A Nearly Linear Time PTAS for Explicit Fractional Packing and Covering Linear Programs
粘弹性固体模型的迟滞回环和能量损耗
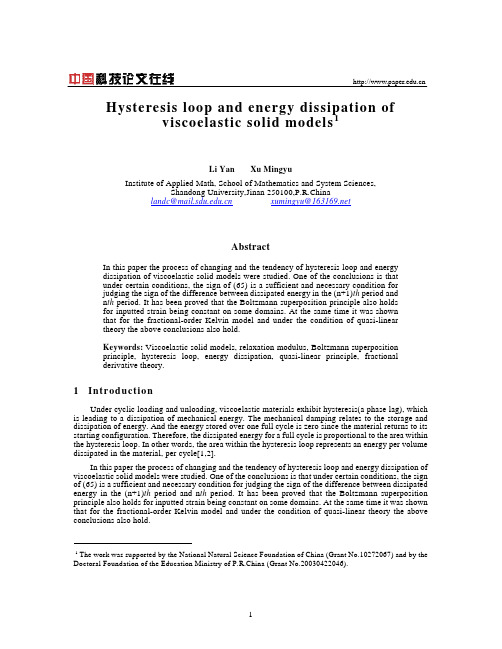
Hysteresis loop and energy dissipation ofviscoelastic solid models1Li Yan Xu MingyuInstitute of Applied Math, School of Mathematics and System Sciences,Shandong University,Jinan 250100,P.R.Chinalandc@ xumingyu@AbstractIn this paper the process of changing and the tendency of hysteresis loop and energydissipation of viscoelastic solid models were studied. One of the conclusions is thatunder certain conditions, the sign of (65) is a sufficient and necessary condition forjudging the sign of the difference between dissipated energy in the (n+1)th period andn th period. It has been proved that the Boltzmann superposition principle also holdsfor inputted strain being constant on some domains. At the same time it was shownthat for the fractional-order Kelvin model and under the condition of quasi-lineartheory the above conclusions also hold.Keywords: Viscoelastic solid models, relaxation modulus, Boltzmann superpositionprinciple, hysteresis loop, energy dissipation, quasi-linear principle, fractionalderivative theory.1IntroductionUnder cyclic loading and unloading, viscoelastic materials exhibit hysteresis(a phase lag), which is leading to a dissipation of mechanical energy. The mechanical damping relates to the storage and dissipation of energy. And the energy stored over one full cycle is zero since the material returns to its starting configuration. Therefore, the dissipated energy for a full cycle is proportional to the area within the hysteresis loop. In other words, the area within the hysteresis loop represents an energy per volume dissipated in the material, per cycle[1,2].In this paper the process of changing and the tendency of hysteresis loop and energy dissipation of viscoelastic solid models were studied. One of the conclusions is that under certain conditions, the sign of (65) is a sufficient and necessary condition for judging the sign of the difference between dissipated energy in the (n+1)th period and n th period. It has been proved that the Boltzmann superposition principle also holds for inputted strain being constant on some domains. At the same time it was shown that for the fractional-order Kelvin model and under the condition of quasi-linear theory the above conclusions also hold.1 The work was supported by the National Natural Science Foundation of China (Grant No.10272067) and by the Doctoral Foundation of the Education Ministry of P.R.China (Grant No.20030422046).2 The basic theories of viscoelastic solid modelsThe dissipation of mechanical energy, for one dimensional situation, can be written as∫∫==ttdt d W 0εσεσ&. (1) It consists of stored energy and dissipated energy. The energy stored over one full cycle is zero sincethe material returns to its starting configuration. Therefore, the area within the hysteresis loop represents an energy per volume dissipated in the material, per cycle [3]. Using the Boltzmann superposition principle, the stress )(t σ is∫−=td t K t 0)()()(ττετσ& (5) or∫−+=td t Kt K t 0)()()()0()(ττετεσ&. (6) Where K(t) is the relaxation modulus and overdots denote derivatives with respect to τ[1,3].is a convex function[1] or is a nonnegative monotone decreasing function and is monotone decreasing to zero. In addition, K(t)K(t))(t K&−)(t ε could be derived almost everywhere on ),0(+∞∈t .Considering the nonlinear effect, we quote the quasi-linear theory[3].Using the quasi-linear theory, we quote the definition of generalized relaxation modulus as follows:)0()()(+=K t K t G .If the strain is step function, the developing process of stress is a function of time t and strain ε. Using ),(t K ε to express this process, we have)()(),()(εεe T t G t K =,1)0(=+G , (22)where is a function of strain and is called elastic response .)()(εe TFig.1: )(t εas a function of time , with T being the period of t )(t ε.3 Hysteresis loop and energy dissipation under nonnegative inputtedstrainIn this section, the inputted strain )(t ε, as shown in Fig.1, satisfies (5)~(8):)),,0[(),()(+∞∈=+t t T t εε (5)),2,1,0(,0)(L ==n nT ε, (6)])2,0[(),()(T a a T a ∈−=εε, (7)⎪⎩⎪⎨⎧++∈<+∈>).)1(,2(,0)2,(,0)(T n T nT t T nT nT t t ε& (8)Moreover, )(t σ satisfies (2) or (3).Using (3) we have∫+−+−=+−Tt td dtT t d KdtT t t d ττετσσ)()]([)]()([&. (9) Let 02012,2a T b a T b +=−=,in which )2,0(0T a ∈, 11b t +=τ and 22b t +=τ, then we have.0)2()()(lim)(01101<+=Δ−−Δ+−=−+→Δdta T d tb T t b T dtT t d t εεετε (10) Similarly, we have.0)2()(02>−=−+dta Td dt T t d ετε (11)Using (7) we obtain.0)2()2(00=−++dta T d dt a T d εε Therefore.0)()(21=−++−+dtT t d dt T t d τετε (12)Further because is a monotone decreasing function, )0)](([>−t K&.0)()]([)()]([)]()([22<−+−+−+−=+−∫∫+++T t Tt T t t d dtT t d K d dtT t d KdtT t t d ττετττετσσ&& (13)What is more,.0)()(00>−+−+a T nT a nT σσ (14)(see the proof of (32)). Using (13) and (14) we obtain that.0)]()([)]()([)]()([)]()([00000000>−++−++−−+−+=−++−−+−++−+a T T nT a T nT a T nT a nT a T T nT a T nT a T nT a nT σσσσσσσσ (15)In the strain(ε)-stress(σ) coordinate system, equation (14) stands for the difference between loading and unloading curves (hysteresis loop’s width[4] in the (n+1)th period and at the point )()(0a t εε=. Moreover, equations (13) ~ (15) indicate that the hysteresis loop's area decreases with increase of period and is decreasing to a positive value. Namely, the energy dissipation for a full cycle decreases with increase of period and is decreasing to a positive constant. The limit area() of hysteresis loops ,when , isit S lim +∞→t .)]()()][([lim lim lim20000)1()1(lim ∫∫∫∫−+−−−+−===∞→+∞→+∞→TnTn T n nTn Tn nTn it da d a nT a T nT K dtd S ττετετεσεσ&& (16)In course of proving (16), we used the equation (24).4 Situation of the strain being constant on some domainsFirstly, we prove that the Boltzmann superposition principle holds for the situation of the strain being constant on some domains.Proof. Letting T be the period of strain )),0((0)(,),(121T t t T T Tt ∈≠+=εε& and 0)(=t ε& . When , the relation between )),((1T T t ∈),0(1T t ∈)(t σ and )(t ε is obviously that∑Δ=→ΔΔ−Δ+Δ−=ttj t t j t j t j t K t 0)}(])1[(){(lim )(εεσ, (17)Namely, at present, )(t σ satisfies (2) or (3).When , because ),(1T T t ∈)(t ε keeps constant on this domain, )(t σ experiences a relaxation process. This process can be written as.)()()()()}(])1[(){(lim )(0001∫∫∑−=−=Δ−Δ+Δ−=Δ=→ΔtT ttj t d t K d t K t j t j t j t K t ττετττετεεσ&& (18)On the analogy of (18), we have.)()()()0()()(),(,)()(()),(,)()(())(011∫∫−+=−=⎪⎩⎪⎨⎧++∈−+∈−=ttd t K t K d t K T nT T nT t d t K T nT nT t d t K t ττετεττετττετττετσ&&&& (19)Similarly, (19) holds for non-periodic functions. Therefore, the Boltzmann superposition principleholds for the situation of the strain being constant on some domains.4.1 S it ua t ion of t h e co nsta nt do ma in d ist ributed sy mmetrica lly in a full periodFig.2: )(t εas a function of time t , with T being the period of )(t ε, and 11T t = and 212T t t =−.This kind of strain, as shown in Fig.2, satisfies (5)~(7) and (20):⎪⎪⎩⎪⎪⎨⎧∈<∈=∈>),,(,0),(,0),0(,0)(2211T t t t t t t t t ε& (20) where ( and are positive constants), 21T T T+=1T 2T 211T t = and 212T t t =−. The second termof equation (20) stands for )(t ε being constant on ]2,2[11T T T −, whose length is .2T Using (5)~(7) and (20) we can obtain that (13) and (15) also hold. Therefore, the hysteresis loop's area decreases with increase of period and is decreasing to a positive value. Namely, the energy dissipation for a full cycle decreases with increase of period and is decreasing to a positive constant. 4.2 Situation of the st rain to be equal to zero on some domainsFigure 3.)(t εas a function of time t, with T being the period of )(t εand .11T t =This kind of strain, shown in Fig.3, satisfies (5),(6) and (21)~(23):⎪⎩⎪⎨⎧∈<∈>),,2(,0)2,0(,0)(111t t t t t t ε& (21)],,[,0)(1T t t t ∈=ε (22)],2,0[),()(11t a a t a ∈−=εε (23)where ( and are positive constants) and 21T T T+=1T 2T 11T t =.Letting ξτξ±=∈2],2,0[2,1TT then we have .)()]([)]()()][([)()(1111∫∫−+++−+−+−+−−−+−=−+−+aT nT anT anT d a T nT Kd a nT a T nT Ka T nT a nT τετττετετσσ&& (24) Obviously, the second term of equation (24) is greater than zero. Further because),2()]2([)()(1111ξεξετετε−+−++−+=−+−−−+a T a T T T a nT a T nT (25)),2()]2([)()(1221ξεξετετε++−−+−+=−+−−−+a T a T T T a nT a T nT (26)and using (5) and (23) we have0)2()]2([1=++−++−+ξεξεa T a T T Tand.0)2()]2([1=−+−−+−+ξεξεa T a T T TTherefore.0)()()()(221111=−+−−−++−+−−−+τετετετεa nT a T nT a nT a T nT (27)Moreover, for convenience, letting),()()(1τετετ−+−−−+=a nT a T nT f (28)then, obviously, . To study the sign of 0)(=nT f )2(ξ−Tf , we divided this problem into two cases: Case1:. a T T 212−≤When ,222121aT T T a −−+=−τwe have ],0(2,0)2(t TnT TnT f ∈−=−. (29) Further because and notice that the solutions of 0)(>a f 0)(=τf are{∈−nT TnT nT ,2,τ, when }],0(t ]2,0[,22TT ∈−==ξξττ, we arrive at 0)2()(2≥−=ξτTf f . (30) Case2:.a T T 212−>In this case, 0)(=τf on ),(11T a nT T a T nT −+−−+∈τ.Becausea T a T T T T T −=−+>+=121212222,and 0)2(2122)(122121>−−=+−+=−−−a T T T T a T Ta T T, we have ),(211T a T a T T −−−∈−, namely ),(221a T a T T+−∈. Then we have 212220T a T T a TT +=+−<≤−=≤ξτ. Further because 0)0(,0)(=>f a f , we arrive at 0)2(≥−ξTf . In sum,0)]2([)]2([)2(1≥−−+−−−−+=−ξεξεξTa nT T a T nT T f . (31) Moreover, using (27) and the monotonicity of , the first term of (24) is also greater than zero. Therefore)0)](([>−τK&0)()(1≥−+−+a T nT a nT σσ. (32)The sign of equality holds, provided and only provided 21T a=. Obviously, (14) holds when(namely ) and 02=T 1T T =)2,0[10T a a ∈= in equation (32).Moreover, for 0)()(>+−T t t σσ, we have∫+−++−=+−1)()]([)]()([12T t td dtT t d T K dtT t t d ττετσσ&. (33) On the analogy of (9), we can obtain0)]()([<+−dtT t t d σσ. (34)Therefore, the hysteresis loop's width decreases with increase of period and is decreasing to a positive value. Namely, the hysteresis loop's area decreases with increase of period and is decreasing to a positive value. In other words, the energy dissipation for a full cycle decreases with increase of period and is decreasing to a positive constant.In addition, using (3) we have0)()]()([)()(1121<−++−=+−+∫+T nT d T nT T K KT nT T nT ττεττσσ&&. (35)Equation (35) implies that )(t σ experience a relaxation process on ),(1T nT T nTt ++∈.Because 0)(=t ε on this domain,)()(1T nT T nT +−+σσ , in the strain(ε)-stress(σ) coordinate system, stands for the length of the overlap between the (n+1)th hysteresis loop and σ- coordinate axis.This length has close relation to )()(1T nT nT +−σσ, the (n+1)th hysteresis loop's widthon )(1T nT+ε,which is shown in (36)~(38).)()()()()()(11T nT nT T nT T nT T nT nT +−=+−+++−σσσσσσ, (36)where0)()]([)()()1(>−+−=+−∫+Tn nTd T nT KT nT nT ττετσσ& , (37) and0)()]([lim)1(=−+−∫+∞→Tn nTn d T nT Kττετ& (38) [4].Namely, )()(1T nT T nT+−+σσ is monotone increasing to )]()([lim 1T nT nT n +−∞→σσ withthe increase of . Therefore, hysteresis loops tend to a closed loop. The area within this closed loop is proportional to the minimum dissipated energy for a full cycle.n 5 Hysteresis loop and energy dissipation under the linearsuperposition of strainsFor linear superposition of strains, it is easy to prove that the Boltzmann superposition principle also holds.For convenience, we use the superposition of two strains to discussthis problem, in this section.5.1 S it ua t ion of th e d e r iva t iv e o f s t ra in b e ing ze r o o n s o me d om a insFigure 4.)(t εas a function of time t, with T being the period.of )(t ε,)(1t εand )(2t εand )()()(21t t t εεε+=.112T t t =−,223T t t =−,231T t =.Let )()()(21t t t εεε+= satisfy (5),(6) and (39)~(41):],2,0[),()(1ta a T a ∈−=εε (39)],2,0[),()(1221t t b b t b t −∈−=+εε (40) ],,[),()()(3232t t t t t t ∈==εεε (41)where⎪⎪⎩⎪⎪⎨⎧∈==∪∈=],,[)()()(),(),0()()(2132211t t t t t t T t t t t t εεεεε (42)⎪⎪⎩⎪⎪⎨⎧∈−∪∈=],,[)()(),(),0(0)(212212t t t t t T t t t t εεε (43)with ( and are positive constants). 321T T T T++=21,T T 3T )(),(1t t εεand )(2t ε are shown inFig.4. Obviously, )(1t ε and )(12t t +ε satisfy (5)~(7) and (20) and (5)~(6) and (21)~(23)respectively. Letting,2,1,)()()()0()()()(0=−+=−=∫∫i d t K t K d t K t tii t i i ττετεττετσ&& (44)2,1,)()()()1(==∫+i dt t t t W Tn nTi ini εσ&. (45)Then we obtain that.)()()()()()()(1333122121)1(∫∫∫∫++++−+++⎟⎟⎠⎞⎜⎜⎝⎛+++==T nT T nT T nT nTTnT T T nT n n Tn nTn dt t t dt t t W W dtt t t W εσεσεσ&&& (46)Using)]()([lim )]()([lim 1111a T nT a T nT a T nT a nT n n −+−++=−+−+∞→∞→σσσσand the same way as is used in arriving at (37), we have0)]()([lim 11>−+−+∞→a T nT a nT n σσ. (47)Using (32) we obtain0)]()([lim 1232>−+−++∞→b T nT b T nT n σσ. (48)Moreover, in the same way as (13), we have0)]()([<+−dtT t t d σσ. (49)Therefore, the last two terms of (46)0)()()()(13331221>+⎟⎟⎠⎞⎜⎜⎝⎛+∫∫∫++++−+T nT T nT T nT nT T nT T T nT dt t t dt t t εσεσ&&. (50) Namely, .21n n n W W W +>What is more, (49) and (50) imply that the hysteresis loop's area decreases with increase of periodand is decreasing to a positive value. Namely, the energy dissipation for a full cycle decreases with increase of period and is decreasing to a positive constant. 5.2 Situa t ion of stra in's va lue being realFigure 5.)(t εas a function of time t, with T being the period of )(t εand .11T t =Letting the inputted strain satisfy (5),(6) and (51)~(54):]),2,0[(),()(11t a a t a ∈−=εε (51) 2,0[(),()(21T b b T b t ∈−=+εε (52) ⎪⎩⎪⎨⎧∈<∈>),,(,0),0(,0)(11T t t t t t ε (53)⎪⎪⎩⎪⎪⎨⎧−∈<−∪∈>),2,2(,0),2()2,0(,0)(2121T T t t T TT t t t ε (54)and⎪⎩⎪⎨⎧∈=∈=],,(,0],0[),()(111T t t t t t t εε (55)⎪⎩⎪⎨⎧∈=∈=],,(),(],0[,0)(111T t t t t t t εε (56)with ( and are positive constants) and 21T T T+=1T 2T 11T t =, as shown in Fig.5. Continuousfunctions )(1t ε and )(2t ε are nonnegative and nonpositive parts of )(t ε, respectively.There exist two ways to find the natures of hysteresis loops and energy dissipation in this kind of inputted strain. The one is to calculate directly the hysteresis loop's area and the other is to use the superposition of )(1t ε and )(2t ε. With the second method, we can see clearly the interaction between)(1t ε and )(2t ε. What is more, using the two methods, we prove out the same conclusions abouthysteresis and energy dissipation. Therefore, we choose the second method to study the changing of hysteresis loops.Using (44) and the proofs of (32) and (48), we have.0)()(111>++−−+b T nT b T nTσσ (57)Obviously, )()(111b T nT b T nT++−−+σσ is an increasing function of . Moreover,n .0)()(122>−+−+a T nT a nT σσ (58)Using (44) we have:)].()([)()()]()([)()()]()([)()(1212211111111a T nT a nT a T T nT a T nT a T nT a nT a T T nT a T nT a T nT a nT a T T nT a T nT −+−+−−++−+++−+−+−−++−++=−+−+−−++−++σσσσσσσσσσσσ (59) Then substituting (56) and (58) into (59) we have:)]()([)()(12122a T nT a nT a T T nT a T nT −+−+−−++−++σσσσ0)()]()([221>−+−+−+=∫+T nT nTd T nT a T K a Kττεττ&&. (60)In terms of Boltzmann superposition principle we have.)