Target speaker separation
1选读shakespeare

William ShakespeareThe first period dates from 1590 ----1600.In this period he wrote most of his historical plays and comedies, and a few early tragedies, and these plays are imbued with an optimistic atmosphere of humanism. Among the best known of this period are Romeo and Juliet(1594) and the Merchant of Venice(1596).The second period, from 1601 ---- 1608, includes chiefly his great tragedies: Hamlet(1601), Othello(1604), King Lear(1605), Macbeth(1605) and Timon of Athens(1609). The outstanding tragi-comedy Measure for Measure(1604) also belong to this period. In the above-mentioned plays are reflected the social contradictions of the age.The third period dates from 1609----1612.In this period Shakespeare chiefly wrote three tragi-comedies, of which The Tempest(1612) is the most significant. In these last plays we see Shakespeare’s optimistic faith in the future of humanity, at the same time we also see the dramatist’s Utopianism.Shakespeare’s masterpiece s are as the following:Four tragedies:Hamlet, Prince of Denmark; Othello); King Lear; Macbeth)Four comedies:Twelfth night; A midsummer night's dream; The merchant of Venice; Much ado about nothing(人教版教材称As you like it)Historical plays:King Henry Ⅳ;The life of King Henry Ⅴ;The life and death of King Richard ⅡThe Sonnets;Two long poems: The Rape of Lucrece鲁克丽丝失贞记Venus and Adonis 维纳斯和阿多尼斯Ben Johnson’s comment on Shakespeare is ―He was not of an age, but for all time, 说他―不属于一个时代而属于千秋万代‖。
外文翻译---说话人识别
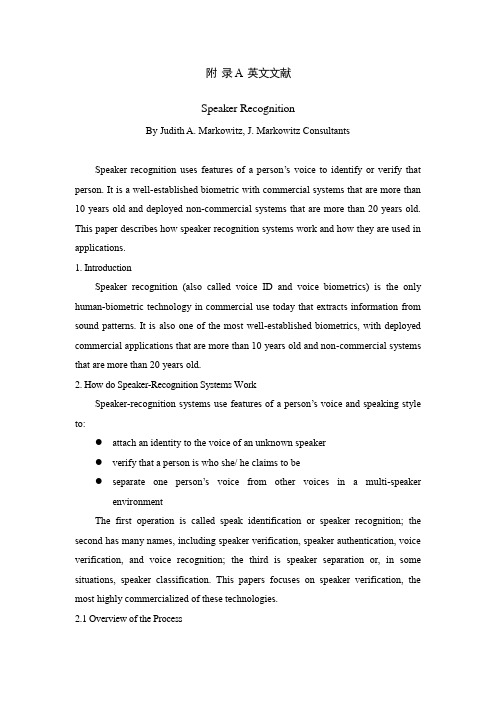
附录A 英文文献Speaker RecognitionBy Judith A. Markowitz, J. Markowitz ConsultantsSpeaker recognition uses features of a person‟s voice to identify or verify that person. It is a well-established biometric with commercial systems that are more than 10 years old and deployed non-commercial systems that are more than 20 years old. This paper describes how speaker recognition systems work and how they are used in applications.1. IntroductionSpeaker recognition (also called voice ID and voice biometrics) is the only human-biometric technology in commercial use today that extracts information from sound patterns. It is also one of the most well-established biometrics, with deployed commercial applications that are more than 10 years old and non-commercial systems that are more than 20 years old.2. How do Speaker-Recognition Systems WorkSpeaker-recognition systems use features of a person‟s voice and speaking style to:●attach an identity to the voice of an unknown speaker●verify that a person is who she/ he claims to be●separate one person‟s voice from other voices in a multi-speakerenvironmentThe first operation is called speak identification or speaker recognition; the second has many names, including speaker verification, speaker authentication, voice verification, and voice recognition; the third is speaker separation or, in some situations, speaker classification. This papers focuses on speaker verification, the most highly commercialized of these technologies.2.1 Overview of the ProcessSpeaker verification is a biometric technology used for determining whether the person is who she or he claims to be. It should not be confused with speech recognition, a non-biometric technology used for identifying what a person is saying. Speech recognition products are not designed to determine who is speaking.Speaker verification begins with a claim of identity (see Figure A1). Usually, the claim entails manual entry of a personal identification number (PIN), but a growing number of products allow spoken entry of the PIN and use speech recognition to identify the numeric code. Some applications replace manual or spoken PIN entry with bank cards, smartcards, or the number of the telephone being used. PINS are also eliminated when a speaker-verification system contacts the user, an approach typical of systems used to monitor home-incarcerated criminals.Figure A1.Once the identity claim has been made, the system retrieves the stored voice sample (called a voiceprint) for the claimed identity and requests spoken input from the person making the claim. Usually, the requested input is a password. The newly input speech is compared with the stored voiceprint and the results of that comparison are measured against an acceptance/rejection threshold. Finally, the system accepts the speaker as the authorized user, rejects the speaker as an impostor, or takes another action determined by the application. Some systems report a confidence level or other score indicating how confident it about its decision.If the verification is successful the system may update the acoustic information in the stored voiceprint. This process is called adaptation. Adaptation is an unobtrusive solution for keeping voiceprints current and is used by many commercial speaker verification systems.2.2 The Speech SampleAs with all biometrics, before verification (or identification) can be performed the person must provide a sample of speech (called enrolment). The sample is used to create the stored voiceprint.Systems differ in the type and amount of speech needed for enrolment and verification. The basic divisions among these systems are●text dependent●text independent●text prompted2.2.1 Text DependentMost commercial systems are text dependent.Text-dependent systems expect the speaker to say a pre-determined phrase, password, or ID. By controlling the words that are spoken the system can look for a close match with the stored voiceprint. Typically, each person selects a private password, although some administrators prefer to assign passwords. Passwords offer extra security, requiring an impostor to know the correct PIN and password and to have a matching voice. Some systems further enhance security by not storing a human-readable representation of the password.A global phrase may also be used. In its 1996 pilot of speaker verification Chase Manhattan Bank used …Verification by Chemical Bank‟. Global phrases avoid the problem of forgotten passwords, but lack the added protection offered by private passwords.2.2.2 Text IndependentText-independent systems ask the person to talk. What the person says is different every time. It is extremely difficult to accurately compare utterances that are totally different from each other - particularly in noisy environments or over poor telephone connections. Consequently, commercial deployment of text-independentverification has been limited.2.2.3 Text PromptedText-prompted systems (also called challenge response) ask speakers to repeat one or more randomly selected numbers or words (e.g. “43516”, “27,46”, or “Friday, c omputer”). Text prompting adds time to enrolment and verification, but it enhances security against tape recordings. Since the items to be repeated cannot