Small-World Networks
脑科学专业术语中英对照

小世界网络( small-world network)无标度网络( scale-free network)随机网络( random network)规则网络( regular network)无向网络( undirected network)加权网络( weighted network)图论( Graph theory)邻接矩阵( adjacency matrix)结构性脑网络( structural brain networks 或anatomical brain networks)功能性脑网络( functional brain networks)因效性脑网络( effective brain networks)感兴趣脑区( region of interest,ROI)血氧水平依赖( BOLD,blood oxygenation level depended)体素( voxel)自发低频震荡( spontaneous low-frequency fluctuations,LFF) 默认功能网络( default mode network,DMN)大范围皮层网络( Large-scale cortical network)效应连接(effective connectivity)网络分析工具箱(Graph Analysis Toolbox,GAT)自动解剖模板(automatic anatomical template,AAL)脑电图(electroencephalogram, EEG)脑磁图(magnetoencephalogram, MEG)功能磁共振成像(Functional magnetic resonance imaging, fMRI)弥散张量成像(Diffusion Tensor Imaging, DTI)弥散谱成像( diffusion spectrum imaging ,DSI)细胞结构量化映射( quantitative cytoarchitecture mapping)正电子发射断层扫描(PET, positron emisson tomography)精神疾病:阿尔茨海默症( Alzheimer’ s disease,AD)癫痫( epilepsy)精神分裂症( Schizophrenia)抑郁症( major depression)单侧注意缺失( Unilateral Neglect)轻度认知障碍(mild cognitive impairment, MCI)正常对照组(normal control, NC)MMSE (Mini-mental state examination) 简易精神状态检查量表CDR (Clinic dementia rating) 临床痴呆量表边( link,edge)节点(vertex 或node)节点度(degree)区域核心节点(provincial hub)度分布(degree distribution)节点强度( node strength)最短路径长度(shortest path length)特征路径长度( characteristic path length) 聚类系数( clustering coefficient)中心度(centrality)度中心度(degree centrality)介数中心度( betweenness centrality)连接中枢点( connector hub)局部效率(local efficiency)全局效率( global efficiency)相位同步( phase synchronization)连接密度(connection density/cost)方法:互相关分析( cross-correlation analysis) 因果关系分析( Causality analysis)直接传递函数分析( Directed Transfer Function,DTF)部分定向相干分析( Partial Directed Coherence,PDC)多变量自回归建模( multivariate autoregressive model,MV AR) 独立成分分析( independent component analysis,ICA)同步似然性(synchronization likelihood, SL)结构方程建模(structural equation modeling, SEM)动态因果建模(dynamic causal modeling, DCM)心理生理交互作用模型(Psychophysiological interaction model)非度量多维定标(non-metric multidimensional scaling)体素形态学(voxel-based morphometry, VBM)统计参数映射(statistical parametric mapping,SPM)皮尔逊相关系数(Pearson correlation)偏相关系数(Partial correlation)DTI指标:MD (Mean diffusivity) 平均扩散率ADC (Apparent diffusion coefficient) 表观弥散系数FA (Fractional anisotropy) 部分各向异性DCavg (Average diffusion coefficient) 平均弥散系数RA (Relative anisotropy) 相对各项异性VR (V olume ratio) 体积比AI (Anisotrop index) 各项异性指数TBSS (Tract-based Spatial Statistics) 基于纤维追踪束体素的空间统计DWI (Diffusion Weight Imaging) 弥散加权成像。
小世界网络综述
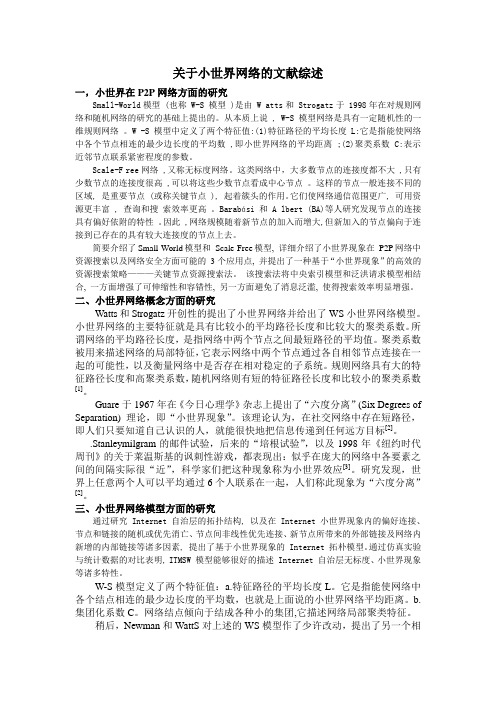
关于小世界网络的文献综述一,小世界在P2P网络方面的研究Small-World模型 (也称 W-S 模型 )是由 W atts和 Strogatz于 1998年在对规则网络和随机网络的研究的基础上提出的。
从本质上说 , W-S模型网络是具有一定随机性的一维规则网络。
W -S模型中定义了两个特征值:(1)特征路径的平均长度 L:它是指能使网络中各个节点相连的最少边长度的平均数 ,即小世界网络的平均距离 ;(2)聚类系数 C:表示近邻节点联系紧密程度的参数。
Scale-F ree网络 ,又称无标度网络。
这类网络中,大多数节点的连接度都不大 ,只有少数节点的连接度很高 ,可以将这些少数节点看成中心节点。
这样的节点一般连接不同的区域, 是重要节点 (或称关键节点 ), 起着簇头的作用。
它们使网络通信范围更广, 可用资源更丰富 , 查询和搜索效率更高。
Barabási和 A lbert (BA)等人研究发现节点的连接具有偏好依附的特性。
因此 ,网络规模随着新节点的加入而增大,但新加入的节点偏向于连接到已存在的具有较大连接度的节点上去。
简要介绍了Small-World模型和Scale-Free模型, 详细介绍了小世界现象在P2P网络中资源搜索以及网络安全方面可能的3个应用点, 并提出了一种基于“小世界现象”的高效的资源搜索策略———关键节点资源搜索法。
该搜索法将中央索引模型和泛洪请求模型相结合, 一方面增强了可伸缩性和容错性, 另一方面避免了消息泛滥, 使得搜索效率明显增强。
二、小世界网络概念方面的研究Watts和Strogatz开创性的提出了小世界网络并给出了WS小世界网络模型。
小世界网络的主要特征就是具有比较小的平均路径长度和比较大的聚类系数。
所谓网络的平均路径长度,是指网络中两个节点之间最短路径的平均值。
聚类系数被用来描述网络的局部特征,它表示网络中两个节点通过各自相邻节点连接在一起的可能性,以及衡量网络中是否存在相对稳定的子系统。
Collective dynamics of 'small-world' networks
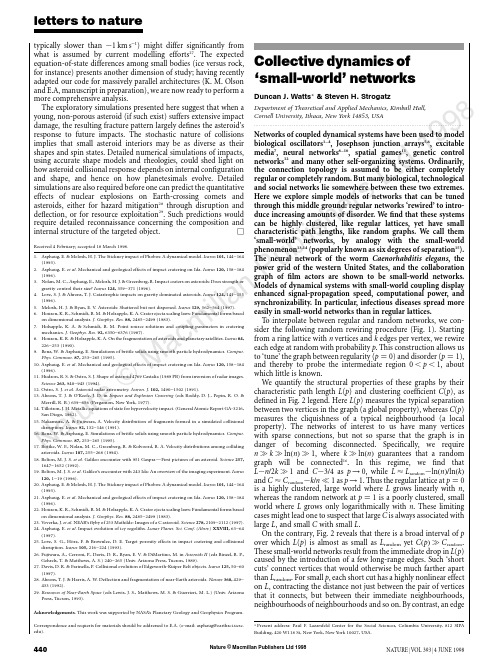
