A Partial Join Approach for Mining Co-location Patterns
布地格福与布地奈德福莫特罗治疗COPD_稳定期效果的对照研究

布地格福与布地奈德福莫特罗治疗COPD稳定期效果的对照研究贺李明(江西萍乡矿业集团有限责任公司总医院呼吸与危重症医学科,萍乡 337000)摘 要 目的:比较布地格福与布地奈德福莫特罗治疗慢性阻塞性肺疾病(COPD)稳定期的临床效果。
方法:收集2021年3月至2022年3月采用药物治疗的COPD稳定期患者60例,用随机数字表法分为观察组和对照组各30例。
观察组给予布地格福吸入气雾剂治疗,2揿/次,2次/d,共10 d。
对照组给予布地奈德福莫特罗吸入粉雾剂治疗,1吸/次,2次/d,共10 d。
比较两组治疗前后血气分析指标、肺功能指标、圣·乔治呼吸问题调查问卷(SGRQ)评分变化情况以及治疗期间药物相关不良反应发生情况。
结果:观察组治疗后血酸碱度(pH)、动脉血氧分压(PaO2)检测结果均高于对照组,动脉血二氧化碳分压(PaCO2)检测结果低于对照组(P<0.05)。
观察组治疗后FEV1%、FEV1/FVC检测结果均高于对照组(P<0.05);症状评分、日常活动评分、心理状态评分及SGRQ总评分均低于对照组(P<0.05)。
观察组和对照组治疗期间各有2例出现药物相关不良反应(P>0.05)。
结论:布地格福与布地奈德福莫特罗治疗COPD 稳定期均有显著的疗效和良好的安全性,但布地格福效果更佳,可作为优选药物。
关键词慢性阻塞性肺疾病;布地格福;布地奈德福莫特罗;血气分析;肺功能中图分类号:R974 文献标志码:A 文章编号:1006-1533(2023)24-0037-04引用本文贺李明. 布地格福与布地奈德福莫特罗治疗COPD稳定期效果的对照研究[J]. 上海医药, 2023, 44(24): 37-40.A comparative study of the effect of budigfu and budesonide formoterol in thetreatment of chronic obstructive pulmonary disease in the stable stageHE Liming(Department of Respiratory and Critical Illness Medicine of General Hospital of Pingxiang Mining Group Co., Ltd, Jiangxi 337000, China) ABSTRACT Objective: To compare the clinical effects of budigfu and budesonide formoterol in the treatment of chronic obstructive pulmonary disease (COPD) in the stable phase. Methods: From March 2021 to March 2022, 60 patients with COPD in the stable stage were collected, and were divided into an observation group and a control group with 30 cases in each group with random number table method. The observation group was treated with budigfu inhalation aerosol, 2 presses/time, 2 times/day, for a total of 10 days, and the control group was treated with budesonide formoterol inhalation powder, 1 presses/time, 2 times/day, for a total of 10 days. The changes of blood gas analysis index, pulmonary function index, St. George’s respiratory questionnaire(SGRQ) score and the occurrence of drug-related adverse reactions were compared between the two groups before and after treatment.Results: After treatment, the detection results of blood pH and arterial partial pressure of oxygen(PaO2) in the observation group were higher than those in the control group, and the result of arterial partial pressure of carbon dioxide(PaCO2) was lower than that in the control group(P<0.05); the detection results of FEV1%, FEV1/FVC in the observation group were higher than those in the control group after treatment(P<0.05); the symptom score, daily activity score, mental state score and SGRQ total score in the observation group were lower than those in the control group(P<0.05). There were two incidence cases of drug-related adverse reactions in the both observation groups(P>0.05). Conclusion: Budigfu and budesonide formoterol have significant efficacy and good safety in the treatment of COPD in the stable stage, but budigfu is more effective and can be used as the preferred drug.通信作者:贺李明。
高三英语学术研究方法创新不断探索单选题30题

高三英语学术研究方法创新不断探索单选题30题1. In academic research, a hypothesis is a ______ that is tested through experiments and observations.A. predictionB. conclusionC. theoryD. assumption答案:D。
本题考查学术研究中“假说”相关的基本概念。
选项A“prediction”意为“预测”,通常是基于现有信息对未来的估计;选项B“conclusion”指“结论”,是在研究后得出的最终判断;选项C“theory”是“理论”,是经过大量研究和验证形成的体系;选项D“assumption”表示“假定、设想”,更符合“假说”的含义,即在研究初期未经充分验证的设想。
2. The main purpose of conducting academic research is to ______ new knowledge and understanding.A. discoverB. createC. inventD. produce答案:A。
此题考查学术研究目的相关的词汇。
选项A“discover”意思是“发现”,强调找到原本存在但未被知晓的事物;选项B“create”意为“创造”,侧重于从无到有地造出新的东西;选项C“invent”指“发明”,通常指创造出新的工具、设备等;选项D“produce”有“生产、产生”的意思,比较宽泛。
在学术研究中,主要是“发现”新知识和理解,所以选A。
3. A reliable academic research should be based on ______ data and methods.A. accurateB. preciseC. correctD. valid答案:D。
本题关于可靠学术研究的基础。
选项A“accurate”侧重于“准确无误”,强调与事实完全相符;选项B“precise”意为“精确的、明确的”,更强调细节的清晰和明确;选项C“correct”指“正确的”;选项D“valid”表示“有效的、有根据的”,强调数据和方法具有合理性和可靠性。
neuro-fuzzy

Combining classifiers of pesticides toxicity through aneuro-fuzzy approachEmilio Benfenati1, Paolo Mazzatorta1, Daniel Neagu2, and Giuseppina Gini21 Istituto di Ricerche Farmacologiche "Mario Negri" Milano,Via Eritrea, 62, 20157 Milano, Italy{Benfenati, Mazzatorta}@ marionegri.it2 Dipartimento di Elettronica e Informazione, Politecnico di Milano,Piazza L. da Vinci 32, 20133 Milano, ItalyNeagu@fusberta.elet.polimi.it, Gini@elet.polimi.ithttp://airlab.elet.polimi.it/imagetoxAbstract. The increasing amount and complexity of data in toxicity predictioncalls for new approaches based on hybrid intelligent methods for mining thedata. This focus is required even more in the context of increasing number ofdifferent classifiers applied in toxicity prediction. Consequently, there exist aneed to develop tools to integrate various approaches. The goal of this researchis to apply neuro-fuzzy networks to provide an improvement in combining theresults of five classifiers applied in toxicity of pesticides. Nevertheless, fuzzyrules extracted from the trained developed networks can be used to performuseful comparisons between the performances of the involved classifiers. Ourresults suggest that the neuro-fuzzy approach of combining classifiers has thepotential to significantly improve common classification methods for the use intoxicity of pesticides characterization, and knowledge discovery.1 IntroductionQuantitative structure–activity relationships (QSARs) correlate chemical structure to a wide variety of physical, chemical, biological (including biomedical, toxicological, ecotoxicological) and technological (glass transition temperatures of polymers, critical micelle concentrations of surfactants, rubber vulcanization rates) properties. Suitable correlations, once established and validated, can be used to predict properties for compounds as yet unmeasured or even unknown.Classification systems for QSAR studies are quite usual for carcinogenicity [9], because in this case carcinogenicity classes are defined by regulatory bodies such as IARC and EPA. For ecotoxicity, most of the QSAR models are regressions, referring to the dose giving the toxic effect in 50% of the animals (for instance LC50: lethal concentration for 50% of the test animals). This dose is a continuous value and regression seems the most appropriate algorithm. However, classification affords some advantages. Indeed, i) the regulatory values are indicated as toxicity classes and ii) classification can allow a better management of noisy data. For this reason we investigated classification in the past [7], [8], [9] and also in this study. No generalrule exists to define an approach suitable to solve a specific classification problem. In several cases, a selection of descriptors is the only essential condition to develop a general system. The next step consists in defining the best computational method to develop robust structure–activity models.Artificial neural networks (ANNs) represent an excellent tool that have been used to develop a wide range of real-world applications, especially when traditional solving methods fail [3]. They exhibit advantages such as ideal learning ability from data, classification capabilities and generalization, computationally fastness once trained due to parallel processing, and noise tolerance. The major shortcoming of neural networks is represented by their low degree of human comprehensibility. More transparency is offered by fuzzy neural networks FNN [14], [16], [18], which represent a paradigm combining the comprehensibility and capabilities of fuzzy reasoning to handle uncertainty, and the capabilities to learn from examples.The paper is organized as follows. Section 2 briefly presents the aspects of data preparation, based on chemical descriptors, some of the most common classification techniques and shows how they behave for toxicology modeling, with a emphasis to pesticides task. Section 3 proposes the neuro-fuzzy approach in order to manage the integration of all the studied classifiers, based on the structure developed as FNN Implicit Knowledge Module (IKM) of the hybrid intelligent system NIKE (Neural explicit&Implicit Knowledge inference system [17]). Preliminary results indicate that combination of several classifiers may lead to the improved performance [5], [11], [12]. The extracted fuzzy rules give new insights about the applicability domain of the implied classifiers. Conclusions of the paper are summarized in the last section.2 Materials and Methods2.1 Data setFor this paper a data set constituted of 57 common organophosphorous compounds has been investigated. The main objective is to propose a good benchmark for the classification studies developed in this area. The toxicity values are the result of a wide bibliographic research mainly from “the Pesticide Manual”, ECOTOX database system, RTECS and HSDB [1]. An important problem that we faced is connected with the variability that the toxicity data presents [2]. Indeed, it is possible to find different fonts showing for the same compound and the same end–point LC50 different for about two orders of magnitude. Such variability is due to different factors, as the different individual reactions of organisms tested, the different laboratory procedures, or is due to different experimental conditions or accidental errors.The toxicity value was expressed using the form Log10 (1/LC50). Then the values were scaled in the interval [-1..1]. Four classes were defined: Class 1 [-1..-0.5), Class 2 [-0.5..0), Class 3 [0..0.5), Class 4 [0.5..1] (Table 2).2.2 DescriptorsA set of about 150 descriptors were calculated by different software: Hyperchem 5.01, CODESSA 2.2.12, Pallas 2.13. They are split into six categories: Constitutional (34 descriptors), Geometrical (14), Topological (38), Electrostatic (57), Quantum–chemicals (6), and Physico–chemical (4). In order to obtain a good model, a selection of the variables, which better describe the molecules, is necessary. There is the risk that some descriptors does not add information, and increase the noise, making more complex the result analysis. Furthermore, using a relatively low number of variables, the risk of overfitting is reduced. The descriptors selection (table 1) was obtained by Principal Components Analysis (PCA), using SCAN4:Table 1. Names of the chemical descriptors involved in the classification task.Cat. Cod.Moment of inertia A G D1Relative number of N atoms C D2Binding energy (Kcal/mol) Q D3DPSA-3 Difference in CPSAs (PPSA3-PNSA3) [Zefirov’s PC] E D4Max partial charge (Qmax) [Zefirov’s PC] E D5ZX Shadow / ZX Rectangle G D6Number of atoms C D7Moment of inertia C G D8PNSA-3 Atomic charge weighted PNSA [Zefirov’s PC] E D9HOMO (eV) E D10LUMO (eV) Q D11Kier&Hall index (order 3) T D122.3 Classification algorithmsThe classification algorithms used for this work are five: LDA (Linear Discriminant Analysis), RDA (Regularized Discriminant Analysis), SIMCA (Soft Independent Modeling of Class Analogy), KNN (K Nearest Neighbors classification), CART (Classification And Regression Tree). The first four are parametric statistical systems based on the Fisher’s discriminant analysis, the fifth and sixth are not parametrical statistical methods, the last one is a classification tree.LDA: the Fischer’s linear discrimination is an empirical method based on p–dimensional vectors of attributes. Thus the separation between classes occurs by an hyperplane, which divides the p–dimensional space of attributes.RDA: The variations introduced in this model have the aim to obviate the principal problems that afflict both the linear and quadratic discrimination. The regulation more efficient was carried out by Friedman, who proposed a compromise between the two previous techniques using a biparametrical method for the estimation (λ and γ).1 Hypercube Inc., Gainsville, Florida, USA2 SemiChem Inc., Shawnee, Kansas, USA3 CompuDrug; Budapest, Hungary4 SCAN (Software for Chemometric Analysis) v.1.1, from Minitab: SIMCA: the model is one of the first used in chemometry for modeling classes and, contrarily to the techniques before described, is not parametrical. The idea is to consider separately each class and to look for a representation using the principal components. An object is assigned to a class on the basis of the residual distance, rsd 2, that it has from the model which represent the class itself:()22ˆigj igj igj x xr −=, )(22j j igjM p r rsd −=∑ (1)where x igj = co –ordinates of the object’s projections on the inner space of the mathematical model for the class, x igj = object’s co –ordinates, p=number of variables, M j = number of the principal components significant for the j class.KNN: this technique classifies each record in a data set based on a combination of the classes of the k record(s) most similar to it in a historical data set (where k = 1). CART is a tree –shaped structure that represents sets of decisions. These decisions generate rules for the classification of a data set. CART provides a set of rules that can be applied to a new (unclassified) data set to predict which records will have a given outcome. It segments a data set by creating two –way splits.The classification obtained using these algorithms is shown in Table 2.2.4 ValidationThe more common methods for validation are: i) Leave –one –out (LOO); ii) Leave –more –out (LMO); iii) Train & Test; iv) Bootstrap. We used LOO, since it is considered the best working on data set of small dimension [10]. According to LOO, given n objects, n models are computed. For each model, the training set consists of n –1 objects and the evaluation set consists of the object left. To estimate the predictive ability, we considered the gap between the experimental (fitting) and the predicted value (cross –validation) for the n objects left, one by one, out from the model.Table 2. True class and class assigned by the algorithms for each compound 5.True Class CART LDA KNN SIMCARDAAnilofos 2 2 2 1 2 2 Chlorpyrifos1 2 2 1 2 2 Chlorpyryfos-methyl 2 2 2 1 2 2 Isazofos 1 1 1 2 1 1 Phosalone 2 2 2 2 2 2 Profenofos 1 2 2 1 2 2 Prothiofos 2 2 2 2 2 2 Azamethiphos 2 2 2 1 4 2 Azinphos methyl 1 1 1 2 1 1 Diazinon 3 3 1 1 4 1 Phosmet2 2 2 1 2 2 Pirimiphos ethyl 1 1 1 1 1 1 Pirimiphos methyl2312115 The 40 molecules with a blank background were used to train the neuro-fuzzy classifier.Pyrazophos 2 2 1 4 2 1Quinalphos 1 1 1 2 1 1Azinphos-ethyl 1 1 1 1 2 1Etrimfos 1 1 1 3 3 1Fosthiazate 4 2 2 2 4 2Methidathion 1 1 1 1 1 1Piperophos 3 3 3 2 2 3Tebupirimfos 4 1 1 3 4 1Triazophos 1 1 1 2 1 1Dichlorvos 2 4 2 2 2 2Disulfoton 3 3 3 1 3 3Ethephon 4 4 4 4 4 4Fenamiphos 1 1 3 2 1 1Fenthion 2 2 3 2 2 3Fonofos 1 1 3 2 1 3Glyphosate 4 4 4 4 4 4Isofenphos (isophenphos) 3 3 3 1 3 3Methamidophos 4 4 4 3 4 4Omethoate 3 3 3 3 3 3Oxydemeton-methyl 3 3 3 3 3 3Parathion ethyl (parathion) 2 2 2 3 1 3Parathion methyl 3 3 3 3 3 3Phoxim 2 2 1 1 1 1Sulfotep 1 1 3 2 2 2Tribufos 2 2 2 2 2 2Trichlorfon 2 2 2 1 2 4Acephate 4 4 1 3 4 4Cadusafos 2 2 3 3 2 2Chlorethoxyfos 2 2 2 3 2 2Demeton-S-methyl 3 3 3 3 3 3Dimethoate 3 3 1 1 3 3Edifenphos 2 2 3 1 2 2EPN 2 2 2 2 2 2Ethion 2 2 2 2 2 2Ethoprophos 3 3 3 2 2 3Fenitrothion 3 2 3 3 3 3Formothion 3 3 2 3 3 3Methacrifos 2 2 2 2 2 3Phorate 1 1 3 2 1 3Propetamphos 3 3 3 4 2 3Sulprofos 3 3 3 2 3 3Temephos 3 3 2 1 3 2Terbufos 1 1 3 2 3 3Thiometon 3 3 3 3 3 33.1 The neuro-fuzzy combination of the classifiers3.2 Motivations and architectureCombining multiple classifiers could be considered as a direction for the development of highly reliable pattern recognition systems, coming from the hybrid intelligent systems approach. Combination of several classifiers may result in improved performances [4], [5]. The necessity of combining multiple classifiers is arising from the main demand of increasing quality and reliability of the final models. There are different classification algorithms in almost all the current pattern recognition application areas, each one having certain degrees of success, but none of them beingas good as expected in applications. The combination technique we propose for the toxicity classification is a neuro-fuzzy gating of the implied classifiers, trained against the correct data. This approach allows multiple classifiers to work together.For this task, the hybrid intelligent system NIKE was used, in order to automate the processes involved, from the data representation for toxicity measurements, to the prediction of toxicity for given new input. It also suggests how the fuzzy inference produced the result, when required [17], based on the effect measure method to combine the weights between the layers of the network in order to select the strongest input-output dependencies [6]. Consequently, for NIKE, we defined the implicit knowledge as the knowledge acquired by neural/neuro-fuzzy nets.Fig. 1. Implicit Knowledge Module implemented as FNN2.The IKM-FNN is implemented as a multilayered neural structure with an input layer, establishing the inputs to perform the membership degrees of the current values, a fully connected three-layered FNN2 [16], and a defuzzification layer [17] (fig.1). The weights of the connections between layer 1 and layer 2 are set to one. A linguistic variable X i is described by m i fuzzy sets, A ij, having the degrees of membership performed by the functions µij(x i), j=1,2,...,m i, i=1,2,..,p., (in our case, p=5, all m i=4, on the classes of the prediction result of the classifiers, as inputs, and on the classes of the toxicity values, as the output y defuz). The layers 1 and 5 are used in the fuzzification process in the training and prediction steps, and the layers 2-4 are organized as a feedforward network to represent the implicit rules through FNN training [15][19]. 