音频去噪 Enhancing Speech by removing noise
音频处理中的噪音消除技术
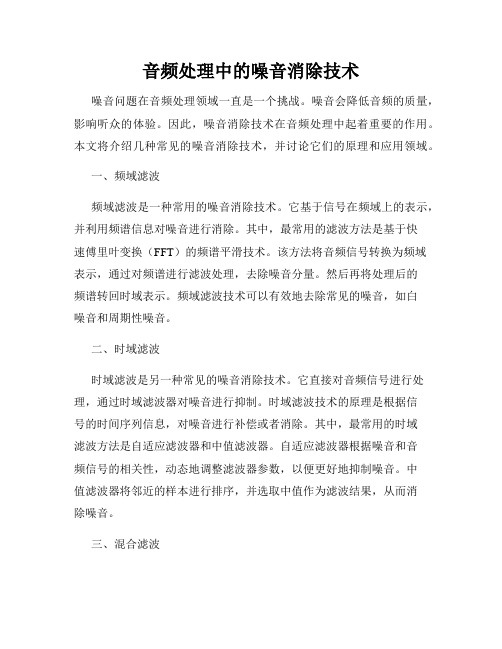
音频处理中的噪音消除技术噪音问题在音频处理领域一直是一个挑战。
噪音会降低音频的质量,影响听众的体验。
因此,噪音消除技术在音频处理中起着重要的作用。
本文将介绍几种常见的噪音消除技术,并讨论它们的原理和应用领域。
一、频域滤波频域滤波是一种常用的噪音消除技术。
它基于信号在频域上的表示,并利用频谱信息对噪音进行消除。
其中,最常用的滤波方法是基于快速傅里叶变换(FFT)的频谱平滑技术。
该方法将音频信号转换为频域表示,通过对频谱进行滤波处理,去除噪音分量。
然后再将处理后的频谱转回时域表示。
频域滤波技术可以有效地去除常见的噪音,如白噪音和周期性噪音。
二、时域滤波时域滤波是另一种常见的噪音消除技术。
它直接对音频信号进行处理,通过时域滤波器对噪音进行抑制。
时域滤波技术的原理是根据信号的时间序列信息,对噪音进行补偿或者消除。
其中,最常用的时域滤波方法是自适应滤波器和中值滤波器。
自适应滤波器根据噪音和音频信号的相关性,动态地调整滤波器参数,以便更好地抑制噪音。
中值滤波器将邻近的样本进行排序,并选取中值作为滤波结果,从而消除噪音。
三、混合滤波混合滤波是一种结合了频域滤波和时域滤波的噪音消除技术。
它通过同时应用频域和时域滤波器,以在多个领域中消除噪音。
混合滤波技术的优点是能够更全面地处理不同类型和频率范围的噪音。
此外,混合滤波还可以根据实际应用需求进行参数调整和优化,以获得更好的噪音消除效果。
四、机器学习方法近年来,机器学习方法在音频处理中的噪音消除中得到了广泛应用。
机器学习方法可以根据大量标注的训练数据,学习出噪音和音频信号之间的映射关系,并对噪音进行预测和消除。
常见的机器学习方法包括支持向量机(SVM)、深度神经网络(DNN)和卷积神经网络(CNN)。
这些方法可以有效地处理复杂的噪音环境,并获得较好的噪音消除效果。
五、应用领域噪音消除技术在多个领域都有重要的应用。
其中,最常见的应用是音频通信和语音识别。
在音频通信中,噪音消除技术可以提高语音的清晰度和可懂度,使通信更加畅顺。
语音识别中的语音增强与去噪技术
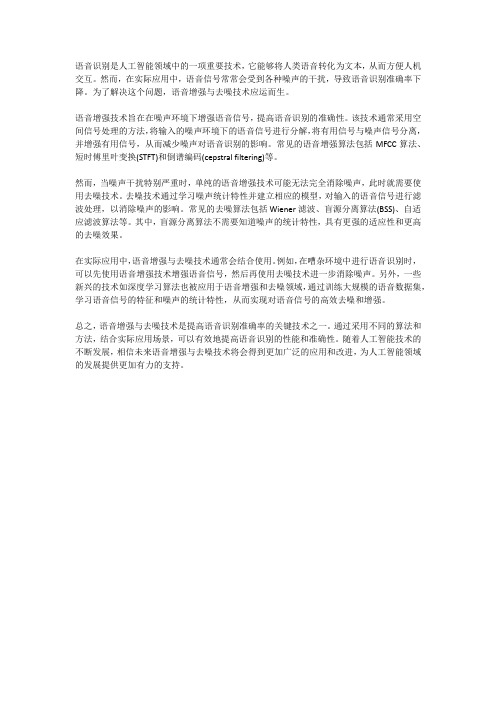
语音识别是人工智能领域中的一项重要技术,它能够将人类语音转化为文本,从而方便人机交互。
然而,在实际应用中,语音信号常常会受到各种噪声的干扰,导致语音识别准确率下降。
为了解决这个问题,语音增强与去噪技术应运而生。
语音增强技术旨在在噪声环境下增强语音信号,提高语音识别的准确性。
该技术通常采用空间信号处理的方法,将输入的噪声环境下的语音信号进行分解,将有用信号与噪声信号分离,并增强有用信号,从而减少噪声对语音识别的影响。
常见的语音增强算法包括MFCC算法、短时傅里叶变换(STFT)和倒谱编码(cepstral filtering)等。
然而,当噪声干扰特别严重时,单纯的语音增强技术可能无法完全消除噪声,此时就需要使用去噪技术。
去噪技术通过学习噪声统计特性并建立相应的模型,对输入的语音信号进行滤波处理,以消除噪声的影响。
常见的去噪算法包括Wiener滤波、盲源分离算法(BSS)、自适应滤波算法等。
其中,盲源分离算法不需要知道噪声的统计特性,具有更强的适应性和更高的去噪效果。
在实际应用中,语音增强与去噪技术通常会结合使用。
例如,在嘈杂环境中进行语音识别时,可以先使用语音增强技术增强语音信号,然后再使用去噪技术进一步消除噪声。
另外,一些新兴的技术如深度学习算法也被应用于语音增强和去噪领域,通过训练大规模的语音数据集,学习语音信号的特征和噪声的统计特性,从而实现对语音信号的高效去噪和增强。
总之,语音增强与去噪技术是提高语音识别准确率的关键技术之一。
通过采用不同的算法和方法,结合实际应用场景,可以有效地提高语音识别的性能和准确性。
随着人工智能技术的不断发展,相信未来语音增强与去噪技术将会得到更加广泛的应用和改进,为人工智能领域的发展提供更加有力的支持。
remove noise翻译
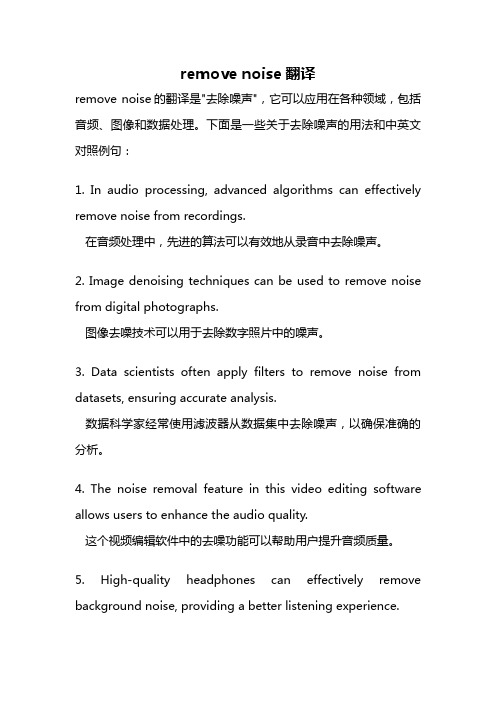
remove noise翻译remove noise的翻译是"去除噪声",它可以应用在各种领域,包括音频、图像和数据处理。
下面是一些关于去除噪声的用法和中英文对照例句:1. In audio processing, advanced algorithms can effectively remove noise from recordings.在音频处理中,先进的算法可以有效地从录音中去除噪声。
2. Image denoising techniques can be used to remove noise from digital photographs.图像去噪技术可以用于去除数字照片中的噪声。
3. Data scientists often apply filters to remove noise from datasets, ensuring accurate analysis.数据科学家经常使用滤波器从数据集中去除噪声,以确保准确的分析。
