Topology optimization 2008
拓扑优化、设计验证、3D打印-案例讲述“三个火枪手”的故事

拓扑优化(Topology Optimization)是一种根据给定的负载情况、约束条件和性能指标,在给定的区域内对材料分布进行优化的数学方法,是结构优化的一种。
设计验证- 基于高端CAE仿真软件进行产品性能的评估,CAE技术为产品设计提供了保障。
拓扑优化与用于设计验证的仿真软件的结合衍生出了新层次的设计自由度,设计师可以轻松地通过拓扑优化找到材料布局,再考虑更多的设计要求,包括应力、屈服强度等通过晶格进行更精细程度的材料分配,达到设计的最优化。
3D打印适合用来制造非常复杂的产品设计,尤其是那些通过传统制造难以或者无法加工出来的设计。
总体来说,拓扑优化技术寻求获得产品设计最佳材料分布的“物善其用”,设计验证基于产品性能出发为拓扑优化结果“保驾护航”。
“拓扑优化、用于设计验证的仿真、3D打印”三者的联袂,实现以“轻量化、结构一体化、高端复杂化”为导向的产品再设计,是面向增材制造的先进设计与制造的“三个火枪手”。
此外,在3D打印领域,关于工艺控制方面的仿真也尤为重要,随着3D打印产业化的深入,仿真贯穿了设计到制造的方方面面。
拓扑优化,设计验证、3D打印,这三者的结合释放了设计的自由度,拓扑优化与仿真的结合将最优的结构形状与最优的产品性能相结合起来设计,这样的设计通过3D打印技术“输出”出来。
三者相互配合,相互促进,相得益彰。
本期,增材专栏与大家一同感受数字制造界的产品再设计。
通过安世亚太分享的案例讲述“三个火枪手”如何进行材料拓扑最优布局、晶格点阵精细化设计、产品轻量化与结构一体化设计、刻面光顺化与重构设计、仿真设计验证等多种内容。
起点-拓扑优化技术安世中德作为安世亚太与德国CADFEM合资公司,将面向增材制造的先进设计与制造构架分为四个环节:(1) 先进设计(2) 工艺设计与优化(3) 增材制造设备(4) 质量检测其中“先进设计”作为“面向增材先进设计与制造”第一环节涉及三个步骤:(1) 起点-拓扑优化技术(2) 过程-设计与模型处理光顺化与重构(3) 验证-仿真计算与评估拓扑优化技术应用能建立在静力学、屈曲、高级非线性、模态、谐响应、随机振动等多种仿真计算基础上,多款仿真软件均有能力不等的拓扑优化分析模块,其中ANSYS Topology Optimization和ANSYS Genesis均有良好的拓扑优化能力表现。
拓扑优化分析
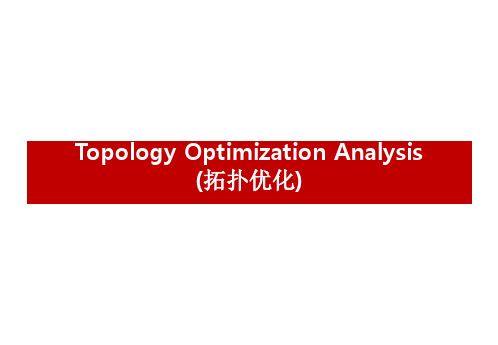
Topology Optimization Analysis(拓扑优化)拓扑优化分析- 单位 : N, mm- 几何模型: Bell crank.x_t边界条件及载荷条件- 刚体上施加多个集中荷载 - 边界条件 (高级选项)- 生成载荷组确认结果- 确认密度- 确认位移- 确认优化模型形状/ 并生成Bell Crank(基于线性静态分析进行的拓扑优化)概要目的承载情况下以位移不超过0.05mm、最小体积为设计目标函数生成的模型分析结果与原模型结果进行比较.- 确认模型的目标函数和约束条件.- 对于优化后模型进行分析并与优化前模型结果进行比较.分析概要模型 (设计对象) 设置边界条件/多种载荷 进行拓扑优化并生成优化模型什么叫拓扑优化?是指对于设计对象在定义约束条件和设计变量后满足目标函数生成优化模型的分析优化问题的构成- 目标函数是指体积和质量的增加或减少. - 约束条件是指位移或者应力等. - 设计变量是指尺寸、材料等特性 .① 定义目标函数② 定义约束条件③ 定义设计变量优化问题的构成设计对象目标函数 最小体积约束条件 位移限制-0.05mm设计变量 单元形状密度优化问题的构成Step54操作步骤231. 选择[ ] (新建)2. 选择模型设定环境 、 [3维/一般模型]3. 选择单位系: [N-mm-J-sec]4. 点击确认[按钮]5. 在窗口中右击鼠标,选择 [隐藏全部导航]分析 >> 设置分析条件 101Step1. 选择模型: bell crank.x_t2. 点击[打开]几何 >> CAD 文件 >> 导入 2102操作顺序• 注意模型格式!!• 确认单位!!1. 创建 >> 选择各向同性2. 选择材料库中Aluminum Alloys3. 选择Al 6061-T64. 点击[确认]5. 点击[关闭]编号 2名称Al 6061-T6 弹性模量 71000 (N/mm²) 泊淞比 0.3密度2.7e-006 (N/mm 3)4操作步骤31251. 创建 >> 选择3D2. 激活[实体]3. 输入特性4. 点击[确认]5. 点击[关闭]操作步骤342编号 1 名称 bell crank 材料2: AL 6061-T615Step1. 选择对象: 模型(1个)2. 3D 网格生成: 选择混合网格(以六面体为主)3. 选择[高级选项]4. 去掉[高阶单元]勾选项5. 点击[确认]05操作步骤3网格 >> 生成 >> 3D 21456Step1. 选择约束窗口中的 [高级]2. 输入约束条件4. 选择[适用]静态/热分析 >> 边界条件 >> 约束 06操作步骤1423名称 约束ty 目标类型 面选择目标 选择4个 (3号) 约束自由度 TY 边界组边界组-1222Step1. 输入约束条件3. 点击[确认]静态/热分析 >> 边界条件 >> 约束 07操作步骤12名称 Rz 自由 目标类型 面 选择目标 2个(2号) 约束自由度 Tx,Ty,Tz,Rx,Ry 边界组 边界组-1111131. 选择[其它]2. 选择[刚体]3. 勾选[刚体] 4 选择[依存节点中心] 5. 选择[依存节点] 6. 视角调整到“上视图” 7. 选择拾取和框选中的圈选8. 鼠标移动到圆孔中心左击鼠标选择圆内表面9. 网格组: 刚体 10. 选择[确认]操作步骤9214 5367810操作步骤1.鼠标移动到圆孔中心左击鼠标选择圆内表面2. 点击[确认]19101. 输入[集中力]3. 点击[适用]操作步骤21名称 3_1 目标类型 节点 选择目标 1个(2号) 荷载成分 X: 400N Y: -187N 荷载组荷载组_131. 输入[集中荷载]3. 点击[适用]操作步骤31名称 4_1 目标类型 节点 选择目标 1个(2号) 荷载成分 X: 311N Y: -1748N 荷载组荷载组_1211. 选择载荷/边界工作目录树的[3_1],[4_1]2. 右击鼠标选择[复制]3. 选择复制的 [3_1-1]4. 右击鼠标选择[编辑]5. 输入名称 [3_2]6. 载荷分量 X: -3438, Y: -9217. 选择[载荷组_2]8. 选择[确认]9. 选择上面第2步中复制的[4_1-1] 10. 右击鼠标选择[编辑] 11. 输入名称 [4_2]12. 荷载分量 X: -1259, Y: 1259 13. 选择荷载组_2 14. 选择[确认]操作步骤1256 7133489101112 141. 选择载荷/边界目录树中[3_1], [4_1]2. 右击鼠标选择[复制]3. 选择复制的[3_1-1]4. 右击鼠标选择[编辑]5. 输入名称[3_3]6. 荷载分量 Z: 4457. 选择[载荷组_3]8. 选择[确认]9. 选择第2步复制的[4_1-1] 10. 右击鼠标选择[编辑] 11. 输入名称[4_3] 12. 输入荷载分量 Z: 445 13. 选择荷载组_3 14. 选择[确认]操作步骤12567133489101112141. 选择载荷/边界目录树[3_1], [4_1]2. 右击鼠标选择[复制 ]3. 选择复制后的[3_1-1]4. 右击鼠标选择[编辑]5. 输入名称 [3_4]6. 输入荷载分量 Z: -4457. 选择[荷载组_4]8. 选择[确认]9. 选择第2步复制的[4_1-1] 10. 右击鼠标选择[编辑] 11. 输入名称[4_4] 12. 输入荷载分量 Z: -445 13. 选择荷载组_4 14. 选择[确认]操作步骤1256713348910111214Step1. 选择[节点]2. 名称: 刚体传感器3. 激活选择目标4. 选择前面创建的中间节点5. 类型: 位移 组成: 总平移6. 方法: 最大绝对值7. 点击[确认]分析 >> 优化设计 >> 传感器 15操作步骤1254367Step1. 分析控制: 选择[拓扑优化 ]2. 名称: bell crank min Vol3. 优化类型: 线性静态拓扑优化/体积最小4. 创建分析子工况: 选择线性静态分析 (生成4个子工况)5. 选择子工况控制6. 通过F2键改变名称 荷载1, 荷载2, 荷载3, 荷载47. 把左面定义的条件全部移到右边分析 >> 分析控制 >> 优化设置 >> 拓扑优化设置 16操作步骤12345 67操作步骤1.子工矿 [荷载组_1]中只选[载荷1]2. [荷载组合_2]中只选[载荷2]3. [荷载组合_3]中只选[载荷3]4. [荷载组合_2]中只选[载荷2]1. 选择子工况 [荷载 1]2. 选择[子工况控制]3. 勾选[位移]4. 传感器选择[刚体传感器 ]5. 条件选择[以下(<=)]输入[0.05]6. 点击[确认]7. [荷载 2,3,4] 子工矿都按照1~6步去操作操作步骤1345672操作步骤1.选择[确认]12操作步骤1.分析各子工况下[总位移]2. 确认位移结果12Step1. 选择[拓扑优化]2. 输入材料密度[0.10]3. 选择[计算]4. 自动计算值5. 输入网格大小[6]6. 自动设置模型路径分析结果 >> 优化设计后处理 >> 生成优化模型 20操作步骤1234567Step21窗格树>> 网格>> 拓扑优化生成网格操作步骤生成拓扑优化模型 .。
ABAQUS拓扑优化分析手册用户手册

ABAQUS拓扑优化分析手册/用户手册分析手册:13. Optimization Techniques优化技术13.1 结构优化:概述13.1.1 概述ABAQUS结构优化是一个帮助用户精细化设计的迭代模块。
结构优化设计能够使得结构组件轻量化,并满足刚度和耐久性要求。
ABAQUS提供了两种优化方法——拓扑优化和形状优化。
拓扑优化(Topology optimization)通过分析过程中不断修改最初模型中指定优化区域的单元材料性质,有效地从分析的模型中移走/增加单元而获得最优的设计目标。
形状优化(Shape optimization)则是在分析中对指定的优化区域不断移动表面节点从而达到减小局部应力集中的优化目标。
拓扑优化和形状优化均遵从一系列优化目标和约束。
最优化方法(Optimization)是一个通过自动化程序增加设计者在经验和直觉从而缩短研发过程的工具。
想要优化模型,必须知道如何去优化,仅仅说要减小应力或者增大特征值是不够,做优化必须有更专门的描述。
比方说,想要降低在两种不同载荷工况下的最大节点力,类似的还有,想要最大化前五阶特征值之和。
这种最优化的目标称之为目标函数(Object Function) 。
另外,在优化过程中可以同时强制限定某些状态参量。
例如,可以指定某节点的位移不超过一定的数值。
这些强制性的指定措施叫做约束(Constraint)。
ABAQUS/CAE可以创建模型然后定义、配置和执行结构优化。
