6 ORDINARY DIFFERENTIAL EQUATIONS
关于常微分方程特解的一个注记
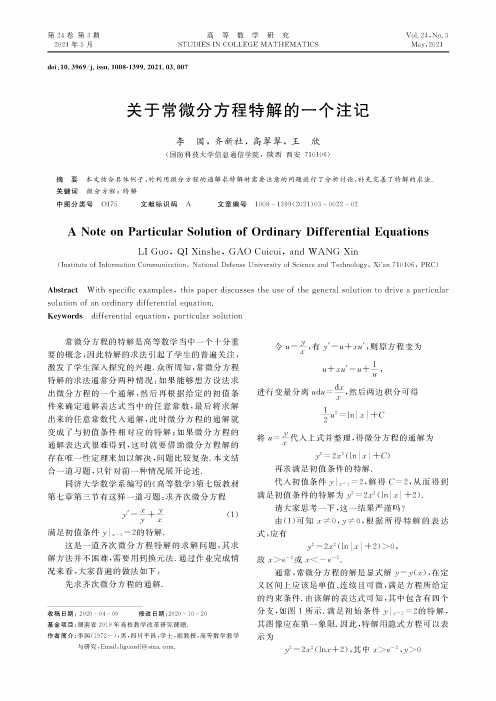
第24卷第3期2021年5月高等数学研究STUDIES IN COLLEGE MATHEMATICSVol.24,No.3May2021doi:10.3969/j.issn.1008-1399.2021.03.007关于常微分方程特解的一个注记李国,齐新社,高翠翠,王欣(国防科技大学信息通信学院,陕西西安710106)摘要本文结合具体例子,对利用微分方程的通解求特解时需要注意的问题进行了分析讨论,补充完善了特解的求法.关键词微分方程;特解中图分类号O175文献标识码A文章编号1008-1399(2021)03-0022-02A Note on Particular Solution of Ordinary Differential EquationsLI Guo,QI Xinshe,GAO Cuicui,and WANG Xin (InstituteofInformationCommunication!NationalDefenseUniversityofScienceandTechnology!>i'an710106!PRC)Abstract With specific examples,this paper discusses the use of the general solution to drive a particular solution of an ordinary differential equation.Keywords differential equation,particular solution常微分方程的特解是高等数学当中一个十分重要的概念,因此特解的求法引起了学生的普遍关注,激发了学生深入探究的兴趣.众所周知,常微分方程特解的求法通常分两种情况:如果能够想方设法求出微分方程的一个通解,然后再根据给定的初值条件来确定通解表达式当中的任意常数,最后将求解出来的任意常数代入通解,此时微分方程的通解就变成了与初值条件相对应的特解;如果微分方程的通解表达式很难得到,这时就要借助微分方程解的存在唯一性定理来加以解决,问题比较复杂•本文结合一道习题,只针对前一种情况展开论述•同济大学数学系编写的《高等数学》第七版教材第七章第三节有这样一道习题:求齐次微分方程'="+工(1)*"满足初值条件*"=1=2的特解•这是一道齐次微分方程特解的求解问题,其求解方法并不困难,需要用到换元法.通过作业完成情况来看,大家普遍的做法如下:先求齐次微分方程的通解•收稿日期:2020-04-09修改日期:2020-10-20基金项目:湖南省2019年高校教学改革研究课题.作者简介:李国(1972—),男,{川平昌,学士,副教授,高等数学教学与研究,Email:liguozdf@.令2=*,有*=2+"2,则原方程变为"2+"2=2+1,2进行变量分离”4"=",然后两边积分可得"2-22=In"+C将2="代入上式并整理,得微分方程的通解为"*2=2"2(In"+C)再求满足初值条件的特解•代入初值条件*|"-1=2,解得C=2,从而得到满足初值条件的特解为*z=2"z(ln"+2).请大家思考一下,这一结果严谨吗?由(1)可知"%0,*%0,根据所得特解的表达应有*2=2"2(In"+2)>0,故"#e—2或"V—e—2.通常,常微分方程的解是显式解*=*("),在定义区间上应该是单值、连续且可微,满足方程所给定的约束条件.由该解的表达式可知,其中包含有四个分支,如图1所示.满足初始条件*|"-1=2的特解,其图像应在第一象限•因此,特解用隐式方程可以表示为*z=2"z(ln"+2),其中">e—2,*>0第24卷第3期李国,齐新社,高翠翠,王欣:关于常微分方程特解的一个注记23或用显式形式可以表示为*=槡槡"(In"+2)2,乂,(e—2,+").如图2所示.同理,如果改变题目中的初值条件,那么特解的表达式也将发生相应地变化•根据以上的求解我们可以得到满足下列初值的特解为:(1)齐次微分方程*="+*满足初值条件*"*|"=—1=2的特解为*2=2"2'n(—")+2(这种情况下,特解始终位于第二象限,即"$—e-2,*〉0;(2)齐次微分方程*="+*满足初值条件*"*"=—1=—2的特解为*2=2"2[ln(—")+2(这种情况下,特解始终位于第三象限,即"$—e—29*$0;(3)齐次微分方程*="+*满足初值条件*"*L=1=—2的特解为*2=2"2(ln"+2),这种情况下,特解始终位于第四象限,即">e—2*$0.进一步,如果将原来的微分方程通过去分母变为"*)="2+*2(2)初始条件依然为*"=1=2其求通解的过程和上面的完全相同,特解的表达式仍然为*2=2"2(In"+2),和方程(1)的特解的不同之处在于,此时,坐标原点(0,0)变成了微分方程(2)的一个特解,而且点(—e—2,0)和点(e—2,0)也都在特解的积分曲线上,如图3所示.结合解的局部性知识进一步深入分析可知,满足初始条件*"=1=2的特解为*="(1"+2),和原微分方程(1)的特解的不同之处在于,此时"2e-2, *20,如图4所示.因此,在利用通解和初值条件来求微分方程特解的时候,不只是简单的求出通解当中的任意常数C就完成了任务,一定要考虑微分方程本身的特点.同时结合微分方程解的存在唯一性定理,兼顾解的局部性,对特解的结构进行深入分析研究.特别是当特解表达式中含有绝对值符号时,一定要谨慎从事,因地制宜对特解的结构进行有针对性地改造.通过以上几个例子的探讨可以看出,同一个微分方程在不同的初值条件下,将会得到不同的特解曲线分支.因此在微分方程特解的教学过程中,一方面,要时刻提醒学生,千万不能忽略初值条件对特解的影响;另一方面,在传授知识的过程中,对学生积极进行价值观的塑造•在这节课的教学设计中,可以有意识的融入课程思政教学理念,教育引导学生一定要不忘初心,牢记使命,方能成就一番事业•参考文献同济大学数学教研室,高等数学[M(7版.北京:高等教育岀版社,2014.姚克俭,常微分方程定性和稳定性方法[M(北京:科学版社2014。
求解常微分方程组的几种方法
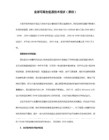
求解常微分方程组的几种方法金晓龙(广东女子职业技术学院 艺信系, 广东 广州 511450)摘要:在建立实际问题的数学模型时,常需要建立各物理量随某自变量变化的常微分方程组及求解,由于处理问题时使用的编程语言不同,经常会遇到如下问题:各种语言如何处理该问题?常用的处理工具?编程语言与工具软件的参数传递? 关键词:常微分方程组 数值解 龙格-库塔法 Matlab 中图分类号:TP311Some Solution of Ordinary Differential EquationsJin Xiaolong(Guangdong Women ’s Polytechnic College, Art and Information D epartment, Guangzhou 511450,China )Abstract :In establishing the the mathematical model of practical problems ,it ofen need to establish the ordinary differential equations which physical quantities change over time , solve the ordinary differential equations for the numerical solutions. Because of using various programming languages,it ofen to encounter the following problems.How to solve the problem by various languages? What is the common tool software? How to pass parameters between programming language and tool software? Key words :ordinary differential equations ;numerical solution ;Runge-Kutta method ;Matlab 1 引言在科学应用中,常需要建立实际问题的数学模型,建立各种变量的常微分方程组及对其求解。
一阶常微分方程

Chapter 1 First-order ordinary differential equations (ODE)一階常微分方程1.1 基本概念()x f y =或()t f y =,y 是x 或t 的函數,y 是因變數(dependent variable ),x 或t 是自變數(independent variable )◎ 微分方程(differential equations):一方程式包含有因變數y 關於自變數x 或t的導數(derivatives)y y ′ ,&或微分(differentials)dy 。
◎ 常微分方程(ordinary differential equations, ODE):一微分方程包含有一個或數個因變數(通常為()x y )關於僅有一個自變數x 的導數。
Ex. 222)2(2 ,09 ,cos y x y e y y x y y x y x +=′′+′′′′=+′′=′◎ 偏微分方程(partial differential equations, PDE):一微分方程包含至少有一個因變數關於兩個以上自變數的部分導數。
Ex. 02222=∂∂+∂∂yux u◎ 微分方程的階數:在微分方程式中所出現最高階導數的階數。
◎ 線性微分方程:在微分方程式中所出現的因變數因變數因變數或其導數僅有一次式(first degree)而無二次以上的乘積(自變數可以有二次以上的乘積)。
Ex. x y y x y cos 24=+′+′′ 因變數:y ,自變數:x ,二階線性常微分方程 x y y y y cos 24=+′+′′ 因變數:y ,自變數:x ,二階非線性常微分方程 222)2(2 y x y e y y x x +=′′+′′′′ 因變數:y ,自變數:x ,三階非線性常微分方程□ 一階常微分方程(first-order ordinary differential equations)隱式形式(implicit form) 表示 0),,(=′y y x F (4)顯式形式(explicit form) 表示 ),(y x f y =′Ex. 隱式形式ODE 0423=−′−y y x ,當0≠x 時,可表示為顯式形式234y x y =′□ 解的概念(concept of solution)在某些開放間隔區間b x a <<,一函數)(x h y =是常微分方程常微分方程0),,(=′y y x F 的解,其函數)(x h 在此區間b x a <<是明確(defined)且可微分的(differentiable),其)(x h 的曲線(或圖形)是被稱為解答曲線(solution curve)。
数学专业英语(Doc版).Word6
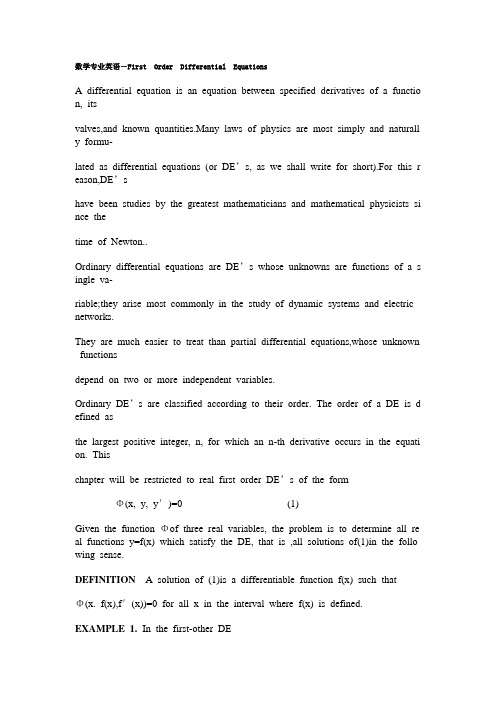