3.2 ResultsSince NIKE modules process only data scaled into the interval [0..1], every class was represented by the centroid of each of the four classes in which the available domain was split: 0.135 (class 1), 0.375 (class 2), 0.625 (class 3), and 0.875 (class 4). The inputs and the output followed a trapezoidal (de)fuzzification (fig. 2): VeryLow (0-0.25), Low (0.25-0.5), Medium (0.5-0.75), High (0.75-1).The neuro-fuzzy network was trained on a training set of 40 objects (70% of the entire set, as depicted in Table 2). The training set was used for the adjustment of the connections of the neural and neuro-fuzzy networks with backpropagation (traingdx) algorithm; traingdx is a network training function that updates weight and bias values according to gradient descent momentum and an adaptive learning rate. The neuro-fuzzy network was a multi-layered structure with the 5x4 above described fuzzy inputs and 4 fuzzy output neurons, the toxicity class linguistic variable (fig. 2.a). The number of hidden neurons parameterized the FNN. After different models (5 to 50 hidden units), a medium number of hidden units is desirable and had the same best results: IKM-FNN with 10, 12 and 19 neurons (fig. 3).(a) (b)Fig. 2. NIKE: (a)The fuzzy terms of the generic linguistic variable Class; (b) the FNN model. Table 3. Performances of the classification algorithms computed.NER% fitting NER%validation DescriptorsLDA 64.91 61.40 D1,D2, D3, D4RDA 84.21 71.93 D1, D2, D3, D4, D6, D7, D8, D11, D12, D13 SIMCA 92.98 77.19 D1, D2, D3, D4, D5, D6, D7, D8, D10, D11, D12 KNN - 61.40 D1, D12CART 85.96 77.19 D1, D2, D3, D4, D5, D9Table 4. Confusion matrix of the neuro-fuzzy combination of classifiers.N° of objectsAssigned Class1 2 3 41 132 15True Class2 20 203 1 15 164 6 6Table 5. True class and class assigned by all the classifiers for each compound wrong predicted by the neuro-fuzzy combination of classifiers.True Class CART LDA KNN SIMCA RDA FNN Chlorpyrifos 1 2 2 1 2 2 2Profenofos 1 2 2 1 2 2 2Fenitrothion 3 2 3 3 3 3 2(a)(b)(c)(d)(e)(f)Fig. 3. The results of training FNNs: (a) 3-5 errors, the best are FNN10H, FNN12H and FNN19H; (b) the chosen model, FNN10H, against the SIMCA results and the real ones; (c) the bad fuzzy inference prediction for 2 cases in class 1 (Chlorpyrifos and Profenofos); (d) the bad fuzzy inference prediction for the case in class 3 (Fenitrothion); two samples of good prediction for test cases: (e) a class 1 sample (Phorate); (f) a class 2 sample (Edinfenphos).A momentum term of 0.95 was used (to prevent too many oscillations of the error function). The nets were trained up to 5000 epochs, giving an error about 0.015. The recognition error for the above models is 5.26% (table 4, 5, fig. 3).The confusion matrix shows the ability in prediction of our approach. Looking of Table 3, we notice that the best performance was obtained by SIMCA, which could correctly classify almost 93% of the molecules. This encouraging result was obtained with whole data set involved in developing the model. If we take a look to the NER% validated with LOO, we can notice that we loss a lot of the reliability of the model when we predict the toxicity of an external object. Such a behavior proves the ability in modeling of these algorithms, but shows also their incapacity in generalization. The neuro-fuzzy approach seems to overcome this problem, succeeding in voting for the best opinion and underling all the considered classification algorithms (fig. 3).3.3 Interpreting the results of the neuro-fuzzy combination of the classifiers The most relevant fuzzy rules were extracted from the IKM-FNN structures using Effect Measure Method [6][13]. Finally, after deleting the contradictory rules, the next list of the most trusty fuzzy rules were considered for the chosen net IKM-FNN10H: IF CarFit1 is:VeryLow THEN class is:High (39.22%)IF CarFit1 is:Low THEN class is:High (82.30%)IF CarFit1 is:Medium THEN class is:High (48.74%)IF CarFit1 is:High THEN class is:High (39.04%)IF SimFit1 is:VeryLow THEN class is:Medium (61.25%)IF SimFit1 is:Low THEN class is:Medium (36.04%)IF SimFit1 is:High THEN class is:Medium (43.72%)IF RdaFit1 is:VeryLow THEN class is:Low (75.65%)IF RdaFit1 is:Low THEN class is:Low (100.00%)IF RdaFit1 is:High THEN class is:High (76.39%)Three types of fuzzy rules were obtained: some could be grouped by the same output, or by having the same fuzzy term in the premise and conclusion, and, finally, rules with mixed terms in premises and conclusion parts. From the first two groups of fuzzy rules (italics), we could conclude that, the opinion of the entry classifier is not important for the given output. More precisely, CART prediction for High values of toxicity (class 4) is better to not be taken in consideration.IF (CarFit1 is:VeryLow) OR (CarFit1 is:Low) OR (CarFit1 is:Medium) OR (CarFit1 is:High) THEN class is:HighSimilarly, SIMCA outputs are not so important for predicting class 3 (Medium toxicity: the second group of fuzzy rules). From the second last group of rules, we could find which is the best classifier from the involved systems. In our case, in order to predict class 2 (Low toxicity) is better to consider the opinion coming from RDA. The same opinion is very important for predicting the class 4 (High toxicity) cases too.ConclusionsClassification of the toxicity requires a high degree of experience from computational chemistry experts. Several approaches were described to generate suitable computer-based classifiers for the considered patterns. We investigated five different classifiers and a neuro-fuzzy correlation of them, to organize and classify toxicity data sets. Our approach shown an improved behaviour as a combination of classifiers. Some results viewing fuzzy rules extraction, as well as the possibility to interpret particular inferences suggest that the Neuro-Fuzzy approach has the potential to significantly improve common classification methods for the use in toxicity characterization. AcknowledgmentThis work is partially funded by the E.U. under the contract HPRN-CT-1999-00015. References1. Benfenati, E., Pelagatti, S., Grasso, P., Gini, G.: COMET: the approach of a project in evaluatingtoxicity. In: Gini, G. C.; Katritzky, A. R. (eds.): Predictive Toxicology of Chemicals: Experiences and Impact of AI Tools. AAAI 1999 Spring Symposium Series. AAAI Press, Menlo Park, CA (1999) 40-43 2. Benfenati, E., Piclin, N., Roncaglioni,A., Varì, M.R.: Factors Influencing Predictive Models ForToxicology. SAR and QSAR in environmental research, 12 (2001) 593-603.3. Bishop, C.M.: Neural networks for pattern recognition. Clarendon Press, Oxford (1995)4. Chen, K., Chi, H.: A method of combining multiple probabilistic classifiers through soft competition ondifferent feature sets. Neurocomputing 20 (1998) 227-2525. Duin, R.P.W., Tax, D.M.J.: Experiments with Classifier Combining Rules. Lecture Notes in ComputerScience, 1857, Springer-Verlag, Berlin (2000) 16-296. Enbutsu, I., Baba, K., Hara, N.: Fuzzy Rule Extraction from a Multilayered Network, in Procs. ofIJCNN'91, Seattle (1991) 461-4657. Gini, G., Benfenati, E., Boley, D.: Clustering and Classification Techniques to Assess Aquatic Toxicity.Procs. of the Fourth Int'l Conf. KES2000, Brighton, UK, Vol. 1 (2000) 166-1728. Gini, G., Giumelli, M., Benfenati, E., Lorenzini, P., Boger, Z.: Classification methods to predict activityof toxic compounds, V Seminar on Molecular Similarity, Girona, Spain (2001 forthcoming)9. Gini, G., Lorenzini, M., Benfenati, E., Brambilla, R., Malvé, L.: Mixing a Symbolic and a SubsymbolicExpert to Improve Carcinogenicity Prediction of Aromatic Compounds. In: Kittler,J.,Roli,F.(eds.):Multiple Classifier Systems, Springler-Verlag, Berlin (2001)126-135.10. Helma, C., Gottmann, E., Kramer, S.: Knowledge discovery and data mining in toxicology. Statisticalmethods in medical research, 9 (2000) 131-13511. Ho, T., Hull, J., Srihari, S.: Decision combination in multiple classifier systems. IEEE Trans. PatternAnal. Mach. Intell. 16/1 (1994) 66-7512. Jacobs, R.A.: Methods for combining experts' probability assessments, Neur. Comp. 7/5(1995)867-88813. Jagielska, I., Matthews, C., Whitfort, T.: An investigation into the application of ANN, FL, GA, andrough sets to automated knowledge acquisition for classification problems. Neurocomp,24(1999)37-5414. Kosko, B.: Neural Networks and Fuzzy System. Prentice-Hall, Englewood Cliffs (1992)15. Lin, C.T., George Lee, C.S.: Neural - Network Based Fuzzy Logic Control and Decision System. IEEETransactions on Computers, 40/12 (1991) 1320-133616. Nauck, D., Kruse, R.: NEFCLASS-X: A Neuro-Fuzzy Tool to Build Readable Fuzzy Classifiers. BTTech. J. 16/3 (1998) 180-192.17. Neagu, C.-D., Avouris, N.M., Kalapanidas, E., Palade, V.: Neural and Neuro-fuzzy Integration in aKnowledge-based System for Air Quality Prediction. App Intell. J. (2001 accepted)18. Palade, V., Neagu, C.-D., Patton, R.J.: Interpretation of Trained Neural Networks by Rule Extraction,Procs. of Int'l Conf. 7th Fuzzy Days in Dortmund (2001) 152-161.19. Rumelhart, D.E., McClelland, J.L.: Parallel Distributed Processing, Explanations in the Microstructureof Cognition. MIT Press (1986)。
外文文献文献列表

- disruption ,: Global convergence vs nationalSustainable-,practices and dynamic capabilities in the food industry: A critical analysis of the literature5 Mesoscopic- simulation6 Firm size and sustainable performance in food -s: Insights from Greek SMEs7 An analytical method for cost analysis in multi-stage -s: A stochastic / model approach8 A Roadmap to Green - System through Enterprise Resource Planning (ERP) Implementation9 Unidirectional transshipment policies in a dual-channel -10 Decentralized and centralized model predictive control to reduce the bullwhip effect in -,11 An agent-based distributed computational experiment framework for virtual -/ development12 Biomass-to-bioenergy and biofuel - optimization: Overview, key issues and challenges13 The benefits of - visibility: A value assessment model14 An Institutional Theory perspective on sustainable practices across the dairy -15 Two-stage stochastic programming - model for biodiesel production via wastewater treatment16 Technology scale and -s in a secure, affordable and low carbon energy transition17 Multi-period design and planning of closed-loop -s with uncertain supply and demand18 Quality control in food -,: An analytical model and case study of the adulterated milk incident in China19 - information capabilities and performance outcomes: An empirical study of Korean steel suppliers20 A game-based approach towards facilitating decision making for perishable products: An example of blood -21 - design under quality disruptions and tainted materials delivery22 A two-level replenishment frequency model for TOC - replenishment systems under capacity constraint23 - dynamics and the ―cross-border effect‖: The U.S.–Mexican border’s case24 Designing a new - for competition against an existing -25 Universal supplier selection via multi-dimensional auction mechanisms for two-way competition in oligopoly market of -26 Using TODIM to evaluate green - practices under uncertainty27 - downsizing under bankruptcy: A robust optimization approach28 Coordination mechanism for a deteriorating item in a two-level - system29 An accelerated Benders decomposition algorithm for sustainable -/ design under uncertainty: A case study of medical needle and syringe -30 Bullwhip Effect Study in a Constrained -31 Two-echelon multiple-vehicle location–routing problem with time windows for optimization of sustainable -/ of perishable food32 Research on pricing and coordination strategy of green - under hybrid production mode33 Agent-system co-development in - research: Propositions and demonstrative findings34 Tactical ,for coordinated -s35 Photovoltaic - coordination with strategic consumers in China36 Coordinating supplier׳s reorder point: A coordination mechanism for -s with long supplier lead time37 Assessment and optimization of forest biomass -s from economic, social and environmental perspectives – A review of literature38 The effects of a trust mechanism on a dynamic -/39 Economic and environmental assessment of reusable plastic containers: A food catering - case study40 Competitive pricing and ordering decisions in a multiple-channel -41 Pricing in a - for auction bidding under information asymmetry42 Dynamic analysis of feasibility in ethanol - for biofuel production in Mexico43 The impact of partial information sharing in a two-echelon -44 Choice of - governance: Self-managing or outsourcing?45 Joint production and delivery lot sizing for a make-to-order producer–buyer - with transportation cost46 Hybrid algorithm for a vendor managed inventory system in a two-echelon -47 Traceability in a food -: Safety and quality perspectives48 Transferring and sharing exchange-rate risk in a risk-averse - of a multinational firm49 Analyzing the impacts of carbon regulatory mechanisms on supplier and mode selection decisions: An application to a biofuel -50 Product quality and return policy in a - under risk aversion of a supplier51 Mining logistics data to assure the quality in a sustainable food -: A case in the red wine industry52 Biomass - optimisation for Organosolv-based biorefineries53 Exact solutions to the - equations for arbitrary, time-dependent demands54 Designing a sustainable closed-loop -/ based on triple bottom line approach: A comparison of metaheuristics hybridization techniques55 A study of the LCA based biofuel - multi-objective optimization model with multi-conversion paths in China56 A hybrid two-stock inventory control model for a reverse -57 Dynamics of judicial service -s58 Optimizing an integrated vendor-managed inventory system for a single-vendor two-buyer - with determining weighting factor for vendor׳s ordering59 Measuring - Resilience Using a Deterministic Modeling Approach60 A LCA Based Biofuel - Analysis Framework61 A neo-institutional perspective of -s and energy security: Bioenergy in the UK62 Modified penalty function method for optimal social welfare of electric power - with transmission constraints63 Optimization of blood - with shortened shelf lives and ABO compatibility64 Diversified firms on dynamical - cope with financial crisis better65 Securitization of energy -s in China66 Optimal design of the auto