更多信息请参考用户手册第十八章。
13.1.2 术语(Terminology)设计区域(Design area): 设计区域即模型需要优化的区域。
这个区域可以是整个模型,也可以是模型的一部分或者数部分。
一定的边界条件、载荷及人为约束下,拓扑优化通过增加/删除区域中单元的材料达到最优化设计,而形状优化通过移动区域内节点来达到优化的目的。
设计变量(Design variables):设计变量即优化设计中需要改变的参数。
增材制造术语

增材制造术语1. 增材制造(Additive Manufacturing):是一种通过逐层堆叠材料来制造三维实体产品的制造技术。
2. 三维打印(3D Printing):是增材制造的一种常见方法,利用计算机控制的逐层堆叠方式将材料逐渐构建成三维物体。
3. 材料堆积(Material Deposition):是增材制造过程中将材料层层堆积以构建产品的步骤。
4. 光固化(Photopolymerization):是一种增材制造技术,利用紫外线或其他光源对光敏材料进行硬化,将其逐层堆积以构建三维物体。
5. 粉末床融合(Powder Bed Fusion):是一种增材制造技术,通过将粉末材料逐层堆积并利用激光或电子束进行局部熔化,最终形成三维物体。
6. 可溶性支撑结构(Soluble Support Structures):是增材制造过程中使用的一种支撑结构,它可以在制造完成后通过特定的溶解剂溶解掉,以减少对产品表面的影响。
7. 层厚(Layer Thickness):是增材制造过程中每一层材料的厚度,决定了最终产品的精度和表面光滑度。
8. 制造方向(Build Orientation):是指在增材制造过程中,选择材料堆积的方向,以最大程度地减少支撑结构的使用和优化产品的物理性能。
9. 预处理(Preprocessing):是增材制造过程中的前期准备工作,包括模型切片、支撑结构的生成等。
10. 后处理(Postprocessing):是增材制造过程中的后期处理工作,包括去除支撑结构、表面光滑、热处理等。
11. 增材制造设备(Additive Manufacturing Equipment):是用于实施增材制造的设备,例如三维打印机、激光烧结机等。
12. 直接制造(Direct Manufacturing):是一种增材制造技术,可以直接从数字模型等电子数据中制造出产品,无需制造模具或工艺准备。
13. 拓扑优化(Topology Optimization):是一种设计方法,通过优化材料分布和结构形状,以最大程度地提高产品性能,同时减少材料使用。
拓扑优化密度法
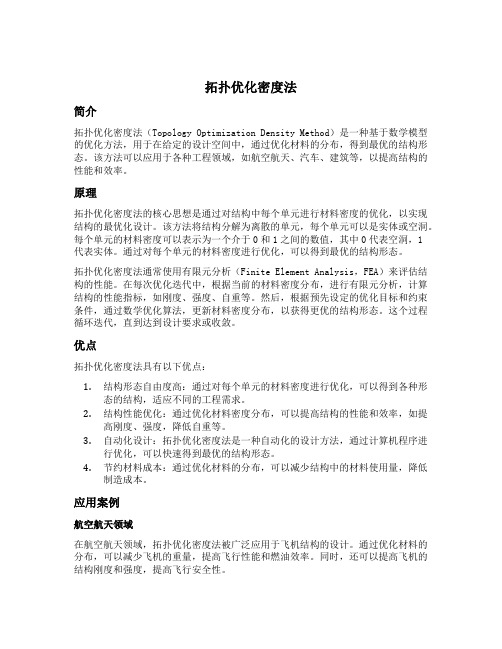
拓扑优化密度法简介拓扑优化密度法(Topology Optimization Density Method)是一种基于数学模型的优化方法,用于在给定的设计空间中,通过优化材料的分布,得到最优的结构形态。
该方法可以应用于各种工程领域,如航空航天、汽车、建筑等,以提高结构的性能和效率。
原理拓扑优化密度法的核心思想是通过对结构中每个单元进行材料密度的优化,以实现结构的最优化设计。
该方法将结构分解为离散的单元,每个单元可以是实体或空洞。
每个单元的材料密度可以表示为一个介于0和1之间的数值,其中0代表空洞,1代表实体。
通过对每个单元的材料密度进行优化,可以得到最优的结构形态。
拓扑优化密度法通常使用有限元分析(Finite Element Analysis,FEA)来评估结构的性能。
在每次优化迭代中,根据当前的材料密度分布,进行有限元分析,计算结构的性能指标,如刚度、强度、自重等。
然后,根据预先设定的优化目标和约束条件,通过数学优化算法,更新材料密度分布,以获得更优的结构形态。
这个过程循环迭代,直到达到设计要求或收敛。
优点拓扑优化密度法具有以下优点:1.结构形态自由度高:通过对每个单元的材料密度进行优化,可以得到各种形态的结构,适应不同的工程需求。
2.结构性能优化:通过优化材料密度分布,可以提高结构的性能和效率,如提高刚度、强度,降低自重等。
3.自动化设计:拓扑优化密度法是一种自动化的设计方法,通过计算机程序进行优化,可以快速得到最优的结构形态。
4.节约材料成本:通过优化材料的分布,可以减少结构中的材料使用量,降低制造成本。
应用案例航空航天领域在航空航天领域,拓扑优化密度法被广泛应用于飞机结构的设计。
通过优化材料的分布,可以减少飞机的重量,提高飞行性能和燃油效率。
同时,还可以提高飞机的结构刚度和强度,提高飞行安全性。
汽车工程领域在汽车工程领域,拓扑优化密度法可以应用于车身结构的设计。
通过优化材料的分布,可以减轻车身重量,提高车辆的燃油经济性和动力性能。
拓扑优化方法
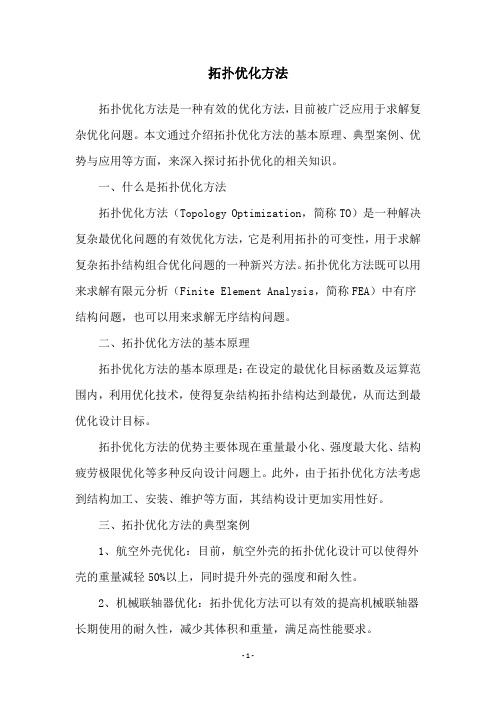
拓扑优化方法拓扑优化方法是一种有效的优化方法,目前被广泛应用于求解复杂优化问题。
本文通过介绍拓扑优化方法的基本原理、典型案例、优势与应用等方面,来深入探讨拓扑优化的相关知识。
一、什么是拓扑优化方法拓扑优化方法(Topology Optimization,简称TO)是一种解决复杂最优化问题的有效优化方法,它是利用拓扑的可变性,用于求解复杂拓扑结构组合优化问题的一种新兴方法。
拓扑优化方法既可以用来求解有限元分析(Finite Element Analysis,简称FEA)中有序结构问题,也可以用来求解无序结构问题。
二、拓扑优化方法的基本原理拓扑优化方法的基本原理是:在设定的最优化目标函数及运算范围内,利用优化技术,使得复杂结构拓扑结构达到最优,从而达到最优化设计目标。
拓扑优化方法的优势主要体现在重量最小化、强度最大化、结构疲劳极限优化等多种反向设计问题上。
此外,由于拓扑优化方法考虑到结构加工、安装、维护等方面,其结构设计更加实用性好。
三、拓扑优化方法的典型案例1、航空外壳优化:目前,航空外壳的拓扑优化设计可以使得外壳的重量减轻50%以上,同时提升外壳的强度和耐久性。
2、机械联轴器优化:拓扑优化方法可以有效的提高机械联轴器长期使用的耐久性,减少其体积和重量,满足高性能要求。
3、结构优化:通过拓扑优化方法,可以有效地减少刚性框架结构的重量,优化结构设计,改善结构性能,大大降低制造成本。
四、拓扑优化方法的优势1、灵活性强:拓扑优化方法允许在设计过程中改变结构形态,可以有效利用具有局部不稳定性的装配元件;2、更容易操作:拓扑优化方法比传统的有序结构模型更容易实现,不需要做过多的运算;3、成本低:拓扑优化方法可以有效降低产品的工艺制造成本,在改进出色性能的同时,可以节省大量人力物力;4、可重复性高:拓扑优化方法可以实现由抽象到具体的可重复的设计,可以实现大量的应用系统。
五、拓扑优化方法的应用拓扑优化方法目前被广泛应用在机械、航空航天、汽车等机械工程领域,具体应用包括但不限于:机械手和夹具的设计优化,汽车机架优化,电器结构优化,机械外壳优化,振动优化,和结构强度优化等等。
汽车工程学院认可的中,英文期刊目录(草案)
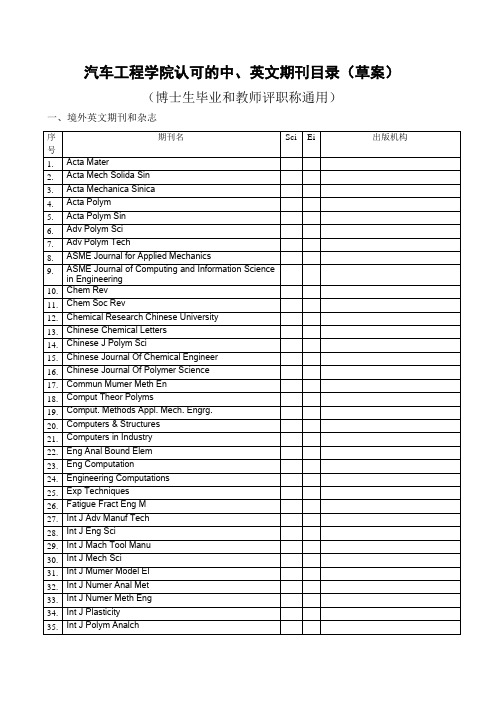
104. Chinese Journal Of Mechanical Engineering (English Edition) 机械工程学报 105. Chinese Optics Letters 106. High Technology Letters 107. Journal Of Beijing Institute Of Technology (English Edition) 108. Journal Of Central South University Of Technology (English Edition) 109. Journal Of Computational Information Systems 110. Journal Of Harbin Institute Of Technology (New Series) 111. Journal Of Hydrodynamics 112. Journal Of Information And Computational Science 113. Journal Of Materials Science And Technology
ห้องสมุดไป่ตู้
∨ ∨ ∨ ∨ ∨ ∨ ∨ ∨ ∨ ∨ ∨ ∨ ∨ ∨ ∨ ∨ ∨ ∨ ∨ ∨ ∨ ∨ ∨ ∨ ∨ ∨ ∨ ∨
130. 计算机科学与技术学报 (国内期刊英文版) 131. 计算数学 (国内期刊英文版) 132. 高等学校计算数学学报 133. 机械工程学报 (国内期刊英文版) 134. 科学通报 (国内期刊英文版) 135. 生物医学与环境科学 (国内期刊英文版) 136. 水动力学研究与进展: A,B辑 (国内期刊英文版) 137. 系统工程与电子技术 (国内期刊英文版) 138. 中国海洋工程 (国内期刊英文版) 139. 中国化学工程学报 (国内期刊英文版) 140. 中国科学 E,G辑 技术科学 (国内期刊英文版) 141. 自然科学进展 (国内期刊英文版) 142. Advances In Structural Engineering 143. Microcomputer Applications 144. 传感技术学报 145. 传感器技术 146. 电源技术 147. 动力工程 148. 中国电机工程学报 149. 钢铁 150. 冶金能源 151. 金属学报 152. 中国有色金属学报 153. 空气动力学学报
深层次动态优化设计理论及应用研究
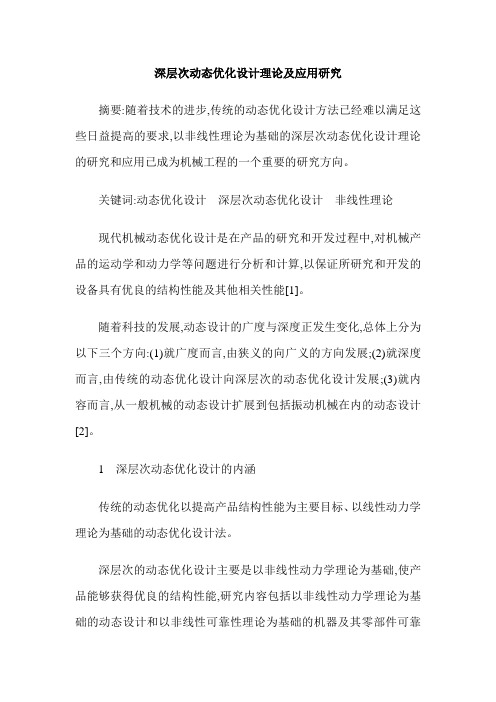
深层次动态优化设计理论及应用研究摘要:随着技术的进步,传统的动态优化设计方法已经难以满足这些日益提高的要求,以非线性理论为基础的深层次动态优化设计理论的研究和应用已成为机械工程的一个重要的研究方向。
关键词:动态优化设计深层次动态优化设计非线性理论现代机械动态优化设计是在产品的研究和开发过程中,对机械产品的运动学和动力学等问题进行分析和计算,以保证所研究和开发的设备具有优良的结构性能及其他相关性能[1]。
随着科技的发展,动态设计的广度与深度正发生变化,总体上分为以下三个方向:(1)就广度而言,由狭义的向广义的方向发展;(2)就深度而言,由传统的动态优化设计向深层次的动态优化设计发展;(3)就内容而言,从一般机械的动态设计扩展到包括振动机械在内的动态设计[2]。
1 深层次动态优化设计的内涵传统的动态优化以提高产品结构性能为主要目标、以线性动力学理论为基础的动态优化设计法。
深层次的动态优化设计主要是以非线性动力学理论为基础,使产品能够获得优良的结构性能,研究内容包括以非线性动力学理论为基础的动态设计和以非线性可靠性理论为基础的机器及其零部件可靠性设计。
2 深层次动态优化设计法的研究意义动态优化设计是机械设计内容中最重要和最具广泛性的问题。
而目前机械设计中,以静态设计为主,或采用传统动态设计方法。
但这对大型机械装备来说,这远远不能满足需要的。
对重大机械产品设备进行深层次的动力学设计,引入非线性动力学理论与方法,是十分必要的[3]。
