A comparison between dissimilarity SOM and kernel SOM for clustering the vertices of a grap
英语修辞隐含比较定义
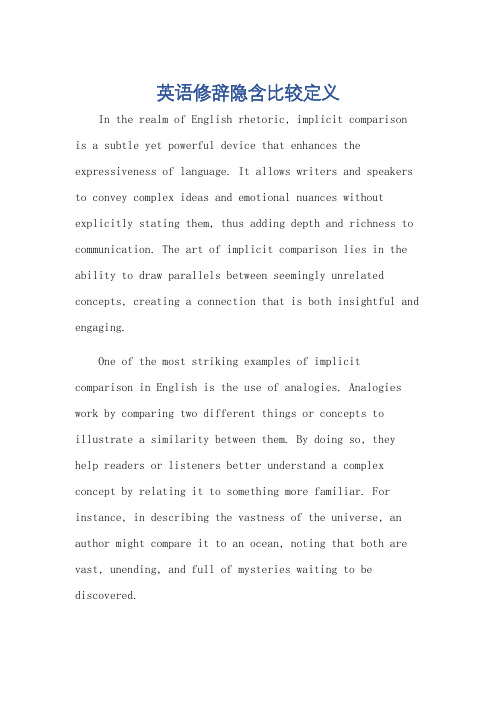
英语修辞隐含比较定义In the realm of English rhetoric, implicit comparison is a subtle yet powerful device that enhances the expressiveness of language. It allows writers and speakers to convey complex ideas and emotional nuances without explicitly stating them, thus adding depth and richness to communication. The art of implicit comparison lies in the ability to draw parallels between seemingly unrelated concepts, creating a connection that is both insightful and engaging.One of the most striking examples of implicit comparison in English is the use of analogies. Analogies work by comparing two different things or concepts to illustrate a similarity between them. By doing so, they help readers or listeners better understand a complex concept by relating it to something more familiar. For instance, in describing the vastness of the universe, an author might compare it to an ocean, noting that both are vast, unending, and full of mysteries waiting to be discovered.Another common form of implicit comparison is the use of hyperbole. Hyperbole involves exaggerating a statement for the purpose of emphasis or to evoke a strong emotional response. By pushing the boundaries of realism, hyperbole allows speakers and writers to convey a sense of urgency or importance that might be difficult to achieve with aliteral statement. For example, when someone says, "I'm starving to death!" they are not literally on the brink of death, but they are emphasizing their extreme hunger through exaggeration.The power of implicit comparison lies in its ability to engage the reader or listener on a deeper level. By making connections between seemingly unrelated ideas, it encourages them to think beyond the literal meaning of words and consider the underlying connections and meanings. This type of rhetorical device is particularly effective in persuasive writing, as it allows the author to subtly guide the reader's thinking without overtly stating their arguments.Implicit comparison also adds a layer of aesthetic pleasure to language. By comparing disparate elements in acreative and unexpected way, it generates a sense of surprise and delight that makes language more enjoyable to read or hear. This is particularly evident in poetry, where poets often use implicit comparison to create images and evoke emotions that are both beautiful and profound.In conclusion, the implicit comparison is a vital tool in the English rhetorician's toolbox. It allows writers and speakers to convey complex ideas and emotional nuances with precision and elegance, engaging their audience on a deeper level. By drawing parallels between seemingly unrelated concepts and using creative language to evoke strong emotional responses, implicit comparison adds a unique and powerful dimension to English rhetoric.**修辞之力:英语中隐含比较的修辞魅力**在英语修辞的世界里,隐含比较是一种微妙而强大的手法,能够增强语言的表达力。
simile and metaphor

Ideas are commodities.
It’s important how you package your ideas. That idea won’t sell. There is always a market for good ideas. That’s a worthless idea. He’s been a source of valuable ideas. Your ideas don’t have a chance in the intellectual marketplace implied comparison between two or more unlike things. His words stabbed at her heart. The tree of liberty must be refreshed from time to time with the blood of patriots and tyrants. It is its natural manure.
Ideas are resources.
He ran out of ideas. Don’t waste your thoughts on small projects. Let’s pool our ideas. We’ve used up all our ideas.
Ideas are money.
General Remarks on Similes and Metaphors
A simile is a direct comparison between two or more unlike things, normally introduced by “like” or “as”. Pop looked so unhappy, almost like a child who has lost his piece of candy. My hands are as cold as ice.
肠道菌群在肠道分布上具有均一性----英文

Increased Proportions of Bifidobacterium and the Lactobacillus Group and Loss of Butyrate-Producing Bacteria in Inflammatory Bowel DiseaseWei Wang,a Liping Chen,a Rui Zhou,a,b Xiaobing Wang,a Lu Song,a Sha Huang,a Ge Wang,a Bing Xia a,bDepartment of Gastroenterology/Hepatology,Zhongnan Hospital of Wuhan University,Wuhan,People’s Republic of China a;Hubei Clinical Center&Key Laboratory of Intestinal&Colorectal Diseases,Wuhan,People’s Republic of China bDysbiosis in the intestinal microbiota of persons with inflammatory bowel disease(IBD)has been described,but there are still varied reports on changes in the abundance of Bifidobacterium and Lactobacillus organisms in patients with IBD.The aim of this investi-gation was to compare the compositions of mucosa-associated and fecal bacteria in patients with IBD and in healthy controls (HCs).Fecal and biopsy samples from21HCs,21and15Crohn’s disease(CD)patients,and34and29ulcerative colitis(UC) patients,respectively,were analyzed by quantitative real-time PCR targeting the16S rRNA gene.The bacterial numbers were transformed into relative percentages for statistical analysis.The proportions of bacteria were uniformly distributed along the colon regardless of the disease state.Bifidobacterium was significantly increased in the biopsy specimens of active UC patients compared to those in the HCs(4.6%versus2.1%,P؍0.001),and the proportion of Bifidobacterium was significantly higher in the biopsy specimens than in the fecal samples in active CD patients(2.7%versus2.0%,P؍0.012).The Lactobacillus group was significantly increased in the biopsy specimens of active CD patients compared to those in the HCs(3.4%versus2.3%,P؍0.036).Compared to the HCs,Faecalibacterium prausnitzii was sharply decreased in both the fecal and biopsy specimens of the active CD patients(0.3%versus14.0%,P<0.0001for fecal samples;0.8%versus11.4%,P<0.0001for biopsy specimens)and the active UC patients(4.3%versus14.0%,P؍0.001for fecal samples;2.8%versus11.4%,P<0.0001for biopsy specimens).In conclusion,Bifidobacterium and the Lactobacillus group were increased in active IBD patients and should be used more cau-tiously as probiotics during the active phase of IBD.Butyrate-producing bacteria might be important to gut homeostasis.C rohn’s disease(CD)and ulcerative colitis(UC)are two formsof inflammatory bowel disease(IBD),a condition driven by an abnormal immune response to the intestinal microbiota in genetically susceptible hosts(1–3).Dysbiosis of the intestinal mi-crobiota is common in IBD.Evidence from antibiotic treatment of IBD,fecal stream diversion in CD,and experimental models of colitis have shown that microbiotas play an important role in the pathogenesis of IBD,and the improvement of dysbiosis in the intestinal microbiota has been propounded as a new strategy for IBD treatment(4).Probiotics are live microorganisms that have health benefits to the host when consumed in adequate amounts,and clinical stud-ies indicate that the quantity of Bifidobacterium and Lactobacillus organisms decreases in the intestinal microbiotas of IBD patients(4).Several clinical trials have demonstrated the efficacy of VSL#3,a mixture of eight different probiotics,for the treatment of UC patients(5,6),and single-species probiotic treatment,such as one with Escherichia coli Nissle1917,Bifidobacterium,or Lactobacillus rhamnosus GG,also displays efficacy in the management of pa-tients with UC(7–9).Meanwhile,experimental studies in colitis mouse models have demonstrated the potential protective mech-anisms of these probiotics,through their reinforcement of the epithelial barrier(10,11),inhibition of proinflammatory cytokine secretion(12,13),and modulation of immune responses(14, 15).Few studies have evaluated the effectiveness of probiotics in CD patients.One study suggested that Faecalibacterium prausnitzii prevents2,4,6-trinitrobenzenesulfonic acid(TNBS)-induced colitis(16).However,studies have shown that the diversity of the genus Bifidobacterium is not decreased in the feces of patients with active CD(17)and that the numbers of Bifidobacterium organisms do not decrease in active CD patients(18).A twin study even found an increased abundance of Bifidobacterium and F.prausnitzii or-ganisms in the mucosal samples of colonic CD patients,as well as an elevated abundance of Lactobacillus organisms in the mucosal samples of ileal CD patients(19).These reports seem to be in conflict with previous data.To investigate the changes caused by common probiotics in IBD patients,we used real-time PCR to quantify bacteria in mu-cosal biopsy specimens and fecal samples of patients with IBD. Furthermore,we also determined the proportional differences of the dominant commensal bacteria between paired fecal and mu-cosal samples.MATERIALS AND METHODSPatients and samples.Chinese patients of Han ethnicity with UC and CD were consecutively recruited from among the outpatients and inpatientsReceived12June2013Returned for modification26July2013Accepted7November2013Published ahead of print13November2013Editor:B.A.ForbesAddress correspondence to Bing Xia,bingxiawh@.W.W.and L.C.contributed equally to this article.Supplemental material for this article may be found at /10.1128/JCM.01500-13.Copyright©2014,American Society for Microbiology.All Rights Reserved.doi:10.1128/JCM.01500-13 Journal of Clinical Microbiology p.398–406February2014Volume52Number2in the Department of Gastroenterology at Zhongnan Hospital of Wuhan University,Wuhan,China.Patients diagnosed with IBD based on data from clinics,radiology,endoscopy,and histology were included in the study.The protocol was approved by the ethics commission of Zhongnan Hospital.The subjects were asked to complete a questionnaire regarding environmental exposure,dietary habits,and antibiotic,probiotic,and drug use.The subjects were required to be adults with an unrestricted diet.Subjects with positive stool cultures of pathogens who were taking anti-biotic or probiotic treatments or colon-cleansing products in the 3months before sampling were excluded.Next,the subjects were invited to participate in the study and provided informed consent.They were askedto expel stool onto a sterile petri dish directly before bowel preparation,and a fresh stool sample was collected on-site and immediately was trans-ferred to the laboratory with an ice box within 1h and stored at Ϫ80°C for further analysis.Subsequently,a magnesium sulfate solution and water were used for bowel preparation,colonoscopy was followed by video en-doscopy,and biopsy specimens were taken from different gut locations.The collection procedure for the fecal and biopsy specimens was accom-plished within 24h.The fecal and biopsy specimens were collected from 76and 63sub-jects,respectively (Table 1).Active CD and active UC were defined as a CD activity index of Ͼ150and a UC activity index of Ͼ3(20,21),respectively.Meanwhile,21healthy controls were matched for stool samples and biopsied tissues,and there were also 8patients with active CD,3patients with CD in remission,16patients with active UC,and 4patients with UC in remission.DNA extraction from biopsy and fecal specimen materials.DNA was extracted from 200mg of feces.Briefly,200mg of stool was added to a 2-ml microcentrifuge tube prefilled with 300mg of 0.1-mm glass beads (Sigma,USA)and incubated on ice until the addition of 1.4ml stool lysis (ASL)buffer from the QIAamp DNA stool minikit (Qiagen,Germany).The samples were immediately subjected to bead beating (45s;speed,6.5m/s)twice using a FastPrep-24machine (MP Biomedicals,USA)before heat and chemical lysis at 95°C for 5min.The subsequent steps of DNA extraction were performed according to the QIAamp kit protocol for pathogen detection.The biopsy specimen DNA was extracted using the QIAamp DNA minikit (Qiagen,Germany)according to the manufactur-er’s instructions,with an additional bead-beating step (45s;speed,6.5,performed twice)using a FastPrep-24at the beginning of the protocol.The extracted DNA was stored at Ϫ80°C for further analysis.Amplification by conventional PCR to check primer specificity.A Bio-Rad PCR machine (Bio-Rad,USA)was used for conventional PCR to check primer specificity.The primers (Table 2)were purchased fromTABLE 1Numbers of specimens by patient group,disease status,and specimen type Patient group Disease status Biopsy location No.of specimens:No.of matched biopsy/fecal specimens Biopsy Feces CDActiveIleum 9158Colon 12Rectum 12Quiescent Ileum 263Colon 3Rectum 3UC Active Colon 222916Rectum 22QuiescentColon 554Rectum 5HC ControlIleum 212121Colon 21Rectum21TABLE 2Group-and species-specific 16S rRNA primers used Target Primer direction Sequence (5=to 3=)Annealing T m (°C)Product size (bp)Reference no.All bacteriaForward ACTCCTACGGGAGGCAGCAGT 6120044Reverse GTATTACCGCGGCTGCTGGCAC Bacteroides Forward GTCAGTTGTGAAAGTTTGC 61.512745Reverse CAATCGGGAGTTCTTCGTG Bifidobacterium Forward AGGGTTCGATTCTGCTCAG 6215645Reverse CATCCGGCATTACCACCC C.coccoides group (XIVa)Forward AAATGACGGTACCTGACTAA 60.744046Reverse CTTTGAGTTTCATTCTTGCGAA C.leptum group (IV)Forward GTTGACAAAACGGAGGAAGG 6024538Reverse GACGGGCGGTGTGTACAA F.prausnitzii Forward AGATGGCCTCGCGTCCGA 61.519934Reverse CCGAAGACCTTCTTCCTCC Lactobacillus group bForward GCAGCAGTAGGGAATCTTCCA 61.534047Reverse GCATTYCACCGCTACACATG E.coli Forward GTTAATACCTTTGCTCATTGA 6134046Reverse ACCAGGGTATCTAATCCTGTT -Globin geneForward CAACTTCATCCACGTTCACC *a26828ReverseGAAGAGCCAAGGACAGGTACa Based on detected bacterial T m .bLactobacillus group PCR primers used to amplify bacteria,including the Lactobacillus ,Pediococcus ,Leuconostoc ,and Weissella group of lactic acid bacteria (LAB).Bacteria in Inflammatory Bowel DiseaseFebruary 2014Volume 52Number 399ShengGong BioTech(ShengGong,China).PCR consisted of35cycles, with an initial DNA denaturation step at95°C(30s),followed by gradi-ent annealing temperature(30s)and elongation at72°C(45s).The procedure was completed with afinal elongation step at72°C(10min). The determinations of optimum temperature were performed using a MyCycler gradient PCR machine,which was adjusted for various tem-perature ranges(Bio-Rad,USA).Real-time PCR.Bacterial16S rRNA gene copies were quantified in mucosal tissue and feces using an iCycler real-time PCR detection system(Bio-Rad,USA).Briefly,standard curves were constructed with a10-fold dilution series of amplified bacterial16S rRNA genes from the reference strains.To determine the influence of biopsy specimen sizes of mucosal tissue,the human cell numbers were quantified using primers specific for the-globin gene to determine the total number of mucosa-associated bacteria in the biopsy specimens.To reduce the quantitative error of the detected bacteria and to characterize the changes in bacterial copies,the abundance of16S rRNA gene copies was calculated from standard curves,and specific bacterial groups were expressed as a percentage of the total bacteria determined by the universal primers.Each reaction was performed in duplicate and re-peated three times.The amplifications were performed in afinal reac-tion volume of20l containing2ϫSYBR mix(GeneCopoeia,USA), 0.4l of each primer at afinal concentration of0.2M,0.4l of ROX (5-carboxy-X-rhodamine)reference dye,2l of bacterial DNA,and ultrapure water to20l.The amplification protocol consisted of one cycle of95°C for10min,followed by40cycles of95°C for10s,an-nealing temperature for30s,and72°C elongation for30s.Thefluo-rescent products were detected at the last step of each cycle.Melting curve analysis was performed from the annealing temperatures to95°C at an increase of0.5°C per10s after amplification to monitor the target PCR product specificity andfidelity.Statistical analysis.Data analysis was conducted using SPSS17.0. Comparisons were made using Student’s t test or a one-way analysis of variance for variables with normal distributions.For nonnormal distribu-tions,the Mann-Whitney U test was used for comparisons between groups,and the Kruskal-Wallis method was used to compare more than two groups.P values ofϽ0.05were considered statistically significant. The total bacterial counts(CFU/g)of each bacterium in the fecal samples were log transformed(log10CFU)for statistical analysis.Specific bacterial counts were expressed as a percentage of the total bacterial counts of each sample.RESULTSClinical characteristics.The demographic and clinical character-istics of the IBD patients are shown in Tables S1and S2in the supplemental material.Percent variation of bacteria in feces.The average bacterial quantifications of feces in each group are summarized in Table3. The comparisons of the fecal bacteria in all groups are shown in Fig.1a and b.The total numbers of bacteria in the fecal samples were similar between the healthy control(HC),CD,and UC pa-tients,and no significant differences were observed.Interestingly,we unexpectedly observed an increase of Bifido-bacterium and the Lactobacillus group in both the active CD(A-CD)and active UC(A-UC)patients,but neither of these popula-tions was significantly different from those in the HCs.However, the proportion of Bifidobacterium was higher in A-UC patients than in A-CD patients.The proportions of Bifidobacterium and the Lactobacillus group were decreased in quiescent-IBD patients compared to active-IBD patients.We also observed a trend of increased Bacteroides organisms in A-CD and A-UC patients compared to healthy controls,but no significant differences were observed.Furthermore,the propor-tion of Bacteroides was lower in quiescent-IBD patients than in active-IBD patients.The Clostridium coccoides group decreased significantly in the feces of both A-CD(Pϭ0.004)and A-UC patients(Pϭ0.015).The Clostridium leptum group,another main group of the Firmicutes phylum,was decreased in A-CD(PϽ0.0001)and A-UC(PϽ0.0001)patients and decreased in R-CDTABLE3Quantification of bacteria in fecal microbiotaDisease group %(meanϮSD)of the indicated bacterial species/group:Bacteroides C.coccoides C.leptum F.prausnitzii Bifidobacterium Lactobacillus E.coliHC14.566Ϯ12.16129.048Ϯ12.75019.618Ϯ10.55814.023Ϯ10.593 1.244Ϯ2.059 2.260Ϯ3.588 1.597Ϯ4.483 A-CD28.444Ϯ22.85015.593Ϯ12.977 1.703Ϯ2.1640.260Ϯ0.575 1.986Ϯ3.442 4.268Ϯ7.073 6.344Ϯ6.505 R-CD23.957Ϯ19.38917.738Ϯ10.466 5.843Ϯ7.541 4.266Ϯ6.078 1.575Ϯ1.673 2.324Ϯ2.537 5.676Ϯ5.687 A-UC26.958Ϯ22.10119.583Ϯ14.767 5.466Ϯ5.106 2.248Ϯ2.860 2.943Ϯ7.410 3.315Ϯ3.43114.742Ϯ17.474 R-UC28.892Ϯ13.47222.617Ϯ8.24711.784Ϯ11.3577.600Ϯ3.795 2.819Ϯ3.326 2.615Ϯ2.630 2.310Ϯ4.607 FIG1(a)Quantification of total bacteria in feces;(b)quantification of dominant bacteria in feces.HC,healthy control;ACD,active Crohn’s disease;RCD, Crohn’s disease in remission;AUC,active ulcerative colitis;RUC,ulcerative colitis in remission.