AnomAlert软件手册
NOXMAT 700 CLD NO NOx数字分析器说明书
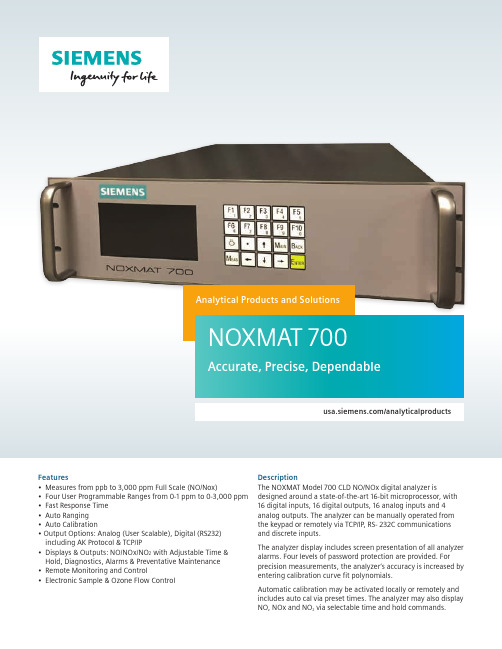
Features• Measures from ppb to 3,000 ppm Full Scale (NO/Nox)• Four User Programmable Ranges from 0-1 ppm to 0-3,000 ppm•FastResponseTime•AutoRanging•AutoCalibration • Output Options: Analog (User Scalable), Digital (RS232)including AK Protocol & TCP/IP• Displays & Outputs: NO/NOx/NO2 with Adjustable Time &Hold, Diagnostics, Alarms & Preventative Maintenance• Remote Monitoring and Control• Electronic Sample & Ozone Flow Control /analyticalproductsAnalytical Products and SolutionsNOXMAT 700Accurate, Precise, DependableDescriptionThe NOXMAT Model 700 CLD NO/NOx digital analyzer isdesigned around a state-of-the-art 16-bit microprocessor, with16 digital inputs, 16 digital outputs, 16 analog inputs and 4analog outputs. The analyzer can be manually operated fromthe keypad or remotely via TCP/IP, RS- 232C communicationsand discrete inputs.The analyzer display includes screen presentation of all analyzeralarms. Four levels of password protection are provided. Forprecision measurements, the analyzer’s accuracy is increased byentering calibration curve fit polynomials.Automatic calibration may be activated locally or remotely andincludes auto cal via preset times. The analyzer may also displayNO, NOx and NO2 via selectable time and hold commands.For more information, please contact:Siemens Industry, Inc.5980 West Sam Houston Parkway North Suite 500Houston, TX 77041Phone: 713-939-7400Email:*************************************************************Method of OperationThe NOXMAT 700 CLD Analyzer utilizes the principle ofchemiluminescence for analyzing the NO or NOx concentration within a gaseous sample. In the NO mode, the method is based upon the chemiluminescent reaction between ozone and nitric oxide (NO) yielding nitrogen dioxide (NO 2) and oxygen. This reaction produces light which has an intensity proportional to the mass flow rate of NO into the reaction chamber. The light is measured by means of a photodiode and associatedamplification electronics. In the NOx mode, NO plus NO 2 is determined as above, however, the sample is first routed through the internal NO 2 in the sample to NO. The resultant reaction is then directly proportional to the total concentration of NOx. Local operation is simplified using the 20 button alphanumeric keypad with data presented on a back lit LCD display. All local operations may be performed remote via RS-232 and/or TCP/IP.Applications • Stack Gases (C E M) • Scrubber E fficiency • Turbine/Generator Feedback Control • Process Chemical Gas Analysis • Personnel Safety • Power Plant Stack De-Nitrification • Vehicle EmissionsSpecificationsDetector: Chemiluminescence (CLD) Photodiode (thermally stabilized with Peltier Cooler) NO/NOx RANGES: 0-1 * to 3,000 ppm NO or NOx (Four user programmable ranges) (Higher ranges available upon request)Response Time: T90 < 2 Seconds to 60 Seconds Adjustable Resolution: 10 ppb NO/NOx (Displays 5 significant digits)Repeatability: Better than 0.5% of Full Scale Linearity: Better than 0.5% of Full ScaleNoise*: Less than 1 % of Full Scale (*1.5% @ 0-1 ppm Full Scale)Zero & Span Drift: Less than 1 % of Full Scale per 24 Hours Zero & Span Adjustment: Via front panel, TCP/IP or RS-232NH 3, HCN & SO 2 Effect: Not detectable with 100 ppm CO 2 Effect: Less than 1 % with 10% CO 2H 2O Effect: Less than 1 % with 1 % H 2OSample Gas Pressure: 8-25 psig (Standard model)Sample Flow Rate: 1.5 to 2.0 LPM (Consult factory for other flow rates)Converter:****************************>98%efficiency Ozonator: Ultraviolet LampAir Or O 2 Requirements: Less than 0.01 ppm NOx at 350 cc/Min. @ 25 psig (Dew Point < -35°C)NO/NOx Control: Manual/Remote/Auto Cycle (Remote NOx mode by dry contact closure)Outputs Available: TCP/IP, RS232, Four Scalable Analog 0- 10 V/4-20 mADiscrete Alarms: (Local & Remote Adjustable) General Fault! TTL Logic (Ground True) Calibration Failure/ TTL Logic (Ground True) High Concentration (2 each)/ TTL Logic (Ground True)Digital Diagnostics: Control Voltages, Temperatures, Pressures, Flow ParametersKeypad Displays: Factory Settings,TCP/IP Address, Passwords (4), Scalable Analog Output Voltages, Full Scale Range Select, Auto Cal Times Special Features: Calculated NO 2 derived from NOx converter efficiency, Auto Ranging, Auto Calibration (adjustable through internal clock) Less than 3 cc Gold Plated Reaction Chamber Display: 3” x 5” Back lit LCD Sample Temperature: Up to 50.C Noncondensing Ambient Temperature: 5 to 40.CAmbient Humidity: Less than 90% RH Noncondensing Warm-Up Time: 1 Hour (Typical)Fittings: 1/4 Inch TubePower Requirements: 115/230 (:t100;0) VAC; 50/60 Hz; 200 Watts (350 watts with pump)Dimensions: 5 1/4 H x 19 W x 17 D (Inches) - short case Weight: 45 Pounds。
PyroMAT 系统用户指南说明书
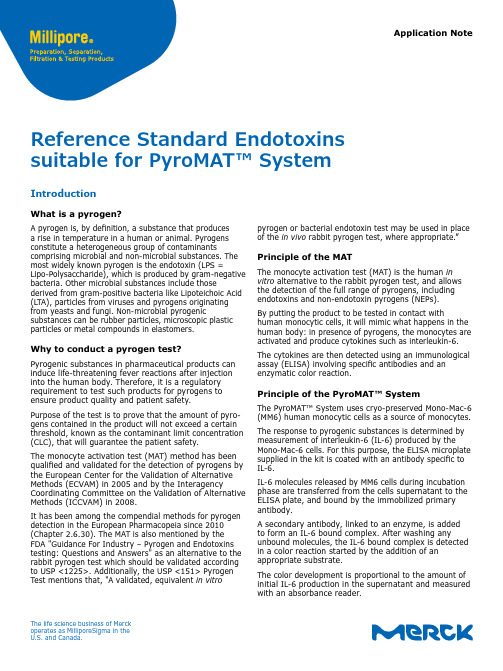
Application Notepyrogen or bacterial endotoxin test may be used in place of the in vivo rabbit pyrogen test, where appropriate.”Principle of the MATThe monocyte activation test (MAT) is the human in vitro alternative to the rabbit pyrogen test, and allows the detection of the full range of pyrogens, including endotoxins and non-endotoxin pyrogens (NEPs).By putting the product to be tested in contact withhuman monocytic cells, it will mimic what happens in the human body: in presence of pyrogens, the monocytes are activated and produce cytokines such as interleukin-6.The cytokines are then detected using an immunological assay (ELISA) involving specific antibodies and an enzymatic color reaction.Principle of the PyroMAT™ SystemThe PyroMAT™ System uses cryo-preserved Mono-Mac-6 (MM6) human monocytic cells as a source of monocytes. The response to pyrogenic substances is determined by measurement of interleukin-6 (IL-6) produced by the Mono-Mac-6 cells. For this purpose, the ELISA microplate supplied in the kit is coated with an antibody specific to IL-6.IL-6 molecules released by MM6 cells during incubation phase are transferred from the cells supernatant to the ELISA plate, and bound by the immobilized primary antibody.A secondary antibody, linked to an enzyme, is added to form an IL-6 bound complex. After washing anyunbound molecules, the IL-6 bound complex is detected in a color reaction started by the addition of an appropriate substrate.The color development is proportional to the amount of initial IL-6 production in the supernatant and measured with an absorbance reader.Reference Standard Endotoxins suitable for PyroMAT™ SystemIntroductionWhat is a pyrogen?A pyrogen is, by definition, a substance that produces a rise in temperature in a human or animal. Pyrogens constitute a heterogeneous group of contaminantscomprising microbial and non-microbial substances. The most widely known pyrogen is the endotoxin (LPS =Lipo-Polysaccharide), which is produced by gram-negative bacteria. Other microbial substances include thosederived from gram-positive bacteria like Lipoteichoic Acid (LTA), particles from viruses and pyrogens originating from yeasts and fungi. Non-microbial pyrogenicsubstances can be rubber particles, microscopic plastic particles or metal compounds in elastomers.Why to conduct a pyrogen test?Pyrogenic substances in pharmaceutical products can induce life-threatening fever reactions after injection into the human body. Therefore, it is a regulatory requirement to test such products for pyrogens to ensure product quality and patient safety.Purpose of the test is to prove that the amount of pyro-gens contained in the product will not exceed a certain threshold, known as the contaminant limit concentration (CLC), that will guarantee the patient safety.The monocyte activation test (MAT) method has been qualified and validated for the detection of pyrogens by the European Center for the Validation of Alternative Methods (ECVAM) in 2005 and by the InteragencyCoordinating Committee on the Validation of Alternative Methods (ICCVAM) in 2008.It has been among the compendial methods for pyrogen detection in the European Pharmacopeia since 2010 (Chapter 2.6.30). The MAT is also mentioned by the FDA "Guidance For Industry – Pyrogen and Endotoxins testing: Questions and Answers" as an alternative to the rabbit pyrogen test which should be validated according to USP <1225>. Additionally, the USP <151> Pyrogen Test mentions that, "A validated, equivalent in vitroThe life science business of Merck operates as MilliporeSigma in the U.S. and Canada.2Resuspension of RSELyophilized RSE were reconstituted and aliquoted according to supplier guidelines.Dilution of Reference Standard Endotoxin aliquotsThe standard endotoxin solutions were prepared from the RSE stock solution at 2000 EU/mL. Seven (7) endotoxin concentrations (0.0125, 0.025, 0.05, 0.1,0.2, 0.4, and 0.8 EU/mL) were prepared to generate the standard curve according to the following procedure:• Thaw a 50 µL-aliquot of RSE and vortex at maximum speed during 1 min.