3 深层次动态优化设计的应用现状3.1 在振动机械中的应用振动机械是泛指利用振动原理而工作的机械,它是化工、冶金、建筑等领域常见的设备,虽种类繁多,但基本工作原理相同[4]。
随着振动机械向大型化发展,对机械性能的要求越来越高。
例如对冶炼设备中的关键设备——振动筛的动负荷的要求随即增大,所以在振动状态下常会引起筛体结构强度和刚度不足,导致筛体变形过大,甚至使筛体出现断梁、侧板断裂等故障,造成严重后果。
- 1、下载文档前请自行甄别文档内容的完整性,平台不提供额外的编辑、内容补充、找答案等附加服务。
- 2、"仅部分预览"的文档,不可在线预览部分如存在完整性等问题,可反馈申请退款(可完整预览的文档不适用该条件!)。
- 3、如文档侵犯您的权益,请联系客服反馈,我们会尽快为您处理(人工客服工作时间:9:00-18:30)。
RESEARCH PAPEROptimal design of periodic structures using evolutionary topology optimizationX.Huang&Y.M.XieReceived:15May2007/Revised:24August2007/Accepted:20September2007/Published online:19October2007 #Springer-Verlag2007Abstract This paper presents a method for topology optimization of periodic structures using the bi-directional evolutionary structural optimization(BESO)technique.To satisfy the periodic constraint,the designable domain is divided into a certain number of identical unit cells.The optimal topology of the unit cell is determined by gradually removing and adding material based on a sensitivity analysis.Sensitivity numbers that consider the periodic constraint for the repetitive elements are developed.To demonstrate the capability and effectiveness of the proposed approach,topology design problems of2D and3D periodic structures are investigated.The results indicate that the optimal topology depends,to a great extent,on the defined unit cells and on the relative strength of other non-designable part,such as the skins of sandwich structures.Keywords Topology optimization.Periodic structure. Sandwich structure.Bi-directional evolutionary structural optimization(BESO)1IntroductionStructural topology optimization has become an effective design tool for obtaining more efficient and lighter structures.In recent years,various optimization methods such as the solid isotropic mircostructure with penalization method(Bendsøe1989;Zhou and Rozvany1991;Mlejnek 1992)and the evolutionary structural optimization(ESO) method(Xie and Steven1993;1997)have found increasing applications.The ESO method was originally introduced by Xie and Steven(1993)to obtain the optimum shape and topology of continuum structures.In this method,ineffi-cient material is slowly removed from the design domain. Bi-directional evolutionary structural optimization(BESO) is an extension of ESO,which allows for material to be added to the structure where it is most needed at the same time as the inefficient material is being removed.The BESO method proves to be more robust than the ESO method(Yang et al.1999;Huang and Xie2007).Periodic structures,e.g.the honeycomb core of a sandwich plate,are widely used in the structural designs because of their lightweight and ease of fabrication(Wadley et al.2003).A lightweight cellular material usually possesses periodic mircostructures.An inverse homogenization method was proposed by Sigmund(1994)and Sigmund and Torquato (1997)using periodic boundary conditions to tailor effective properties of cellular materials.As a result,the cellular material with extreme macroscopic properties has been found. However,the design of macrostructures with periodic geometries,e.g.the core of a lightweight sandwich for the mean compliance minimization,is different from the pure material design of microstructures.Recently,Zhang and Sun (2006)investigated scale-rated effects of the cellular material by combining the macroscopic design aimed at finding a preliminary layout of materials and the refined design to determine locally the optimal material microstructure.The method can be directly applied to the design of periodic structures,but the computational cost is expensive because it has to perform two finite-element analyses in each iteration, one for the macroscale optimization problem and the other for the microscale sub-optimization problem.For the design of periodic structures,the macroscopic distribution of theStruct Multidisc Optim(2008)36:597–606 DOI10.1007/s00158-007-0196-1X.Huang(*):Y.M.XieRMIT University,GPO Box2476V,Melbourne3001,Australia e-mail:huang.xiaodong@.auY.M.Xiee-mail:mike.xie@.audesignable material must be periodic,although the stress/straindistribution may not exhibit any periodic characteristics.Therefore,a general macroscopic optimization method with additional periodic constraints needs to be established to solve the optimization problems of periodic structures efficiently.This paper proposes a method for topology optimization of periodic structures under given boundary and loading conditions using the BESO method.Sensitivity numbers that consider the periodic constraint for the repetitive elements are developed.Then,the optimal topology of the unit cell is determined by gradually removing and adding material according to the sensitivity numbers.Finally,several 2D and 3D examples are presented.2Problem statement and sensitivity number 