lecture8 Matrix Completion
《IEEEsignalprocessingletters》期刊第19页50条数据
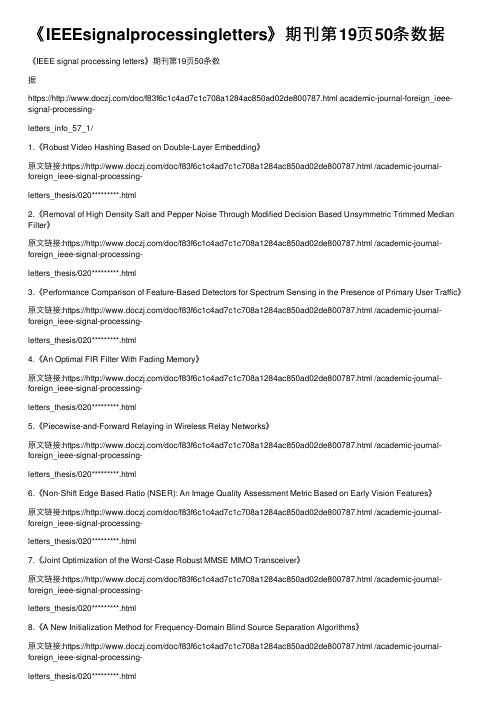
《IEEEsignalprocessingletters》期刊第19页50条数据《IEEE signal processing letters》期刊第19页50条数据https:///doc/f83f6c1c4ad7c1c708a1284ac850ad02de800787.html academic-journal-foreign_ieee-signal-processing-letters_info_57_1/1.《Robust Video Hashing Based on Double-Layer Embedding》原⽂链接:https:///doc/f83f6c1c4ad7c1c708a1284ac850ad02de800787.html /academic-journal-foreign_ieee-signal-processing-letters_thesis/020*********.html2.《Removal of High Density Salt and Pepper Noise Through Modified Decision Based Unsymmetric Trimmed Median Filter》原⽂链接:https:///doc/f83f6c1c4ad7c1c708a1284ac850ad02de800787.html /academic-journal-foreign_ieee-signal-processing-letters_thesis/020*********.html3.《Performance Comparison of Feature-Based Detectors for Spectrum Sensing in the Presence of Primary User Traffic》原⽂链接:https:///doc/f83f6c1c4ad7c1c708a1284ac850ad02de800787.html /academic-journal-foreign_ieee-signal-processing-letters_thesis/020*********.html4.《An Optimal FIR Filter With Fading Memory》原⽂链接:https:///doc/f83f6c1c4ad7c1c708a1284ac850ad02de800787.html /academic-journal-foreign_ieee-signal-processing-letters_thesis/020*********.html5.《Piecewise-and-Forward Relaying in Wireless Relay Networks》原⽂链接:https:///doc/f83f6c1c4ad7c1c708a1284ac850ad02de800787.html /academic-journal-foreign_ieee-signal-processing-letters_thesis/020*********.html6.《Non-Shift Edge Based Ratio (NSER): An Image Quality Assessment Metric Based on Early Vision Features》原⽂链接:https:///doc/f83f6c1c4ad7c1c708a1284ac850ad02de800787.html /academic-journal-foreign_ieee-signal-processing-letters_thesis/020*********.html7.《Joint Optimization of the Worst-Case Robust MMSE MIMO Transceiver》原⽂链接:https:///doc/f83f6c1c4ad7c1c708a1284ac850ad02de800787.html /academic-journal-foreign_ieee-signal-processing-letters_thesis/020*********.html8.《A New Initialization Method for Frequency-Domain Blind Source Separation Algorithms》原⽂链接:https:///doc/f83f6c1c4ad7c1c708a1284ac850ad02de800787.html /academic-journal-foreign_ieee-signal-processing-letters_thesis/020*********.html9.《A Method For Fine Resolution Frequency Estimation From Three DFT Samples》原⽂链接:https:///doc/f83f6c1c4ad7c1c708a1284ac850ad02de800787.html /academic-journal-foreign_ieee-signal-processing-letters_thesis/020*********.html10.《Position-Patch Based Face Hallucination Using Convex Optimization》原⽂链接:https:///doc/f83f6c1c4ad7c1c708a1284ac850ad02de800787.html /academic-journal-foreign_ieee-signal-processing-letters_thesis/020*********.html11.《Signal Fitting With Uncertain Basis Functions》原⽂链接:https:///doc/f83f6c1c4ad7c1c708a1284ac850ad02de800787.html /academic-journal-foreign_ieee-signal-processing-letters_thesis/020*********.html12.《Optimal Filtering Over Uncertain Wireless Communication Channels》原⽂链接:https:///doc/f83f6c1c4ad7c1c708a1284ac850ad02de800787.html /academic-journal-foreign_ieee-signal-processing-letters_thesis/020*********.html13.《The Student's -Hidden Markov Model With Truncated Stick-Breaking Priors》原⽂链接:https:///doc/f83f6c1c4ad7c1c708a1284ac850ad02de800787.html /academic-journal-foreign_ieee-signal-processing-letters_thesis/020*********.html14.《IEEE Signal Processing Society Information》原⽂链接:https:///doc/f83f6c1c4ad7c1c708a1284ac850ad02de800787.html /academic-journal-foreign_ieee-signal-processing-letters_thesis/020*********.html15.《Acoustic Model Adaptation Based on Tensor Analysis of Training Models》原⽂链接:https:///doc/f83f6c1c4ad7c1c708a1284ac850ad02de800787.html /academic-journal-foreign_ieee-signal-processing-letters_thesis/020*********.html16.