CMP design space exploration subject to physical constraints
华工第十六届数理大赛赛题发布会
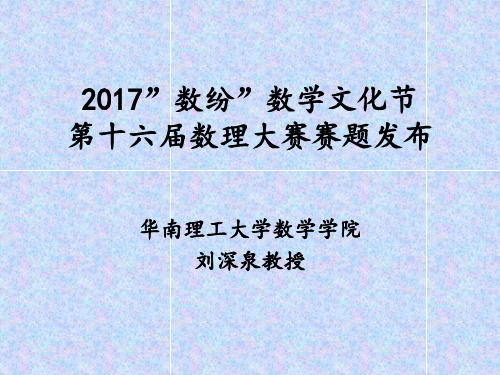
2014全国数学建模竞赛题目 2015美国数学建模竞赛题目
2014全国数学建模竞赛题目 A题 嫦娥三号软着陆轨道设计与控制策略 B题 创意平板折叠桌 C题 生猪养殖场的经营管理 D题 储药柜的设计
2015 MCM&ICM Problems • Problem A:Eradicating Ebola • Problem B:Searching for a lost plane • Problem C:Managing Human Capital in Organizations • Problem D:Is it sustainable?
• Convex Optimization • Duality-Theory • Lagrange Multipliers • Kernels function
Deep Learning Neural Network
• • • • AlphaGo Zero强化学习战胜AlphaGo 人工智能推动数学建模 数学建模制造新的信息机器 丘成桐:工程上取得很大发展 但理论基础仍非常 薄弱 • 人工智能需要一个可以被证明的理论作为基础。 • 人工智能需要新数学理论
SVHN – real world image dataset
Image classification
Convolutiona Neural Network
全 连 接 卷 积
池 化
CNN- 图像分类和场景特色
数学建模生产的图形处理机器
LSTM- 翻译语言和语音识别机器
RNN - 语音识别和自然语言分析
2017A题
CT系统参数标定及成像
• 请建立相应的数学模型和算法,解决以下问题: • (1) 在正方形托盘上放置两个均匀固体介质组成的标定模板,模板的 几何信息如图2所示,相应的数据文件见附件1,其中每一点的数值反 映了该点的吸收强度,这里称为“吸收率”。对应于该模板的接收信 息见附件2。请根据这一模板及其接收信息,确定CT系统旋转中心在 正方形托盘中的位置、探测器单元之间的距离以及该CT系统使用的X 射线的180个方向。 • (2) 附件3是利用上述CT系统得到的某未知介质的接收信息。利用(1) 中得到的标定参数,确定该未知介质在正方形托盘中的位置、几何形 状和吸收率等信息。另外,请具体给出图3所给的10个位置处的吸收 率,相应的数据文件见附件4。 • (3) 附件5是利用上述CT系统得到的另一个未知介质的接收信息。利 用(1)中得到的标定参数,给出该未知介质的相关信息。另外,请具 体给出图3所给的10个位置处的吸收率。 • (4) 分析(1)中参数标定的精度和稳定性。在此基础上自行设计新模 板、建立对应的标定模型,以改进标定精度和稳定性,并说明理由。
(完整版)人工智能原理MOOC习题集及答案北京大学王文敏课件
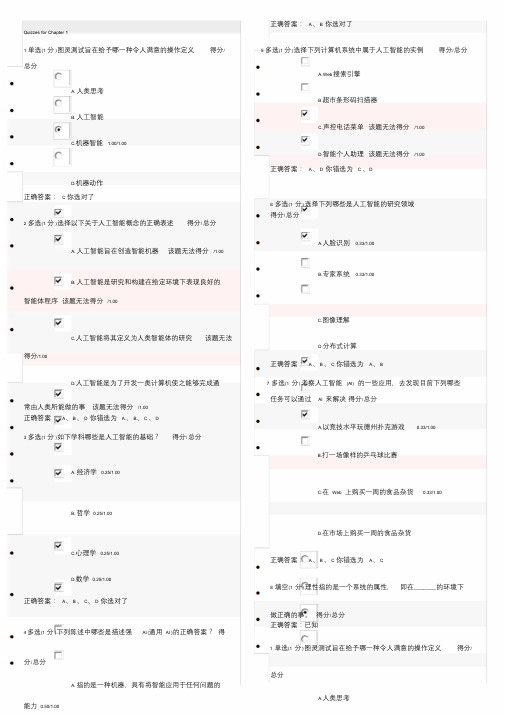
正确答案:A、B 你选对了Quizzes for Chapter 11 单选(1 分)图灵测试旨在给予哪一种令人满意的操作定义得分/ 5 多选(1 分)选择下列计算机系统中属于人工智能的实例得分/总分总分A. W eb搜索引擎A. 人类思考B.超市条形码扫描器B. 人工智能C.声控电话菜单该题无法得分/1.00C.机器智能 1.00/1.00D.智能个人助理该题无法得分/1.00正确答案:A、D 你错选为C、DD.机器动作正确答案: C 你选对了6 多选(1 分)选择下列哪些是人工智能的研究领域得分/总分2 多选(1 分)选择以下关于人工智能概念的正确表述得分/总分A.人脸识别0.33/1.00A. 人工智能旨在创造智能机器该题无法得分/1.00B.专家系统0.33/1.00B. 人工智能是研究和构建在给定环境下表现良好的智能体程序该题无法得分/1.00C.图像理解C.