parts - for JIT operations: Sequential bifurcation factor screening and multi-response surface methodology67 Achieving sustainable -s through energy justice68 - agility: Securing performance for Chinese manufacturers69 Energy price risk and the sustainability of demand side -s70 Strategic and tactical mathematical programming models within the crude oil - context - A review71 An analysis of the structural complexity of -/s72 Business process re-design methodology to support - integration73 Could - technology improve food operators’ innovativeness? A developing country’s perspective74 RFID-enabled process reengineering of closed-loop -s in the healthcare industry of Singapore75 Order-Up-To policies in Information Exchange -s76 Robust design and operations of hydrocarbon biofuel - integrating with existing petroleum refineries considering unit cost objective77 Trade-offs in - transparency: the case of Nudie Jeans78 Healthcare - operations: Why are doctors reluctant to consolidate?79 Impact on the optimal design of bioethanol -s by a new European Commission proposal80 Managerial research on the pharmaceutical - – A critical review and some insights for future directions81 - performance evaluation with data envelopment analysis and balanced scorecard approach82 Integrated - design for commodity chemicals production via woody biomass fast pyrolysis and upgrading83 Governance of sustainable -s in the fast fashion industry84 Temperature ,for the quality assurance of a perishable food -85 Modeling of biomass-to-energy - operations: Applications, challenges and research directions86 Assessing Risk Factors in Collaborative - with the Analytic Hierarchy Process (AHP)87 Random / models and sensitivity algorithms for the analysis of ordering time and inventory state in multi-stage -s88 Information sharing and collaborative behaviors in enabling - performance: A social exchange perspective89 The coordinating contracts for a fuzzy - with effort and price dependent demand90 Criticality analysis and the -: Leveraging representational assurance91 Economic model predictive control for inventory ,in -s92 -,ontology from an ontology engineering perspective93 Surplus division and investment incentives in -s: A biform-game analysis94 Biofuels for road transport: Analysing evolving -s in Sweden from an energy security perspective95 -,executives in corporate upper echelons Original Research Article96 Sustainable -,in the fast fashion industry: An analysis of corporate reports97 An improved method for managing catastrophic - disruptions98 The equilibrium of closed-loop - super/ with time-dependent parameters99 A bi-objective stochastic programming model for a centralized green - with deteriorating products100 Simultaneous control of vehicle routing and inventory for dynamic inbound -101 Environmental impacts of roundwood - options in Michigan: life-cycle assessment of harvest and transport stages102 A recovery mechanism for a two echelon - system under supply disruption103 Challenges and Competitiveness Indicators for the Sustainable Development of the - in Food Industry104 Is doing more doing better? The relationship between responsible -,and corporate reputation105 Connecting product design, process and - decisions to strengthen global - capabilities106 A computational study for common / design in multi-commodity -s107 Optimal production and procurement decisions in a - with an option contract and partial backordering under uncertainties108 Methods to optimise the design and ,of biomass-for-bioenergy -s: A review109 Reverse - coordination by revenue sharing contract: A case for the personal computers industry110 SCOlog: A logic-based approach to analysing - operation dynamics111 Removing the blinders: A literature review on the potential of nanoscale technologies for the ,of -s112 Transition inertia due to competition in -s with remanufacturing and recycling: A systems dynamics mode113 Optimal design of advanced drop-in hydrocarbon biofuel - integrating with existing petroleum refineries under uncertainty114 Revenue-sharing contracts across an extended -115 An integrated revenue sharing and quantity discounts contract for coordinating a - dealing with short life-cycle products116 Total JIT (T-JIT) and its impact on - competency and organizational performance117 Logistical - design for bioeconomy applications118 A note on ―Quality investment and inspection policy in a supplier-manufacturer -‖119 Developing a Resilient -120 Cyber - risk ,: Revolutionizing the strategic control of critical IT systems121 Defining value chain architectures: Linking strategic value creation to operational - design122 Aligning the sustainable - to green marketing needs: A case study123 Decision support and intelligent systems in the textile and apparel -: An academic review of research articles124 -,capability of small and medium sized family businesses in India: A multiple case study approach125 - collaboration: Impact of success in long-term partnerships126 Collaboration capacity for sustainable -,: small and medium-sized enterprises in Mexico127 Advanced traceability system in aquaculture -128 - information systems strategy: Impacts on - performance and firm performance129 Performance of - collaboration – A simulation study130 Coordinating a three-level - with delay in payments and a discounted interest rate131 An integrated framework for agent basedinventory–production–transportation modeling and distributed simulation of -s132 Optimal - design and ,over a multi-period horizon under demand uncertainty. Part I: MINLP and MILP models133 The impact of knowledge transfer and complexity on - flexibility: A knowledge-based view134 An innovative - performance measurement system incorporating Research and Development (R&D) and marketing policy135 Robust decision making for hybrid process - systems via model predictive control136 Combined pricing and - operations under price-dependent stochastic demand137 Balancing - competitiveness and robustness through ―virtual dual sourcing‖: Lessons from the Great East Japan Earthquake138 Solving a tri-objective - problem with modified NSGA-II algorithm 139 Sustaining long-term - partnerships using price-only contracts 140 On the impact of advertising initiatives in -s141 A typology of the situations of cooperation in -s142 A structured analysis of operations and -,research in healthcare (1982–2011143 - practice and information quality: A - strategy study144 Manufacturer's pricing strategy in a two-level - with competing retailers and advertising cost dependent demand145 Closed-loop -/ design under a fuzzy environment146 Timing and eco(nomic) efficiency of climate-friendly investments in -s147 Post-seismic - risk ,: A system dynamics disruption analysis approach for inventory and logistics planning148 The relationship between legitimacy, reputation, sustainability and branding for companies and their -s149 Linking - configuration to - perfrmance: A discrete event simulation model150 An integrated multi-objective model for allocating the limited sources in a multiple multi-stage lean -151 Price and leadtime competition, and coordination for make-to-order -s152 A model of resilient -/ design: A two-stage programming with fuzzy shortest path153 Lead time variation control using reliable shipment equipment: An incentive scheme for - coordination154 Interpreting - dynamics: A quasi-chaos perspective155 A production-inventory model for a two-echelon - when demand is dependent on sales teams׳ initiatives156 Coordinating a dual-channel - with risk-averse under a two-way revenue sharing contract157 Energy supply planning and - optimization under uncertainty158 A hierarchical model of the impact of RFID practices on retail - performance159 An optimal solution to a three echelon -/ with multi-product and multi-period160 A multi-echelon - model for municipal solid waste ,system 161 A multi-objective approach to - visibility and risk162 An integrated - model with errors in quality inspection and learning in production163 A fuzzy AHP-TOPSIS framework for ranking the solutions of Knowledge ,adoption in - to overcome its barriers164 A relational study of - agility, competitiveness and business performance in the oil and gas industry165 Cyber - security practices DNA – Filling in the puzzle using a diverse set of disciplines166 A three layer - model with multiple suppliers, manufacturers and retailers for multiple items167 Innovations in low input and organic dairy -s—What is acceptable in Europe168 Risk Variables in Wind Power -169 An analysis of - strategies in the regenerative medicine industry—Implications for future development170 A note on - coordination for joint determination of order quantity and reorder point using a credit option171 Implementation of a responsive - strategy in global complexity: The case of manufacturing firms172 - scheduling at the manufacturer to minimize inventory holding and delivery costs173 GBOM-oriented ,of production disruption risk and optimization of - construction175 Alliance or no alliance—Bargaining power in competing reverse -s174 Climate change risks and adaptation options across Australian seafood -s – A preliminary assessment176 Designing contracts for a closed-loop - under information asymmetry 177 Chemical - modeling for analysis of homeland security178 Chain liability in multitier -s? Responsibility attributions for unsustainable supplier behavior179 Quantifying the efficiency of price-only contracts in push -s over demand distributions of known supports180 Closed-loop -/ design: A financial approach181 An integrated -/ design problem for bidirectional flows182 Integrating multimodal transport into cellulosic biofuel- design under feedstock seasonality with a case study based on California183 - dynamic configuration as a result of new product development184 A genetic algorithm for optimizing defective goods - costs using JIT logistics and each-cycle lengths185 A -/ design model for biomass co-firing in coal-fired power plants 186 Finance sourcing in a -187 Data quality for data science, predictive analytics, and big data in -,: An introduction to the problem and suggestions for research and applications188 Consumer returns in a decentralized -189 Cost-based pricing model with value-added tax and corporate income tax for a -/190 A hard nut to crack! Implementing - sustainability in an emerging economy191 Optimal location of spelling yards for the northern Australian beef -192 Coordination of a socially responsible - using revenue sharing contract193 Multi-criteria decision making based on trust and reputation in -194 Hydrogen - architecture for bottom-up energy systems models. Part 1: Developing pathways195 Financialization across the Pacific: Manufacturing cost ratios, -s and power196 Integrating deterioration and lifetime constraints in production and - planning: A survey197 Joint economic lot sizing problem for a three—Layer - with stochastic demand198 Mean-risk analysis of radio frequency identification technology in - with inventory misplacement: Risk-sharing and coordination199 Dynamic impact on global -s performance of disruptions propagation produced by terrorist acts。
高三英语英语学习大数据分析单选题40题

高三英语英语学习大数据分析单选题40题1.In the era of big data, we need to analyze large amounts of information _____.A.thoroughlyB.approximatelyC.randomlyD.occasionally答案:A。
thoroughly 意为“彻底地、完全地”;approximately 意为“大约、近似地”;randomly 意为“随机地、任意地”;occasionally 意为“偶尔、间或”。
在大数据时代,我们需要彻底地分析大量信息,所以选A。
2.Big data can provide _____ insights into customer behavior.A.preciousB.valuableC.worthlessD.trivial答案:B。
precious 意为“珍贵的、宝贵的”,通常用于形容物品或情感;valuable 意为“有价值的”,可用于形容信息、建议等;worthless 意为“无价值的”;trivial 意为“琐碎的、不重要的”。
大数据能提供有价值的关于客户行为的见解,所以选B。
3.The analysis of big data requires powerful _____ tools.putationalB.manualC.primitiveD.ineffective答案:A。
computational 意为“计算的”;manual 意为“手工的”;primitive 意为“原始的”;ineffective 意为“无效的”。
大数据分析需要强大的计算工具,所以选A。
4.Big data analytics can help businesses make more _____ decisions.rmedB.uninformedC.randomD.hasty答案:A。
informed 意为“有根据的、明智的”;uninformed 意为“无知的、未被通知的”;random 意为“随机的”;hasty 意为“匆忙的”。
Value Function Approximation on Non-Linear Manifolds for Robot Motor Control
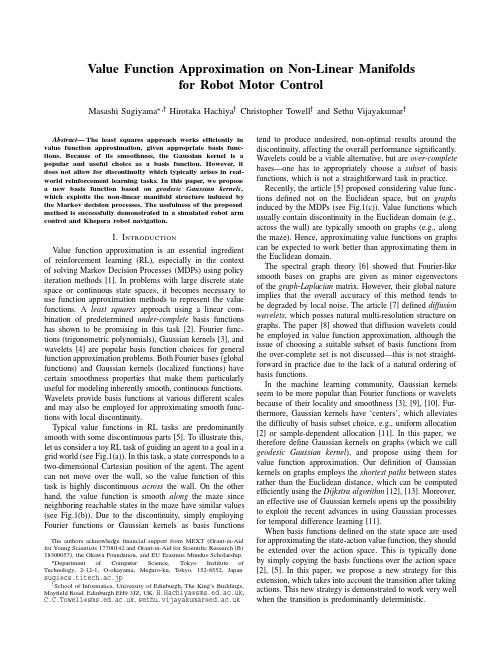
Value Function Approximation on Non-Linear Manifoldsfor Robot Motor ControlMasashi Sugiyama Hirotaka Hachiya Christopher Towell and Sethu VijayakumarAbstract—The least squares approach works efficiently in value function approximation,given appropriate basis func-tions.Because of its smoothness,the Gaussian kernel is a popular and useful choice as a basis function.However,it does not allow for discontinuity which typically arises in real-world reinforcement learning tasks.In this paper,we propose a new basis function based on geodesic Gaussian kernels, which exploits the non-linear manifold structure induced by the Markov decision processes.The usefulness of the proposed method is successfully demonstrated in a simulated robot arm control and Khepera robot navigation.I.I NTRODUCTIONV alue function approximation is an essential ingredient of reinforcement learning(RL),especially in the context of solving Markov Decision Processes(MDPs)using policy iteration methods[1].In problems with large discrete state space or continuous state spaces,it becomes necessary to use function approximation methods to represent the value functions.A least squares approach using a linear com-bination of predetermined under-complete basis functions has shown to be promising in this task[2].Fourier func-tions(trigonometric polynomials),Gaussian kernels[3],and wavelets[4]are popular basis function choices for general function approximation problems.Both Fourier bases(global functions)and Gaussian kernels(localized functions)have certain smoothness properties that make them particularly useful for modeling inherently smooth,continuous functions. Wavelets provide basis functions at various different scales and may also be employed for approximating smooth func-tions with local discontinuity.Typical value functions in RL tasks are predominantly smooth with some discontinuous parts[5].To illustrate this, let us consider a toy RL task of guiding an agent to a goal in a grid world(see Fig.1(a)).In this task,a state corresponds to a two-dimensional Cartesian position of the agent.The agent can not move over the wall,so the value function of this task is highly discontinuous across the wall.On the other hand,the value function is smooth along the maze since neighboring reachable states in the maze have similar values (see Fig.1(b)).Due to the discontinuity,simply employing Fourier functions or Gaussian kernels as basis functions The authors acknowledgefinancial support from MEXT(Grant-in-Aid for Y oung Scientists17700142and Grant-in-Aid for Scientific Research(B) 18300057),the Okawa Foundation,and EU Erasmus Mundus Scholarship.Department of Computer Science,Tokyo Institute of Technology,2-12-1,O-okayama,Meguro-ku,Tokyo,152-8552,Japan sugi@cs.titech.ac.jpSchool of Informatics,University of Edinburgh,The King’s Buildings, Mayfield Road,Edinburgh EH93JZ,UK.H.Hachiya@, C.C.Towell@,sethu.vijayakumar@ tend to produce undesired,non-optimal results around the discontinuity,affecting the overall performance significantly. Wavelets could be a viable alternative,but are over-complete bases—one has to appropriately choose a subset of basis functions,which is not a straightforward task in practice. Recently,the article[5]proposed considering value func-tions defined not on the Euclidean space,but on graphs induced by the MDPs(see Fig.1(c)).V alue functions which usually contain discontinuity in the Euclidean domain(e.g., across the wall)are typically smooth on graphs(e.g.,along the maze).Hence,approximating value functions on graphs can be expected to work better than approximating them in the Euclidean domain.The spectral graph theory[6]showed that Fourier-like smooth bases on graphs are given as minor eigenvectors of the graph-Laplacian matrix.However,their global nature implies that the overall accuracy of this method tends to be degraded by local noise.The article[7]defined diffusion wavelets,which posses natural multi-resolution structure on graphs.The paper[8]showed that diffusion wavelets could be employed in value function approximation,although the issue of choosing a suitable subset of basis functions from the over-complete set is not discussed—this is not straight-forward in practice due to the lack of a natural ordering of basis functions.In the machine learning community,Gaussian kernels seem to be more popular than Fourier functions or wavelets because of their locality and smoothness[3],[9],[10].Fur-thermore,Gaussian kernels have‘centers’,which alleviates the difficulty of basis subset choice,e.g.,uniform allocation [2]or sample-dependent allocation[11].In this paper,we therefore define Gaussian kernels on graphs(which we call geodesic Gaussian kernel),and propose using them for value function approximation.Our definition of Gaussian kernels on graphs employs the shortest paths between states rather than the Euclidean distance,which can be computed efficiently using the Dijkstra algorithm[12],[13].Moreover, an effective use of Gaussian kernels opens up the possibility to exploit the recent advances in using Gaussian processes for temporal difference learning[11].When basis functions defined on the state space are used for approximating the state-action value function,they should be extended over the action space.This is typically done by simply copying the basis functions over the action space [2],[5].In this paper,we propose a new strategy for this extension,which takes into account the transition after taking actions.This new strategy is demonstrated to work very well when the transition is predominantly deterministic.