《On Estimating the Number of Co-Channel Interferers in MIMO Cellular Systems》原⽂链接:https:///doc/f83f6c1c4ad7c1c708a1284ac850ad02de800787.html /academic-journal-foreign_ieee-signal-processing-letters_thesis/020*********.html17.《Period Estimation in Astronomical Time Series Using Slotted Correntropy》原⽂链接:https:///doc/f83f6c1c4ad7c1c708a1284ac850ad02de800787.html /academic-journal-foreign_ieee-signal-processing-letters_thesis/020*********.html18.《Multidimensional Shrinkage-Thresholding Operator and Group LASSO Penalties》原⽂链接:https:///doc/f83f6c1c4ad7c1c708a1284ac850ad02de800787.html /academic-journal-foreign_ieee-signal-processing-letters_thesis/020*********.html19.《Enhanced Seam Carving via Integration of Energy Gradient Functionals》letters_thesis/020*********.html20.《Backtracking-Based Matching Pursuit Method for Sparse Signal Reconstruction》原⽂链接:https:///doc/f83f6c1c4ad7c1c708a1284ac850ad02de800787.html /academic-journal-foreign_ieee-signal-processing-letters_thesis/020*********.html21.《Performance Bounds of Network Coding Aided Cooperative Multiuser Systems》原⽂链接:https:///doc/f83f6c1c4ad7c1c708a1284ac850ad02de800787.html /academic-journal-foreign_ieee-signal-processing-letters_thesis/020*********.html22.《Table of Contents》原⽂链接:https:///doc/f83f6c1c4ad7c1c708a1284ac850ad02de800787.html /academic-journal-foreign_ieee-signal-processing-letters_thesis/020*********.html23.《Bayesian Estimation With Imprecise Likelihoods: Random Set Approach》原⽂链接:https:///doc/f83f6c1c4ad7c1c708a1284ac850ad02de800787.html /academic-journal-foreign_ieee-signal-processing-letters_thesis/020*********.html24.《Low-Complexity Channel-Estimate Based Adaptive Linear Equalizer》原⽂链接:https:///doc/f83f6c1c4ad7c1c708a1284ac850ad02de800787.html /academic-journal-foreign_ieee-signal-processing-letters_thesis/020*********.html25.《Tensor Versus Matrix Completion: A Comparison With Application to Spectral Data》原⽂链接:https:///doc/f83f6c1c4ad7c1c708a1284ac850ad02de800787.html /academic-journal-foreign_ieee-signal-processing-letters_thesis/020*********.html26.《Joint DOD and DOA Estimation for MIMO Array With Velocity Receive Sensors》原⽂链接:https:///doc/f83f6c1c4ad7c1c708a1284ac850ad02de800787.html /academic-journal-foreign_ieee-signal-processing-letters_thesis/020*********.html27.《Regularized Subspace Gaussian Mixture Models for Speech Recognition》原⽂链接:https:///doc/f83f6c1c4ad7c1c708a1284ac850ad02de800787.html /academic-journal-foreign_ieee-signal-processing-letters_thesis/020*********.html28.《Handoff Optimization Using Hidden Markov Model》原⽂链接:https:///doc/f83f6c1c4ad7c1c708a1284ac850ad02de800787.html /academic-journal-foreign_ieee-signal-processing-letters_thesis/020*********.html29.《Standard Deviation for Obtaining the Optimal Direction in the Removal of Impulse Noise》letters_thesis/020*********.html30.《Energy Detection Limits Under Log-Normal Approximated Noise Uncertainty》原⽂链接:https:///doc/f83f6c1c4ad7c1c708a1284ac850ad02de800787.html /academic-journal-foreign_ieee-signal-processing-letters_thesis/020*********.html31.《Joint Subspace Learning for View-Invariant Gait Recognition》原⽂链接:https:///doc/f83f6c1c4ad7c1c708a1284ac850ad02de800787.html /academic-journal-foreign_ieee-signal-processing-letters_thesis/020*********.html32.《GMM-Based KLT-Domain Switched-Split Vector Quantization for LSF Coding》原⽂链接:https:///doc/f83f6c1c4ad7c1c708a1284ac850ad02de800787.html /academic-journal-foreign_ieee-signal-processing-letters_thesis/020*********.html33.《Complexity Reduced Face Detection Using Probability-Based Face Mask Prefiltering and Pixel-Based Hierarchical-Feature Adaboosting》原⽂链接:https:///doc/f83f6c1c4ad7c1c708a1284ac850ad02de800787.html /academic-journal-foreign_ieee-signal-processing-letters_thesis/020*********.html34.