数学专业英语-First Order Differential EquationsA differential equation is an equation between specified derivatives of a functio n, itsvalves,and known quantities.Many laws of physics are most simply and naturall y formu-lated as differential equations (or DE’s, as we shall write for short).For this r eason,DE’shave been studies by the greatest mathematicians and mathematical physicists si nce thetime of Newton..Ordinary differential equations are DE’s whose unknowns are functions of a s ingle va-riable;they arise most commonly in the study of dynamic systems and electric networks.They are much easier to treat than partial differential equations,whose unknown functionsdepend on two or more independent variables.Ordinary DE’s are classified according to their order. The order of a DE is d efined asthe largest positive integer, n, for which an n-th derivative occurs in the equati on. Thischapter will be restricted to real first order DE’s of the formΦ(x, y, y′)=0 (1)Given the function Φof three real variables, the problem is to determine all re al functions y=f(x) which satisfy the DE, that is ,all solutions of(1)in the follo wing sense.DEFINITION A solution of (1)is a differentiable function f(x) such thatΦ(x. f(x),f′(x))=0 for all x in the interval where f(x) is defined.EXAMPLE 1. In the first-other DEthe function Φis a polynomial function Φ(x, y, z)=x+ yz of three variables i n-volved. The solutions of (2) can be found by considering the identityd(x²+y²)/d x=2(x+yyˊ).From this identity,one sees that x²+y²is a con-stant if y=f(x) is any solution of (2).The equation x²+y²=c defines y implicitly as a two-valued function of x,for any positive constant c.Solving for y,we get two solutions,the(single-valued) functions y=±(c-x²)0.5,for each positive constant c.The graphs of these so-lutions,the so-called solution curves,form two families of scmicircles,which fill t he upper half-plane y>0 and the lower half-plane y>0,respectively.On the x-axis,where y=0,the DE(2) implies that x=0.Hence the DE has no solu tionswhich cross the x-axis,except possibly at the origin.This fact is easily overlook ed,because the solution curves appear to cross the x-axis;hence yˊdoes not exist, and the DE (2) is not satisfied there.The preceding difficulty also arises if one tries to solve the DE(2)for yˊ. Div iding through by y,one gets yˊ=-x/y,an equation which cannot be satisfied if y=0.The preceding difficulty is thus avoided if one restricts attention to regions where the DE(1) is normal,in the following sense.DEFINITION. A normal first-order DE is one of the formyˊ=F(x,y) (3)In the normal form yˊ=-x/y of the DE (2),the function F(x,y) is continuous i n the upper half-plane y>0 and in the lower half-plane where y<0;it is undefin ed on the x-axis.Fundamental Theorem of the Calculus.The most familiar class of differential equations consists of the first-order DE’s of the formSuch DE’s are normal and their solutions are descried by the fundamental tho rem of the calculus,which reads as follows.FUNDAMENTAL THEOREM OF THE CALCULUS. Let the function g(x)i n DE(4) be continuous in the interval a<x<b.Given a number c,there is one an d only one solution f(x) of the DE(4) in the interval such that f(a)=c. This sol ution is given by the definite integralf(x)=c+∫a x g(t)dt , c=f(a) (5)This basic result serves as a model of rigorous formulation in several respects. First,it specifies the region under consideration,as a vertical strip a<x<b in the xy-plane.Second,it describes in precise terms the class of functions g(x) consid ered.And third, it asserts the existence and uniqueness of a solution,given the “initial condition”f(a)=c.We recall that the definite integral∫a x g(t)dt=lim(maxΔt k->0)Σg(t k)Δt k , Δt k=t k-t k-1 (5ˊ)is defined for each fixed x as a limit of Ricmann sums; it is not necessary to find a formal expression for the indefinite integral ∫g(x) dx to give meanin g to the definite integral ∫a x g(t)dt,provided only that g(t) is continuous.Such f unctions as the error function crf x =(2/(π)0.5)∫0x e-t²dt and the sine integral f unction SI(x)=∫x∞[(sin t )/t]dt are indeed commonly defined as definite int egrals.Solutions and IntegralsAccording to the definition given above a solution of a DE is always a functi on. For example, the solutions of the DE x+yyˊ=0 in Example I are the func tions y=±(c-x²)0.5,whose graphs are semicircles of arbitrary diameter,centered at the origin.The graph of the solution curves are ,however,more easily describ ed by the equation x²+y²=c,describing a family of circles centered at the origi n.In what sense can such a family of curves be considered as a solution of th e DE ?To answer this question,we require a new notion.DEFINITION. An integral of DE(1)is a function of two variables,u(x,y),whic h assumes a constant value whenever the variable y is replaced by a solution y=f(x) of the DE.In the above example, the function u(x,y)=x²+y²is an integral of the DE x +yyˊ=0,because,upon replacing the variable y by any function ±( c-x²)0.5,we obtain u(x,y)=c.The second-order DEd²x/dt²=-x (2ˊ)becomes a first-order DE equivalent to (2) after setting dx/dx=y:y ( dy/dx )=-x (2)As we have seen, the curves u(x,y)=x²+y²=c are integrals of this DE.When th e DE (2ˊ)is interpreted as equation of motion under Newton’s second law,the integrals c=x²+y²represent curves of constant energy c.This illustrates an important prin ciple:an integral of a DE representing some kind of motion is a quantity that r emains unchanged through the motion.Vocabularydifferential equation 微分方程 error function 误差函数ordinary differential equation 常微分方程 sine integral function 正弦积分函数order 阶,序 diameter 直径derivative 导数 curve 曲线known quantities 已知量replace 替代unknown 未知量substitute 代入single variable 单变量strip 带形dynamic system 动力系统 exact differential 恰当微分electric network 电子网络line integral 线积分partial differential equation 偏微分方程path of integral 积分路径classify 分类 endpoints 端点polynomial 多项式 general solution 通解several variables 多变量parameter 参数family 族rigorous 严格的semicircle 半圆 existence 存在性half-plane 半平面 initial condition 初始条件region 区域uniqueness 唯一性normal 正规,正常Riemann sum 犁曼加identity 恒等(式)Notes1. The order of a DE is defined as the largest positive integral n,for which an nth derivative occurs i n the question.