→→→↓→→→→→→→→→→↑→↑→→→→↓→↓→→→→→→→→→→↑→→→↓↓→↓↓→→→→→↑→↑→↑→→→→↓→→→→→→→↑↑→↑→→→→→↓↓→↓→→→→→→→→↑→→→→→→↓→→→→→→→→↑→→→→→→→→↓↓↓↓↓→→→↑↑→→→↑→↓↓→↓↓↓↓↓→→→↑→→↑→↑→↓↓↓↓↓↓↓↓→→↑↑↑↑↑↑↑↑→↑→→→→→↑↑→→→→↑↑↑↑↑→↑→→↑→→→→→↑↑↑→→→→→→↑→→↑→↑↑↑↑↑→→→↑↑→↑↑↑→→→→→↑→↑→↑↑↑↑↑↑↑↑↑→→→↑↑↑↑↑↑↑→↑↑↑↑↑↑↑→→↑↑↑↑↑↑↑↑↑↑→↑↑↑→↑→↑↑↑↑↑↑↑↑↑↑↑↑↑↑↑↑↑→↑↑↑↑↑↑↑↑↑↑↑↑↑↑↑↑↑↑↑↑↑↑↑↑↑↑↑↑↑↑↑↑↑↑123456789101112131415161718192021 1234567891011121314151617181920(a)(b)(c)Fig.1.An illustrative example of an RL task of guiding an agent to a goal in the grid world.(a)Black areas are walls over which the agent cannot move while the goal is represented in gray.Arrows on the grids represent one of the optimal policies.(b)Optimal state value function(in log-scale).(c)Graph induced by the MDP and a random policy.II.F ORMULATION OF THE R EINFORCE MENT L EARNINGP ROBLEMIn this section,we briefly introduce the notation and reinforcement learning(RL)formulation that we will use across the manuscript.A.Markov Decision ProcessesLet us consider a Markov decision process(MDP),where is afinite1 set of states,is afinite set of actions,is the joint probability of making a transition to state if action is taken in state,is an immediate reward for making a transition from to by action, and is the discount factor for future rewards.The expected reward for a state-action pair is given as(1)Let be a deterministic policy which the agent follows.In this paper,we focus on deterministic policies since there always exists an optimal deterministic policy[2]. Let be a state-action value function for policy,which indicates the expected long-term discounted sum of rewards the agent receives when the agent takes action in state and follows policy thereafter.satisfies the following Bellman equation:(2)The goal of RL is to obtain a policy which results in maximum amount of long-term rewards.The optimal policy is defined as,whereis the optimal state-action value function defined by.1For the moment,we focus on discrete state spaces.In Sec.III-D,we extend the proposed method to the continuous state space.B.Least Squares Policy IterationIn practice,the optimal policy can not be directly obtained since and are usually unknown; even when they are known,direct computation of is often computationally intractable.To cope with this problem,the paper[2]proposed ap-proximating the state-action value function using a linear model:(3)where is the number of basis functions which is usu-ally much smaller than the number of states,are the parameters to be learned, denotes the transpose,and are pre-determined basis functions.Note that and can depend on policy,but we do not show the explicit dependence for the sake of simplicity.Assume we have roll-out samples from a sequence of actions:,where each tuple denotes the agent experiencing a transition fromto on taking action with immediate reward.Under the Least Squares Policy Iteration(LSPI)formulation[2], the parameter is learned so that the Bellman equation (2)is optimally approximated in the least squares sense2. Consequently,based on the approximated state-action value function with learned parameter,the policy is updated as(4)Approximating the state-action value function and updating the policy is iteratively carried out until some convergence criterion is met.III.G AUSSIA N K ERNELS ON G RAPHSIn the LSPI algorithm,the choice of basis functionsis an open design issue.Gaussian kernels have traditionally been a popular choice[2],[11],but they 2There are two alternative approaches:Bellman residual minimization andfixed point approximation.We take the latter approach following the suggestion in the reference[2].can not approximate discontinuous functions well.Recently, more sophisticated methods of constructing suitable basis functions have been proposed,which effectively make use of the graph structure induced by MDPs[5].In this section, we introduce a novel way of constructing basis functions by incorporating the graph structure;while relation to the existing graph-based methods is discussed in the separate report[14].A.MDP-Induced GraphLet be a graph induced by an MDP,where states are nodes of the graph and the transitions with non-zero transition probabilities from one node to another are edges. The edges may have weights determined, e.g.,based on the transition probabilities or the distance between nodes. The graph structure corresponding to an example grid world shown in Fig.1(a)is illustrated in Fig.1(c).In practice, such graph structure(including the connection weights)are estimated from samples of afinite length.We assume that the graph is connected.Typically,the graph is sparse in RL tasks,i.e.,,where is the number of edges and is the number of nodes.B.Ordinary Gaussian KernelsOrdinary Gaussian kernels(OGKs)on the Euclidean space are defined as(5) where are the Euclidean distance between states and;for example,when the Cartesian positions of and in the state space are given by and,respectively.is the variance parameter of the Gaussian kernel.The above Gaussian function is defined on the state space ,where is treated as a center of the kernel.In order to employ the Gaussian kernel in the LSPI algorithm,it needs to be extended over the state-action space. This is usually carried out by simply‘copying’the Gaussian function over the action space[2],[5].More precisely:let the total number of basis functions be,where is the number of possible actions and is the number of Gaussian centers.For the-th action and for the-th Gaussian center,the-th basis function is defined as(6)where is the indicator function,i.e.,if otherwise.Gaussian kernels are shift-invariant,i.e.,they do not directly depend on the absolute positions and,but depend only on the difference between two positions;more specifically,Gaussian kernels depend only on the distance between two positions.C.Geodesic Gaussian KernelsOn graphs,a natural definition of the distance would be the shortest path.So we define Gaussian kernels on graphs based on the shortest path:(7) where denotes the shortest path from state to state.The shortest path on a graph can be interpreted as a discrete approximation to the geodesic distance on a non-linear manifold[6].For this reason,we call Eq.(7)a geodesic Gaussian kernel(GGK).Shortest paths on graphs can be efficiently computed using the Dijkstra algorithm[12].With its naive implementation, computational complexity for computing the shortest paths from a single node to all other nodes is,where is the number of nodes.If the Fibonacci heap is employed,the computational complexity can be reduced to[13],where is the number of edges.Since the graph in value function approximation problems is typically sparse (i.e.,),using the Fibonacci heap provides signifi-cant computational gains.Furthermore,there exist various approximation algorithms which are computationally very efficient(see[15]and and references therein). Analogous to OGKs,we need to extend GGKs to the state-action space for using them in the LSPI method.A naive way is to just employ Eq.(6),but this can cause a‘shift’in the Gaussian centers since the state usually changes when some action is taken.To incorporate this transition,we propose defining the basis functions as the expectation of Gaussian functions after transition,i.e.,(8) This shifting scheme is expected to work well when the transition is predominantly deterministic(see Sec.IV and Sec.V-A for experimental evaluation).D.Extension to Continuous State SpacesSo far,we focused on discrete state spaces.However,the concept of GGKs can be naturally extended to continuous state spaces,which is explained here.First,the continuous state space is discretized,which gives a graph as a discrete approximation to the non-linear manifold structure of the continuous state space.Based on the graph,we construct GGKs in the same way as the discrete case.Finally,the discrete GGKs are interpolated,e.g.,using a linear method to give continuous GGKs.Although this procedure discretizes the continuous state space,it must be noted that the discretization is only for the purpose of obtaining the graph as a discrete approximation of the continuous non-linear manifold;the resulting basis func-tions themselves are continuously interpolated and hence,the state space is still treated as continuous as opposed to other conventional discretization procedures.→→→→↓→→→→↑→→→→→→→↓→↓→↑→→↓→→↑↓↓↓↓→↑→→↓→↑↑↓↓↓↓→↑→→↓→→→→→→→→→→→→↓→→→↓→→↑→→→→→→→→→→→↑↑↑→→→→→→↓→→→→↓→→→→↓→↑→→↓↓↓↓→↓→↑↑→→→→→↓↓→↓→→→→→→↓↓↓↓↓↓→→→→↑→↓↓↓↓↓↓→→→↑→→→→→↑↑↑→→↑→↑↑↑↑↑↑↑↑↑↑↑↑↑↑↑↑↑↑↑↑↑↑↑↑12345678910111213141516171819201234567891011121314(a)Sutton’s maze→→↓→→↓→↓→→→→↑→→→→↑→→→→→↓→→→→→→→→→→→↑→→→→→↓→→→→→↑→→→↑↑↑↓→→→→↓→↓→→→↑→↑↑↑↑↑↓↓↓↓↓↓↓↓→→↑↑↑↑↑↑↑↑↓→→↓↓↓↓↓↓↓↓→→→→→↑↑↑↑↑↓↓→→→↓↓↓→→↑→→→→↑↑↑↓↓↓↓↓↓↓↓↓→→→→→↑↑↑↑↓↓↓↓↓→→→→→→→→↑↑↑↑↑↓↓↓↓↓↓↓→→→↑↑↑↑↑↑↑↑↓↓→→→↓↓→→→→↓↓→→→→→→↓→↓→→→→→→↓→↓↓→→↓→→↓→↓↓↓→→→↓↓↓↓↓↓↓↓→→→→↓↓↓↓↓↓→→→→↓↓↓↓↓↓↓→↓↓↓↓↓↓↓↓↓↓↓↓→→↓→↓→↓↓↓↓↓↓↓↓↓↓↓↓↓↓↓↓↓12345678910111213141516171819201234567891011121314151617181920(b)Three-room maze(a)Sutton’s maze(b)Three-room mazeFig.3.Mean squared error of approximated value functions averaged overtrials for the Sutton and three room mazes.In the legend,the standard deviation of GGKs and OGKs is denoted in the bracket.(b)Three-room mazeFig.4.Fraction of optimal states averaged over trials for the Sutton and three room mazes.IV.E XPERIMENTAL C OMPARISONIn this section,we report the results of extensive and systematic experiments for illustrating the difference between GGKs and other basis functions.We employ two standard grid world problems illustrated in Fig.2,and evaluate the goodness of approximated value functions by computing the mean squared error (MSE)with respect to the optimal value function and the goodness of obtained policies by calculating the fraction of states from which the agent can get to the goal optimally (i.e.,in the shortest number of steps).series of random walk of length are gathered as training samples,which are used for estimating the graph as well as the transition probability and expected reward.We set the edge weights in the graph to (which is equivalent to the Euclidean distance between two nodes).We test GGKs,OGKs,graph-Laplacian eigenfunctions (GLEs)[5],and diffusion wavelets (DWs)[8].This simulation is repeated times for each maze and each method,randomly changing training samples in each run.The mean of the above scores as a function of the number of bases is plotted in Fig.4.Note that the actual number of bases is four times more becauseof the extension of basis functions over the action space (see Eq.(6)and Eq.(8)).GGKs and OGKs are tested with small/medium/large Gaussian widths.Fig.3depicts MSEs of the approximated value functions for each method.They show that MSEs of GGKs with width ,OGKs with width ,GLEs,and DWs are very small and decrease as the number of kernels increases.On the other hand,MSEs of GGKs and OGKs with medium/large width are large and increase as the number of kernels increases.Therefore,from the viewpoint of approximation quality of the value functions,the width of GGKs and OGKs should be small.Fig.4depicts the fraction of optimal states in the obtained policy.They show that overall GGKs with medium/large width give much better policies than OGKs,GLEs,and DWs.An interesting finding from the graphs is that GGKs tend to work better if the Gaussian width is large,while OGKs show the opposite trend;this may be explained as follows.Tails of OGKs extend across the wall.Therefore,OGKs with large width tend to produce undesired value function and erroneous policies around the partitions.This tail effect can be alleviated if the Gaussian width is made small.However,this in turn makes the approximated value function fluctuating;so the resulting policies are still erroneous.The fluctuation problem with a small Gaussian width seems to be improved if the number of bases is increased,while the tail effect with a large Gaussian width still remains even when the number of bases is increased.On the other hand,GGKs do not suffer from the tail problem thanks to the geodesic construction.Therefore,GGKs allows us to make the width large without being affected by the discontinuity across the wall.Consequently,smooth value functions along the maze are produced and hence better policies can be obtained by GGKs with large widths.This result highlights a helpful property since it alleviates the practical issue of determining the values of the Gaussian width parameter.V.A PPL ICATIONSAs discussed in the previous section,the proposed GGKs bring a number of preferable properties for making value function approximation effective.In this section,we in-vestigate the application of the GGK-based method to the challenging problems of a(simulated)robot arm control and mobile robot navigation and demonstrate its usefulness. A.Robot Arm ControlWe use a simulator of a two-joint robot arm(moving in a plane)illustrated in Fig.5(a).The task is to lead the end effector(‘hand’)of the arm to an object while avoiding the obstacles.Possible actions are to increase or decrease the angle of each joint(‘shoulder’and‘elbow’)by degrees in the plane,simulating coarse stepper motor joints.Thus the state space is the-dimensional discrete space consisting of two joint angles as illustrated in Fig.5(b).The black area in the middle corresponds to the obstacle in the joint angle state space.The action space involves actions:increase or decrease one of the joint angles.We give a positive immediate reward when the robot’s end effector touches the object;otherwise the robot receives no immediate reward. Note that actions which make the arm collide with obstacles are not allowed.The discount factor is set to. In this environment,we can change the joint angle exactly by degrees,so the environment is deterministic.However, because of the obstacles,it is difficult to explicitly compute an inverse kinematic model;furthermore,the obstacles intro-duce discontinuity in value functions.Therefore,this robot arm control task is an interesting test bed for investigating the behaviour of GGKs.We collected training samples from series of random arm movements,where the start state is chosen randomly in each trial.The graph induced by the above MDP consists of nodes and we assigned uniform weights to the edges.There are totally goal states in this environment (see Fig.5(b)),so we put thefirst Gaussian centers at the goals and the remaining centers are chosen randomly in the state space.For GGKs,kernel functions are extended over the action space using the shifting scheme(see Eq.