2.1Topology optimization problem for periodic design The objective of the present optimization problem is to find an optimal periodic topology of the structure for a given amount of material.Thus,the resulting structure will have the maximum stiffness to carry the prescribed force under the given boundary conditions.To consider the periodicity of a structure in a given designable domain,for example,for 2D cases,the designable domain is divided into m =m 1×m 2unit cells where m 1and m 2denote the numbers of unit cells along direction x and direction y ,respectively,as shown in Fig.1.The total number of the unit cell is usually prescribed by the client ’s or end-user ’s design specifications.It can be seen that the limit case of m=1×1corresponds to the conventional topology optimization problem.Thus,we can formulate the optimization problem related to the overall mean compliance minimization in terms of the binary design variable X i,j ,where i and j denote the cell number and element number in the cell,respectively (see Fig.1),as Minimize C ¼1f T u ð1a ÞSubject to :V ÃÀmV i ¼0ð1b ÞV i ¼X n j ¼1V i ;j x i ;jð1c Þx 1j ¼x 2j ¼ÁÁÁ¼x mj ð1d Þx i ;j 20;1f gj ¼1;2;ÁÁÁ;n ðÞi ¼1;2;ÁÁÁ;m ðÞð1e Þwhere f and u are the applied load and displacement vectors and C is known as the mean compliance.V i is the total volume of i th unit cell,V ij the volume of the j th element in the i th unit cell and V *the prescribed total structural volume.n is the total element number in a unit cell.The binary design variable X i,j declares the absence (0)or presence (1)of an individual element.Equation (1d)denotes that the status (1or 0)of elements for the same position in all unit cells remains to be the same so as to ensure the periodicity of the design as shown in Fig.1.2.2Elemental sensitivity numbersIn the conventional evolutionary optimization method,the sensitivity number is defined by the first-order variation of the mean compliance because of removing one element from the design domain.When i th solid element (1)is removed from a structure,the change of the mean compliance is approximately equal to the elemental strain energy as (Chu et al .1996)ΔC i ¼12u TiK i u i ð2Þwhere u i is the nodal displacement vector of the i th element and K i is the element stiffness matrix.The original ESO procedure is directly driven by gradually removing the lowest sensitivity elements.Similarly,when the j th element in the i th unit cell is removed,the change of the overall mean compliance is ΔC i ;j ¼12u Ti ;jK i ;j u i ;j ð3ÞBecause of the periodicity of the cells,the status (1or 0)of the j th element in all cells should be the same.In other words,these elements should be removed or added simultaneously.Therefore,the optimization process can be conducted in a representative unit cell,which can be selected from any unit cell by the user.According to (3),the change of the overall mean compliance because of the removal of a series of elements is approximately equal to the sum of the total strain energy stored in these elements.Therefore,the sensitivity number of the j th element in the representative unit cell is defined by the changes oftheFig.1A 2D design domain with m =6unit cells (where m =m 1×m 2and m 1and m 2denote the number of unit cells along the x and y direction;X i,j is the design variables where i and j denote the cell number and element number in the cell,respectively)598X.Huang,Y .M.Xieoverall mean compliance because of the removal of j th elements in all unit cells together a j ¼X m i ¼1ΔC i ;jð4ÞThe mesh of the representative unit cell can be selected from any unit cell in the structure.Topology of the structure can be solely expressed by the representative unit cell because the whole structure is divided into m -independent and identical unit cells.Therefore,the following optimization algorithm can only be applied in the representative unit cell.3Filtering and update sensitivity numbersTopology optimization can often exhibit an instability for which the resulting topology contains checkerboard pat-terns of solid and void elements.It has been established that the appearance of the checkerboard patterns because of the mixed variable problem does not represent an optimal topology (Sigmund and Petersson 1998).A most popular heuristic treatment for preventing checkerboards is filtering sensitivity numbers,which is based on image-processing techniques and works as a low-pass filter eliminate features below a certain length scale in the optimal designs.First,the nodal sensitivity numbers a n i ÀÁ,which do not carry any physical meaning on their own,are defined by averaging the sensitivity numbers of connected elements.Then,these nodal sensitivity numbers must be converted back into elements before the topology can be determined.In