()()()]2()([)()(20110111111∫∫−+−−+−−+−+−=−+−+aT anT d a T nT Kd a nT a T K Ka T nT a nT ττετττεττσσ&&& (61)Therefore,)]()([)()(111111a T nT a nT a T T nT a T nT −+−+−−++−++σσσσ0)()]()([221<−+−+−+=∫++TnT T nT d T nT a TK a Kττεττ&&. (61)Substituting (60) and (62) into (59) yields).,()()]()([)]()([)()(.)1(111n a M d T nT a T K a Ka T nT a nT a T T nT a T nT def Tn nT=−+−+−+=−+−+−−++−++∫+ττεττσσσσ&& (63)Similarly, we have).,()()]()([)]()([)()2()1(111n b N d T nT b T K b T Kb T nT b T nT b T T nT b T nT Tn nT=−+++−−+=++−−+−+++−−+∫+ττεττσσσσ&& (64)It is obvious that the sign of or depends only on and ),(n a M ),(n b N )(t K )(t ε. That is to say, the dissipated energy for a full cycle is unnecessary monotone function of . The tendency of change of dissipated energy for full cycles is determined by (65)n ∫∫+2221),(),(T T db n b N da n a M . (65)Equation (65) stands for the difference between dissipated energies in the (n+1)th period and n th period. Under the conditions of (5),(6) and (51)~(54), the sign of (65) is a sufficient and necessary condition for judging the sign of the difference between dissipated energy, per volume, in the (n+1)th period and n th period.Moreover, because of the precondition,0),(),(2221=+∞++∞∫∫T T db b N da a M . (66)holds for arbitrary strain[4].Obviously, (65) converts into (15) with 02=T .For example, letting strain)sin()(t t =ε, then we have ],)sin()[sin(21)(1t t t +=ε])sin()[sin(21)(2t t t −=εand 221T T T ==. Substituting )()(1t t εε, and )(2t ε into (65), it isobtained that (65) is greater than zero for arbitrary ),2,1,0(L ∈n . Namely, the dissipated energy for a full cycle increases with increase of period.On the real domain and letting +∞→t, we have,)cos()sin()(lim t B t A t t +=+∞→σ, (67)where])cos()()0([lim 0τττd KK A tt ∫+=+∞→&, (68) })sin()]([{lim 0∫−=+∞→tt d KB τττ&. (69) Using the nature of yields)(t K 0})sin()]([{lim 0>−=∫+∞→tt d KB τττ&. (70) After a straight calculation we have222222)(B A B A =−++εσσε. (71)Obviously, in σε− coordinate system, (71) is an inclined ellipse whose center is the origin(just likediscuss in [1]. The area B π represents the most energy per volume dissipated in the material, full cycle. Namely, the dissipated energy for full cycle is monotone increasing to a positive value, which is proportional to B π [5~7].6 Applying of fractional Kelvin model and quasi-linear theoryUsage of the quasi-linear theory corresponds with substituting and )]([)(t T e ε),(t K ε into )(t εand respectively. Moreover, provided satisfies (72) and (73):)(t K ][)(εe T⎪⎪⎪⎩⎪⎪⎪⎨⎧<<==>>,0,00,00,0][)(εεεεe T (72),0][)(>εεd dT e (73) all the above conclusions also hold.Using the Riemann-Liouville derivative theory[8,9] and fractional Kelvin model, the relaxation modulus[10] is)()]((1[)(t H t E E t K R αεααεασαεαττττ−−−=, (74) where )10(≤<αα is the fractal order of evolution,∑∞=+Γ−=−0)1(])([))((n nn ttE αττασασαis Mittag-Leffler function and is unit step function.)(t H Because is a complete monotone function[11], is a convex function or is anonnegative monotone decreasing functions and is monotone decreasing to zero. Therefore, substituting into , the above conclusions also hold.)(t K α)(t K α)(t K α)(t K α&−)(t K α)(t K 7 Results and discussionIn this paper, we discuss the process of changing and the tendency to one-dimensional viscoelasticsolid models' hysteresis loops and energy dissipation. We assume that is a convex function oris a nonnegative monotone decreasing function and is monotone decreasing to zero. One of our conclusions is that under the conditions of (5),(6) and (51)~(54), (65) implies that thedissipated energy in the (n+1)th period is greater or equal to it in the n th period, as shown in the example of section 5.2. On the other hand, (65))(t K )(t K )(t K&−0≥0< implies that the dissipated energy in the (n+1)th period is less than it in the n th period, as discusses in sections 3~5.1. This is a sufficient and necessary condition. Moreover, because of the precondition, (66) holds for arbitrary strain. We have proved that the Boltzmann superposition principle also holds for inputted strain being constant on some domains. At the same time, we prove that for the fractional-order Kelvin model and under the condition of quasi-linear theory the above conclusions also hold.8 acknowledgementsThis work was supported by the National Natural Science Foundation of China (Grant No.10272067) and by the Doctoral Foundation of the Education Ministry of P.R.China (Grant No.20030422046). References[1] Roderic S. Lakes(1999),Viscoelastic Solids,CRC Press, Boca Raton London New York Washington,D.C.[2] G. Carbone and B.N.J. Persson(2005), Crack motion in viscoelastic solids: The role of the flashtemperature. Eur. Phys. J. E 17, 261{281}[3] Fung.Y.C.Biomechanics:Mechanical(1993), Properties of Living Tessues.2nd ed. New York:Springer.[4] Li Yan and Xu Mingyu(2006), Hysteresis and preconditon of viscoelastic solid models.Mech Time-DependMater (2006) 10:113–123.[5] Anna Vainchtein and P. Rosakis(1999), Hysteresis and Stick-Slip Motion of Phase Boundaries in DynamicModels of Phase Transitions. J. Nonlinear Sci. Vol. 9: pp. 697–719.[6] Anna Vainchtein(1999), Dynamics of Phase Transitions and Hysteresis in a Viscoelastic Ericksen’s Bar on anElastic foundation.Journal of Elasticiy 57:243-280.[7] Ian D. Aiken, Douglas K. Nims, Andrew S. Whittaker, James M. Kelly(1993), Testing of Passive EnergyDissipation Systems. EARTHQUAKE SPECTRA, VOL. 9, NO. 3, EARTHQUAKE ENGINEERING RESEARCH INSTITUTE CALIFORNIA AUGUST.[8] I. Podlubny(1999), Fractional Differential Equations.Academic Press, San Diego-Boston-New Yor –London-Tokyo-Toronto.[9] R.L.Bagley and P.J.Torvik(1983),A theoretical basis for the application of fractional calculus toviscoelasticity, Journal of Rheology 27,201-210.[10] Alan Freed , Kai Diethelm,et al.(2002), Fractional-Order Viscoelasticity(FOV):Constitutivedevelopment using the fractional calculus:First annual report.NASA/TM-2002-211914.[11]Kenneth ler,Stefan G. Samko(2001).Completely monotonic functions.Integr.Transf.and Spec.Funct.2001,Vol.12,No4,389-402.。
Modelling of Reflections and Air Absorption in Acoustical Spaces – A Digital Filter Design
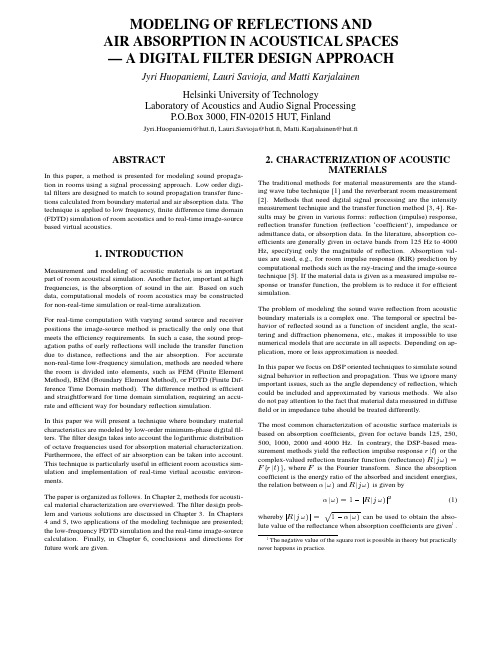
MODELING OF REFLECTIONS AND AIR ABSORPTION IN ACOUSTICAL SPACES —A DIGITAL FILTER DESIGN APPROACH Jyri Huopaniemi,Lauri Savioja,and Matti KarjalainenHelsinki University of TechnologyLaboratory of Acoustics and Audio Signal ProcessingP.O.Box3000,FIN-02015HUT,FinlandJyri.Huopaniemi@hut.fi,Lauri.Savioja@hut.fi,Matti.Karjalainen@hut.fiABSTRACTIn this paper,a method is presented for modeling sound propaga-tion in rooms using a signal processing approach.Low order digi-talfilters are designed to match to sound propagation transfer func-tions calculated from boundary material and air absorption data.The technique is applied to low frequency,finite difference time domain (FDTD)simulation of room acoustics and to real-time image-source based virtual acoustics.1.INTRODUCTIONMeasurement and modeling of acoustic materials is an important part of room acoustical simulation.Another factor,important at high frequencies,is the absorption of sound in the air.Based on such data,computational models of room acoustics may be constructed for non-real-time simulation or real-time auralization.For real-time computation with varying sound source and receiver positions the image-source method is practically the only one that meets the efficiency requirements.In such a case,the sound prop-agation paths of early reflections will include the transfer function due to distance,reflections and the air absorption.For accurate non-real-time low-frequency simulation,methods are needed where the room is divided into elements,such as FEM(Finite Element Method),BEM(Boundary Element Method),or FDTD(Finite Dif-ference Time Domain method).The difference method is efficient and straightforward for time domain simulation,requiring an accu-rate and efficient way for boundary reflection simulation.In this paper we will present a technique where boundary material characteristics are modeled by low-order minimum-phase digitalfil-ters.Thefilter design takes into account the logarithmic distribution of octave frequencies used for absorption material characterization. Furthermore,the effect of air absorption can be taken into account. This technique is particularly useful in efficient room acoustics sim-ulation and implementation of real-time virtual acoustic environ-ments.The paper is organized as follows.In Chapter2,methods for acousti-cal material characterization are overviewed.Thefilter design prob-lem and various solutions are discussed in Chapter3.In Chapters 4and5,two applications of the modeling technique are presented; the low-frequency FDTD simulation and the real-time image-source calculation.Finally,in Chapter6,conclusions and directions for future work are given.2.CHARACTERIZATION OF ACOUSTICMATERIALSThe traditional methods for material measurements are the stand-ing wave tube technique[1]and the reverberant room measurement [2].Methods that need digital signal processing are the intensity measurement technique and the transfer function method[3,4].Re-sults may be given in various forms:reflection(impulse)response, reflection transfer function(reflection‘coefficient’),impedance or admittance data,or absorption data.In the literature,absorption co-efficients are generally given in octave bands from125Hz to4000 Hz,specifying only the magnitude of reflection.Absorption val-ues are used,e.g.,for room impulse response(RIR)prediction by computational methods such as the ray-tracing and the image-source technique[5].If the material data is given as a measured impulse re-sponse or transfer function,the problem is to reduce it for efficient simulation.The problem of modeling the sound wave reflection from acoustic boundary materials is a complex one.The temporal or spectral be-havior of reflected sound as a function of incident angle,the scat-tering and diffraction phenomena,etc.,makes it impossible to use numerical models that are accurate in all aspects.Depending on ap-plication,more or less approximation is needed.In this paper we focus on DSP oriented techniques to simulate sound signal behavior in reflection and propagation.Thus we ignore many important issues,such as the angle dependency of reflection,which could be included and approximated by various methods.We also do not pay attention to the fact that material data measured in diffuse field or in impedance tube should be treated differently.The most common characterization of acoustic surface materials is based on absorption coefficients,given for octave bands125,250, 500,1000,2000and4000Hz.In contrary,the DSP-based mea-surement methods yield the reflection impulse response or the complex-valued reflection transfer function(reflectance),where is the Fourier transform.Since the absorption coefficient is the energy ratio of the absorbed and incident energies, the relation between and is given by(1) wherebyThe negative value of the square root is possible in theory but practically never happens in practice.The relation between the normalized impedance and the re-flectance isFigure3:Two-dimensional waveguide mesh structure with bound-aryfilters.Figure3shows the basic structure of a digital waveguide mesh.In the mesh each node is connected to its neighbours by unit delays. Nodes on the boundary(on the left side of thefigure)have one con-nection to boundaryfilter with transfer function H(z),which rep-resent the acoustical properties of the boundary surface.Each sur-face material has its own transfer function.Each boundaryfilter has connections only to one node inside the mesh.This structure sim-ulates locally reacting surfaces,which is usually valid for surface materials in normal acoustic spaces.Although Fig.3represents a two-dimensional case this same structure is suitable also to three-dimensional meshes which are used in simulation of room acoustics. Figure4represents numerical simulation results of a2D waveg-uide mesh with different boundaryfilters and incident angles of the reflected plane wave.Figures show the magnitude attenuation of boundaryfilter and simulation results,also the target response is plotted.In the upperfigure the wall is hard and target absorption is low and both simulation results follow thefilter response reason-ably well.In the lowerfigure the required material is more absorb-ing.The simulation result of90incident angle is not as good as in the upperfigure,but the shape of the attenuation response is cor-rect.Still the simulation of incident angle of45is correct.Wave propagation characteristics are direction-dependent in the waveg-uide mesh.The same applies also to the reflection characteristics. This anisotropic behaviour may be reduced by using an interpola-tion technique[16].The sampling frequency of the waveguide mesh is determined by the spacing of the nodes,i.e.,the length of the unit delays.For example if a3D mesh is discretized by10cm spacing,the update frequency will be ca.6kHz.The simulation results are valid on the frequency band that covers approximately the lowest tenth of the whole band (update frequency).In this case also the boundaryfilters should be designed to cover the same band.5.REAL-TIME ROOM ACOUSTICSRENDERINGIn real-time rendering of room acoustics,efficient techniques for modeling the direct sound and early reflections have to be imple-mented.Although the geometrical acoustics approach is limited to high frequencies[10],it is the only possible approach for real-timeFigure4:Reflection responses of two2D FDTD simulations. dynamic auralization.We have used the methods discussed in Chap-ter3to design reflection and air absorptionfilters.The DIV A vir-tual acoustics simulation system[17]uses the image-source method [18,19]for computing the early reflections in a computer model of an acoustical spacesuch as a concert hall.The hall used in this study is depicted in Fig.5[8].The room acoustics processing is divided into three parts:direct sound,early reflections and late reverberation processing.It is known from room acoustics theory that consider-able emphasis should be put on the accurate reproduction of early reflections in order to achieve natural sounding simulation.An overview of processing for the direct sound and early reflections in the DIV A system is given in the form of aflowgraph in Fig.6. The inputs to calculation are the room geometry and the positions of the source and the listener.Visibility checking is carried out to determine valid image sources.The image-source calculation algorithm keeps track of the surface hits for each image,and afirst-order IIR approximation isfitted to the given magnitude response data obtained by cascading the ap-propriate material absorption characteristics.A precalculated set of all the boundary reflection combinations has been stored to enable efficient calculation.Thefiltering structure is illustrated in Fig.7. For each image a reflectionfilter M(z)and a distance-dependent air absorptionfilter A(z)is attached(for the direct sound,only air ab-sorption is valid).To reduce the needed calculation,grouping of reflections according to arrival times can be carried out[20].The Figure5:The geometry of the Sigyn hall[8].Room geometrySource and receiverFigure6:Image-source calculation process in the DIV A system[17].frequency-independent attenuation of sound is taken into account by a gain factor proportional to distance(law[10]).The output from the early reflections calculation algorithm is directed to binau-ral processing and late reverberation units.Generally the cascaded reflectionfilter magnitude characteristics fall into straightforward low-or highpass structures.There are,however, exceptions in the case of more complex materials such as resonators with air cavities.To enable accurate modeling also in these cases, we introduced an estimation algorithm for requiredfilter order based on least squares error calculation.6.CONCLUSIONSIn this paper,we have presented a signal processing approach to modeling reflections and air absorption in acoustical spaces.Low order digitalfilters may be designed tofit air absorption character-istics,the measured absorption coefficient data or real-valued data found from the literature.The methods described are useful in sim-ulation of room acoustics and real-time image-source based virtual acoustics.The approach is currently limited to specular reflections. Extensions to our model could include such effects as diffuse reflec-tions and direction-dependent absorption coefficients[21].References1.Standard ISO10534-1:1996,Acoustics—Determination ofSound Absorption Coefficient and Impedance in Impedance Tubes—Part1:Method Using Standing Wave Ratio.2.Standard ISO354:1985,Acoustics—Measurement of SoundAbsorption in a Reverberation Room.3.Fahy,F.J.,Sound Intensity,2nd Edition,E&FN SPON,1995.4.Chung,J.Y.and Blaser,D.A.,“Transfer Function Method ofMeasuring the In-Duct Acoustic Properties.Part I:Theory,and Part II:Experiment”J.Acoust.Soc.Am.,V ol.68,No.3,1980.5.Kuttruff,H.,“Sound Field Prediction in Rooms,”in Proc.15thInt.Congr.Acoust,Trondheim,Norway,1995June,vol.2,pp.545-552.6.MATLAB,Signal Processing Toolbox,MathWorks Inc.7.Naylor,G.and Rindel,J.,Odeon Room Acoustics Program,Version 2.5,User Manual,Technical Univ.of Denmark, Acoustics Laboratory,Publication49,Denmark,1994.hti,T.and M¨o ller,H.,“The Sigyn Hall,Turku-A Con-cert Hall of Glass,”in Proc.1996Nordic Acoustical Meeting, Helsinki,Finland,1996June,pp.43-48.Figure7:Structure for image-source implementation with reflection and air absorptionfilters.9.Bass,H.,and Bauer,H.