4. The noise removal feature in this video editing software allows users to enhance the audio quality.这个视频编辑软件中的去噪功能可以帮助用户提升音频质量。
5. High-quality headphones can effectively remove background noise, providing a better listening experience.高品质的耳机可以有效地去除背景噪声,提供更好的听音体验。
6. The noise-canceling feature on this microphone eliminates unwanted background noise during recording.这款麦克风上的降噪功能可以在录音过程中消除不必要的背景噪声。
音频去噪与音效优化技巧
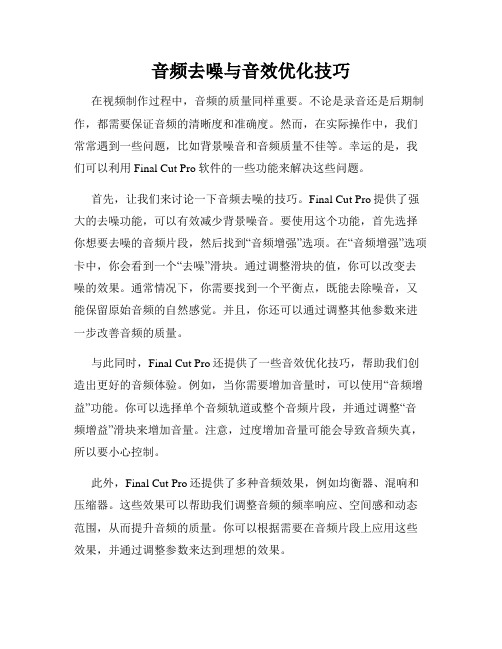
音频去噪与音效优化技巧在视频制作过程中,音频的质量同样重要。
不论是录音还是后期制作,都需要保证音频的清晰度和准确度。
然而,在实际操作中,我们常常遇到一些问题,比如背景噪音和音频质量不佳等。
幸运的是,我们可以利用Final Cut Pro软件的一些功能来解决这些问题。
首先,让我们来讨论一下音频去噪的技巧。
Final Cut Pro提供了强大的去噪功能,可以有效减少背景噪音。
要使用这个功能,首先选择你想要去噪的音频片段,然后找到“音频增强”选项。
在“音频增强”选项卡中,你会看到一个“去噪”滑块。
通过调整滑块的值,你可以改变去噪的效果。
通常情况下,你需要找到一个平衡点,既能去除噪音,又能保留原始音频的自然感觉。
并且,你还可以通过调整其他参数来进一步改善音频的质量。
与此同时,Final Cut Pro还提供了一些音效优化技巧,帮助我们创造出更好的音频体验。
例如,当你需要增加音量时,可以使用“音频增益”功能。
你可以选择单个音频轨道或整个音频片段,并通过调整“音频增益”滑块来增加音量。
注意,过度增加音量可能会导致音频失真,所以要小心控制。
此外,Final Cut Pro还提供了多种音频效果,例如均衡器、混响和压缩器。
这些效果可以帮助我们调整音频的频率响应、空间感和动态范围,从而提升音频的质量。
你可以根据需要在音频片段上应用这些效果,并通过调整参数来达到理想的效果。
另一个常见的问题是音频同步。
有时候,在录制过程中,视频和音频的同步会出现偏差。
Final Cut Pro提供了一个简单而强大的工具来解决这个问题,那就是“音频调整”。
你只需要选择需要调整的音频片段,然后在顶部的工具栏中选择“音频调整”选项。
在弹出的“音频调整”面板中,你可以通过调整“时间刻度”和“百分比”来实现音频的微调。
最后,还有一些其他小技巧可以帮助我们更好地处理音频问题。
例如,你可以使用“音频淡入淡出”功能来平滑音频的起始和结束部分。
此外,通过使用“音频分割”功能,你可以对音频进行剪裁和编辑,以满足你的需要。
语音信号处理中的语音增强与噪声消除算法
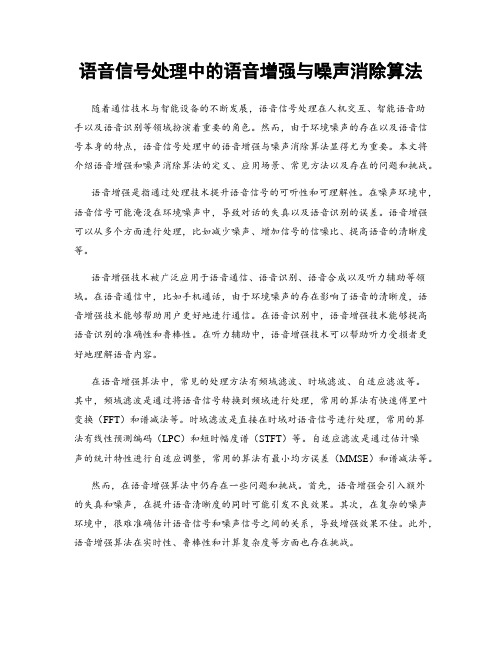
语音信号处理中的语音增强与噪声消除算法随着通信技术与智能设备的不断发展,语音信号处理在人机交互、智能语音助手以及语音识别等领域扮演着重要的角色。
然而,由于环境噪声的存在以及语音信号本身的特点,语音信号处理中的语音增强与噪声消除算法显得尤为重要。
本文将介绍语音增强和噪声消除算法的定义、应用场景、常见方法以及存在的问题和挑战。
语音增强是指通过处理技术提升语音信号的可听性和可理解性。
在噪声环境中,语音信号可能淹没在环境噪声中,导致对话的失真以及语音识别的误差。
语音增强可以从多个方面进行处理,比如减少噪声、增加信号的信噪比、提高语音的清晰度等。
语音增强技术被广泛应用于语音通信、语音识别、语音合成以及听力辅助等领域。
在语音通信中,比如手机通话,由于环境噪声的存在影响了语音的清晰度,语音增强技术能够帮助用户更好地进行通信。
在语音识别中,语音增强技术能够提高语音识别的准确性和鲁棒性。
在听力辅助中,语音增强技术可以帮助听力受损者更好地理解语音内容。
在语音增强算法中,常见的处理方法有频域滤波、时域滤波、自适应滤波等。
其中,频域滤波是通过将语音信号转换到频域进行处理,常用的算法有快速傅里叶变换(FFT)和谱减法等。
时域滤波是直接在时域对语音信号进行处理,常用的算法有线性预测编码(LPC)和短时幅度谱(STFT)等。
自适应滤波是通过估计噪声的统计特性进行自适应调整,常用的算法有最小均方误差(MMSE)和谱减法等。
然而,在语音增强算法中仍存在一些问题和挑战。
首先,语音增强会引入额外的失真和噪声,在提升语音清晰度的同时可能引发不良效果。
其次,在复杂的噪声环境中,很难准确估计语音信号和噪声信号之间的关系,导致增强效果不佳。
此外,语音增强算法在实时性、鲁棒性和计算复杂度等方面也存在挑战。
与语音增强算法相对应,噪声消除算法着重于降低环境噪声对语音信号的影响,使得语音信号更加清晰可听。
噪声消除广泛应用于语音通信、音频处理、语音识别以及语音合成等领域。
语音上行去噪经典算法

语音上行去噪经典算法语音去噪是指在语音通信或语音处理过程中,采用算法来减少或消除噪声对语音信号的影响,使语音更加清晰。
常用的语音去噪算法包括经典的谱减法、Wiener 滤波器和子空间方法等。
1. 谱减法是一种经典的语音去噪算法。