this process,a filter scheme is used to carry it out.The defined filter functions are based on a length scale r min .The primary role of the scale parameter r min in the filter scheme is to identify the nodes that influence the sensitivity of i th element.This can be visualized by drawing a circle of radius r min centred at the centroid of i th element,thus generating the circular sub-domain Ωi .Nodes located inside Ωi contribute to the computation of the improved sensitivity number of j th element asa 1j ¼PM i ¼1w r ij ÀÁa n iPi ¼1w r ijÀÁð5Þwhere a 1j denotes the sensitivity number after filtering,M is the total number of nodes in the sub-domain Ωi and w (r ij )is the linear weight factor defined by w r ij ÀÁ¼r min Àr ij i ¼1;2;ÁÁÁ;M ðÞð6Þwhere r ij is the distance between the centre of the element j and node i .It can be seen that the above filter scheme is similar to the mesh-independency filter used in Sigmundand Petersson (1998)except that the nodal sensitivitynumbers are used.If the filter is based on the elemental sensitivities,the BESO method will be converted into the ESO method without the capability of adding material when the filter domain only covers one element.As a result,the checkerboard pattern would be unavoidable.The main function of the filter is to smooth the sensitivity number within the design domain of the base cell.It should be pointed out that Sigmund and Petersson (1998)consid-ers the elemental density in the filter,thus the sensitivity number will be infinite for void elements.However,the present filter does not consider the element states (0or 1),and the initial sensitivity number for void (0)elements is ing the above filter technique,non-zero sensitivity numbers for void (0)elements are obtained as a result of filtering the sensitivity numbers of solid (1)elements.Some void (0)elements may be added in the later design.It can be seen that the sensitivity numbers of the solid (1)and void (0)elements are based on the different status (1or 0)of the element.Therefore,there is an abrupt change of the sensitivity number when an element changes the status.As a result,the objective function and topology are unstable and hard to putational experience has shown that averaging the sensitivity number with its historical information is an effective way to avoid this problem (Huang and Xie 2007).It can be simply expressed by a 2j¼a 1j ;k þa 1j ;k À12ð7Þwhere k is the current iteration number.Thus,the updated sensitivity number includes all sensitivity information in the previous iterations.Note that the sensitivity number is a measure of the approximate variation of the objective function because of element removal or addition in the representative unit cell.To minimize the mean compliance,the evolutionary process will be conducted by removing elements with the smallest sensitivity numbers and adding the elements with the highest ones.Mathematically,such a procedure is known as the ‘hill-climb ’method or the ‘steepest descent ’algorithm.4Numerical implementationThe evolutionary iteration procedure for solving the optimization problems for periodic structures can be outlined as follows:1.Create the analysis model,and apply the boundaryand loading conditions.2.Discretize the model using an finite-element mesh,and assign the initial property values (0or 1)of elements to construct the initial design.Optimal design of periodic structures using evolutionary topology optimization 5993.Perform finite-element analysis on the current designto obtain elemental sensitivity numbers in (3).4.Identify the group of elements according to theperiodic constraint and obtain elemental sensitivity numbers of the representative unit cell using (4).5.Conduct filtering and averaging sensitivity numbers withits history information in the representative unit cell.6.Determine the target volume for the next iteration bygradually decreasing or increasing material asV i þ1¼V i 1ÆER ðÞi ¼1;2;3ÁÁÁðÞð8a Þwhere ER is called the evolutionary volume ratio.Once the objective volume is reached,the volume will be kept constant for the remaining iterations as V i þ1¼V Ãð8b Þ7.Reset the property values of elements for therepresentative unit cell.For solid elements,the property value is switched from 1to 0if the following criterion is satisfied.a i a thð9a ÞFor void elements,the property value is switched from 0to 1if the following criterion is satisfied.a i >a thð9b ÞWhere αth is the threshold of the sensitivity number that is determined by the target material volume in each iteration.For example,if the target volume for the present iteration is 70%of the unit cell,then elements for which sensitivity numbers are ranked at the top 70%of all element in the unit cell will remainto be solid or be added,and all other elements will be removed or remain to be void.8.Construct a new representative unit cell using ele-ments with property value 1.9.Reassign property values of elements in all unit cells ac-cording to the representative unit cell.As a result,a new design is constructed for the next finite-element analysis.10.Repeat steps 3–9until a convergent solution isobtained.The following convergence criterion will be applied after the volume constraint is satisfied.t ¼P N i ¼1C k Ài þ1ÀC k ÀN Ài þ1ðÞP i ¼1C k Ài þ1t max ð10Þwhere k is the current iteration number,τmax is a allowable convergence error and N is integral number.