be predicted, it is extremely difficult to play a recording. Furthermore, there is no problem of forgetting a password, even though the PIN, if used, may still be forgotten.2.3 Anti-speaker ModellingMost systems compare the new speech sample with the stored voiceprint for the claimed identity. Other systems also compare the newly input speech with the voices of other people. Such techniques are called anti-speaker modelling. The underlying philosophy of anti-speaker modelling is that under any conditions a voice sample from a particular speaker will be more like other samples from that person than voice samples from other speakers. If, for example, the speaker is using a bad telephone connection and the match with the speaker‟s voiceprint is poor, it is likely that the scores for the cohorts (or world model) will be even worse.The most common anti-speaker techniques are●discriminate training●cohort modeling●world modelsDiscriminate training builds the comparisons into the voiceprint of the new speaker using the voices of the other speakers in the system. Cohort modelling selects a small set of speakers whose voices are similar to that of the person being enrolled. Cohorts are, for example, always the same sex as the speaker. When the speaker attempts verification, the incoming speech is compared with his/her stored voiceprint and with the voiceprints of each of the cohort speakers. World models (also called background models or composite models) contain a cross-section of voices. The same world model is used for all speakers.2.4 Physical and Behavioural BiometricsSpeaker recognition is often characterized as a behavioural biometric. This description is set in contrast with physical biometrics, such as fingerprinting and iris scanning. Unfortunately, its classification as a behavioural biometric promotes the misunderstanding that speaker recognition is entirely (or almost entirely) behavioural. If that were the case, good mimics would have no difficulty defeating speaker-recognition systems. Early studies determined this was not the case and identified mimic-resistant factors. Those factors reflect the size and shape of a speaker‟s speaking mechanism (called the vocal tract).The physical/behavioural classification also implies that performance of physical biometrics is not heavily influenced by behaviour. This misconception has led to the design of biometric systems that are unnecessarily vulnerable to careless and resistant users. This is unfortunate because it has delayed good human-factors design for those biometrics.3. How is Speaker Verification Used?Speaker verification is well-established as a means of providing biometric-based security for:●telephone networks●site access●data and data networksand monitoring of:●criminal offenders in community release programmes●outbound calls by incarcerated felons●time and attendance3.1 Telephone NetworksToll fraud (theft of long-distance telephone services) is a growing problem that costs telecommunications services providers, government, and private industry US$3-5 billion annually in the United States alone. The major types of toll fraud include the following:●Hacking CPE●Calling card fraud●Call forwarding●Prisoner toll fraud●Hacking 800 numbers●Call sell operations●900 number fraud●Switch/network hits●Social engineering●Subscriber fraud●Cloning wireless telephonesAmong the most damaging are theft of services from customer premises equipment (CPE), such as PBXs, and cloning of wireless telephones. Cloning involves stealing the ID of a telephone and programming other phones with it. Subscriber fraud, a growing problem in Europe, involves enrolling for services, usually under an alias, with no intention of paying for them.Speaker verification has two features that make it ideal for telephone and telephone network security: it uses voice input and it is not bound to proprietary hardware. Unlike most other biometrics that need specialized input devices, speaker verification operates with standard wireline and/or wireless telephones over existing telephone networks. Reliance on input devices created by other manufacturers for a purpose other than speaker verification also means that speaker verification cannot expect the consistency and quality offered by a proprietary input device. Speaker verification must overcome differences in input quality and the way in which speech frequencies are processed. This variability is produced by differences in network type (e.g. wireline v wireless), unpredictable noise levels on the line and in the background, transmission inconsistency, and differences in the microphone in telephone handset. Sensitivity to such variability is reduced through techniques such as speech enhancement and noise modelling, but products still need to be tested under expected conditions of use.Applications of speaker verification on wireline networks include secure calling cards, interactive voice response (IVR) systems, and integration with security forproprietary network systems. Such applications have been deployed by organizations as diverse as the University of Maryland, the Department of Foreign Affairs and International Trade Canada, and AMOCO. Wireless applications focus on preventing cloning but are being extended to subscriber fraud. The European Union is also actively applying speaker verification to telephony in various projects, including Caller Verification in Banking and Telecommunications, COST250, and Picasso.3.2 Site accessThe first deployment of speaker verification more than 20 years ago was for site access control. Since then, speaker verification has been used to control access to office buildings, factories, laboratories, bank vaults, homes, pharmacy departments in hospitals, and even access to the US and Canada. Since April 1997, the US Department of Immigration and Naturalization (INS) and other US and Canadian agencies have been using speaker verification to control after-hours border crossings at the Scobey, Montana port-of-entry. The INS is now testing a combination of speaker verification and face recognition in the commuter lane of other ports-of-entry.3.3 Data and Data NetworksGrowing threats of unauthorized penetration of computing networks, concerns about security of the Internet, and increases in off-site employees with data access needs have produced an upsurge in the application of speaker verification to data and network security.The financial services industry has been a leader in using speaker verification to protect proprietary data networks, electronic funds transfer between banks, access to customer accounts for telephone banking, and employee access to sensitive financial information. The Illinois Department of Revenue, for example, uses speaker verification to allow secure