这是另一种定义句型,请参看附录IV.此外要注意nth derivative 之前用an 不用a .2. This chapter will be restricted to real first order differential equations of the formΦ(x,y,yˊ)=0意思是;文章限于讨论形如Φ(x,y,yˊ)=0的实一阶微分方程.有时可以用of the type代替of the form 的用法.The equation can be rewritten in the form yˊ=F(x,y).3. Dividing through by y,one gets yˊ=-x/y,…划线短语意思是:全式除以y4. As we have seen, the curves u(x,y)=x²+y²=c are integrals of this DE这里x²+y²=c 因c是参数,故此方程代表一族曲线,由此”曲线”这一词要用复数curves.5. Their solutions are described by the fundamental theorem of the calculus,which reads as follows.意思是:它们的解由微积分基本定理所描述,(基本定理)可写出如下.句中reads as follows 就是”写成(读成)下面的样子”的意思.注意follows一词中的”s”不能省略.ExerciseⅠ.Translate the following passages into Chinese:1.A differential M(x,y) dx +N(x,y) dy ,where M, N are real functions of two variables x and y, is called exact in a domain D when the line integral ∫c M(x,y) dx +N(x,y) dy is the same for all paths of int egration c in D, which have the same endpoints.Mdx+Ndy is exact if and only if there exists a continuously differentiable function u(x,y) such that M= u/ x, N=u/ y.2. For any normal first order DE yˊ=F(x,y) and any initial x0 , the initial valve problem consists of finding the solution or solutions of the DE ,for x>x0 which assumes a given initial valve f(x0)=c.3. To show that the initial valve problem is well-set requires proving theorems of existence (there isa solution), uniqueness (there is only one solution) and continuity (the solution depends continuously on t he initial value).Ⅱ. Translate the following sentences into English:1) 因为y=ч(x) 是微分方程dy/ dx=f(x,y)的解,故有dч(x)/dx=f (x,ч(x))2) 两边从x0到x取定积分得ч(x)-ч(x0)=∫x0x f(x,ч(x)) dx x0<x<x0+h3) 把y0=ч(x0)代入上式, 即有ч(x)=y0+∫x0x f(x,ч(x)) dx x0<x<x0+h4) 因此y=ч(x) 是积分方程y=y0+∫x0x f (x,y) dx定义于x0<x<x0+h 的连续解.Ⅲ. Translate the following sentences into English:1) 现在讨论型如 y=f (x,yˊ) 的微分方程的解,这里假设函数f (x, dy/dx) 有连续的偏导数.2) 引入参数dy/dx=p, 则已给方程变为y=f (x,p).3) 在y=f (x,p) x p=dy/dx p= f/ x+f/ p dp/dx4) 这是一个关于x和p的一阶微分方程,它的解法我们已经知道.5) 若(A)的通解的形式为p=ч(x,c) ,则原方程的通解为y=f (x,ч(x,c)).6) 若(A) 有型如x=ψ(x,c)的通解,则原方程有参数形式的通解 x=ψ(p,c)y=f(ψ(p,c)p)其中p是参数,c是任意常数.。
常微分方程课件,中山版
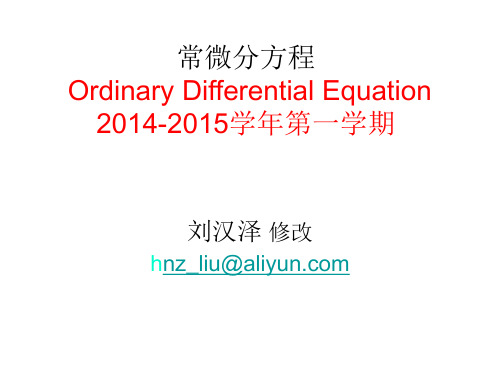
证明: 对y sinx,由于
y y sin x sin x 0
"
y cosx,y sin x 故对x (, ), 有
' "
故y sinx是微分方程 y" y 0在(,)上的一个解 . 同理y cosx是微分方程 y" y 0在(,)上的一个解 .
与其他学科的关系
• 常微分方程的形成与发展是和力学、天文学、物 理学,以及其他科学技术的发展密切相关的 • 数学的其他分支的新发展,如复变函数、李群、 组合拓扑学等,都对常微分方程的发展产生了深 刻的影响 • 当前计算机的发展更是为常微分方程的应用及理 论研究提供了非常有力的工具
1.1 常微分方程模型
SI模型 易感染者:Susceptible 已感染者:Infective
SIS模型
• 对无免疫性的传染病,假设病人治愈后会再次被 感染,设单位时间治愈率为mu
SIR模型(R:移出者(Removed))
• 对有很强免疫性的传染病,假设病人治愈后不会在 被感染,设在时刻t的愈后免疫人数为r(t),称为移出 者,而治愈率l为常数
如
dy (2) xdy ydx 0 ; (1) 2 x; dx 3 2 d x dx (3) tx x 0; 2 dt dt
d 4x d 2x (4) 5 2 3x sin t; 4 dt dt
都是常微分方程
偏微分方程
如果在一个微分方程中,自变量的个数为两个或两 个以上,称为偏微分方程,PDE 如
n阶线性微分方程的一般形式
d y d y a1 ( x) n1 an ( x) y f ( x) n dx dx
高阶常系数齐次线性微分方程的解法
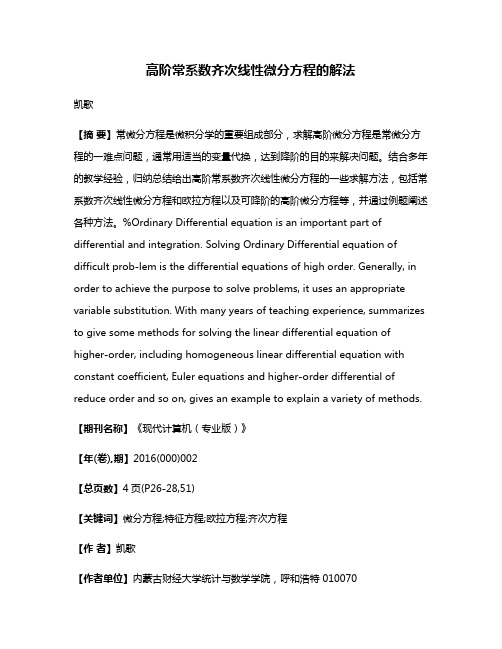
高阶常系数齐次线性微分方程的解法凯歌【摘要】常微分方程是微积分学的重要组成部分,求解高阶微分方程是常微分方程的一难点问题,通常用适当的变量代换,达到降阶的目的来解决问题。
结合多年的教学经验,归纳总结给出高阶常系数齐次线性微分方程的一些求解方法,包括常系数齐次线性微分方程和欧拉方程以及可降阶的高阶微分方程等,并通过例题阐述各种方法。
%Ordinary Differential equation is an important part of differential and integration. Solving Ordinary Differential equation of difficult prob-lem is the differential equations of high order. Generally, in order to achieve the purpose to solve problems, it uses an appropriate variable substitution. With many years of teaching experience, summarizes to give some methods for solving the linear differential equation of higher-order, including homogeneous linear differential equation with constant coefficient, Euler equations and higher-order differential of reduce order and so on, gives an example to explain a variety of methods.【期刊名称】《现代计算机(专业版)》【年(卷),期】2016(000)002【总页数】4页(P26-28,51)【关键词】微分方程;特征方程;欧拉方程;齐次方程【作者】凯歌【作者单位】内蒙古财经大学统计与数学学院,呼和浩特 010070【正文语种】中文求解常微分方程的问题,常常通过变量分离、两边积分,如果是高阶微分方程则通过适当的变量代换,达到降阶的目的来解决问题。
Ordinarydifferentialequations
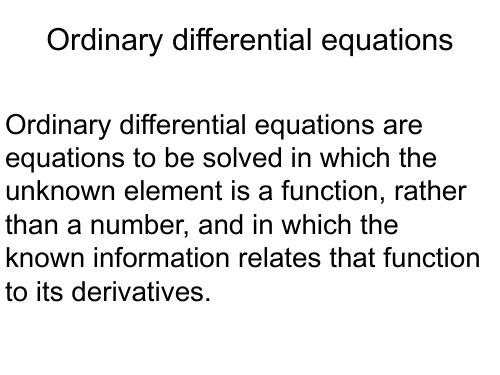
• Theorem. Let M(x,y) and N(x,y) be continuous and have continuous partial derivatives with respect x and y in the rectangle R consisting of those points (x,y) with a<x<b and c<y<d. There exists a function Q(x,y) such that
• Then, the initial-value problem
• dy/dx=f(x,y), y(x0)= y0
(6)
• has a unique solution y(x) on the interval
x0=< x <= x0 + α. In other words, if y(x) and z(x) are two solutions of (6), the y(x)
these functions is a constant multiple of the
other on I. The functions y1(x) and y2(x) are said to be linear independent on an interval I if there
are not linear dependent on I.
Review of terms for classifying firstorder ODEs.
• Although this really means F(x, y, dy/dx) = 0 for some F of 3 variables, all further adjectives assume the equation has the form dy/dx = f(x,y) for some real-valued f defined on a subset of R^2.