-J.,“Atmospheric Absorption of Sound:Analytical Expressions,”J.Acoust.Soc.Am.,V ol.52,No.3, 1972,pp.821-825.10.Kuttruff,H.,Room Acoustics,3rd Edition,Elsevier SciencePublishers,Essex,England,1991.11.Botteldooren,D.,“Finite-difference time-domain simulationof low-frequency room acoustic problems,”J.Acoust.Soc.Am.,V ol.98,No.6,1995,pp.3302-3308.12.Savioja,L.,J¨a rvinen,A.,Melkas,K.,and Saarinen,K.,“De-termination of the Low Frequency Behavior of an IEC Lis-tening Room,”In Proc.Nordic Acoustical Meeting(NAM’96), Helsinki,Finland,June12-14,1996,pp.55-58.13.Savioja,L.,Rinne,T.,and Takala,T.,“Simulation of roomacoustics with a3-Dfinite difference mesh,”in Proc.1994Int.Computer Music Conf.,˚Arhus,Denmark,Sept.12-17,1994, pp.463-466.14.Van Duyne,S.and Smith,J.O.,“The2-D digital waveguidemesh,”in Proc.1993IEEE Workshop on Applications of Sig nal Processing to Audio and Acoustics,New Paltz,NY,Oct.17-20,1993.15.Savioja,L.,Karjalainen,M.,and Takala,T.,“DSP Formula-tion of a Finite Difference Method for Room Acoustics Simu-lation.,”In NORSIG’96IEEE Nordic Signal Processing Sym-posium,Espoo,Finland,Sept.24-27,1996,pp.455-458. 16.Savioja,L.,and V¨a lim¨a ki,V.,“Improved Discrete-Time Mod-eling of Multi-Dimensional Wave Propagation Using the In-terpolated Digital Waveguide Mesh,”In Proc.ICASSP’97, M¨u nchen,April19-24,1997,V ol.1,pp.459-462.17.Takala,T.,H¨a nninen,R.,V¨a lim¨a ki,V.,Savioja,L.,Huopaniemi,J.,Huotilainen,T.,Karjalainen,M.1996.“An integrated system for virtual audio reality,”in Proc.100th Au-dio Engineering Society(AES)Convention,Preprint no.4229, Copenhagen,Denmark,May11-14,1996.18.Allen,J.,Berkley,D.,“Image Method for Efficiently Simulat-ing Small-Room Acoustics,”J.Acoust.Soc.Am.,V ol.65,No.4,1979,pp.943-950.19.Borish,J.,“Extension of the Image Model to Arbitrary Polyhe-dra,”J.Acoust.Soc.Am.,V ol.75,No.6,1984,pp.1827-1836.20.Jot,J.-M.,Larcher,V.,and Warusfel,O.,“Digital SignalProcessing Issues in the Context of Binaural and Transaural Stereophony,”in Proc.98th Audio Engineering Society(AES) Convention,Preprint no.3980,Paris,France,February25-28, 1995.21.Dalenb¨a ck,B.-I.,A New Model for Room Acoustic Predictionand Auralization,Dr.Phil.Thesis,Chalmers Univ.of Tech., G¨o teborg,1995.。
Dependable Computing Systems Centre
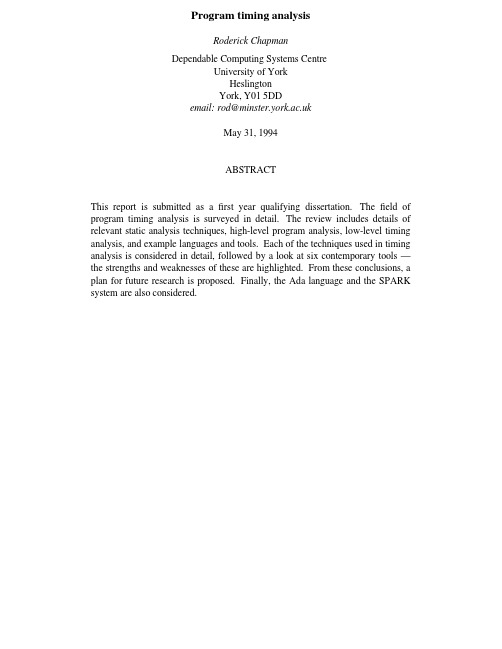
Program timing analysisRoderick ChapmanDependable Computing Systems CentreUniversity of YorkHeslingtonYork,Y015DDemail:rod@May31,1994ABSTRACTThis report is submitted as afirst year qualifying dissertation.Thefield of program timing analysis is surveyed in detail.The review includes details of relevant static analysis techniques,high-level program analysis,low-level timing analysis,and example languages and tools.Each of the techniques used in timing analysis is considered in detail,followed by a look at six contemporary tools—the strengths and weaknesses of these are highlighted.From these conclusions,a plan for future research is proposed.Finally,the Ada language and the SPARK system are also considered.Table of ContentsPart I-Introduction1.Introduction and overview (3)Part II-Field Survey and Review2.Static analysis (5)2.1Controlflow analysis (5)2.2Dataflow analysis (5)2.3Informationflow analysis (6)2.4Worst case variable range analysis (7)2.5Program dependencies (7)2.6Sequencing constraint analysis (8)3.High level timing analysis (10)3.1Language issues (10)3.2Simple WCET construction (11)3.3Timing annotations (12)3.4Future annotations (19)3.5Systems and scheduling (22)4.Low level timing analysis (23)4.1Architectural assumptions (24)4.2Source of instruction stream (26)4.3Implicit controlflows (26)4.4Processor caches and pipelining (27)4.5Input and output instructions (28)4.6Micro-analysis (29)5.Example languages and tools (31)5.1Timing schema (31)5.2Real-time Euclid (32)5.3MARS/C (32)5.4The FLEX system (33)5.5Real-Time Concurrent-C (35)5.6The SPIRITS timing tool (36)5.7Comparison of methods (37)Part III-Proposed Future Work and Results6.Conclusions and proposal (39)7.Preliminary results (42)7.1The SPARK Toolset (42)7.2Timing analysis of Ada programs (46)7.3Application of SPARK to timing analysis (50)7.4Conclusions (51)8.References (52)Part I-Introduction1.Introduction and overviewThis report concerns the problem of computing the worst-case execution time of computer software written in imperative high level languages.The study of the timing properties of software is a critical issue in the construction of highly dependable computing systems.In hard real-time systems,the timing properties of a program must be totally predictable even under worst-case conditions.Halang[Hal89]cites several major reasons why the timing behaviour of a program should be known:Predictability of system behaviour.Allowing the supervision of timely task execution.Application of predictable task scheduling algorithms.Detection and handling of transient overloads.Halang also notes that a priori knowledge of execution times allows the designer to select hardware components that will meet the required performance as specified in a system’s design. This makes for a cheaper and more effective design process.Structure of the reportThis report is split into three parts.Thefirst part contains an overview of the general issues.Part two("Field survey and review")forms the main body of the report,and is subdivided into four chapters.Chapter2considers the general problem of static analysis of computer programs,looking at each of the major techniques in turn.Chapter3looks at the problem of performing timing analysis of code written in high level languages.The modelling of the timing properties of such code is shown to be a major problem,owing mainly to a lack of information provided by the source code.The techniques used by current systems are then reviewed,including the so-called annotations that are added to programs to express their timing properties.An example of such annotations is given and studied in detail.We also consider the problems caused by concurrent and distributed systems where many processes communicate,synchronise,and interact with their environment.The impact of scheduling is therefore noted.Chapter4considers the timing-analysis of small pieces of assembly-language code.These are required to construct the worst-case execution time of larger blocks of code,but clearly depend on the target computer.The problem of constructing this low-level timing information is considered in detail.Chapter5reviewsfive current state-of-the-art timing tools.These tools take subtly different approaches to timing analysis;the pros and cons of each are discussed.Their similarities are also highlighted,showing that some common ground has already emerged in thisfield.The SPIRITS project is also considered in this chapter.The strengths and weaknesses of each of these tools are highlighted,considering the assumptions and restrictions they impose.Part three of the report presents conclusions and a proposal for further work.This is followed by a report on some early results:a study of the applicability of timing analysis to the Ada language.Ada is of particular interest in thisfield because of its growing use in the military and avionics industries.It is argued that Ada presents many unique problems for a timing system. These are given in some detail.A commercially available‘safety-critical’Ada toolset(SPARK) is then considered.Its goals(chiefly the proof and security of a program)are shown to bepotentially useful in timing analysis.It is argued that the SPARK system presents a potentially powerful new approach to timing analysis that is significantly different from those used by the tools considered in chapter5.Part II-Field survey and review2.Static analysisThis section aims to cover the background information required before timing analysis can be discussed in detail.In particular,we are interested in the static analysis of programs.These techniques have been in use for many years,but have only recently been used in timing analysis. We therefore aim to review these ideas briefly,looking at their traditional uses and how they might be applied in timing analysis.2.1.Control-flow analysisThe notion of control-flow analysis dominates most static analysis and optimisation techniques. Similarly,the modelling of control-flow in a program is central to any timing analysis system. Some basic concepts and terminology are therefore required;the following is summarised from the tutorial given by Aho at al[Aho86].The control-flow graph of a program is represented as a set of nodes and arcs.The nodes represent the basic blocks of code.These are blocks of maximal length with single entry and exit points.If any instruction in a basic block is executed,then they all are.The arcs in the graph representflow of control between the basic blocks.The node where control-flow enters the graph is called the initial node.A node d is said to dominate another node n if every path from the initial node to n passes through d.In particular,every node dominates itself.Loops are of particular interest to us:these are detected as follows:Loops have a single entry node or‘header’which dominates all other nodes in the loop.There must exist a path from inside the loop back to the header.This path is called a back edge.If a path exists from node a→b,and b dominates a,then that path is a back edge.The natural loop of an edge n→d is d plus the set of nodes that can reach n without passing through d.Another important property of the control-flow graph is reducibility.Most static analysis techniques rely on the control-flow graph being reducible.A graph is reducible if the edges can be partitioned into two disjoint sets,forward and backward,such that:Forward edges form an acyclic graph in which every node can be accessed from the initial node.Back edges always have their heads dominating their tails.In practice,most modern programming languages always produce reducibleflow graphs. Older languages,such as FORTRAN-77,or the misuse of modern languages(eg.abuse of the GOTO statement)can produce unreducible graphs.Algorithms forfinding basic blocks,natural loops,the dominates relation,and the reducibility of a graph are all given in[Aho86].2.2.Dataflow analysisUsing the control-flow graph,it is possible to collect much useful information about theflow of data in a program.This is useful in detecting common programming errors and in optimisation of the program.Within a single statement S,the following information can be collected:out[S]The information leaving Sin[S]The information entering Skill[S]The information killed in Sgen[S]The information generated in Sgen[S]can be thought of as the definition of variables,and kill[S]as the‘killing’of variables. From these data,so-called data-flow equations can be constructed to describe the program.For example:out[S]=gen[S]∪(in[S]−kill[S])This equation reads‘the information leaving S is that which entered S plus that generated in S minus the information killed in S.’These equations are composed for each basic block of a subprogram.These equations can then be solved using a variety of techniques tofind a wide range of information about a program.This includes:Variables used when not defined.Variables defined but never used.The‘reaching definitions’problem.This analysisfinds the points in a program that each definition‘reaches’without being killed.The‘available’expressions at a point in a program.An expression x+y is available at a point p if every path from the initial node to p evaluates x+y and does not subsequently redefine x or y.This is useful in the optimisation of common-subexpressions.Detection of loop-invariant computations.More details of these techniques are presented in[Aho86]and[Ken81].A unified model of data-flow problems has also been developed recently[Mar90].rmationflow analysisThese techniques,developed by Carre[Car85],are closely related to dataflow analysis,but generate relations describing theflow of information in a program.These are very useful in automated program checking and testing.Three relations are built for a piece of code with this technique.These are:1)The relation from input variables and parameters to output variables and parameters.2)The relation from input variables and parameters to expressions.3)The relation from expressions to output variables and parameters.The latter two relations are computed using a post-order traversal of the program’s syntax tree, looking at variable definitions and kills as above.Thefirst relation is computed by composing the latter two.These can then be used in the following ways:The statements affecting a given variable can be found.The effect of adding or removing statements can be found.Thefirst relation can be checked against user-supplied annotations.Ineffective variables,ineffective statements,and undefined variable uses can all be detected. Thefirst and fourth of these are potentially useful in timing analysis.For example,where boolean expressions are responsible for controlflow decisions,the ability tofind which statements and variables can affect those expressions is particularly attractive.2.4.Worst-case variable range analysisThis technique has been developed in some optimising compilers to aid the suppression of run-time checks and exceptions.It is based upon a traversal of the control-flow graph,collecting information about the worst case possible range of variables.This works well in languages such as Ada where strong typing and range-checking are enforced.For example,given the following declarationssubtype A is INTEGER range1..9;subtype B is INTEGER range0..20;X,Y:A;Z:B;a compiler can analyse the following assignment statementZ:=X+Y;and deduce that the worst-case range of the expression X+Y is 2..18using its knowledge of the type A and the properties of the"+"operator.A run-time check that the expression does not exceed the range0..20is therefore eliminated.The worst-case range of variables is constructed using the rules given by Morel[Mor84]for each program construct.This technique is potentially very useful in timing analysis.Knowledge of variable ranges could be used to eliminate or guarantee the execution of certain paths in a program.We will return to this idea in section3.4.2.5.Program dependenciesThe program dependence graph(or PDG)is an alternative representation of a program’s control and data-flow proposed by Ferrante et al[Fer87]which has found use in program testing, debugging and optimisation[Agr90,Pod89,Ott84].It encompasses the relations:‘‘A statement s is dataflow dependent on statement s’if there is a sequence of variable assignments that potentially propagates data from s’to s.’’[Bur91a]‘‘A statement s is control dependent on s’if s’is a conditional branch statement and the control structure potentially allows s’to decide whether s is executed or not.’’