• Perform serial dilutions in endotoxin-free water, using endotoxin-free glass tubes, as described below. Make sure to vortex all the dilutions before using.Comparison of Reference Standard Endo-toxins (RSE) from different suppliersPreparation of endotoxin standard solutions is needed to assess the limit of detection (LOD) of the system, to build a standard curve for quantification or to estimate the pyrogen content of a sample, depending on the MAT method used.The use of a validated Reference Standard Endotoxin is required and such a standard can be supplied by the European Pharmacopeia (EDQM) or the United States Pharmacopeia (USP).Control Standard Endotoxins (CSE) provided by LAL suppliers should not be used for MAT test.The reference standard endotoxin (RSE) supplied by the USP / EDQM is a reference endotoxin preparation with a certified activity upon reconstitution. Control standard endotoxin preparations (CSEs) are qualified using the RSE, but their activity is certified only in combination with a test system, e.g. a defined preparation of limulus amoebocyte lysate used for the bacterial endotoxin test. Using these CSEs outside their test system might lead to unexpected results and is not recommended by the respective suppliers. As there is currently no dedicated reference standard for the pyrogen test available,standardization is achieved using a strong pyrogen like the RSE whose production and standardization is not depending on the use of a specific bacterial endotoxin test.The scope of this application note is to show the suitability of Reference Standard Endotoxins from different suppliers (USP/EDQM) for MAT with the PyroMAT™ System.PyroMAT™ Kit Pyr0MATkit PyroMAT™ Cells Pyr0MATcellsPyroMAT™ Endotoxin StandardEuropean Pharmacopoeia (EP) Reference Standard Endotoxin1.44161.0001Sigma-Aldrich RSEEuropean Pharmacopoeia (EP) Reference Standard EndotoxinE0150000ACILA RSE USP Reference Standard Endotoxin (USP) 10.000 E.U./Fl1220200NIBSC RSE 3rd International Standard 10,000 USP Endotoxin units Replacement I.S. for 94/58010/178Table 1: Materials used to generate standard curvesMaterialsFigure 2:Materials used to generate standard curves3MAT quick procedure with PyroMAT™Step 1: Preparation and incubation with PyroMAT™ cellsStep 2: Detection of IL-6 with ELISA1• Prepare suitable endotoxin standard dilutions• Load the different solutions on the 96-wells cell culture plate • Prepare the PyroMAT™ cells and dispense in each well2• Incubate the plate for 22 ±2 hours at 37 °C with humidified atmosphere, without CO 23• Transfer the cell supernatants into IL-6 microplate• Add the IL-6 conjugate to each well • Incubate 2 hours at room temperature 4• Remove the liquid and wash the plate 4 times• Prepare the substrate solution by mixing color reagent A and B and add the mixture to each well • Incubate 30 minutes at room temperature, in the dark5• Add the stop solution6• Read the plate at 450 nm and 630 nm within 30 minutes after adding the stop solutionFigure 3: PyroMAT TMworkflow with standard ELISA procedureResultsEndotoxin standard curves were generated using RSE from different suppliers. To be considered “VALID”, the endotoxin standard curve must fulfill the following acceptance criteria described in the EP Chapter 2.6.30 : Effect of dose criteria: a statistical test thatconfirms a positive dose/effect response.Goodness of fit: a statistical test that confirms the suitability of the regression model to describe the raw data. The data are modeled with a 5-parameter logistics regression model.Blank criteria: the mean of blank OD value should be below 0.1.LOD criteria: the test is valid if an LOD ≤ 0.05 EU/mL is reached.An additional criterion was implemented in the protocol to assess the reactivity of the standard curve:Minimum of reactivity: OD of the 4 replicates of the highest standard (0.8 EU/mL) should be above 3.It is not required by the European pharmacopeia and is given as an additional indication for the customer.Data analysis was performed using the PyroMAT™data analysis tool, which consists of a specific protocol developed for PyroMAT™ using Gen5 Software (Biotek).The Figure 4 presents the curves that were obtained with the PyroMAT™ system using Reference Standard Endotoxins (RSE) from four different suppliers as described in table 1.Figure 4: Comparison of standard curves generated with various Reference Standard EndotoxinsThe validity of the acceptance criteria for the endotoxin standard curve was determined using the PyroMAT™ data analysis tool (protocol for Gen5 Software).The Figure 5 shows the results obtained and the legend used in the software for data interpretation:All standard curves generated with RSE from different suppliers passed the acceptance criteria of a valid standard curve according to the EP chapter 2.6.30.ConclusionAll four different Reference Standard Endotoxins (RSE) tested led to the generation of a valid standard curve and can be used to perform MAT with PyroMAT™ system.MaterialEffect of close Goodness of Fit Blank Delta OD LOD criteria Minimum of reactivityPyroMAT™ Endotoxin Standard VALID VALID VALID VALID CONFORM Sigma-Aldrich RSE VALID VALID VALID VALID CONFORM ACILA RSE VALID VALID VALID VALID CONFORM NIBSC RSEVALIDVALIDVALIDVALIDCONFORMLegendEffect of Dose Criteria: VALID: p<0.01 LOD Criteria VALID: LOD ≤ 0.05 EU/mLINVALID: p≥0.01INVALID:LOD > 0.05 EU/mLGoodness of Fit Criteria: VALID: p>0.05 Additional Criteria – Minimum of reactivity CONFORM: All replicates of Delta OD at STD7 are above 3INVALID: p≤0.05 NOT REACHED: At least one replicate of Delta OD at STD7 is below 3BLK Delta OD Criteria: VALID: MEAN(BLK)<0.1 : Unable to EvaluateINVALID: MEAN(BLK)≥0.1Figure 5: Acceptance criteria for the endotoxin standard curves and legend for data interpretationTo place an order or receive technical assistanceIn Europe, please call Customer Service:France: 0825 045 645 Germany************ I taly: 848 845 645Spain: 901 516 645 Option 1Switzerland: 0848 645 645UnitedKingdom************For other countries across Europe, p lease call: +44 (0) 115 943 0840 Or visit: /officesFor Technical Service visit: /techservice /pyromatMerck, the vibrant M, Millipore and PyroMAT are trademarks of Merck KGaA or its afiliates. All other trademarks are the property of their respective owners. Detailed information on trademarks is available via publicly accessible resources.© 2018 Merck KGaA, Darmstadt, Germany and/or its affiliates. All Rights Reserved.MK_AN2150EN 2018-1255306/2018Merck KGaAFrankfurter Str. 250 64293 Darmstadt。
Minitab Statistical Software 入门说明书
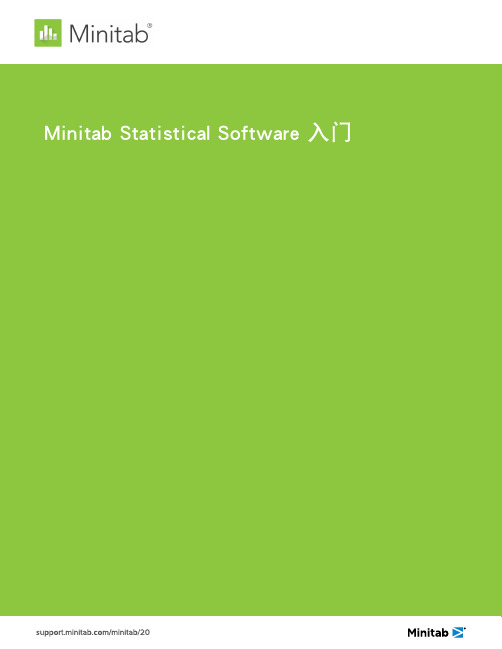
Minitab Statistical Software入门内容1简介4概述4案例4 Minitab用户界面4数据类型6打开并检查工作表6在下一章中72用图形表示数据8概述8创建、解释和编辑直方图8创建并解释散点图13在一个布局上排列多个图形16保存Minitab项目19在下一章中193分析数据20概述20对数据进行汇总摘要20比较两个或更多均值22保存项目28在下一章中284评估质量29概述29创建并解释控制图29创建并解释能力统计量33保存项目35在下一章中355设计实验36概述36创建设计的试验36查看设计39将数据输入到工作表40分析设计并解释结果40使用存储的模型进行其他分析44保存项目47在下一章中486重新执行分析49概述49使用会话命令执行分析49复制会话命令50使用exec文件重复进行分析51保存项目52在下一章中527导入和准备数据53概述53从不同来源导入数据53准备数据以进行分析55当数据值更改时57保存工作表57索引591.简介概述Minitab Statistical Software入门介绍了Minitab中一些最常用的功能和任务。
备注:本指南中某些功能仅在桌面应用中可用。
使用Web应用时在Windows计算机或Mac上,您可以打开桌面应用来访问Minitab提供的所有功能。
大部分统计分析都需要执行一系列步骤,这些步骤通常由背景知识或您要调查的主题领域来指导完成。
第2章到第5章介绍以下步骤:•利用图形研究数据并显示结果•进行统计分析•评估质量•设计试验在第6章和第7章中,您学习如何执行以下操作:•使用快捷方式自动执行将来的分析•将数据从不同文件类型导入到Minitab中,并准备数据以进行分析。
案例某家在网上销售图书的公司具有三个区域出货中心。
每个出货中心都用不同的计算机系统来输入和处理订单。
该公司想要确定最高效的计算机系统,并在每个出货中心使用该计算机系统。
在整个Minitab Statistical Software入门中,您学习了使用Minitab来分析来自出货中心的数据。
Amattrol T5554分析过程控制学习系统说明书

and instrumenta on diagrams before moving into more concentrated analy cal process control
topics like pH electrodes, pH meters and transmi ers,
and loop controllers. Within this curriculum, learners
searchable course content.
Expand Training with Optional Control Modules and Data Acquisition
The Advanced Analy cal Control Module (T5554-A2) adds to the T5554 to enable integra on with the other Amatrol process control learning system to form very sophis cated process control loops. The T5554 also requires a PID control, offering a choice between a single loop (T5554C1) or dual loop (T5554-C2). It also offers a 3-channel data acquisi on op on (T5554-R1-A).
more systems UƟliƟes:
Electricity (120 VAC/60 Hz/1 phase) Water
Hands-On Training Using Real Industrial Process Control Components
Anova Table说明

Anova TableSourcea | SSb dfc MSd -------------+------------------------------Model | 9543.72074 4 2385.93019Residual | 9963.77926 195 51.0963039 -------------+------------------------------Total | 19507.5 199 98.0276382a. Source - This is the source of variance, Model, Residual, and Total. The Total variance is partitioned into the variance which can be explained by the independent variables (Model) and the variance which is not explained by the independent variables (Residual, sometimes called Error). Note that the Sums of Squares for the Model and Residual add up to the Total Variance, reflecting the fact that the Total Variance is partitioned into Model and Residual variance.b. SS - These are the Sum of Squares associated with the three sources of variance, Total, Model and Residual. These can be computed in many ways. Conceptually, these formulas can be expressed as:SSTotal The total variability around the mean. S(Y - Ybar)2.SSResidual The sum of squared errors in prediction. S(Y - Ypredicted)2.SSModel The improvement in prediction by using the predicted value of Y over just using the mean of Y. Hence, this would be the squared differences between the predicted value of Y and the mean of Y, S(Ypredicted - Ybar)2. Another way to think of this is the SSModel is SSTotal - SSResidual. Note that the SSTotal = SSModel + SSResidual. Note that SSModel / SSTotal is equal to .4892, the value of R-Square. This is because R-Square is the proportion of the variance explained by the independent variables, hence can be computed by SSModel / SSTotal.c. df - These are the degrees of freedom associated with the sources of variance. The total variance has N-1 degrees of freedom. In this case, there were N=200 students, so the DF for total is 199. The model degrees of freedom corresponds to the number of predictors minus 1 (K-1). You may think this would be 4-1 (since there were 4 independent variables in the model, math, female, socst and read). But, the intercept is automatically included in the model (unless you explicitly omit the intercept). Including the intercept, there are 5 predictors, so the model has 5-1=4 degrees of freedom. The Residual degrees of freedom is the DF total minus the DF model, 199 - 4 is 195.d. MS - These are the Mean Squares, the Sum of Squares divided by their respective DF. For the Model, 9543.72074 / 4 = 2385.93019. For the Residual, 9963.77926 / 195 = 51.0963039. These are computed so you can compute the F ratio, dividing the Mean Square Model by the Mean Square Residual to test the significance of the predictors in the model.