Ordinarydifferentialequation
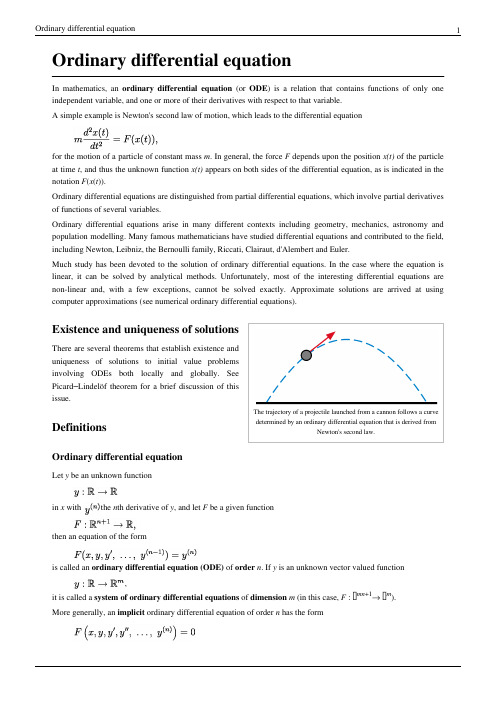
Ordinary differential equationIn mathematics, an ordinary differential equation (or ODE ) is a relation that contains functions of only one independent variable, and one or more of their derivatives with respect to that variable.A simple example is Newton's second law of motion, which leads to the differential equationfor the motion of a particle of constant mass m . In general, the force F depends upon the position x(t) of the particle at time t , and thus the unknown function x(t) appears on both sides of the differential equation, as is indicated in the notation F (x (t )).Ordinary differential equations are distinguished from partial differential equations, which involve partial derivatives of functions of several variables.Ordinary differential equations arise in many different contexts including geometry, mechanics, astronomy and population modelling. Many famous mathematicians have studied differential equations and contributed to the field,including Newton, Leibniz, the Bernoulli family, Riccati, Clairaut, d'Alembert and Euler.Much study has been devoted to the solution of ordinary differential equations. In the case where the equation is linear, it can be solved by analytical methods. Unfortunately, most of the interesting differential equations are non-linear and, with a few exceptions, cannot be solved exactly. Approximate solutions are arrived at using computer approximations (see numerical ordinary differential equations).The trajectory of a projectile launched from a cannon follows a curve determined by an ordinary differential equation that is derived fromNewton's second law.Existence and uniqueness of solutionsThere are several theorems that establish existence anduniqueness of solutions to initial value problemsinvolving ODEs both locally and globally. SeePicard –Lindelöf theorem for a brief discussion of thisissue.DefinitionsOrdinary differential equationLet ybe an unknown function in x with the n th derivative of y , and let Fbe a given functionthen an equation of the formis called an ordinary differential equation (ODE) of order n . If y is an unknown vector valued function,it is called a system of ordinary differential equations of dimension m (in this case, F : ℝmn +1→ ℝm ).More generally, an implicit ordinary differential equation of order nhas the formwhere F : ℝn+2→ ℝ depends on y(n). To distinguish the above case from this one, an equation of the formis called an explicit differential equation.A differential equation not depending on x is called autonomous.A differential equation is said to be linear if F can be written as a linear combination of the derivatives of y together with a constant term, all possibly depending on x:(x) and r(x) continuous functions in x. The function r(x) is called the source term; if r(x)=0 then the linear with aidifferential equation is called homogeneous, otherwise it is called non-homogeneous or inhomogeneous. SolutionsGiven a differential equationa function u: I⊂ R→ R is called the solution or integral curve for F, if u is n-times differentiable on I, andGiven two solutions u: J⊂ R→ R and v: I⊂ R→ R, u is called an extension of v if I⊂ J andA solution which has no extension is called a global solution.A general solution of an n-th order equation is a solution containing n arbitrary variables, corresponding to n constants of integration. A particular solution is derived from the general solution by setting the constants to particular values, often chosen to fulfill set 'initial conditions or boundary conditions'. A singular solution is a solution that can't be derived from the general solution.Reduction to a first order systemAny differential equation of order n can be written as a system of n first-order differential equations. Given an explicit ordinary differential equation of order n (and dimension 1),define a new family of unknown functionsfor i from 1 to n.The original differential equation can be rewritten as the system of differential equations with order 1 and dimension n given bywhich can be written concisely in vector notation aswithandLinear ordinary differential equationsA well understood particular class of differential equations is linear differential equations. We can always reduce an explicit linear differential equation of any order to a system of differential equation of order 1which we can write concisely using matrix and vector notation aswithHomogeneous equationsThe set of solutions for a system of homogeneous linear differential equations of order 1 and dimension nforms an n-dimensional vector space. Given a basis for this vector space , which is called a fundamental system, every solution can be written asThe n × n matrixis called fundamental matrix. In general there is no method to explicitly construct a fundamental system, but if one solution is known d'Alembert reduction can be used to reduce the dimension of the differential equation by one.Nonhomogeneous equationsThe set of solutions for a system of inhomogeneous linear differential equations of order 1 and dimension ncan be constructed by finding the fundamental system to the corresponding homogeneous equation and one particular solution to the inhomogeneous equation. Every solution to nonhomogeneous equation can then be written asA particular solution to the nonhomogeneous equation can be found by the method of undetermined coefficients or the method of variation of parameters.Concerning second order linear ordinary differential equations, it is well known thatSo, if is a solution of: , then such that:So, if is a solution of: ; then a particular solution of , isgiven by:. [1]Fundamental systems for homogeneous equations with constant coefficientsIf a system of homogeneous linear differential equations has constant coefficientsthen we can explicitly construct a fundamental system. The fundamental system can be written as a matrix differential equationwith solution as a matrix exponentialwhich is a fundamental matrix for the original differential equation. To explicitly calculate this expression we first transform A into Jordan normal formand then evaluate the Jordan blocksof J separately asTheories of ODEsSingular solutionsThe theory of singular solutions of ordinary and partial differential equations was a subject of research from the time of Leibniz, but only since the middle of the nineteenth century did it receive special attention. A valuable but little-known work on the subject is that of Houtain (1854). Darboux (starting in 1873) was a leader in the theory, and in the geometric interpretation of these solutions he opened a field which was worked by various writers, notably Casorati and Cayley. To the latter is due (1872) the theory of singular solutions of differential equations of the first order as accepted circa 1900.Reduction to quadraturesThe primitive attempt in dealing with differential equations had in view a reduction to quadratures. As it had been the hope of eighteenth-century algebraists to find a method for solving the general equation of the th degree, so it was the hope of analysts to find a general method for integrating any differential equation. Gauss (1799) showed, however, that the differential equation meets its limitations very soon unless complex numbers are introduced. Hence analysts began to substitute the study of functions, thus opening a new and fertile field. Cauchy was the first to appreciate the importance of this view. Thereafter the real question was to be, not whether a solution is possible by means of known functions or their integrals, but whether a given differential equation suffices for the definition of a function of the independent variable or variables, and if so, what are the characteristic properties of this function.Fuchsian theoryTwo memoirs by Fuchs (Crelle, 1866, 1868), inspired a novel approach, subsequently elaborated by Thomé and Frobenius. Collet was a prominent contributor beginning in 1869, although his method for integrating a non-linear system was communicated to Bertrand in 1868. Clebsch (1873) attacked the theory along lines parallel to those followed in his theory of Abelian integrals. As the latter can be classified according to the properties of