人工智能将其定义为人类智能体的研究该题无法D.分布式计算得分/1.00正确答案:A、B、C 你错选为A、BD.人工智能是为了开发一类计算机使之能够完成通7 多选(1 分)考察人工智能(AI) 的一些应用,去发现目前下列哪些任务可以通过AI 来解决得分/总分常由人类所能做的事该题无法得分/1.00正确答案:A、B、D 你错选为A、B、C、DA.以竞技水平玩德州扑克游戏0.33/1.003 多选(1 分)如下学科哪些是人工智能的基础?得分/总分B.打一场像样的乒乓球比赛A. 经济学0.25/1.00C.在Web 上购买一周的食品杂货0.33/1.00B. 哲学0.25/1.00D.在市场上购买一周的食品杂货C.心理学0.25/1.00正确答案:A、B、C 你错选为A、CD.数学0.25/1.008 填空(1 分)理性指的是一个系统的属性,即在_________的环境下正确答案:A、B、C、D 你选对了做正确的事。
得分/总分正确答案:已知4 多选(1 分)下列陈述中哪些是描述强AI (通用AI )的正确答案?得1 单选(1 分)图灵测试旨在给予哪一种令人满意的操作定义得分/ 分/总分总分A. 指的是一种机器,具有将智能应用于任何问题的A.人类思考能力0.50/1.00B.人工智能B. 是经过适当编程的具有正确输入和输出的计算机,因此有与人类同样判断力的头脑0.50/1.00C.机器智能 1.00/1.00C.指的是一种机器,仅针对一个具体问题D.机器动作正确答案: C 你选对了D.其定义为无知觉的计算机智能,或专注于一个狭2 多选(1 分)选择以下关于人工智能概念的正确表述得分/总分窄任务的AIA. 人工智能旨在创造智能机器该题无法得分/1.00B.专家系统0.33/1.00B. 人工智能是研究和构建在给定环境下表现良好的C.图像理解智能体程序该题无法得分/1.00D.分布式计算C.人工智能将其定义为人类智能体的研究该题无法正确答案:A、B、C 你错选为A、B得分/1.00 7 多选(1 分)考察人工智能(AI) 的一些应用,去发现目前下列哪些任务可以通过AI 来解决得分/总分D.人工智能是为了开发一类计算机使之能够完成通A.以竞技水平玩德州扑克游戏0.33/1.00常由人类所能做的事该题无法得分/1.00正确答案:A、B、D 你错选为A、B、C、DB.打一场像样的乒乓球比赛3 多选(1 分)如下学科哪些是人工智能的基础?得分/总分C.在Web 上购买一周的食品杂货0.33/1.00A. 经济学0.25/1.00D.在市场上购买一周的食品杂货B. 哲学0.25/1.00正确答案:A、B、C 你错选为A、CC.心理学0.25/1.008 填空(1 分)理性指的是一个系统的属性,即在_________的环境下D.数学0.25/1.00 做正确的事。
胡文美教授cuda中文讲座_lecture1

© David Kirk/NVIDIA and Wen-mei W. Hwu Taiwan, June 30-July 2, 2008
Historic GPGPU Constraints
• Dealing with graphics API
– Working with the corner cases of the graphics API
• The same computation executed on many data elements in parallel – low control flow overhead with high SP floating point arithmetic intensity • Many calculations per memory access • Currently also need high floating point to integer ratio
© David Kirk/NVIDIA and Wen-mei W. Hwu Taiwan, June 30-July 2, 2008
CUDA - No more shader functions.