《RLS Algorithm With Convex Regularization》原⽂链接:https:///doc/f83f6c1c4ad7c1c708a1284ac850ad02de800787.html /academic-journal-foreign_ieee-signal-processing-letters_thesis/020*********.html35.《Solvability of the Zero-Pinning Technique to Orthonormal Wavelet Design》原⽂链接:https:///doc/f83f6c1c4ad7c1c708a1284ac850ad02de800787.html /academic-journal-foreign_ieee-signal-processing-letters_thesis/020*********.html36.《Power Spectrum Blind Sampling》原⽂链接:https:///doc/f83f6c1c4ad7c1c708a1284ac850ad02de800787.html /academic-journal-foreign_ieee-signal-processing-letters_thesis/020*********.html37.《Noise Folding in Compressed Sensing》原⽂链接:https:///doc/f83f6c1c4ad7c1c708a1284ac850ad02de800787.html /academic-journal-foreign_ieee-signal-processing-letters_thesis/020*********.html38.《Fast Maximum Likelihood Scale Parameter Estimation From Histogram Measurements》原⽂链接:https:///doc/f83f6c1c4ad7c1c708a1284ac850ad02de800787.html /academic-journal-foreign_ieee-signal-processing-letters_thesis/020*********.html39.《Elastic-Transform Based Multiclass Gaussianization》原⽂链接:https:///doc/f83f6c1c4ad7c1c708a1284ac850ad02de800787.html /academic-journal-foreign_ieee-signal-processing-letters_thesis/020*********.html40.《Improving Detection of Acoustic Signals by Means of a Time and Frequency Multiple Energy Detector》原⽂链接:https:///doc/f83f6c1c4ad7c1c708a1284ac850ad02de800787.html /academic-journal-foreign_ieee-signal-processing-letters_thesis/020*********.html41.《Efficient Multiple Kernel Support Vector Machine Based Voice Activity Detection》原⽂链接:https:///doc/f83f6c1c4ad7c1c708a1284ac850ad02de800787.html /academic-journal-foreign_ieee-signal-processing-letters_thesis/020*********.html42.《Performance Analysis of Dual-Hop AF Systems With Interference in Nakagami-$m$ Fading Channels》原⽂链接:https:///doc/f83f6c1c4ad7c1c708a1284ac850ad02de800787.html /academic-journal-foreign_ieee-signal-processing-letters_thesis/020*********.html43.《Illumination Normalization Based on Weber's Law With Application to Face Recognition》原⽂链接:https:///doc/f83f6c1c4ad7c1c708a1284ac850ad02de800787.html /academic-journal-foreign_ieee-signal-processing-letters_thesis/020*********.html44.《A Robust Replay Detection Algorithm for Soccer Video》原⽂链接:https:///doc/f83f6c1c4ad7c1c708a1284ac850ad02de800787.html /academic-journal-foreign_ieee-signal-processing-letters_thesis/020*********.html45.《Regularized Adaptive Algorithms-Based CIR Predictors for Time-Varying Channels in OFDM Systems》原⽂链接:https:///doc/f83f6c1c4ad7c1c708a1284ac850ad02de800787.html /academic-journal-foreign_ieee-signal-processing-letters_thesis/020*********.html46.《A Novel Semi-Blind Selected Mapping Technique for PAPR Reduction in OFDM》原⽂链接:https:///doc/f83f6c1c4ad7c1c708a1284ac850ad02de800787.html /academic-journal-foreign_ieee-signal-processing-letters_thesis/020*********.html47.《Widely Linear Simulation of Continuous-Time Complex-Valued Random Signals》原⽂链接:https:///doc/f83f6c1c4ad7c1c708a1284ac850ad02de800787.html /academic-journal-foreign_ieee-signal-processing-letters_thesis/020*********.html48.《A Generalized Poisson Summation Formula and its Application to Fast Linear Convolution》原⽂链接:https:///doc/f83f6c1c4ad7c1c708a1284ac850ad02de800787.html /academic-journal-foreign_ieee-signal-processing-letters_thesis/020*********.html49.《Multiple-Symbol Differential Sphere Detection Aided Differential Space-Time Block Codes Using QAM Constellations》原⽂链接:https:///doc/f83f6c1c4ad7c1c708a1284ac850ad02de800787.html /academic-journal-foreign_ieee-signal-processing-letters_thesis/020*********.html50.《Low Rank Language Models for Small Training Sets》原⽂链接:https:///doc/f83f6c1c4ad7c1c708a1284ac850ad02de800787.html /academic-journal-foreign_ieee-signal-processing-letters_thesis/020*********.html。