--------------------------------------------------------------------------------Overall Model FitNumber of obse = 200 F( 4, 195)f = 46.69 Prob > Ff = 0.0000 R-squaredg = 0.4892 Adj R-squaredh = 0.4788 Root MSEi = 7.1482e. Number of obs - This is the number of observations used in the regression analysis.f. F and Prob > F - The F-value is the Mean Square Model (2385.93019) divided by the Mean Square Residual (51.0963039), yielding F=46.69. The p-value associated with this F value is very small (0.0000). These values are used to answer the question "Do the independent variables reliably predict the dependent variable?". The p-value is compared to your alpha level (typically 0.05) and, if smaller, you can conclude "Yes, the independent variables reliably predict the dependent variable". You could say that the group of variables math and female can be used to reliably predict science (the dependent variable). If the p-value were greater than 0.05, you would say that the group of independent variables does not show a statistically significant relationship with the dependent variable, or that the group of independent variables does not reliably predict the dependent variable. Note that this is an overall significance test assessing whether the group of independent variables when used together reliably predict the dependent variable, and does not address the ability of any of the particular independent variables to predict the dependent variable. The ability of each individual independent variable to predict the dependent variable is addressed in the table below where each of the individual variables are listed.g. R-squared - R-Squared is the proportion of variance in the dependent variable (science) which can be predicted from the independent variables (math, female, socst and read). This value indicates that 48.92% of the variance in science scores can be predicted from the variables math, female, socst and read. Note that this is an overall measure of the strength of association, and does not reflect the extent to which any particular independent variable is associated with the dependent variable.h. Adj R-squared - Adjusted R-square. As predictors are added to the model, each predictor will explain some of the variance in the dependent variable simply due to chance. One could continue to add predictors to the model which would continue to improve the ability of the predictors to explain the dependent variable, although some of this increase in R-square would be simply due to chance variation in that particular sample. The adjusted R-square attempts to yield a more honest value to estimate the R-squared for the population. The value of R-square was .4892, while the value of Adjusted R-square was .4788 Adjusted R-squared is computed using the formula 1 - ((1 - Rsq)((N - 1) /( N - k - 1)). From this formula, you can see that when the number of observations is small and the number of predictors is large, there will be a much greater difference between R-square and adjusted R-square (because the ratio of (N - 1) / (N - k - 1) will be much greater than 1). By contrast, when the number of observations is very large compared to the number of predictors, the value of R-square and adjusted R-square will be much closer because the ratio of (N - 1)/(N - k - 1) will approach 1.i. Root MSE - Root MSE is the standard deviation of the error term, and is the square root of the Mean Square Residual (or Error).--------------------------------------------------------------------------------Parameter Estimates------------------------------------------------------------------------------ sciencej | Coef.k Std. Err.l tm P>|t|m [95% Conf. Interval]n -------------+---------------------------------------------------------------- math | .3893102 .0741243 5.25 0.000 .243122 .5354983 female | -2.009765 1.022717 -1.97 0.051 -4.026772 .0072428 socst | .0498443 .062232 0.80 0.424 -.0728899 .1725784 read | .3352998 .0727788 4.61 0.000 .1917651 .4788345 _cons | 12.32529 3.193557 3.86 0.000 6.026943 18.62364 ------------------------------------------------------------------------------j. science - This column shows the dependent variable at the top (science) with the predictor variables below it (math, female, socst, read and _cons). The last variable (_cons) represents the constant, also referred to in textbooks as the Y intercept, the height of the regression line when it crosses the Y axis. In other words, this is the predicted value of science when all other variables are 0.k. Coef. - These are the values for the regression equation for predicting the dependent variable from the independent variable. The regression equation is presented in many different ways, for example:Ypredicted = b0 + b1*x1 + b2*x2 + b3*x3 + b4*x4The column of estimates (coefficients or parameter estimates, from here on labeled coefficients) provides the values for b0, b1, b2, b3 and b4 for this equation. Expressed in terms of the variables used in this example, the regression equation issciencePredicted = 12.32529 + .3893102*math + -2.009765*female+.0498443*socst+.3352998*readThese estimates tell you about the relationship between the independent variables and the dependent variable. These estimates tell the amount of increase in science scores that would be predicted by a 1 unit increase in the predictor. Note: For the independent variables which are not significant, the coefficients are not significantly different from 0, which should be taken into account when interpreting the coefficients. (See the columns with the t-value and p-value about testing whether the coefficients are significant).math - The coefficient (parameter estimate) is .3893102. So, for every unit (i.e., point, since this is the metric in which the tests are measured) increase in math, a .3893102 unit increase in science is predicted, holding all other variables constant. (It does not matter at what value you hold the other variables constant, because it is a linear model.) Or, for every increase of one point on the math test, your science score is predicted to be higher by .3893102 points. This is significantly different from 0.female - For every unit increase in female, there is a -2.009765 unit decrease in the predicted science score, holding all other variables constant. Since female is coded 0/1 (0=male, 1=female) the interpretation can be put more simply. For females the predicted science score would be 2 points lower than for males. The variable female is technically not statistically significantly different from 0, because the p-value is greater than .05. However, .051 is so close to .05 that some researchers would still consider it to be statistically significant.socst - The coefficient for socst is .0498443. This means that for a 1-unit increase in thesocial studies score, we expect an approximately .05 point increase in the science score. This is not statistically significant; in other words, .0498443 is not different from 0.read - The coefficient for read is .3352998. Hence, for every unit increase in reading score we expect a .34 point increase in the science score. This is statistically significant.l. Std. Err. - These are the standard errors associated with the coefficients. The standard error is used for testing whether the parameter is significantly different from 0 by dividing the parameter estimate by the standard error to obtain a t-value (see the column with t-values and p-values). The standard errors can also be used to form a confidence interval for the parameter, as shown in the last two columns of this table.m. t and P>|t| - These columns provide the t-value and 2-tailed p-value used in testing the null hypothesis that the coefficient (parameter) is 0. If you use a 2-tailed test, then you would compare each p-value to your preselected value of alpha. Coefficients having p-values less than alpha are statistically significant. For example, if you chose alpha to be 0.05, coefficients having a p-value of 0.05 or less would be statistically significant (i.e., you can reject the null hypothesis and say that the coefficient is significantly different from 0). If you use a 1-tailed test (i.e., you predict that the parameter will go in a particular direction), then you can divide the p-value by 2 before comparing it to your preselected alpha level. With a 2-tailed test and alpha of 0.05, you may reject the null hypothesis that the coefficient for female is equal to 0. The coefficient of -2.009765 is significantly greater than 0. However, if you used a 2-tailed test and alpha of 0.01, the p-value of .0255 is greater than 0.01 and the coefficient for female would not be significant at the 0.01 level. Had you predicted that this coefficient would be positive (i.e., a one tail test), you would be able to divide the p-value by 2 before comparing it to alpha. This would yield a 1-tailed p-value of 0.00945, which is less than 0.01 and then you could conclude that this coefficient is greater than 0 with a one tailed alpha of 0.01.The coefficient for math is significantly different from 0 using alpha of 0.05 because its p-value is 0.000, which is smaller than 0.05.The coefficient for socst (.0498443) is not statistically significantly different from 0 because its p-value is definitely larger than 0.05.The coefficient for read (.3352998) is statistically significant because its p-value of 0.000 is less than .05.The constant (_cons) is significantly different from 0 at the 0.05 