(Also from[Bur91a.)Informally,we can imagine each node of the control-flow graph having labels attached to each of its control-flow arcs.These labels may be data values or dataflow expressions which define under which conditions each of the arcs can be traversed.More detailed information can therefore be computed by also considering the data-dependence graph of the program.This is computed by considering the definitions and uses of variables in each basic block.An algorithm for computing the control and data dependencies from the control-flow graph is presented by Ferrante et al[Fer87]and also in[Bur91a].These ideas have found application is many areas.Ferrante cites the following possible uses of the program-dependency graph:Detection of potential parallelism in programs.Automated parallelisation of programs for vector and parallel computers.Optimisation techniques such as invariant code motion.Use as the central data structure in an incremental software development environment.The use of the program-dependency graph in timing analysis will be considered in section 3.4.1.2.6.Sequencing Constraint AnalysisThis technique,developed by Osterweil et al[Ole89,Ole90],seeks to generalise many traditional static analysis techniques into a single model.Osterweil’s observation is that many static analysis techniques are concerned with the detection of violations of constraints on the sequencing of some events in a program.For example,traditional dataflow analysis detects anomalies in the sequences of definitions and uses of program variables.Osterweil therefore aims to generalise this notion to include general sequencing-constraints covering any class of program events.His system comprises two main parts:1)CECIL:A language for expressing sequencing constraints.CECIL is based upon a regular-expression language that allows the specification of alternatives and repetition of events.2)CESAR:A tool that can deduce if a given program will satisfy or violate a given CECILconstraint.These ideas are best illustrated by way of an example.Consider the following program: OPEN;while not DONE loopcompute;WRITE;end loop;CLOSE;A CECIL constraint has the following syntax:{Alphabet}[start-anchor]quantifier reg-exp[end-anchor]Alphabet is the set of events we wish to analyse.The start and end anchors are events which delimit the parts of the program under analysis.The regular expression uses the notation ’;’for sequencing,’*’for0or more repetitions and’+’for1or more repetitions.For example,a CECIL constraint relating to the above program might be:{open,close,write}([s]--start anchorforall(open;write*;close)*[t]--end anchor)This states that between the start of the program(s)and its termination(t),all paths must satisfy the given regular expression.The CESAR tool would,in this case,determine that the program satisfies this constraint.If the regular expression is changed toforall(open;write+;close)*(ie.we require at least one write event)then CESAR determines that the program can violate this constraint(because the value of the DONE expression is unknown.)CECIL is aflexible and expressive language;it can easily express the traditional static analysis constraints-for example,the‘all variables must be defined before use’constraint is expressed in CECIL as follows:{read,define,undefine}forall(read|define)[read]Similar ideas were pursued by Silberschatz et al[Kie83]in their access right expressionnotation.This work,though,concentrated on specifying the sequences of allowable operations on monitors and abstract-data types in languages such as Concurrent-Pascal[Bri75].Their notation is a regular-expression language similar to CECIL,but Silberschatz also realises that the expressions can be translated into deterministicfinite state machines.These can then be incorporated into the program under analysis to provide run-time checking that the constraints are not violated.This idea has potential for use in timing analysis;we will return to this in section3.4.1.3.High level timing analysisThis section looks at the problem of determining the timing characteristics of a high-level program.For hard real-time and safety critical systems,a program should be proven to not only meet its logical specification,but also its timing requirements.Three techniques have emerged to tackle this problem:Testing and measurement.Simulation of the target machine.Worst-case timing analysis.This section is specifically concerned with the third of these.Thefirst two are not entirely appropriate for our needs,especially in hard real-time systems,where the worst-case behaviour of programs must be known.Traditionally,measurement has been used to test the run-time behaviour of a program;this often leads to well-known average case behaviour,but unpredictable performance in the worst-case.The idea of analysing programs to determine their timing has been attempted by various projects.Some have worked at the assembly language level while others have considered the high-level source code of a program.These efforts have all found the following problems:1)Some programming language constructs inherently demand an unbounded amount of time,space,or both.2)A program’s source code does not express enough information about the paths through thecode for a realistic timing analysis to be performed.3)Modern computer architectures are not amenable to timing analysis.In their drive for greaterand greater speed,recent computers have introduced many non-deterministic features.These may improve the overall speed of the CPU,but they are not predictable:a central goal of any timing analysis.4)Worst-case execution times(WCETs)must be produced.Furthermore,these must be non-pessimistic ie.close to the actual worst-case running times.This is required so that processes,input-output,and communication can be scheduled in a system.If the values of WCET for a set of processes are too high,then the system will falsely appear to be unschedulable.We will look at each of these points in more detail below.We start with considering how programming languages must be restricted to enable timing analysis.nguage issuesMost programming languages were not designed with timing analysis in mind.A handful of research languages such as Real-time Euclid[Kli86]and NewSpeak[Cur86]have attacked this idea,but none of these have found widespread nguages such as Ada,C and Pascal include many features that make timing analysis infeasible.These features have to be removed to form a suitable subset of the original language.In particular,we require that:The control-flow graph of a program is reducible.Most static-analysis techniques already rely on this property.All constructs in the language have bounded and predictable timing properties.To this end,current timing analysis systems have enforced the following restrictions:The use of the GOTO statement is prohibited.This would allow the programmer to violatethefirst of the above goals.Recursion.This is particularly difficult to handle,and can also lead to unpredictable space requirements.Although some theory does exist for the analysis of simple,tail-recursive functions,the analysis of general recursive programs remains well beyond the state-of-the-art.Loops must be bounded in some way.Note that this restriction does not ban the use of potentially indefinite‘while’or‘repeat’loops,but merely requires that all loops should terminate within a known,fixed time or number of iterations.Program units,such as procedures and functions,should have a single entry and a single exit point(including error return paths).This simplifies their analysis and allows them to be analysed in isolation.Dynamic memory allocation.Current schemes for the implementation of dynamic memory allocation have unpredictable timing behaviour.Such facilities are therefore not permitted. These restrictions may seem somewhat Draconian,but the reader should remember that timing analysis is still in its infancy.With further experience and research,these language features might be reintroduced.We will consider how this might be achieved in section3.4below.3.2.Simple WCET constructionWe will now consider how the WCET can be constructed for a small fragment of high-level code. We will assume a suitably constrained language,in line with the restrictions above.The basic technique,common to all timing analysis systems,is the composition of basic-blocks.A basic-block(or BB)is defined as a sequence of instructions of maximal length which has a single entry point(at the‘top.’)If one instruction in a BB is executed,then they all are.It is assumed that BBs have a singlefixed worst-case execution time(how this is computed will be discussed in section4.)A timing analysis system must therefore model the control-flow of a program.As an example,consider the following‘if’statement:if(expression){stm1}else{stm2}This comprises of three blocks(expression,stm1,and stm2.)These blocks may themselves be comprised of other basic-blocks.The control-flow graph for this statement looks like this:There are clearly two possible paths through this graph.The worst-case execution time is therefore given by:WCET(if−statement)=WCET(expression)+max(WCET(stm1),WCET(stm2))Of course,stm1and stm2may themselves be complex statement-sequences comprising of many basic blocks.Similar rules are applied to construct WCET‘bottom-up.’The complete set of ‘WCET formulae’used by the MARS timing tool are given below in section3.3.5.This simple-minded approach to timing analysis forms the basis for all current tools.It exhibits the problem,though,that its results are overly pessimistic.This is because the analysis does not take into account any contextual information about the run-time behaviour of the program.For example if the‘expression’part of the above if-statement happened to always have the value‘False’at run-time,then clearly one path through the graph could be eliminated.Our simple timing analysis would not detect this,producing a potentially pessimistic WCET.The same argument applies to most other language constructs(particularly loops):more information is needed than can be supplied by a simple analysis of the source code alone.We therefore turn to the annotations which have been suggested to remedy this situation.3.3.Timing annotationsWe have argued above that the source code of a program alone does not supply enough information to enable to production of a non-pessimistic WCET.So called annotations are therefore needed.The goal of these annotations is to add information to the source code,enabling non-pessimistic WCETs to be constructed.This section will consider what types of annotation have been used in current timing analysis systems.An example of how such annotations are used will also be given.3.3.1.Task specificationThe Real-time Euclid[Kli86]language(or‘RTE’)is a language specifically designed for programming hard real-time systems.Timing information in RTE is embedded in the syntax of the language,not as comments.An RTE program is built from one or more processes which synchronise and communicate using a variant of the monitor[Hoa74].The RTE language allows the programmer to define explicitly the start time and period of a process.For instance,a process with a period of60 seconds and afirst activation at10minutes is written:process NAME:periodic frame60first activation at600This information is use by RTE in its schedulability analysis.This is needed to test whether a multi-process program will meet all its deadlines or not.Real-time Euclid is the only language we will see that includes processes in the core of the language.Other contemporary systems(eg. MARS and Park/Shaw)handle scheduling differently;these will be considered in section5below.3.3.2.Loop annotationsLoops are the most difficult control structure to handle in a timing analysis system.They lead to unpredictable timing and an explosion in the number of possible paths through a program.Timing systems,then,all seek to bound the number of iterations that a loop may perform.This does not imply that the number of iterations is constant(effectively banning the‘while’loop)but that the upper bound on the number of iterations is known.Contemporary timing systems have handled this problem in much the same way.The MARS/C language introduces a new construct called a scope,defined as‘part of a program’s instruction code,limited by a special scope language construct,that is embedded into the syntax of the language.’[Pus89]As loops are the only construct of interest,scopes and loops coincide in MARS/C.The scope construct allows the expression of either a maximum number of iterations or a maximum time for a loop as follows:FOR(i=0;i<MAX_ROWS;i++)SCOPE MAX_COUNT(const_expr)stm1FOR(i=0;i<MAX_ROWS;i++)SCOPE MAX_TIME(const_texpr);stm2The WHILE and REPEAT-UNTIL versions of the scoped loops are similar.A sequence of statements can also be given that will be executed if the bound is exceeded at run-time: FOR(i=0;i<MAX_ROWS;i++)SCOPE MAX_COUNT(const_expr)stm1ON_OVERRUN stm2FOR(i=0;i<MAX_ROWS;i++)SCOPE MAX_TIME(const_texpr)stm1ON_TIMEOUT stm2Note that the loop bounds are checked at run-time.This implies a small overhead,but allows the greaterflexibility and expressive power of the while-loop to be exploited.Time-bounded loops are converted to a maximum number of iterations by the timing analyser.These are also checked at run-time,allowing the ON_OVERRUN or ON_TIMEOUT statement to be executed if a loop breaks its iteration bound.In Real-Time Euclid,loop bounds are expressed as part of the language.Again,bounds may be expressed as a number of iterations or as a time.The original paper[Kli86]gives more details.The timing tool developed by Shaw et al[Par91]takes a very different approach.The tool is designed to produce both best and worst case execution times for program fragments written in a subset of the C language.Loop bounds(lower and upper)are obtained interactively.The tool simply stops when it encounters a loop and asks the programmer what the bounds are.This approach is simple and avoids having to modify the syntax of the source language too much.Onthe other hand,the correctness of user-supplied bounds might be questionable.3.3.3.MarkersThe marker is an annotation introduced in MARS/C to complement the scope.It is defined as follows:‘A Marker is a special mark located within a scope.It specifies the maximal number of times the marked position in the program may be passed by the programflow between entering and leaving the scope.’[Pus89]Markers are used when there is more than one path through the body of a scoped loop body.A marker is usually placed within a computationally expensive path,thus limiting the maximum number of times that that path may be taken.A timing analysis tool can take advantage of this information to favour the cheaper paths through the scope.An example of the use of scopes and markers is given in section3.3.5.It should be noted that markers in MARS are checked at run-time.A count is kept of how often each marker is passed which is incremented and tested by a small code-sequence every time the marked path is executed.This allows an exception to be raised and handled at run-time,but adds a small overhead to the code’s execution time.The developers of MARS argue that this cost is acceptable,given that run-time marker checking gives confidence that markers are indeed correct and even some small level of fault-tolerance.Markers(or any equivalent annotation)are not found in Real-time Euclid or the Park/Shaw system.3.3.4.Loop sequencesThefinal annotation used in MARS/C is the loop sequence.This annotation states that the sum of the bounds for a set of nested loops cannot exceed a given value.Consider the following example: a:FOR(...)MAX_COUNT(10)b:FOR(...)MAX_COUNT(20)Here we see two nested loops with bounds10and20respectively.A normal WCET analysis will assume that the body of loop b will be executed200times.The loop-sequence comes into play when the actual bounds on the loops at run-time complement each other in some way and do not exceed a limit which is smaller than the sum given by adding up the MAX_COUNT annotations.For example:LOOP-SEQUENCE ITERATION_SUM(15)a:FOR(...)IN_SEQUENCE MAX_COUNT(10)b:FOR(...)IN_SEQUENCE MAX_COUNT(20)ON_OVERRUN statementsNow,there are many possible ways that the loops could execute at run-time.The following table shows some of these and the corresponding worst-case iteration count for the body of loop b:。