access to tax data by its off-site auditors.3.4 CorrectionsIn 1993, there were 4.8 million adults under correctional supervision in the United States and that number continues to increase. Community release programmes, such as parole and home detention, are the fastest growing segments of this industry. It is no longer possible for corrections officers to provide adequate monitoring ofthose people.In the US, corrections agencies have turned to electronic monitoring systems. Since the late 1980s speaker verification has been one of those electronic monitoring tools. Today, several products are used by corrections agencies, including an alcohol breathalyzer with speaker verification for people convicted of driving while intoxicated and a system that calls offenders on home detention at random times during the day.Speaker verification also controls telephone calls made by incarcerated felons. Inmates place a lot of calls. In 1994, US telecommunications services providers made $1.5 billion on outbound calls from inmates. Most inmates have restrictions on whom they can call. Speaker verification ensures that an inmate is not using another inmate‟s PIN to make a forbidden contact.3.5 Time and AttendanceTime and attendance applications are a small but growing segment of the speaker-verification market. SOC Credit Union in Michigan has used speaker verification for time and attendance monitoring of part-time employees for several years. Like many others, SOC Credit Union first deployed speaker verification for security and later extended it to time and attendance monitoring for part-time employees.4. StandardsThis paper concludes with a short discussion of application programming interface (API) standards. An API contains the function calls that enable programmers to use speaker-verification to create a product or application. Until April 1997, when the Speaker Verification API (SV API) standard was introduced, all available APIs for biometric products were proprietary. SV API remains the only API standard covering a specific biometric. It is now being incorporated into proposed generic biometric API standards. SV API was developed by a cross-section of speaker-recognition vendors, consultants, and end-user organizations to address a spectrum of needs and to support a broad range of product features. Because it supports both high level functions (e.g. calls to enrol) and low level functions (e.g. choices of audio input features) itfacilitates development of different types of applications by both novice and experienced developers.Why is it important to support API standards? Developers using a product with a proprietary API face difficult choices if the vendor of that product goes out of business, fails to support its product, or does not keep pace with technological advances. One of those choices is to rebuild the application from scratch using a different product. Given the same events, developers using a SV API-compliant product can select another compliant vendor and need perform far fewer modifications. Consequently, SV API makes development with speaker verification less risky and less costly. The advent of generic biometric API standards further facilitates integration of speaker verification with other biometrics. All of this helps speaker-verification vendors because it fosters growth in the marketplace. In the final analysis active support of API standards by developers and vendors benefits everyone.附录B 中文翻译说话人识别作者:Judith A. Markowitz, J. Markowitz Consultants 说话人识别是用一个人的语音特征来辨认或确认这个人。
专八语言学试题【答案版本】

1. F. de. Saussure is a (n) __________linguist.A. AmericanB. BritishC. SwissD. RussianSwiss linguist. The founder of structural linguistics, he declared that there is only an arbitrary relationship between a linguistic sign and that which it signifies. The posthumously published collection of his lectures,Course in General Linguistics (1916), is a seminal work of modern linguistics.索绪尔,费迪南德·德:(1857-1913) 瑞士语言学家,结构主义语言的创始人,他声称在语言符号和其所指含义之间仅有一种模糊的关系。
他死后,他的讲演集出版为《普通语言学教程》(1916年),是现代语言学的开山之作2.N. Chomsky is a(n) ______linguist.Canadian B. American C. French D. SwissAmerican linguist who revolutionized the study of language with his theory of generative grammar, set forth inSyntactic Structures (1957).乔姆斯基,诺阿姆:(生于1928) 美国语言学家,他在《句法结构》(1957年)一书中所阐述的关于生成语法的理论曾使语言学研究发生突破性进展3.___________is the study of speech sounds in language or a language with reference totheir distribution and patterning and to tacit rules governing pronunciation.A.PhonologyB. Lexicography 词典编纂C. lexicology词典学D.Morphology词态词态学音位学研究的是一种语言的整个语音系统及其分布,包括某一特定语言里的语音和音位分部和结合的规律。
一种基于卷积神经网络的端到端语音分离方法

Abstract:Mostofspeechseparationsystemsusuallyenhancethemagnitudespectrumofthemixture.Thephasespectrum isleftunchanged,whichisinherentintheshorttimeFouriertransform (STFT)coefficientsoftheinputsignal.However, recentstudieshavesuggestedthatphasewasimportantforperceptualquality.Inordertosimultaneouslymakefulluseof magnitudeandphase,thisworkdevelopsanovelendtoendmethodfortwotalkerspeechseparation,basedonanencoder decoderfullyconvolutionalstructure.Differentfrom traditionalspeechseparationsystems,inthispaper,deepneuralnet workoutputsonespeaker’ssignalsexclusively.WeevaluatetheproposedmodelontheTIMITdataset.Theexperimental resultsshowthattheproposedmethodsignificantlyoutperformsthepermutationinvarianttraining(PIT)baselinemethod, witharelativeimprovementof1606 insignaltodistortionratio(SDR). Keywords:speakerindependentspeechseparation;cocktailpartyproblem;endtoend;convolutionencoderdecoder
跨文化交际要点整理

1. Culture: All the material and spiritual things created by man.2. Communication: Transmission of information/feelings between a sender and a receiver.3. Intercultural/Cross-cultural communication: Communication between people of any two different cultures between countries or within a country.4. Intercultural communication: Usually communication between members of the same culture.5. Intercultural (communicative) competence: Knowledge, attitudes and ability / skills required for intercultural communication.6. Racial, ethnic, international communication: Communication between races, ethnic groups or nations.7. Contact, encounter, interaction, exchange, dialog, negotiation, etc.Culture The total sum of material and spiritual wealth created by the mankind in the process of the social and historical development, especially literature, art, science, technology, architecture, education, traditions and customs, etc.Characteristics of culture:(1) Culture is learned:(2) Culture is dynamic and adaptive:(3) Culture is pervasive (传遍):(4) Culture is interrelated: Spring Festival(5) Culture is largely invisible:(6) Culture is ethnocentric:种族优越感Cultural identity:One’s sense of belonging to a particular culture or ethnic group. intercultural