alpha level. However, having a significant intercept is seldom interesting.n. [95% Conf. Interval] - This shows a 95% confidence interval for the coefficient. This is very useful as it helps you understand how high and how low the actual population value of the parameter might be. The confidence intervals are related to the p-values such that the coefficient will not be statistically significant if the confidence interval includes 0. If you look at the confidence interval for female, you will see that it just includes 0 (-4 to .007). Because .007 is so close to 0, the p-value is close to .05. If the upper confidence level had been a little smaller, such that it did not include 0, the coefficient for female would have been statistically significant. Also, consider the coefficients for female (-2) and read (.34). Immediately you see that the estimate for female is so much bigger, but examine the confidence interval for it (-4 to .007). Now examinethe confidence interval for read (.19 to .48). Even though female has a bigger coefficient (in absolute terms) it could be as small as -4. By contrast, the lower confidence level for read is .19, which is still above 0. So, even though female has a bigger coefficient, read is significant and even the smallest value in the confidence interval is still higher than 0. The same cannot be said about the coefficient for socst. Such confidence intervals help you to put the estimate from the coefficient into perspective by seeing how much the value could vary.。
tabularaster 0.7.2 用户指南说明书
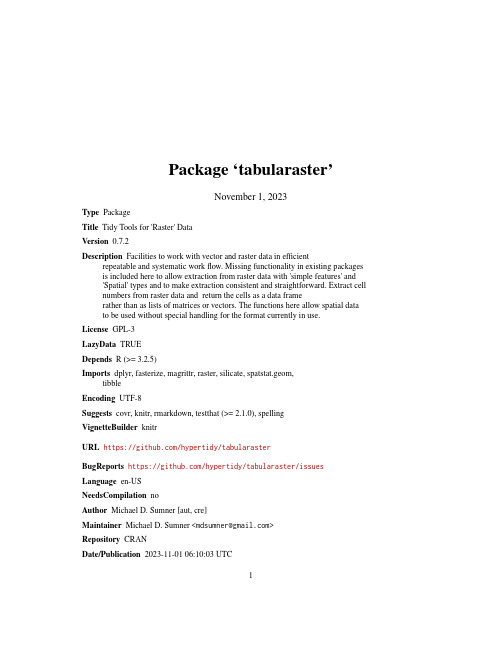
Package‘tabularaster’November1,2023Type PackageTitle Tidy Tools for'Raster'DataVersion0.7.2Description Facilities to work with vector and raster data in efficientrepeatable and systematic workflow.Missing functionality in existing packages is included here to allow extraction from raster data with'simple features'and'Spatial'types and to make extraction consistent and straightforward.Extract cell numbers from raster data and return the cells as a data framerather than as lists of matrices or vectors.The functions here allow spatial data to be used without special handling for the format currently in use.License GPL-3LazyData TRUEDepends R(>=3.2.5)Imports dplyr,fasterize,magrittr,raster,silicate,spatstat.geom,tibbleEncoding UTF-8Suggests covr,knitr,rmarkdown,testthat(>=2.1.0),spellingVignetteBuilder knitrURL https:///hypertidy/tabularasterBugReports https:///hypertidy/tabularaster/issues Language en-USNeedsCompilation noAuthor Michael D.Sumner[aut,cre]Maintainer Michael D.Sumner<******************>Repository CRANDate/Publication2023-11-0106:10:03UTC12as_tibble R topics documented:as_tibble (2)cellnumbers (3)decimate (5)ghrsst (5)index_extent (6)oisst (7)polycano (7)rastercano (7)sharkcano (8)tabularaster (8)Index10 as_tibble Convert a Raster to a data frame.DescriptionGenerate a data frame version of any raster e the arguments’cell’,’dim’,’split_date’and ’value’to control the columns that are included in the output.Usage##S3method for class BasicRasteras_tibble(x,cell=TRUE,dim=nlayers(x)>1L,value=TRUE,split_date=FALSE,xy=FALSE,...)Argumentsx a RasterLayer,RasterStack or RasterBrickcell logical to include explicit cell numberdim logical to include slice indexvalue logical to return the values as a column or notsplit_date logical to split date into componentsxy logical to include the x and y centre coordinate of each cell...unusedDetailsIf the raster has only one layer,the slice index is not e’dim=FALSE’to not include the slice index value.Valuea data frame(tibble)with columns:•cellvalue the actual value of the raster cell•cellindex the index of the cell(numbered from1to ncell()in the raster way).Columns cellindex or cellvalue may be omitted if either or both of cell and/or value are FALSE,respectivelyOther columns might be included depending on the properties of the raster and the arguments to the function:•year,month,day if split_date is TRUE•x,y if xy is TRUE•dimindex if the input has more than1layer and dim is TRUE.Examples##basic data frame version of a basic rasteras_tibble(raster::raster(volcano))##data frame with time column since raster has that setr<-raster::raster(volcano)br<-raster::brick(r,r)as_tibble(raster::setZ(br,Sys.Date()+1:2),cell=TRUE)cellnumbers Extract cell numbers from a Raster object.DescriptionProvide the’cellnumbers’capability of raster::extract and friends directly,returning a data frame of query-object identifiers’object_’and the cell number.Usagecellnumbers(x,query,...)##Default S3method:cellnumbers(x,query,...)##S3method for class SpatialLinescellnumbers(x,query,...)##S3method for class sfccellnumbers(x,query,...)##S3method for class sfcellnumbers(x,query,...)Argumentsx Raster objectquery Spatial object or matrix of coordinates...unusedDetailsRaster data is inherently2-dimensional,with a time or’level’dimension treated like a layers of these2D forms.The’raster’package cell number is counted from1at the top-left,across the rows and down.This corresponds the the standard"raster graphics"convention used by’GDAL’and the ’sp’package,and many other implementations.Note that this is different to the convention used by the graphics::image function.Currently this function only operates as if the input is a single layer objects,it’s not clear if adding an extra level of grouping for layers would be sensible.Valuea data frame(tibble)with columns•object_-the object ID(what row is it from the spatial object)•cell_-the cell number of the rasterExampleslibrary(raster)library(dplyr)r<-raster(volcano)%>%aggregate(fact=4)cellnumbers(r,rasterToContour(r,level=120))library(dplyr)cr<-cut(r,pretty(values(r)))suppressWarnings(tt<-cellnumbers(cr,polycano))library(dplyr)tt%>%mutate(v=extract(r,cell_))%>%group_by(object_)%>%summarize(mean(v))head(pretty(values(r)),-1)decimate5 decimate Decimate swiftly and ruthlesslyDescriptionReduce the resolution of a raster by ruthless decimation.Usagedecimate(x,dec=10)Argumentsx raster object(single layer).dec decimation factor,raw multiplier for the resolution of the outputDetailsThis is fast,it’s just fast extraction with no care taken for utility purposes when you need to reduce the detail.Valueraster layerExampleslibrary(raster)plot(decimate(raster(volcano)))contour(raster(volcano),add=TRUE)ghrsst Sea surface temperature data.DescriptionA smoothed subset of GHRSST.FormatA raster created GHRSST data and raster smoothing.DetailsSee"data-raw/ghrsst.R"and"data-raw/ghrsst-readme.txt"for details.sst_regions is a simple polygon region layer to sit over the SST data.6index_extent Exampleslibrary(raster)plot(ghrsst,col=hcl.colors(12,"YlOrRd",rev=TRUE))plot(sst_regions,add=TRUE,col=NA)cellnumbers(ghrsst,sst_regions)index_extent Index extentDescriptionExtent in index space.Usageindex_extent(x,ex)Argumentsx raster layerex extentDetailsConvert a geographic extent into purely index space.Valueextent objectExamples##the index extent is the rows/colsindex_extent(raster::raster(volcano),raster::extent(0,1,0,1))index_extent(raster::raster(volcano),raster::extent(0,1,0,.5))oisst7 oisst Optimally interpolated SST in near-native form.DescriptionSee data-raw/oisst.R in the source repository.Thefile was avhrr-only-v2.20170729.nc,its extent -180,180,-90,90with dimensions1440x720in the usual raster configuration.FormatA data frame of sst values created from OISST data.Examplesoisstpolycano The raster volcano as polygons.DescriptionSee data-raw/rastercano.r in the source repository.FormatA sp::SpatialPolygonsDataFrame with variables:volcano_elevation.Examplesexists("polycano")rastercano The raster volcano.DescriptionSee data-raw/rastercano.r in the source repository.FormatA raster created from the volcano data.Exampleslibrary(raster)plot(rastercano)sharkcano Sharkcano,the shark and the volcano.DescriptionThis is just a free image off the internet.The image was read in and all non-essential items dropped.The dimensions in raster::raster terms is stored in attr(sharkcano,"rasterdim").FormatA data frame with117843rows and2variables:cell_integer,cell indexbyte integer,byte value of shark image pixelsThese are cell values on a grid that is648x958.ReferencesThis is the small version from here,see script in data-raw/sharkcano.r /stockphoto/16214 Thanks to@jennybc for pointers onfinding free stuff:https:///jennybc/free-photosExampleslibrary(raster)rd<-attr(sharkcano,"rasterdim")rastershark<-raster(matrix(NA_integer_,rd[1],rd[2]))rastershark[sharkcano$cell_]<-sharkcano$byte##byte,heh##I present to you,Sharkcano!(Just wait for the3D version,Quadshark).plot(rastercano)contour(rastershark,add=TRUE,labels=FALSE)plot(rastershark,col="black")##another wayplot(rastercano)points(xyFromCell(rastershark,sharkcano$cell_),pch=".")tabularaster Tabular tools for rasterDescriptionExtract and index with raster tidy tools for raster.DetailsTabularaster includes these main functions.as_tibble convert raster data to data frame form,with control over output and form of dimension/coordinate columns cellnumbers extract a data frame of query identifiers and cell,pixel index numbersdecimate fast and loose resizing of a raster to coarser resolutionindex_extent build an extent in row column form,as opposed to coordinate value formIndexas_tibble,2,9cellnumbers,3,9decimate,5,9ghrsst,5graphics::image,4index_extent,6,9oisst,7polycano,7raster,5raster::extract,3rastercano,7sharkcano,8sst_regions(ghrsst),5tabularaster,8volcano,710。
Mata手册说明书
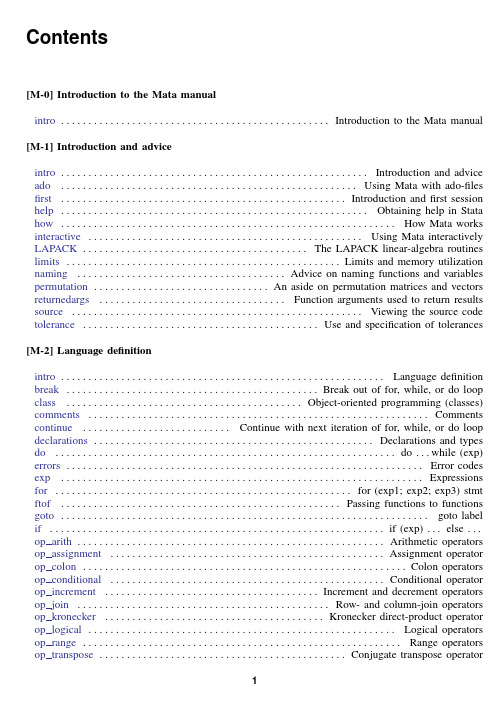