它基于傅里叶分析,将语音信号从时域转换到频域,通过对频域幅度进行修剪来减少噪声。
该算法的基本原理是在短时傅里叶变换(STFT)的基础上,对每个频带的幅度进行修正,减小低信噪比(SNR)的频带的幅度,然后进行逆变换得到去噪后的语音。
2. Wiener滤波器是一种以最小均方误差为准则的自适应滤波器。
该算法假设语音信号和噪声信号是高斯随机过程,通过最小化均方误差来估计信号和噪声的功率谱密度。
Wiener滤波器的基本原理是在频域采用逐帧处理,通过估计语音信号和噪声信号的功率谱密度比值,计算出每个频带的Wiener滤波器增益,然后将滤波器增益应用到频谱上得到去噪结果。
3. 子空间方法是一种基于信号与噪声在子空间中的性质来进行去噪的方法。
该算法利用信号与噪声在统计上的互相独立性,在子空间中对语音信号和噪声信号进行分离。
子空间方法常用的算法包括主成分分析(PCA)、独立成分分析(ICA)和稀疏表示等。
谱减法、Wiener滤波器和子空间方法是常用的语音去噪算法,它们在实际应用中有各自的优缺点。
谱减法简单易实现,适用于低噪声的情况,但在高噪声环境中会产生伪声;Wiener滤波器对于高噪声环境和非高斯噪声具有较好的去噪效果,但在弱信号和非平稳噪声环境下效果较差;子空间方法具有较好的去噪效果,对于非线性噪声具有较好的适应性,但计算复杂度较高。
除了经典算法外,近年来也出现了一些使用深度学习进行语音去噪的方法,如基于卷积神经网络(CNN)的去噪自编码器、基于循环神经网络(RNN)的长短时记忆网络(LSTM)等。
这些算法通过学习大量训练数据,利用神经网络的强大拟合能力来进行语音信号和噪声信号之间的映射,从而实现去噪效果。
remove noise翻译

remove noise翻译remove noise的翻译可以是“去除噪音”。
下面是一些关于remove noise的用法和中英文对照例句:1. Remove noise from audio recordings:去除音频录音中的噪音。
Example: The audio editing software can remove noise from the recording.例句:这款音频编辑软件可以去除录音中的噪音。
2. Remove noise from images:去除图像中的噪点。
Example: The photo editing app has a feature to remove noise from images.例句:这款照片编辑应用程序有一个功能可以去除图像中的噪点。
3. Remove background noise during video calls:在视频通话中去除背景噪音。
Example: The noise-canceling microphone can effectively remove background noise during video calls.例句:这款降噪麦克风可以有效地在视频通话中去除背景噪音。
4. Use noise reduction techniques in music production:在音乐制作中使用降噪技术。
Example: The producer applied noise reduction techniques to clean up the audio tracks.例句:制作人运用降噪技术来清理音频轨道。
5. Remove noise pollution in urban areas:减少城市地区的噪音污染。
Example: The government implemented measures to remove noise pollution in busy city centers.例句:政府采取了措施来减少繁忙城市中的噪音污染。
音频去噪与降噪技巧详解

音频去噪与降噪技巧详解在音频处理中,噪音是一个普遍存在的问题,它们可以来自录音设备、外部环境或其他因素。
这些噪音会严重影响音频质量和听感。
Adobe Premiere Pro是一个功能强大的视频编辑软件,同时也提供了丰富的音频处理功能。
本文将为大家介绍一些Adobe Premiere Pro中常用的音频去噪与降噪技巧,帮助大家改善音频质量。
1. 使用噪音消除器Adobe Premiere Pro内置了一个噪音消除器效果,可以有效消除背景噪音。
首先,在音轨上选中需要去噪的音频片段,然后点击“效果”菜单,选择“音频效果”,再选择“噪音消除器”。
在“噪音消除器”面板中,调整参数直至满意的效果。
主要的参数包括“减少噪音门限”、“噪音削弱量”和“噪音调整”。
减少噪音门限控制了噪音被消除的最小阈值,噪音削弱量控制了消除的噪音幅度,噪音调整则可以调整噪音消除前后的相对音量。
2. 使用均衡器均衡器可以调整频谱中不同频段的音量,从而控制音频的质量和平衡。
在Adobe Premiere Pro中,可以使用内置的均衡器效果来调整音频。
选中需要调整的音频片段,点击“效果”菜单,选择“音频效果”,再选择“均衡器”。
在“均衡器”面板中,可以通过上下拖动不同频段的滑块来调整音量。
如果想要去掉某个频段的噪音,可以将对应频段的滑块下拉到最低。
3. 使用降噪插件除了内置的噪音消除器和均衡器外,Adobe Premiere Pro还支持第三方降噪插件。
这些插件通常具有更高级的降噪算法和更丰富的调节选项,可以提供更好的降噪效果。