the fundamental curve which remains unchanged under a rational transformation, so Clebsch proposed to classify the transcendent functions defined by the differential equations according to the invariant properties of the corresponding surfaces f = 0 under rational one-to-one transformations.Lie's theoryFrom 1870 Sophus Lie's work put the theory of differential equations on a more satisfactory foundation. He showed that the integration theories of the older mathematicians can, by the introduction of what are now called Lie groups, be referred to a common source; and that ordinary differential equations which admit the same infinitesimal transformations present comparable difficulties of integration. He also emphasized the subject of transformations of contact.A general approach to solve DE's uses the symmetry property of differential equations, the continuous infinitesimal transformations of solutions to solutions (Lie theory). Continuous group theory, Lie algebras and differential geometry are used to understand the structure of linear and nonlinear (partial) differential equations for generating integrable equations, to find its Lax pairs, recursion operators, Bäcklund transform and finally finding exact analytic solutions to the DE.Symmetry methods have been recognized to study differential equations arising in mathematics, physics, engineering, and many other disciplines.Sturm–Liouville theorySturm–Liouville theory is a theory of eigenvalues and eigenfunctions of linear operators defined in terms of second-order homogeneous linear equations, and is useful in the analysis of certain partial differential equations.Software for ODE solving•FuncDesigner (free license: BSD, uses Automatic differentiation, also can be used online via Sage-server [2])•VisSim [3] - a visual language for differential equation solving•Mathematical Assistant on Web [4] online solving first order (linear and with separated variables) and second order linear differential equations (with constant coefficients), including intermediate steps in the solution.•DotNumerics: Ordinary Differential Equations for C# and [5] Initial-value problem for nonstiff and stiff ordinary differential equations (explicit Runge-Kutta, implicit Runge-Kutta, Gear’s BDF and Adams-Moulton).•Online experiments with JSXGraph [6]References[1]Polyanin, Andrei D.; Valentin F. Zaitsev (2003). Handbook of Exact Solutions for Ordinary Differential Equations, 2nd. Ed.. Chapman &Hall/CRC. ISBN 1-5848-8297-2.[2]/welcome[3][4]http://user.mendelu.cz/marik/maw/index.php?lang=en&form=ode[5]/NumericalLibraries/DifferentialEquations/[6]http://jsxgraph.uni-bayreuth.de/wiki/index.php/Differential_equationsBibliography• A. D. Polyanin and V. F. Zaitsev, Handbook of Exact Solutions for Ordinary Differential Equations (2nd edition)", Chapman & Hall/CRC Press, Boca Raton, 2003. ISBN 1-58488-297-2• A. D. Polyanin, V. F. Zaitsev, and A. Moussiaux, Handbook of First Order Partial Differential Equations, Taylor & Francis, London, 2002. ISBN 0-415-27267-X• D. Zwillinger, Handbook of Differential Equations (3rd edition), Academic Press, Boston, 1997.•Hartman, Philip, Ordinary Differential Equations, 2nd Ed., Society for Industrial & Applied Math, 2002. ISBN 0-89871-510-5.•W. Johnson, A Treatise on Ordinary and Partial Differential Equations (/cgi/b/bib/ bibperm?q1=abv5010.0001.001), John Wiley and Sons, 1913, in University of Michigan Historical Math Collection (/u/umhistmath/)• E.L. Ince, Ordinary Differential Equations, Dover Publications, 1958, ISBN 0486603490•Witold Hurewicz, Lectures on Ordinary Differential Equations, Dover Publications, ISBN 0-486-49510-8•Ibragimov, Nail H (1993), CRC Handbook of Lie Group Analysis of Differential Equations Vol. 1-3, Providence: CRC-Press, ISBN 0849344883.External links•Differential Equations (/Science/Math/Differential_Equations//) at the Open Directory Project (includes a list of software for solving differential equations).•EqWorld: The World of Mathematical Equations (http://eqworld.ipmnet.ru/index.htm), containing a list of ordinary differential equations with their solutions.•Online Notes / Differential Equations (/classes/de/de.aspx) by Paul Dawkins, Lamar University.•Differential Equations (/diffeq/diffeq.html), S.O.S. Mathematics.• A primer on analytical solution of differential equations (/mws/gen/ 08ode/mws_gen_ode_bck_primer.pdf) from the Holistic Numerical Methods Institute, University of South Florida.•Ordinary Differential Equations and Dynamical Systems (http://www.mat.univie.ac.at/~gerald/ftp/book-ode/ ) lecture notes by Gerald Teschl.•Notes on Diffy Qs: Differential Equations for Engineers (/diffyqs/) An introductory textbook on differential equations by Jiri Lebl of UIUC.Article Sources and Contributors7Article Sources and ContributorsOrdinary differential equation Source: /w/index.php?oldid=433160713 Contributors: 48v, A. di M., Absurdburger, AdamSmithee, After Midnight, Ahadley,Ahoerstemeier,AlfyAlf,Alll,AndreiPolyanin,Anetode,Ap,Arthena,ArthurRubin,BL,BMF81,********************,Bemoeial,BenFrantzDale,Benjamin.friedrich,BereanHunter,Bernhard Bauer, Beve, Bloodshedder, Bo Jacoby, Bogdangiusca, Bryan Derksen, Charles Matthews, Chilti, Chris in denmark, ChrisUK, Christian List, Cloudmichael, Cmdrjameson, Cmprince, Conversion script, Cpuwhiz11, Cutler, Delaszk, Dicklyon, DiegoPG, Dmitrey, Dmr2, DominiqueNC, Dominus, Donludwig, Doradus, Dysprosia, Ed Poor, Ekotkie, Emperorbma, Enochlau, Fintor, Fruge, Fzix info, Gauge, Gene s, Gerbrant, Giftlite, Gombang, HappyCamper, Heuwitt, Hongsichuan, Ht686rg90, Icairns, Isilanes, Iulianu, Jack in the box, Jak86, Jao, JeLuF, Jitse Niesen, Jni, JoanneB, John C PI, Jokes Free4Me, JonMcLoone, Josevellezcaldas, Juansempere, Kawautar, Kdmckale, Krakhan, Kwantus, L-H, LachlanA, Lethe, Linas, Lingwitt, Liquider, Lupo, MarkGallagher,MathMartin, Matusz, Melikamp, Michael Hardy, Mikez, Moskvax, MrOllie, Msh210, Mtness, Niteowlneils, Oleg Alexandrov, Patrick, Paul August, Paul Matthews, PaulTanenbaum, Pdenapo, PenguiN42, Phil Bastian, PizzaMargherita, Pm215, Poor Yorick, Pt, Rasterfahrer, Raven in Orbit, Recentchanges, RedWolf, Rich Farmbrough, Rl, RobHar, Rogper, Romanm, Rpm, Ruakh, Salix alba, Sbyrnes321, Sekky, Shandris, Shirt58, SilverSurfer314, Ssd, Starlight37, Stevertigo, Stw, Susvolans, Sverdrup, Tarquin, Tbsmith, Technopilgrim, Telso, Template namespace initialisation script, The Anome, Tobias Hoevekamp, TomyDuby, TotientDragooned, Tristanreid, Twin Bird, Tyagi, Ulner, Vadimvadim, Waltpohl, Wclxlus, Whommighter, Wideofthemark, WriterHound, Xrchz, Yhkhoo, 今古庸龍, 176 anonymous editsImage Sources, Licenses and ContributorsImage:Parabolic trajectory.svg Source: /w/index.php?title=File:Parabolic_trajectory.svg License: Public Domain Contributors: Oleg AlexandrovLicenseCreative Commons Attribution-Share Alike 3.0 Unported/licenses/by-sa/3.0/。
- 1、下载文档前请自行甄别文档内容的完整性,平台不提供额外的编辑、内容补充、找答案等附加服务。
- 2、"仅部分预览"的文档,不可在线预览部分如存在完整性等问题,可反馈申请退款(可完整预览的文档不适用该条件!)。
- 3、如文档侵犯您的权益,请联系客服反馈,我们会尽快为您处理(人工客服工作时间:9:00-18:30)。