Contents[M-0]Introduction to the Mata manualintro.................................................Introduction to the Mata manual[M-1]Introduction and adviceintro........................................................Introduction and advice ing Mata with ado-files first....................................................Introduction andfirst session help........................................................Obtaining help in Stata how.............................................................How Mata works ing Mata interactively LAPACK.........................................The LAPACK linear-algebra routines limits..................................................Limits and memory utilization naming......................................Advice on naming functions and variables permutation................................An aside on permutation matrices and vectors returnedargs..................................Function arguments used to return results source.....................................................Viewing the source code e and specification of tolerances[M-2]Language definitionnguage definition break..............................................Break out of for,while,or do loop class...........................................Object-oriented programming(classes) ments continue...........................Continue with next iteration of for,while,or do loop declarations...................................................Declarations and types do..............................................................do...while(exp) errors.................................................................Error codes exp..................................................................Expressions for......................................................for(exp1;exp2;exp3)stmt ftof...................................................Passing functions to functions goto...................................................................goto label if.............................................................if(exp)...else... op arith........................................................Arithmetic operators op assignment..................................................Assignment operator op colon...........................................................Colon operators op conditional..................................................Conditional operator op increment.......................................Increment and decrement operators op join..............................................Row-and column-join operators op kronecker........................................Kronecker direct-product operator op logical........................................................Logical operators op range..........................................................Range operators op transpose.............................................Conjugate transpose operator12Contents optargs.........................................................Optional arguments pointers..................................................................Pointers pragma...............................................Suppressing warning messages reswords...........................................................Reserved words return........................................................return and return(exp) e of semicolons struct..................................................................Structures e of subscripts syntax............................................Mata language grammar and syntax version............................................................Version control void................................................................V oid matrices while.............................................................while(exp)stmt[M-3]Commands for controlling Matamands for controlling Mata end...................................................Exit Mata and return to Stata mata.....................................................Mata invocation command mata clear.....................................................Clear Mata’s memory mata describe.....................................Describe contents of Mata’s memory mata drop...................................................Drop matrix or function mata help......................................................Obtain help in Stata mata matsave..............................................Save and restore matrices mata memory.........................................Report on Mata’s memory usage mata mlib....................................................Create function library mata mosave................................Save function’s compiled code in objectfile mata rename..............................................Rename matrix or function mata set......................................Set and display Mata system parameters mata stata...................................................Execute Stata command mata which........................................................Identify function namelists........................................Specifying matrix and function names [M-4]Index and guide to functions intro....................................................Index and guide to functions io...................................................................I/O functions manipulation....................................................Matrix manipulation mathematical.........................................Important mathematical functions matrix............................................................Matrix functions programming................................................Programming functions scalar..................................................Scalar mathematical functions solvers................................Functions to solve AX=B and to obtain A inverse standard..........................................Functions to create standard matrices stata........................................................Stata interface functions statistical........................................................Statistical functions string..................................................String manipulation functions utility.......................................................Matrix utility functions [M-5]Mata functions intro...............................................................Mata functionsContents3 abbrev().........................................................Abbreviate strings abs().......................................................Absolute value(length) adosubdir().........................................Determine ado-subdirectory forfile all()..........................................................Element comparisons args()........................................................Number of arguments asarray().........................................................Associative arrays ascii().....................................................Manipulate ASCII codes assert().....................................................Abort execution if false blockdiag()...................................................Block-diagonal matrix bufio()........................................................Buffered(binary)I/O byteorder().............................................Byte order used by computer C()...............................................................Make complex c()...............................................................Access c()value callersversion()........................................Obtain version number of caller cat()....................................................Loadfile into string matrix chdir().......................................................Manipulate directories cholesky().........................................Cholesky square-root decomposition cholinv()...................................Symmetric,positive-definite matrix inversion cholsolve()............................Solve AX=B for X using Cholesky decomposition comb()binatorial function cond()...........................................................Condition number conj()plex conjugate corr()....................................Make correlation matrix from variance matrix cross().............................................................Cross products crossdev()..................................................Deviation cross products cvpermute().................................................Obtain all permutations date()...................................................Date and time manipulation deriv()........................................................Numerical derivatives designmatrix().....................................................Design matrices det()........................................................Determinant of matrix diag().................................................Replace diagonal of a matrix diag().......................................................Create diagonal matrix diag0cnt()..................................................Count zeros on diagonal diagonal().........................................Extract diagonal into column vector dir()....................................................................File list direxists()..................................................Whether directory exists direxternal().....................................Obtain list of existing external globals display().............................................Display text interpreting SMCL displayas()........................................................Set display level displayflush()............................................Flush terminal-output buffer Dmatrix().......................................................Duplication matrix docx*().......................................Generate Office Open XML(.docx)file dsign()............................................FORTRAN-like DSIGN()function e()...................................................................Unit vectors editmissing()..........................................Edit matrix for missing values edittoint()......................................Edit