τmax =0.1%and N =5are selected throughout this paper,which means a stable compliance in ten successive iterations.5Examples and discussion5.1Numerical verification of the optimal design for a periodic structureThe topology optimization for a continuum structure is complex because of a large number of design variables.Evolutionary structural optimization offers an alternative method for solving various topology optimizationproblemsFig.2Design domain of the optimization problem in Zhang and Sun (2006)600X.Huang,Y .M.Xieof continuum structures (Xie and Steven 1997).Theoreti-cally,it is noted that the sequential linear programming-based approximate optimization method followed by the Simplex algorithm is equivalent to ESO/BESO (Tanskanen 2002).However,had the optimization problem been solved with a periodic constraint,the structure would still evolve to an optimal solution.To verify the proposed method,an optimization problem in Zhang and Sun (2006)is tested.The 2D rectangular domain of the problem with L =32and H =20is shown in Fig.2.The designable domain refers to the inner core with H 1=16.The plate is fixed on the left end and loaded vertically with F =100(force/length)on the right end.Young ’s modulus and Poisson ’s ratio of material are E =1000and ν=0.3.The objective of the problem is to find the optimal topology of the core with a volume fraction of 50%over the core area.To avoid the singularity of the problem,a small non-designable elastic portion is added artificially along the right edge to transfer the applied load.Four cases for m =2×1,4×2,8×4and 16×8will be studied and compared.In consistence with the periodic pattern in Zhang and Sun (2006),the symmetric unit cells about the neutral axis are arranged for later three cases.The filter radius is selected to be r min =1.5,1.0,0.5and 0.3for m =21,4×2,8×4and 16×8,respectively.BESO starts from the full design and gradually decreases the volume fraction with the parameter ER=2%until the constraint of the volume fraction 50%is achieved.Then,the volume keeps constant until the defined convergent criterion is satisfied.Figure 3shows the evolutionary his-tories of topology,volume fraction and objective function (mean compliance)for m =2×1.It can be seen that the topology volume fraction and objective function are all con-vergent at the end of the optimization process.Figure 4shows the final optimal topologies for all four cases,which are very similar to the topologies of Zhang and Sun (2006).The mean compliance are 72,899.3,73,843.3,77,617.1and 79,706.2for m =2×1,4×2,8×4and 16×8,which are much lower than the corresponding solutions of Zhang and Sun (2006),which are 82,530.6,84,012.9,88,308.3and 90,547.5,respectively.This difference mainly attributes to the effect of the soft material whose strain energy is arti-ficially increased because of the penalized parameter,p>1.Fig.3Evolutionary histories of volume fraction,mean compli-ance and topology for the peri-odic condition with m=2×1Fig.4Optimal designs for various periodic constraints a m =2×1,b m =4×2,c m =8×4and d m =16×8Optimal design of periodic structures using evolutionary topology optimization 6015.2Example 1:2D sandwich designThe proposed method can be applied to the cellular core design of a sandwich structure as shown in Fig.5where the sandwich structure is fixed at both ends of skins.The designable core is a rectangle of size 160by 40mm,which is divided into 320×80four-node plane stress elements,and the two skins have 1mm thickness,which is divided into 320beam elements.It is assumed that the skins and core are tied together.A vertical point force P =1N is applied at the middle point of the top skin.The materials of the skins and core are assumed with Young ’s modulus 100GPa and Poisson ’s ratio 0.3and Young ’s modulus 1GPa and Poisson ’s ratio 0.3,respectively.Assume that only 30%of the material is available to construct the final design.The filter radius and evolutionary ratio are selected to be r m =2mm and ER=1%.Figure 6shows the evolutionary histories of topology,volume fraction and objective function for m =2×1.It can beseen that all topologies satisfy the defined periodic con-ditions