6 ORDINARY DIFFERENTIAL EQUATIONSDifferential equations are mathematical descriptions of how the variables and their derivatives (rates of change) with respect to one or more independent variable affect each other in a dynamical way. Their solutions show us how the dependent variable(s) will change with the independent variable(s). Many problems in natural sciences and engineering fields are formulated into a scalar differential equation or a vector differential equation—that is, a system of differential equations. In this chapter, we look into several methods of obtaining the numerical solutions to ordinary differential equations (ODEs) in which all dependent variables (x) depend on a single independent variable (t). First, the initial value problems (IVPs) will be handled with several methods including Runge–Kutta method and predictor–corrector methods in Sections 6.1 to 6.5. The final section (Section 6.6) will introduce the shooting method and the finite difference and collocation method for solving the two-point boundary value problem (BVP). ODEs are called an IVP if the values x(t0)of dependent variables are given at the initial point t0 of the independent variable, while they are called a BVP if the values x(t0) and x(t f)are given at the initial/final points t0 and t f.6.1 EULER’S METHODWhen talking about the numerical solutions to ODEs, everyone starts with the Euler’s method, since it is easy to understand and simple to program. Even though its low accuracy keeps it from being widely used for solving ODEs, it gives us a clue to the basic concept of numerical solution for a differential equation simply and clearly. Let’s consider a first-order differential equation:It has the following form of analytical solution:which can be obtained by using a conventional method or the Laplace transform technique. However, such a nice analytical solution does not exist for every differential equation; even if it exists, it is not easy to find even by using a computer equipped with the capability of symboliccomputation. That is why we should study the numerical solutions to differential equations.Then, how do we translate the differential equation into a form that can easily be handled by computer? First of all, we have to replace the derivative y’(t)= dy/dt in the differential equation by a numerical derivative (introduced before), where the step-size h is determined based on the accuracy requirements and the computation time constraints. Euler’s method approximates the derivative in Eq. (6.1.1) with Eq. (5.1.2) asand solves this difference equation step-by-step with increasing t by h each time from t = 0.This is a numeric sequence {y(kh)}, which we call a numerical solution of Eq. (6.1.1).To be specific, let the parameters and the initial value of Eq. (6.1.1) be a = 1, r = 1, and y0 = 0. Then, the analytical solution (6.1.2) becomesy(t)= 1 −e−at(6.1.5) and the numerical solution (6.1.4) with the step-size h = 0.5 and h = 0.25 are as listed in Table 6.1 and depicted in Fig. 6.1. We make a MATLAB program “nm610.m”, which uses Euler’s method for the differential equation (6.1.1), actually solving the difference equation (6.1.3) and plots the graphs of the numerical solutions in Fig. 6.1.%nm610: Euler method to solve a 1st-order differential equationclear, clfa = 1; r = 1; y0 = 0; tf = 2;t = [0:0.01:tf]; yt = 1 - exp(-a*t); %Eq.(6.1.5): true analytical solutionplot(t,yt,’k’), hold onklasts = [8 4 2]; hs = tf./klasts;y(1) = y0;for itr = 1:3 %with various step size h = 1/8,1/4,1/2 klast = klasts(itr); h = hs(itr); y(1)=y0;for k = 1:klasty(k + 1) = (1 - a*h)*y(k) +h*r; %Eq.(6.1.3):plot([k - 1 k]*h,[y(k) y(k+1)],’b’, k*h,y(k+1),’ro’)if k < 4, pause; endendendFigure 6.1 Examples of numerical solution obtained by using the Euler’s method.The graphs seem to tell us that a small step-size helps reduce the error so as to make the numerical solution closer to the (true) analytical solution. But, as will be investigated thoroughly in Section 6.2, it is only partially true. In fact, a too small step-size not only makes the computation time longer (proportional as 1/h), but also results in rather larger errors due to the accumulated round-off effect.This is why we should look for other methods to decrease the errors rather than simply reduce the step-size.Euler’s method can also be applied for solving a first-order vector differential equationwhich is equivalent to a high-order scalar differential equation. The algorithm can be described byand is cast into the MATLAB routine “ode_Euler()”.function [t,y] = ode_Euler(f,tspan,y0,N)%Euler’s method to solve vector differential equation y’(t) = f(t,y(t)) % for tspan = [t0,tf] and with the initial value y0 and N time stepsif nargin<4 | N <= 0, N = 100; endif nargin<3, y0 = 0; endh = (tspan(2) - tspan(1))/N; %stepsizet = tspan(1)+[0:N]’*h; %time vectory(1,:) = y0(:)’; %al ways make the initial value a row vectorfor k = 1:Ny(k + 1,:) = y(k,:) +h*feval(f,t(k),y(k,:)); %Eq.(6.1.7)end6.2 HEUN’S METHOD: TRAPEZOIDAL METHODAnother method of solving a first-order vector differential equation like Eq. (6.1.6) comes from integrating both sides of the equation.If we assume that the value of the (derivative) function f(t ,y) is constant as f(t k,y(t k)) within one time step [t k,t k+1), this becomes Eq. (6.1.7) (with h = t k+1−t k), amounting to Euler’s method. If we use the trapezoidal rule (5.5.3), it becomesfunction [t,y] = ode_Heun(f,tspan,y0,N)%Heun method to solve vector differential equation y’(t) = f(t,y(t)) % for tspan = [t0,tf] and with the initial value y0 and N time steps if nargin<4 | N <= 0, N = 100; endif nargin<3, y0 = 0; endh = (tspan(2) - tspan(1))/N; %stepsizet = tspan(1)+[0:N]’*h; %time vectory(1,:) = y0(:)’; %always make the initial value a row vectorfor k = 1:Nfk = feval(f,t(k),y(k,:)); y(k+1,:) = y(k,:)+h*fk; %Eq.(6.2.3)y(k+1,:) = y(k,:) +h/2*(fk +feval(f,t(k+1),y(k+1,:))); %Eq.(6.2.4) endBut, the right-hand side (RHS) of this equation has y k+1, which is unknown at t k. To resolve this problem, we replace the y k+1 on the RHS by the following Euler’s approximation:so that it becomesThis is Heu n’s method, which is implemented in the MATLAB routine “ode_Heun()”. It is a kind of predictor-and-corrector method in that it predicts the value of y k+1 by Eq. (6.2.3) at t k and then corrects the predicted value by Eq. (6.2.4) at t k+1. The truncation erro r of Heun’s method is O(h2)(proportional to h2) as shown in Eq. (5.6.1), while the error of Euler’s method is O(h).6.3 RUNGE–KUTTA METHODAlthough Heun’s method is a little better than the Euler’s method, it is still not accurate enough for most real-world problems. Thefourth-order Runge–Kutta (RK4) method having a truncation error of O(h4)is one of the most widely used methods for solving differential equations. Its algorithm is described below.whereEquation (6.3.1) is the core of RK4 method, which may be obtained by substituting Simpson’s rule (5.5.4)into the integral form (6.2.1) of differential equation and replacing f k+1/2 with the average of the successive function values (f k2 + f k3)/2. Accordingly, the RK4 method has a truncation error of O(h4)as Eq. (5.6.2) and thus is expected to work better than the previous twomethods.The fourth-order Runge–Kutta (RK4) method is cast into the MATLAB routine “ode_RK4()”. The program “nm630.m” uses this routine to solve Eq. (6.1.1) with the step size h = (t f−t0)/N = 2/4 = 0.5 and plots the numerical result together with the (true) analytical solution. function [t,y] = ode_RK4(f,tspan,y0,N,varargin)%Runge-Kutta method to solve vector differential eqn y’(t) = f(t,y(t))% for tspan = [t0,tf] and with the initial value y0 and N time stepsif nargin < 4 | N <= 0, N = 100; endif nargin < 3, y0 = 0; endy(1,:) = y0(:)’; %make it a row vectorh = (tspan(2) - tspan(1))/N; t = tspan(1)+[0:N]’*h;for k = 1:Nf1 = h*feval(f,t(k),y(k,:),varargin{:}); f1 = f1(:)’; %(6.3.2a)f2 = h*feval(f,t(k) + h/2,y(k,:) + f1/2,varargin{:}); f2 = f2(:)’;%(6.3.2b)f3 = h*feval(f,t(k) + h/2,y(k,:) + f2/2,varargin{:}); f3 = f3(:)’;%(6.3.2c)f4 = h*feval(f,t(k) + h,y(k,:) + f3,varargin{:}); f4 = f4(:)’; %(6.3.2d)y(k + 1,:) = y(k,:) + (f1 + 2*(f2 + f3) + f4)/6; %Eq.(6.3.1)end%nm630: Heun/Euer/RK4 method to solve a differential equation (d.e.) clear, clftspan = [0 2];t = tspan(1)+[0:100]*(tspan(2) - tspan(1))/100;a = 1; yt = 1 - exp(-a*t); %Eq.(6.1.5): true analytical solutionplot(t,yt,’k’), hold ondf61 = inline(’-y + 1’,’t’,’y’); %Eq.(6.1.1): d.e. to be solvedy0 = 0; N = 4;[t1,ye] = oed_Euler(df61,tspan,y0,N);[t1,yh] = ode_Heun(df61,tspan,y0,N);[t1,yr] = ode_RK4(df61,tspan,y0,N);plot(t,yt,’k’, t1,ye,’b:’, t1,yh,’b:’, t1,yr,’r:’)plot(t1,ye,’bo’, t1,yh,’b+’, t1,yr,’r*’)N = 1e3; %to estimate the time for N iterationstic, [t1,ye] = ode_Euler(df61,tspan,y0,N); time_Euler = toctic, [t1,yh] = ode_Heun(df61,tspan,y0,N); time_Heun = toctic, [t1,yr] = ode_RK4(df61,tspan,y0,N); time_RK4 = tocComparison of this result with those of Euler’s method (“ode_Euler()”) and Heun’s method (“ode_Heun()”) is given in Fig. 6.2, which shows that the RK4 method is better than Heun’s method, while Euler’s method is the worst in terms of accuracy with the same step-size. But,Figure 6.2 Numerical solutions for a first-order differential equation.in terms of computational load, the order is reversed, because Euler’s method, Heun’s method, and the RK4 method need 1, 2, and 4 function evaluations (calls) per iteration, respectively.