在使用第三方插件时,需要先将插件文件安装到Premiere Pro的插件目录,然后在效果面板中找到对应的插件并将其应用到音频上。
具体的操作步骤可以参考插件厂商提供的使用说明。
4. 使用音频关键帧调节音频关键帧是指在音频中添加关键点,并通过调整关键点的音量来控制音频的变化。
在Adobe Premiere Pro中,可以使用音频关键帧来调整音频的噪音水平。
- 1、下载文档前请自行甄别文档内容的完整性,平台不提供额外的编辑、内容补充、找答案等附加服务。
- 2、"仅部分预览"的文档,不可在线预览部分如存在完整性等问题,可反馈申请退款(可完整预览的文档不适用该条件!)。
- 3、如文档侵犯您的权益,请联系客服反馈,我们会尽快为您处理(人工客服工作时间:9:00-18:30)。
L ABORATORY 5 Enhancing Speech by removing noisePurposeThe main purpose of this lab is to design different IIR and FIR filters in the context of enhancing a peech corrupted by an additive noise. By doing so, this will help us to better understand the properties of different types of filters, the meanings of the design parameters, and some of the trade‐offs between filter classes.In order to see the behavior of these different types of filters, we were given a noisy speech signal and were asked to design a low-pass filter in order to remove the high-pass noise. After doing this, we had to pass the audio file through each of these filters in order to see the if the speech was filtered correctly and what is the effect of each filter on the filtered signal. The noisy signal was sampled at a frequency Fs = 44100 Hz and the filter specifications are:Part I : Matlab RipplePart II: Butterworth filterIn this part, we had to design a Butterworth filter that satisfies the given specifications.We used Matlab fdatool to design this filter. In order to use this Matlab built-in tool, we had to convert the above given specifications into IIR filter specifications. Thus, we took:Apass = Gpbmax – Gpbmin = 40 – 37 = 3dBAstop = Gpbmax – Gsbmax = 40 -(- 55) = 95dBAs for Fpass, Fstop and Fs, we put 2500 Hz, 4000Hz and 44100 Hz respectively.After designing this filter, we found by using the Matlab ‘’order’’ function that the the order of this filter is 23.The next step was to determine the number of multiplication operations per input sample required to implement the filter. In order to do this, we used the Ma tlab ‘’cost’’ function and found that the number of multiplication operations per input sample required to implement the filter is 46.We also had to plot the magnitude response of this filter in dB and in linear scale along with the group delay. Figure 1 shows the corresponding results.Figure 1 – Magnitude and group delay of the Butterworth filterAfter plotting the magnitude and