*,PϽ0.05;**,PϽ0.0001.Wang et al. Journal of Clinical Microbiologypatients (P ϭ0.036)compared to in the HCs.We found that the decreased proportion of C.leptum was higher in A-CD patients than in A-UC patients (P ϭ0.014).Although the proportions of C.coccoides and C.leptum in feces showed a rising trend in patients with quiescent IBD,there was no significant difference between quiescent IBD and active IBD patients.F.prausnitzii ,a represen-tative bacterium of the C.leptum group,was decreased both in patients with A-CD (P Ͻ0.0001)and in those with A-UC (P ϭ0.001).The decrease in the proportion of F.prausnitzii in patients with A-CD was significant compared with that in A-UC patients (P ϭ0.01).F.prausnitzii was increased in quiescent IBD patients,but no significant differences were observed compared with pa-tients with active IBD.E.coli ,the most abundant bacterium in the Gammaproteobacteria ,was increased in both CD and UC patients.The proportion of E.coli increased in active-CD (P ϭ0.005)and quiescent-CD (P ϭ0.026)patients compared to that in the HCs.Additionally,the proportion of E.coli increased in active-UC pa-tients (P ϭ0.001)compared to HCs,and the proportion de-creased in quiescent-UC (P ϭ0.05)patients compared with active-UC patients.Moreover,we found that the increased pro-portion of E.coli was more striking in the active-UC than in the active-CD patients (P ϭ0.027).Percent variation of bacteria in different gut locations.To determine whether the percentages of commensals varied signifi-cantly in the different gut locations,we compared the bacterialproportions among the three biopsied locations (Fig.2).The total number of mucosa-associated bacteria in the healthy controls was consistent across the different biopsied locations.The percentages of detected bacteria were almost uniformly distributed along the colon in the healthy controls.The percentages of detected bacteria were also consistent across the different biopsied locations in pa-tients with A-CD.Interestingly,the same results were observed in patients with A-UC and UC in remission (R-UC),in whom the bacteria were almost uniformly distributed along the colon,re-gardless of whether the area was inflamed.Percent variation of bacteria in mucosal biopsy specimens.The average bacterial quantifications of the biopsy specimens in each group are summarized in Table 4.The results were also com-pared to those for HCs.In the present study,we observed a de-creased trend in total mucosa-associated bacteria in patients with CD and UC compared to in the HCs,but no significant difference was observed.Because the biopsied sample size of the CD patients in remission (R-CD)group was limited,we did not compare it with that of the healthy controls.A comparison of the bacteria found in the biopsy specimens from all groups is shown in Fig.3a and b .Bifidobacterium was increased in patients with A-UC (P ϭ0.001)compared to in the HCs,and the increased proportion of Bifidobacterium in the biopsy specimens was higher in A-UC than A-CD patients (P ϭ0.032).Again,the Lactobacillus groupunex-FIG 2Ratios of bacteria in different gut locations and feces.Shown in the upper left graph is the total number of mucosa-associated bacteria at different biopsied locations in different groups.The other five graphs show the dominant probiotic ratios in the feces and different gut locations.Bacteria in Inflammatory Bowel DiseaseFebruary 2014Volume 52Number 2 401pectedly presented a significant increase in patients with A-CD (Pϭ0.036)compared to in the HCs,and although the increased proportion of the Lactobacillus group was higher in patients with A-CD than A-UC,no significant difference was observed.We also observed a rising trend in patients with A-UC,but this trend was not significant.In contrast,the percentages of Bifidobacterium and the Lactobacillus group presented a decreasing trend in patients with quiescent UC,but no significant differences were observed.We observed a trend of increased Bacteroides in the biopsy specimens from patients with A-CD and A-UC compared to in healthy controls,but no significant difference was observed.The proportion of the C.coccoides group in biopsy specimens was de-creased in A-CD patients(PϽ0.0001)compared to in the HCs, while no significant decrease was found in patients with A-UC. The decreased proportion of the C.coccoides group was more striking in patients with A-CD compared to A-UC(Pϭ0.003). The C.leptum group was decreased in patients with A-CD(PϽ0.0001)and A-UC(PϽ0.0001)compared to HCs,and the de-creased proportion was higher in A-CD than A-UC patients,al-though no significant difference was observed.We observed a sig-nificant decrease in the C.leptum group in patients with R-UC (Pϭ0.016)compared to in the HCs.F.prausnitzii was also de-creased in patients with A-CD(PϽ0.0001)and A-UC(PϽ0.0001)compared to in the HCs,and the decreased proportion ofF.prausnitzii was significantly higher in patients with A-CD than in patients with A-UC(Pϭ0.006).Both the C.coccoides group and F.prausnitzii exhibited a rising trend in patients with quies-cent UC compared to those with active UC,but no significant difference was observed.Additionally,E.coli significantly in-creased in the biopsy specimens in IBD patients.The proportion of E.coli was at a high level in patients with active CD(Pϭ0.018) compared to in the HCs.Moreover,E.coli also increased in active UC patients(Pϭ0.016)compare to in the HCs.Although the proportion of E.coli was higher in active CD than in active UC patients,no significant differences were was observed.Comparison of the ratio between fecal and biopsy specimens. As the detected bacteria in the intestinal mucosal biopsy spec-imens showed similar proportions regardless of the biopsied location,we determined whether the proportion was different between biopsy and fecal specimens(Fig.4).The proportion of E.coli was significantly higher in the biopsy specimens(Pϭ0.002) than in fecal samples in21healthy controls,but no significant differences were observed in the other comparisons.In eight paired A-CD cases,the proportion of Bifidobacterium was in-creased in biopsy specimens of the active CD patients(Pϭ0.012) compared to in the fecal samples.The C.coccoides group showed a decrease in the biopsy specimens of A-CD patients(Pϭ0.003) compared to the fecal samples,but this result was not found in the UC patients.Conversely,the C.leptum group and its representa-tive bacterium F.prausnitzii were decreased in the fecal samples of A-CD patients compared to in the biopsy specimens,but no sig-nificant difference was observed.Thisfinding was partly due to the small number of paired cases.However,the C.leptum group showed a decrease in the fecal samples of patients with A-UC(Pϭ0.001)compared to biopsy specimens,but not in R-UC patients. DISCUSSIONIn the present study,we investigated mucosa-associated com-mensal bacteria,as they adhere strictly to the epithelium and can provide access to the mucosa-associated microbiota of the subjects,which may play a more critical role than fecal mi-crobes in IBD pathogenesis(22).In our study,we found that the proportions of detected mucosa-associated bacteria in healthy gastrointestinal tracts were uniformly distributed along the colon,which was in accordance with thefindings from a previous study(23,24).The total bacterial counts and detected bacteria were similar across the different gut locations in the colon,regardless of the disease state,which was in line with some previous data(24,25),although reports with con-flicts data have also been published(26–30).TABLE4Quantification of bacteria in mucosal microbiotaDisease group %(meanϮSD)of the indicated bacterial species/group:Bacteroides C.coccoides C.leptum F.prausnitzii Bifidobacterium Lactobacillus E.coliHC19.030Ϯ6.59926.182ϮA.98021.957Ϯ8.08911.415Ϯ6.085 2.147Ϯ1.514 2.262Ϯ2.887 4.872Ϯ8.83 A-CD32.263Ϯ22.400 6.286Ϯ3.5148.578Ϯ7.6040.817Ϯ0.976 2.793Ϯ2.600 3.420Ϯ2.16911.666Ϯ8.796 A-UC28.393Ϯ15.35619.045Ϯ14.10613.326Ϯ6.679 2.844Ϯ2.243 4.653Ϯ2.889 3.267Ϯ2.5909.831Ϯ10.984 R-UC31.477Ϯ22.29619.542Ϯ14.44412.754Ϯ7.027 3.849Ϯ4.238 3.527Ϯ1.981 2.349Ϯ2.0080.875Ϯ0.459 FIG3(a)Total mucosa-associated bacteria in different groups.(b)Quantification of dominant bacteria in biopsy specimens.*,PϽ0.05;**,PϽ0.0001. Wang et al. Journal of Clinical MicrobiologyAs common probiotics,Bifidobacterium and Lactobacillus have received considerable attention.Surprisingly,the proportion of Bifidobacterium was found to be increased in patients with active IBD.These data were partly in agreement with previous data (17),although conflicting data have also been published (31).Compar-atively,the proportion of Bifidobacterium was reduced in quies-cent CD and UC patients.However,the quantitative PCR (qPCR)results had good agreement only with 454pyrosequencing in the fecal samples.Moran et al.(32)reported that germ-free interleu-kin-10-deficient (IL-10Ϫ/Ϫ)mice administered Bifidobacterium animalis had marked duodenal and mild colonic inflammation and immune responses.Moreover,Medina et al.(33)showed that B.longum diverted immune responses toward a proinflammatory or regulatory profile,consequently producing different effects.In contrast,another study demonstrated that oral Bifidobacterium administration prevented intestinal inflammation through the in-duction of intestinal IL-10-producing Tr1cells and ameliorated colitis in immunocompromised mice (35).In the current study,the Lactobacillus group PCR primers used to amplify bacteria belong to the Lactobacillus ,Pediococcus ,Leuconostoc ,and Weissella groups of lactic acid bacteria (LAB)(25).Unexpectedly,we observed that the Lactobacillus group pre-sented marked increases in patients with active IBD,despite no significant differences in those with active UC.However,in pa-tients with quiescent IBD,the proportion of the Lactobacillus group was similar to that of the HCs in both the fecal and biopsy samples.Because it was difficult to design genus-specific primers to definitively discriminate Lactobacillus ,Pediococcus ,Leuconos-toc ,and Weissella group organisms,we quantified the Lactobacillus group with the genus primer,and the species of the Lactobacillus genus are phylogenetically diverse,with Ͼ100species docu-mented to date (36).This result may suggest that other species of the Lactobacillus genus or LAB-producing bacteria were also in-creased in active-IBD patients.A previous study showed that Lac-tobacillus can secrete lactocepin and exert anti-inflammatory ef-fects by selectively degrading proinflammatory chemokines (12).Mileti et al.(37)found that Lactobacillus paracasei displayed a delay in the development of colitis and a decreased severity of disease but that L.plantarum and L.rhamnosus GG exacerbated the development of dextran sodium sulfate (DSS)-induced colitis.In contrast,Tsilingiri et al.(39)found that L.plantarum induced an inflammatory response in the healthy tissue cultured ex vivo at the end of incubation that resembled the response induced by Salmonella .Moreover,L.paracasei ,L.plantarum ,and L.rhamno-sus GG were detrimental in the inflamed tissue derived from IBD patients cultured ex vivo ,whereas the supernatant from the cul-ture system of L.paracasei directly acted on the tissue and down-regulated the proinflammatory activities of the existing leukocytes (39).It remains to be determined which species of Lactobacillus group is increased in patients during the active phase of IBD.Thus,the effects of Bifidobacterium and Lactobacillus in the gut lumen of active IBD patients are of importance and should be determined.Although the bacteria of the Firmicutes phylum presented a varied degree of decline,the decrease in proportion was greater in patients with A-CD than in patients with A-UC.Moreover,weFIG 4Comparison of the ratios in paired fecal and biopsy samples.*,P Ͻ0.05;**,P Ͻ0.0001.Bacteria in Inflammatory Bowel DiseaseFebruary 2014Volume 52Number 2 403found that the C.coccoides group,which comprises Clostridiumcluster XIVa,including members of other genera,such as Copro-coccus,Eubacterium,Lachnospira,and Ruminococcus(38),wasmore deficient in the biopsy specimens of the A-CD patients thanin the fecal samples,and that the reduced proportion was higherthan that of C.leptum in the biopsy specimens.In contrast,previ-ous studies reported that F.prausnitzii within the C.leptum groupwas strikingly low in mucosa-associated microbiotas(40,41).Based on these results,it is tantalizing to hypothesize that the C.coccoides group was more effective in adhering to the mucosalsurface and that the decrease in the C.coccoides group in both thefecal and biopsy specimens of active CD patients,especially with astrikingly decreased proportion in the biopsy specimens,was spe-cific to CD in genetically susceptible individuals.In our study,we found that the representative bacterium ofthe C.leptum group,F.prausnitzii,nearly disappeared in bothdifferent gut locations and in feces but increased in patientswith quiescent IBD.Previous reports showed that F.prausnitziiproduces formate and butyrate and that its fermented product D-lactate provides energy for colonic epithelial cells and plays an important role in epithelial barrier integrity and immunemodulation(41,42).Additionally,Sokol et al.(16)demon-strated that F.prausnitzii exhibits a butyrate-independent anti-inflammatory effect in IBD models.Interestingly,however,Hansen et al.(43)found that F.prausnitzii was increased inpediatric CD patients at the onset of disease,but not in patientswith UC,suggesting a more dynamic role for this organism inthe development of IBD.Moreover,Willing et al.(19)reportedan increase in F.prausnitzii in colonic CD in twins with inflam-matory bowel disease but a decrease in F.prausnitzii in ilealCD.The biopsy specimens in the study by Hansen et al.weretaken from a single site:from the distal colon in controls,orfrom the most distal inflamed site in IBD.The biggest differ-ence in their data was the inclusion of subjects regardless ofwhether they accepted the conventional IBD treatment.There-fore,pharmacological treatment may be a potential con-founder in the microbial study of IBD.Previous data showedthat the abundance of F.prausnitzii decreased strikingly in pa-tients with ileal CD(28,40),and Sokol et al.