(cf) Note that a function call takes much more time than a multiplication and thus the number of function calls should be a criterion in estimating and comparing computational time.The MATLAB built-in routines “ode23()” and “ode45()” implement the Runge–Kutta method with an adaptive step-size adjustment, which uses a large/small step-size depending on whether f (t)is smooth or rough. In Section 6.4.3, we will try applying these routines together with our routines to solve a differential equation for practice rather than for comparison.6.4 PREDICTOR–CORRECTOR METHOD6.4.1 Adams–Bashforth–Moulton MethodThe Adams–Bashforth–Moulton (ABM) method consists of two steps. The first step is to approximate f(t ,y) by the (Lagrange) polynomial of degree 4 matching the four points{(t k−3, f k−3), (t k−2, f k−2), (t k−1, f k−1), (t k , f k)}and substitute the polynomial into the integral form (6.2.1) ofdifferential equation to get a predicted estimate of y k+1.The second step is to repeat the same work with the updated four points {(t k−2, f k−2), (t k−1, f k−1), (t k , f k), (t k+1, f k+1)} (f k+1 = f(t k+1, p k+1))to get a corrected estimate of y k+1.The coefficients of Eqs. (6.4.1a) and (6.4.1b) can be obtained by using the MATLAB routines “lagranp()” and “polyint()”, each of which generates Lagrange (coefficient) polynomials and integrates a polynomial, respectively.Let’s try running the program “ABMc.m”.>>abmccAP = -3/8 37/24 -59/24 55/24cAC = 1/24 -5/24 19/24 3/8%ABMc.m% Predictor/Corrector coefficients in Adams–Bashforth–Moulton methodclearformat rat[l,L] = lagranp([-3 -2 -1 0],[0 0 0 0]); %only coefficient polynomial Lfor m = 1:4iL = polyint(L(m,:)); %indefinite integral of polynomialcAP(m) = polyval(iL,1)-polyval(iL,0); %definite integral over [0,1]endcAP %Predictor coefficients[l,L] = lagranp([-2 -1 0 1],[0 0 0 0]); %only coefficient polynomial Lfor m = 1:4iL = polyint(L(m,:)); %indefinite integral of polynomialcAC(m) = polyval(iL,1) - polyval(iL,0); %definite integral over [0,1] endcAC %Corrector coefficientsformat shortAlternatively, we write the Taylor series expansion of y k+1 about t k and that of y k about t k+1 asand replace the first, second, and third derivatives by their difference approximations.From these equations and under the assumption that K kk ≅≅+(4)(4)1f f , we can write the predictor/corrector errors asWe still cannot use these formulas to estimate the predictor/corrector errors, since K is unknown. But, from the difference between these two formulaswe can get the practical formulas for estimating the errors asThese formulas give us rough estimates of how close thepredicted/corrected values are to the true value and so can be used to improve them as well as to adjust the step-size.These modification formulas are expected to reward our efforts that we have made to derive them.The Adams–Bashforth–Moulton (ABM) method with the modification formulas can be described by Eqs. (6.4.1a), (6.4.1b), and (6.4.7a), (6.4.7b) summarized below. This scheme needs only two function evaluations (calls) per iteration, while having a truncation error of O(h5) and thus is expected to work better than the methods discussed so far. It is implemented by the MATLAB built-in rou tine “ode113()” with many additional sophisticated techniques.6.4.2 Hamming MethodIn this section, we introduce just the algorithm of the Hamming method [H-1] summarized in the box above, which is another multistep predictor–corrector method like the Adams–Bashforth–Moulton (ABM) method.This scheme also needs only two function evaluations (calls) per iteration, while having the error of O(h5)and so is comparable with the ABM method discussed in the previous section.6.4.3 Comparison of MethodsThe major factors to be considered in evaluating/comparing different numerical methods are the accuracy of the numerical solution and its computation time. In this section, we will compare the routines“ode23()”, “ode45()”, and “ode113()” by trying them out on the same differential equations, hopefully to make some conjectures about their performances. It is important to note that the evaluation/comparison of numerical methods is not so simple because their performances maydepend on the characteristic of the problem at hand. It should also be noted that there are other factors to be considered, such as stability, versatility, proof against run-time error, and so on. These points are being considered in most of the MATLAB built-in routines.The first thing we are going to do is to validate the effectiveness of the modifiers (Eqs. (6.4.8b,d) and (6.4.9b,d)) in the ABM(Adams–Bashforth–Moulton)method and the Hamming method. For this job, we write and run the program“nm643_1.m” to get the results depicted in Fig. 6.3 for the differential equationy_(t) = −y(t) + 1 with y(0) = 0 (6.4.10)which was given at the beginning of this chapter. Fig. 6.3 shows us an interestingfact that, although the ABM method and the Hamming method, even withoutmodifiers, are theoretically expected to have better accuracy than the RK4 (fourthorderRunge–Kutta) method, they turn out to work better than RK4 only with modifiers. Of course, it is not always the case, as illustrated in Fig. 6.4, whichPREDICTOR–CORRECTOR METHOD 27510.50 2 4 6 8 10(a1) Numerical solutions without modifierstrue analytical solution y (t ) = 1 −e−tand numerical solutions10.50 2 4 6 8 10(a2) Numerical solutions with modifierstrue analytical solution y (t ) = 1 − e−tand numerical solutions0 2 4 6 8 10642(b1) Relative errors without modifiers× 10−510.50 2 4 6 8 10(b2) Relative errors with modifiersRK4ABMHamming1.5× 10−5Figure 6.3 Numerical solutions and their errors for the differential equation y_(t) = −y(t) + 1.true analytical solution y (t ) = et − 1and numerical solutions0 2 4 6 8 10123× 104(a3) Numerical solutions by ode23,ode45, ode1130 2 4 6 8 10× 10–310.5ode23 ( )ode45 ( )ode113 ( )(b3) Their relative errorstrue analytical solution y (t ) = et − 1and numerical solutions22 4 6 8 10 0 2 4 6 8 103× 104 × 10–4(a1) Numerical solutions without modifiers11.510.5(b1) Relative errors without modifierstrue analytical solution y (t ) = et − 1and numerical solutions20 2 4 6 8 1013× 104(a2) Numerical solutions with modifiers0 2 4 6 8 10× 10–41.510.5RK4ABMHamming(b2) Relative errors with modifiersFigure 6.4 Numerical solutions and their errors for the differential equation y_(t) = y(t) + 1.276 ORDINARY DIFFERENTIAL EQUATIONSwe obtained by applying the same routines to solve another differential equationy_(t) = y(t) + 1 with y(0) = 0 (6.4.11)where the true analytical solution isy(t) = et − 1 (6.4.12)%nm643_1: RK4/Adams/Hamming method to solve a differential eq clear, clft0 = 0; tf = 10; y0 = 0; %starting/final time, initial valueN = 50; %number of segmentsdf643 = inline(’-y+1’,’t’,’y’); %differential equation to solvef643 = inline(’1-exp(-t)’,’t’); %true analytical solutionfor KC = 0:1tic, [t1,yR] = ode_RK4(df643,[t0 tf],y0,N); tR = toctic, [t1,yA] = ode_ABM(df643,[t0 tf],y0,N,KC); tA = toctic, [t1,yH] = ode_Ham(df643,[t0 tf],y0,N,KC); tH = tocyt1 = f643(t1); %true analytical solution to plotsubplot(221 + KC*2) %plot analytical/numerical solutionsplot(t1,yt1,’k’, t1,yR,’k’, t1,yA,’k--’, t1,yH,’k:’)tmp = abs(yt1)+eps; l_t1 = length(t1);eR = abs(yR - yt1)./tmp; e_R=norm(eR)/lt1eA = abs(yA - yt1)./tmp; e_A=norm(eA)/lt1eH = abs(yH - yt1)./tmp; e_H=norm(eH)/lt1subplot(222 + KC*2) %plot relative errorsplot(t1,eR,’k’, t1,eA,’k--’, t1, eH,’k:’)end%nm643_2: ode23()/ode45()/ode113() to solve a differential eq clear, clft0 = 0; tf = 10; y0 = 0; N = 50; %starting/final time, initial valuedf643 = inline(’y + 1’,’t’,’y’); %differential equation to solvef643 = inline(’exp(t) - 1’,’t’); %true analytical solutiontic, [t1,yR] = ode_RK4(df643,[t0 tf],y0,N); time(1) = toc;tic, [t1,yA] = ode_ABM(df643,[t0 tf],y0,N); time(2) = toc;yt1 = f643(t1);tmp = abs(yt1)+ eps; l_t1 = length(t1);eR = abs(yR-yt1)./tmp; err(1) = norm(eR)/l_t1;eA = abs(yA-yt1)./tmp; err(2) = norm(eA)/l_t1;options = odeset(’RelTol’,1e-4); %set the tolerance of relative errortic, [t23,yode23] = ode23(df643,[t0 tf],y0,options); time(3) = toc; tic, [t45,yode45] = ode45(df643,[t0 tf],y0,options); time(4) = toc; tic, [t113,yode113] = ode113(df643,[t0 tf],y0,options); time(5) = toc; yt23 = f643(t23); tmp = abs(yt23) + eps;eode23 = abs(yode23-yt23)./tmp; err(3) = norm(eode23)/length(t23); yt45 = f643(t45); tmp = abs(yt45) + eps;eode45 = abs(yode45 - yt45)./tmp; err(4) = norm(eode45)/length(t45); yt113 = f643(t113); tmp = abs(yt113) + eps;eode113 = abs(yode113 - yt113)./tmp; err(5) =norm(eode113)/length(t113);subplot(221), plot(t23,yode23,’k’, t45,yode45,’b’, t113,yode113,’r’) subplot(222), plot(t23,eode23,’k’, t45,eode45,’b--’, t113,eode113,’r:’) err, timeVECTOR DIFFERENTIAL EQUATIONS 277Table 6.2 Results of Applying Several Routines to solve a Simple Differential Equationode RK4() ode ABM() ode Ham() ode23() ode45() ode113() Relative error 0.0925 × 10−4 0.0203 × 10−4 0.0179 × 10−4 0.4770 ×10−4 0.0422 × 10−4 0.1249 × 10−4Computing time 0.05 sec 0.03 sec 0.03 sec 0.07 sec 0.05 sec 0.05 sec Readers are invited to supplem ent the program “nm643_2.m” in such a waythat “ode_Ham()” is also used to solve Eq. (6.4.11). Running the program yieldsthe results depicted in Fig. 6.4 and listed in Table 6.2. From Fig. 6.4, it is noteworthythat, without the modifiers, the ABM method seems to be better than theHamming method; however, with the modifiers, it is the other way around or atleast they run a neck-and-neck race. Anyone will see that the predictor–correctormethods such as the ABM method (ode_ABM()) and the Hamming method(ode_Ham()) give us a better numerical solution with less error and shorter computationtime than the MATLAB built-in routines “ode23()”, “ode45()”, and“ode113()” as well as the RK4 method (ode_RK4()), as listed in Table6.2. But,a general conclusion should not be deduced just from one example.6.5 VECTOR DIFFERENTIAL EQUATIONS6.5.1 State EquationAlthough we have tried using the MATLAB routines only for scalar differentialequations, all the routines made by us or built inside MATLAB are ready toentertain first-order vector differential equations, called state equations, as below.x1_(t) = f1(t, x1(t), x2(t), . . .) with x1(t0) = x10x2_(t) = f2(t, x1(t), x2(t), . . .) with x2(t0) = x20. . . . . . . . . . . . . . . . . . . . . . . . . . . . . . . . . .x_(t) = f(t, x(t)) with x(t0) = x0 (6.5.1)For example, we can define the system of first-order differential equationsx1_(t) = x2(t) with x1(0) = 1x2_(t) = −x2(t) + 1 with x2(0) = −1(6.5.2)in a file named “df651.m” and solve it by running the MATLAB program“nm651_1.m”, which uses the routines “ode_Ham()”/“ode45()” to get thenumerical solutions and plots the results as depicted in Fig. 6.5. Note that thef unction given as the first input argument of “ode45()” must be fabricated togenerate its value in a column vector or at least, in the same form of vector asthe input argument ‘x’ so long as it is a vector-valued function.278 ORDINARY DIFFERENTIAL EQUATIONS%nm651_1 to solve a system of differential eqs., i.e., state equationdf = ’df651’;t0 = 0; tf = 2; x0 = [1 -1]; %start/final time and initial valueN = 45; [tH,xH] = ode_Ham(df,[t0 tf],x0,N); %with N = number of segments[t45,x45] = ode45(df,[t0 tf],x0);plot(tH,xH), hold on, pause, plot(t45,x45)function dx = df651(t,x)dx = zeros(size(x)); %row/column vector depending on the shape of x dx(1) = x(2); dx(2) = -x(2) + 1;Especially for the state equations having only constant coefficients like Eq.(6.5.2), we can change it into a matrix–vector form as_x1_(t)x2_(t) _ = _0 10 −1__x1(t)x2(t) _ + _01 _us (t) (6.5.3)with _x1(0)x2(0) _ = _ 1−1 _ and us (t) = 1 ∀t ≥ 0x_(t) = A x(t) + Bu(t) with the initial state x(0) and the input u(t) (6.5.4) which is called a linear time-invariant (LTI) state equation, and then try to findthe analytical solution. For this purpose, we take the Laplace transform of bothsides to writesX(s) −x(0) = AX(s) + BU(s) with X(s) = L{x(t)}, U(s) = L{u(t)}[sI −A]X(s) = x(0) + BU(s), X(s) = [sI −A]−1x(0) + [sI −A]−1BU(s) (6.5.5)where L{x(t)} and L−1{X(s)} denote the Laplace transform of x(t) and theinverse Laplace transform of X(s), respectively. Note that[sI −A]−1 = s−1[I −As−1]−1 = s−1 I + As−1 + A2s−2 + ·· ·φ(t) = L−1{[sI −A]−1} (6.5.6)= I + At +A22t2 +A33!t3 + ·· · = eAt with φ(0) = IBy applying the convolution property of Laplace transform (Table D.2(4) inAppendix D)L−1{[sI −A]−1BU(s)} = L−1{[sI −A]−1} ∗L−1{BU(s)} = φ(t) ∗Bu(t)= _ ∞−∞φ(t −τ)Bu(τ) dτu(τ )=0 for τ<0 or τ>t = _ tφ(t −τ)Bu(τ) dτ (6.5.7)VECTOR DIFFERENTIAL EQUATIONS 279we can take the inverse Laplace transform of Eq. (6.5.5) to write x(t) = φ(t)x(0) + φ(t) ∗Bu(t) = φ(t)x(0) + _ tφ(t −τ)Bu(τ) dτ (6.5.8)For Eq. (6.5.3), we use Eq. (6.5.6) to findφ(t) = L−1{[sI −A]−1}= L−1 ___s 00 s _ − _0 10 −1 __−1_ = L−1 __s −10 s + 1 _−1_= L−1 1s(s + 1) _s +1 10 s __= L−1 _1/s 1/s − 1/(s + 1)0 1/(s + 1) __ = _1 1−e−t0 e−t _ (6.5.9)and use Eqs. (6.5.8), (6.5.9), and u(t) = us (t) = 1 ∀t ≥ 0 to obtain x(t) = _1 1−e−t0 e−t __ 1−1 _ + _ t0 _1 1−e−(t−τ)0 e−(t−τ) __01 _1 dτ= _ e−t−e−t _ + _τ −e−(t−τ)e−(t−τ) _____t0 = _t − 1 + 2e−t1 − 2e−t _ (6.5.10)Alternatively, we can directly take the inverse transform of Eq. (6.5.5) to getX(s) = [sI −A]−1{x(0) + [sI −A]−1BU(s)}=1s(s + 1) _s +1 10 s __ 1−1 _ + _01 _ 1s _=1s2(s + 1) _s +1 10 s __ s−s + 1 _ =1s2(s + 1) _ s2 + 1s(1 −s) _ (6.5.11)X1(s) =s2 + 1s2(s + 1) =1s2 −1s +2s + 1, x1(t) = t − 1 + 2e−t (6.5.12a)X2(s) =1 −ss(s + 1) =1s −2s + 1, x2(t) = 1 − 2e−t (6.5.12b)which conforms with Eq. (6.5.10).The MATLAB program “nm651_2.m” uses a symbolic computation routine“ilaplace()” to get the inverse Laplace transform, uses “eval()” to evaluate280 ORDINARY DIFFERENTIAL EQUATIONS−11.510.5−0.50.5x1(t )1 1.5 2x2(t )Figure 6.5 Numerical/analytical solutions of the continuous-time state equation (6.5.2)/(6.5.3).it, and plots the result as depicted in Fig. 6.5, which supports this derivation procedure.Additionally, it uses another symbolic computation routi ne “dsolve()”to get the analytical solution directly.>>nm651_2Solution of Differential Equation based on Laplace transformXs = [ 1/s + 1/s/(s + 1)*(-1 + 1/s) ][ 1/(s + 1)*(-1 + 1/s) ]xt = [ -1 + t + 2*exp(-t) ][ -2*exp(-t) + 1 ]Analytical solutionxt1 = -1 + t + 2*exp(-t)xt2 = -2*exp(-t) + 1%nm651_2: Analytical solution for state eq. x’(t) = Ax(t) +Bu(t)(6.5.3)clearsyms s t %declare s,t as symbolic variablesA = [0 1;0 -1];B = [0 1]’; %Eq.(6.5.3)x0 = [1 -1]’; %initial valuedisp(’Solution of Differential Eq based on Laplace transform’)disp(’Laplace transformed solution X(s)’)Xs = (s*eye(size(A)) - A)^-1*(x0 + B/s) %Eq.(6.5.5)disp(’Inverse Laplace transformed solution x(t)’)xt = ilaplace(Xs) %inverse Laplace transform %Eq.(6.5.12)t0 = 0; tf = 2; N = 45; %initial/final timet = t0 + [0:N]’*(tf - t0)/N; %time vectorxtt = eval(xt:); %evaluate the inverse Laplace transformplot(t,xtt)disp(’Analytical solution’)xt = dsolve(’Dx1 = x2, Dx2 = -x2 + 1’, ’x1(0) = 1, x2(0) = -1’);xt1 = xt.x1, xt2 = xt.x2 %Eq.(6.5.10)VECTOR DIFFERENTIAL EQUATIONS 2816.5.2 Discretization of LTI State EquationIn this section, we consider a discretization method of converting a continuoustimeLTI (linear time-invariant) state equationx_(t) = A x(t) + Bu(t) with the initial state x(0) and the input u(t)(6.5.13)into an equivalent discrete-time LTI state equation with the sampling period Tx[n + 1] = Ad x[n] + Bdu[n] (6.5.14)with the initial state x[0] and the input u[n] = u(nT ) for nT ≤ t < (n+ 1)Twhich can be solved easily by an iterative scheme mobilizing just simple multiplicationsand additions.For this purpose, we rewrite the solution (6.5.8) of the continuous-time LTIstate equation with the initial time t0 asx(t) = φ(t −t0)x(t0) + _ tt0φ(t −τ)Bu(τ) dτ (6.5.15)Under the assumption that the input is constant as the initial value within eachsampling interval—that is, u[n] = u(nT ) for nT ≤ t < (n+ 1)T —we substitutet0 = nT and t = (n + 1)T into this equation to write the discrete-time LTI stateequation asx((n + 1)T ) = φ(T )x(nT ) + _ (n+1)TnTφ((n + 1)T −τ)Bu(nT ) dτx[n + 1] = φ(T )x[n] + _ (n+1)TnTφ(nT + T −τ) dτBu[n]x[n + 1] = Ad x[n] + Bdu[n] (6.5.16)where the discretized system matrices areAd = φ(T ) = eAT (6.5.17a)Bd = _ (n+1)TnTφ(nT + T −τ) dτBσ=nT+T−τ = −_ 0Tφ(σ) dσB = _ Tφ(τ) dτB(6.5.17b)Here, let us consider another way of computing these system matrices, whichis to the taste of digital computers. It comes from making use of the definitionof a matrix exponential function in Eq. (6.5.6) to rewrite Eq. (6.5.17) as Ad = eAT =∞_m=0AmT mm! = I + AT∞_m=0。