identity:One’s sense of belonging to more than one particular culture or ethnic group in an intercultural situation.Types of intercultural identity /acculturation(1) Assimilation 融入型: Breaking away from one’s ethnic culture and trying to identify with the dominant culture;(2) Integration 融合型: Maintaining contact with the dominant culture while retaining the core values of one’s ethnic culture;(3) Separation 分离型: Adhering to one’s ethnic culture and shunning the dominant culture in the foreign environment;(4) Marginalization 边缘型: Alienated from and unidentified with either one’s ethnic culture or the dominant culture.Communication: Transmission of information between a sender and a receiver. Ingredients/process of communication:Source:The source is the person with an idea he or she desires to communicate. Encoding:Encoding is the process of putting an idea into a symbol.Message:The term message identifies the encoded thought. Encoding is the process, the verb; the message is the resulting object.Channel:The term channel is used technically to refer to the means by which the encoded message is transmitted. The channel or medium, then, may be print,electronic, or the light and sound waves of the face-to-face communication.Noise:The term noise technically refers to anything that distorts the message the source encodes.Receiver:The receiver is the person who attends to the message.Decoding:Decoding is the opposite process of encoding and just as much an active process.Receiver response:It refers to anything the receiver does after having attended to and decoded the message.Feedback:Feedback refers to that portion of the receiver response of which the source has knowledge and to which the source attends and assigns meaning.Context:The final component of communication is context. Generally, context can be defined as the environment in which the communication takes place and which helps define the communication.Characteristics of communication:(1) Communication is dynamic: p44(2) Communication is symbolic:(3) Communication is irreversible(4) Communication is transactional:(5) Communication is self-reflective: Thinking about oneself.(6) Communication is contextual and systematic:The necessity and importance of intercultural communication1) It is a historical trend of the modern world: globalization and glocalization;2) It improves ties and enhances understanding between different peoples;3) It reduces the possibilities of conflicts and the dangers of war between nations4) It increases international trade and stimulates domestic economy5) It promotes exchanges in various fields including culture, education, technology, et6) It helps environmental protection on a global scale.Types and features of intercultural communication:1) Intercultural communication: Between different cultures;2) Interethnic communication: Between different ethnic groups;3) Interracial communication: Between different races;4) International communication: Between different nations;5) Inter-class, inter-professional, inter-age, etc. Communication.Major causes of intercultural misunderstanding1) Culture shock 文化震撼/文化休克: 2) Ethnocentrism 民族中心主义: 3) Projected similarity误以为相似: 4) Stereotypes刻板印象/固见: 5) Misevaluation错误评判;6) Prejudice偏见;7) Discrimination歧视Relation between language and culture:1) Language is part of culture;2) Culture can be affected by language;3) Language reflects culture:Relation between language and (intercultural) communication:1) Language is the major tool used in (intercultural) communication;2) Language may pose problems for translation due to its cultural loading;3) Language may cause misunderstanding because of differences in interpreting speech acts (the real intension / meaning of the speaker):Conversational principles1) The Cooperative Principle (CP) (H.P. Grice, 1975, 1989):The maxim of quantity:The maxim of quality:The maxim of relationThe maxim of manner:2) The Politeness Principle (PP) (G. N. Leech, 1983):Tact maxim (得体):Generosity maxim (慷慨):Approbation maxim (赞誉):Modesty maxim (谦逊):Agreement maxim (一致):Sympathy maxim (同情):Speech acts:The way in which speech or words are used to cause acts or actions Assertive (阐述):Directives (指令):Commissives (承诺):Expressives (表情):Declarations (宣告):Nonverbal communication:All types of communication that take place without words.Importance of nonverbal communication:1) It often occurs unconsciously;2) It can define relationship between people;3) It tends to be a more reliable demonstration of the interlocutor’s true meaning (because it is less consciously controlled);4) It can be used to help verbal communication;5) It may easily be misunderstood and cause problems or trouble, especially in cross-cultural communication.Function of non-verbal communication:1) Emphasizing:2) Repeating:3) Substituting:4) Regulating:5) Contradicting:Categories of nonverbal communication:1) Body movement / posture2) Gestures, facial expressions and eye contact3) Touching4) Paralanguage: 副语言High context culture (强语境文化) :A culture in which much is understood in communication by referring to the (extra-linguistic) context so that people tend tospeak out the least.Low context culture (弱语境文化) : A culture in which less is understood in communication by referring to the (extra-linguistic) context so that people tend to speak out the most.China: High-context culture:English-speaking countries: Low-context culture:。
Pyle Audio PSS4 PSS6 高功率立体声扬声器选择器用户手册说明书
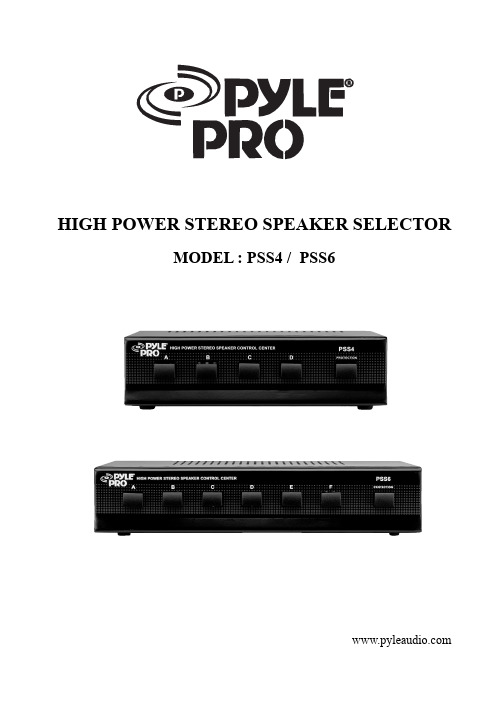
1
PREPARATIONS • Use the PYLE PRO Speaker Selectors only with amplifiers rated at 100 watts per
channel or less. • Your PYLE PRO Speaker Selector is designed to accept any size cable up to 14 gauge
中考高频词汇关联记忆

Ill-illness
Imagine-imagination-imaginable Important-importance-unimportant Injure-injury-injured Instruct-instruction-instructive Introduce-introduction Invent-invention-inventor Invite-invitation Late-later-latest-lately Live-alive-living-lively Luck-lucky-unlucky-luckily-unluckily
Fail-failure Feel-feeling Final-finally Follow-following Fool-foolish Foreign-foreigner Free-freedom-freely Friend-friendly-friendship Fun-funny
General-generally Gentle-gentlemangently Germany-German Grow-growth Happy-hapiness-happily Harm-harmful-harmless Humor-humorous Hungerf Beautiful Believable-Unbelievable Bored Boring Broken
Care-careful-carefully Choose-choice Clear-clearly Close Colour-colourful Communicate-communication Compare-comparatively Compete-competition Complete-completely Create-creation Culture-Cultural Collect-collection-collecter
音响专业术语

音响专业术语音响专业术语平衡(balance):指在音频频谱的高段和低段之间在相对响度上所存在的客观关系;也指双声道立体声左声道和右声道之间的信号的相同(平衡)。
平衡连接(balanced connection):指音响器材间的一种连接方式,在单根电缆中有3根导线,一根用来传送音频信号,另一根用于传送极性相反的音频信号,而另一根则为地线。
香蕉插座(banana jack)指装于音箱和功率放大器上用于和音箱线的香蕉插头连接的一种小型圆状插座。
香蕉插头(banana plug):普遍装于音箱线两端的供插入香蕉插座的一种插头。