(16)also foundthat F.prausnitzii presented a reduction in resected ileal Crohnmucosa and was associated with endoscopic recurrence at6months.However,our data show that F.prausnitzii was con-sistent at different gut locations in patients with CD.This maybe caused by various lifestyle and dietary habits.Our study wasfocused on the populations of central China,most of whomprefer a high-fiber diet,according to the results of our ques-tionnaire.Additionally,F.prausnitzii represented a higher av-erage proportion(11.4%)in the biopsy specimens of the HCs,and organisms with such high proportions may display variedfunctions in different mucosal sites.This remains an interest-ing pursuit for further research.This study design was based on the analysis of bacterial16S rRNAgenes and reflected the gene copy number rather than true cellcounts.Also,the rRNA gene analysis did not reflect the functionalchanges in gastrointestinal tract microbes,such as enhanced viru-lence,mucosal adherence,and invasion,which do not influence therelative proportions of species in the microbiota.Therefore,furtherstudies should be conducted on the functions of commensal bacteria.We identified specific commensal bacteria that were signif-icantly increased or decreased in individuals with CD and UC.The butyrate-producing bacteria of Clostridium clusters IV and XIVa were found to be decreased;in particular,F.prausnitzii was decreased in IBD patients.However,Bifidobacterium and the Lactobacillus group were increased in patients with active IBD. Thus,more attention should be paid to butyrate-producing bac-teria,and Bifidobacterium and Lactobacillus could then be used more cautiously as probiotics in patients during the acute phase of IBD.ACKNOWLEDGMENTSWe thank all the subjects who volunteered to participate in this study.This study was supported by Hubei Science&Technology Bureau (grant no.303131796),the Fundamental Research Funds of the Central University of Ministry of Education of China(grant no.2012303020201 and201130302020004),and the National Support Project of the Ministry of Science&Technology of China(grant no.2012BAI06B03).We declare no conflicts of interest.REFERENCES1.Chassaing B,Darfeuille-Michaud A.2011.The commensal microbiota andenteropathogens in the pathogenesis of inflammatory bowel diseases.Gastro-enterology140:1720–1728./10.1053/j.gastro.2011.01.054.2.Sartor RB.2006.Mechanisms of disease:pathogenesis of Crohn’s diseaseand ulcerative colitis.Nature Clin.Pract.Gastroenterol.Hepatol.3:390–407./10.1038/ncpgasthep0528.3.Sartor RB.2008.Microbial influences in inflammatory bowel diseases.Gastroenterology134:577–594./10.1053/j.gastro.2007 .11.059.4.Neish AS.2009.Microbes in gastrointestinal health and disease.Gastro-enterology136:65–80./10.1053/j.gastro.2008.10.080.5.Miele E,Pascarella F,Giannetti E,Quaglietta L,Baldassano RN,StaianoA.2009.Effect of a probiotic preparation(VSL#3)on induction andmaintenance of remission in children with ulcerative colitis.Am.J.Gas-troenterol.104:437–443./10.1038/ajg.2008.118.6.Tursi A,Brandimarte G,Papa A,Giglio A,Elisei W,Giorgetti GM,FortiG,Morini S,Hassan C,Pistoia MA,Modeo ME,Rodino’S,D’Amico T, Sebkova L,Sacca’N,Di Giulio E,Luzza F,Imeneo M,Larussa T,Di Rosa S,Annese V,Danese S,Gasbarrini A.2010.Treatment of relapsing mild-to-moderate ulcerative colitis with the probiotic VSL#3as adjunc-tive to a standard pharmaceutical treatment:a double-blind,randomized, placebo-controlled study.Am.J.Gastroenterol.105:2218–2227.http://dx /10.1038/ajg.2010.218.7.Kruis W,Fric P,Pokrotnieks J,Lukás M,Fixa B,Kascák M,Kamm MA,Weismueller J,Beglinger C,Stolte M,Wolff C,Schulze J.2004.Main-taining remission of ulcerative colitis with the probiotic Escherichia coli Nissle1917is as effective as with standard mesalazine.Gut53:1617–1623./10.1136/gut.2003.037747.8.Kato K,Mizuno S,Umesaki Y,Ishii Y,Sugitani M,Imaoka A,OtsukaM,Hasunuma O,Kurihara R,Iwasaki A,Arakawa Y.2004.Randomized placebo-controlled trial assessing the effect of bifidobacteria-fermented milk on active ulcerative colitis.Aliment.Pharmacol.Ther.20:1133–1141./10.1111/j.1365-2036.2004.02268.x.9.Zocco MA,dal Verme LZ,Cremonini F,Piscaglia AC,Nista EC,Candelli M,Novi M,Rigante D,Cazzato IA,Ojetti V,Armuzzi A, Gasbarrini G,Gasbarrini A.2006.Efficacy of Lactobacillus GG in main-taining remission of ulcerative colitis.Aliment.Pharmacol.Ther.23: 1567–1574./10.1111/j.1365-2036.2006.02927.x.10.Zakostelska Z,Kverka M,Klimesova K,Rossmann P,Mrazek J,Ko-pecny J,Hornova M,Srutkova D,Hudcovic T,Ridl J,Tlaskalova-Hogenova H.2011.Lysate of probiotic Lactobacillus casei DN-114001 ameliorates colitis by strengthening the gut barrier function and changing the gut microenvironment.PLoS One6:e27961./10.1371 /journal.pone.0027961.11.Patel RM,Myers LS,Kurundkar AR,Maheshwari A,Nusrat A,Lin PW.2012.Probiotic bacteria induce maturation of intestinal claudin3expres-sion and barrier function.Am.J.Pathol.180:626–635. /10.1016/j.ajpath.2011.10.025.12.von Schillde MA,Hörmannsperger G,Weiher M,Alpert CA,Hahne H,Bäuerl C,van Huynegem K,Steidler L,Hrncir T,Pérez-Martínez G,Wang et al. Journal of Clinical Microbiology。
An empirical comparison between direct and indirect test result checking approaches

An Empirical Comparison betweenDirect and Indirect Test Result Checking Approaches∗†‡§Peifeng HuThe University of Hong KongPokfulamHong Kongpfhu@cs.hku.hkZhenyu ZhangThe University of Hong KongPokfulamHong Kong zyzhang@cs.hku.hkW.K.ChanCity University of Hong Kong T at Chee AvenueHong Kong wkchan@.hkT.H.TseThe University of Hong KongPokfulamHong Kongthtse@cs.hku.hkABSTRACTAn oracle in software testing is a mechanism for checking whether the system under test has behaved correctly for any executions.In some situations,oracles are unavailable or too expensive to apply. This is known as the oracle problem.It is crucial to develop techniques to address it,and metamorphic testing(MT)was one of such proposals.This paper conducts a controlled experiment to investigate the cost effectiveness of using MT by38testers on three open-source programs.The fault detection capability and time cost of MT are compared with the popular assertion checking method. Our results show that MT is cost-efficient and has potentials for detecting more faults than the assertion checking method.∗c ACM,2006.This is the authors’version of the work.It is posted here by permission of ACM for your personal use.Not for redistribution.The definitive version was published in Proceedings of the3rd International Workshop on Software Quality Assurance(SOQUA2006)(in conjunction with the14th ACM SIGSOFT International Symposium on Foundations of Software Engineering(SIGSOFT2006/FSE-14)),pages6–13.ACM Press, New York,2006./10.1145/1188895.1188901.†This research is supported in part by a grant of the Research Grants Council of Hong Kong(project no.HKU7145/04E),a grant of City University of Hong Kong and a grant of The University of Hong Kong.‡All correspondence should be addressed to Prof.T.H.Tse at Department of Computer Science,The University of Hong Kong,Pokfulam,Hong Kong.Tel:(+852)28592183.Fax:(+852)25578447.Email: thtse@cs.hku.hk.§Part of the work was done when Chan was with The Hong Kong University of Science and Technology,Clear Water Bay,Hong Kong.Permission to make digital or hard copies of all or part of this work for personal or classroom use is granted without fee provided that copies are not made or distributed for profit or commercial advantage and that copies bear this notice and the full citation on thefirst page.To copy otherwise,to republish,to post on servers or to redistribute to lists,requires prior specific permission and/or a fee.SOQUA’06,November6,2006,Portland,OR,USA.Copyright2006ACM1-59593-584-3/06/0011...$5.00.Categories and Subject DescriptorsD.2.5[Software Engineering]:Testing and Debugging—Testingtools;D.2.8[Software Engineering]:Metrics—Product metricsGeneral TermsExperimentationKeywordsMetamorphic testing,test oracle,controlled experiment,empirical evaluation1.INTRODUCTIONSoftware testing assures programs by executing test cases overthe programs with the intent to reveal failures[3].To do so, software testers need to evaluate test results through an oracle,which is a mechanism for checking whether a program has behaved correctly[35].In many situations,however,oracles are unavailableor too expensive to apply.This is known as the oracle problem[35]. Usually,the main purpose of implementing a specific program is to compute unknown results.If the expected results could easily be computed through other automatic means,then there would not bea need to implement the program in thefirst place.On the other hand,manual checking of program outputs is slow,ineffective,and costly,especially for a large number of test cases.Assessing the correctness of program outcomes has,therefore,been recognizedas“one of the most difficult tasks in software testing”[27].As we shall review in Section2,assertion checking[4]and metamorphic testing(MT)[9,17,18,11]are techniques to alleviatethe oracle problem.Assertion checking verifies the result or intermediate program states of a test case.It directly confirms the execution behavior of a program in terms of a checking condition.MT takes another direction,which verifies follow-up test casesbased on existing test cases.It cross-checks the test resultsof existing test cases and their follow-up test cases.In other words,MT indirectly verifies the behaviors of multiple program executions in terms of a checking condition.It would be interestingto compare the two approaches on their effectiveness to identify failures.HKU CS Tech Report TR-2006-13There have been various case studies in applying metamorphic testing to different types of programs,ranging from conventional programs and object-oriented programs,to pervasive programs and web services.Chen et al.[16]reported on the testing of programs for solving partial differential equations.They further investigated the integration of metamorphic testing with fault-based testing and global symbolic evaluation[18].Gotlieb and Botella[22]developed an automated framework to check against a restricted class of metamorphic relations.Tse and others applied metamorphic approach to the unit testing[33]and integration testing[10]of context-sensitive middleware-based applications. Chan et al.[13,14]developed a metamorphic approach to the online testing of service-oriented software applications. Throughout these studies,both the testing and the evaluation of experimental results were conducted by the researchers themselves. The programs under test were from academic sources and relatively small.There is a need for systematic empirical research on how well MT can be applied in practical situations and how effective it is compared with other testing strategies1.Like other comparisons of testing strategies such as between controlflow and dataflow criteria[21]and among different data flow criteria[25],controlled experimental evaluations are essential. They should answer the following research questions:(a)Can testers be trained to apply MT properly?(b)How does the fault detection effectiveness of MT compare with other effective strategies?(c)What is the effort for applying MT?This paper reports and discusses the results in such a controlled experiment.We restricted the scope to object-oriented testing at the class level[4].The subjects were38postgraduate students enrolled in an advanced software testing course.Before doing the experiment,they were taught the concepts of MT and a reference strategy—assertion checking[4]—to alleviate the oracle problem.The training sessions for either concept were similar in duration.Three open-source programs were selected as target programs.The subjects were required to apply both MT and assertion checking strategies to test these programs independently. We ran their test cases over faulty versions of the target programs to assess the capability of these two testing strategies in detecting faults[1].Results were analyzed to compare the costs and effectiveness between MT and assertion checking.The main contributions of this paper are four-fold:(i)It is the first controlled experiment to study the above questions.(ii)The experiment shows that metamorphic testing is more effective than assertion checking for identifying faults for object-oriented programs.(iii)It confirms the belief that the subjects can formulate metamorphic relations and implement MT without much difficulty. In fact,the experiment shows that all subjects manage to propose metamorphic relations after a brief introduction,and identical or very similar metamorphic relations are proposed by different subjects.(iv)It also indicates that metamorphic testing is worth applying in terms of time cost.The paper is organized as follows:Section2discusses the related literature.Section3introduces the fundamental notions and procedures of metamorphic testing.Section4describes the experiment,and the result is presented and discussed in Section5. Finally,Section6concludes the paper.1Other researchers have evaluated the selection of metamorphic relations. However,their work is not yet publicly accessible at the time of submission of this paper.Thus,we shall exclude them from our discussions.2.RELATED WORKMany approaches have been proposed to alleviate the test oracle problem.Instead of checking the output directly,these approaches generated various types of oracle to verify the correctness of a program.Chapman[15]suggested that a previous version of a program could be used to verify the correctness of the current version.Weyuker[35]suggested checking whether some identity relations would be preserved by the program under test.Blum and others[6,2]proposed a program checker,which was an algorithm for checking the output of computation for numerical programs. Their theory was subsequently extended into the theory of self-testing/correcting[5].Xie and Memon[36]studied different types of oracle for graphic user interface(GUI)testing.Binder[4] discussed four categories and eighteen oracle patterns in object-oriented program testing.Assertion checking[32]is another method to verify the execution results of programs.An assertion,which is embedded directly in the source code,is a Boolean expression that verifies whether the execution of a test case satisfies some necessary properties for correct implementation.Assertions are supported by many programming languages and are easy to implement. Assertion checking has been widely used in object-oriented testing. For example,state invariants[4,23],represented by assertions,can be used to check the stated-based behaviors of a system.Briand et al.[8]investigated the effectiveness of using state-invariant assertions as oracles and compared it with the results using precise oracles for object-oriented programs.It was shown that state-invariant assertions were effective in detecting state-related errors. Since our target programs are also object-oriented programs,we have chosen assertion checking as the alternative testing strategy in our experimental comparison.Some researchers have proposed to prepare test specifications, either manually or automatically,to alleviate the test oracle problem.Memon et al.[28]assumed that a test specification of internal object interactions was available and used it to identify non-conformance of the execution traces.This type of approach is common in conformance testing for telecommunication protocols. Sun et al.[31]proposed a similar approach to test the harnesses of st and others[24,34]trained pattern classifiers to learn the casual input-output relationships of a legacy system. They then used the classifiers as test oracles.Podgurski and others [30,20]classified failure reports into categories via classifiers,and then refined the classification by further means.Bowring et al.[7] used a progressive approach to train a classifier to help regression testing.Chan et al.