• CUDA integrated CPU+GPU application C program
– Serial or modestly parallel C code executes on CPU – Highly parallel SPMD kernel C code executes on GPU
Input Registers
per thread per Shader per Context
Fragment Program
• Addressing modes
第10章-数字集成电路物理设计方法

20
功耗分析
• 静态功耗分析
– 利用时序库提供的器件功耗数据进行计算 – 利用物理库提供的金属物理工艺数据分析电压降和电迁移效应 – 能提供平均功耗的预估值,迅速简洁
• 动态功耗分析
– 需要提供设计的仿真数据 – VCD文件(Value Change Format):文件太大造成使用不便 – TCF文件(Toggle Count Format):结果比较粗糙,与实际情况存在差异 – CPF文件(Common Power Format):适用于功能验证、RTL综合、形式 验证、布局布线约束
7
数字集成电路物理设计流程
• 250nm工艺物理设计流程
– Timing-Driven Design, TDD – Timing-Driven Routing – Power Grid – Zero Skew
– Static Timing Analysis, STA
– Worst Case, WC – Best Case, BC – In-Place Optimization, IPO – Design Rule Violation, DRV
– Standard Design Constraints, SDC
13
展平式物理设计
自底向上的展平式设计流程及调用关系
14
硅虚拟原型设计
• 布图
– 考虑将整个设计进行分割
• 布线
– 采用快速近似的实验布线(Trial Routing)
– 所需时间约为详细布线的1/10
– 与最终结果在性能上的差距为5%~10% – 快速的设计收敛评估
– Design for Testability, DFT
– On-Chip Variation, OCV
【2024版】模电-考试大纲pcl
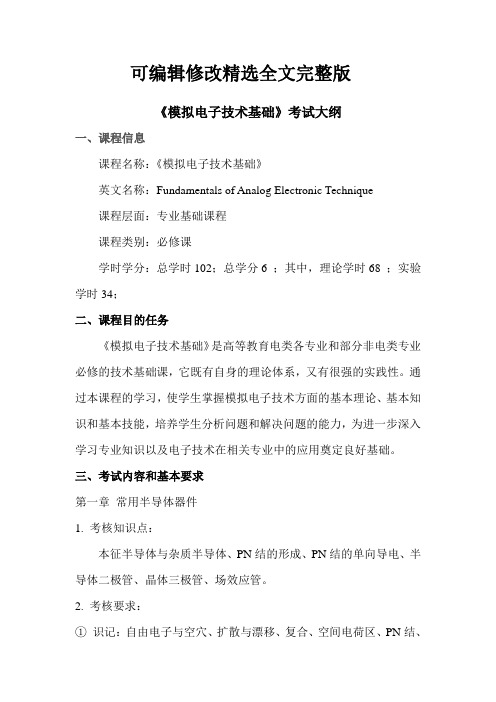
可编辑修改精选全文完整版《模拟电子技术基础》考试大纲一、课程信息课程名称:《模拟电子技术基础》英文名称:Fundamentals of Analog Electronic Technique课程层面:专业基础课程课程类别:必修课学时学分:总学时102;总学分6 ;其中,理论学时68 ;实验学时34;二、课程目的任务《模拟电子技术基础》是高等教育电类各专业和部分非电类专业必修的技术基础课,它既有自身的理论体系,又有很强的实践性。
通过本课程的学习,使学生掌握模拟电子技术方面的基本理论、基本知识和基本技能,培养学生分析问题和解决问题的能力,为进一步深入学习专业知识以及电子技术在相关专业中的应用奠定良好基础。
三、考试内容和基本要求第一章常用半导体器件1. 考核知识点:本征半导体与杂质半导体、PN结的形成、PN结的单向导电、半导体二极管、晶体三极管、场效应管。
2. 考核要求:①识记:自由电子与空穴、扩散与漂移、复合、空间电荷区、PN结、耗尽层、导电沟道等概念。
②领会:二极管的单向导电;稳压管的稳压原理;晶体管与场效应管的放大作用、外特性及主要参数的物理意义、三个工作区域的特点。
③简单应用:利用外加电压判断三极管三个电极和管子的类型,根据三个电极电位判断管子的工作状态;根据特性曲线判断场效应管的类型。