高中精华双语文章:不紧不慢

高中精华双语文章:不紧不慢在企业中,随处可见“不紧不慢〞的劳动者,这是个普遍现象。
事实上,办公制度专家还未曾遇到过工作效率高于60%的商业网点。
管理部门为进步效率,研究产生了装配线工序,试图减少“不紧不慢〞,为有更大的产量。
Take It Easy不紧不慢How can someone, hour after hour, day after day, year in and year out, tighten approximately the same nut to the same bolt and not go mad? That most working people do not, in fact, going mad is due in large measure to a phenomenon so common that it is found wherever people labor in industry: taking it easy. It would take some kind of real mental case to do all the work one could all day long. No one expects it. Taking it easy on the job while someone else covers your work, or "working on and off," as it is usually called in America, is an established part of the working life.一个人怎么能时复一时,年复一年地把几乎一样的螺帽拧到一样的螺栓上而不发疯的呢?事实上,多数劳动者并不发疯多半是由于"不紧不慢"的现象很平常,在企业中只要有人工作的地方随处可见这种现象。
Escaping Nash Inflation

ESCAPING NASH INFLATIONIN-KOO CHO,NOAH WILLIAMS,AND THOMAS J.SARGENTA BSTRACT.Mean dynamics describe the convergence to self-confirming equilibria of self-referential systems under discounted least squares learning.Escape dynamics recurrentlypropel away from a self-confirming equilibrium.In a model with a unique self-confirmingequilibrium,the escape dynamics make the government discover too strong a version ofthe natural rate hypothesis.The escape route dynamics cause recurrent outcomes close tothe Ramsey(commitment)inflation rate in a model with an adaptive government.Key Words:Self-confirming equilibrium,mean dynamics,escape route,large deviation,natural rate of unemployment,adaptation,experimenta-tion trap.‘If an unlikely event occurs,it is very likely to occur in the most likely way.’Michael Harrison1.I NTRODUCTIONBuilding on work by Sims(1988)and Chung(1990),Sargent(1999)showed how a government adaptivelyfitting an approximating Phillips curve model recurrently sets inflation near the optimal time-inconsistent ouctome,although later inflation creeps back to the time-consistent suboptimal outcome of Kydland and Prescott(1977).The good outcomes emerge when the government temporarily learns the natural rate hypothe-sis.The temporary escapes from the time-consistent outcome symptomize a remarkable type of escape dynamics that promote experimentation and that are induced by unusual shock patterns that interact with the government’s adaptive algorithm and its imperfect model.The escapes lead to dramatic changes in the government’s inflation policy as it temporarily overcomes its inflationary bias.Some simulated time paths of inflation for different specifications of the model are shown in Figure1.Inflation starts and remains near the high time-consistent value for a while,is rapidly cut to zero,but then gradually2IN-KOO CHO,NOAH WILLIAMS,AND THOMAS J.SARGENTF IGURE ofthe model.approaches the time-consistent high value again.This paper explains the dynamic forces that drive these outcomes.Escape dynamics from self-confirming equilibria can occur in a variety of models with large agents who use adaptive algorithms to estimate approximating models.1For con-creteness,this paper focuses on the Phillips curve model studied by Sargent(1999).The model has the following features:(1)the monetary authority controls the inflation rate, apart from a random disturbance;(2)the true data generating mechanism embodies a version of the natural rate hypothesis in an expectational Phillips curve;(3)as in Kydland and Prescott(1977),a purposeful government dislikes inflation and unemployment and a private sector forecasts inflation optimally;but(4)the monetary policy makers don’t know the true data generating mechanism and instead use a goodfitting approximating model.The fundamentals in the economy arefixed,including the true data generating mechanism,preferences,and agents’methods for constructing behavior rules.Changes in the government’s beliefs about the Phillips curve,and how it approximates the natural rate hypothesis,drive the inflation rate.Inspired by econometric work about approximat-ing models by Sims(1972)and White(1982),we endow the monetary authority,not with the correct model,but with an approximating model that it nevertheless estimates with good econometric procedures.We use the concept of a self-confirming equilibrium,a natural equilibrium concept for behavior induced by an approximating model.2In a self-confirming equilibrium,beliefs are correct about events that occur with positive probability in equilibrium.The approxi-mating model is‘wrong’only in its description of events that occur with zero probability in equilibrium.Among the objects determined by a self-confirming equilibrium are theESCAPING NASH INFLATION3 parameters of the government’s approximating model.While the self-confirming equi-librium concept differs formally from a Nash(or time consistent)equilibrium,3it turns out that the self-confirming equilibrium outcomes are the time-consistent ones.Thus,the suboptimal time consistent outcome continues to be our benchmark.Like a Nash equilibrium,a self-confirming equilibrium restricts population objects (mathematical expectations,not sample means).We add adaptation by requiring the government to estimate its model from historical data in real time.We form an adap-tive model by having the monetary authority adjust its behavior rule in light of the latest model estimates.Thus,we attribute‘anticipated utility’behavior(see Kreps(1998))to the monetary authority.Following Sims(1988),we study a‘constant gain’estimation al-gorithm that discounts past observations.Called a‘tracking algorithm’,it is useful when parameter drift is suspected(see e.g.Marcet and Nicolini(1997)).Results from the literature on least squares learning(e.g.,Marcet and Sargent(1989a), Woodford(1990),Evans and Honkapohja(1998))apply and take us part way,but only part way,to our goal of characterizing the dynamics of the adaptive system.That litera-ture shows how the limiting behavior of systems with least squares learning is described by an ordinary differential equation called the‘mean dynamics’.They describe the(un-conditionally)average path of the government’s beliefs,in a sense that we shall describe precisely.For our model,the mean dynamics converge to the self-confirming equilibrium and the time consistent outcome.Thus,the mean dynamics do not account for the recur-rent stabilizations in the simulations of Sims(1988),Chung(1990),and Sargent(1999). We show that these stabilizations are governed by another deterministic component of the dynamics,described by another ODE,the‘escape’dynamics.They point away from the self-confirming equilibrium and toward the Ramsey(or optimal-under-commitment) equilibrium outcome.So two sorts of dynamics dominate the behavior of the adaptive system.(1)The mean dynamics come from an unconditional moment condition,the least squaresnormal equations.These dynamics drive the system toward a self-confirmingequilibrium.4(2)The escape route dynamics propel the system away from a self-confirming equilib-rium.They emerge from the same least squares moment conditions,but they areconditioned on a particular“most likely”unusual event,defined in terms of the disturbance sequence.This most likely unusual event is endogenous.The escape route dynamics have a compelling behavioral interpretation.Within the confines of its approximate model,learning the natural rate hypothesis requires that the government generate a sufficiently wide range of inflation experiments.To learn even an imperfect version of the natural rate hypothesis,the government must experiment more than it does within a self-confirming equilibrium.The government is caught in an experimentation trap.The adaptive algorithm occasionally puts enough movement into the government’s beliefs to produce informative experiments.4IN-KOO CHO,NOAH WILLIAMS,AND THOMAS J.SARGENT1.1.Related literature.Evans and Honkapohja(1993)investigated a model with mul-tiple self-confirming equilibria having different rates of inflation.When agents learn through a recursive least squares algorithm,outcomes converge to a self-confirming equi-librium that is stable under the learning algorithm.When agents use afixed gain algo-rithm,Evans and Honkapohja(1993)demonstrated that the outcome oscillates among different locally stable self-confirming equilibria.They suggested that such a model can explain widefluctuations of market outcomes in response to small shocks.In models like Evans and Honkapohja(1993)and Kasa(1999),the time spent in a neighborhood of a locally stable equilibrium and the escape path from its basin of at-traction are determined by a large deviation property of the recursive algorithm.As the stochastic perturbation disappears,the outcome stays in a neighborhood of a particular locally stable self-confirming equilibrium(exponentially)longer than the others.This observation provided Kandori,Mailath,and Rob(1993)and Young(1993)with a wayto select a unique equilibrium in evolutionary models with multiple locally stable Nash equilibria.An important difference from the preceding literature is that our model has a unique self-confirming equilibrium.Despite that,the dynamics of the model resemble those for models with multiple equilibria such as Evans and Honkapohja(1993).With multiple locally stable equilibria,outcomes escape from the basin of attraction of a locally stable outcome to the neighborhood of another locally stable equilibrium.The fact that our model has a globally unique stable equilibrium creates an additional challenge for us, namely,to characterize the most likely direction of the escape from a neighborhood of the unique self-confirming equilibrium.As we shall see,the most likely direction entails the government’s learning a good,but not self-confirming,approximation to the natural rate hypothesis.anization.Section2describes the model in detail.Section3defines a self-confirming equilibrium.Section4describes a minimal modification of a self-confirming equilibrium formed by giving the government an adaptive algorithm for its beliefs.Section5uses re-sults from the theory of large deviations to characterize convergence to and escape froma self-confirming equilibrium.Section6shows that numerical simulations of escape dy-namics,like those in Sargent(1999),are well described by the numerically calculated theoretical escape paths.For the purpose of giving intuition about the escape dynamics, Section7specializes the shocks to be binomial,then adduces a transformed measure of the shocks that tells how particular endogenously determined unusual shock sequences drive the escape dynamics.Section8concludes.The remainder of this introduction de-scribes the formal structure of the model andfindings of the paper.1.3.Overview.The government’s beliefs about the economy are described by a vector of regression coefficients.It chooses a decision rule that makes the stochastic process for the economy be.But for the stochastic process,the bestfitting model ofthe economy has coefficients.A self-confirming equilibrium is afixed point of .The orthogonality conditions pinning down the bestfitting model can be expressed (1.1)ESCAPING NASH INFLATION5 We shall show thatwhereA self-confirming equilibrium is a set of population regression coefficients.We form an adaptive model by slightly modifying a self-confirming equilibrium.Rather than usingpopulation moments tofit its regression model,the government uses discounted leastsquares estimates from historical samples.We study how the resulting adaptive systemconverges to or diverges from a self-confirming equilibrium.Each period the govern-ment uses the most recent data to update a least squares estimate of its model co-efficients,then sets its policy according to.This is what Kreps(1998)calls an anticipated utility model.The literature on least squares learning in self-referential sys-tems(see Marcet and Sargent(1989a),Marcet and Sargent(1989b),Woodford(1990),andEvans and Honkapohja(2000))give conditions under which the limiting behavior of thegovernment’s beliefs are nearly deterministic and approximated by the following ordi-nary differential equation(ODE)is governed by the uniqueness and stability of the stationary points of the ODE.Our model has a unique self-confirming equilibrium.It supports the high inflationtime-consistent outcome of Kydland and Prescott(1977).The ODE(1.3),(1.4),is veryinformative about the behavior of our adaptive model.It is globally stable about theself-confirming equilibrium,and describes how the adaptive system is gradually drawnto the self-confirming equilibrium.But to understand how the sample paths recurrentlyvisit the better low-inflation outcome,we need more than the ODE(1.3,1.4).Until our work,such‘escape dynamics’had not been characterized analytically.Thispaper shows that they are governed by the ODE6IN-KOO CHO,NOAH WILLIAMS,AND THOMAS J.SARGENTrate hypothesis.Thus,like the mean dynamics,the escape dynamics are deterministic. We verify that these deterministic dynamics do a good job of describing the simulations. As Sims(1988)and Sargent(1999)emphasize,the evolution of beliefs during an es-cape is economically interesting because then the government discovers a good enough approximate version of the natural rate hypothesis to cause it to pursue superior policy that is supported by beliefs that are‘wrong’in the sense that they are not a self-confirming equilibrium.Nevertheless,in another sense those beliefs are more‘correct’than those in a self-confirming equilibrium because they inspire the government to leave the‘experi-mentation trap’that confines it within a self-confirming equilibrium.2.S ETUPTime is discrete and indexed by.Let be an i.i.d.sequence of random vectors with mean zero and covariance matrix.Let,respectively,be the unemployment rate,the rate of inflation,the public’s expected rate of inflation,and the systematic part of inflation determined by government policy.The government sets ,the public sets,then nature chooses shocks that determine and.The economy is described by the following version of a model of Kydland and Prescott(1977):(2.8)(2.9)(2.10)(2.11)where(2.12)Equation(2.8)is a natural rate Phillips curve;(2.9)says that the government sets infla-tion up to a random term;(2.10)imposes rational expectations for the public;(2.11)is the government’s decision rule for setting the systematic part of inflation.The de-cision rule is a function of the government’s beliefs about the economy,which are parameterized by a vector.For some purposes below we consider the simpler model in which the government only estimates a static regression of unemployment on inflation and a constant(i.e. ).We call this the static model.Since there is no temporal dependence in(2.8),(2.9),all of the temporal dependence in the model comes through the government’s beliefs.Under the static model specification,the government’s control rule can be calculated explicitly, allowing some of our characterizations to be sharper.2.1.The government’s beliefs and control problem.The government’s model of the economy is a linear Phillips curve(2.13)where the government treats as a mean zero,serially uncorrelated random term beyond its control.We shall eventually restrict,but temporarily regard it as arbitrary.TheESCAPING NASH INFLATION7 government’s decision rule(2.11)solves the problem:(2.14)where denotes the expectations operator induced by(2.13)and the minimization is subject to(2.13)and(2.9).We call problem(2.14)the Phelps problem.Versions of it were studied by Phelps(1967), Kydland and Prescott(1977),Barro and Gordon(1983),and Sargent(1999).We identify three salient outcomes associated with different hypothetical government’s beliefs: Belief1.If,then the Phelps problem tells the government to set for all.This is the Nash outcome of Sargent(1999),i.e.,the time-consistent outcome of