四元数矩阵补全代码

四元数矩阵补全代码四元数矩阵补全是一种在矩阵中填补缺失值的技术。
这种技术可以通过使用四元数来处理矩阵中的缺失值,从而提高矩阵的鲁棒性和精度。
以下是一个四元数矩阵补全的代码实现:```pythonimport numpy as npfrom scipy.optimize import minimize# 定义四元数类class Quaternion:def __init__(self, a, b, c, d):self.a = aself.b = bself.c = cself.d = d# 定义四元数加法def __add__(self, q2):return Quaternion(self.a + q2.a, self.b + q2.b, self.c + q2.c, self.d + q2.d)# 定义四元数减法def __sub__(self, q2):return Quaternion(self.a - q2.a, self.b - q2.b, self.c -q2.c, self.d - q2.d)# 定义四元数数乘def __mul__(self, q2):a = self.a * q2.a - self.b * q2.b - self.c * q2.c - self.d * q2.db = self.a * q2.b + self.b * q2.a + self.c * q2.d - self.d * q2.cc = self.a * q2.c - self.b * q2.d + self.c * q2.a + self.d * q2.bd = self.a * q2.d + self.b * q2.c - self.c * q2.b + self.d * q2.areturn Quaternion(a, b, c, d)# 定义四元数共轭def conj(self):return Quaternion(self.a, -self.b, -self.c, -self.d)# 定义四元数求模def norm(self):return np.sqrt(self.a ** 2 + self.b ** 2 + self.c ** 2 + self.d ** 2)# 定义四元数单位化def normalize(self):norm = self.norm()if norm == 0:return Quaternion(0, 0, 0, 0)else:return Quaternion(self.a / norm, self.b / norm, self.c / norm, self.d / norm)# 定义四元数逆def inv(self):return self.conj() * (1 / self.norm() ** 2)# 定义四元数矩阵补全类class QuaternionMatrixCompletion:def __init__(self, X, p, q, r):self.X = Xself.p = pself.q = qself.r = r# 定义四元数矩阵补全目标函数def objective(self, theta):A = Quaternion(theta[:self.p],theta[self.p:self.p+self.q],theta[self.p+self.q:self.p+2*self.q], theta[-self.r:])Z = np.zeros((self.p, self.q), dtype=Quaternion)for i in range(self.p):for j in range(self.q):if not np.isnan(self.X[i][j]):Z[i][j] = Quaternion(self.X[i][j], 0, 0, 0)E = np.linalg.norm(A.dot(Z).flatten() - self.X.flatten(), ord=2)return E# 定义四元数矩阵补全方法def complete(self):theta = np.random.rand(self.p + 2 * self.q + self.r) # 初始化参数向量bounds = [(None, None)] * self.p + [(0, None)] * self.q + [(None, None)] * self.q + [(None, None)] * self.r # 参数上下界res = minimize(self.objective, theta, bounds=bounds) # 最小化目标函数A = Quaternion(res.x[:self.p],res.x[self.p:self.p+self.q],res.x[self.p+self.q:self.p+2*self.q], res.x[-self.r:])X_hat = A.dot(Z) # 恢复矩阵return X_hat```这个代码实现了一个四元数矩阵补全的类,其中包括了四元数的基本运算,以及矩阵补全的目标函数和方法。
机器学习中矩阵低秩与稀疏近似
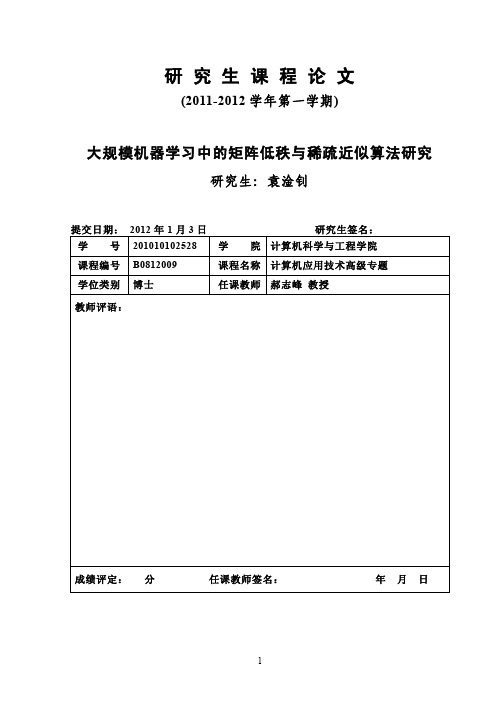
3、课程论文用 A4 纸双面打印。字体全部用宋体简体,题目 要求用小二号字加粗,标题行要求用小四号字加粗,正文内容要求 用小四号字;经学院同意,课程论文可以用英文撰写,字体全部用 Times New Roman,题目要求用 18 号字加粗;标题行要求用 14 号字加粗,正文内容要求用 12 号字;行距为 2 倍行距(方便教师 批注);页边距左为 3cm、右为 2cm、上为 2.5cm、下为 2.5cm;其 它格式请参照学位论文要求。
1.2 l0正则
l0正则是最直接最根本的稀疏学习技术。然而不幸的是,它具有组合的性质,是 个非凸正则子,难于分析。最小化l0范数是一个NP难的问题,在理论和实践中,均只 存在指数复杂度(相对于向量维数)的算法。一般来说,绝大多数算法对求l0只能得 到一个非精确解,有的直接求解最接近l0正则的凸l1正则(显然在lp正则中,p越少越
3
华南理工大学工学博士研究生课程论文
a) p ≥ 1
b) 0 < p < 1
图 1 当p ≥ 1与0 < p < 1时,lp正则子的形状示意图。
接近l0正则),也有的研究者使用如下函数逼近来逼近l0: x 0 ≈ i log(ε + |xi|),其 中ε是一个很小的正数,它是为了避免出现log 0数值上的无意义。但对于需要直接优
2
华南理工大学工学博士研究生课程论文
统计学习是当今机器学习领域的主流技术。向量空间的统计学习算法已经比较 成熟,近几年来,许多研究者主要把目光放在矩阵空间上。与向量空间相比,基于矩 阵空间的学习技术由于缺少扩展性,会随着问题的大小在空间和时间复杂度上分别 呈二次方与三次方增长,所以如何逼近一个目标矩阵而令机器学习技术更鲁棒更精 确更适合于大规模的情况已成为当今机器学习领域十分热门的话题。受到支持向量 机、压缩感知和非负矩阵分解等技术的启发,基于稀疏和低秩性质的假设,人们开发 了一系列基于矩阵方法的机器学习算法。
矩阵集中不等式MATRIX CONCENTRATION INEQUALITIES
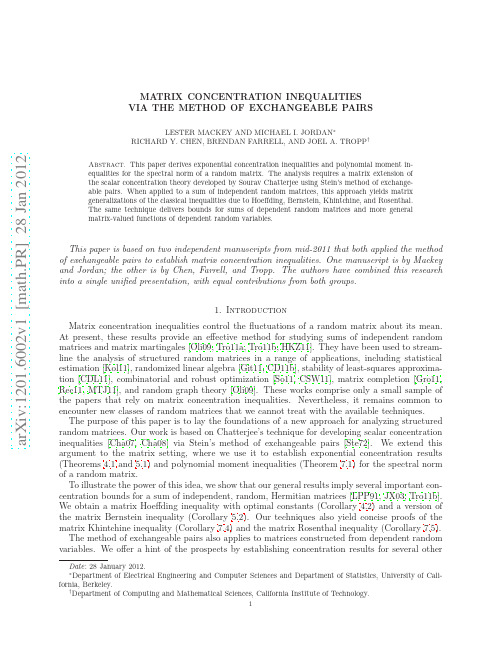
LESTER MACKEY AND MICHAEL I. JORDAN∗ RICHARD Y. CHEN, BRENDAN FARRELL, AND JOEL A. TROPP†