第二章基本放大电路1. 考核知识点:放大的概念,放大电路的组成原则,放大电路的主要性能指标,放大电路的分析方法,晶体管三种基本放大电路共射、共集、共基接法,场效应管放大电路共源、共漏接法,基本放大电路的派生电路。
2. 考核要求:①识记:以下基本概念和定义——放大、静态工作点、饱和失真与截止失真、直流通道和交流通道、直流负载线和交流负载线、H参数等效模型、放大倍数、输入电阻和输出电阻、最大不失真输出电压、静态工作点的稳定。
②领会:组成放大电路的原则和各种基本放大电路的工作原理及特点。
③简单应用:能够根据具体要求选择电路的类型,了解稳定静态工作点的必要性及稳定方法。
第四章DC综合的设计约束
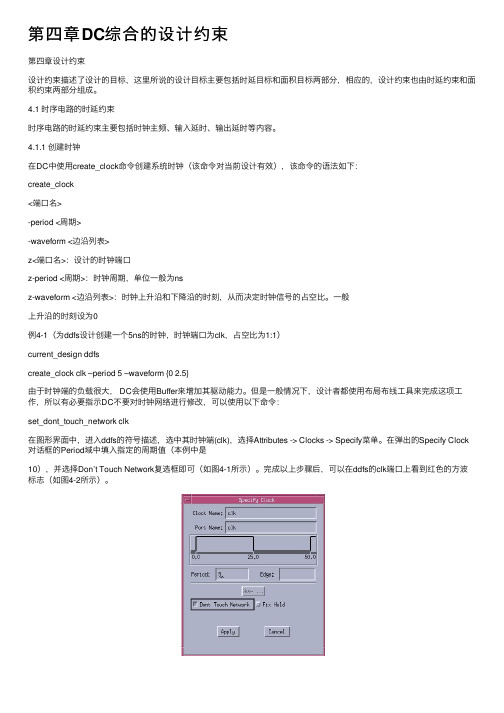
第四章DC综合的设计约束第四章设计约束设计约束描述了设计的⽬标,这⾥所说的设计⽬标主要包括时延⽬标和⾯积⽬标两部分,相应的,设计约束也由时延约束和⾯积约束两部分组成。
4.1 时序电路的时延约束时序电路的时延约束主要包括时钟主频、输⼊延时、输出延时等内容。
4.1.1 创建时钟在DC中使⽤create_clock命令创建系统时钟(该命令对当前设计有效),该命令的语法如下:create_clock<端⼝名>-period <周期>-waveform <边沿列表>z<端⼝名>:设计的时钟端⼝z-period <周期>:时钟周期,单位⼀般为nsz-waveform <边沿列表>:时钟上升沿和下降沿的时刻,从⽽决定时钟信号的占空⽐。
⼀般上升沿的时刻设为0例4-1(为ddfs设计创建⼀个5ns的时钟,时钟端⼝为clk,占空⽐为1:1)current_design ddfscreate_clock clk –period 5 –waveform {0 2.5}由于时钟端的负载很⼤, DC会使⽤Buffer来增加其驱动能⼒。
但是⼀般情况下,设计者都使⽤布局布线⼯具来完成这项⼯作,所以有必要指⽰DC不要对时钟⽹络进⾏修改,可以使⽤以下命令:set_dont_touch_network clk在图形界⾯中,进⼊ddfs的符号描述,选中其时钟端(clk),选择Attributes -> Clocks -> Specify菜单。
在弹出的Specify Clock 对话框的Period域中填⼊指定的周期值(本例中是10),并选择Don’t Touch Network复选框即可(如图4-1所⽰)。
完成以上步骤后,可以在ddfs的clk端⼝上看到红⾊的⽅波标志(如图4-2所⽰)。
(a) Specify Clock菜单 (b) 创建时钟对话框中创建时钟图4-1 在Design Analyzer4.1.2 设置输⼊延时输⼊延时的概念可⽤图4-3来解释。
散射波成像技术在地铁空洞探测中的应用

散射波成像技术在地铁空洞探测中的应用李纯阳;周官群;章俊【摘要】城市地铁工程快速发展,以空洞为主的不良地质体诱发的地面塌陷事故层出不穷.传统的地震反射波法无法满足高精度探测小尺度、不均匀复杂地质体和城市施工空间受限的要求.在考虑散射波成像方法需求以及城市施工环境限制的情况,设计快速可移动城市散射波观测系统,将等效偏移距(EOM)散射成像技术应用于地铁空洞探测中,取得较好效果.