and the mean compliance of the final topology is 8.33Nm.Table 1lists the optimal topologies and their mean compliances for various number of unit cells (m ).A typical unit cell is given inside dash lines except for m =1×1.Related mean compliance values are plotted against the number of the unit cells in Fig.7.In general,the mean compliance increases with the number of unit cells because the number of constraints associated with the design variables increases.Therefore,the solution of the conven-tional BESO method corresponding to a limit case with m =1×has the minimum mean compliance.On the other hand,the optimal topology depends on the aspect ratio of the unit cell.For example,the optimal topologies for m =2×1and m =1×2are different,although their total numbers of unit cells are equal.The present BESO method may starts from an initial guess design,which may be highly non-optimal.As an example,Fig.8shows the evolutionary histories of topology,volume fraction and mean compliance for m =2×1.The compliance and volume are both decreasing at the beginning,which indicates that the overall stiffness of the structure has improved because of the optimization algorithm,although the volume of the structure becomes less.The volume fraction remains constant after the seventh iteration,and the compliance continues to decrease until it converges to a constant value,8.33Nm,which is equal to the solution shown in Fig.7.The advantage ofthisFig.5Design domain of 2D sandwichstructureFig.6Evolutionary histories of volume fraction,mean compli-ance and topology for 2D sand-wich structure with m =2×1when BESO starts from the initial full design602X.Huang,Y .M.Xieprocedure is that the optimization process is straightforward from the history of the objective function.Another advantage is that this procedure possesses a high compu-tational efficiency because only a portion of all elements in the full model is involved in the finite-element analysis.Nevertheless,the BESO starting from an initial guess design may converge to a local optimal solution because some void elements in the initial guess design may never be included in the finite-element analysis during the whole optimization process.To eliminate or reduce the likelihood of a local optimum,sometimes it might be necessary that BESO should start from the initial full design;thus,allelements are involved in the finite-element analysis at least once (Huang and Xie 2007).5.3Example 2:2D bridge designFigure 9shows an optimization problem for designing a bridge.The design domain is a rectangle of size L =240,H =60and thickness t =1,the bottom deck with length L =240,height h =5and thickness t =1is supported at two bottom corners,and a vertical force P =100is applied at the middle point of the bottom deck.The design domain is discretized by a 240×60four-node quadrilateral element mesh,and the non-designable deck is meshed with 240beam elements.The nodes of the beam elements are connected with those of the plate elements at the bottom side of the design domain.The materials for the design domain and the deck are same to be Young ’s modulus E =200and Poisson ’s ratio ν=0.3.Suppose only 30%of the designable domain material is available for constructing the final structure.The evolutionary ratio ER=2%is used in this example.Figure 10a shows the optimal design with m =1×1,which is the same to the conventional optimal design,and the mean compliance of the design is 1.12.When the design domain is divided with unit cells,the final designs are shown in Fig.10b and c for m =4×1and m =6×1,respectively.Their mean compliances are 1.53and 1.78,which are higher than that of the conventional design.Similar to the above example,the mean compliance increases with the total number of unit cells.To study the influence of the deck,the above problem with the height of the deck h =50is solved with m =4×1.Table 1Optimal designs and their mean compliance for 2D sandwich structures under various periodicconstraintFig.7Variation of mean compliance against the number of total unit cells,mOptimal design of periodic structures using evolutionary topology optimization603The final design is shown in Fig.11,which totally differs from the above one shown in Fig.10b.It demonstrates that the optimal topology may also depend on the strength of other non-designable parts.5.43D sandwich designsThe proposed optimization approach can also be extended for designing 3D periodic structures.Figure 12shows a sandwich cantilever undergoing four vertical concentrated loads P =1.The designable core of the size 100×20×40is divided into a 100×20×40mesh using eight-node cubic elements,and both non-designable skins with a unit thickness are divided into a 100×20mesh using four-node plate elements.The materials are assumed