This paper is based on two independent manuscripts from mid-2011 that both applied the method of exchangeable pairs to establish matrix concentration inequalities. One manuscript is by Mackey and Jordan; the other is by Chen, Farrell, and Tropp. The authors have combined this research into a single unified presentation, with equal contributions from both groups. 1. Introduction Matrix concentration inequalities control the fluctuations of a random matrix about its mean. At present, these results provide an effective method for studying sums of independent random matrices and matrix martingales [Oli09, Tro11a, Tro11b, HKZ11]. They have been used to streamline the analysis of structured random matrices in a range of applications, including statistical estimation [Kol11], randomized linear algebra [Git11, CD11b], stability of least-squares approximation [CDL11], combinatorial and robust optimization [So11, CSW11], matrix completion [Gro11, Rec11, MTJ11], and random graph theory [Oli09]. These works comprise only a small sample of the papers that rely on matrix concentration inequalities. Nevertheless, it remains common to encounter new classes of random matrices that we cannot treat with the available techniques. The purpose of this paper is to lay the foundations of a new approach for analyzing structured random matrices. Our work is based on Chatterjee’s technique for developing scalar concentration inequalities [Cha07, Cha08] via Stein’s method of exchangeable pairs [Ste72]. We extend this argument to the matrix setting, where we use it to establish exponential concentration results (Theorems 4.1 and 5.1) and polynomial moment inequalities (Theorem 7.1) for the spectral norm of a random matrix. To illustrate the power of this idea, we show that our general results imply several important concentration bounds for a sum of independent, random, Hermitian matrices [LPP91, JX03, Tro11b]. We obtain a matrix Hoeffding inequality with optimal constants (Corollary 4.2) and a version of the matrix Bernstein inequality (Corollary 5.2). Our techniques also yield concise proofs of the matrix Khintchine inequality (Corollary 7.4) and the matrix Rosenthal inequality (Corollary 7.5). The method of exchangeable pairs also applies to matrices constructed from dependent random variables. We offer a hint of the prospects by establishing concentration results for several other
平面四边形八节点等参元matlab程序
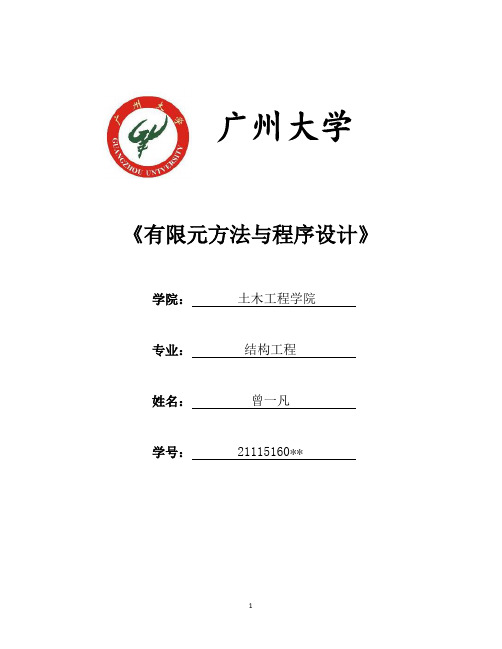
广州大学《有限元方法与程序设计》学院:土木工程学院专业:结构工程姓名:***学号: **********% 平面四边形八节点等参元MATLAB程序% 变量说明&(2015级——结构工程——曾一凡)% YOUNG POISS THICK% 弹性模量泊松比厚度% NPOIN NELEM NVFIX NFORCE% 总结点数单元数约束结点个数受力结点数% COORD LNODS FORCE% 结构节点整体坐标数组单元定义数组结点力数组% ALLFORCE FIXED HK DISP% 总体荷载向量约束信息数组总体刚度矩阵结点位移向量% 1本程序计算了节点位移和单元中心应力并输出到nonde8out.txt文本里% 2在第四页举了一个实例用MATLAB算出结果再用ANSYS算出的结果对比%======================主程序=====================format short e %设定输出类型clear %清除内存变量FP1=fopen('nonde8.txt','rt'); %打开初始数据文件FP2=fopen('nonde8out.txt','wt'); %打开文件存放计算结果NPOIN=fscanf(FP1,'%d',1); %结点数NELEM=fscanf(FP1,'%d',1); %单元数NFORCE=fscanf(FP1,'%d',1); %作用荷载的结点个数NVFIX=fscanf(FP1,'%d',1); %约束数YOUNG=fscanf(FP1,'%e',1); %弹性模量POISS=fscanf(FP1,'%f',1); %泊松比THICK=fscanf(FP1,'%f',1);%厚度LNODS=fscanf(FP1,'%d',[8,NELEM])';%单元结点号数组(逆时针)COORD=fscanf(FP1,'%f',[2,NPOIN])'; % 结点号x,y坐标(整体坐标下) FORCE=fscanf(FP1,'%f',[3,NFORCE])';%结点力数组% 节点力:结点号、X方向力(向右正),Y方向力(向上正)FIXED=fscanf(FP1,'%d',NVFIX)';% 约束信息:约束对应的位移编码(共计NVFIX 组)EK=zeros(2*8,2*8); % 单元刚度矩阵并清零HK=zeros(2*NPOIN,2*NPOIN); % 张成总刚矩阵并清零X=zeros(1,8); %存放单元8个x方向坐标的向量并清零Y=zeros(1,8); %存放单元8个y方向坐标的向量并清零%--------------------------求总刚-------------------------------for i=1:NELEM % 对单元个数循环for m=1:8% 对单元结点个数循环X(m)=COORD(LNODS(i,m),1); %单元8个x方向坐标的向量Y(m)=COORD(LNODS(i,m),2); %单元8个y方向坐标的向量endEK=eKe(X,Y,YOUNG,POISS,THICK);%调用单元刚度矩阵a=LNODS(i,:); %临时向量,用来记录当前单元的结点编号for j=1:8 %对行进行循环---按结点号循环for k=1:8 %对列进行循环---按结点号循环HK((a(j)*2-1):a(j)*2,(a(k)*2-1):a(k)*2)=HK((a(j)*2-1):a(j)*2,(a(k)*2-1):a(k)*2)+...EK(j*2-1:j*2,k*2-1:k*2); % 