%With the rapid development of city underground project , bad geological body induced by the cavity collapse accidents emerge in an endless stream.The traditional seismic reflection wave method can not to solve the high precision detection of the small scale or complex geological body and city construction space limited . The paper considering the scattering wave imaging method and city construction constraints , to design the rapid city scattering wave observation system , the equivalent offset (EOM) application of scattering imaging technology in subway hole exploration , has achieved good effect.【期刊名称】《安徽理工大学学报(自然科学版)》【年(卷),期】2018(038)003【总页数】5页(P40-44)【关键词】地铁;空洞;共散射点道集;偏移成像;散射波技术【作者】李纯阳;周官群;章俊【作者单位】安徽惠洲地质安全研究院股份有限公司研发部,安徽合肥 231202;安徽惠洲地质安全研究院股份有限公司研发部,安徽合肥 231202;中国矿业大学深部岩土力学与地下工程国家重点实验室,江苏徐州 221008【正文语种】中文【中图分类】P613截至2015 年末,中国大陆地区共26座城市开通运营城轨交通,共计116 条线路,其中地下线占总运营里程的57.8%[1]。
ICCAD流程-课堂问题答案
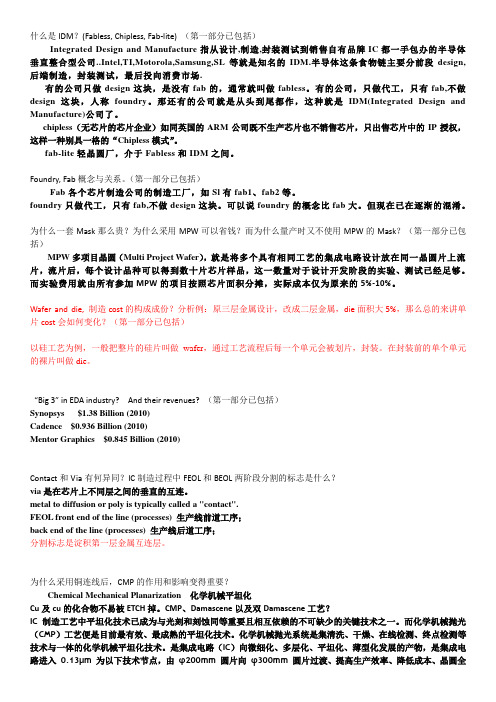
什么是IDM?(Fabless, Chipless, Fab-lite) (第一部分已包括)Integrated Design and Manufacture指从设计,制造,封装测试到销售自有品牌IC都一手包办的半导体垂直整合型公司..Intel,TI,Motorola,Samsung,SL等就是知名的IDM.半导体这条食物链主要分前段design,后端制造,封装测试,最后投向消费市场.有的公司只做design这块,是没有fab的,通常就叫做fabless。
有的公司,只做代工,只有fab,不做design这块,人称foundry。
那还有的公司就是从头到尾都作,这种就是IDM(Integrated Design and Manufacture)公司了。
chipless(无芯片的芯片企业)如同英国的ARM公司既不生产芯片也不销售芯片,只出售芯片中的IP授权,这样一种别具一格的“Chipless模式”。
fab-lite轻晶圆厂,介于Fabless和IDM之间。
Foundry, Fab概念与关系。
(第一部分已包括)Fab各个芯片制造公司的制造工厂,如Sl有fab1、fab2等。
foundry只做代工,只有fab,不做design这块。
可以说foundry的概念比fab大。
但现在已在逐渐的混淆。
为什么一套Mask那么贵?为什么采用MPW可以省钱?而为什么量产时又不使用MPW的Mask?(第一部分已包括)MPW多项目晶圆(Multi Project Wafer),就是将多个具有相同工艺的集成电路设计放在同一晶圆片上流片,流片后,每个设计品种可以得到数十片芯片样品,这一数量对于设计开发阶段的实验、测试已经足够。
而实验费用就由所有参加MPW的项目按照芯片面积分摊,实际成本仅为原来的5%-10%。