group delay of this filter, we had to plot the pole-zero diagram. Figure 2 shows the corresponding result.Figure 2 – Pole-zero plot of the Butterworth filterAnother important aspect of the filter that we have to consider is the impulse response. Using the Matlab ‘’filter’’ and ‘’stem’’ functions, we plotted the impulse response if this filter. Figure 3 shows the corresponding result.Figure 3 – Impulse response of the Butterworth filterFinally, we filtered the noisy signal with this filter and noticed that the noise was almost completely removed and that we can listen clearly to the speech without any noise. However, the filtered signal was not strong enough and needed to be amplified en order for the sound to be loud enough. So we amplified the filtered signal by a factor of 100 and noticed that the speech was loud enough and very clear.Part III: Chebyshev Type I filterIn this part, we had to design a Chebyshev Type I filter that satisfies the given specifications. We used Matlab fdatool to design this filter. In order to use this Matlab built-in tool, we had to convert the above given specifications into IIR filter specifications. Thus, we took the same parameters as we used with the Butterworth filter:Apass = Gpbmax – Gpbmin = 40 – 37 = 3dBAstop = Gpbmax – Gsbmax = 40 -(- 55) = 95dBAs for Fpass, Fstop and Fs, we put 2500 Hz, 4000Hz and 44100 Hz respectively.After designing this filter, we found by using the Matlab ‘’order’’ function that the the order of this filter is 11.The next step was to determine the number of multiplication operations per input sample required to implement the filter. In order to do this, we used the Matlab ‘’cost’’ function and found that the number of multiplication operations per input sample required to implement the filter is 22.We also had to plot the magnitude response of this filter in dB and in linear scale along with the group delay. Figure 4 shows the corresponding results.Figure 4 – Magnitude and group delay of the Chebyshev Type I filterAfter plotting the magnitude and group delay of this filter, we had to plot the pole-zero diagram. Figure 5 shows the corresponding result.Figure 5 – Pole-zero plot of the Chebyshev Type I filterAnother important aspect of the filter that we have to consider is the impulse response. Using the Matlab ‘’filter’’ and ‘’stem’’ functions, we plotted the impulse response if this filter. Figure 6 shows the corresponding result.Figure 6 – Impulse response of the Chebyshev Type I filterFinally, we filtered the noisy signal with this filter and noticed that the noise was almost completely removed and that we can listen clearly to the speech without any noise. However, the filtered signal was not strong enough and needed to be amplified en order for the sound to be loud enough. So we amplified the filtered signal by a factor of 100 and noticed that the speech was loud enough and very clear.Part IV: Chebyshev Type II filterIn this part, we had to design a Chebyshev Type II filter that satisfies the given specifications. We used Matlab fdatool to design this filter. In order to use this Matlab built-in tool, we had to convert the above given specifications into IIR filter specifications. Thus, we took the same parameters as we used with the Butterworth filter:Apass = Gpbmax – Gpbmin = 40 – 37 = 3dBAstop = Gpbmax – Gsbmax = 40 -(- 55) = 95dBAs for Fpass, Fstop and Fs, we put 2500 Hz, 4000Hz and 44100 Hz respectively.After designing this filter, we found by using the Matlab ‘’order’’ function that the the order of this filter is 11.The next step was to determine the number of multiplication operations per input sample required to implement the filter. In order to do this, we used the Matlab ‘’cost’’ function and found that the number of multiplication operations per input sample required to implement the filter is 22.We also had to plot the magnitude response of this filter in dB and in linear scale along with the group delay. Figure 7 shows the corresponding results.Figure 7– Magnitude and group delay of the Chebyshev Type II filterAfter plotting the magnitude and group delay of this filter, we had to plot the pole-zero diagram. Figure 8 shows the corresponding result.Figure 8 – Pole-zero plot of the Chebyshev Type II filterAnother important aspect of the filter that we have to consider is the impulse response. Using the Matlab ‘’filter’’ and ‘’stem’’ functions, we plotted the impulse response if this filter. Figure 9 shows the corresponding result.Figure 9 – Impulse response of the Chebyshev Type II filterFinally, we filtered the noisy signal with this filter and noticed that the noise