Nature © Macmillan Publishers Ltd 19988typically slower than ϳ1km s −1)might differ significantly from what is assumed by current modelling efforts 27.The expected equation-of-state differences among small bodies (ice versus rock,for instance)presents another dimension of study;having recently adapted our code for massively parallel architectures (K.M.Olson and E.A,manuscript in preparation),we are now ready to perform a more comprehensive analysis.The exploratory simulations presented here suggest that when a young,non-porous asteroid (if such exist)suffers extensive impact damage,the resulting fracture pattern largely defines the asteroid’s response to future impacts.The stochastic nature of collisions implies that small asteroid interiors may be as diverse as their shapes and spin states.Detailed numerical simulations of impacts,using accurate shape models and rheologies,could shed light on how asteroid collisional response depends on internal configuration and shape,and hence on how planetesimals evolve.Detailed simulations are also required before one can predict the quantitative effects of nuclear explosions on Earth-crossing comets and asteroids,either for hazard mitigation 28through disruption and deflection,or for resource exploitation 29.Such predictions would require detailed reconnaissance concerning the composition andinternal structure of the targeted object.ⅪReceived 4February;accepted 18March 1998.1.Asphaug,E.&Melosh,H.J.The Stickney impact of Phobos:A dynamical model.Icarus 101,144–164(1993).2.Asphaug,E.et al .Mechanical and geological effects of impact cratering on Ida.Icarus 120,158–184(1996).3.Nolan,M.C.,Asphaug,E.,Melosh,H.J.&Greenberg,R.Impact craters on asteroids:Does strength orgravity control their size?Icarus 124,359–371(1996).4.Love,S.J.&Ahrens,T.J.Catastrophic impacts on gravity dominated asteroids.Icarus 124,141–155(1996).5.Melosh,H.J.&Ryan,E.V.Asteroids:Shattered but not dispersed.Icarus 129,562–564(1997).6.Housen,K.R.,Schmidt,R.M.&Holsapple,K.A.Crater ejecta scaling laws:Fundamental forms basedon dimensional analysis.J.Geophys.Res.88,2485–2499(1983).7.Holsapple,K.A.&Schmidt,R.M.Point source solutions and coupling parameters in crateringmechanics.J.Geophys.Res.92,6350–6376(1987).8.Housen,K.R.&Holsapple,K.A.On the fragmentation of asteroids and planetary satellites.Icarus 84,226–253(1990).9.Benz,W.&Asphaug,E.Simulations of brittle solids using smooth particle put.mun.87,253–265(1995).10.Asphaug,E.et al .Mechanical and geological effects of impact cratering on Ida.Icarus 120,158–184(1996).11.Hudson,R.S.&Ostro,S.J.Shape of asteroid 4769Castalia (1989PB)from inversion of radar images.Science 263,940–943(1994).12.Ostro,S.J.et al .Asteroid radar astrometry.Astron.J.102,1490–1502(1991).13.Ahrens,T.J.&O’Keefe,J.D.in Impact and Explosion Cratering (eds Roddy,D.J.,Pepin,R.O.&Merrill,R.B.)639–656(Pergamon,New York,1977).14.Tillotson,J.H.Metallic equations of state for hypervelocity impact.(General Atomic Report GA-3216,San Diego,1962).15.Nakamura,A.&Fujiwara,A.Velocity distribution of fragments formed in a simulated collisionaldisruption.Icarus 92,132–146(1991).16.Benz,W.&Asphaug,E.Simulations of brittle solids using smooth particle put.mun.87,253–265(1995).17.Bottke,W.F.,Nolan,M.C.,Greenberg,R.&Kolvoord,R.A.Velocity distributions among collidingasteroids.Icarus 107,255–268(1994).18.Belton,M.J.S.et al .Galileo encounter with 951Gaspra—First pictures of an asteroid.Science 257,1647–1652(1992).19.Belton,M.J.S.et al .Galileo’s encounter with 243Ida:An overview of the imaging experiment.Icarus120,1–19(1996).20.Asphaug,E.&Melosh,H.J.The Stickney impact of Phobos:A dynamical model.Icarus 101,144–164(1993).21.Asphaug,E.et al .Mechanical and geological effects of impact cratering on Ida.Icarus 120,158–184(1996).22.Housen,K.R.,Schmidt,R.M.&Holsapple,K.A.Crater ejecta scaling laws:Fundamental forms basedon dimensional analysis.J.Geophys.Res.88,2485–2499(1983).23.Veverka,J.et al .NEAR’s flyby of 253Mathilde:Images of a C asteroid.Science 278,2109–2112(1997).24.Asphaug,E.et al .Impact evolution of icy regoliths.Lunar Planet.Sci.Conf.(Abstr.)XXVIII,63–64(1997).25.Love,S.G.,Ho¨rz,F.&Brownlee,D.E.Target porosity effects in impact cratering and collisional disruption.Icarus 105,216–224(1993).26.Fujiwara,A.,Cerroni,P .,Davis,D.R.,Ryan,E.V.&DiMartino,M.in Asteroids II (eds Binzel,R.P .,Gehrels,T.&Matthews,A.S.)240–265(Univ.Arizona Press,Tucson,1989).27.Davis,D.R.&Farinella,P.Collisional evolution of Edgeworth-Kuiper Belt objects.Icarus 125,50–60(1997).28.Ahrens,T.J.