matrix for roundoff error(integers) edittozero().......................................Edit matrix for roundoff error(zeros) editvalue()............................................Edit(change)values in matrix eigensystem()............................................Eigenvectors and eigenvalues4Contentseigensystemselect()pute selected eigenvectors and eigenvalues eltype()..................................Element type and organizational type of object epsilon().......................................Unit roundoff error(machine precision) equilrc()..............................................Row and column equilibration error().........................................................Issue error message errprintf()..................................Format output and display as error message exit()..........................................................Terminate execution exp().................................................Exponentiation and logarithms factorial()..............................................Factorial and gamma function favorspeed()...................................Whether speed or space is to be favored ferrortext()......................................Text and return code offile error code fft().............................................................Fourier transform fileexists().......................................................Whetherfile exists fillmissing()..........................................Fill matrix with missing values findexternal().................................Find,create,and remove external globals findfile().................................................................Findfile floatround().................................................Round tofloat precision fmtwidth().........................................................Width of%fmt fopen()..................................................................File I/O fullsvd()............................................Full singular value decomposition geigensystem().................................Generalized eigenvectors and eigenvalues ghessenbergd()..................................Generalized Hessenberg decomposition ghk()...................Geweke–Hajivassiliou–Keane(GHK)multivariate normal simulator ghkfast().....................GHK multivariate normal simulator using pregenerated points gschurd()...........................................Generalized Schur decomposition halton().........................................Generate a Halton or Hammersley set hash1()...........................................Jenkins’one-at-a-time hash function hessenbergd()..............................................Hessenberg decomposition Hilbert()..........................................................Hilbert matrices I()................................................................Identity matrix inbase()...........................................................Base conversion indexnot()..................................................Find character not in list invorder()............................................Permutation vector manipulation invsym().............................................Symmetric real matrix inversion invtokens()...............................Concatenate string rowvector into string scalar isdiagonal()..............................................Whether matrix is diagonal isfleeting()...........................................Whether argument is temporary isreal()......................................................Storage type of matrix isrealvalues()..................................Whether matrix contains only real values issymmetric().................................Whether matrix is symmetric(Hermitian) isview()....................................................Whether matrix is view J().............................................................Matrix of constants Kmatrix()mutation matrix lapack()PACK linear-algebra functions liststruct()...................................................List structure’s contents Lmatrix().......................................................Elimination matrix logit()...........................................Log odds and complementary log-logContents5 lowertriangle().........................................Extract lower or upper triangle lud()...........................................................LU decomposition luinv()......................................................Square matrix inversion lusolve()...................................Solve AX=B for X using LU decomposition makesymmetric().............................Make square matrix symmetric(Hermitian) matexpsym().......................Exponentiation and logarithms of symmetric matrices matpowersym().........................................Powers of a symmetric matrix mean()............................................Means,variances,and correlations mindouble().................................Minimum and maximum nonmissing value minindex().......................................Indices of minimums and maximums minmax().................................................Minimums and maximums missing().......................................Count missing and nonmissing values missingof()................................................Appropriate missing value mod()..................................................................Modulus moptimize().....................................................Model optimization more().....................................................Create–more–condition negate().......................................................Negate real matrix norm()....................................................Matrix and vector norms normal()................................Cumulatives,reverse cumulatives,and densities optimize().....................................................Function optimization panelsetup()...................................................Panel-data processing pathjoin()....................................................File path manipulation pinv().................................................Moore–Penrose pseudoinverse polyeval()........................................Manipulate and evaluate polynomials printf().............................................................Format output qrd()...........................................................QR decomposition qrinv().............................Generalized inverse of matrix via QR decomposition qrsolve()...................................Solve AX=B for X using QR decomposition quadcross().............................................Quad-precision cross products range()..................................................Vector over specified range rank().............................................................Rank of matrix Re()..................................................Extract real or imaginary part reldif()..................................................Relative/absolute difference rows()........................................Number of rows and number of columns rowshape().........................................................Reshape matrix runiform()..............................Uniform and nonuniform pseudorandom variates runningsum().................................................Running sum of vector schurd()......................................................Schur decomposition select()..............................................Select rows,columns,or indices setbreakintr()..................................................Break-key processing sign()...........................................Sign and complex quadrant functions sin()..........................................Trigonometric and hyperbolic functions sizeof().........................................Number of bytes consumed by object solve tol().....................................Tolerance used by solvers and inverters solvelower()..........................................Solve AX=B for X,A triangular solvenl().........................................Solve systems of nonlinear equations sort().......................................................Reorder rows of matrix6Contentssoundex().............................................Convert string to soundex code spline3()..................................................Cubic spline interpolation sqrt().................................................................Square root st addobs()....................................Add observations to current Stata dataset st addvar().......................................Add variable to current Stata dataset st data()...........................................Load copy of current Stata dataset st dir()..................................................Obtain list of Stata objects st dropvar()...........................................Drop variables or observations st global()........................Obtain strings from and put strings into global macros st isfmt()......................................................Whether valid%fmt st isname()................................................Whether valid Stata name st local()..........................Obtain strings from and put strings into Stata macros st macroexpand().......................................Expand Stata macros in string st matrix().............................................Obtain and put Stata matrices st numscalar().......................Obtain values from and put values into Stata scalars st nvar()........................................Numbers of variables and observations st rclear().....................................................Clear r(),e(),or s() st store().................................Modify values stored in current Stata dataset st subview()..................................................Make view from