[12]used classifiers to identify different types of behaviors related to the synchronization failures of objects in a multimedia application.3.PRELIMINARIES OF METAMORPHICRELATIONS AND TESTINGThis section introduces metamorphic testing.As we have briefed in Section1,metamorphic testing relies on a checking condition that relates multiple test cases and their results in order to verify whether any failures are revealed.Such a checking condition is known as a metamorphic relation.We shallfirst revisit metamorphic relations and then discuss how they are used in the metamorphic approach to software testing.3.1Metamorphic RelationsA metamorphic relation(MR)is an existing or expected relation over a set of distinct inputs and their corresponding outputs for multiple executions of the target function[17].Consider,forinstance,the sine function.For any inputs x1and x2such that x1+x2=π,we must have sin x1=sin x2.Definition1(Metamorphic Relation)[11]Let x1,x2,...,x k be a series of inputs to a function f,where k≥1, and f(x1),f(x2),...,f(x k) be the corresponding series ofresults.Suppose f(x i1),f(x i2),...,f(x im) is a subseries,possibly an empty subseries,of f(x1),f(x2),...,f(x k) .Let x k+1,x k+2,...,x n be another series of inputs to f,where n≥k+1,and f(x k+1),f(x k+2),...,f(x n) be the corresponding series of results.Suppose,further,that there exists relationsr(x1,x2,...,x k,f(x i1),f(x i2),...,f(x im),x k+1,x k+2,...,x n)andr′(x1,x2,...,x n,f(x1),f(x2),...,f(x n))such that r′must be true whenever r is satisfied.We say thatMR={(x1,x2,...,x n,f(x1),f(x2),...,f(x n))|r(x1,x2,...,x k,f(x i1),f(x i2),...,f(x im),x k+1,x k+2,...,x n)→r′(x1,x2,...,x n,f(x1),f(x2),...,f(x n))}is a metamorphic relation.When there is no ambiguity,we simply write the metamorphic relation asMR:If r(x1,x2,...,x k,f(x i1),f(x i2),...,f(x im),x k+1,x k+2,...,x n)then r′(x1,x2,...,x n,f(x1),f(x2),...,f(x n)). Furthermore,x1,x2,...,x k are known as source test cases and x k+1,x k+2,...,x n are known as follow-up test cases.Similar to assertions in the mathematical sense,metamorphic relations are also necessary properties of the function to be implemented.They can,therefore,be used to detect inconsistencies in a program.They can be any relations involving the inputs and outputs of two or more executions of the target program.They may include inequalities,periodicity properties,convergence properties, subsumption relationships,and so on.Intuitively,human testers are needed to study the problem domain related to a target program and formulate metamorphic relations accordingly.This is akin to requirements engineering, in which humans instead of automatic requirements engines are necessary for formulating systems requirements.Is there a systematic methodology guiding testers to formulate metamorphic relations like the methodologies that guide systems analysts to specify requirements?This remains an open question.We shall further investigate along this line in the future.We observe that other researchers are also beginning to formulate important properties in the form of specifications to facilitate the verification of system behaviors[19].3.2Metamorphic TestingIn practice,if the program is written by a competent programmer, most test cases are“successful test cases”,which do not reveal any failure.These successful test cases have been considered useless in conventional testing.Metamorphic testing(MT)uses information from such successful test cases,which will be referred to as source test cases.Consider a program p for a target function f in the input domain D.A set of source test cases T={t1,t2,...,t k}can be selected according to any test case selection strategy.Executing the program p on T produces outputs p(t1),p(t2),...,p(t k). When there is an oracle,the test results can be verified against f(t1),f(t2),...,f(t k).If these results reveal any failure,testing stops.On the other hand,when there is no oracle or when no failure is revealed,the metamorphic testing approach can continue to be applied to automatically generate follow-up test cases T′={t k+1,t k+2,...,t n}based on source test cases T,so thatthe program can be verified against metamorphic relations.For example,given a source test case x1for a program that implements the sine function,we can construct a follow-up test case x2based on the metamorphic relation x1+x2=π.Definition2(Metamorphic Testing)[11]Let P be an imple-mentation of a target function f.The metamorphic testing of the metamorphic relationMR:If r(x1,x2,...,x k,f(x i1),f(x i2),...,f(x im),x k+1,x k+2,...,x n),then r′(x1,x2,...,x n,f(x1),f(x2),...,f(x n)) involves the following steps:(1)Given a series of source test cases x1,x2,...,x k and their respective results P(x1),P(x2),..., P(x k) ,generate a series of follow-up test cases x k+1,x k+2,...,x naccording to the relation r(x1,x2,...,x k,P(x i1),P(x i2),...,P(x im), x k+1,x k+2,...,x n)over the implementation P.(2)Check the relation r′(x1,x2,...,x n,P(x1),P(x2),...,P(x n))over P.If r′is false,then the metamorphic testing of MR reveals a failure.3.3Metamorphic Testing ProcedureGotlieb and Botella[22]developed an automated framework for a subclass of metamorphic relations.The framework translates a specification into a constraint logic programming language program.Test cases can be automatically be generated according to metamorphic testing.Their framework only works on a restricted subset of the C language and is not applicable to test cases involving objects.Since we want to apply MT to test real-world object-oriented programs,we adopt the original procedure[9]as follows: Firstly,testers identify and formulate metamorphic relations MR1,MR2,...,MR n from the target function f.For each metamorphic relation MR i,testers construct a function gen i to generate follow-up test cases from the source test cases.Next, for each metamorphic relation MR i,testers construct a function ver i,which will be used to verify whether multiple inputs and the corresponding outputs satisfy MR i.After that,testers generate a set of source test cases T according to a preferred test case selection strategy.Finally,for every test case in T,the test driver invokes the function gen i to generate follow-up test cases and apply the function ver i to check whether the test cases satisfy the given metamorphic relation MR i.If a metamorphic relation MR i is violated by any test case,ver i reports that an error is found in the program under test.4.EXPERIMENTThis section describes the set up of the controlled experiment.It firstly formulates the research questions to be investigated and then describes the experimental design and experimental procedure. 4.1Research QuestionsThe research questions to be investigated are summarized as follows:(a)Can the subjects properly apply MT after training?Can thesubjects identify correct and useful metamorphic relationsfrom target programs?(b)Is MT an effective testing method?Does MT have acomparative advantage over other testing strategies suchas assertion checking in terms of the number of mutantsdetected?To address this question,we shall use the standardstatistical technique of null hypothesis testing.Null Hypothesis H0:difference between MTterms of the number ofAlternativesignificant differencechecking in terms ofdetected.We aim at applying thethe Mann-Whitney test tofindshould be rejected,with athat the difference betweenstatistically significant rather(c)What is the effort,in terms of4.2Experimental DesignOur experiment identifies fourvariables.The independentsubjects,target programs,and faultyThe dependent variables are effort inof metamorphic relations/assertions,terms of mutation detection ratio.strategies,we incorporate MT andof this section,we describe the otherSection5will analyze the resultsvariables.Subjects:All the38subjectscomputer science who attended theSoftware Engineering:SoftwareHong Kong.These students had atcomputer science,computerThe majority of them wereindustrial experience.The rests were MPhil and PhD students.We controlled that the training sessions of either approach are comparable in duration and in content.Since differences in software engineering background might affect the students’capability to apply metamorphic testing or assertion checking,we conducted a brief survey prior to the experimentation.It showed that most of them had real-life or academic experience in object-oriented design,Java programming, software testing,and assertion checking.Figure1lists the survey result.As most of subjects were knowledgeable about object-oriented design and Java programming,they were deemed to be competent in the experimental tasks.On the other hand,we found a few students having rather limited experience in software testing and assertion checking.Since they did not have prior concepts of metamorphic testing either,the experiment did not specifically favor the metamorphic approach.Target Programs:We used three open-source programs as target programs.All of them were Java programs selected from real-world software systems.Thefirst target program Boyer is a program using the Boyer-Moore algorithm to support the applications in Canadian Mind Products,an online commercial software company2.The program returns the index of thefirst occurrence of a specified pattern within a given text.The second target program BooleanExpression evaluates Boolean expressions and returns the resulting Boolean value.For example,the evaluation result of“!(true&&false)||true”is“true”. 2URL /products1.html.The program is part of a popular open-source project jboolexpr3 in SourceForge4,which is the largest open-source project website. The target program is a core part of the project.The third target program is TxnT ableSorter.It is taken from a popular open-source project Eurobudget5in the SourceForge website.Eurobudget is an office application written in Java,similar to Microsoft Money or Quicken.Table1specifies the statistics of the three target programs.The sizes of these programs are in line with the sizes of the target programs used in typical software testing researches such as[1] or the famous Siemens test suites.Thefirst program is a piece of commercial software.The second program is a core part of a standard library.The third one is selected from real office software with hundreds of classes and more than100,000lines of code in total.All of them are open source.Faulty Versions of Target Programs:To investigate the relative effectiveness of metamorphic testing and assertion checking,we used mutation operators to seed faults to programs.A previous study[1]showed that well-defined mutation operators were valid for testing experiments6.In our experiment,mutants were seeded using the tool muJava[26].The tool uses two types of mutation operator:class 3Available at /projects/jboolexpr.4URL .5Available at .6We also attempted to use publicly accessible real faults of these programs to conduct the experiments.However,descriptions of these faults in the source repositories were either too vague or not available.Table2:Categories of Mutation OperatorsCategory DescriptionAOD Delete Arithmetic OperatorsAOI Insert Arithmetic OperatorsAOR Replace Arithmetic OperatorsROR Replace Relational OperatorsCOR Replace Conditional OperatorsCOI Insert Conditional OperatorsCOD Delete Conditional OperatorsSOR Replace Shift OperatorsLOR Replace Logical OperatorsLOI Insert Logical OperatorLOD Delete Logical OperatorASR Replace Assignment Operatorslevel and method level.Class level mutation operators are operators specific to generating faults in object-oriented programs at the class level.Method level mutation operators defined in[29]are operators specific for statement faults.We only seeded method level mutation operators to the programs under study,because our experiment concentrated on unit testing and because this set of operators had been studied extensively[29,1].Table2list all the mutation operators used in the controlled experiment.A total of151mutants were generated by muJava for the class Boyer,145for the class BooleanExpression,and378for TxnT ableSorter.Note that faults were only seeded into the methods supposedly covered by the test cases for unit testing.Table3lists the number of mutants under each category of operators.We used all of them in the controlled experiment.4.3Experimental ProcedureBefore the experiment,the subjects were given a six-hour training to use MT and assertion checking.The target programs and the tasks to be performed were also presented to the subjects.The subjects were briefed about the main functionality of each target program and the algorithm used,thus simulating the process in real-life in which a tester acquires the background knowledge of the program under test.They were blind to the use of mutants in the controlled experiment.For each program,the subjects were required to apply MT strictly following the procedure in Section3.3,as well as to add assertions to the source code for checking.We did not restrict the number of metamorphic relations and assertions.The subjects were told to develop metamorphic relations and assertions as they sawfit,with a view to thoroughly test each target program.We did not mandate the use of a particular testing case generation strategy,such as all-def-use criterion,for MT or assertion checking. The subjects were simply asked to provide adequate test cases for testing the target programs.This avoided the possibility that some particular test case selection strategy,when applied in large scale, might favor either MT or assertion checking.We asked the students to submit metamorphic relations, functions to generate follow-up test cases,functions to verify metamorphic relations,test cases for metamorphic testing,source code with inserted assertions,and test cases for assertion checking. They were also asked to report the time costs in applying metamorphic testing and assertion checking.Before testing the faulty versions with these functions,assertions,and test cases,we checked the student submissions carefully to ensure that there was no implementation error.4.4Addressing the Threats to ValidityWe briefly describe the threats to validity in this section before we present our main results in the next section.Internal Validity:Internal validity refers to whether the observed effects depend only on the intended experimental variables.For this experiment,we provided the subjects with all the background materials and confirmed with them that they had sufficient time to perform all the tasks.On the other hand,we appreciate that students might be interrupted by minor Internet activities when they performed their tasks.Hence,the time costs reported by the subjects should be conservative.Furthermore,the subjects did not know the nature and details of the faults seeded. This measure ensured that their“designed”metamorphic relations and assertions were unbiased with respect to the seeded faults. External Validity:External validity is the degree to which the results are generalizable to the testing of real-world systems.The programs used in our experiment were from real-life applications. For example,Eurobudget is widely used and has been downloaded more than10000times from SourceForge.On the other hand, some real-world programs can be much larger and less well documented than the open-source programs studied.More future studies may be in order for the testing of large complex systems using the MT method.5.EXPERIMENTAL RESULTSThis section presents the experimental results of applying metamorphic testing and assertion checking.They are structured according to the dependent variables presented in the last section.5.1Metamorphic Relations and AssertionsA critical and difficult step in applying MT and assertion checking is to develop metamorphic relations and assertions for target programs.Table4reports on the number of metamorphic relations and assertions identified by the subjects for the three target programs.The mean numbers of metamorphic relations developed by the subjects for the respective programs were2.79, 2.68,and5.00.The total numbers of different metamorphic relations identified by all subjects for the respective programs were 18,39,and25.The mean numbers of assertions for the respective programs were6.96,11.35,and10.97.For the sake of brevity, we list in Table5only the metamorphic relations identified by the subjects for the Boyer program.The results show that all the subjects could properly apply metamorphic testing and assertion checking after training.In general,they could identify a larger number of assertions than metamorphic relations.Furthermore,their abilities to identify metamorphic relations varied.In particular,we observe that all38subjects managed to propose metamorphic relations after some training for each of the three open-source programs.It confirms the belief by the originators of MT that testers can formulate metamorphic relations effectively.5.2Comparison of Fault DetectionCapabilitiesWe use the subjects’metamorphic relations,assertions,and source and follow-up test cases to test the faulty versions of the target programs.The mutation detection ratio[1]is used to compare the fault detection capabilities of MT and assertion checking strategies.The mutation detection ratio of a test set is defined as the number of mutants detected by the test set over the total number of mutants.For metamorphic testing,a mutant is detected if a source test case and follow up test cases executed。
comparison between expository essay and argumentative essay

Essays that define;
Two methods of logical reasoning. One is deduction, and the other is induction. Deductive reasoning moves from the general to the specific; inductive reasoning works to the other way.