&Harris,A.W.Deflection and fragmentation of near-Earth asteroids.Nature 360,429–433(1992).29.Resources of Near-Earth Space (eds Lewis,J.S.,Matthews,M.S.&Guerrieri,M.L.)(Univ.ArizonaPress,Tucson,1993).Acknowledgements.This work was supported by NASA’s Planetary Geology and Geophysics Program.Correspondence and requests for materials should be addressed to E.A.(e-mail:asphaug@).letters to nature440NATURE |VOL 393|4JUNE 1998Collective dynamics of ‘small-world’networksDuncan J.Watts *&Steven H.StrogatzDepartment of Theoretical and Applied Mechanics,Kimball Hall,Cornell University,Ithaca,New York 14853,USA.........................................................................................................................Networks of coupled dynamical systems have been used to model biological oscillators 1–4,Josephson junction arrays 5,6,excitable media 7,neural networks 8–10,spatial games 11,genetic control networks 12and many other self-organizing systems.Ordinarily,the connection topology is assumed to be either completely regular or completely random.But many biological,technological and social networks lie somewhere between these two extremes.Here we explore simple models of networks that can be tuned through this middle ground:regular networks ‘rewired’to intro-duce increasing amounts of disorder.We find that these systems can be highly clustered,like regular lattices,yet have small characteristic path lengths,like random graphs.We call them ‘small-world’networks,by analogy with the small-world phenomenon 13,14(popularly known as six degrees of separation 15).The neural network of the worm Caenorhabditis elegans ,the power grid of the western United States,and the collaboration graph of film actors are shown to be small-world networks.Models of dynamical systems with small-world coupling display enhanced signal-propagation speed,computational power,and synchronizability.In particular,infectious diseases spread more easily in small-world networks than in regular lattices.To interpolate between regular and random networks,we con-sider the following random rewiring procedure (Fig.1).Starting from a ring lattice with n vertices and k edges per vertex,we rewire each edge at random with probability p .This construction allows us to ‘tune’the graph between regularity (p ¼0)and disorder (p ¼1),and thereby to probe the intermediate region 0Ͻp Ͻ1,about which little is known.We quantify the structural properties of these graphs by their characteristic path length L (p )and clustering coefficient C (p ),as defined in Fig.2legend.Here L (p )measures the typical separation between two vertices in the graph (a global property),whereas C (p )measures the cliquishness of a typical neighbourhood (a local property).The networks of interest to us have many vertices with sparse connections,but not so sparse that the graph is in danger of becoming disconnected.Specifically,we require n q k q ln ðn Þq 1,where k q ln ðn Þguarantees that a random graph will be connected 16.In this regime,we find that L ϳn =2k q 1and C ϳ3=4as p →0,while L ϷL random ϳln ðn Þ=ln ðk Þand C ϷC random ϳk =n p 1as p →1.Thus the regular lattice at p ¼0is a highly clustered,large world where L grows linearly with n ,whereas the random network at p ¼1is a poorly clustered,small world where L grows only logarithmically with n .These limiting cases might lead one to suspect that large C is always associated with large L ,and small C with small L .On the contrary,Fig.2reveals that there is a broad interval of p over which L (p )is almost as small as L random yet C ðp Þq C random .These small-world networks result from the immediate drop in L (p )caused by the introduction of a few long-range edges.Such ‘short cuts’connect vertices that would otherwise be much farther apart than L random .For small p ,each short cut has a highly nonlinear effect on L ,contracting the distance not just between the pair of vertices that it connects,but between their immediate neighbourhoods,neighbourhoods of neighbourhoods