带宽(band width):指音响装置能够处理或通过的一段频率范围。
比方说,杜比环绕声的环绕声道的带宽便是100Hz-7kHz。
环绕声道只通过频率在100Hz (低音)和7kHz(高音的低段)之间的频率。
人耳能听到的频率范围为20Hz-20kHz。
在谈到电气或声学器材的带宽时,往往指-3dB之间的频率范围。
低音(bass):指在音频低段的声音,通常低于500Hz(另一说则指低于160Hz)。
低频延伸(bass extension):指音响器材所能重放的最低频率。
系用于测定在重放低音时音响系统或音箱所能下潜到什么程度的尺度。
比方说,小型超低音音箱的低频延伸可以到40Hz,而大型超低音音箱则下潜到16Hz。
低音管理(bass management):指A/V功放接收机或A/V前置放大器中的综合控制电路,系用于确定应该给相应的音箱送去多少低频信号。
倒相式音箱(bass reflex):也称倒相式开孔箱,系在音箱面板上开有倒相孔(槽)的一类音箱。
由于开有孔,箱内的声音便可以辐射到外面来。
倒相式音箱比密闭式音箱的低频延伸要好些,但低音往往不那么结实紧凑。
比较“无限障板”(infinite baffle)双路功放推动(bi-amping):指用两台功率放大器去推动同一音箱的一种特殊连接方式,系用一台功率放大器去推动低音单元;另用一台功率放大器去推动中音和高音单元。
- 1、下载文档前请自行甄别文档内容的完整性,平台不提供额外的编辑、内容补充、找答案等附加服务。
- 2、"仅部分预览"的文档,不可在线预览部分如存在完整性等问题,可反馈申请退款(可完整预览的文档不适用该条件!)。
- 3、如文档侵犯您的权益,请联系客服反馈,我们会尽快为您处理(人工客服工作时间:9:00-18:30)。
TARGET SPEAKER SEPARATION IN A MULTISOURCE ENVIRONMENT USING SPEAKER-DEPENDENT POSTFILTER AND NOISE ESTIMATIONPejman Mowlaee†and Rahim Saeidi‡†Signal Processing and Speech Communication Laboratory,Graz University of Technology,Graz,Austria ‡Centre for Language and Speech Technology,Radboud University Nijmegen,The Netherlandspejman.mowlaee@tugraz.at rahim.saeidi@let.ru.nlABSTRACTIn this paper,we present a novel system for enhancing a target speech corrupted in a non-stationary real-life noise scenario.The proposed system consists of one spatial beamformer based on GCC-PHAT-estimated time-delay of arrival followed by three postfilters applied in a sequential way,namely:Wienerfilter,minimum mean square error estimator(MMSE)of the log-amplitude,and a model-driven postfilter(MDP)that relies on particular speech signal statistics cap-tured by target speaker Gaussian mixture model.The beamformer accounts for the directional interferences while the MMSE speech enhancement suppresses the stationary background noise,and MDP contributes to suppress the non-stationary sources from the binau-ral mixture.In our evaluation,multiple objective quality metrics are used to report the speech enhancement and separation perfor-mance,averaged on the CHiME development set.The proposed system performs better than standard state-of-the-art techniques and shows comparable performance with other systems submitted to the CHiME challenge.More precisely,it is successful in suppressing the non-stationary interfering sources at different SNR levels supported by the relatively high scores for signal-to-interference-ratio. Index Terms:Multisource noise,speech enhancement,speech qual-ity,non-stationary noise.1.INTRODUCTIONTarget speaker separation describes the problem of estimating an unknown clean speech signal recorded by one or several micro-phones in a noisy environment with possible presence of competing speaker(s).The problemfinds applications in many different areas of speech communications,including mobile telephony,robust au-tomatic speech recognition and hearing aids.The research in this area has been carried on for decades-with reporting some success-ful high quality speech enhancement systems.As a noise reduc-tion device is expected to work in noisy environment without a prior knowledge of the noise type,recent research effort has been directed toward studying the robustness of these algorithms in nonstationary noise,including low signal-to-noise ratios(SNRs)[1].As one step toward studying the problem of enhancing a tar-get speech signal in a multisource environment with nonstationary background noise,recently,the PASCAL challenge,termed as com-putational hearing in multisource environments(CHiME)was orga-nized[2].The challenge addresses several critical aspects on the The work of Pejman mowlaee was partially funded by the European project DIRHA(FP7-ICT-2011-7-288121),by ASD(Acoustic Sensing& Design)and Speech Processing Solutions GmbH Vienna.The work of Rahim Saeidi was funded by the European Community’s Seventh Framework Pro-gramme(FP72007-2013)under grant agreement no.238803.original problem of enhancing and recognizing of a target speech from its noisy version observed in a real-life listening environment mainly characterized by rather low SNR ratios whereas the noise sources are unpredictable,abrupt and highly non-stationary.Motivated by the recent advances for handling non-stationary noise in speech enhancement[3–8],in this paper we propose a com-binative approach to deal with multisource background noise(sta-tionary as well as non-stationary noise sources)in a binaural setup. The proposed system utilizes several postfilters for handling the sta-tionary part of interferences and novel GMM-based speaker models to estimate target speech and further to estimate the non-stationary part of the noise.The performance of the proposed algorithm is evaluated on the CHiME challenge corpus using several instrumen-tal metrics.The performance of the proposed combinative signal-dependent approach is compared to two well-known state-of-the-art signal-independent algorithms in[9,10]as well as the two top-performing systems[11,12]that participated in CHiME challenge. Throughout our study we report how much improvement is achiev-able by incorporating speaker-dependentfilters inside the speech en-hancement algorithm to successfully handle the nonstationary noise.2.PREVIOUS METHODSPrevious noise reduction techniques are classified as single and multi-channel.In a multichannel scenario,a beamformer algorithm leads to a promising cancellation of directional noise sources.Still, the usefulness of the beamforming techniques for enhancement pur-pose gets quite limited,especially when used individually under highly non-stationary or diffused noise scenarios[13].For single-channel speech enhancement methods,a minimum mean square er-ror(MMSE)estimator in the amplitude(MMSE-STSA)[10]and in the log-amplitude(MMSE-LSA)[9]domain are well-known for dealing well with the stationary additive noise scenario while other algorithms were suggested to handle non-stationary noise types [3,14].These techniques mainly rely on noise estimates typically provided by a noise estimation scheme(noise power spectral density (PSD)trackers[4,14])in a decision-directed manner,and further assume that the noise signal shows less changes in its second order statistics compared to that of the target speech signal.Such an as-sumption is not valid for real-life scenarios where the noise signal is highly time-varying and unpredictable or when the noise signal has a statistical characteristic close to the speech.Therefore,the achiev-able performance obtained by the methods in this group,gets limited when used in such adverse noise conditions[15].To take advantage of both groups,several methods on com-bining a beamforming stage with a speech enhancement stage as a post-processor have been suggested[5,16].The post-processor at-Fig.1.Block diagram for the proposed system.Algorithm1Steps taken