TB P. 94
Cause and Effect
reasons why; if...then; as a result; therefore; because
To develop the arguments that support the main idea
Moreover, in addition, further…
批注本地保存成功开通会员云端永久保存去开通
Comparison between Expository Essay and Argumentative Essay
Expository Essay
Argumentative Essay
definition
Exposition is a type of oral or written discourse that is used toexplain, describe, give information or inform.
Express the purpose of the essay in the first paragraph when the essay is short; don’t keep the readers guessing and leave the purpose in the body part
第二语言习得 考试复习题
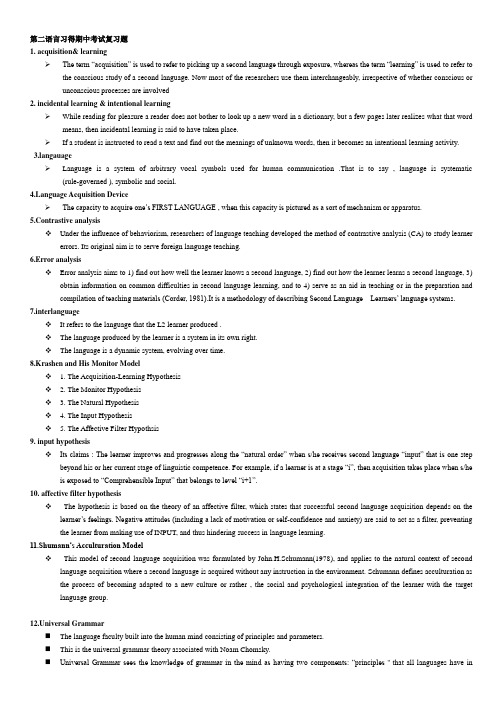
第二语言习得期中考试复习题1. acquisition& learning➢The term “acquisition” is used to refer to picking up a second language through exposure, whereas the term “learning” is used to refer to the conscious study of a second language. Now most of the researchers use them interchangeably, irrespective of whether conscious or unconscious processes are involved2. incidental learning & intentional learning➢While reading for pleasure a reader does not bother to look up a new word in a dictionary, but a few pages later realizes what that word means, then incidental learning is said to have taken place.➢If a student is instructed to read a text and find out the meanings of unknown words, then it becomes an intentional learning activity. ngauage➢Language is a system of arbitrary vocal symbols used for human communication .That is to say , language is systematic (rule-governed ), symbolic and social.nguage Acquisition Device➢The capacity to acquire one’s FIRST LANGUAGE , when this capacity is pictured as a sort of mech anism or apparatus.5.Contrastive analysis❖Under the influence of behaviorism, researchers of language teaching developed the method of contrastive analysis (CA) to study learner errors. Its original aim is to serve foreign language teaching.6.Error analysis❖Error analysis aims to 1) find out how well the learner knows a second language, 2) find out how the learner learns a second language, 3) obtain information on common difficulties in second language learning, and to 4) serve as an aid in teaching or in the preparation and compilation of teaching materials (Corder, 1981).It is a methodology of describing Second Language Learners’ language system s.7.interlanguage❖It refers to the language that the L2 learner produced .❖The language produced by the learner is a system in its own right.❖The language is a dynamic system, evolving over time.8.Krashen and His Monitor Model❖ 1. The Acquisition-Learning Hypothesis❖ 2. The Monitor Hypothesis❖ 3. The Natural Hypothesis❖ 4. The Input Hypothesis❖ 5. The Affective Filter Hypothsis9. input hypothesis❖Its claims : The learner improves and progresses along the “natural order” when s/he receives second language “input” that is one step beyond his or her current stage of linguistic competence. For example, if a learner is at a stage “i”, then acquisition takes place when s/he is exposed to “Comprehensible Input” that belongs to level “i+1”.10. affective filter hypothesis❖The hypothesis is based on the theory of an affective filter, which states that successful second language acquisition depends on the learner’s feelings. Negative attitudes (including a lack of motivation or self-confidence and anxiety) are said to act as a filter, preventing the learner from making use of INPUT, and thus hindering success in language learning.11.Shumann’s Acculturation Model❖This model of second language acquisition was formulated by John.H.Schumann(1978), and applies to the natural context of second language acquisition where a second language is acquired without any instruction in the environment. Schumann defines acculturation as the process of becoming adapted to a new culture or rather , the social and psychological integration of the learner with the target language group.12.Universal Grammar⏹The language faculty built into the human mind consisting of principles and parameters.⏹This is the universal grammar theory associated with Noam Chomsky.⏹Universal Grammar sees the knowledge of grammar in the mind as having two components: “principles"that all languages have incommon and “parameters” on which they vary.13.M acLaughlin’s Information processing model☐SLA is the acquisition of a complex cognitive skill that must progress from controlled processing to automatic processing.14.Anderson’s ACT☐This is another general theory of cognitive learning that has been applied to SLA☐Also emphasizes the automatization process.☐Conceptualizing three types of memory:1. Working memory2. Declarative long term memory3. Procedural long-term memory15.fossilization☐It refers to the phenomenon in which second language learners often stop learning even though they might be far short of native-like competence. The term is also used for specific linguistic structures that remain incorrect for lengthy periods of time in spite of plentiful input.munication strategies⏹Communication strategies, known as CSs, consist of attempts to deal with problems of communication that have arisen in interaction.They are characterized by the negotiation of an agreement on meaning between the two parties.1.What it is that needs to be learnt in language acquisition?➢Phonetics and Phonology➢Syntax➢Morphology➢Semantics➢Pragmatics2.How experts study the children’s acquisition➢Observe young children’s learning to talk.➢Record the speech of their children➢Create a database➢Have a single hypothesis3.What are learning strategies? Give examples ?➢Intentional behaviour and thoughts that learners make use of during learning in order to better help them understand, learn or remember new information .➢Learning strategies are classified into :1. meta-cognitive strategies2. cognitive strategies3. socio-affective strategies4.What are the factors influencing the success of SLA ?●Cognitive factors :1. Intelligence2. Language aptitudenguage learning strategies●Affective factors:nguage attitudes2.Motivation5.What are the differences between the Behaviorist learning model and that of Mentalist?➢Behaviorist learning model claims that children acquired the L1 by trying to imitate utterances produced by people around them and by receiving negative or positive reinforcement of their attempts to do so. Language acquisition, therefore, was considered to be environmentally determined.6.What are the beneficial views obtained from the studies on children’s L1 acquisition?1. Children’s language acquisition goes through several stages2. These stages are very similar across children for a given language, although the rate at which individual children progress through them ishighly variable;3. These stages are similar across languages;4. Child language is rule-governed and systematic, and the rules created by the child do not necessarily correspond to adult ones;5. Children are resistant to correction;6. Children’s mental capacity limits the n umber of rules they can apply at any one time, and they will revert to earlier hypotheses when two ormore rules compete.7.What are the differences of error analysis from contrastive analysisContrastive analysis stresses the interfering effects of a first language on second language learning and claims that most errors come from interference of the first language. (Corder ,1967). However, such a narrow view of interference ignores the intralingual effects of language learning among other factors. Error an alysis is the method to deal with intralingual factors in learners’ language (Corder, 1981).it is a methodology of describing Second Language Learners’ language systems .Error analysis is a type of bilingual comparison, a comparison between learners’ inte rlanguage and a target language, while contrastive analysis between languages. (native language and target language)8.What are UG principles and parameters?➢The universal principle is the principle of structure-dependency, which states that language is organized in such a way that it crucially depends on the structural relationships between elements in a sentence.➢Parameters are prnciples that differ in the way they work or function from language to language. That is to say there are certain linguistic features that vary across languages.9.What role does UG play in SLA?➢Three possibilities :1. UG operates in the same way for L2 as it does for L1.2. The learner’s Core grammar is fixed and UG is no longer available to the L2 learner, particularly not to th e adult learner.3. UG is partly available but it is only one factor in the acquisition of L2. There are other factors and they may interfere with the UGinfluence.10.What are classifications of communication strategies?Faerch and Kasper characterizes CSs in the light of learners’ attempts at governing two different behaviors and their taxonomies are achievement and reduction strategies , and they are based on the psycholinguistics.➢Achievement Strategies:⏹Paraphrase⏹Approximation⏹Word coinage⏹Circumlocution⏹Conscious Transfer⏹Literal translation⏹Language switch (borrowing)⏹Mime⏹Use body language and gestures to make communication open⏹Appeal for assistance➢Reduction Strategies⏹Message abandonment(topic shift):Ask a student to answer the question :How old are you ? She must utter two orthree sentences to answer the question, but she mustn’t tell her age.⏹Topic avoidance(Silence)。
Literature Comparison Methods
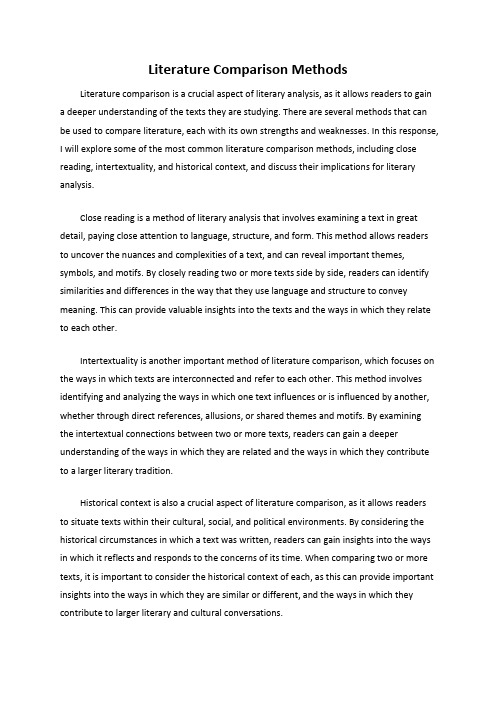
Literature Comparison MethodsLiterature comparison is a crucial aspect of literary analysis, as it allows readers to gain a deeper understanding of the texts they are studying. There are several methods that can be used to compare literature, each with its own strengths and weaknesses. In this response, I will explore some of the most common literature comparison methods, including close reading, intertextuality, and historical context, and discuss their implications for literary analysis.Close reading is a method of literary analysis that involves examining a text in great detail, paying close attention to language, structure, and form. This method allows readers to uncover the nuances and complexities of a text, and can reveal important themes, symbols, and motifs. By closely reading two or more texts side by side, readers can identify similarities and differences in the way that they use language and structure to convey meaning. This can provide valuable insights into the texts and the ways in which they relate to each other.Intertextuality is another important method of literature comparison, which focuses on the ways in which texts are interconnected and refer to each other. This method involves identifying and analyzing the ways in which one text influences or is influenced by another, whether through direct references, allusions, or shared themes and motifs. By examining the intertextual connections between two or more texts, readers can gain a deeper understanding of the ways in which they are related and the ways in which they contribute to a larger literary tradition.Historical context is also a crucial aspect of literature comparison, as it allows readersto situate texts within their cultural, social, and political environments. By considering the historical circumstances in which a text was written, readers can gain insights into the ways in which it reflects and responds to the concerns of its time. When comparing two or more texts, it is important to consider the historical context of each, as this can provide important insights into the ways in which they are similar or different, and the ways in which they contribute to larger literary and cultural conversations.In addition to these methods, there are several other approaches to literature comparison that can be valuable for literary analysis. For example, readers can compare texts based on their genre, style, or thematic content, in order to gain insights into the ways in which they are similar or different. They can also consider the ways in which texts have been received and interpreted by different audiences over time, in order to gain insights into the ways in which they have been understood and valued.Overall, literature comparison is a complex and multifaceted process that requires careful attention to detail and a willingness to explore texts from multiple perspectives. By utilizing a variety of methods, including close reading, intertextuality, and historical context, readers can gain a deeper understanding of the texts they are studying and the ways in which they relate to each other. This can enrich their appreciation of literature and provide valuable insights for further analysis and interpretation.。
The Panda framework for comparing patterns

The P ANDA framework for comparing patterns qIlaria Bartolini a ,Paolo Ciaccia a ,Irene Ntoutsi b ,Marco Patella a,*,Yannis Theodoridis baDEIS,University of Bologna,viale Risorgimento,2,40136Bologna,Italy b Department of Informatics,University of Piraeus,Greece and Research Academic Computer Technology Institute,Athens,Greecea r t i c l e i n f o Article history:Received 10July 2008Received in revised form 3October 2008Accepted 4October 2008Available online 25October 2008Keywords:Pattern comparison Pattern base management systems Data models Knowledge discoverya b s t r a c tData Mining techniques are commonly used to extract patterns ,like association rules anddecision trees,from huge volumes of data.The comparison of patterns is a fundamentalissue,which can be exploited,among others,to synthetically measure dissimilarities inevolving or different datasets and to compare the output produced by different data miningalgorithms on a same dataset.In this paper,we present the P ANDA framework for computingthe dissimilarity of both simple and complex patterns,defined upon raw data and other pat-terns,respectively.In P ANDA the problem of comparing complex patterns is decomposedinto simpler sub-problems on the component (simple or complex)patterns and so-obtained partial solutions are then smartly aggregated into an overall dissimilarity score.This intrinsically recursive approach grants P ANDA with a high flexibility and allows it toeasily handle patterns with highly complex structures.P ANDA is built upon a few basic con-cepts so as to be generic and clear to the end user.We demonstrate the generality and flex-ibility of P ANDA by showing how it can be easily applied to a variety of pattern types,including sets of itemsets and clusterings.Ó2008Elsevier B.V.All rights reserved.1.IntroductionA huge amount of heterogeneous data is collected nowadays from a variety of data sources (e.g.,business,health care,telecommunication,science).The storage rate of these data collections is growing at a phenomenal rate (over 1exabyte per year,according to a recent survey [1]).Due to their quantity and complexity it is impossible for humans to thoroughly investigate these data collections through a manual process.Knowledge discovery in data (KDD)tries to solve this problem by discovering hidden information using data mining (DM)techniques.DM results,called patterns ,constitute compact and rich in semantics representations of raw data [2].Well-known examples of patterns are decision trees,clusterings,and fre-quent itemsets.Patterns reduce the complexity and size of data collections,while preserving most of the information of the original raw data;the degree of preservation,however,strongly depends on the parameters of the DM algorithms used for their extraction.The wide spreading of DM technology makes the problem of efficiently managing patterns an important research issue.Ideally,patterns should be treated by pattern management systems as ‘‘first-class citizens”,in the same fashion that raw data are treated by traditional database management systems.Along this line of research some interesting results,mainly con-centrated on representation and querying issues,have been obtained [2,3].In this paper,we address the relevant issue of pattern comparison ,i.e.,how to establish whether two patterns are similar or not.Pattern comparison is valuable in0169-023X/$-see front matter Ó2008Elsevier B.V.All rights reserved.doi:10.1016/j.datak.2008.10.004qThis work was partially supported by the IST-2001-33058Thematic Network P ANDA ‘‘PAtterns for Next-generation DAtabase systems”.