and so on.By contrast,an edge*Present address:Paul zarsfeld Center for the Social Sciences,Columbia University,812SIPA Building,420W118St,New York,New York 10027,USA.Nature © Macmillan Publishers Ltd 19988letters to natureNATURE |VOL 393|4JUNE 1998441removed from a clustered neighbourhood to make a short cut has,at most,a linear effect on C ;hence C (p )remains practically unchanged for small p even though L (p )drops rapidly.The important implica-tion here is that at the local level (as reflected by C (p )),the transition to a small world is almost undetectable.To check the robustness of these results,we have tested many different types of initial regular graphs,as well as different algorithms for random rewiring,and all give qualitatively similar results.The only requirement is that the rewired edges must typically connect vertices that would otherwise be much farther apart than L random .The idealized construction above reveals the key role of short cuts.It suggests that the small-world phenomenon might be common in sparse networks with many vertices,as even a tiny fraction of short cuts would suffice.To test this idea,we have computed L and C for the collaboration graph of actors in feature films (generated from data available at ),the electrical power grid of the western United States,and the neural network of the nematode worm C.elegans 17.All three graphs are of scientific interest.The graph of film actors is a surrogate for a social network 18,with the advantage of being much more easily specified.It is also akin to the graph of mathematical collaborations centred,traditionally,on P.Erdo¨s (partial data available at /ϳgrossman/erdoshp.html).The graph of the power grid is relevant to the efficiency and robustness of power networks 19.And C.elegans is the sole example of a completely mapped neural network.Table 1shows that all three graphs are small-world networks.These examples were not hand-picked;they were chosen because of their inherent interest and because complete wiring diagrams were available.Thus the small-world phenomenon is not merely a curiosity of social networks 13,14nor an artefact of an idealizedmodel—it is probably generic for many large,sparse networks found in nature.We now investigate the functional significance of small-world connectivity for dynamical systems.Our test case is a deliberately simplified model for the spread of an infectious disease.The population structure is modelled by the family of graphs described in Fig.1.At time t ¼0,a single infective individual is introduced into an otherwise healthy population.Infective individuals are removed permanently (by immunity or death)after a period of sickness that lasts one unit of dimensionless time.During this time,each infective individual can infect each of its healthy neighbours with probability r .On subsequent time steps,the disease spreads along the edges of the graph until it either infects the entire population,or it dies out,having infected some fraction of the population in theprocess.p = 0p = 1Regular Small-worldRandomFigure 1Random rewiring procedure for interpolating between a regular ring lattice and a random network,without altering the number of vertices or edges in the graph.We start with a ring of n vertices,each connected to its k nearest neighbours by undirected edges.(For clarity,n ¼20and k ¼4in the schematic examples shown here,but much larger n and k are used in the rest of this Letter.)We choose a vertex and the edge that connects it to its nearest neighbour in a clockwise sense.With probability p ,we reconnect this edge to a vertex chosen uniformly at random over the entire ring,with duplicate edges forbidden;other-wise we leave the edge in place.We repeat this process by moving clockwise around the ring,considering each vertex in turn until one lap is completed.Next,we consider the edges that connect vertices to their second-nearest neighbours clockwise.As before,we randomly rewire each of these edges with probability p ,and continue this process,circulating around the ring and proceeding outward to more distant neighbours after each lap,until each edge in the original lattice has been considered once.