(8))since the transition is deterministic in this experiment.Fig.6illustrates the value functions approximated using GGKs and OGKs3.The graphs show that GGKs give a nice smooth surface with obstacle-induced discontinuity sharply preserved,while OGKs tend to smooth out the discontinuity. This makes a significant difference in avoiding the obstacle: from‘A’to‘B’in Fig.5(b),the GGK-based value function results in a trajectory that avoids the obstacle(see Fig.6(a)). On the other hand,the OGK-based value function yields a trajectory that tries to move the arm through the obstacle by following the gradient upward(see Fig.6(b)).The latter causes the arm to get stuck behind the obstacle.Fig.7summarizes the performance of GGKs and OGKs measured by the percentage of successful movements(i.e., the end effector reaches the target)averaged over indepen-dent runs.More precisely,in each run,totally training samples are collected using a different random seed,a policy is then computed by the GGK-or OGK-based method using LSPI,and the obtained policy is tested.This graph shows that GGKs remarkably outperform OGKs since the arm can successfully avoid the obstacle.The performance of OGK does not go beyond even when the number of kernels is increased.This is caused by the‘tail effect’of ordinary Gaussian functions;the OGK-based policy can not lead the end effector to the object if it starts from the bottom-left half of the state spaceWhen the number of kernels is increased,the performance of both GGKs and OGKs once gets worse at around.This would be caused by our kernel center allocation strategy:thefirst kernels are put at the goal states and the remaining kernel centers are chosen randomly.When is less than or equal to,the approximated value function tends to have a unimodal profile since all kernels are put at the goal states.However,when is larger than,this unimodality is broken and the surface of the approximated value function gets slightlyfluctuated.This smallfluctuation can cause an error in policies and therefore the performance is degraded at around.This performance degradation tends to be improved as the number of kernels is further increased.Overall,the above result shows that when GGKs are combined with our kernel center allocation strategy,almost perfect policies can be obtained with a very small number of kernels.Therefore,the proposed method is computationally very advantageous.B.Robot Agent NavigationThe above simple robot arm control simulation shows that the GGK method is promising.Here we apply GGKs to a more challenging task of a mobile robot navigation,which involves a high-dimensional and continuous state space. We employ a Khepera robot illustrated in Fig.8(a)on a navigation task.A Khepera is equipped with infra-red 3For illustration purposes,let us display the state value function ,which is the expected long-term discounted sum of rewards the agent receives when the agent takes actions following policy from state. From the definition,it can be confirmed that is expressed.(a)Aschematic(b)State space(a)Geodesic Gaussian kernels(b)Ordinary Gaussian kernelsFig.6.Approximated value functions.Fig.7.Number of successful trials.sensors (‘s1’to ‘s8’in the figure)which measure the strength of the reflected light returned from surrounding obstacles.Each sensor produces a scalar value between and(which may be regarded as continuous):the sensor obtains the maximum value if an obstacle is just in front of the sensor and the value decreases as the obstacle gets farther till it reaches the minimum value .Therefore,the state space is -dimensional and continuous.The Khepera has two wheels and takes the following defined actions:forward,left-rotation,right-rotation and backward (i.e.,the action space contains actions).The speed of the left and right wheels for each action is described in Fig.8(a)in the bracket (the unit is pulse per 10milliseconds).Note that the sensor values and the wheel speed are highly stochastic due to the change of the ambient light,noise,the skid etc.Furthermore,perceptual aliasing occurs due to the limited range and resolution of sensors.Therefore,the state transition is highly stochastic.We set the discount factor to .The goal of the navigation task is to make the Khepera explore the environment as much as possible.To this end,we give a positive reward when the Khepera moves forward and a negative reward when the Khepera collides with an obstacle.We do not give any reward to the left/right rotation and backward actions.This reward design encourages the Khepera to go forward without hitting obstacles,through which extensive exploration in the environment could be achieved.We collected training samples fromseries of random movements in a fixed environment with several ob-stacles (see Fig.9(a)).Then we constructed a graph from the gathered samples by discretizing the continuous state space using the Self-Organizing Map (SOM)[16].The number of nodes (states)in the graph is set to (equivalent with the SOM map size of );this value is computed by the standard rule-of-thumb formula [17],where is the number of samples.The connectivity of the graph is determined by the state transition probability computed from the samples,i.e.,if there is a state transition from one node to another in the samples,an edge is established between these two nodes and the edge weight is set according to the Euclidean distance between them.Fig.8(b)illustrates an example of the obtained graph structure—for visualization purposes,we projected the -dimensional state space onto a -dimensional subspace spanned by(9)The -th element in the above bases corresponds to the output of the -th sensor (see Fig.8(a)).Therefore,the projection onto this subspace roughly means that the horizontal axis corresponds to the distance to the left/right obstacle,while the vertical axis corresponds to the distance to the front/back obstacle.For clear visibility,we only displayed the edges whose weight is less than .This graph has a notable feature:the nodes around the region ‘B’in the figure are(a)A schematic(b)State space projected onto a -dimensional subspace for visualization.Fig.8.Khepera robot.(a)Training(b)TestFig.9.Simulation environment(a)Geodesic Gaussian kernels(b)Ordinary Gaussian kernels Fig.10.Examples of obtained policy .Fig.11.Average amount of exploration.putation time.directly connected to the nodes at ‘A ’,but are not directly connected to the nodes at ‘C’,‘D’,and ‘E’.This implies that the geodesic distance from ‘B’to ‘C’,‘D’,or ‘E’is large,although the Euclidean distance is small.Since the transition from one state to another is highly stochastic in the current experiment,we decided to simply duplicate the GGK function over the action space (see Eq.(6)).For obtaining continuous GGKs,GGK functions need to be interpolated (see Sec.III-D).We may employ a simple linear interpolation method in general.However,the current experiment has unique characteristics—at least one of the sensor values is always zero since the Khepera is never completely surrounded by obstacles.Therefore,samples are always on the surface of the -dimensional hypercube-shaped state space.On the other hand,the node centers determined by the SOM are not generally on the surface.This means thatany sample is not included in the convex hull of its nearest nodes and we need to extrapolate the function value.Here,we simply add the Euclidean distance between the sample and its nearest node when computing kernel values;more precisely,for a state that is not generally located on a node center,the GGK-based basis function is defined as(10)where is the node closest to in the Euclidean distance.Fig.10illustrates an example of actions selected at each node by the GGK-based and OGK-based policies.We usedkernels and set the width to .The symbols ‘’,’’,‘’,and ‘’in the figure indicates forward,backward,left rotation,and right rotation actions.This shows that there is。
FastSHAPES

Shapes can be completed in a fraction of the time manually lofted patterns would allow and importantly, they are fabricated “right first Simply model the item in 3D T HE F AST WAY TO P RODUCE P ATTERNS R EADY FOR C UTTING“R IGHT F IRST T IME ”Take out the guesswork and eliminate the need for Calculations! This computer based systemcan handle thousands morecomputations which isreflected in the capacity and accuracy of the system. Particularly when compared to hand lofted pattern developments. FastSHAPES ® produces clean and precise geometry that you can export as a DXF (CAD) fileor into your CAM system forediting and/or nesting ifdesired.Highly accurate components offer a marked improvementin weld qu a lit y a n d consistency resulting in better fitup. ®System I NSTANT P LATE D EVELOPMENTSFastSHAPES ® completed this complex hydro-electric component in only minutesready for burning, with all forming detailsand even plate edge preparation (PEP).H IGHLIGHTS :• S PECIFICALLY DESIGNED FORTHICK PLATE!• I NCLUDES C OMMON , C OMPLEX AND H EAVY C ONSTRUCTION S HAPES • PRODUCTION-READY FLAT PATTERNS• F ULL M ANUFACTURING AND W ELD D ETAILS• A UTOMATIC & E ASY !• M ULTI -LANGUAGE SUPPORT • M ICROSOFT W INDOWSNuts and Bolts:Dynamic Display changes shape as data is enteredFull 3D Viewing inc stereoscopic, Plan, Elevation & Isometric ‘Cutaway’ feature lets you see inside the development Calculates Bend Lines, Forming Angle, Longitudinal Seam Off-sets and moreEvery component of a construc-tion can be viewed independently Plotted layouts2D & 3D DXF CAD output of shapes and flat patternIdeal for Boilermakers, Draftsmen & EngineersCommon ShapesFull welding preparation detailsFastSHAPES® is for medium to heavy plate manufacturing where the main jointing technology is welding. Complex multi componentsFastSHAPES ® has proven to offer substantial time & material savings ranging from mining buckets to wind-farm towers.FastSHAPES ® has also been used in the development of Naval Frigates and nuclear and conventional submarines.D ESIGNED FOR R EALWORLD A PPLICATIONSGreen Allowances Forming Angle Back to Back “Stitch” CuttingMarking of bend lines Mass of all Parts Assembly ListLongitudinal Seam OffsetsF ULL M ANUFACTURING D ETAILS TO I NTERNATIONAL S TANDARDSPower Transmission Power Transmission Chain Sprocket, Universal Sprocket, Rack and Pinion, Involute Gears.Heavy/Complex Transitions Heavy/Complex TransitionsTapered & Transforming Lobster-back Bends, Offset & Mitred Transformers, Conical Branches,Conical & Tubular Runs, Simple & Complex Birfircations.Common Blowpipe Common BlowpipeRectangle to Round, Elbow,Pipe Branch, Lobster, Multi Segment Cone, Oblique Cone, Cone Bifircate, Plate Branches.1976FastSHAPES ® offers themost dramatic savings when working with : • Large fabricated shapes which require a lot of additional fabrication information (often multi-segmented). • Complex equation based developments such as gears. • Predictable multi-component develop-ments.F a s t S H A P E S ® w a s specifically designed to be able to handle medium and thick plate. The program has been used in real world production solutions since 1989.Pipe branches, transitions, heavy ducting, anything for Hydro-electric, mining infra-structure or general heavy fabrication are handled superbly.FastSHAPES ® allows for multi strake, variable thickness construction with internal, mean or outside data entry.D EVELOP A FULL RANGE OF ‘P RODUCTION R EADY ’ F LAT P ATTERNSNote: With over 20 shapes in the library, FastSHAPES ® covers most of the shape development needs of fabricators and service centers.Full fabrication development includes Welds, Full/Partial Penetrations, Fillet, Butt/Groove. These large conical structures are used to support Turbines at a Wind Farm.FastSHAPES ® can rapidly produce useful estimation for a particular development via a Parts List (Cut Distance, Marking Distance, Mass of Cut Components).Annual Service Maintenance Agreements are also available. SMA’s give you Priority Support, Upgrades and M e m b e r D i s c o u n tEntitlements.Contact FastCAM for current pricing.The FastSHAPES ® System includes 30 days FREE technical support*technical support* to ensure that you are up and runningquickly. Free self-help is available via our web site and pay per incident support is availableas and when required.Training can be tailored to meet your needs. CAD experience is not r e q u i r e d h o w e v e rFastSHAPES ® does presumea working knowledge of fabrication techniques. Contact your local FastCAMOffice or reseller for more information. S PEED , A CCURACY & D ETAILING BENEFITS E STIMATION AND P ROTOTYPINGO FFERING S OLID S ERVICE , M AINTENANCE & S UPPORTA VAILABLE IN 3 DIFFERENT VERSIONS TO MEET YOUR N EEDS* Technical support by phone, fax, email. Excludes on-site, installation and O/S support.1976Tradesman in a Box™ Tradesman in a Box™Full shape development set to print co ordinates andtemplates.Ideal for hand cutting oroptical pattern production.FastSHAPES ® System SystemFull Shape Set. Multi Plate, Multi ThicknessOutput: 2D & 3D DXF, FastCAMfile, Coordinate Table.FastSHAPES ® / / FastCAM FastCAM ® NC Bundle NC BundleCombines FastSHAPES ® with the FastCAM ® System for a complete NC programming and nesting solution with integrated post processors.Refer to the FastCAM ® System brochure for more details.Note: For more details refer the FastSHAPES® Complete Technical Reference..FastSHAPES ® lets you develop even the most com-plex parts in minutes, model the item in 3D (if required) and produce a flat pattern with all manufacturing details.Design Draftsmen and Engineers use FastSHAPES ® to prototype designs knowing that they can be easily fabricated from plate. 2D & 3D DXF CAD outputsare very useful to take designs and patterns back into a CAD system for d o c u m e n t a t i o n a n d verification.Note: FastSHAPES ® can be used in conjunction with a CAD system however CAD is not a requirement.FastCAM Inc.—USA FastCAM Pty. Ltd.—Asia Pacific FastCAM China 8700 West Bryn Mawr, 96 Canterbury Road, No.34, 377 Chenhui Road, Suite 800 South, Middle Park, Victoria, 3206, Zhangjuang Tomson Garden, Chicago, 60631 3507 Australia Zhangjuang High Tech, Phone: 312 715 1535 Phone: 61 3 9699 9899 Pudong, Shanghai, 201203 Fax: 312 715 1536 Fax: 61 3 9699 7501 Phone: 8621 5080 3069 Email: fastcam@ Email: fastcam@.au Fax: 8621 5080 3071Email: fastcam@ Web: FastCAM has been supplying PC-based software for Burning, Shearing and Sawing/Drilling machines for over 25 years. The flagship product FastCAM ® offers unique integrated postprocessors, NC verification and NC code nesting that still sets it apart from other CAM and CAD/CAM systems. The new generation of FastCAM ® software is used in many countries, in many languages and in many different environments.Today the product line has been expanded to include dozens of trademarked products offering a wide range of solutions for metal fabrication and Service Center operation. FastCAM has OEM and Business Partners in North America, South America, China, Australia, New Zealand and Europe (Poland, UK, Czech, Hungary). We welcome all enquiries.F AST CAM W ORLDWIDE O FFICESFASTCAM SUPPORTS: BURNY, LYNX, WESTINGHOUSE, ESAB, PICOPATH, CREONICS, HANCOCK , FANUC, C & G, PCS, KOIKE SANSO, TANAKA, MESSER, FAGOR, FARLEY, ANCA,SIEMENS, JHE, HYBRID, MYNUC, ANCA, PDF32 AND MANY OTHERS. THIS IS NOT A COMPLETE LISTING — CONTACT YOUR RESELLER OR FASTCAM DIRECTLY FOR MORE. FastCAM ® will operate on most Pentium based PC’s with Microsoft Windows 98/ME/XP or NT4/2000 however the recommended system, particularly for nesting, is a Pentium IV 256/512Mb RAM 80Gb HDD 17” Monitor & Windows XP.Using FastSHAPES ®, this square to round transition was completed in less than 30 seconds ready to send to the burning machine. The development even included the line marking and number of degrees for forming every bend! Handles simple and complex shapes. All read directly into 2D and 3D CAD systems.。