view st tempname()...............................................Temporary Stata names st tsrevar().....................................Create time-series op.varname variables st updata()....................................Determine or set data-have-changedflag st varformat().................................Obtain/set format,etc.,of Stata variable st varindex()...............................Obtain variable indices from variable names st varname()...............................Obtain variable names from variable indices st varrename()................................................Rename Stata variable st vartype()............................................Storage type of Stata variable st view()..........................Make matrix that is a view onto current Stata dataset st viewvars().......................................Variables and observations of view st vlexists()e and manipulate value labels stata()......................................................Execute Stata command stataversion().............................................Version of Stata being used strdup()..........................................................String duplication strlen()...........................................................Length of string strmatch()....................................Determine whether string matches pattern strofreal().....................................................Convert real to string strpos().....................................................Find substring in string strreverse()..........................................................Reverse string strtoname()...........................................Convert a string to a Stata name strtoreal().....................................................Convert string to real strtrim()...........................................................Remove blanks strupper()......................................Convert string to uppercase(lowercase) subinstr()...........................................................Substitute text sublowertriangle()...........................Return a matrix with zeros above a diagonal substr()......................................................Substitute into string substr()...........................................................Extract substring sum().....................................................................Sums svd()..................................................Singular value decomposition svsolve()..........................Solve AX=B for X using singular value decomposition swap()..............................................Interchange contents of variablesContents7 Toeplitz().........................................................Toeplitz matrices tokenget()........................................................Advanced parsing tokens()..................................................Obtain tokens from string trace().......................................................Trace of square matrix transpose()..................................................Transposition in place transposeonly().......................................Transposition without conjugation trunc()............................................................Round to integeruniqrows()..............................................Obtain sorted,unique values unitcircle()plex vector containing unit circle unlink().................................................................Erasefile valofexternal().........................................Obtain value of external global Vandermonde()................................................Vandermonde matrices vec().........................................................Stack matrix columns xl()............................................................Excelfile I/O class[M-6]Mata glossary of common terms Glossary........................................................................ Subject and author index...........................................................。
slanter软件包说明说明书

Package‘slanter’October14,2022Version0.2-0Date2021-05-09Title Slanted Matrices and Ordered ClusteringDescription Slanted matrices and ordered clustering for better visualization of similarity data. RoxygenNote7.0.2Encoding UTF-8License MIT+file LICENSEImports Matrix,pheatmap,pracma,statsLazyData trueSuggests knitr,rmarkdownVignetteBuilder knitrNeedsCompilation noAuthor Oren Ben-Kiki[aut,cre],Weizmann Institute of Science[cph]Maintainer Oren Ben-Kiki<*****************>Depends R(>=2.10)Repository CRANDate/Publication2021-05-0908:10:02UTCR topics documented:meristems (2)oclust (2)reorder_frame (3)reorder_hclust (4)sheatmap (4)slanted_orders (7)slanted_reorder (8)Index1012oclustmeristems Sample RNA data of similarity between batches of1000cells of tomatomeristem cells.DescriptionThis is a simple matrix where each entry is the similarity(correlation)between a pair of batches.Negative correlations were changed to zero to simplify the analysis.Usagedata(meristems)FormatA simple square matrix.Examplesdata(meristems)similarity<-meristemssimilarity[similarity<0]=0slanter::sheatmap(meristems,order_data=similarity,show_rownames=FALSE,show_colnames=FALSE) oclust Hierarchically cluster ordered data.DescriptionGiven a distance matrix for sorted objects,compute a hierarchical clustering preserving this order.That is,this is similar to hclust with the constraint that the result’s order is always1:N.Usageoclust(distances,method="ward.D2",order=NULL,members=NULL)Argumentsdistances A distances object(as created by stats::dist).method The clustering method to use(only ward.D and ward.D2are supported).order If specified,assume the data will be re-ordered by this order.members Optionally,the number of members for each row/column of the distances(bydefault,one each).reorder_frame3 DetailsIf an order is specified,assumes that the data will be re-ordered by this order.That is,the indices in the returned hclust object will refer to the post-reorder data locations,**not**to the current data locations.This can be applied to the results of slanted_reorder,to give a"plausible"clustering for the data.ValueA clustering object(as created by hclust).Examplesclusters<-slanter::oclust(dist(mtcars),order=1:dim(mtcars)[1])clusters$orderreorder_frame Reorder the rows of a frame.DescriptionYou’d expect data[order,]to"just work".It doesn’t for data frames with a single column,which happens for annotation data,hence the need for this function.Sigh.Usagereorder_frame(frame,order)Argumentsframe A data frame to reorder the rows of.order An array containing indices permutation to apply to the rows.ValueThe data frame with the new row orders.Examplesdf<-data.frame(foo=c(1,2,3))df[c(1,3,2),]slanter::reorder_frame(df,c(1,3,2))reorder_hclust Given a clustering of some data,and some ideal order we’d like touse to visualize it,reorder(but do not modify)the clustering to be asconsistent as possible with this ideal order.DescriptionGiven a clustering of some data,and some ideal order we’d like to use to visualize it,reorder(but do not modify)the clustering to be as consistent as possible with this ideal order.Usagereorder_hclust(clusters,order)Argumentsclusters The existing clustering of the data.order The ideal order we’d like to see the data in.ValueA reordered clustering which is consistent,wherever possible,the ideal order.Examplesclusters<-hclust(dist(mtcars))clusters$orderclusters<-slanter::reorder_hclust(clusters,1:length(clusters$order))clusters$ordersheatmap Plot a heatmap with values as close to the diagonal as possible.DescriptionGiven a matrix expressing the cross-similarity between two(possibly different)sets of entities,this will reorder it to move the high values close to the diagonal,for a better visualization.Usagesheatmap(data,...,order_data=NULL,annotation_col=NULL,annotation_row=NULL,order_rows=TRUE,order_cols=TRUE,squared_order=TRUE,same_order=FALSE,patch_cols_order=NULL,patch_rows_order=NULL,discount_outliers=TRUE,cluster_rows=TRUE,cluster_cols=TRUE,oclust_rows=TRUE,oclust_cols=TRUE,clustering_distance_rows="euclidian",clustering_distance_cols="euclidian",clustering_method="ward.D2",clustering_callback=NA)Argumentsdata A rectangular matrix to plot,of non-negative values(unless order_data is spec-ified)....Additionalflags to pass to pheatmap.order_data An optional matrix of non-negative values of the same size to use for computing the orders.annotation_col Optional data frame describing each column.annotation_row Optional data frame describing each row.order_rows Whether to reorder the rows.Otherwise,use the current order.order_cols Whether to reorder the columns.Otherwise,use the current order.squared_order Whether to reorder to minimize the l2norm(otherwise minimizes the l1norm).same_order Whether to apply the same order to both rows and columns(if reordering both).For a square matrix,may also contain’row’or’column’to force the order ofone axis to apply to both.patch_cols_orderOptional function that may be applied to the columns order,returning a betterorder.patch_rows_orderOptional function that may be applied to the rows order,returning a better order.discount_outliersWhether to do afinal order phase discounting outlier values far from the diago-nal.cluster_rows Whether to cluster the rows,or the clustering to use.cluster_cols Whether to cluster the columns,or the clustering to use.oclust_rows Whether to use oclust instead of hclust for the rows(if clustering them).oclust_cols Whether to use oclust instead of hclust for the columns(if clustering them).clustering_distance_rowsThe default method for computing row distances(by default,euclidian).clustering_distance_colsThe default method for computing column distances(by default,euclidian).clustering_methodThe default method to use for hierarchical clustering(by default,ward.D2and*not*complete).clustering_callbackIs not supported.DetailsIf you have an a-priori order for the rows and/or columns,you can prevent reordering either or bothby specifying order_rows=FALSE and/or order_cols=FALSE.Otherwise,slanted_orders is usedto compute the"ideal"slanted order for the data.By default,the rows and columns are ordered independently from each other.If the matrix isasymmetric but square(e.g.,a matrix of weights of a directed graph such as a K-nearest-neighborsgraph),then you can can specify same_order=TRUE to force both rows and columns to the sameorder.You can also specify same_order= row to force the columns to use the same order as therows,or same_order= column to force the rows to use the same order as the columns.You can also specify a patch_cols_order and/or a‘patch_rows_order‘function that takes thecomputed"ideal"order and returns a patched order.For example,this can be used to force specialvalues(such as"outliers")to the side of the heatmap.There are four options for controlling clustering:*By default,sheatmap will generate a clustering tree using oclust,to generate the"best"cluster-ing that is also compatible with the slanted order.