in the proposed system in Fig.1.Spatial Filtering(Pre-processor)Align the two channels based on the time-delay estimateˆτ[19].Filter-and-sum beamformer.Wiener postfilterApply coherence-based Wiener postfilter[20].MMSE-LSA postfilterUsing MMSE-LSA in(5)with noise tracking algorithm of[4].Model-driven postfilterML speech estimation using target speaker GMM model in(8).Recover target signal by applying a mask on the noisy signal(11).tempts to reduce the stationary part of noise that was not canceled by the spatialfilter.Also,to reduce the amount of the spectral out-liers responsible for the musical noise produced at the output of the single-channel speech enhancement algorithms,some previous stud-ies developed the idea of applying a post-filter based on a pre-trained model on the clean target speech spectra as a constraint for speech enhancement purposes[6–8].Exploiting an adaptive postfilter was first suggested to enhance the perceptual quality of coded speech by emphasizing formants and pitch harmonics of speech[17].More recently,the authors in[6]showed that a clean speech codebook is effective in introducing intraframe constrains.A two passfiltering technique composed of a logSTSAfilter followed by a post-filter based on vector quantization(VQ)trained on the linear predictor co-efficients of the clean speech was presented in[7].They reported satisfactory improvement in perceptual quality of speech by remov-ing the musical noise of MMSE-LSA for pink and white noise[7]. Finally,we recently suggested the idea of incorporating a VQ code-book of the target speaker as a postfilter and fused it to a noise tracker in a single-channel scenario[18].The postfilter stage pro-vides the maximum likelihood(ML)speech estimate based on the target speaker model,while the noise tracker provides an estimation of the background noise.The preliminary results on multisource noisy data provided in[1]showed improvement over state-of-the-art signal-independent single-channel speech enhancement techniques which solely rely on noise statistics[9,10,14].3.PROPOSED SYSTEMThe block diagram of the proposed system is shown in Fig.1. First,the time delay between channels is estimated using the phase transform generalized cross correlation(GCC-PHAT)method[19]. Based on the time-aligned signals,a coherence-based Wiener beam-former[20]is applied.The enhanced single-channel output by the spatialfiltering stage is further sent to the MMSE-LSA algorithm[9] using the noise tracker in[4].Finally,we apply a model-driven postfilter(MDP)which provides the ML speech estimate based on trained speaker models in the form of Gaussian mixture models (GMMs),taking advantage of a good interference cancellation prop-erty by model-driven separation systems[21]and perceptual quality enhancement in speech coding[17].The steps taken are described in Alg.1.In the following,we present each step in detail.3.1.Spatialfiltering(pre-processor)Assume x l(n)and x r(n)with n=0,···,N−1denote the n th sample of the left and right time-domain clean speech signals at each frame where N is the signal length in samples.The received signal at each channel experiences the reverberation effect introduced by the acoustic transfer function from the source to each microphone de-noted by h l(n)and h r(n)with additive background noise denoted by d l(n)and d r(n),respectively.Then the binaural noisy observa-tion at the left/right channels is given byz c(n)=x c(n)∗h c(n)+d c(n),(1)where c=l and c=r gives the signal for the left and right channels, respectively.Taking the K-point discrete Fourier transform(DFT) with k∈0,...,K/2+1,we obtainZ c,k=X c,k H c,k+D c,k.(2)Assuming the left channel as the reference signal,the PHAT-weighted generalized cross-correlation(GCC-PHAT)algorithm in [19]is used to provide the time-delay estimate(TDE)of arrivalˆτbetween the channels.The output of the spatialfilter is given as the sum of the time aligned right and left signals˜Z r,k=Z r,k e jkˆτand˜Z l,k=Z l,k and we have:Y BF k=˜Z l,k+˜Z r,k2.Letφij,k with i=x l,j=x r be the cross-power spectral density between left and right microphones while for i=j={x l,x r},it denotes the auto-power spectral density of left and right microphones,respectively. The Wiener beamformer given by[20]:W postk=2φ˜zl,k˜z r,kφxl,k x l,k+φxr,k x r,k,(3)is known as a good approximation when there is no correlation be-tween the desired signal and noise as well as if the noise at each channel is uncorrelated.The power spectral densities are approxi-mated using a time recursive averaging,with smoothing parameterof0.9.The enhanced output is given by:Y BFok=W postkY BF k.3.2.Handling stationary noiseGiven the beamformer output signal,we apply a single-channel speech enhancement gain function in order to reduce the stationary background noise in the noisy signal.For this we apply the MMSE-LSA noise suppression rule[9]and the noise tracker in[4].The periodogram of the input signal is smoothed by afirst order recur-sive equation.Based on pilot experiments,we set the key param-eters in[4]as:η=0.7,γ=0.998andαd=0.95,whereηis the smoothing factor used to smooth the power spectrum of noisy speech,γis the parameter used to track the minimum of the pe-riodogram of the noisy speech via continuously averaging spectral values of the noisy speech at previous frames,andαd is the coef-ficient used in updating the speech-presence probability.The gain function,G k,is calculated based on estimations of a priori and a posteriori SNR values denoted byξk andγk[15],and is given by:G k=ξk1+ξkexp 12 ∞νk e−t t dt ,(4)withνk=ξkξk+1γk.Applying G k to the beamformer output,|Y BFok| we obtain|Y LSA k|=G k|Y BFo k|,(5) which together with the background noise estimate|ˆD st k|is passed to the next step called a model-driven postfilter(MDP).3.3.Handling non-stationary noiseSo far,both spatial and spectral speech estimations function indepen-dently from the spectral constraint of the target source,and as a con-sequence,the gain function G k leads to musical noise.To suppress the remaining musical noise,we propose to incorporate a postfilter by imposing the target speaker’s spectral constraints captured by the Gaussian mixture models learned from the channel-distorted clean speech training data.The proposed model-driven postfilter(MDP) is implemented in two steps:1)ML speech estimation,and2)signal reconstruction using a soft mask gain function.In the following,we explain the two steps in details.3.3.1.ML speech estimationBased on the estimated background noise,|ˆD st k|found by the noise tracker,we produce a binary maskˆG k,0as belowˆG k,0= 1,|ˆD st k|<|Y LSA k|0,Otherwise.