单刚子块叠加到总刚中endendendALLFORCE=FOECEXL(NPOIN,NFORCE,FORCE); % 调用函数生成荷载向量%-------------------------处理约束--------------------------------for j=1:NVFIX % 对约束个数进行循环N1=FIXED(j);HK(1:2*NPOIN,N1)=0; HK(N1,1:2*NPOIN)=0; HK(N1,N1)=1;% 将零位移约束对应的行、列变成零,主元变成1ALLFORCE(N1)=0;endDISP=HK\ALLFORCE; % 方程求解,HK先求逆再与力向量左乘得到位移%-------------------------求应力---------------------------------stress=zeros(3,NELEM); % 应力向量并清零for i=1:NELEM % 对单元个数进行循环D=(YOUNG/(1-POISS*POISS))*[1 POISS 0;POISS 1 0;0 0 (1-POISS)/2]; %弹性矩阵for k=1:8 % 对单元结点个数进行循环N2=LNODS(i,k); % 取单元结点号U(k*2-1:k*2)=DISP(N2*2-1:N2*2); %从总位移向量中取出当前单元的结点位移B=eBe(X,Y,0,0); %调用单元中心的应变矩阵endstress(:,i)=D*B*U';end%===============计算单元刚度矩阵函数===================function EK=eKe(X,Y,YOUNG,POISS,THICK)EK=zeros(16,16); % 张成16*16矩阵并清零D=(YOUNG/(1-POISS*POISS))*[1 POISS 0;POISS 1 0;0 0 (1-POISS)/2]; %弹性矩阵%高斯积分采用3*3个积分点A1=5/9;A2=8/9;A3=5/9; %对应积分的加权系数A=[A1 A2 A3];r=(3/5)^(1/2);x=[-r 0 r]; %积分点for i=1:3for j=1:3B=eBe(X,Y,x(i),x(j)); %调用应变矩阵BJ=Jacobi(X,Y,x(i),x(j)); %调用雅可比矩阵JEK=EK+A(i)*A(j)*B'*D*B*det(J)*THICK;endend%===============计算雅可比矩阵函数===================function J=Jacobi(X,Y,s,t)[N_s,N_t]=DHS(s,t);x_s=0;y_s=0;x_t=0;y_t=0;for j=1:8x_s=x_s+N_s(j)*X(j);y_s=y_s+N_s(j)*Y(j);x_t=x_t+N_t(j)*X(j);y_t=y_t+N_t(j)*Y(j);endJ=[x_s y_s;x_t y_t];%===============计算应变矩阵函数===================function B=eBe(X,Y,s,t)[N_s,N_t]=DHS(s,t);J=Jacobi(X,Y,s,t);B=zeros(3,16);for i=1:8B1=J(2,2)*N_s(i)-J(1,2)*N_t(i);B2=-J(2,1)*N_s(i)+J(1,1)*N_t(i);B(1:3,2*i-1:2*i)=[B1 0;0 B2;B2 B1];endB=B/det(J);%===============计算形函数的求导函数================ ==function [N_s,N_t]=DHS(s,t)N_s(1)=1/4*(1-t)*(s-t-1)+1/4*(1+s)*(1-t);N_s(2)=1/2*(1+t)*(1-t);N_s(3)=1/4*(1+t)*(s+t-1)+1/4*(1+s)*(1+t);N_s(4)=1/2*(1-s)*(1+t)-1/2*(1+s)*(1+t);N_s(5)=-1/4*(1+t)*(-s+t-1)-1/4*(1-s)*(1+t);N_s(6)=-1/2*(1+t)*(1-t);N_s(7)=-1/4*(1-t)*(-s-t-1)-1/4*(1-s)*(1-t);N_s(8)=1/2*(1-s)*(1-t)-1/2*(1+s)*(1-t);N_t(1)=-1/4*(1+s)*(s-t-1)-1/4*(1+s)*(1-t);N_t(2)=1/2*(1+s)*(1-t)-1/2*(1+s)*(1+t);N_t(3)=1/4*(1+s)*(s+t-1)+1/4*(1+s)*(1+t);N_t(4)=1/2*(1+s)*(1-s);N_t(5)=1/4*(1-s)*(-s+t-1)+1/4*(1-s)*(1+t);N_t(6)=1/2*(1-s)*(1-t)-1/2*(1-s)*(1+t);N_t(7)=-1/4*(1-s)*(-s-t-1)-1/4*(1-s)*(1-t);N_t(8)=-1/2*(1+s)*(1-s);end%===============计算总荷载矩阵函数===================function ALLFORCE=FOECEXL(NPOIN,NFORCE,FORCE) % 本函数生成荷载向量ALLFORCE=zeros(2*NPOIN,1); % 张成特定大小的向量,并赋值0for i=1:NFORCEALLFORCE((FORCE(i,1)*2-1):FORCE(i,1)*2)=FORCE(i,2:3);%FORCE(i,1)为作用点,FORCE(i,2:3)为x,y方向的结点力end%-------------------------输出节点位移和单元中心应力-------------------------------for i=1:NPOINfprintf(FP2,'x%d=%d\n',i, DISP(2*i-1)); %输出结点x方向的位移fprintf(FP2,'y%d=%d\n',i, DISP(2*i)); %输出结点y方向的位移endfor j=1:NELEMfprintf(FP2,'%d x=%f\n',j,stress(1,j)); %输出单元x方向的应力fprintf(FP2,'%d y=%f\n',j,stress(2,j)); %输出单元y方向的应力fprintf(FP2,'%d xy=%f\n',j,stress(3,j)); %输出单元切应力end%------------------------实例计算并用ANSYS进行对比结果----------------------------如图所示一个4m*1m悬臂梁,在3节点作用1*105N的竖向力,参数如下:弹性模量2.0*108,泊松比0.3,划分成四个单元,每个单元八个节点,单元尺寸是1m*1m。
Matrix Completion from a Few Entries
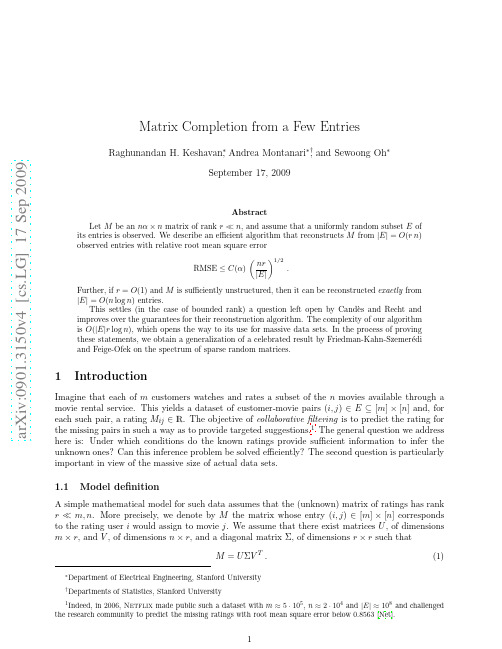
1
Introduction
Imagine that each of m customers watches and rates a subset of the n movies available through a movie rental service. This yields a dataset of customer-movie pairs (i, j ) ∈ E ⊆ [m] × [n] and, for each such pair, a rating Mij ∈ R. The objective of collaborative filtering is to predict the rating for the missing pairs in such a way as to provide targeted suggestions.1 The general question we address here is: Under which conditions do the known ratings provide sufficient information to infer the unknown ones? Can this inference problem be solved efficiently? The second question is particularly important in view of the massive size of actual data sets.