Kydland and Prescott(1977).Belief2.If,for any,the government setsfor all.This is the Ramsey outcome,i.e.,the optimal time-inconsistent outcome of Kydland and Prescott(1977).Belief3.If the coefficients on current and lagged’s sum to zero,then asfrom below,the Phelps problem eventually sends arbitrarily close to.Under the actual probability distribution generated by(2.8),(2.9),(2.10),the value of the government’s objective function(2.14)is larger under the outcome than under outcome.Under Belief1,the government perceives a trade-off between in-flation and unemployment and sets inflation above zero to exploit that trade-off.Under Belief2,the government perceives no trade-off,sets inflation at zero,and accepts what-ever unemployment emerges.Under Belief3,the government thinks that although there is a short-term trade-off between inflation and unemployment when,there is no ‘long-term’trade-off.Through the workings of an‘induction hypothesis’that opens an apparent avenue by which the government can manipulate the current position of the Phillips curve(see Cho and Matsui(1995)and Sargent(1999)),the Phelps problem tells the government eventually to set inflation close to zero when is close to.In a common-knowledge model in which(2.13)is dropped and replaced by the as-sumption that the government knows the model,the outcome emerges as what Stokey(1989)and Sargent(1999)call the Nash outcome,and emerges as the Ram-sey outcome.In the common-knowledge model,these varying outcomes reflect different timing protocols and characterize a time-consistency problem analyzed by Kydland and Prescott.The mapping from government beliefs to outcomes is interesting only when the gov-ernment’s beliefs might be free.Our equilibrium concept,a self-confirming equilibrium, restricts those beliefs,and thereby narrows the outcomes relative to those enumerated above.However,the mapping from beliefs to outcomes play a role during escapes from self-confirming equilibria.8IN-KOO CHO,NOAH WILLIAMS,AND THOMAS J.SARGENT3.S ELF-CONFIRMING EQUILIBRIUM3.1.Restrictions on government’s beliefs.Define and(3.15)Let denote the history of the joint shock process up to.Evidently,from(2.8),(2.9),(2.10),(2.11),and therefore the process are both functions of:(3.16)Definition3.1.A self-confirming equilibrium is a that satisfies(3.17)The expectation in(3.17)is taken with respect to the probability distribution generated by(2.8),(2.9),(2.10),(2.11).Notice that is the time value of the object set to zero by the following least squares orthogonality condition:(3.18)Equations(3.18)are the orthogonality conditions that make in(2.13)a least-squares regression.Condition(3.17)thus renders the government’s beliefs consistent with the data.Condition(3.17)can be interpreted as asserting that is afixed point in a mapping from the government’s beliefs about the Phillips curve to the actual Phillips curve.Thus, let(3.19)and.Then notice that(3.20)(3.22)Given a government model in the form of a perceived regression coefficient vector and the associated government best response function,is the actual least squares regression coefficient induced by.Thus,maps government model to a bestfitting model.Equation(3.22)shows that(3.17)asserts that,so thatESCAPING NASH INFLATION9 the government’s model is the bestfitting model.See Marcet and Sargent(1989a)for a discussion of the operator in a related class of models.Elementary calculations show that there is a unique self-confirming equilibrium.It cor-responds to the beliefs(1)mentioned above.These beliefs support the Nash equilibrium outcome in the sense of Stokey(1989)and Sargent(1999).4.A DAPTATION4.1.Discounted least squares updating of.We modify the model now to consist of (2.8),(2.9),(2.10)as before,but replace(2.11)with(4.23)where remains the best-response function generated by the Phelps problem,and is the government’s time estimate of the empirical Phillips curve.The government estimates by the following recursive least squares algorithm:(4.24)(4.25)where is a gain parameter that determines the weight placed on current observations relative to the past.In this paper we consider the case in which the gain is constant.We want to study the behavior of system formed by(2.8),(2.9),(2.10),(4.23),(4.24)and(4.25).4.2.Mean dynamics.Wefind thefirst important component of dynamics by adapting the stochastic approximation methods used by Woodford(1990),Marcet and Sargent (1989a),and Evans and Honkapohja(2000).We call this component the mean dynamics because it governs the(unconditionally)expected evolution of the government’s beliefs. While previous applications of stochastic approximation results in economics have gener-ally considered recursive least squares with decreasing gain,we consider the case where the gain is constant.5Broadly similar results obtain in the constant and decreasing gain cases,but there are important differences in the asymptotics and the sense of convergence that we discuss below.To present convergence proofs,it helps to group together the components of the gov-ernment’s beliefs into a single vector.Define(4.26)Then the updating equations(4.24),(4.25)can be written(4.27)Now break the“update part”into its expected and random components.Defineis the mean of defined as(4.28)5See Evans and Honkapohja(2000)for extensive discussion of constant gain algorithms.10IN-KOO CHO,NOAH WILLIAMS,AND THOMAS J.SARGENTwhere(4.29)Then we can write the composite dynamics as(4.30))over time.As in the decreasing gain case,we can show that the asymptotic behavior of(4.30)is governed by an ODE,but the estimates converge in a weaker sense.Specifically,decreas-ing gain algorithms typically converge with probability one along a sequence of iterations as,but constant gain algorithms converge weakly(or in distribution)as across sequences of iterations,each of which is indexed by the gain.Note that we can rewrite(4.30)as(4.31)This equation resembles afinite-difference approximation of a derivative with time step ,but is perturbed by a noise term.The convergence argument defines a continuous time scale as,and interpolates between the discrete iterations to get a continuous process.Then by letting,the approximation error in thefinite difference goes to zero,and a weak law of large numbers insures that the noise term becomes negligible. We are left with the ODE:(4.33)We need the following set of assumptions.For reference,we also list the original num-ber in Kushner and Yin(1997).To emphasize the asymptotics,we include the superscript on the parameters denoting the gain setting.Assumptions A.A8.5.0:The random sequence is tight.6A8.5.1:For each compact set is uniformly integrable.7A8.5.3:For each compact set the sequence6A random sequence is tight if7A random sequence is uniformly integrable ifA8.5.4a:The ODE that is asymptotically stable.8A8.1.6:The functionthat is the self-confirming equilibrium,the estimate sequence converges weakly to the self-confirming equilibrium.Therefore,with high probability,as and we would expect the government’s beliefs to be near their self-confirming values,and the economy to be near the Nash outcome.However,in the next section we shall see that the beliefs can recur-rently escape the self-confirming equilibrium.Although the impact of noise terms goes to zero with the gain,for a given,“rare”sequences of shocks can have a large impact on the estimates and the economy.5.E SCAPEIn this section we determine the most likely rare events and how they push the gov-ernment’s beliefs away from a self-confirming equilibrium.To this end,wefirst present some general results from the theory of large deviations,a general method for analyzing small probability events.We then present results from Williams(2000),who applies these general results analytically to characterize the escape dynamics.5.1.Escape dynamics as a control problem.Throughout,we will only be interested in characterizing the escape problem for the Phillips curve coefficients.This motivates the following definition.Definition5.1.An escape path is a sequence of estimates that leave a set containing the limit pointfor someFollowing a convention in the large deviation literature,we set the initial point of an escape path to be the stable point,let be the set of all escape paths.For each,define8A point as and for each there exists an such that if for allDefinition5.2.Let be the(first)exit time associated with escape path. An absolutely continuous trajectory is a dominant escape path ifwill occur along with very high probability,if an escape ever occurs.To analyze the escape dynamics,we adapt the general results of Dupuis and Kushner (1989),which are themselves extensions of the theory of Freidlin and Wentzell(1984) for stochastic approximation models.After presenting some general results,we apply results of Williams(2000),who obtains explicit solutions of the escape dynamics that can be used to interpret the simulations calculated earlier by Sims(1988),Chung(1990),and Sargent(1999).Given the recursive formula(4.30),define the-functional as(5.35),and with the evolution of following the mean dynamics conditional on .(We let for trajectories that are not absolutely continuous.)In the context of continuous time diffusions,Freidlin and Wentzell(1984)characterized the dominant escape path as a solution of a variational problem.Their results have been extended to discrete time stochastic approximation models by Dupuis and Kushner(1985)and Dupuis and Kushner(1989).We adapt these results in the following theorem,whose main object is the solution of the following variational problem:(5.38)for someThe minimized value(1)Suppose that the shocks are i.i.d.and unbounded but that there exists a algebraand constants such that for all anda.s.Then we have:for some(2)If the shocks are i.i.d.and bounded,andbe the terminal point of the dominant escape path.Then for any and:.The next three parts establish stronger results under the assumption that the errors are bounded.Part(2)shows that under bounded errors,the asymptotic inequality in part(1)becomes an asymptotic equality. Part(3)shows that for small the time it takes beliefs to escape the self-confirming equi-librium becomes close to.It is known(see Benveniste,Metivier,and Priouret(1990)for example)that the asymptotic distribution of Markov processes can be characterized by the Poisson equa-tion,so it is natural that it appears here.This analysis then leads to a representation of the-functional as a quadratic form in,with a normalizing matrix that depends on the solution of the Poisson equation associated with.In general the solution of the Poisson equation can itself be a difficult problem,as it involves solving a functional equation.However in the important linear-quadratic-Gaussian case(which includes our model),the problem can be solved in the space of quadratic functions,and therefore the Poisson equation reduces to a matrix Lyapunov equation.This provides a tremendous simplification,as there are efficient numerical methods for solving Lyapunov equations. We summarize these arguments in the following theorem and remark.Theorem5.4.Suppose that Assumptions A hold,that follows a stationary functional au-toregression with a unique stationary distribution and Lipschitz continuous mean and variance functions,and that the function is Lipschitz continuous in.Then there is a matrix-valued function such that the dominant escape path and rate function can be determined by solving the following variational problem:(5.39)subject to(5.41)(5.42)for someProof.See Williams(2000).Remark5.5.In our model,follows a linear autoregression,the are i.i.d.normal,and is a quadratic function of.Then is a fourth moment matrix that can be calculated explicitly by solving matrix Lyapunov equations described in Appendix C.This theorem provides a clearer interpretation and analysis of the variational problem. The escape dynamics perturb the mean dynamics by a forcing sequence.Then is a quadratic cost function that measures the magnitude of the perturbations during the episode of an escape.In particular,we can think of(5.39)as a least squares problem, where plays the role of a covariance matrix.If we had then the beliefs adhere to the mean dynamics,and the cost would be zero.For the beliefs to escape from.Tofind the dominant escape path,we solve the control problem in(5.39).We form the Hamiltonian with co-states for the evolution of:It is easy to verify that the Hamiltonian is convex,so thefirst order conditions are nec-essary and sufficient.Taking thefirst order conditions,we see that the dominant escape path is characterized by the following set of differential equations:The path that achieves the minimum is the dominant escape path.This path characterizes the evolution of the parameters on the most likely path away from the stable point.The minimized value.There is a unique self-confirming equilibrium,depicted in Figure2.It has.To solve the problem numerically,it helps to recast the boundary value problem as an initial value problem.In the ODE system(5.43)and boundaries(5.42),the only com-ponents left undetermined are the initial conditions for the co-states.We can solve the problem by minimizing over these initial conditions,and determine the escape times and。
浙江省杭州2022-2023学年高二下学期3月月考英语试题含解析

A.thatB.whatC.whetherD.where
【答案】D
【答案】infectious##nfectious
【解析】
【详解】考查形容词。句意:笑是最具感染力的表达方式之一。根据句意和首字母提示可知,此处填形容词infectious“感染的”,放在expressions前作定语,故填infectious。
3.S________nothing, he walked right into the trap.(根据首字母单词拼写)
【点睛】名词性从句考查的关键是连接词的选用和语序,因此,我们首先要搞清从句的性质,掌握各连接词的用法,在此基础上判断.此外,关注名词性从句用陈述语序.题干中is being accepted 是谓语动词,前面是主语从句,第一个空用that引导,因为主语从句中不缺少成分,也不需要翻译,第二个空是be动词后面的表语从句的引导词,表示"职业",用whatever,去掉逗号中间插入的部分可知,is前的主语从句"girls can be whatever they would like to be"从意思到成分都是完整的,故选择在名词性从句中不担任成分,无意义的that,
14.________leaves the classroom last should turn off the lights.
A.WhichB.WhoC.WhicheverD.Whoever
conservation of linear momentum
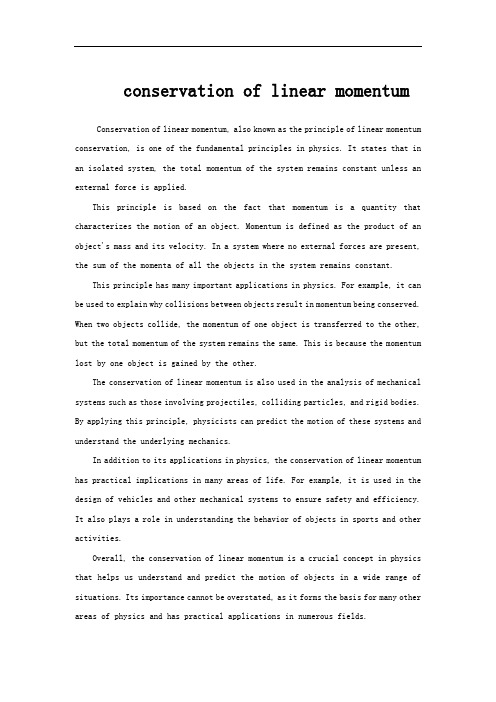
conservation of linear momentumConservation of linear momentum, also known as the principle of linear momentum conservation, is one of the fundamental principles in physics. It states that in an isolated system, the total momentum of the system remains constant unless an external force is applied.This principle is based on the fact that momentum is a quantity that characterizes the motion of an object. Momentum is defined as the product of an object's mass and its velocity. In a system where no external forces are present, the sum of the momenta of all the objects in the system remains constant.This principle has many important applications in physics. For example, it can be used to explain why collisions between objects result in momentum being conserved. When two objects collide, the momentum of one object is transferred to the other, but the total momentum of the system remains the same. This is because the momentum lost by one object is gained by the other.The conservation of linear momentum is also used in the analysis of mechanical systems such as those involving projectiles, colliding particles, and rigid bodies. By applying this principle, physicists can predict the motion of these systems and understand the underlying mechanics.In addition to its applications in physics, the conservation of linear momentum has practical implications in many areas of life. For example, it is used in the design of vehicles and other mechanical systems to ensure safety and efficiency. It also plays a role in understanding the behavior of objects in sports and other activities.Overall, the conservation of linear momentum is a crucial concept in physics that helps us understand and predict the motion of objects in a wide range of situations. Its importance cannot be overstated, as it forms the basis for many other areas of physics and has practical applications in numerous fields.。
华科奥本海姆讲义三

T
H(s) or H(z) is in general a function of the complex variable s or z .