A partial order approach to noisy fitness functions

A Partial Order Approach to Noisy Fitness FunctionsG¨unter RudolphDepartment of Computer ScienceUniversity of Dortmund44221Dortmund/Germanyrudolph@LS11.cs.uni-dortmund.deAbstract-If thefitness values are perturbed by noise then they do not have a definitive total order.As a consequence,tra-ditional selection procedures in evolutionary algorithms may lead to obscure solutions.A potential remedy is as follows:Construct a partial order on the set of noisyfit-ness values and apply those evolutionary algorithms that have been designed forfinding the minimal elements of partially ordered sets.These minimal elements are the only reasonable candidates for the unperturbed true so-lution.A method for reducing the number of candidate solutions is suggested.From a theoretical point of view it is worth mentioning that all convergence results for evo-lutionary algorithms with partially orderedfitness sets re-main valid for the approach considered here.1IntroductionThe Gaussian distribution is the predominant choice for mod-eling noise frequently observable in measurings of various kinds.Here,we hold the view that a noise distribution with unbounded support(like the Gaussian,Cauchy,Laplace,Lo-gistic,and others)may be quite unrealistic.Actually it is at least equally plausible to assume that the noise cannot exceed certain limits due to technical characteristics of the involved measurement unit.Even if a distributional shape close to a Gaussian appears reasonable we can resort to a symmetrical Beta distribution which can converge weakly to a Gaussian distribution under continuously increasing but bounded sup-port(see e.g.Evans et al.1993,p.36).This assumption will have significant theoretical and practical impacts on the evo-lutionary algorithms(EAs)considered here.Traditional measures for coping with noisyfitness func-tions in evolutionary algorithms include the resampling of the randomfitness value with averaging,the appropriate adjust-ment(i.e.,enlargement)of the population size,and in case of continuous search spaces also the rescaling of inherited mu-tations;see Beyer(2000)for a summary of work on EAs for noisyfitness functions.Here,we add yet another avenue for dealing with noisy fitness functions:Instead of using a selection procedure that is based on the totally ordered set of noisyfitness values we endow the probabilisticfitness set with an appropriate par-tial order and deploy EAs with those selection methods being explicitly designed for coping with arbitrary partially ordered fitness sets(Rudolph1998,2001;Rudolph and Agapie2000).Section2offers a brief introduction to partially orderedsets in general and in particular to interval orders(Fishburn 1985)which constitute thefirst step towards the partial or-der to be used later on.Since the noise is supposed to have bounded support we can easily equip these intervals with a probability measure(representing the noise distribution).Thus, the interval order turns to a partial order on random variables.This subject is detailed in Section3which also contains the presentation of the EA along with a discussion of the in-herited theoretical properties from the general case(Rudolph 2001).Preliminary experimental results can be found in Sec-tion4.2Partially Ordered SetsLet be a set.A reflexive,antisymmetric,and transitive re-lation“”on is termed a partial order relation whereas a strict partial order relation“”must be antireflexive,asym-metric,and transitive.The latter relation may be obtained by the former relation by setting.After these preparations one is in the position to turn to the actual objects of interest.Definition1Let be some set.If the partial order relation “”is valid on then the pair is called a partially ordered set(or short:poset).If for some thenis said to dominate.Distinct points are said to be comparable when either or.Otherwise,andare incomparable which is denoted by.If each pair of distinct points of a poset is comparable thenis called a totally ordered set or a chain.Dually,if each pair of distinct points of a poset are incomparable then is termed an antichain.For example,let be the set of closed intervals of and defineiffiffiffIt is easily seen that is a partially ordered set in which distinct intervals with a nonvoid intersection are incompara-ble.Similarly,the infinitely large but countable setwith withis a poset with incomparable elements whereas with is totally ordered and therefore a chain.An example for an antichain is the set of“minimal elements”introduced next.1Definition2An element is called a minimal element of the poset if there is no such that. The set of all minimal elements,denoted,is said to be complete if for each there is at least onesuch that.In contrast to infinitely large posets the completeness of is guaranteed forfinitely large posets.Of course, completeness of infinitely large posets is not precluded.For example,the set with is infinitely large and the set of minimal elements is complete.3Coping with Noisy Fitness Functions3.1AssumptionsLet be thefinite search set and assume that the determinis-ticfitness function is perturbed by additive noise ,i.e.,for.As mentioned ear-lier,here we insist that random variable has bounded and known support in form of a closed interval of.For exam-ple,may have a uniform or symmetric beta distribution on its support with.Atfirst it is assumed that every point/individual is evaluated only ter on this assumption is dropped.3.2Partial Order ApproachWhen an individual is evaluated viathen the noisyfitness value is an element of the interval.Since the EA only has knowledge of the support bound and in no case of the truefitness value,the noisy evaluation of only leads to the information that the truefitness value must be in the interval.Thus,each point or individual is associated with a realization of a random interval.Next we declare a strict partial order on these intervals and thereby also a strict partial order on the individuals.Let and w.l.o.g..If(1) then we define and thereby.This choice is reasonable because we can immediately infer fromthat with probability.One should mention that this partial order is a special case of a partial order introduced in Guddat et al.(1985),p.29.Moreover,notice that the connection to interval orders gets evident by the equivalence between equation(1)and(2) Thus,whenever two intervals as those above have a nonvoid intersection then the noisyfitness values and therefore also the individuals are incomparable,in symbols:resp..It remains to examine whether the set of minimal elements of such posets represents a reasonable and useful set of can-didate solutions.For this purpose definewith andwithIn general,and are random objects.But since it is as-sumed that each element is evaluated only once,one can hold the view that each element of has been evalu-ated already before the EA is run such that the set and the quantity are deterministic during the run of the EA.In this manner one obtains a unique partial order on and on for each run.The set of minimal elements is then given by(3)Needless to say,it is reasonable to postulate that the noisy image of an unperturbed optimal point is con-tained in the set of minimal elements.As shown below,this requirement is fulfilled.Theorem1For all with holds regard-less of the value of.Proof:First notice the equivalencewhich is easily deduced from equation(3).Since the support of random variable is the relation(4)holds with probability.For the same reason one obtains.Insertion in equation(4)leads to.The next result offers an assessment of the’solutions’con-tained in the set.Theorem2.Proof:Owing to equation(3)each element of is upper bounded by.Since the support of is one obtains.Putting all together yields the desired inequality.Notice that under this partial order the set of minimal ele-ments of a givenfinite population is determinable in linear time:Find the individual with the smallest perturbedfitness value in the population.This takes time.Each in-dividual withfitness moves to the set of minimal elements.Since this takes time the entire run time is .23.3The Base AlgorithmThe pseudo code given in Figure1is taken from Rudolph (2001).Notice that an individual of a population at generation gathers all quantities of interest,i.e.,.The partial ordering of the individuals is based on their noisyfitness values of course.The expressionin phase1encapsulates the operation of generating new individuals from the current population of size with at generation.initialize;setrepeat(*PHASE1*)(*PHASE2*)for each:if thenendifif for all thenendifendfor(*PHASE3*)if thenfill with elements from:1.2.3.untilendifuntil stopping criterion fulfilledFigure1:Pseudo code of the evolutionary algorithm with par-tially orderedfitness.3.4The Theoretical Property InheritedSince the base algorithms’properties are valid for arbitrary partially orderedfitness sets,any instantiation inherits the the-oretical properties from the general case(Rudolph2001). Theorem3Let the search space of the base algorithm in Figure1befinite and the partial order of thefitness set be as described in Section3.2.If every collection of offspring can be generated from an arbitrary collection of parents with some positive minimum probability,then the entire popula-tion will enter the set after afinite number of generations with probability and stays there forever.As we know from Theorems1and2the set of minimal elements contains the noisy version of the global mini-mum and each member of is at most away from the true minimum.In this sense,we may call also the set of-optimal solutions with.3.5The Virtue of Resampling RevisitedA-optimal solution may be sufficient or may not.For the latter case one should look for a method of decreasing this bound.Here we use the technique of resampling that is common practice in EAs with noisyfitness ually each point/individual is sampled several times and the thereby obtained noisyfitness values are averaged.This makes the es-timator of the truefitness value more and more reliable by re-ducing its variance by a factor ofwhere denotes the th smallest outcome of samples in total.Thus,after samples one knows for sure that thetrue value is somewhere in the interval given in equation(5).The uncertainty interval shrinks to for.The speed of narrowing can be deter-mined as follows:Let and. Then is the relative size of the uncertainty or incomparability interval after samples and the probability that it is then still larger than percent of its initial size is given byPP(6)then E.Thus,the closer should re-semble Gaussian noise the slower is the narrowing of the uncertainty or incomparability interval.4First Numerical Experiments4.1Instantiation of the Base AlgorithmThe search space is afinite subset of the-dimensional set of integers with box constraints.An individual is represented by a-tuple of integers(the chromosomes)and the bounds of the confidence interval that is needed for the comparison of the individuals according to equation(2).Mutations of the chromosomes obey a bilateral geometrical distribution on the integers(Rudolph1994)that is truncated at the box con-straints.If the self-adaptation of the mutation distribution is switched on then an additional parameter(chromosome)must be represented in the individual.The recombination of two chromosomes is realized by uniform crossover,the potential real-valued chromosomes for self-adaptation are averaged.4.2Realization of the Noise GeneratorThe noise used here is represented by a Beta random.A Beta random variable on with pa-rameter and probability density function as given in equation(6)can be generated via whereandwith uniformly distributed random numbers.4.3Preliminary ResultsThe search space is for the test functionFigure 2shows a typical run for a population size ofand a support bound for the uniformly dis-tributed noise ,whereas the support bound was in-creased to in Figure 3and in Figure 4.051015202530354045500500100015002000250030003500400045005000n u m b e r o f s u r v i v i n g p a r e n t sgenerations051015202530354045500500100015002000250030003500400045005000n u m b e r o f (3a )-o p t i m a l s o l u t i o n sgenerationsFigure 3:Number of surviving parents and -optimal so-lutions for noise bound .As can be seen in all three cases the number of survivingparents rapidly increases at about the maximum size as soon as the population enters the set of -optimal solutions.It appears plausible that this happens the earlier the larger is the noise bound ,but it must be noted that there is no statistical support for this conjecture at the moment.Nevertheless,we dare to use the number of surviving par-ents as an indicator for the event of entering the -optimal set.When this happens then the individuals cannot be com-pared due to the size of the noise interval.Therefore,the re-sampling of the fitness values should begin right now.Many rules for the indicator mechanism are possible.Here,we introduce a width parameter that is initiallyset to.If two individuals are incomparable and at least one of them has a confidence interval larger than ,then the individual with the largest interval is re-evaluated and its interval bounds are updated.This is repeated as long as the individuals are incomparable or both confidence intervals are smaller than .The decrease of by provided that.The EA is stopped as soon as and no parent has been replaced in the last selection process.Of course,these parameters are chosen arbitrarily for thenext experiment—other values may yields far better results.The identification of “good”parameter settings is not the goal here;rather,we like to gain first insights concerning the be-havior of this EA.51015202530354045500500100015002000250030003500400045005000n u m b e r o f s u r v i v i n g p a r e n t sgenerations051015202530354045500500100015002000250030003500400045005000n u m b e r o f (3a )-o p t i m a l s o l u t i o n sgenerationsFigure 4:Number of surviving parents and-optimal so-lutions for noise bound .For this purpose the noise bound is set to and the EA is run.Figure 5shows some characteristic quantities recorded during a typical run of the EA.At about generation 2000the population begins to enter the set of -optimal solutions.No re-evaluation of the in-dividuals was triggered until now.The number of surviving parents oscillates considerably while the width parameter is continuously decreased.This leads to a rapid increase of the number of re-evaluations along with a significant improve-ment of the solutions as can be seen from the number of par-ents below certain (normally unavailable)true fitness values.The number of re-evaluations,however,is much too large for practical use.This might be caused by an unlucky choice of the EA parameters.In the next experiment the width thresh-old was chosen a magnitude larger,namely,.Table 1summarizes the results obtained from 50independent runs.The number of re-evaluations is considerably smaller now,but the optimal true solution was never contained in the finalpopulation,in contrast to some other tests with.Needless to say,some parameter studies are necessary to find5mean std.dev.skew.Table1:Summary of results for50runs with.a useful parametrization of this EA.AcknowledgmentsThis work was supported by the Deutsche Forschungsgemein-schaft(DFG)as part of the Collaborative Research Center “Computational Intelligence”(SFB531). BibliographyB.C.Arnold,N.Balakrishnan,and H.N.Nagaraja(1992).AFirst Course in Order Statistics.New York:Wiley.H.-G.Beyer(2000).Evolutionary algorithms in noisy envi-ronments:Theoretical issues and guidelines for practice.Computer Methods in Applied Mechanics and Engineer-ing186(2-4),239–267.M.Evans,N.Hastings,and B.Peacock(1993).Statistical Distributions(2nd ed.).New York:Wiley.P.C.Fishburn(1985).Interval Orders and Interval Graphs:A Study of Partially Ordered Sets.New York:Wiley.J.Guddat,F.Guerra Vasquez,K.Tammer,and K.Wendler (1985).Multiobjective and Stochastic Optimization Based on Parametric Optimization.Berlin:Akademie-Verlag.G.Rudolph(1994).An evolutionary algorithm for inte-ger programming.In Y.Davidor,H.-P.Schwefel,and R.M¨a nner(Eds.),Parallel Problem Solving From Nature, 3,pp.139–148.Berlin and Heidelberg:Springer.G.Rudolph(1998).Evolutionary search for minimal ele-ments in partially orderedfinite sets.In V.W.Porto, N.Saravanan,D.Waagen,and A.E.Eiben(Eds.),Evolu-tionary Programming VII,Proceedings of the7th Annual Conference on Evolutionary Programming,pp.345–353.Berlin:Springer.G.Rudolph(2001).Evolutionary search under partially or-deredfitness sets.In Proceedings of the International Symposium on Information Science Innovations in En-gineering of Natural and Artificial Intelligent Systems (ENAIS2001).ICSC Academic Press.G.Rudolph and A.Agapie(2000).Convergence properties ofsome multi-objective evolutionary algorithms.In A.Za-lzala et al.(Eds.),Proceedings of the2000Congress on Evolutionary Computation(CEC2000),V ol.2,pp.1010–1016.Piscataway(NJ):IEEE Press.51015202530354045500500100015002000250030003500 numberofsurvivingparentsgenerations51015202530354045500500100015002000250030003500 numberofparentsbelowcertainvaluegenerations< 30000< 3000< 300< 30<3< 0.3< 0.030.0010.010.11101000500100015002000250030003500 decayofwidththresholdgenerations1101001000100001000001e+061e+071e+080500100015002000250030003500 cumulatednumberofre-evaluationsgenerationsFigure5:From top to bottom:Number of surviving parents, number of parents below certainfitness thresholds,decay of width threshold and cumulated number of re-evaluations.6。