Wafer and die, 制造cost的构成成份?分析例:原三层金属设计,改成二层金属,die面积大5%,那么总的来讲单片cost会如何变化?(第一部分已包括)以硅工艺为例,一般把整片的硅片叫做wafer,通过工艺流程后每一个单元会被划片,封装。
- 1、下载文档前请自行甄别文档内容的完整性,平台不提供额外的编辑、内容补充、找答案等附加服务。
- 2、"仅部分预览"的文档,不可在线预览部分如存在完整性等问题,可反馈申请退款(可完整预览的文档不适用该条件!)。
- 3、如文档侵犯您的权益,请联系客服反馈,我们会尽快为您处理(人工客服工作时间:9:00-18:30)。
CMPDesignSpaceExplorationSubjecttoPhysicalConstraintsYingminLi†,BenjaminLee‡,DavidBrooks‡,ZhigangHu††,KevinSkadron††Dept.ofComputerScience,UniversityofVirginia††IBMT.J.WatsonResearchCenter
‡DivisionofEngineeringandAppliedSciences,HarvardUniversity
{yingmin,skadron}@cs.virginia.edu,zhigangh@us.ibm.com,{dbrooks,bclee}@eecs.harvard.edu
AbstractThispaperexploresthemulti-dimensionaldesignspaceforchipmultiprocessors,exploringtheinter-relatedvari-ablesofcorecount,pipelinedepth,superscalarwidth,L2cachesize,andoperatingvoltageandfrequency,undervariousareaandthermalconstraints.Theresultsshowtheimportanceofjointoptimization.Thermalconstraintsdominateotherphysicalconstraintssuchaspin-bandwidthandpowerdelivery,demonstratingtheimportanceofcon-sideringthermalconstraintswhileoptimizingtheseotherparameters.Foraggressivecoolingsolutions,reducingpowerdensityisatleastasimportantasreducingtotalpower,whileforlow-costcoolingsolutions,reducingto-talpowerismoreimportant.Finally,thepapershowsthechallengesofaccommodatingbothCPU-boundandmemory-boundworkloadsonthesamedesign.Theirre-spectivepreferencesformorecoresandlargercachesleadtoincreasinglyirreconcilableconfigurationsasareaandotherconstraintsarerelaxed;ratherthanaccommodat-ingahappymedium,theextraresourcessimplyencouragemoreextremeoptimizationpoints.