It turns out that, if |E | = Θ(n), this algorithm performs very poorly. The reason is that the matrix M E contains columns and rows with Θ(log n/ log log n) non-zero (revealed) entries. The largest singular values of M E are of order Θ( log n/ log log n). The corresponding singular vectors are highly concentrated on high-weight column or row indices (respectively, for left and right singular vectors). Such singular vectors are an artifact of the high-weight columns/rows and do not provide useful information about the hidden entries of M . This motivates the definition of the following operation (hereafter the degree of a column or of a row is the number of its revealed entries). Trimming. Set to zero all columns in M E with degree larger that 2|E |/n. Set to 0 all rows with degree larger than 2|E |/m. Figure 1 shows the singular value distributions of M E and M E for a random rank-3 matrix M . The surprise is that trimming (which amounts to ‘throwing out information’) makes the underlying rank-3 structure much more apparent. This effect becomes even more important when the number of revealed entries per row/column follows a heavy tail distribution, as for real data. In terms of the above routines, our algorithm has the following structure. Spectral Matrix Completion( matrix M E ) 1: Trim M E , and let M E be the output; 2: Project M E to Tr (M E ); 3: Clean residual errors by minimizing the discrepancy F (X, Y ). 2
colmap八点法计算本质矩阵
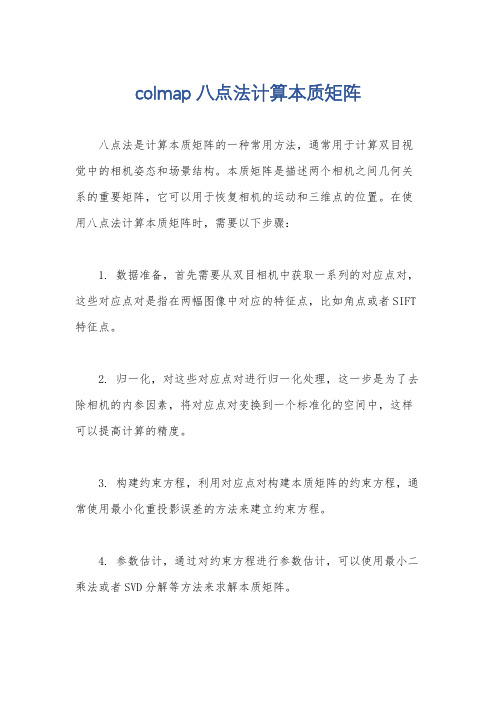
colmap八点法计算本质矩阵
八点法是计算本质矩阵的一种常用方法,通常用于计算双目视觉中的相机姿态和场景结构。
本质矩阵是描述两个相机之间几何关系的重要矩阵,它可以用于恢复相机的运动和三维点的位置。
在使用八点法计算本质矩阵时,需要以下步骤:
1. 数据准备,首先需要从双目相机中获取一系列的对应点对,这些对应点对是指在两幅图像中对应的特征点,比如角点或者SIFT 特征点。
2. 归一化,对这些对应点对进行归一化处理,这一步是为了去除相机的内参因素,将对应点对变换到一个标准化的空间中,这样可以提高计算的精度。
3. 构建约束方程,利用对应点对构建本质矩阵的约束方程,通常使用最小化重投影误差的方法来建立约束方程。
4. 参数估计,通过对约束方程进行参数估计,可以使用最小二乘法或者SVD分解等方法来求解本质矩阵。
5. 约束处理,由于计算得到的矩阵可能不满足本质矩阵的性质,需要对其进行约束处理,比如通过SVD分解将本质矩阵约束为满足
特定条件的形式。
6. 解算结果验证,最后,需要对计算得到的本质矩阵进行验证,通常可以通过对极约束、三角化等方法来验证本质矩阵的准确性。
总的来说,八点法计算本质矩阵是一个复杂的过程,需要对双
目图像进行深入的理解和处理,同时需要熟练掌握相关的数学知识
和计算机视觉算法。
同时,还需要注意处理数据的精度和噪声等因素,以获得准确的本质矩阵计算结果。
求解低秩矩阵填充的改进的交替最速下降法
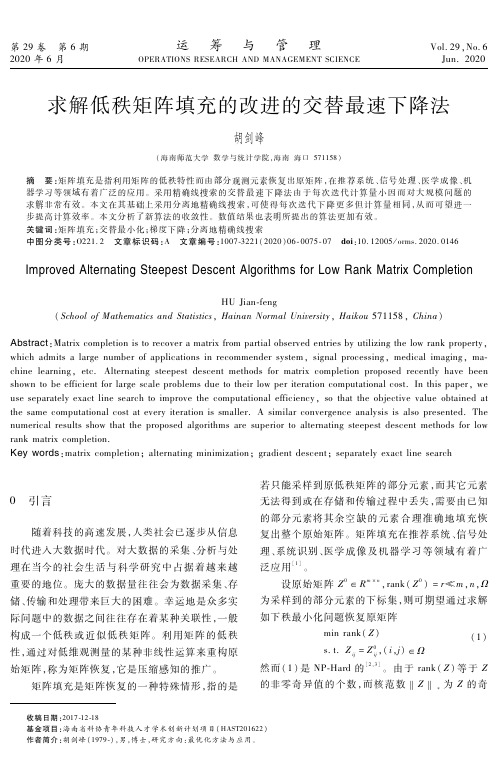
第29卷 第6期运 筹 与 管 理Vol.29,No.62020年6月OPERATIONSRESEARCHANDMANAGEMENTSCIENCEJun.2020收稿日期:2017 12 18基金项目:海南省科协青年科技人才学术创新计划项目(HAST201622)作者简介:胡剑峰(1979 ),男,博士,研究方向:最优化方法与应用。
求解低秩矩阵填充的改进的交替最速下降法胡剑峰(海南师范大学数学与统计学院,海南海口571158)摘 要:矩阵填充是指利用矩阵的低秩特性而由部分观测元素恢复出原矩阵,在推荐系统、信号处理、医学成像、机器学习等领域有着广泛的应用。
采用精确线搜索的交替最速下降法由于每次迭代计算量小因而对大规模问题的求解非常有效。
本文在其基础上采用分离地精确线搜索,可使得每次迭代下降更多但计算量相同,从而可望进一步提高计算效率。
本文分析了新算法的收敛性。
数值结果也表明所提出的算法更加有效。
关键词:矩阵填充;交替最小化;梯度下降;分离地精确线搜索中图分类号:O221.2 文章标识码:A 文章编号:1007 3221(2020)06 0075 07 doi:10.12005/orms.2020.0146ImprovedAlternatingSteepestDescentAlgorithmsforLowRankMatrixCompletionHUJian feng(SchoolofMathematicsandStatistics,HainanNormalUniversity,Haikou571158,China)Abstract:Matrixcompletionistorecoveramatrixfrompartialobservedentriesbyutilizingthelowrankproperty,whichadmitsalargenumberofapplicationsinrecommendersystem,signalprocessing,medicalimaging,machinelearning,etc.Alternatingsteepestdescentmethodsformatrixcompletionproposedrecentlyhavebeenshowntobeefficientforlargescaleproblemsduetotheirlowperiterationcomputationalcost.Inthispaper,weuseseparatelyexactlinesearchtoimprovethecomputationalefficiency,sothattheobjectivevalueobtainedatthesamecomputationalcostateveryiterationissmaller.Asimilarconvergenceanalysisisalsopresented.Thenumericalresultsshowthattheproposedalgorithmsaresuperiortoalternatingsteepestdescentmethodsforlowrankmatrixcompletion.Keywords:matrixcompletion;alternatingminimization;gradientdescent;separatelyexactlinesearch0 引言随着科技的高速发展,人类社会已逐步从信息时代进入大数据时代。
- 1、下载文档前请自行甄别文档内容的完整性,平台不提供额外的编辑、内容补充、找答案等附加服务。
- 2、"仅部分预览"的文档,不可在线预览部分如存在完整性等问题,可反馈申请退款(可完整预览的文档不适用该条件!)。
- 3、如文档侵犯您的权益,请联系客服反馈,我们会尽快为您处理(人工客服工作时间:9:00-18:30)。
Σ = Σ1I2
0
0 diag(σ3 · · · σn)
Q0 0I
Q0
QT 0
=
Σ
,
0I
0I
QT 0 0I
where QQT = I. Then we have
UΣVT = U Q 0 Σ QT 0 VT .