- 1、下载文档前请自行甄别文档内容的完整性,平台不提供额外的编辑、内容补充、找答案等附加服务。
- 2、"仅部分预览"的文档,不可在线预览部分如存在完整性等问题,可反馈申请退款(可完整预览的文档不适用该条件!)。
- 3、如文档侵犯您的权益,请联系客服反馈,我们会尽快为您处理(人工客服工作时间:9:00-18:30)。
A Partial Join Approach for Mining Co-location Patterns∗Jin Soung Y oo Department of Computer Science andEngineeringUniversity of Minnesota200Union ST SE4-192Minneapolis,MN55414jyoo@Shashi Shekhar Department of Computer Science andEngineeringUniversity of Minnesota200Union ST SE4-192Minneapolis,MN55414shekhar@ABSTRACTSpatial co-location patterns represent the subsets of events whose instances are frequently located together in geographic space.We identified the computational bottleneck in the execution time of a current co-location mining algorithm.A large fraction of the join-based co-location miner algo-rithm is devoted to computing joins to identify instances of candidate co-location patterns.We propose a novel partial-join approach for mining co-location patterns efficiently.It transactionizes continuous spatial data while keeping track of the spatial information not modeled by transactions.It uses a transaction-based Apriori algorithm as a building block and adopts the instance join method for residual in-stances not identified in transactions.We show that the algorithm is correct and complete infinding all co-location rules which have prevalence and conditional probability above the given thresholds.An experimental evaluation using syn-thetic datasets and a real dataset shows that our algorithm is computationally more efficient than the join-based algo-rithm.Categories and Subject DescriptorsH.2.8[Database Applications]:Data mining,GIS and Spatial databasesGeneral TermsAlgorithms∗This work was partially supported by Digital Technology Center of University of Minnesota,NASA grant No.NCC21231and the Army High Performance Computing Research Center under the auspices of the Department of the Army, Army Research Laboratory cooperative agreement number DAAD19-01-2-0014,the content of which does not necessar-ily reflect the position or the policy of the government,and no official endorsement should be inferredPermission to make digital or hard copies of all or part of this work for personal or classroom use is granted without fee provided that copies are not made or distributed for profit or commercial advantage and that copies bear this notice and the full citation on thefirst page.To copy otherwise,to republish,to post on servers or to redistribute to lists,requires prior specific permission and/or a fee.GIS’04,November12–13,2004,Washington,DC,USA.Copyright2004ACM1-58113-979-9/04/0011...$5.00.KeywordsSpatial data mining,Association rule,Co-location,Join1.INTRODUCTIONA co-location represents a subset of spatial boolean events whose instances are often located in a neighborhood.Boolean spatial events describe the presence or absence of geographic object types at different locations in a two dimensional or three dimensional metric space,e.g.,surface of the Earth. Examples of boolean spatial events include business types, mobile service request,disease,crime,climate,plant species, etc.Spatial co-location patterns may yield important in-sights for many applications.For example,a mobile service provider may be interested in service patterns frequently re-quested in a close location,e.g.,‘today sales’and‘nearby stores’.The frequent neighboring request sets may be used for providing attractive location-sensitive advertisements, promotion,etc.Figure1shows the locations of different types of business in a downtown area of Minneapolis,Min-nesota.We can notice two prevalent co-location patterns, i.e.,{‘auto dealers’,‘auto repair shops’}and{‘department stores’,‘gift stores’}.Other application domains for co-locations are Earth science,environmental management,gov-ernment services,public health,public safety,transporta-tion,tourism,etc.Figure1:An example of co-location pat-terns found among different types of businesses in a city.A=Auto dealers,R=auto Repair shops,D=Department stores,G=Gift stores and H=Hotels.{A, B, C}’s instances={(A.2, B.4, C.2),(A.3, B.3, C.1)}(a)An example dataset{A, B, C}’s instances={(A.2, B.4, C.2)}(b){A, B, C}’s instances={(A.2, B.4, C.2)}or {(A.2, B.4, C.2) , (A.3, B.3, C.1)}(c)B.4C.2A BA.1B.1A.3B.3A.4B.3A.1 C.1A.3 C.3A.3 C.1B CA CB.3C.1A.3B.3C.1A.2B.4C.2A B C={(A.2, B.4, C.2) , (A.3, B.3, C.1)}A.2 C.2{A, B, C}’s instancesA.2B.4(d)Figure2:Examples to illustrate different approaches to discover co-location patterns(b)An explicit transac-tionization of a spatial dataset can split instances of co-locations.(c)The non-overlapping grouping method can generate sets of different instances.(d)The instance join method generates complete instances but computation is expensive.Co-location rule discovery is a process to identify co-location patterns from an instance dataset of spatial boolean events. It is not trivial to adopt association rule mining algorithms[1, 8,13,18]to mine co-location patterns since instances of spa-tial events are embedded in a continuous space and share a variety of spatial relationships.Reusing association rule al-gorithms may require transactionizing spatial datasets,which is challenging due to the risk of transaction boundaries split-ting co-location pattern instances across distinct transac-tions.Figure2(a)shows an example spatial dataset with three spatial events,A,B,and C.Each instance is repre-sented by its event type and unique instance id,e.g.,A.1. Solid lines show neighbor relationships over event instances. For example,{A.2,B.4,C.2}and{A.3,B.3,C.1}are the instances of co-location{A,B,C}since their event instances are neighbors of each other.Figure2(b)shows the problem of explicit transactionization.Rectangular grids are used to produce transactions over the spatial dataset.As can be seen by the solid line circle,the only identified instance of co-location{A,B,C}is{A.2,B.4,C.2}.The instance{A.3, B.3,C.1}is missed due to the split caused by the transaction boundaries.Related Work:In previous work on co-location pattern discovery,a few approaches have been developed to iden-tify instances of candidate co-location patterns.One ap-proach[12]groups neighboring instances arbitrarily with a non-overlapping instance grouping constraint.This disjoint grouping method may yield different instance sets by the order of grouping.For example,Figure2(c)illustrates dif-ferent instance sets of co-location{A,B,C}by the order of grouping instances of size2co-location{A,B}.If an in-stance{A.4,B.3}isfirst grouped,the instance{A.3,B.3} is not identified since B.3already belongs to instance{A.4, B.3}even if it is a neighborhood instance.Consequently, the instance{A.3,B.3,C.1}of co-location{A,B,C}is also not found.Another approach[15]generates instances of candidate co-locations without any missing by using an instance join method.For example,in Figure2(d),the instances of co-location{A,B}and the instances of co-location{A,C}are joined and their neighbor relations are checked for gener-ating instances of co-location{A,B,C}.{A.2,B.4,C.2} and{A.3,B.3,C.1}are correctly generated.The join-based algorithm may be useful in analyzing datasets of sparse in-stances.However,scaling the algorithm to substantially large dense spatial datasets is challenging due to the in-creasing number of co-location patterns and their instances. Other co-location mining work[17]presents a framework for extended spatial objects,e.g.,polygons and line strings.It also uses an instance join method to identify nearby spatial objects.This paper proposes a novel approach for efficient co-location pattern mining.We make the following contribu-tions.Our Contributions:First,we identified the computa-tional bottleneck in the execution time of the join-based co-location mining algorithm[15].A large fraction of the al-gorithm is devoted to computing joins to identify instances of candidate co-location patterns.Second,we propose a novel partial-join approach for mining co-location patterns efficiently.It transactionizes continuous spatial data while keeping track of the spatial information not modeled by transactions.This approach is based on an important ob-servation that only event instances having at least one cut neighbor relation are related to co-location instances split over transactions.Third,we present an efficient co-location mining algorithm to concretize the partial-join approach.It uses a transaction-based Apriori algorithm[1]as a building block and adopts the instance join method[15]of the join-based co-location mining algorithm for generating residual co-location instances not identified by transactions.Fourth, we prove that the partial join algorithm is correct and com-plete infinding all co-location rules with prevalence and con-ditional probability above the given thresholds.Fifth,we provide an algebraic cost model to characterize the dom-inance zone of the performance between our partial-join algorithm and the join-based algorithm.Finally,we con-ducted experiments using a real dataset as well as syntheticdatasets.The experimental evaluation shows that our algo-rithm is computationally more efficient than the full join-based mining algorithm.The remainder of the paper is organized as follows.Sec-tion2presents an overview of basic concepts of co-location pattern mining.In Section3,we present the partial join ap-proach for efficient co-location mining.Section4describes the partial join co-location mining algorithm.The proofs of correctness and completeness of the algorithm,and an alge-braic cost model are given in Section5.Section6presents experimental evaluations.We give the conclusion and dis-cuss future work in Section7.2.CO-LOCATION PATTERN MINING:BASIC CONCEPTSThis section describes the basic concepts for mining co-location patterns.Given a set of boolean spatial events E={e1,...,e k}, a set S of their instances{i1,...,i n},and a reflexive and symmetric neighbor relation R over S,a co-location C is a subset of boolean spatial events,i.e.,C⊆E whose instances I⊆S form a clique[3]using neighbor relation R.For simplicity,we use a metric-based neighbor relation R,i.e., neighbor(i1,i2)between event instances i1and i2defined by Euclidean distance(i1,i2)≤a user-specified threshold is used as a neighbor relation R.A co-location rule is of the form:C1→C2(p,cp),where C1and C2are disjoint co-locations,p is a value representing the prevalence measure,and cp is the conditional probabil-ity.A neighborhood instance I of a co-location C is a row instance(simply,instance)of C if I contains instances of all events in C and no proper subset of I does so.For example, in Figure2(d),{A.1,B.1}is a row instance of co-location {A,B}.{A.3,C.1,C.3}is a neighborhood in Figure2(a) but it is not a row instance of co-location{A,C}because its subset{A.3,C.1}contains instances of all events in{A, C}.The table instance of a co-location C is the collection of all row instances of C.For example,the table instance of{B,C}in Figure2(d)has two row instances,{B.3,C.1} and{B.4,C.2}.The conditional probability,P r(C1|C2),of a co-location rule C1→C2is the probability offinding an instance of C2 in the neighborhood of an instance of C1.Formally,it isestimated as|πC1(table instance of C1∪C2)|1,whereπis a pro-jection operation with duplication elimination.The participation index,P i(C)is used as a co-location prevalence measure.The participation index of a co-locationC={e1,...,e k}is defined as min ei∈C {P r(C,e i)},whereP r(C,e i)is the participation ratio for event type e i in a co-location C.P r(C,e i)is the fraction of instances of e i which participate in any instance of co-location C, |πei(table instance of C)|i,whereπis a projection operation with duplication elimination.For example,in Figure2(a),the total number of instances of event type A is4and the to-tal number of instances of event type C is3.From Fig-ure2(d),the participation index of co-location c={A,C}is min{P r(c,A),P r(c,C)}=3/4because P r(c,A)is3/4and P r(c,C)is3/3.A high participation index value indicates that the spatial events in a co-location pattern likely show up together.Lemma 1.The participation ratio and the participation index are monotonically non increasing with the size of the co-location increasing.Proof.Please refer to[15]for the proof.Lemma1ensures that the participation index can be used to effectively prune the search space of co-location pattern mining.3.PARTIAL JOIN APPROACH FORCO-LOCATION PATTERN MININGThis section defines our partial join approach for efficient co-location pattern mining.3.1Problem DefinitionWe formalize the co-location mining problem as follows: Given:1)A set of k spatial event types E={e1,...,e k}and a set of their instances S={i1,...,i n},each i∈S is a vector< instance id,spatial event type,location>,where location∈a spatial framework2)A symmetric and reflexive neighbor relation R over loca-tions3)A minimal prevalence threshold(min prev)and a mini-mal conditional probability threshold(min cond prob) Find:Find a correct and complete set of co-location rules with participation index>min prev and conditional probability >min cond prob.Objective:Minimize computation cost.Constraints:1)R is a distance metric based neighbor relation.2)Ignore edge effects in R.3)Correct and complete infinding all co-location rules sat-isfying given thresholds.4)Spatial dataset is a point dataset.3.2Partial Join ApproachThe basic idea of the partial join approach is to reduce the number of instance joins for identifying instances of candi-date co-locations by transactionizing a spatial dataset un-der a neighbor relationship and tracing only residual neigh-borhood instances cut apart via the transactions.The key component of our approach is how we identify instances of co-locations split across explicit transactions.It is based on an observation that only event instances having at least one cut neighbor relationship are related to the neighborhood instances split over transactions.To formalize this idea,we provide a set of definitions of key terms related to the partial join approach.Definition 1.A neighborhood transaction(simply, transaction)is a set of instances T⊆S that forms a clique using a neighbor relation R.A spatial