*Request that sheatmap will use the same hclust as pheatmap(e.g.,oclust_rows=FALSE).Inthis case,the tree is reordered to be the"most compatible"with the target slanted order.That is, sheatmap will invoke reorder_hclust so that,for each node of the tree,the order of the two sub-trees will be chosen to best match the target slanted order.The end result need not be identical tothe slanted order,but is as close as possible given the hclust clustering tree.*Specify an explicit clustering(e.g.,cluster_rows=hclust(...)).In this case,sheatmap willagain merely reorder the tree but will not modify it.In addition,you can give this function any of the pheatmapflags,and it will just pass them on.Thisallows full control over the diagram’s features.Note that clustering_callback is not supported.In addition,the default clustering_methodhere is ward.D2instead of complete,since the only methods supported by oclust are ward.D and ward.D2.ValueWhatever pheatmap returns.Examplesslanter::sheatmap(cor(t(mtcars)))slanter::sheatmap(cor(t(mtcars)),oclust_rows=FALSE,oclust_cols=FALSE)pheatmap::pheatmap(cor(t(mtcars)))slanted_orders Compute rows and columns orders which move high values close tothe diagonal.DescriptionFor a matrix expressing the cross-similarity between two(possibly different)sets of entities,this produces better results than clustering(e.g.as done by pheatmap).This is because clustering does not care about the order of each two sub-partitions.That is,clustering is as happy with((2,1), (4,3))as it is with the more sensible((1,2),(3,4)).As a result,visualizations of similarities using naive clustering can be misleading.Usageslanted_orders(data,order_rows=TRUE,order_cols=TRUE,squared_order=TRUE,same_order=FALSE,discount_outliers=TRUE,max_spin_count=10)Argumentsdata A rectangular matrix containing non-negative values.order_rows Whether to reorder the rows.order_cols Whether to reorder the columns.squared_order Whether to reorder to minimize the l2norm(otherwise minimizes the l1norm).same_order Whether to apply the same order to both rows and columns.discount_outliersWhether to do afinal order phase discounting outlier values far from the diago-nal.max_spin_count How many times to retry improving the solution before giving up.ValueA list with two keys,rows and cols,which contain the order.Examplesslanter::slanted_orders(cor(t(mtcars)))slanted_reorder Reorder data rows and columns to move high values close to the diag-onal.DescriptionGiven a matrix expressing the cross-similarity between two(possibly different)sets of entities, this uses slanted_orders to compute the"best"order for visualizing the matrix,then returns the reordered monly used in pheatmap(slanted_reorder(data),...),and of course sheatmap does this internally for you.Usageslanted_reorder(data,order_data=NULL,order_rows=TRUE,order_cols=TRUE,squared_order=TRUE,same_order=FALSE,discount_outliers=TRUE)Argumentsdata A rectangular matrix to reorder,of non-negative values(unless order_data is specified).order_data An optional matrix of non-negative values of the same size to use for computing the orders.order_rows Whether to reorder the rows.order_cols Whether to reorder the columns.squared_order Whether to reorder to minimize the l2norm(otherwise minimizes the l1norm).same_order Whether to apply the same order to both rows and columns.discount_outliersWhether to do afinal order phase discounting outlier values far from the diago-nal.ValueA matrix of the same shape whose rows and columns are a permutation of the input.Examplesslanter::slanted_reorder(cor(t(mtcars)))Index∗datasetsmeristems,2meristems,2oclust,2reorder_frame,3reorder_hclust,4sheatmap,4slanted_orders,7slanted_reorder,810。
- 1、下载文档前请自行甄别文档内容的完整性,平台不提供额外的编辑、内容补充、找答案等附加服务。
- 2、"仅部分预览"的文档,不可在线预览部分如存在完整性等问题,可反馈申请退款(可完整预览的文档不适用该条件!)。
- 3、如文档侵犯您的权益,请联系客服反馈,我们会尽快为您处理(人工客服工作时间:9:00-18:30)。
电话 传真 因特网
ii
补充信息
警告
该设备输入端子处存在致命的危险电压和电流。因此, AnomAlert*装置应该由受过高压及强电流设备培训后的合格并有 经验的人员安装、维护。AnomAlert 的使用必须遵守所在地区和 国家有关电气设备安装与操作的全部安全法规。仔细审阅包括加 标锁定在内的所有现场相关规程。 时时刻刻都应当遵守推荐的安全预防措施。当电流和电压互感器 原边通电时都会存在致命的电流和电压,因此在安装或今后任何 日常维护过程中,必须遵守标准操作规程范例(例如:短接电流 互感器副边和拆除电压互感器保险丝)。 本装置被设计成工作在某个控制机柜内,装置后部的接线端子在 工作过程中禁止触碰;它不应在任何非上述安装要求的环境中使 用。AnomAlert 不应当作为电气设备的主要保护目的而使用。 声明 维修计划信息及时间安排是基于现场观测制定的,因此是大致近似的、并且把不同类 型设备的运行特点作为平均来进行考虑。由于设备每个部分及其状态都不尽相同,所 以实际故障周期也会有所差异。因此,建议把AnomAlert软件所生成的诊断报告应当仅 作为维修工作和维修工期安排的一个方向性指导,同时对于AnomAlert检测到的异常应 进行核实并尽快予以解决。 产品处置声明 在产品达到寿命期限或者使用期限时拥有所有权的非欧盟成员国客户和第三方是适当 处置产品废弃物的唯一责任人。拥有该产品所有权的任何个人、公司、组织或者机构 在产品都不应违反联邦、州、国家、或者国际法规对产品废弃物处置的规定。本特利 内华达有限公司不承担产品达到寿命期限或使用期限时处置废弃物的责任。
2.1 2.2 2.3 3.1 3.1.1 3.1.2 3.1.3 3.2 3.3 3.4 3.4.1 3.5 3.6 3.7 3.8 3.8.1 3.9 3.9.1 3.9.2 3.10 4.1 4.2 4.3 4.4 4.5 4.6 4.7 4.8
iv
在本地节点添加位置 ........................................................................................................................ 3 在某个位置添加 AnomAlert 装置 ............................................................................................... 3 开始监测 ................................................................................................................................................. 4 设置和使用密码 .................................................................................................................................. 5 修改密码 ....................................................................................................................................... 6 添加新用户或删除现有用户............................................................................................... 6 登出 ................................................................................................................................................. 7 添加和操控远程节点 ........................................................................................................................ 7 添加和删除位置 .................................................................................................................................. 9 设置通讯参数 .................................................................................................................................... 10 高级设定 .................................................................................................................................... 11 从子网添加和删除 AnomAlert 设备 ....................................................................................... 12 联机到远程服务器 .......................................................................................................................... 13 修改本地节点属性 .......................................................................................................................... 13 联系人列表 ......................................................................................................................................... 14 添加人员到联系人列表 ...................................................................................................... 14 电动机故障通知 ............................................................................................................................... 15 邮件服务器设定 ..................................................................................................................... 16 报警列表 .................................................................................................................................... 16 定期参数报告 .................................................................................................................................... 18 监测器启/停 ....................................................................................................................................... 19 指令 ........................................................................................................................................................ 19 删除 ........................................................................................................................................................ 19 属性 ........................................................................................................................................................ 20 通知(警告,报警以及错误) ................................................................................................. 20 信息 ........................................................................................................................................................ 21 趋势 ........................................................................................................................................................ 22 诊断 ........................................................................................................................................................ 24