0I
0I
In general, for any Ui and Vi that satisfy A = UiΣViT , we can rewrite in the following form as
tr(X−1) − ttr(X−1YX−1) + t2tr((X−1Y)2X−1) − · · · − tr(X−1)
= lim
t↓0
t
= −tr(X−1YX−1)
= −tr(X−2Y)
8.3 Subgradient
Definition 8.2. If function f is convex and proper (which means domf = {x ∈ E|f (x) < ∞} is non-empty), φ is said to be subgradient of f (x) at xˆ, if it satisfies φ, x − xˆ ≤ f (x) − f (xˆ) for all x ∈ E.
Let Z = (zij) denote user i rates movie j with ranking zij ∈ {1, 2, 3, 4, 5}. However, some movies are not rated by any people, i.e. zij is missing. Our purpose is to complete the ranking matrix. Let X be the m × n complete matrix and Ω = {(i, j)|zij is observed}. We can reasonably suppose that X is a low rank matrix. Our purpose is to minimize the error between Z and X with the constraint that X is low rank, i.e.
simply say f is differentiable (on E).
Example 8.1. f (X) = log |X|, X ∈ Sn++, find f (X; Y), where Y ∈ Sn++.
Solution:
log |(X + tY)| − log |X|
f (X, Y) = lim
8-4
There are three examples of Schatten p-norm:
• p = 1, A ∗ =
n i=1
σi.
• p = 2, A F =
n i=1
σi2.
• p = ∞, A ∞ = σ1. We call σ1 the spectrum radius, and A ∞ is also called spectral norm.
f (xˆ; d) = lim
(2)
t↓0
t
when the limit exists. When the directional derivative f (xˆ; d) is actually linear in d, that
is f (xˆ; d) = a, d for some element a of E. Then, we say f is (Gaˆteanx) differentiable at xˆ with (Gˆateanx) derivative ∇f (xˆ) = a. If f is differentiable at every point in E, then we
1 min X2
(zij − xij )2
(i,j)∈Ω
s.t. X a low rank matrix
Since X is a low rank matrix, we cannot merely use Z ≈ UT X as an approximation of Z. We need to add a sparse matrix S, i.e. Z = X + S, for a matrix can always be represented as the sum of a low rank matrix and a sparse matrix. Then our objective function is:
=
X−
1 2
X−
1 2
YX−
1 2
X
1 2
,
we
have
X−1Y
∼
X−
1 2
YX−
1 2
,
i.e.
X−1Y
and
X−
1 2
YX−
1 2
have the same eigenvalues.
Consider that X and Y are positive definite
symmetric
matrices,
then
X−
1 2
=
(X−
1 2
)T
= 0 and for any z = 0, zT Yz > 0.
Hence
zT
X−
1 2
YX−
1 2
z
>
0
and
X−
1 2
YX−
1 2
is
positive
definite.
Example 8.2. f (X) = tr(AT X), A ∈ Rp×m, X ∈ Rp×n, find f (X; Y)
Lemma 8.1. Let A and R be given m × n matrices, · is Schatten p-norm, φ(·) is the corresponding norm on singular vector, then there is a SVD of A such that
Machine Learning
Matrix Completion
Lecture Notes 8: Matrix Completion
Professor: Zhihua Zhang
Scribe:Yuxi Zhang, Ruotian Luo
8 Matrix Completion
8.1 Problem Background
min
X,S
1 2
Z−X−S
2 F
+
λ1
X
∗ + λ2
S
1
(1)
However, this objective function is not differentiable. Therefore, we need to introduce the definition of directional derivative and subgradient.
t↓0
t
log |X(I + tX−1Y)| − log |X|
=| + log |I + tX−1Y| − log |X|
= lim
t↓0
t
log |I + tX−1Y|
= lim
t↓0
t
= lim
n i=1
log
(1
+
tλi
(X−1
Y))
t↓0
t
=
lim
t↓0
n i=1
Proposition 8.1. For any convex proper function f : E → (−∞, ∞), the point xˆ is a global minimizer of f iff the condition 0 ∈ ∂f (xˆ) holds.
Recall the definition of general matrix norms: Definition 8.3. For A ∈ Rm×n, A is a function of A which satisfies the following conditions.
1. A ≥ 0
2. A = 0 iff A = 0
3. A + B ≤ A + B
8-3
4. αA = |α| A According to the definition, it’s easy to derive that matrix norm is convex:
αA + (1 − α)B ≤ α A + (1 − α) B
8-2
Solution:
tr(AT (X + tY)) − tr(AT X)
f (X, Y) = lim
t↓0
t
tr(AT X) + tr(tAT Y)) − tr(AT X)
= lim
t↓0
t
= tr(AT Y)
= A, Y
Example 8.3. f (X) = tr(X−1), find f (X, Y)
Addition condition: Definition 8.4. A matrix norm · is called consistent if:
AB ≤ A B .
We here consider a kind of norm function which satisfy UT AV = A , U,V are orthogonal matrices. UT U = UUT = I and VT V = VVT = I. A = UΣVT , A is m × n matrix, U is m × m matrix; Σ is m × n; V is n × n.