1IntroductionRecentproductannouncementsshowatrendtowardag-gressiveintegrationofmultiplecoresonasinglechiptomaximizethroughput.However,thistrendpresentsanex-pansivedesignspaceforchiparchitects,encompassingthenumberofcoresperdie,coresizeandcomplexity(pipelinedepthandsuperscalarwidth),memoryhierarchydesign,operatingvoltageandfrequency,andsoforth.Identify-ingoptimaldesignsisespeciallydifficultbecausethevari-ablesofinterestareinter-relatedandmustbeconsideredsimultaneously.Furthermore,trade-offsamongthesede-signchoicesvarydependingbothonworkloadsandphysi-cal(e.g.,areaandthermal)constraints.Weexplorethismulti-dimensionaldesignspaceacrossarangeofpossiblechipsizesandthermalconstraints,forbothCPU-boundandmemory-boundworkloads.Fewpriorworkshaveconsideredsomanycores,andtoourknowledge,thisisthefirstworktooptimizeacrosssomanydesignvariablessimultaneously.Weshowtheinter-relatednatureoftheseparametersandhowtheoptimumchoiceofdesignparameterscanshiftdramaticallydependingonsys-temconstraints.Specifically,thispaperdemonstratesthat:•Asimple,fastapproachtosimulatealargenumberofcoresbyobservingthatcoresonlyinteractthroughtheL2cacheandsharedinterconnect.Ourmethodologyusessingle-coretracesandonlyrequiresfastcachesimulationformulti-coreresults.•CPU-andmemory-boundapplicationsdesiredramat-icallydifferentconfigurations.Adaptivityhelps,butanycompromiseincursthroughputpenalties.•Thermalconstraintsdominate,trumpingevenpin-bandwidthandpower-deliveryconstraints.Oncether-malconstraintshavebeenmet,throughputisthrottledbacksufficientlytomeetcurrentpin-bandwidthandITRSpower-deliveryconstraints.•Adesignmustbeoptimizedwiththermalconstraints.Scalingfromthethermal-blindoptimumleadstoaconfigurationthatisinferior,sometimesradicallyso,toathermallyoptimizedconfiguration.•Simpler,smallercoresarepreferredundersomecon-straints.Inthermallyconstraineddesigns,themaindeterminantisnotsimplymaximizingthenumberofcores,butmaximizingtheirpowerefficiency.Ther-malconstraintsgenerallyfavorshallowerpipelinesandlowerclockfrequencies.•Additionalcoresincreasethroughput,despitethere-sultingvoltageandfrequencyscalingrequiredtomeetthermalconstraints,untilperformancegainsfromanadditionalcoreisnegatedbytheimpactofvoltageandfrequencyscalingacrossallcores.•Foraggressivecoolingsolutions,reducingpowerden-sityisatleastasimportantasreducingtotalpower.Forlow-costcoolingsolutions,however,reducingto-talpowerismoreimportant.Thispaperisorganizedasfollows.Section2isthere-latedwork.Weintroduceourmodelinfrastructureandval-idationinsection3.Wepresentdesignspaceexplorationresultsandexplanationsinsection4.Weendwithconclu-sionsandproposalsforfutureworkinsection5.2RelatedWorkTherehasbeenaburstofworkinrecentyearstounder-standtheperformance,energy,andthermalefficiencyofdifferentCMPorganizations.Fewhavelookedatalargenumbersofcoresandnone,ofwhichweareaware,have
150-7803-9368-6/06/$20.00/©2006 IEEEjointlyoptimizedacrossthelargenumberofdesignparam-etersweconsiderwhileaddressingtheassociatedmethod-ologychallenges.LiandMart´ınez[17]presentthemostaggressivestudyofwhichweareaware,exploringupto16-wayCMPsforSPLASHbenchmarksandconsideringpowerconstraints.Theirresultsshowthatparallelexecu-tiononaCMPcanimproveenergyefficiencycomparedtothesameperformanceachievedviasingle-threadedex-ecution,andthatevenwithinthepowerbudgetofasin-glecore,aCMPallowssubstantialspeedupscomparedtosingle-threadedexecution.Kongetiraetal.[12]describetheSunNiagaraprocessor,aneight-wayCMPsupportingfourthreadspercoreandtar-getingworkloadswithhighdegreesofthread-levelparal-lelism.Chaudhryetal.