(6)The mask acts like a target speaker activity detector and mostly re-jects the speech pauses and noise only regions in the observed noisy signal.This is needed to avoid modeling these regions using the GMM inference.For the regions recognized as noise-only,we ap-ply the spectral gainfloor of20log10G min=−25dB,as suggestedby[3].Letλbe the probability density function for modeling the spec-tral amplitudes of the target speaker signal.Here,we assume that λ∼{N(w m,µm,Σm)}M m=1is modeled by a GMM where the model parameters are Gaussian weights,means and covariance re-spectively and M is the model order.The mixture weights are posi-tive and further satisfy the constraint M m=1w m=1.Hence,giventhe model of target speaker and the input enhanced spectrum,|Y LSAk|, the goal is tofind the Gaussian of the model that provides the high-est likelihood defined in(7).Assuming diagonal covariance matri-ces for each Gaussian,from the maximization of the log-likelihood function,the selected mean vector is found as the solution to the following minimization criterion:µm∗=minm K/2+1k=0 (|Y LSA k|−µk,m)22σ2k,m−ln(w m√2πσk,m) ,(8)whereµm∗is the mean of the Gaussian in the speaker GMM that maximizes the a posteriori probability of the model given the input. We obtain the ML speech estimate as|ˆX ML k|=µk,m∗.3.3.2.Signal reconstruction using soft maskThe ML speech estimate|ˆX ML k|,as an estimate for reverberated clean speech,and|ˆD st k|,as our estimate for the stationary noise spectrum are used tofind the non-stationary part of noise,ˆd nst n,as belowˆd nst n =y BFon−ˆx ML n−ˆd st n.(9)Calculation ofˆd nst n in the time-domain is motivated by the fact that performing the calculation in the spectral-domain leads to negative spectrum amplitudes in some frequency bins,whereflooring these amplitudes introduces musical noise.To recover the speech signal of the target speaker,we produce the following soft mask gain functionˆG k = |ˆX ML k|√|ˆX MLk|2+max(|ˆD stk|2,|ˆD nstk|2),|ˆX ML k|>|ˆD st k|G min,Otherwise,(10)where we define|ˆD nst k|=(1−˜G2k)|Y BFo|and|ˆZ w k|=|ˆX ML k|2+|ˆD st k|2.with|ˆD nst k|as the estimation for the non-stationary noise with˜G k=|ˆZ w k||Y BFok|.Finally,using a K-point inverseDFT,the time domain enhanced speechˆx n is obtained asˆx n=DFT−1{ˆG k|Y BFo|e j∠Y BFo}.(11)4.EXPERIMENTAL SETUP4.1.System configuration and speech corpusA window length of32ms and a frame shift of8ms were used atthe sampling frequency of16kHz.GMMs were used to model forthe spectral amplitude of the target speaker.The speaker models aretrained using the binaural clean reverberated training data providedfor each speaker[2].In this way,the GMMs learn the average roomimpulse responses and the speaker characteristics.All500utterancesfrom the training set are utilized to train a512component GMM foreach speaker using10iterations of the EM algorithm[22].For performance evaluation,we conducted our experiments onthe PASCAL CHiME corpus produced by[2]via convolving theclean speech signals with the real room impulse response to simulatethe reverberant environment as well as adding a wide range of noisescoming from sources at different locations.The CHiME corpus con-sists of34,000utterances from18males and16females where thesentences follow a unique grammatical structure.The training set isused to train speaker models,while the development set is used toreport the system performance in terms of target speaker separationquality.Averaged on the whole development set,we report segmen-tal SNR(SSNR)to measure speech enhancement performance andBSS EV AL[23]metrics including signal-to-distortion ratio(SDR),signal-to-interference ratio(SIR)and signal-to-artifact ratio(SAR)to report the separation performance.In all our evaluations,the ob-jective metric is calculated at the left ear using the reverberant targetspeech as the reference signal.5.EXPERIMENTAL RESULTS5.1.Experiment1:spectrogram analysisFigure2illustrates an example to give indications about how the pro-posed system deals with background noise composed of stationaryand non-stationary parts.The results are shown for two utterancesselected from the SiSEC[24]development database corrupted at asignal-to-noise ratio of-3dB.The reverberated version of the cleansignals are used as reference signal to calculate the metrics.Theproposed system is capable recovering the most parts of the targetspeaker spectrogram via effectively rejecting the interference sig-nal.The SSNR improvement is shown in subplot5where for furtherhighlight the capability of the proposed system in recovering the tar-get speech signal;in the spectrograms,the regions where SSNR getsimproved are marked by black dashed boxes.5.2.Experiment2:improvements in speech qualityWe compare the performance of the model-driven speech enhance-ment system with the state-of-the-art speech enhancement methods:MMSE-STSA[10]and MMSE-LSA[9].For a fair comparison,thebeamformer output is used as the input signal to the speech enhance-ment methods studied here.The SDR and SIR results are shownin Table1,and averaged on600sentences of the development setp m (|YLSA|)=1(2π)K/2+12|Σm |12exp −(|Y LSA |−µm )T Σ−1m (|Y LSA|−µm )2 (7)Method-6-30369Noisy-6.6±0.1-4.2±0.0-1.8±0.10.7±0.1 3.3±0.05.5±0.1MMSE-LSA [9]-5.5±0.2-2.7±0.3-0.1±0.3 2.6±0.3 5.4±0.37.8±0.3MMSE-STSA [10]-5.4±0.2-2.6±0.3-0.1±0.3 2.6±0.3 5.4±0.37.8±0.3Proposed0.4±0.31.23±0.32.57±0.23.6±0.24.5±0.25.1±0.1Method-6-30369Noisy-6.6±0.1-4.2±0.1-1.8±0.10.7±0.1 3.3±0.1 5.5±0.1MMSE-LSA [9]-5.5±0.2-2.7±0.3-0.1±0.3 2.6±0.3 5.4±0.37.8±0.3MMSE-STSA [10]-5.4±0.2-2.6±0.3-0.1±0.3 2.7±0.3 5.4±0.37.8±0.3Proposed6.77±0.37.86±0.310.2±0.212.4±0.214.4±0.217.0±0.1Table paring SDR (left)and SIR (right)results for the proposed method versus two state-of-the-art speech enhancement algorithms02000400002000400002000400002000400000.511.522.5320Time (sec)Mixture Enhanced∆ SDR= +4.5 , ∆ SIR= +6.5∆ SDR= +3.0 , ∆ SIR= +4.7Showing spectrogram of clean,noisy input,enhanced speech,noise reference signals for input SNR of -3(dB).Absolute improvement com-pared to noisy signal in terms of SDR and SIR are shown,per clip.group to six input SNRs of -6to 9decibels.Considerable improve-ment versus the state-of-the-art speech enhancement techniques are attained in SDR for input SNR ≤3dB using the proposed method in this paper.In terms of SIR,we consistently outperform the standard approaches in [9,10]with a wide margin.We further compare the results of our method with those who participated in the CHiME challenge.We have received the full en-hanced development set files from two participants whose systems are called:1)data separation based on target signal cancellation and noise masking [12],and 2)non-negative matrix factorization bidirec-tional long short-term memory (NMF-BLSTM)[11].Figure 3shows the BSS EV AL results averaged over 600sentences in the develop-ment set,grouped at different input SNRs.In terms of SDR,it is evident that the proposed system is in line with other