dataset S is parti-tioned to a set of disjoint transactions{T1,...,T n}where T i∩T j=∅,i=j and∪(T1,...,T n)=S.We assume a spatial dataset S can be partitioned to a set of distinct transactions,i.e.,each event instance i∈S belongs to one transaction.For example,Figure4shows a set of transactions on the same example spatial dataset of Figure2(a).The dashed circle represents a neighborhoodregion centered at an arbitrary location on a spatial frame-work.The instances within the dashed circle are neighbors of each other and thus forms a transaction.For example,B.2 and B.5form a transaction.A spatial dataset can be differ-ently transactionized according to the partitioning method used.Thus the transactions generated using rectangular grids in Figure2(b)are a little different from the trans-actions illustrated in Figure4.For example,in Figure4, {A.3,C.1,C.3}forms a single transaction.By contrast,in Figure2(b),it is divided into two transactions,{A.3,C.3} and{C.1}.We will examine the effect of different transac-tionization methods in future work.Definition 2.A row instance I of a co-location C is an intraX row instance(simply,intraX instance)of C if all instances i∈I belong to a common transaction T.The intraX table instance of C is the collection of all intraX row instances of C.For example,in Figure4,{A.3,C.1}is an intraX instance of co-location{A,C}but{A.1,C.1}is not since its event instances A.1and C.1are members of different transactions. The intraX table instance of{A,C}consists of{A.3,C.1}, {A.3,C.3}and{A.2,C.2}.Definition 3.A neighbor relation r∈R between two event instances,i1,i2∈S,i1=i2is called a cut neighbor relation if i1and i2are neighbors of each other but belong to distinct transactions.Figure4presents cut neighbor relations as dotted lines. {A.1,C.1},{A.3,B.3}and{B.3,C.1}has cut neighbor relations.Definition 4.A row instance I of a co-location C is an interX row instance(simply,interX instance)of C if all instances i∈I have at least one cut neighbor relation.The interX table instance of C is the collection of all interX row instances of C.For example,in Figure4,{A.3,B.3}is an interX instance of co-location{A,B}because A.3has a cut neighbor relation with B.3and B.3also has cut neighbor relations with A.3 and with C.1.Note{A.3,C.1}is an interX instance as well as an intraX instance of{A,C}.InterX table instance of {A,C}has two interX instances{A.1,C.1}and{A.3,C.1}.Figure3:The cases of possible instances of size3 and of size4co-locations over transactionsFigure3illustrates the possible instances of size3co-location and of size4co-location located over neighborhood transactions.Black dots signify event instances,circles are transactions,and lines show neighbor relations between twoFigure4:An illustration of the partial join co-location mining algorithmevent instances.Especially,dotted lines signify cut neighbor relations.There are two types of instances of co-locations. One is all event instances of a co-location instance belong to a single transaction.The other is the event instances are distributed across two or more transactions.The for-mer is the case of an intraX instance and the latter is an interX instance.We can notify all event instances of in-terX instances are related to at least one cut neighbor rela-tion(dotted lines).Lemma 2.For a co-location C,the table instance of C is the union of intraX table instance of C and interX table instance of C.Proof.The table instance of a co-location C is the col-lection of all(row)instances of C.First,we will show any instance,I={i1,...,i n}of C is an intraX instance of C or an interX instances of C.Since I forms a clique using a neighbor relation,all event instances of I can be included in a single neighborhood transaction according to definition1.I becomes an intraX instance.By contrast,if all event in-stances of I are not in a single transaction,each member should have at least one cut neighborhood relation with the other members in different transactions due to their clique relation.Thus,I becomes an interX instance.Second,all instances of intraX table instance and interX table instance of C are row instances whose event instances form a clique according to definition2and definition4.4.PARTIAL JOIN CO-LOCATION MININGALGORITHMThis section describes the partial join co-location min-ing algorithm.A transaction-based Apriori algorithm[1]is used as a building block to identify all intraX instances of co-locations.InterX instances are generated using general-ized apriori gen function[15]of the join-based co-location mining algorithm.This approach is expected to provide a framework for efficient co-location mining since all instancesin the transaction are neighbors of each other and no spa-tial operation and combinatorial operation,i.e.,join,is re-quired tofind instances of candidate co-locations within a transaction,i.e.,intraX instances.The computation cost of instance join operations for generating only interX instances not identified in the transactions is relatively cheaper than one forfinding all instances of co-locations.The partial-join mining algorithm for co-location patterns is described as follows.InputsE:a set of boolean spatial event typesS:a set of instances<event type,event instance id,location> R:a spatial neighbor relationmin prev:prevalence value thresholdmin cond prob:conditional probability threshold OutputA set of all prevalent co-location rules withparticipation index greater than min prevand conditional probability greater thanmin cond probVariablesk:co-location sizeT:a set of transactionsC k:a set of size k candidate co-locationsP k:a set of size k prevalent co-locationsR k:a set of size k co-location rulesIntraX k:intraX table instances of C kInterX k:interX table instances of C k,P kMethod1)(T,InterX2)=transactionize(S,R);2)k=1;C1=E;P1=E;3)while(not empty P k)do{4)C k+1=gen candidate co-location(P k);5)for all transaction t∈T6)IntraX k+1=gather intraX instances(C k+1,t);7)if k≥28)InterX k+1=gen interX intances(C k+1,InterX k,R);9)P k+1=select prevalent co-location10)(C k+1,IntraX k+1InterX k+1,min prev);11)R k+1=gen co-location rule(P k+1,min cond prob);12)k=k+1;13)}14)return(R2,...,R k+1);Algorithm1:Partial join co-location algorithm Transactionization of a spatial dataset:Given a spa-tial dataset and a neighbor relation,the spatial dataset is partitioned for generating neighborhood transactions.There are several partitioning methods adopted for neighborhood transactions,e.g.,grids[14],maximal cliques[3],max-clique agglomerative clustering[20],min cut partitioning[6]etc. The ideal case is a method to generate a set of maximal cliques with minimizing the number of edges cut by parti-tions.In the case of a simple grid partitioning,rectangular grids of a proximity neighborhood size d×d,where d is a neighbor distance metric,are posed on a spatial frame-work,and event instances in each cell are gathered for a transaction.Cut neighbor relations can be detected by ex-amining all pairs(i1,i2)of instances in neighboring cells, i.e.,(i1,i2)∈R and i1.trans no=i2.trans no,where R is a neighbor relation.It can be implemented using geo-metric approaches, e.g.,plane sweep[2],space partition-ing[9],tree matching[10].Size2interX instances are gen-erated from all pairs(i1,i2)of instances having cut neigh-bor relations in each transaction,i.e.,i1∈B,i2∈B and i1.trans no=i2.trans no,where B is a set of event in-stances having cut neighbor relations,as well as cut neigh-borhood instances.Generation of candidate co-locations:We use the apriori gen[1]for generating candidate co-location sets. Size k+1candidate co-locations are generated from size k prevalent co-locations.The anti-monotonic property of the participation index makes event level pruning feasible. Scanning transactions and gathering intraX instances :In each iteration step,the transactions are scanned and the intraX instances of candidate co-locations are enumer-ated.This step is similar to the apriori algorithm.However, notice that the transactions of a spatial event dataset differ from the transactions of a market basket dataset.The tradi-tional market basket data transaction has only boolean item types,i.e.,an item is present in a transaction or not.By contrast,each item of our neighborhood transaction consists of an event type and its instance id as described in Figure4. One event type can have several instances in a transaction. To reuse an efficient trie data structure[4,7]in determining instances of candidate co-locations in a transaction,we con-vert several items of same event type with different instance ids to one event type item having a bitmap structure[5]in which corresponding instance id bits are set.The converted transactions are searched for gathering intraX instances of co-locations.Figure4shows a conceptual set of intraX table instances.Actually,all instances are enumulated in the trie structure of itemsets using bitmaps.Generation of interX table instances:The interX table instance of C k+1,k≥2are generated from interX table in-stance of C k using the generalized apriori gen function[15]. The SQL-like syntax is described below.forall co-location c k+1∈C k+1insert into c k+1.interX table instanceselect p.instance1,p.instance2,...,p.instance k ,q.instance kfrom c k.interX table instance1p,c k.interX table instance2qwhere(p.instance1,...,p.instance k−1)=(q.instance1,...,q.instance k−1)and(p.instance k,q.instance k)∈R;end;In Figure4,an interX table instance of{A,B}having {A.3,B.3}and an interX table instance of{A,C}having {A.1,C.1}and{A.3,C.1}are joined to produce interX ta-ble instance of{A,B,C}.Selection of Prevalent Co-locations:The participation index of co-location C k+1is calculated from the union of in-traX table instance(C k+1)and interX table instance(C k+1). Candidate co-locations are pruned using a given prevalence threshold,min prev.In Figure4,co-location{B,C}has two instances,i.e.,one is an intraX instance,{B.4,C.2}and the other is an interX instance{B.3,C.1}.The participa-tion index of co-location{B,C}is min{2/5,2/3}=2/5.If min prev is given as1/2,the candidate co-location{B, C}is pruned because its prevalence measure is less than1/2.Generation of Co-location Rules:This step generates all co-location rules with high conditional probability above a given min cond prob.5.ANALYSIS OF THE PARTIAL JOINCO-LOCATION MINING ALGORITHM In this section,we analyze the partial join co-location min-ing algorithm for completeness,correctness and computa-tional pleteness implies that no co-location rule satisfying given prevalence and conditional probability thresholds is missed.Correctness means that the participa-tion index values and conditional probability of generated co-location rules meet the user specified threshold.5.1Completeness and CorrectnessLemma 3.The partial join co-location mining algorithm is correct.Proof.The partial join co-location mining algorithm is correct if co-location patterns produced by algorithm1meets the thresholds of prevalence value and conditional probabil-ity.First,we will show that intraX instances and interX instances are correct in the neighbor relation.Step1in al-gorithm1generates neighborhood transactions according to definition1.Thus the intraX instances gathered in step6 are correct in the neighbor relation.The interX instances generated in step8are proved by the correctness of gener-alized apriori gen algorithm[15].That is,all instances of a generated interX instance are neighbor of each other.Sec-ond,step9ensures that only prevalent co-location sets are selected.Thus step11returns co-location rules above given thresholds correctly.Lemma 4.The partial join co-location mining algorithm is complete.Proof.We prove if a co-location is prevalent,it is found by algorithm1.First,the monotonicity of the participation index in lemma1proves the completeness of the event level pruning of candidate co-locations using apriori gen in step 4.Second,we will show that the intraX table instances and the interX table instances generated from algorithm1are complete,which will imply that all instances of co-locations are complete according to lemma2.All intraX table in-stances are completely found by the apriori algorithm in step6.Size2interX table instances generated from step 1are a superset of all neighboring instances necessary to generate size k+1,k≥2interX instances.In step8,the completeness of the instance join method to generate interX instances is the same as that of generalized apriori gen[15]. In step11,enumeration of the subsets of each of the preva-lence co-locations ensures that no spatial co-location rules satisfying given prevalence and conditional probabilities are missed.5.2Computational Complexity AnalysisThis section compares the computational cost of the join-based co-location mining algorithm and the partial join al-gorithm.Let T jb(k+1)and T pj(k+1)represent the costs of iteration k of the join-based algorithm and the partial join algorithm respectively.T jb(k+1)=T gen candi(P k)+T gen inst(table insts of P k)+T prune(C k+1)≈T gen inst(table insts of P k)T pj(k+1)=T gen candi(P k)+T gath intraX inst(transactions) +T gen interX inst(interX table insts of P k)+T prune(C k+1)≈T gen interX inst(interX table insts of P k)In the above equations,T gen candi(P k)represents the costof generating size k+1candidate co-location with the preva-lent size k co-locations.T gen inst(table insts of P k)repre-sents the cost of generating table instances of size k+1 candidate co-locations with size k table instances.T gath intraX inst(transactions)is the cost of scanning trans-actions and gathering the instances of the size k+1candi-date co-locations.T gen interX inst(interX table inst of P k)is the cost of generating interX table instances of the sizek+1candidate co-locations with size k interX table in-stances.T prune(C k+1)represents the cost for pruning non prevalent size k+1co-locations.The bulk of time is consumed in generating instances. We assume that the cost of gathering intraX instances from transactions is relatively cheaper than instance join cost, and that the other factors,T gen candi(P k)and T prune(C k+1) are illegible.Thus the computational ratio of the partial join algorithm over the join-based algorithm can be simplified asT pj(k+1)jb≈T gen interX inst(interX table insts of P k)gen inst insts kThe computational ratio is affected by the size of interX table instances and the size of table instances of co-locationP k.The dominance factors affecting the number of interX instances and the number of total instances can be the num-ber of cut neighbor relations and the data density of the neighborhood area.When the number of cut neighbor rela-tions isfixed and the data density in a neighborhood area grows,the size of table instances increases rapidly and the cost to generate the table instances is much greater than the cost to generate interX table instances.By contrast,as the number of cut neighbor relations increases,the size of interX table instances increases.Thus the average cost to gener-ate interX table instances grows.When all instances have cut neighbor relations,they are involved in interX table in-stances thus the cost to generate the interX table instancesis similar to the cost to generate table instances in the join-based algorithm.In our experiments,as described in the next section,we use the data density in neighborhood area and the ratio of cut neighbor relations as key parameters to evaluate the algorithms.We can expect that the partial join approach is likely more efficient than the join-based method when the locations of spatial events are clustered in neigh-borhood areas and the number of cut neighbor relations is smaller.6.EXPERIMENTAL EV ALUATIONWe evaluated the performance of the partial join algo-rithm with the join-based approach using synthetic and real datasets.In Subsection6.1,we describe an overall exper-imental design and a synthetic data generator.In Subsec-tion6.2,we evaluate the computational efficiency gained。