Eigenfunction( of the system): an input signal for which the system output is a constant times the input.
1, xt 0, t T1 T1 t T 2
…
-T -T/2 -T1 T1 T/2 T
x(t) … t
T1 T1
Represent it in Fourier series.
2T1 1 a0 dt T T1 T
T1
1 T1 jk0t 1 ak e dt e jk 0t T T1 jk 0T
x(t )
k
a e
k
jk0 t
0 2 / T
where the coefficients ak is generally a complex function of k0 . The signals in the set e jk0 t , k 0, 1, 2, are harmonically related complex exponentials.
synthesis equation: analysis equation:
1 ak T
T
- 1、下载文档前请自行甄别文档内容的完整性,平台不提供额外的编辑、内容补充、找答案等附加服务。
- 2、"仅部分预览"的文档,不可在线预览部分如存在完整性等问题,可反馈申请退款(可完整预览的文档不适用该条件!)。
- 3、如文档侵犯您的权益,请联系客服反馈,我们会尽快为您处理(人工客服工作时间:9:00-18:30)。
a r X i v :0801.1987v 2 [c s .D S ] 13 M a r 2013n ).For moderately dense instances –as long asr +c =o (n/log n )–the 1/ε2factor multiplies a sub-linear term.Generally,the time is linear in the input size n as long as ε≥Ω(Department of Computer Science and Engineering,University of California,Riverside.The first author would like to thank the Greek State Scholarship Foundation (IKY).The second author’s research was partially supported by NSF grants 0626912,0729071,and 1117954.1Accepted to Algorithmica,2013.The conference version of this paper was “Beating Simplex for fractional packing and covering linear programs”[13].The running times of algorithms of this type increase as the approximation parameterεgets small.For algorithms that rely on linear approximation of the penalty changes in each iteration,the running timesgrow at least quadratically in1/ε(times a polynomial in the other parameters).For explicitly given packing and covering,the fastest previous such algorithm that we know of runs in time O((r+c)¯c log(n)/ε2),where¯c is the maximum number of columns in which any variable appears[21].That algorithm applies to mixed packing and covering—a more general ing some of the techniques in this paper,one canimprove that algorithm to run in time O(n log(n)/ε2)(an unpublished result),which is slower than the algorithm here for dense problems.Technically,the starting point for the work here is a remarkable algorithm by Grigoriadis and Khachiyan for the following special case of packing and covering[9].The input is a two-player zero-sum matrix gamewith payoffs in[−1,1].The output is a pair of mixed strategies that guarantee an expected payoffwithin an additiveεof optimal.(Note that achieving additive errorεis,however,easier than achieving multiplicative error1+ε.)The algorithm computes the desired output in O((r+c)log(n)/ε2)time.This is remarkable in that,for dense matrices,it is sub-linear in the input size n=Θ(rc).2(For a machine-learning algorithm closely related to Grigoriadis and Khachiyan’s result,see[5,6].)We also use the idea of non-uniform increments from algorithms by Garg and K¨o nemann[8,12,7].Dependence onε.Building on work by Nesterov(e.g.,[16,17]),recent algorithms for packing and cov-ering problems have reduced the dependence on1/εfrom quadratic to linear,at the expense of increased dependence on other parameters.Roughly,these algorithms better approximate the change in the penaltyfunction in each iteration,leading to fewer iterations but more time per iteration(although not to the same extent as interior-point algorithms).For example,Bienstock and Iyengar give an algorithm for concurrentmulticommodityflow that solves O∗(ε−1k1.5|V|0.5)shortest-path problems,where k is the number of com-modities and|V|is the number of vertices[3].Chudak and Eleuterio continue this direction—for example,they give an algorithm for fractional set cover running in worst-case time O∗(c1.5(r+c)/ε+c2r)[4].Comparison to Simplex and Interior-Point methods.Currently,the most commonly used algorithms for solving linear programs in practice are Simplex and interior-point methods.Regarding Simplex algorithms, commercial implementations algorithms use many carefully tuned heuristics(e.g.pre-solvers and heuristics for maintaining sparsity and numerical stability),enabling them to quickly solve many practical problems with millions of non-zeros to optimality.But,as is well known,their worst-case running times are expo-nential.Also,for both Simplex and interior-point methods,running times can vary widely depending on the structure of the underlying problem.(A detailed analysis of Simplex and interior-point running times is outside the scope of this paper.)These issues make rigorous comparison between the various algorithms difficult.Still,here is a meta-argument that may allow some meaningful comparison.Focus on“square”con-straint matrices,where r=Θ(c).Note that at a minimum,any Simplex implementation must identify a non-trivial basic feasible solution.Likewise,interior-point algorithms require(in each iteration)a Cholesky decomposition or other matrix factorization.Thus,essentially,both methods require implicitly(at least) solving an r×r system of linear equations.Solving such a system is a relatively well-understood problem, both in theory and in practice,and(barring special structure)takesΩ(r3)time,orΩ(r2.8)time using Strassen’s algorithm.Thus,on“square”instances,Simplex and interior-point algorithms should have run-ning times growing at least withΩ(r2.8)(and probably more).This reasoning applies even if Simplex or interior-point methods are terminated early so as tofind approximately optimal solutions.In comparison,on“square”matrices,the algorithm in this paper takes time O(n+r log(r)/ε2)where n=O(r2)or less.If the meta-argument holds,then,for applications where(1+ε)-approximate solutions suffice for somefixed and moderateε(say,ε≈1%),for very large instances(say,r≥104),the algorithm here should be orders of magnitude faster than Simplex or interior-point algorithms.This conclusion is consistent with experiments reported here,in which the running times of Simplex andinterior-point algorithms on large random instances exceedΩ(r2.8).Concretely,withε=1%,the algorithm here is faster when r is on the order of103,with a super-linear(in r)speed-up for larger r.1.2Technical roadmapBroadly,the running times of iterative optimization algorithms are determined by(1)the number of iter-ations and(2)the time per iteration.Various algorithms trade offthese two factors in different ways.The technical approach taken here is to accept a high number of iterations—(r+c)log(n)/ε2,a typical bound for an algorithm of this class(see e.g.[11]for further discussion)—and to focus on implementing each iteration as quickly as possible(ideally in constant amortized time).Coupling.Grigoriadis and Khachiyan’s sub-linear time algorithm uses an unusual technique of coupling primal and dual algorithms that is critical to the algorithm here.As a starting point,to explain coupling, consider the following“slow”coupling algorithm.(Throughout,assume without loss of generality by scaling that a j=b i=1for all i,j.)The algorithm starts with all-zero primal and dual solutions,x andˆx, respectively.In each iteration,it increases one coordinate x j of the primal solution x by1,and increases one coordinateˆx i of the dual solutionˆx by1.The index j of the primal variable to increment is chosen randomly from a distributionˆp that depends on the current dual solution.Likewise,the index i of the dual variable to increment is chosen randomly from a distribution p that depends on the current primal solution.The distributionˆp is concentrated on the indices of dual constraints M Tˆx that are“most violated”byˆx.Likewise,the distribution p is concentrated on the indices of primal constraints Mx that are“most violated”by x.Specifically,p i is proportional to(1+ε)M i x,whileˆp j is proportional to(1−ε)M T jˆx.3 Lemma1in the next section proves that this algorithm achieves the desired approximation guarantee. Here,broadly,is why coupling helps reduce the time per iteration in comparison to the standard approach. The standard approach is to increment the primal variable corresponding to a dual constraint that is“most violated”by p—that is,to increment x j′where j′(approximately)minimizes M T j′p(for p defined as above). This requires at a minimum maintaining the vector M T p.Recall that p i is a function of M i x.Thus,a change in one primal variable x j′changes many entries in the vector p,but even more entries in M T p.(In the r×c bipartite graph G=([r],[c],E)where E={(i,j):M ij=0},the neighbors of j′change in p,while all neighbors of those neighbors change in M T p.)Thus,maintaining M T p is costly.In comparison,to implement coupling,it is enough to maintain the vectors p andˆp.The further product M T p is not needed(nor is Mˆp). This is the basic reason why coupling helps reduce the time per iteration.Non-uniform increments.The next main technique,used to make more progress per iteration,is Garg and K¨o nemann’s non-uniform increments[8,12,7].Instead of incrementing the primal and dual variables by a uniform amount each time(as described above),the algorithm increments the chosen primal and dual variables x j′andˆx i′by an amountδi′j′chosen small enough so that the left-hand side(LHS)of each constraint(each M i x or M T jˆx)increases by at most1(so that the analysis still holds),but large enough so that the LHS of at least one such constraint increases by at least1/4.This is small enough to allow the same correctness proof to go through,but is large enough to guarantee a small number of iterations.The number of iterations is bounded by(roughly)the following argument:each iteration increases the LHS of some constraint by1/4,but,during the course of the algorithm,no LHS ever exceeds N≈log(n)/ε2.(The particular N is chosen with foresight so that the relative error works out to1+ε.)Thus,the number of iterations is O((r+c)N)=O((r+c)log(n)/ε2).Using slowly changing estimates of Mx and M Tˆx.In fact,we will achieve this bound not just for the number of iterations,but also for the total work done(outside of pre-and post-processing).The key to this is the third main technique.Most of the work done by the algorithm as described so far would be in maintaining the vectors Mx and M Tˆx and the distributions p andˆp(which are functions of Mx and M Tˆx). This would require lots of time in the worst case,because,even with non-uniform increments,there can still be many small changes in elements of Mx and M Tˆx.To work around this,instead of maintaining Mx and M Tˆx exactly,the algorithm maintains more slowly changing estimates for them(vectors y andˆy,respectively), using random sampling.The algorithm maintains y≈Mx as follows.When the algorithm increases a primal variable x j′during an iteration,this increases some elements in the vector Mx(specifically,the elements M i x where M ij>0).For each such element M i x,if the element increases by,say,δ≤1,then the algorithm increases the corresponding y i not byδ,but by1,but only with probabilityδ.This maintains not only E[y i]=M i x,but also,with high probability,y i≈M i x.Further,the algorithm only does work for a y i (e.g.updating p i)when y i increases(by1).The algorithm maintains the estimate vectorˆy≈M Tˆx similarly, and defines the sampling distributions p andˆp as functions of y andˆy instead of Mx and M Tˆx.In this wayeach unit of work done by the algorithm can be charged to an increase in|Mx|+|M Tˆx|(or more precisely, an increase in|y|+|ˆy|,which never exceeds(r+c)N).(Throughout the paper,|v|denotes the1-norm of any vector v.)Section2gives the formal intuition underlying coupling by describing and formally analyzing thefirst (simpler,slower)coupling algorithm described above.Section3describes the full(main)algorithm and its correctness proof.Section4gives remaining implementation details and bounds the run time.Section5 presents basic experimental results,including a comparison with the GLPK Simplex algorithm.1.3PreliminariesFor the rest of the paper,assume the primal and dual problems are of the following restricted forms, respectively:max{|x|:Mx≤1,x≥0},min{|ˆx|:M Tˆx≥1,ˆx≥0}.That is,assume a j=b i=1for each i,j.This is without loss of generality by the transformation M′ij=M ij/(b i a j).Recall that|v|denotes the 1-norm of any vector v.2Slow algorithm(coupling)To illustrate the coupling technique,in this section we analyze thefirst(simpler but slower)algorithm described in the roadmap in the introduction,a variant of Grigoriadis and Khachiyan’s algorithm[9].We show that it returns a(1−2ε)-approximate primal-dual pair with high probability.We do not analyze the running time,which can be large.In the following section,we describe how to modify this algorithm(using non-uniform increments and the random sampling trick described in the previous roadmap)to obtain the full algorithm with a good time bound.For just this section,assume that each M ij∈[0,1].(Assume as always that b i=a j=1for all i,j;recall that|v|denotes the1-norm of v.)Here is the algorithm:4It may be instructive to compare this algorithm to the more standard algorithm.In fact there are two standard algorithms related to this one:a primal algorithm and a dual algorithm.In each iteration,the primal algorithm would p and increments x j′.Separately and simultaneously,the dual algorithm would choose i′to choose j′to minimize M Tj′maximize(Mˆp)i′,then incrementsˆx i′.(Note that the primal algorithm and the dual algorithm are independent,and in fact either can be run without the other.)To prove the approximation ratio for the primal algorithm,one would bound the increase in|p|relative to the increase in the primal objective|x|.To prove the approximation ratio for the dual algorithm,Lemma1The slow algorithm returns a(1−2ε)-approximate primal-dual pair(feasible primal and dual solutions x⋆andˆx⋆such that|x⋆|≥(1−2ε)|ˆx⋆|)with probability at least1−1/(rc).Proof In a given iteration,let p andˆp denote the vectors at the start of the iteration.Let p′andˆp′denote the vectors at the end of the iteration.Let∆x denote the vector whose j th entry is the increase in x j during the iteration(or if z is a scalar,∆z denotes the increase in z).Then,using that each∆M i x=M ij′∈[0,1],|p′|=ip i(1+ε)M i∆x≤ip i(1+εM i∆x)=|p| 1+εp T|p|TM∆x−ε∆ˆx T Mˆpone would bound the decrease in|ˆp|relative to the increase in the dual objective|ˆx|.In this view,the coupled algorithm can be obtained by taking these two independent primal and dual algorithms and randomly coupling their choices of i′and j′.The analysis of the coupled algorithm uses as a penalty function|p||ˆp|,the product of the respective penalty functions |p|,|ˆp|of the two underlying algorithms.To implement the above non-uniform increments and the adjusted sampling distribution,the algorithm maintains the following data structures as a function of the current primal and dual solutions x andˆx:a set J of indices of still-active(not yet met)covering constraints(columns);for each column M T j its maximum entry u j=max i M ij;and for each row M i a close upper boundˆu i on its maximum active entry max j∈J M ij (specifically,the algorithm maintainsˆu i∈[1,2]×max j∈J M ij).Then,the algorithm takes the incrementδi′j′to be1/(ˆu i′+u j′).This seemingly odd choice has two key properties:(1)It satisfiesδi′j′=Θ(1/max(ˆu i′,u j′)),which ensures that when x j′andˆx i′are increased by δi′j′,the maximum increase in any LHS(any M i x,or M T jˆx with j∈J)isΩ(1).