*Corresponding author.E-mail addresses:i.bartolini@unibo.it (I.Bartolini),paolo.ciaccia@unibo.it (P.Ciaccia),ntoutsi@unipi.gr (I.Ntoutsi),marco.patella@unibo.it (M.Patella),ytheod@unipi.gr (Y.Theodoridis).Data &Knowledge Engineering 68(2009)244–260Contents lists available at ScienceDirectData &Knowledge Engineeringjo ur na l h o me pa ge :w w w.e ls ev ie r.c o m/lo c a t e/da t a kI.Bartolini et al./Data&Knowledge Engineering68(2009)244–260245 monitoring and detecting changes in patterns describing evolving data(e.g.,the purchasing behavior of customers over time),as well as in a number of other scenarios,some of which are sketched in Section1.1.A principled approach to pattern comparison needs to address several problems.First,there is a large amount of heter-ogeneous patterns for which a dissimilarity operator should be defined:since each of these pattern types could have its own specific requirements on how the dissimilarity should be assessed,it seems almost impossible(and possibly meaningless)to define a‘‘universal”dissimilarity measure.Second,besides patterns defined over raw data(hereafter called simple patterns), there also exist patterns defined upon other patterns,e.g.,a cluster of frequent itemsets,an association rule of clusters,a forest of decision trees,etc.For these patterns,hereafter called complex patterns,dissimilarity operators should also be de-fined:how these are related to the corresponding ones defined for component patterns needs to be addressed.Third,one should consider that two patterns can be more or less similar both in the data they represent and in the way they represent such data.For instance,two clusters might differ either because of their‘‘shape”or because of the amount of raw data they summarize(or because of both).Given the above,we can state a series of high-level methodological requirements that a framework for dissimilarity assessment should satisfy:General applicability:The framework should be applicable to arbitrary types of patterns.Flexibility:The framework should allow for the definition of alternative dissimilarity functions,even for the same pattern type.Indeed,the end user should be able to easily adjust the dissimilarity criterion to her specific needs.Simplicity:The framework should be built upon a few basic concepts,so as to be understandable to the end user.Efficiency:It should be possible to define the dissimilarity between patterns without the need of accessing the underlying raw data.This requirement also encompasses privacy issues,e.g.,when raw data are not publicly available.The framework we propose,called P ANDA,1addresses above requirements as follows.Generality is achieved by considering that patterns can be(recursively)defined by means of a set of type constructors.To gain the necessaryflexibility in defining dissimilarity operators,P ANDA adopts a modular approach.In particular,the problem of comparing complex patterns is reduced to the one of comparing the corresponding sets(or lists,etc.)of component(simpler)ponent patterns arefirst paired(using a specific matching type)and their scores are then aggregated(through some aggregation function)so as to obtain the overall dissimilarity score.This recursive definition of dissimilarity allows highly complex patterns to be easily handled and, due to modularity,to change any component with an alternative one.To address the requirement of simplicity,P ANDA adopts a consistent approach to model patterns,which are viewed as entities composed of two parts:the structure component identifies ‘‘interesting”regions in the attribute space,e.g.,the head and the body of an association rule,whereas the measure component describes how the pattern is related to the underlying raw data,e.g.,the support and the confidence of the rule.When compar-ing two simple patterns,the dissimilarity of their structure components(hereafter,structure dissimilarity)and the dissimilarity of their measure components(hereafter,measure dissimilarity)are combined(through some combining function)in order to de-rive the total dissimilarity score.Finally,considering the efficiency issue,P ANDA only works in‘‘pattern space”,i.e.,raw data need not to be accessed to evaluate patterns’dissimilarity.It has to be remarked that is not in the P ANDA scope the issue of determining the‘‘best”measure for every comparison problem.Indeed,P ANDA represents a conceptual environment within which specific,user-and/or application-dependent,dis-similarity measures can be framed.Obviously,P ANDA is also amenable to act as a software framework,in which case further advantages are that of favoring the reusability of components and the easy development of user-defined building blocks into ready-to-use libraries.1.1.Motivating examplesIn this section,we provide some illustrative examples which demonstrate the usefulness of a pattern comparison oper-ation.Afirst application is as an alternative to the comparison of raw data collections,e.g.,the monthly sales of a supermar-ket.Approaches which use pattern sets in order to compare the original raw datasets already exist in the literature,e.g.[4,5]: such approaches are based on the intuition that,since patterns condensate the information existing in the raw data,their dissimilarity is a(either lossless or lossy)representation of the dissimilarity of the originating data[6].Defining such a map-ping between dissimilarity in the raw data space and that in the corresponding pattern space is really useful:if the compar-ison between patterns does not show substantial differences,it is possible to avoid a thorough(and costly)analysis on the raw datasets.In the same direction,pattern comparison might be helpful in the distributed database domain to analyze,for example,differences of data characteristics across distributed datasets(e.g.,customer transactions in branches of a super-market or human reactions to chemical/biological substances).Other applications include pattern base synchronization (i.e.,keeping patterns up to date with respect to the original raw data),versioning support in a pattern management system (getting a differential backup of the new version or compare versions of the pattern base so as to discover changes and outliers),the discovery of unexpected or outlier patterns(by comparing them to a target pattern),the evaluation of DM 1P ANDA stands for PAtterns for Next-generation DAtabase systems,an acronym used for the IST-2001-33058project of the European Union,which proposed and studied the PBMS(Pattern Base Management Systems)concept.246I.Bartolini et al./Data&Knowledge Engineering68(2009)244–260algorithms(through the comparison of their outcomes),or secure DM where,due to privacy considerations,only patterns (and not the underlying raw data)are available;in this latter case,the comparison should involve only pattern space char-acteristics,since connection to raw data is lost.We conclude this section by describing a few scenarios where similarity between patterns plays an important role. Example1.Consider a telecommunication company providing a package of new generation services with respect to different customer profiles.Let a decision maker of the company request a monthly report depicting the aggregated usage information of this package as extracted from a data warehouse.Such a report would be far more translatable by the decision maker,e.g.,for target marketing,if it was accompanied by the monthly comparison of the classification of the customer profiles using such services,as these are portrayed,say,via decision tree models.Example2.A spatial DM application analyzes how much the density of population in a town correlates with the number of car accidents.For privacy reasons,raw data are not available,rather only the distributions of population and car accidents in the areas of the town can be used.Such distributions cannot only be compared on a per-area basis,because a high correlation is only detected when the distributions of neighboring areas are compared.The definition of a similarity operator between distributions should beflexible enough to take such correlation into account.Example3.A copy detection system developer has to experiment with different techniques for comparing multimedia doc-uments,in order to select the most effective one.She is given a feature-based representation of the documents(e.g.,list of keywords with weights for the text,distribution of color for the images),and needs to setup a set of methods that take into account all such features and return a score assessing how similar two documents are.The rest of the paper is organized as follows:in Section2,we describe the pattern model underlying the framework and introduce two running examples.Section3is devoted to explain the basic concepts and mechanisms of the P ANDA framework, whereas Section4demonstrates how several comparison measures proposed in the literature can be modeled within the framework.Further examples are included in Appendix A,together with actual experimental results as obtained from a pro-totype software implementation described in Section5.Related work is discussed in Section6,while Section7concludes.2 2.Pattern representationOur approach to pattern representation builds upon the logical pattern base model proposed in[9];in the sequel we de-scribe only the parts of the model relevant to our purposes(for a detailed presentation,please refer to[9]).The model assumes a set of base types(e.g.,Int,Real,Boolean,and String)and a set of type constructors,including list ð<ÁÁÁ>Þ,setðfÁÁÁgÞ,arrayð½ÁÁÁ Þ,and tupleððÁÁÁÞÞ.Let us call T the set of types including all the base types and all the types that can be derived from them through repeated application of the type constructors.Types to which a(unique)name is assigned are called named types.Some examples of types are:{Int}set of integersXYPair=(x:Int,y:Int)named tuple type with attributes x and y <XYPair>list of XYPair sDefinition1(Pattern type).A pattern type is a named pair,PT¼ðSS;MSÞ,where SS is the structure schema and MS is the measure schema.Both SS and MS are types in T.A pattern type PT is called complex if its structure schema SS includes another pattern type,otherwise PT is called simple.The structure schema SS defines the pattern space by describing the structure of the patterns which are instances of the particular pattern type.The complexity of the pattern space depends on the expressiveness of the typing system T.The mea-sure schema MS describes measures that relate the pattern to the underlying raw data or,more in general,provides quan-titative information about the pattern itself.It is clear that the measure complexity also depends exclusively on T.A pattern is an instance of a pattern type,thus it instantiates both the structure and the measure schemas.Assuming that each base typeB is associated with a set of values domðBÞ,it is immediate to define values for any type in T.Definition2(Pattern).Let PT¼ðSS;MSÞbe a pattern type.A pattern p,instance of PT,is defined as p¼ðs;mÞ,where p is the pattern identifier,s(the structure of p,also denoted as p:s)is a value for type SS,p:s2domðSSÞ,and m(the measure of p,also denoted as p:m)is a value for type MS,p:m2domðMSÞ.Before describing the main concepts of the P ANDA framework,we introduce here two running examples that will be used throughout the paper to show the applicability of our framework to real cases,namely the comparison of clusterings and of collections of documents.In particular,in thefirst example,each clustering(set of clusters)represent an image in a2This paper extends the concepts introduced in[7,8]by providing a more formal presentation,along with a significant number of new experiments and examples of application of the framework.region-based image retrieval(RBIR)system,where images are retrieved according to their similarity to a provided query im-age.Experiments on both running examples are detailed in Appendix A.Example4(Clusterings(images)).We illustrate here the case of the W INDSURF image retrieval system[10],which applies a clustering algorithm on visual characteristics of images so as to divide each image into regions of homogeneous pixels (clusters),but the behavior of other RBIR systems can be modeled in a similar way.In details,W INDSURF applies a Discrete Wavelet Transform to each image and the k-means algorithm is used to cluster together pixels sharing common visual characteristic,like color and texture.Each region is then represented as a cluster using the centroid and the corresponding covariance matrix for each color channel and wavelet sub-band(details can be found in[10]),while the cluster support(i.e., the fraction of image pixels contained in the region)is used as the pattern measure.In terms of the P ANDA model,each region(simple pattern)is modeled asRegion¼ðSS:ðbands:½ðcenter:½Real 31;cov:½½Real 3131Þ 41Þ;MS:ðsupp:RealÞÞ:Images are then defined as sets of regions(clusters)with no measure:Image¼ðSS:f Region g;MS:?Þ;where?denotes the null type.Example5(Collections of documents).The problem of comparing collections of documents is quite common in web mining where,for example,it is used tofind sites selling similar products.The problem,in its basic form,assumes a collection(set)of textual documents,where each document consists of a set of keywords.Each keyword k in a document is associated to its(normalized)weight in the document itself(e.g.,representing its frequency using tf=idf measures),and can therefore be modeled as a simple pattern:Keyword¼ðSS:ðterm:StringÞ;MS:ðweight:RealÞÞ:A possible instance of this type isp407¼ððterm¼databaseÞ;ðweight¼0:5ÞÞ:Consequently,documents and collections are represented respectively asDocument¼ðSS:f Keyword g;MS:?Þ;Collection¼ðSS:f Document g;MS:?