(As there are nk /2edges in the entire graph,the rewiring process stops after k /2laps.)Three realizations of this process are shown,for different values of p .For p ¼0,the original ring is unchanged;as p increases,the graph becomes increasingly disordered until for p ¼1,all edges are rewired randomly.One of our main results is that for intermediate values of p ,the graph is a small-world network:highly clustered like a regular graph,yet with small characteristic path length,like a random graph.(See Fig.2.)T able 1Empirical examples of small-world networksL actual L random C actual C random.............................................................................................................................................................................Film actors 3.65 2.990.790.00027Power grid 18.712.40.0800.005C.elegans 2.65 2.250.280.05.............................................................................................................................................................................Characteristic path length L and clustering coefficient C for three real networks,compared to random graphs with the same number of vertices (n )and average number of edges per vertex (k ).(Actors:n ¼225;226,k ¼61.Power grid:n ¼4;941,k ¼2:67.C.elegans :n ¼282,k ¼14.)The graphs are defined as follows.Two actors are joined by an edge if they have acted in a film together.We restrict attention to the giant connected component 16of this graph,which includes ϳ90%of all actors listed in the Internet Movie Database (available at ),as of April 1997.For the power grid,vertices represent generators,transformers and substations,and edges represent high-voltage transmission lines between them.For C.elegans ,an edge joins two neurons if they are connected by either a synapse or a gap junction.We treat all edges as undirected and unweighted,and all vertices as identical,recognizing that these are crude approximations.All three networks show the small-world phenomenon:L ՌL random but C q C random.00.20.40.60.810.00010.0010.010.11pFigure 2Characteristic path length L (p )and clustering coefficient C (p )for the family of randomly rewired graphs described in Fig.1.Here L is defined as the number of edges in the shortest path between two vertices,averaged over all pairs of vertices.The clustering coefficient C (p )is defined as follows.Suppose that a vertex v has k v neighbours;then at most k v ðk v Ϫ1Þ=2edges can exist between them (this occurs when every neighbour of v is connected to every other neighbour of v ).Let C v denote the fraction of these allowable edges that actually exist.Define C as the average of C v over all v .For friendship networks,these statistics have intuitive meanings:L is the average number of friendships in the shortest chain connecting two people;C v reflects the extent to which friends of v are also friends of each other;and thus C measures the cliquishness of a typical friendship circle.The data shown in the figure are averages over 20random realizations of the rewiring process described in Fig.1,and have been normalized by the values L (0),C (0)for a regular lattice.All the graphs have n ¼1;000vertices and an average degree of k ¼10edges per vertex.We note that a logarithmic horizontal scale has been used to resolve the rapid drop in L (p ),corresponding to the onset of the small-world phenomenon.During this drop,C (p )remains almost constant at its value for the regular lattice,indicating that the transition to a small world is almost undetectable at the local level.Nature © Macmillan Publishers Ltd 19988letters to nature442NATURE |VOL 393|4JUNE 1998Two results emerge.First,the critical infectiousness r half ,at which the disease infects half the population,decreases rapidly for small p (Fig.3a).Second,for a disease that is sufficiently infectious