was almost completely removed and that we can listen clearly to the speech without any noise. However, the filtered signal was not strong enough and needed to be amplified en order for the sound to be loud enough. So we amplified the filtered signal by a factor of 100 and noticed that the speech was loud enough and very clear.Part V: Elliptic filterIn this part, we had to design an Elliptic filter that satisfies the given specifications. We used Matlab fdatool to design this filter. In order to use this Matlab built-in tool, we had to convert the above given specifications into IIR filter specifications. Thus, we took the same parameters as we used with the Butterworth filter:Apass = Gpbmax – Gpbmin = 40 – 37 = 3dBAstop = Gpbmax – Gsbmax = 40 -(- 55) = 95dBAs for Fpass, Fstop and Fs, we put 2500 Hz, 4000Hz and 44100 Hz respectively.After designing this filter, we found by using the Matlab ‘’order’’ function that the the order of this filter is 8.The next step was to determine the number of multiplication operations per input sample required to implement the filter. In order to do this, we used the Matlab ‘’cost’’ function and found that the number of multiplication operations per input sample required to implement the filter is 16.We also had to plot the magnitude response of this filter in dB and in linear scale along with the group delay. Figure 10 shows the corresponding results.Figure 10– Magnitude and group delay of the Elliptic filterAfter plotting the magnitude and group delay of this filter, we had to plot the pole-zero diagram. Figure 11 shows the corresponding result.Figure 11 – Pole-zero plot of the Elliptic filterAnother important aspect of the filter that we have to consider is the impulse response. Using the Matlab ‘’filter’’ and ‘’stem’’ functions, we plotted the impulse response if this filter. Figure 12 shows the corresponding result.Figure 12 – Impulse response of the Elliptic filterFinally, we filtered the noisy signal with this filter and noticed that the noise was almost completely removed and that we can listen clearly to the speech without any noise. However, the filtered signal was not strong enough and needed to be amplified en order for the sound to be loud enough. So we amplified the filtered signal by a factor of 100 and noticed that the speech was