Þ:3.The P ANDA frameworkIn this section,we provide a framework for assessing the dissimilarity of two patterns,p1and p2,of the same type PT.From Section2,it is evident that the complexity of PT can widely vary and is only restricted by the adopted typing system T.Our framework is built upon two basic principles:1.The dissimilarity between two patterns should be evaluated by taking into account both the dissimilarity of their struc-tures and the dissimilarity of their measures.2.The dissimilarity between two complex patterns should(recursively)depend on the dissimilarity of their componentpatterns.Thefirst principle is a direct consequence of having allowed for arbitrarily complex structures in patterns.Since the struc-ture of a complex pattern might include measures of its component patterns,neglecting the structure dissimilarity could easily result in misleading results.For instance,comparing two Image s,as defined in Example4,obviously needs to take into account the structure component,since the measure one is empty.Another motivation underlying this principle arises from the need of building an efficient framework,which does not force accessing the underlying dataset(s)in order to deter-mine the dissimilarity of two patterns,e.g.,in terms of their common instances.To this end,we use all pieces of information that are available in the pattern space,namely the structural description of the patterns and their quantitative measures with respect to the underlying raw data.The second principle provides the necessaryflexibility to the P ANDA framework.Although,for the case of complex patterns,one could devise arbitrary models for their comparison,it is useful and,at the same time,sufficient for practical purposes,to consider solutions that decompose the‘‘difficult”problem of comparing complex patterns into simpler sub-problems like those of comparing simple patterns,and then‘‘smartly”aggregate the so-obtained partial solutions into an overall score.Besides the above principles,it is also sometimes convenient,in order to offer a better and more intuitive interpretation of the results,to assume that the dissimilarity between two patterns yields a score value,normalized in the½0;1 range(the higher the score,the higher the dissimilarity).Unless otherwise stated,we will implicitly make this assumption throughout the paper.I.Bartolini et al./Data&Knowledge Engineering68(2009)244–260247We start by describing how thefirst principle is applied to the basic case of simple patterns,after that we show how to generalize the framework to the case of complex patterns.3.1.Dissimilarity between simple patternsThe dissimilarity between two patterns,p1and p2,of a simple pattern type PT is based on three key ingredients:a structure dissimilarity function,dis struct,that evaluates the dissimilarity of the structure components of the two patterns,p 1:s and p2:s,a measure dissimilarity function,dis meas,used to assess the dissimilarity of the corresponding measure components,p1:mand p2:m,anda combining function,Comb,also called the combiner,yielding an overall score from the structure and measure dissimilar-ity scores.The dissimilarity of two patterns is consequently determined as(see also Fig.1)disðp1;p2Þ¼Combðdis structðp1:s;p2:sÞ;dis measðp1:m;p2:mÞÞ:ð1ÞIf p1and p2share the same structure,then dis structðp1:s;p2:sÞ¼0.In the general case,in which the patterns have differentstructures,two alternatives exist:1.The structural components are somewhat‘‘compatible”,in which case we interpret dis structðp1:s;p2:sÞas the‘‘additionaldissimilarity”one wants to charge with respect to the case of identical structures.2.Structures are completely unrelated(in a sense that depends on the case at hand),i.e.,dis structðp1:s;p2:sÞ¼1.In this case,regardless of the measure dissimilarity,we also require the overall dissimilarity to be maximum,i.e.,disðp1;p2Þ¼1.Thisrestriction is enforced to prevent cases where two completely different patterns might be considered somehow similar due to low differences in their measures.Example6.Continuing Example5,consider two keywords k1¼ðt1;w1Þand k2¼ðt2;w2Þto be compared.For the structure dissimilarity function,if the two terms are the same,then dis structðt1;t2Þ¼0.When t1–t2,if some information about the semantics of the terms is available,such as a thesaurus or a hierarchical hypernymy/hyponymy ontology,like WordNet[11], then one could set dis structðt1;t2Þ<1to reflect the‘‘semantic distance”between t1and t2[12];on the other hand,if no such information is available,then dis structðt1;t2Þ¼1.A possible choice for the measure dissimilarity function is the absolute difference of measures,i.e.,dis measðw1;w2Þ¼j w1Àw2j.Finally,a possible combiner for this example is,say,the algebraic disjunction of the two dissimilarities:disðk1;k2Þ¼dis structðt1;t2Þþdis measðw1;w2ÞÀdis structðt1;t2ÞÁdis measðw1;w2Þ;ð2Þthat correctly yields disðk1;k2Þ¼1when dis structðt1;t2Þ¼1,and disðk1;k2Þ¼dis measðw1;w2Þwhen dis structðt1;t2Þ¼0. Example7.Continuing our other running example(Example4),W INDSURF uses the Bhattacharyya distance[10]to compare regions,i.e.,clusters structures:dis structðp1:s;p2:sÞ2¼X4b¼11=2Álndet p1:bands½b :covþp2:bands½b :covffiffiffiffiffiffiffiffiffiffiffiffiffiffiffiffiffiffiffiffiffiffiffiffiffiffiffiffiffiffiffiffiffiffiffiffiffiffiffiffiffiffiffiffiffiffiffiffiffiffiffiffiffiffiffiffiffiffiffiffiffiffiffiffiffiffiffiffiffiffiffiffiffiffiffiffiffiffiffiffiffiffiffiffiffiffiffiffiffidetðp1:bands½b :covÞÁdetðp2:bands½b :covÞp@þ1=8ðp1:bands½b :cÀp2:bands½b :cÞTÁp1:bands½b :covþp2:bands½b :cov2À1Áp1:bands½b :cÀp2:bands½b :c ðÞ!!;where detðÁÞdenotes the determinant of a matrix.The measure dissimilarity is defined as dis measðp1:m;p2:mÞ¼j p1:suppÀp2:supp j:248I.Bartolini et al./Data&Knowledge Engineering68(2009)244–260Finally,the combining function simply averages the two distances.It has to be observed that,in several cases,patterns have no measure at all;for instance,sets of strings have type: Set¼ðSS:f String g;MS:?Þ.In this case the assessed dissimilarity will depend only on how much the structural compo-nents of the patterns differ,i.e.,disðp1;p2Þ¼dis structðp1:s;p2:sÞ.It has to be remarked that our framework does not preclude the possibility of defining different dis struct,dis meas and Comb functions for each pattern type of interest.Rather,the functions best suited to the case at hand should be chosen,possibly depending on specific user’s needs,e.g.,to focus the comparison only on some patterns’properties,to trade-off accuracy for computational costs,etc.(see also Section5).3.2.Dissimilarity between complex patternsAlthough in line of principle one could define simple patterns with arbitrarily complicated structural components,this would necessarily force dissimilarity functions to be complex as well and hardly reusable.Among the requirements stated in the introduction,this‘‘monolithic”approach would only comply with that of efficiency,however,failing to address any of the other ones.In P ANDA,we pursue a modular approach that,by definition,is better suited to guaranteeflexibility,sim-plicity,and reusability.Moreover,as it will be discussed later,this does not rule out the possibility of efficient implementations.Coherently with the second principle inspiring our approach,the dissimilarity of complex patterns is evaluated starting from the dissimilarities of the corresponding component patterns.In particular,the structure of complex patterns plays here a major role,since it is where pattern composition occurs.Without loss of generality,in what follows it is assumed that the component patterns,p1;p2;...;p N,of a complex pattern cp completely describe the structure of cp(no additional information is present in cp:s)and that they form a set,i.e., cp:s¼f p1;p2;...;p N g.At the end of this section,we describe how complex patterns built using other type constructors(lists, vectors,and tuples)can be dealt with.The structure dissimilarity of complex patterns cp1:s¼f p11;p21;...;p N11g and cp2:s¼f p12;p22;...;p N22g depends on two fun-damental abstractions,namely:the matching type,which is used to establish how the component patterns of cp1and cp2can be matched,andthe aggregation logic,which is used to combine the dissimilarity scores of the matched component patterns into a single value representing the total dissimilarity between the structures of the complex patterns.3.2.1.Matching typeA matching between the complex patterns cp1:s¼f p11;p21;...;p N11g and cp2:s¼f p12;p22;...;p N22g is a matrix X N1ÂN2¼ðx i;jÞij,where each element x i;j2½0;1 ði¼1;...;N1;j¼1;...;N2Þrepresents the(amount of)matching between the i th componentpattern of cp1and the j th component pattern of cp2,i.e.,between p i1and p j2.A matching type is a set of constraints on the x i;j coefficients so that only some matchings are valid.Relevant cases of matching types include:1–1matching:In this case,each component pattern of cp1(resp.,cp2)might be matched to at most one component patternof cp2(resp.,cp1).Partial matching occurs if N1–N2.The1–1matching type corresponds to the following set ofconstraints:X N1 i¼1x i;j618j;X N2j¼1x i;j618iX N1i¼1X N2j¼1x i;j¼min f N1;N2g;x i;j2f0;1g8i;jN—M(complete)matching:In this case,each component pattern of cp1(resp.,cp2)is matched to every component patternof cp2and vice versa,i.e.,x i;j¼1;8i;j.EMD matching:This matching type,introduced for defining the earth mover’s distance(EMD)[13,14],differs from previ-ous ones in that each x i;j might be real-valued,and represents the amount of p i1‘‘mass”that is matched with p j2.The cor-responding constraints on the matching matrix are:X N1 i¼1x i;j6w j28jX N2j¼1x i;j6w i18i;X N1i¼1X N2j¼1x i;j¼minX N1i¼1w i1;X N2j¼1w j2();x i;j2½0;1 8i;j;where w i1(resp.,w j2)is the weight(mass amount)associated to each component pattern p i1(resp.,p j2)of cp1(resp.,cp2).Finally,note that dissimilarity functions rely,either explicitly or implicitly,on a specific matching type.For instance,the N—M complete matching is used by the complete linkage algorithm to compare clusters.Variations of matching types de-scribed above are also common,such as the one used by the dynamic time warping(DTW)distance[15]as well as related distances for time series(see also Section4.3).I.Bartolini et al./Data&Knowledge Engineering68(2009)244–260249。
- 1、下载文档前请自行甄别文档内容的完整性,平台不提供额外的编辑、内容补充、找答案等附加服务。
- 2、"仅部分预览"的文档,不可在线预览部分如存在完整性等问题,可反馈申请退款(可完整预览的文档不适用该条件!)。
- 3、如文档侵犯您的权益,请联系客服反馈,我们会尽快为您处理(人工客服工作时间:9:00-18:30)。
A comparison between dissimilarity SOM and kernel SOM forclustering the vertices of a graphNathalie Villa(1)and Fabrice Rossi(2)(1)Institut de Math´e matiques de Toulouse,Universit´e Toulouse III118route de Narbonne,31062Toulouse cedex9,France(2)Projet AxIS,INRIA RocquencourtDomaine de V oluceau,Rocquencourt,B.P.105,78153Le Chesnay cedex,France email:(1)nathalie.villa@math.ups-tlse.fr,(2)fabrice.rossi@inria.frKeywords:kernel SOM,dissimilarity,graphAbstract—Flexible and efficient variants of the Self Organizing Map algorithm have been proposed for non vector data,including,for example,the dissimilarity SOM (also called the Median SOM)and several kernelized ver-sions of SOM.Although thefirst one is a generalization of the batch version of the SOM algorithm to data described by a dissimilarity measure,the various versions of the sec-ond ones are stochastic SOM.We propose here to introduce a batch version of the kernel SOM and to show how this one is related to the dissimilarity SOM.Finally,an application to the classification of the vertices of a graph is proposed and the algorithms are tested and compared on a simulated data set.1IntroductionDespite all its qualities,the original Self Organizing Map (SOM,[13])is restricted to vector data and cannot there-fore be applied to dissimilarity data for which only pair-wise dissimilarity measures are known,a much more gen-eral setting that the vector one.This motivated the intro-duction of modified version of the batch SOM adapted to such data.Two closely related dissimilarity SOM were pro-posed in1996[12,1],both based on the generalization of the definition of the mean or median to any dissimilarity measure(hence the alternative name Median SOM).Fur-ther variations and improvements of this model are pro-posed in[11,6,8].Another way to build a SOM on non vector data is to use the kernelized version of the algorithm.Kernel methods have become very popular in the past few years and numer-ous learning algorithm(especially supervised ones)have been“kernelized”:original data are mapped into an high dimensional feature space by the way of a nonlinear feature map.Both the high dimensional space and the feature map are obtained implicitly from a kernel function.The idea is that difficult problems can become linear ones while being mapped nonlinearly into high dimensional spaces.Classi-cal(often linear)algorithms are then applied in the feature spaces and the chosen kernel is used to compute usual oper-ations such as dot products or norms;this kernelization pro-vides extensions of usual linear statistical tools into nonlin-ear ones.This is the case,among others,for Support Vector Machine(SVM,[20])which corresponds to linear discrim-ination or Kernel PCA([17])which is built on Principal Component Analysis.More recently,kernelized version of the SOM has been studied:[10]first proposes a kernelized version of SOM that aims at optimizing the topographic mapping.Then,[2,16]present kernel SOM that applies to the images of the original data by a mapping function;they obtain improvements in classification performances of the algorithm.[15,23]also studied these algorithms:thefirst one gives a comparison of various kernel SOM on several data sets for classification purposes and the second proves the equivalence between kernel SOM and self-organizing mixture density network.In this work,we present a batch kernel SOM algorithm and show how this algorithm can be seen as a particular ver-sion of the dissimilarity SOM(section2).We target specif-ically non vector data,more precisely vertices of a graph for which kernels can be used to define global proximi-ties based on the graph structure itself(section3.1).Ker-nel SOM provides in this context an unsupervised classi-fication algorithm that is able to cluster the vertices of a graph into homogeneous proximity groups.This applica-tion is of a great interest as graphs arise naturally in many settings,especially in studies of social networks such as World Wide Web,scientific network,P2P network([3])or medieval peasant community([4,22]).Wefinally propose to explore and compare the efficiency of these algorithms to this kind of problems on a simulated example(section 3.2).2A link between kernel SOM and Dissimilarity SOMIn the following,we consider n input data,x1,...,x n from an arbitrary input space,G.In this section,we present self-organizing algorithms using kernels,i.e.functions k: G×G→R such that are symmetric(k(x,x )=k(x ,x))and positive (for all m ∈N ,all x 1,...,x m ∈G and all α1,...,αm ∈R , mi =1αi αj k (x i ,x j )≥0).These functions are dot products of a mapping func-tion,φ,which is often nonlinear.More precisely,it exists an Hilbert space,(H , .,. ),called a Reproducing Kernel Hilbert Space (RKHS),and a mapping function φ:G →H such that k (x,x )= φ(x ),φ(x ) .Then,algorithms that use the input data by the way of their norms or dot products are easily kernelized using the images by φof the original data set:φand H are not explicitely known as the opera-tions are defined by the way of the kernel function.2.1On-line kernel SOMA kernel SOM based on the k -means algorithm has beenfirst proposed by [16].The input data of this algorithm are the images by φof x 1,...,x n and,as in the original SOM,they are mapped into a low dimensional grid made of M neurons,{1,...,M },which are related to each others by a neighborhood relationship,h .Each neuron j is represented by a prototype in the feature space H ,p j ,which is a linear combination of {φ(x 1),...,φ(x n )}:p j = n i =1γji φ(x i ).This leads to the following algorithm:Algorithm 1:On-line kernel SOM(1)For all j =1,...,M and all i =1,...,n ,initialize γ0jirandomly in R ;(2)For l =1,...,L ,do (3)assignment step :x l is assigned to the neuron,f l(x l )which has the closest prototype:f l (x l )=argminj =1,...,Mφ(x l )−p l −1j(4)representation step :for all j =1,...,M ,the pro-totype p j is recomputed:for all i =1...,n ,γl ji =γl −1ji +α(l )h (f l (x l ),j )“δil −γl −1ji ”End for.where step (3)leads to the minimization ofni,i =1γl −1ji γl −1ji k (x i ,x i )−2 n i =1γl −1ji k (x i ,x l ).As shown in [23],the kernel SOM can be seen as a result of minimizing the energy E = n j =1h (f (x ),j ) φ(x )−p j 2stochastically.Another version of the kernel SOM is also proposed by [2]:it uses prototypes chosen in the original set and then computes the algorithm with the images by φof this pro-totype.It comes from the minimization of the following energy E = n j =1h (f (x ),j ) φ(x )−φ(p j ) 2.2.2Dissimilarity SOM with dissimilaritiesbased on kernelsDissimilarity SOM ([11,6,8])is a generalization of the batch version of SOM to data described by a dissimilar-ity measure.We assume given,for all i,i =1,...,n ,ameasure δ(x i ,x i ),that is symmetric (δ(x,x )=δ(x ,x )),positive (δ(x,x )≥0)and such that δ(x,x )=0.The Dissimilarity SOM proceeds as follows:Algorithm 2:Dissimilarity SOM(1)For all j =1,...,M ,initialize p 0j randomly to one of theelement of the data set {x 1,...,x n };(2)For l =1,...,L ,do (3)assignment step :for all i =1,...,n ,x i is assigned to the neuron,f l (x i )which has the closest prototype:f l (x i )=argminj =1,...,Mδ(x i ,p l −1j)(4)representation step :for all j =1,...,M ,the pro-totype p j is recomputed:p l j=argminx ∈(x i )i =1,...,nn X i =1h (f l (x i ),j )δ(x i ,x )End for.As shown in step (4),the purpose is to choose prototypesin the data set that minimize the generalized energy E = M j =1 ni =1h (f (x i ),j )δ(x i ,p j ).