(2)It allows the algorithm to select the random pair(i′,j′)in constant time using the following subroutine,called random-pair(the notation p׈u denotes the vector with i th entry p iˆu i):r ×c+,ε)—return a (1−6ε)-approximate primal-dual pair w/high prob.1.Initialize vectors x,ˆx ,y,ˆy ←0,and scalar N =⌈2ln(rc )/ε2⌉.2.Precompute u j .=max {M ij :i ∈[r ]}for j ∈[c ].(The max.entry in column M j .)As x and ˆx are incremented,the alg.maintains y and ˆy so E[y ]=Mx ,E[ˆy ]=M T ˆx .It maintains vectors p defined by p i .=(1+ε)y i and,as a function of ˆy:J .={j ∈[c ]:ˆy j ≤N }(the active columns)ˆu i ∈[1,2]×max {M ij :j ∈J }(approximates the max.active entry in row i of M )ˆp j .= (1−ε)ˆy j if j ∈J 0otherwise.It maintains vectors p ׈u and ˆp ×u ,where a ×b is a vector whose i th entry is a i b i .3.Repeat until max i y i =N or min j ˆy j =N :4.Let (i ′,j ′)←random-pair (p,ˆp ,p ׈u ,ˆp ×u ).5.Increase x j ′and ˆx i ′each by the same amount δi ′j ′.=1/(ˆu i ′+u j ′).6.Update y ,ˆy ,and the other vectors as follows:7.Choose random β∈[0,1]uniformly,and 8.for each i ∈[r ]with M ij ′δi ′j ′≥β,increase y i by 19.(and multiply p i and (p ׈u )i by 1+ε);10.for each j ∈J with M i ′j δi ′j ′≥β,increase ˆy j by 111.(and multiply ˆp j and (ˆp ×u )j by 1−ε).12.For each j leaving J ,update J ,ˆu ,and p ׈u .13.Let (x ⋆,ˆx ⋆).=(x/max i M i x,ˆx /min j M T j ˆx ).Return (x ⋆,ˆx ⋆).Fig.1The full algorithm.[i ]denotes {1,2,...,i }.Implementation details are in Section 4.Lemma 2In each iteration,1.The largest change in any relevant LHS is at least 1/4:max {max i ∆M i x,max j ∈J∆M T j ˆx }∈[1/4,1].2.Let α.=|p ||ˆp |/ ij p i ˆp j /δij .The expected changes in each x j ,x j ,y i ,ˆy j satisfyE [∆x j ]=αˆp j /|ˆp |,E[∆y i ]=E[∆M i x ]=αM ˆp i /|ˆp |,E[∆ˆx i ]=αp i /|p |,E[∆ˆy j ]=E[∆M T j ˆx ]=αM T p j /|p |.Proof (i)By the choice of ˆu and u ,for the (i ′,j ′)chosen,the largest change in a relevant LHS isδi ′j ′max max i M ij ′,max j ∈J M i ′j∈[1/2,1]δi ′j ′max(ˆu i ′,u j ′)⊆[1/4,1]δi ′j ′(ˆu i ′+u j ′)=[1/4,1].(ii)First,we verify that the probability that random-pair returns a given (i,j )is α(p i /|p |)(ˆp j /|ˆp |)/δij .Here is the calculation.By inspection of random-pair,the probability is proportional to|p ׈u ||ˆp |p i ˆu i|ˆp |+|p ||ˆp ×u |p i|ˆp ×u |which by algebra simplifies to p i ˆp j (ˆu i +u j )=p i ˆp j /δij .Hence,the probability must be α(p i /|p |)(ˆp j /|ˆp |)/δij ,because the choice of αmakes the sum over all i and j of the probabilities equal 1.Next,note that part (i)of the lemma implies that in line 8(given the chosen i ′and j ′)the probability that a given y i is incremented is M ij ′δi ′j ′,while in line 10the probability that a given ˆy j is incremented is M i ′j δi ′j ′.Now,the remaining equalities in (ii)follow by direct calculation.For example:E[∆x j ]= i (αp i /|p |)(ˆp j /|ˆp |)/δij )δij =αˆp j /|ˆp |.⊓⊔The next lemma shows that(with high probability)the estimate vectors y andˆy suitably approximate Mx and M Tˆx,respectively.The proof is simply an application of an appropriate Azuma-like inequality (tailored to deal with the random stopping time of the algorithm).Lemma31.For any i,with probability at least1−1/(rc)2,at termination(1−ε)M i x≤y i+εN.2.For any j,with probability at least1−1/(rc)2,after the last iteration with j∈J,it holds that(1−ε)ˆy j≤M T jˆx+εN.Proof(i)By Lemma2,in each iteration each M i x and y i increase by at most1and the expected increases in these two quantities are the same.So,by the Azuma inequality for random stopping times(Lemma10), Pr[(1−ε)M i x≥y i+εN]is at most exp(−ε2N)≤1/(rc)2.This proves(i).The proof for(ii)is similar,noting that,while j∈J,the quantity M T jˆx increases by at most1each iteration.⊓⊔Finally,here is the main utility lemma.Recall that the heart of the analysis of the slow algorithm (Lemma1)was showing that in expectation|p||ˆp|was non-increasing.This allowed us to conclude that (with high probability at the end)max i M i x was not much larger than min j M T jˆx.This was the key to proving the approximation ratio.The next lemma gives the analogous argument for the full algorithm.It shows that the quantity|p||ˆp| is non-increasing in expectation,which,by definition of p andˆp,implies that(with high probability at the end)max i y i is not much larger than min jˆy j.The proof is essentially the same as that of Lemma1,but with some technical complications accounting for the deletion of covering constraints.Since(with high probability by Lemma3)the estimates y andˆy approximate Mx and Mˆx,respectively, this implies that(with high probability at the end)max i M i x is not much larger than min j M T jˆx.Since the algorithm maintains|x|=|ˆx|,this is enough to prove the approximation ratio.Lemma4With probability at least1−1/rc,when the algorithm stops,max i y i≤N and min jˆy j≥(1−2ε)N. Proof Let p′andˆp′denote p andˆp after a given iteration,while p andˆp denote the values before the iteration.We claim that,given p andˆp,E[|p′||ˆp′|]≤|p||ˆp|—with each iteration|p||ˆp|is non-increasing in expectation.To prove it,note|p′|= i p i(1+ε∆y i)=|p|+εp T∆y and,similarly,|ˆp′|=|ˆp|−εˆp T∆ˆy(recall ∆y i,∆ˆy j∈{0,1}).Multiplying these two equations and dropping a negative term gives|p′||ˆp′|≤|p||ˆp|+ε|ˆp|p T∆y−ε|p|ˆp T∆ˆy.The claim follows by taking expectations of both sides,then,in the right-hand side applying linearity of expectation and substituting E[∆y]=αMˆp/|ˆp|and E[∆ˆy]=αM T p/|p|from Lemma2.By Wald’s equation(Lemma9),the claim implies that E[|p||ˆp|]for p andˆp at termination is at most its initial value rc.Applying the Markov bound,with probability at least1−1/rc,at termination max i p i max jˆp j≤|p||ˆp|≤(rc)2≤exp(ε2N).Assume this event happens.The index set J is not empty at termination,so the minimumˆy j is achieved for j∈J.Substitute in the definitions of p i andˆp j and take log to get max i y i ln(1+ε)≤min jˆy j ln(1/(1−ε))+ε2N.Divide by ln(1/(1−ε)),apply1/ln(1/(1−ε))≤1/εand also ln(1+ε)/ln(1/(1−ε))≥1−ε.This gives (1−ε)max i y i≤min jˆy j+εN.By the termination condition max i y i≤N is guaranteed,and either max i y i=N or min jˆy j=N.If min jˆy j=N,then the event in the lemma occurs.If not,then max i y i=N,which(with the inequality in previous paragraph)implies(1−ε)N≤min jˆy j+εN,again implying the event in the lemma.⊓⊔Finally,here is the approximation guarantee(Theorem1).It follows from the three lemmas above by straightforward algebra.Theorem1With probability at least1−3/rc,the algorithm in Fig.1returns feasible primal and dual solutions(x⋆,ˆx⋆)with|x⋆|/|ˆx⋆|≥1−6ε.Proof Recall that the algorithm returns(x⋆,ˆx⋆).=(x/max i M i x,ˆx/min j M T jˆx).By the naive union bound,with probability at least1−3/rc(for all i and j)the events in Lemma3occur,and the event in Lemma4 occurs.Assume all of these events happen.Then,at termination,for all i and j,(1−ε)M i x≤y i+εN(1−2ε)N≤ˆy jy i≤N and(1−ε)ˆy j≤M T jˆx+εN.By algebra,using(1−a)(1−b)≥1−a−b and1/(1+ε)≥1−ε,it follows for all i and j that(1−2ε)M i x≤N and(1−4ε)N≤M T jˆx.This implies min j M T jˆx/max i M i x≥1−6ε.The scaling at the end of the algorithm assures that x⋆andˆx⋆are feasible.Since the sizes|x|and |ˆx|increase by the same amount each iteration,they are equal.Thus,the ratio of the primal and dual objectives is|x⋆|/|ˆx⋆|=min j M T jˆx/max i M i x≥1−6ε.⊓⊔4Implementation details and running timeThis section gives remaining implementation details for the algorithm and bounds the running time.The remaining implementation details concern the maintenance of the vectors(x,ˆx,y,ˆy,p,ˆp,u,ˆu,p׈u,ˆp×u)so that each update to these vectors can be implemented in constant time and random-pair can be implemented in constant time.The matrix M should be given in any standard sparse representation,so that the non-zero entries can be traversed in time proportional to the number of non-zero entries.4.1Simpler implementationFirst,here is an implementation that takes O(n log n+(r+c)log(n)/ε2)time.(After this we describe how to modify this implementation to remove the log n factor from thefirst term.)Theorem2The algorithm can be implemented to return a(1−6ε)-approximate primal-dual pair for packing and covering in time O(n log n+(r+c)log(n)/ε2)with probability at least1−4/rc.Proof To support random-pair,store each of the four vectors p,ˆp,p׈u,ˆp×u in its own random-sampling data structure[15](see also[10]).This data structure maintains a vector v;it supports random sampling from the distribution v/|v|and changing any entry of v in constant time.Then random-pair runs in constant time,and each update of an entry of p,ˆp,p׈u,orˆp×u takes constant time.Updating the estimates y andˆy in each iteration requires,given i′and j′,identifying which j and i are such that M i′j and M ij′are at leastβ/δi′j′(the corresponding elements y i andˆy j get increased).To support this efficiently,at the start of the algorithm,preprocess the matrix M.Build,for each row and column,a doubly linked list of the non-zero entries.Sort each list in descending order.Cross-reference the lists so that,given an entry M ij in the i th row list,the corresponding entry M ij in the j th column list can be found in constant time.The total time for preprocessing is O(n log n).Now implement each iteration as follows.Let I t denote the set of indices i for which y i is incremented in line8in iteration t.From the randomβ∈[0,1]and the sorted list for row j′,compute this set I t by traversing the list for row j′in order of decreasing M ij′,collecting elements until an i with M ij′<β/δi′j′is encountered.Then,for each i∈I t,update y i,p i,and the i th entry in p׈u in constant time.Likewise, let J t denote the set of indices j for whichˆy j is incremented in pute J t from the sorted list for column i′.For each j∈J t,updateˆp j,and the j th entry inˆp×u.The total time for these operations during the course of the algorithm is O( t1+|I t|+|J t|).For each element j that leaves J during the iteration,updateˆp j.Delete all entries in the j th column list from all row lists.For each row list i whosefirst(largest)entry is deleted,update the correspondingˆu i by settingˆu i to be the next(nowfirst and maximum)entry remaining in the row list;also update(p׈u)i. The total time for this during the course of the algorithm is O(n),because each M ij is deleted at most once.This completes the implementation.By inspection,the total time is O(n log n)(for preprocessing,and deletion of covering constraints)plus O( t1+|I t|+|J t|)(for the work done as a result of the increments).Thefirst term O(n log n)above is in itsfinal form.The next three lemmas bound the second term(the sum).Thefirst lemma bounds the sum except for the“1”.That is,it bounds the number of times any y i orˆy j is incremented.(There are r+c elements,and each can be incremented at most N times during the course of the algorithm.)Lemma5|I t|+|J t|≤(r+c)N=O((r+c)log(n)/ε2).tProof First, t|I t|≤rN because each y i can be increased at most N times before max i y i≥N(causing termination).Second, t|J t|≤cN because eachˆy j can be increased at most N times before j leaves J and ceases to be updated.⊓⊔The next lemma bounds the remaining part of the second term,which is O( t1).Given that t|I t|+ |J t|≤(r+c)N,it’s enough to bound the number of iterations t where|I t|+|J t|=0.Call such an iteration empty.(The1’s in the non-empty iterations contribute at most t|I t|+|J t|≤(r+c)N to the sum.) Wefirst show that each iteration is non-empty with probability at least1/4.This is so because,for any(i′,j′)pair chosen in an iteration,for the constraint that determines the incrementδi′j′,the expected increase in the corresponding y i orˆy j must be at least1/4,and that element will be incremented(making the iteration non-empty)with probability at least1/4.Lemma6Given the state at the start of an iteration,the probability that it is empty is at most3/4.Proof Given the(i′,j′)chosen in the iteration,by(1)of Lemma2,by definition ofδi′j′,there is either an i such that M ij′δi′j′≥1/4or a j such that M i′jδi′j′≥1/4.In the former case,i∈I t with probability at least1/4.In the latter case,j∈J t with probability at least1/4.⊓⊔This implies that,with high probability,the number of empty iterations does not exceed three times the number of non-empty iterations by much.(This follows from the Azuma-like inequality.)We have already bounded the number of non-empty iterations,so this implies a bound(with high probability)on the number of empty iterations.Lemma7With probability at least1−1/rc,the number of empty iterations is O((r+c)N).Proof Let E t be1for empty iterations and0otherwise.By the previous lemma and the Azuma-like in-equality tailored for random stopping times(Lemma10),for anyδ,A≥0,Pr (1−δ) T t=1E t≥3 T t=1(1−E t)+A ≤exp(−δA).Takingδ=1/2and A=2ln(rc),it follows that with probability at least1−1/rc,the number of empty iterations is bounded by a constant times the number of non-empty iterations plus2ln(rc).The number of non-empty iterations is at most(r+c)N,hence,with probability at least1−1/rc the number of empty iterations is O((r+c)N).⊓⊔Finally we complete the proof of Theorem2,stated at the top of the section.As discussed above,the total time is O(n log n)(for preprocessing,and deletion of covering constraints) plus O( t1+|I t|+|J t|)(for the work done as a result of the increments).By Lemma5, t|I t|+|J t|=O((r+c)log(n)/ε2).By Lemma7,with probability1−1/rc,the number of iterations t such that|I t|+|J t|=0is O((r+c)log(n)/ε2).Together,these imply that,with probability 1−1/rc,and the total time is O(n log n+(r+c)log(n)/ε2).This and Theorem1imply Theorem2.⊓⊔4.2Faster implementation.To prove the main result,it remains to describe how to remove the log n factor from the n log n term in the time bound in the previous section.The idea is that it suffices to approximately sort the row and column lists,and that this can be done in linear time.Theorem3The algorithm can be implemented to return a(1−7ε)-approximate primal-dual pair for packing and covering in time O(n+(c+r)log(n)/ε2)with probability at least1−5/rc.。