[4]describethebenefitsofmultiplecoresandmultiplethreads,sharingeightcoreswithasin-gleL2cache.TheyalsodescribetheSunRockprocessor’s“scouting”mechanismthatusesahelperthreadtoprefetchinstructionsanddata.El-Moursyetal.[6]showtheadvantagesofclusteredarchitecturesandevaluateaCMPofmulti-threaded,multi-clustercoreswithsupportforuptoeightcontexts.Huhetal.[10]categorizedtheSPECbenchmarksintoCPU-bound,cache-sensitive,orbandwidth-limitedgroupsandexploredcorecomplexity,areaefficiency,andpinband-widthlimitations,concludingduetopin-bandwidthlimi-tationsthatasmallernumberofhigh-performancecoresmaximizesthroughput.EkmanandStenstrom[5]useSPLASHbenchmarkstoexploreasimilardesignspaceforenergy-efficiencywiththesameconclusions.Kumaretal.[14]considertheperformance,power,andareaimpactoftheinterconnectionnetworkinCMParchi-tecture.Theyadvocatelowdegreesofsharing,butusetransactionorientedworkloadswithhighdegreesofinter-threadsharing.Sincewearemodelingthroughput-orientedworkloadsconsistingofindependentthreads,wefollowtheexampleofNiagara[12]andemploymoreaggressiveL2sharing.Inourexperiments,eachL2cachebankissharedbyhalfthetotalnumberofcores.Interconnectiondesignparametersarenotvariableinourdesignspaceatthistime,andinfactconstituteasufficientlyexpansivedesignspaceoftheirownthatweconsiderthistobebeyondthescopeofthecurrentpaper.Theresearchpresentedinthispaperdiffersfrompriorworkinthelargenumberofdesignparametersandmetricsweconsider.WeevaluateCMPdesignsforperformance,powerefficiency,andthermalefficiencywhilevaryingthenumberofcoresperchip,pipelinedepthandwidth,chipthermalpackagingeffectiveness,chiparea,andL2cachesize.Thisevaluationisperformedwithafastdecou-pledsimulationinfrastructurethatseparatescoresimula-tionfrominterconnection/cachesimulation.Byconsider-ingmanymoreparametersinthedesignspace,wedemon-stratetheeffectivenessofthisinfrastructureandshowtheinter-relatednessoftheseparameters.ThemethodologiesforanalyzingpipelinedepthandwidthbuildonpriorworkbyLeeandBrooks[16]bydevelopingfirst-ordermodelsforcapturingchangesincoreareaaspipelinedimensionschange,therebyenablingpowerdensityandtemperatureanalysis.Weidentifyop-timalpipelinedimensionsinthecontextofCMParchi-tectures,whereasmostpriorpipelineanalysisconsiderssingle-coremicroprocessors[8,9,22],Furthermore,mostpriorworkinoptimizingpipelinesfocusedexclusivelyonperformance,althoughZyubanetal.found18FO4delaystobepower-performanceoptimalforasingle-threadedmi-croprocessor[26].Otherresearchershaveproposedsimplifiedprocessormodels,withthegoalofacceleratingsimulation.Withinthemicroprocessorcore,KarkhanisandSmith[11]de-scribeatrace-driven,first-ordermodelingapproachtoestimateIPCbyadjustinganidealIPCtoaccountforbranchmisprediction.Incontrast,ourmethodologyadjustspower,performance,andtemperatureestimatesfromde-tailedsingle-coresimulationstoaccountforfabricevents,suchascachemissesandbuscontention.Inordertomodellargescalemultiprocessorsystemsrunningcommercialworkloads,Kunkeletal.[15]utilizeanapproachthatcom-binesfunctionalsimulation,hardwaretracecollection,andprobabilisticqueuingmodels.However,ourdecoupledanditerativeapproachallowsustoaccountforeffectssuchaslatencyoverlapduetoout-of-orderexecution,effectsnoteasilycapturedbyqueuingmodels.Althoughdecoupledsimulationframeworkshavebeenproposedinthecontextofsingle-coresimulation(e.g.,KumarandDavidson[13])withargumentssimilartoourown,ourmethodologyisap-pliedinthecontextofsimulatingmulti-coreprocessors.