loud enough and very clear.ConclusionThe purpose of this experiment was to verify a conjecture that states that the ear is insensitive to phase. In order to do this, we had to implement all-pass filters that would keep the same magnitude of the frequency response of the audio signal but will introduce a phase shift.By doing this, we had the opportunity to better understand some concepts relating to all pass filters. We also saw the behaviour of these filters when we have an impulse input. Also, we plotted the magnitude, the group delay and the pole-zero plot of the all-pass filters in order tostudy the magnitude of the frequency response and the phase introduced by an all pass filter. We also had the opportunity to see the relation that exists between the poles and zeros in an all pass filters and noticed that the poles and zeros are at conjugate reciprocal locations. Finally, we concluded that the ear is insensitive to phase shift. However, for very big phase shifts, the ear can detect some distortions in the sound.AppndiceMain MATLAB codeload projIA.mat%Part a)%Impulse responsefigure(1)impz(b,a,100)%Magnitudefigure(2)[H,W]=freqz(b,a)plot(W,20*log10(abs(H)))xlabel('Frequency in rad/s');ylabel('Magnitude in DB');title('Magnitude Response of the all-pass filter with N=1')%Group Delayfigure(3)grpdelay(b,a)title('Group delay of the all-pass filter with N=1')%Part b)%Pole-zero plotfigure(4)zplane(b,a)title('Pole-zero plot of the all-pass filter with N=1')%Part c)%Listening to soundy = filter(b,a,speech)sound(y,fs)%Part d)%Implementing the filter with N=50n = [0:5000];h2 = [n == 0]% First, we define h2 as an impulse input signalfor (n=1:50)h2 = filter(b,a,h2); %We filter the impulse input with the all pass filter with N=1 50 timesend%Impulse responsefigure (5)stem(h2)title('Impulse response');xlabel('n samples');ylabel('Amplitude')%Magnitudefigure(6)[H,W]=freqz(b,a)plot(W,(20*log10((abs(H)).^50)))xlabel('Frequency in rad/s');ylabel('Magnitude in DB');title('Magnitude Response of the all-pass filter with N=50')%Group Delayfigure(7)plot(W/pi,50.*grpdelay(b,a))xlabel('Normalized frequency (x pi rad/sample)');ylabel('Group delay (samples)');title('Group Delay of the all-pass filter with N=50')grid%Listening to soundy2 = filter(b,a,speech)for(n=1:49)y2=filter(b,a,y2);endsound(y2,fs)。