top-performing systems submitted to the CHiME challenge and marginally outper-forms the NMF-BLSTM in [11]in SNR =−6dB.However,the SIR results reveal that the proposed method achieves a consistent im-provement at all SNR levels compared to the NMF-BLST approach in [11]but only better than [12]for SNR ≥3dB.The system in [11]appears to be the best performing system in terms of SAR.In analysis of the scores of the instrumental quality metrics re-ported in Figure 3and Table 1,one should remind the differencebetween the noise characteristic at low and high SNR scenarios.Low SNRs down to -6dB are designed as background highly non-stationary energetic events while SNRs up to 9dB are fairly sta-tionary ambient noise.Therefore,the improvement in performance indicates that the model-driven postfilter stage is capable of handling non-stationary noises.From the experimental results,it was observed that the proposed system offers a high interference cancellation property,especially at low SNR levels.At high SNR levels,the results indicate that the proposed model-based system will not exceed the metrics evaluated on the unprocessed signal,because of the saturation behavior offered by the model-driven enhancement method.Fig.method versus systems participated in the CHiME challenge.AcknowledgementsThe authors would like to thank Dr.Felix Weninger,and Dr.Zbynek Koldovsky for their help in sharing wave files of their systems sub-mitted to the CHiME challenge as benchmarks in our experiments.6.CONCLUSIONWe presented a multi-stage target speech separation system for pro-cessing binaural recordings in environments that may be corrupted by stationary or non-stationary noise.The proposed system com-bined a spatial beamformer and a GMM-based model-driven post-filter to handle spatial interference and non-stationary noise,respec-tively.The performance of the proposed system was compared with the state-of-the art speech enhancement methods as well as two benchmark systems submitted to CHiME challenge.The presented system provides consistent improvement over benchmarks in terms of SSNR and pared to noisy observation,the proposed sys-tem,at -3dB input SNR on average achieves 4.5dB improvement in SDR and 9.8dB in SIR.7.REFERENCES[1]J.Barker,E.Vincent,N.Ma,H.Christensen,and P.Green,“The Pascal CHiME speech separation and recognition chal-lenge,”to appear in Computer Speech and Language,2012.[2]H.Christensen,J.Barker,N.Ma,and P.Green,“The CHiMEcorpus:a resource and a challenge for computational hearing in multisource environments,”in Proc.INTERSPEECH,2010.[3]I.Cohen and B.Berdugo,“Speech enhancement for non-stationary noise environments,”Signal Processing,vol.81,no.11,pp.2403–2418,2001.[4]S.Rangachari and P.C.Loizou,“A noise-estimation algorithmfor highly non-stationary environments,”Speech Communica-tion,vol.48,no.2,pp.220–231,2006.[5]I.Cohen,S.Gannot,and B.Berdugo,“An integrated real-timebeamforming and postfiltering system for nonstationary noise environments,”EURASIP Journal on Applied Signal Process-ing,,no.11,pp.1064–1073,2003.[6]T.V.Sreenivas and P.Kirnapure,“Codebook constrainedwienerfiltering for speech enhancement,”Acoustics,Speech and Signal Processing,IEEE Transactions on,vol.4,no.5, pp.383–389,Sept.1996.[7]J.Wung,S.Miyabe,and B.-H.Juang,“Speech enhancementusing minimum mean-square error estimation and a post-filter derived from vector quantization of clean speech,”in Proc.IEEE Int.Conf.Acoust.,Speech,Signal Processing,2009,pp.4657–4660.[8]J.Wung,S.Miyabe,and B.-H.Juang,“Speech enhancementbased on a log-spectral amplitude estimator and a postfilter de-rived from clean speech codebook,”in Proc.European Signal Processing Conf.,2010,pp.999–1003.[9]Y.Ephraim and D.Malah,“Speech enhancement using aminimum mean-square error log-spectral amplitude estimator,”Acoustics,Speech and Signal Processing,IEEE Transactions on,vol.33,no.2,pp.443–445,Apr1985.[10]Y.Ephraim and D.Malah,“Speech enhancement using aminimum-mean square error short-time spectral amplitude es-timator,”Acoustics,Speech and Signal Processing,IEEE Transactions on,vol.32,no.6,pp.1109–1121,1984. [11]F.Weninger,J.Geiger,M.Wöllmer,B.Schuller,and G.Rigoll,“The Munich2011CHiME Challenge Contribution:NMF-BLSTM speech enhancement and recognition for reverberated multisource environments,”in Proceedings Machine Listening in Multisource Environments,CHiME2011,satellite workshop of INTERSPEECH2011,2011,pp.24–29.[12]Z.Koldovsky,J.Malek,M.Balik,and J.Nouza,“CHiME dataseparation based on target signal cancellation and noise mask-ing,”in Proceedings Machine Listening in Multisource Envi-ronments,CHiME2011,satellite workshop of INTERSPEECH 2011,2011,pp.47–50.[13]O.L.Frost,“An algorithm for linearly constrained adaptivearray processing,”Proceedings of the IEEE,vol.60,no.8,pp.926–935,Aug.1972.[14]R. C.Hendriks,R.Heusdens,and J.Jensen,“MMSEbased noise PSD tracking with low complexity,”in Acous-tics,Speech,and Signal Processing,International Conference, 2010,pp.4266–4269.[15]P.Loizou,Speech Enhancement:Theory and Practice,CRCPress,Boca Raton,2007.[16]S.Gannot and I.Cohen,“Speech enhancement based on thegeneral transfer function GSC and postfiltering,”Speech and Audio Processing,IEEE Transactions on,vol.12,no.6,pp.561–571,nov.2004.[17]J.Chen and A.Gersho,“Adaptive postfiltering for quality en-hancement of coded speech,”Acoustics,Speech and Signal Processing,IEEE Transactions on,vol.3,no.1,pp.59–71, 1995.[18]P.Mowlaee,R.Saeidi,and R.Martin,“Model-driven speechenhancement for multisource reverberant environment:signal separation evaluation campaign(SiSEC2011),”in Proc.the 10th Int.Conf.on Latent Variable Analysis and Signal Separa-tion(LVA),2012,pp.454–461.[19]M.S.Brandstein and H.F.Silverman,“A robust method forspeech signal time-delay estimation in reverberant rooms,”in Acoustics,Speech,and Signal Processing,International Con-ference,Apr.1997,vol.1,pp.375–378.[20]C.Marro,Y.Mahieux,and K.U.Simmer,“Analysis of noisereduction and dereverberation techniques based on microphone arrays with postfiltering,”Acoustics,Speech and Signal Pro-cessing,IEEE Transactions on,vol.6,no.3,pp.240–259, May1998.[21]M.Cooke,J.R.Hershey,and S.J.Rennie,“Monaural speechseparation and recognition challenge,”Elsevier Computer Speech and Language,vol.24,no.1,pp.1–15,2010. [22]S.Young,The HTK Book(for HTK Version3.4),CambridgeUniversity Engineering Department,2006.[23]E.Vincent,R.Gribonval,and C.Fevotte,“Performance mea-surement in blind audio source separation,”Acoustics,Speech and Signal Processing,IEEE Transactions on,vol.14,no.4, pp.1462–1469,July2006.[24]S.Araki, F.Nesta, E.Vincent,Z.Kodovsky,G.Nolte,A.Ziehe,and A.Benichoux,“The2011signal separationevaluation campaign SiSEC2011:Audio source separation,”in Proc.the10th Int.Conf.on Latent Variable Analysis and Signal Separation,2012.。