[6,8]propose variants of this algorithm:the first one allows the use of several prototypes for a single neuron and the second describes a faster version of the algorithm.In [22],a dissimilarity based on a kernel is described:it is designed for the clustering of the vertices of a graph.To construct their dissimilarity,the authors take advantage of the fact that the kernel can be interpreted as a norm;then computing the distance induced by this norm leads to the definition of a dissimilarity measure on {x 1,...,x n }:δmed (x,x )= φ(x )−φ(x ) (1)= k (x,x )+k (x ,x )−2k (x,x ).We can also define a variant of this dissimilarity measure by,for all x ,x in G ,δmean (x,x )= φ(x )−φ(x ) 2(2)=k (x,x )+k (x ,x )−2k (x,x ).We now show that the dissimilarity SOM based on this last measure can be seen as a particular case of a batch version of the kernel SOM.2.3Batch kernel SOMReplacing the value of the dissimilarity (2)in the represen-tation step of the Dissimilarity SOM algorithm leads to the following equation:p l j =argminx ∈(x i )i =1,...,nn i =1h (f l (x i ),j ) φ(x i )−φ(x ) 2.In this equation,the prototypes are the images by φof some vertices;if we now allow the prototypes to belinear combinations of {φ(x i )}i =1,...,n as in the kernel SOM (section 2.1),the previous equation becomes p l j = ni =1γlji φ(x i )whereγl j=arg min γ∈Rnn i =1h (f l(x i ),j ) φ(x i )−n i =1γi φ(x i ) 2.(3)Equation (3)has a simple solution:p j = ni =1h (f l(x i ),j )φ(x i ) n i =1h (f l(x i ),j )(4)which is the weighted mean of the (φ(x i ))i .As a conse-quence,equation (3)is the representation step of a batch SOM computed in the feature space H .We will call this algorithm kernel batch SOM :Algorithm 3:Kernel batch SOM(1)For all j =1,...,M and all i =1,...,n ,initialize γ0jirandomly in R ;(2)For l =1,...,L ,do (3)assignment step :for all i =1,...,n ,x i is assigned to the neuron,f l (x i )which has the closest prototype:f l (x i )=argminj =1,...,Mφ(x i )−p l −1j(4)representation step :for all j =1,...,M ,the pro-totype p j is recomputed:γlj=arg min γ∈Rnn X i =1h (f l(x i ),j ) φ(x i )−n X i =1γi φ(x i ) 2End for.where,as shown in (4),the representation step simplyreduces toγlji =h (f l (x i ),j ) n i =1h (f l (x i ,j )).Like in the on-line version,the assignment is run by di-rectly using the kernel without explicitly defining φandH :in fact,and all x ∈{x i ,...,x n },it leads to the mini-mization on j ∈{1,...,M }of ni,i =1γji γji k (x i ,x i )−2 ni =1γji k (x,x i ).Then,the kernel batch SOM is simply a batch kernel SOM performed in a relevant space so it shares its consistancy properties [7].Finally,we conclude that the dissimilarity SOM de-scribed in section 2.2can be seen as the restriction of a kernel batch SOM to the case where the prototypes are ele-ments of the original data set.Formally,dissimilarity SOM is the batch kernel SOM for which the feature space is not Hilbertian but discrete.3Application to graphsThe fact that the prototypes are defined in the feature space H from the original data {x 1,...,x n }allows to apply thealgorithms described in section 2to a wide variety of data,as long as a kernel can be defined on the original set G (for which no vector structure is needed).In particular,this algorithm can be used to cluster the vertices of a weighted graph into homogeneous proximity groups using the graph structure only,without any assumption on the vertices set.The problem of clustering the vertices of a graph is of a great interest,for instance as a tool for understanding the organization of social networks ([3]).This approach has already been tested for the dissimilarity SOM on a graph extracted from a medieval database ([22]).We use in the rest of the paper the following notations.The dataset {x 1,...,x n }consists in the vertices of a graph G ,with a set of edges in E .Each edge (x i ,x i )has a positive weight w i,i (with w i,i =0⇔(x i ,x i )/∈E ).Weights are assumed to be symmetric (w i,i =w i ,i ).We call d i the degree of the vertex x i given by d i = ni =1w i,i.3.1The Laplacian and related kernelsIn [19],the authors investigate a family of kernels based on regularization of the Laplacian matrix.The Laplacian of the graph is the positive matrix L =(L i,i )i,i =1,...,n such that L i,i =−w i,i if i =id i if i =i (see [5,14]for a comprehensive review of the propertiesof this matrix).In particular,[19]shows how this discrete Laplacian can be derived from the usual Laplacian defined on continuous spaces.Applying regularization functions to the Laplacian,we obtain a family of matrices including •Regularized Laplacian :for β>0,K β=(I n +βL )−1;•and Diffusion matrix :for β>0,K β=e −βL ;These matrices are easy to compute for graphs having a few hundred of vertices via an eigenvalue decomposition:their eigensystem is deduced by applying the regularizing func-tions to the eigenvalues of the Laplacian (the eigenvectors are the same).Moreover,these matrices can be interpreted as regularizing matrices because the norm they induced pe-nalizes more the vectors that vary a lot over close vertices.The way this penalization is taken into account depends on the regularizing function applied to the Laplacian ing these regularizing matrices,we can define associ-ated kernel by k β(x i ,x i )=K βii .Moreover,the diffusion kernels (see also [14])can be interpreted as the quantity of energy accumulated after a given time in a vertex if energy is injected at time 0in the other vertex and if the diffusion is done along the edges.It has then become very popular to summarize both the global structure and the local proxim-ities of a graph (see [21,18]for applications in computa-tional biology).In [9],the authors investigate the distances induced by kernels of this type in order to rank the vertices of a weighted graph;they compare them to each others andshow their good performances compared to standard meth-ods.3.2SimulationsIn order to test the 3algorithms presented in section 2for clustering the vertices of a graph,we simulated 50graphs having a structure close to the ones described in [4,22].We made them as follow:•We built 5complete sub-graphs (C i )i =1,...,5(cliques)having (n i )i =1,...,5vertices where n i are generated from a Poisson distribution with parameter 50;•For all i =1,...,5,we generated l i random links between C i and vertices of the other cliques:l i is generated from a uniform distribution on the set {1,...,100n i }.Finally,multiple links are removed;thus the simulated graphs are “non-weighted”(i.e.w i,i ∈{0,1}).A simplified version of these types of graphs is shown in Figure 1:we restricted the mean of x i to 5and l i is gener-ated from a uniform distribution on {1,...,10n i }in order to make the visualizationpossible.Figure 1:Example of a simulated graph:the vertices of the 5cliques are represented by different labels (+ *x o)Algorithms 1to 3were tested on these graphs by using the diffusion kernel and the dissimilarities (equations (1)and (2))built from it.The grid chosen is a 3×3rectangular grid for which the central neuron has a neighborhood of size 2,as illustrated in Figure 2.We ran all the algorithms until the stabilization is ob-tained,which leads to:•500iterations for the on-line kernel SOM (algorithm 1);•20iterations for the dissimilarity SOM with both dis-similarities (algorithm 2);•10iterations for the batch SOM (algorithm 3).Then,to avoid the influence of the initialization step,the algorithms were initialized randomly 10times.For each graph and each algorithm,the best classification,whichFigure 2:SOM grid used (dark gray is the 1-neighborhood and light gray the 2-neighborhood of the central neuron)minimizes the energy of the final grid,is kept.The compu-tational burden of the algorithms is summarize in Table 1:it gives the total running time for 50graphs and 10initial-izations per graph.Batch kernel SOM is the fastest whereas Algorithm on-line k-SOMd-SOM batch k-SOMTime (min)2608020Table 1:Computation timesthe on-line kernel SOM is very slow because it needs a high number of iterations to stabilize.For the batch kernel SOM,we initialize the prototypes with random elements of the data set (as in the dissimilarity SOM)in order to obtain a good convergence of the algorithm.Finally,we tested three parameters for the diffusion ker-nel (β=0.1,β=0.05and β=0.01).Higher parameters were not tested because we had numerical instabilities in the computation of the dissimilarities for some of the 50graphs.In order to compare the classifications obtained by the different algorithms,we computed the following crite-ria:•the mean energy (except for the dissimilarity SOM with dissimilarity (1)which does not have a compa-rable energy).Notice also that the energies computed for different values of βcan also not be compared;•the standard deviation of the energy;•the mean number of classes found by the algorithm;•after having associated each neuron of the grid to one of the cliques by a majority vote law,the mean pour-centage of good classified vertices (vertices assigned to a neuron associated to its clique);•the number of links divided by the number of possible links between 2vertices assigned to 2neurons having distance 1(or 2,3,4)between them on the grid.The results are summarized in Tables 2to 5and an example of a classification obtained for the batch kernel SOM for the graph represented in Figure 1is given in Figure 3.First of all,we see that the quality of the classification heavily depends on the choice of β.For this application,performances decrease with β,with very bad performances for all the algorithms with the parameter β=0.01.β=0.1β=0.05β=0.01 Mean energy0.10 3.21196Sd of energy0.40 4.739 Mean nb of classes8.048.929 Mean%of79.8478.2839.72 good classif.%of links for54.558.451.31-neighborhood%of links for39.140.048.02-neighborhood%of links for34.933.145.63-neighborhood%of links for24.228.943.84-neighborhoodTable2:Performance criteria for the on-line kernel SOMβ=0.1β=0.05β=0.01 Mean energy NA NA NASd of energy NA NA NA Mean nb of classes999 Mean%of77.3440.4929.56 good classif.%of links for48.645.452.51-neighborhood%of links for42.045.555.82-neighborhood%of links for38.048.157.13-neighborhood%of links for34.851.557.04-neighborhoodTable3:Performance criteria for the dissimilarity SOM (dissimilarity(1))Then,we can also remark that the performances highly depend on the graph:the standard deviation of the energy is high compared to its mean.In fact,5graphs always ob-tained very bad performances and removing them divides the standard deviation by20.Comparing the algorithms to each others,we see that the batch kernel SOM seems tofind the best clustering for the vertices of the graph:this is the one for which the mean number of classes found by the algorithm is the closest to the number of cliques(5).It has also the best pourcentage of good classified vertices and the smallest number of links for all neighborhoods,proving that the vertices classified in the same cluster are also frequently in the same clique. Then comes the on-line kernel SOM that suffers from a long computational time andfinally,the dissimilarity SOM with slightly better performances for the dissimilarity(2). Comparing thefirst three Tables,we can say that the perfor-mance gain of the on-line kernel SOM is really poor com-pared to the computational time differences between the algorithms.Moreover,interpretation of the clusters mean-β=0.1β=0.05β=0.01 Mean energy0.13 3.99300Sd of energy0.557.365 Mean nb of classes999 Mean%of77.8940.6929.77 good classif.%of links for49.645.052.31-neighborhood%of links for41.745.755.82-neighborhood%of links for36.948.056.83-neighborhood%of links for34.051.058.84-neighborhoodTable4:Performance criteria for the dissimilarity SOM (dissimilarity(2))β=0.1β=0.05β=0.01 Mean energy0.10 3.00172Sd of energy0.38 4.4535 Mean nb of classes 6.567.569 Mean%of94.3492.7232.81 good classif.%of links for44.948.047.61-neighborhood%of links for37.837.646.62-neighborhood%of links for29.132.348.83-neighborhood%of links for28.628.546.44-neighborhoodTable5:Performance criteria for batch kernel SOMing can take benefit of the fact that prototypes are elements of the data set.On the contrary,dissimilarity SOM totally fails in decreasing the number of relevant classes(the mean number of clusters in thefinal classification is always the biggest possible,9);this leads to a bigger number of links between two distinct neurons than in the both versions of kernel SOM.4ConclusionsWe show in this paper that the dissimilarity SOM used with a kernel based dissimilarity is a particular case of a batch kernel SOM.This leads us to the definition of a batch un-supervised algorithm for clustering the vertices of a graph. The simulations made on randomly generated graphs prove that this batch version of kernel SOM is both efficient and fast.The dissimilarity SOM,which is more restricted,is less efficient but still have good performances and produces prototypes that are more easily interpretable.We also em-phasized the importance of a good choice of the parameterFigure3:Example of a classification obtained for the batch kernel SOM on graph represented in Figure1of the kernel,a problem for which an automatic solution would be very useful in practice. AcknowledgementsThis project is supported by“ANR Non Th´e matique 2005:Graphes-Comp”.The authors also want to thank the anonymous referees for their helpful comments. References[1] C.Ambroise and aert.Analyzing dissimilarity ma-trices via Kohonen maps.In Proceedings of5th Conference of the International Federation of Classification Societies (IFCS1996),volume2,pages96–99,Kobe(Japan),March 1996.[2]P.Andras.Kernel-Kohonen networks.International Journalof Neural Systems,12:117–135,2002.[3]S.Bornholdt and H.G.Schuster.Handbook of Graphs andNetworks-From the Genome to the Internet.Wiley-VCH, Berlin,2002.[4]R.Boulet and B.Jouve.Partitionnement d’un r´e seau desociabilit´e`a fort coefficient de clustering.In7`e mes Journ´e es Francophones“Extraction et Gestion des Connaissances”, pages569–574,2007.[5] F.Chung.Spectral Graph Theory.Number92in CBMS Re-gional Conference Series in Mathematics.American Math-ematical Society,1997.[6] B.Conan-Guez,F.Rossi,and A.El Golli.Fast algorithmand implementation of dissimilarity self-organizing maps.Neural Networks,19(6-7):855–863,2006.[7]M.Cottrell,B.Hammer,A.Hasenfuss,and T.Villmann.Batch and median neural gas.Neural Networks,19:762–771,2006.[8] A.El Golli,F.Rossi,B.Conan-Guez,and Y.Lecheval-lier.Une adaptation des cartes auto-organisatrices pour des donn´e es d´e crites par un tableau de dissimilarit´e s.Revue de Statistique Appliqu´e e,LIV(3):33–64,2006.[9] F.Fouss,L.Yen,A.Pirotte,and M.Saerens.An experimen-tal investigation of graph kernels on a collaborative recom-mendation task.In IEEE International Conference on Data Mining(ICDM),pages863–868,2006.[10]T.Graepel,M.Burger,and K.Obermayer.Self-organizingmaps:generalizations and new optimization techniques.Neurocomputing,21:173–190,1998.[11]T.Kohohen and P.J.Somervuo.Self-Organizing maps ofsymbol strings.Neurocomputing,21:19–30,1998.[12]T.Kohonen.Self-organizing maps of symbol strings.Tech-nical report a42,Laboratory of computer and information science,Helsinki University of technoligy,Finland,1996.[13]T.Kohonen.Self-Organizing Maps,3rd Edition.In SpringerSeries in Information Sciences,volume30.Springer,Berlin, Heidelberg,New York,2001.[14]R.I.Kondor and fferty.Diffusion kernels on graphs andother discrete structures.In Proceedings of the19th Inter-national Conference on Machine Learning,pages315–322, 2002.[15]u,H.Yin,and S.Hubbard.Kernel self-organisingmaps for classification.Neurocomputing,69:2033–2040, 2006.[16] D.Mac Donald and C.Fyfe.The kernel self organisingmap.In Proceedings of4th International Conference on knowledge-based intelligence engineering systems and ap-plied technologies,pages317–320,2000.[17] B.Sch¨o lkopf,A.Smola,and K.R.M¨u ller.Nonlinear com-ponents analysis as a kernel eigenvalue problem.Neural Computation,10:1299–1319,1998.[18] B.Sch¨o lkopf,K.Tsuda,and J.P.Vert.Kernel methods incomputational biology.MIT Press,London,2004.[19] A.J.Smola and R.Kondor.Kernels and regularization ongraphs.In M.Warmuth and B.Sch¨o lkopf,editors,Pro-ceedings of the Conference on Learning Theory(COLT)and Kernel Workshop,2003.[20]V.Vapnik.The Nature of Statistical Learning Theory.Springer Verlag,New York,1995.[21]J.P.Vert and M.Kanehisa.Extracting active pathways fromgene expression data.Bioinformatics,19:238ii–244ii,2003.[22]N.Villa and R.Boulet.Clustering a medieval social networkby SOM using a kernel based distance measure.In M.Ver-leysen,editor,Proceedings of ESANN2007,pages31–36, Bruges,Belgium,2007.[23]H.Yin.On the equivalence between kernel self-organisingmaps and self-organising map mixture density networks.Neural Networks,19:780–784,2006.。