with Young ’s modulus E =1,Poisson ’s ratio ν=0.3for the core and E =100,ν=0.3for both skin plates.The objective is to obtain the optimal periodic layout of the core while minimizing the mean compliance of the design and at the same timesatisfying the requirement of allowable material volume,10%of the designable domain.The evolutionary ratio ER=2%is used in this example.Figure 13a shows that the optimal topology with m =1×1×1and its mean compliance is 40.9.Figure 13b and c show that the final topologies for the number of unit cells m =4×1×1and m =4×2×1and their mean compliance are 45.4andFig.8Evolutionary histories of volume fraction,mean compli-ance and topology for 2D sand-wich structure with m =2×1when BESO starts from the initial guessdesignFig.9The optimization problem for 2D bridgestructureFig.10Optimal designs and their mean compliance for 2D bridge structure a m =1×1,C =1.12,b m =4×1,C =1.53,c m =6×1,C =1.78604X.Huang,Y .M.Xie49.5,respectively.It is also found that the mean compliance increases with the total number of 3D unit cells.6ConclusionsA method for topology optimization of periodic structures has been developed in this paper.Additional periodic constraint has been added to the optimization formulation to ensure that the structure comprises a prescribed number of identical unit cells.The optimal topology of the unit cell is found by gradually removing and adding material using the BESO method.Several 2D and 3D examples are presented.The following conclusions can be drawn:1.The proposed method can be used to effectivelyoptimize 2D and 3D periodic structures.With a given amount of material,the optimal design from the proposed method will have a substantially lower mean compliance than the initial,guess design.2.The optimal topology highly depends on the totalnumber and the aspect ratio of the unit cells.3.The value of the objective function (mean compliance)becomes higher when the total number of unit cells increases.Thus,the solution of the conventionaltopology optimization corresponding to the limit case with m =1×1has the lowest mean compliance.How-ever,the advantage of a periodic design is that the manufacturing or construction cost could be much reduced.4.The optimal topology of the designable domain mayalso depend on the relative strength of other non-designable parts,such as the skins of the sandwichstructures.Fig.11Optimal designs with m =4×1for 2D bridge structure with strong deck h=50Fig.12The optimization problem for 3D sandwichstructureFig.13Optimal designs for 3D sandwich structure a m =1×1×1,C =40.9,b m =4×1×1,C =45.4,c m =4×2×1,C =49.5Optimal design of periodic structures using evolutionary topology optimization 605Acknowledgements The authors wish to acknowledge the financial support from the Australian Research Council for this work. ReferencesBendsøe MP(1989)Optimal shape design as a material distribution problem.Struct Optim1:193–202Chu DN,Xie YM,Hira A,Steven GP(1996)Evolutionary structural optimization for problems with stiffness constraints.Finite Elem Anal Des21:239–51Huang X,Xie YM(2007)Convergent and mesh-independent solutions for the bi-directional evolutionary structural optimization method.Finite Elem Anal Des,doi:10.1016/j.finel.2007.06.006 Mlejnek HP(1992)Some aspects of the genesis of structures.Struct Optim5:64–69Querin OM,Young,V,Steven,GP,Xie,YM(2000)Computational efficiency and validation of bi-directional evolutionary structural put Methods Appl Mech Eng189:559–573 Sigmund O(1994)Materials with prescribed constitutive parameters: an inverse homogenization problem.Int J Solids Struct31(17):2313–2329Sigmund O,Petersson J(1998)Numerical instabilities in topology optimization:a survey on procedures dealing with checkerboards, mesh-dependencies and local minima.Struct Optim16:68–75 Sigmund O,Torquato S(1997)Design of materials with extreme thermal expansion using a three-phase topology optimization method.J Mech Phys Solids45(6):1037–1067Tanskanen P(2002)The evolutionary structural optimization method: theoretical put Methods Appl Mech Eng191:5485–5498 Wadley HNG,Fleck NA,Evan AG(2003)Fabrication and structural performance of periodic cellular metal sandwich structures.Compos Sci Technol63:2331–2343Xie YM,Steven GP(1993)A simple evolutionary procedure for structural put Struct49:885–896Xie YM,Steven GP(1997)Evolutionary structural optimization.Springer,LondonYang XY,Xie,YM,Steven,GP,Querin,OM(1999)Bidirectional evolutionary method for stiffness optimization.AIAA Journal37(11):1483–1488Zhang W,Sun S(2006)Scale-related topology optimization of cellular materials and structures.Int J Numer Methods Eng68:993–1011 Zhou M,Rozvany GIN(1991)The COC algorithm,part II: topological,geometry and generalized shape p Methods Appl Mech Eng89:197–224606X.Huang,Y.M.Xie。