to infect the entire population regardless of its structure,the time T (p )required for global infection resembles the L (p )curve (Fig.3b).Thus,infectious diseases are predicted to spread much more easily and quickly in a small world;the alarming and less obvious point is how few short cuts are needed to make the world small.Our model differs in some significant ways from other network models of disease spreading 20–24.All the models indicate that net-work structure influences the speed and extent of disease transmis-sion,but our model illuminates the dynamics as an explicit function of structure (Fig.3),rather than for a few particular topologies,such as random graphs,stars and chains 20–23.In the work closest to ours,Kretschmar and Morris 24have shown that increases in the number of concurrent partnerships can significantly accelerate the propaga-tion of a sexually-transmitted disease that spreads along the edges of a graph.All their graphs are disconnected because they fix the average number of partners per person at k ¼1.An increase in the number of concurrent partnerships causes faster spreading by increasing the number of vertices in the graph’s largest connected component.In contrast,all our graphs are connected;hence the predicted changes in the spreading dynamics are due to more subtle structural features than changes in connectedness.Moreover,changes in the number of concurrent partners are obvious to an individual,whereas transitions leading to a smaller world are not.We have also examined the effect of small-world connectivity on three other dynamical systems.In each case,the elements were coupled according to the family of graphs described in Fig.1.(1)For cellular automata charged with the computational task of density classification 25,we find that a simple ‘majority-rule’running on a small-world graph can outperform all known human and genetic algorithm-generated rules running on a ring lattice.(2)For the iterated,multi-player ‘Prisoner’s dilemma’11played on a graph,we find that as the fraction of short cuts increases,cooperation is less likely to emerge in a population of players using a generalized ‘tit-for-tat’26strategy.The likelihood of cooperative strategies evolving out of an initial cooperative/non-cooperative mix also decreases with increasing p .(3)Small-world networks of coupled phase oscillators synchronize almost as readily as in the mean-field model 2,despite having orders of magnitude fewer edges.This result may be relevant to the observed synchronization of widely separated neurons in the visual cortex 27if,as seems plausible,the brain has a small-world architecture.We hope that our work will stimulate further studies of small-world networks.Their distinctive combination of high clustering with short characteristic path length cannot be captured by traditional approximations such as those based on regular lattices or random graphs.Although small-world architecture has not received much attention,we suggest that it will probably turn out to be widespread in biological,social and man-made systems,oftenwith important dynamical consequences.ⅪReceived 27November 1997;accepted 6April 1998.1.Winfree,A.T.The Geometry of Biological Time (Springer,New Y ork,1980).2.Kuramoto,Y.Chemical Oscillations,Waves,and Turbulence (Springer,Berlin,1984).3.Strogatz,S.H.&Stewart,I.Coupled oscillators and biological synchronization.Sci.Am.269(6),102–109(1993).4.Bressloff,P .C.,Coombes,S.&De Souza,B.Dynamics of a ring of pulse-coupled oscillators:a group theoretic approach.Phys.Rev.Lett.79,2791–2794(1997).5.Braiman,Y.,Lindner,J.F.&Ditto,W.L.Taming spatiotemporal chaos with disorder.Nature 378,465–467(1995).6.Wiesenfeld,K.New results on frequency-locking dynamics of disordered Josephson arrays.Physica B 222,315–319(1996).7.Gerhardt,M.,Schuster,H.&Tyson,J.J.A cellular automaton model of excitable media including curvature and dispersion.Science 247,1563–1566(1990).8.Collins,J.J.,Chow,C.C.&Imhoff,T.T.Stochastic resonance without tuning.Nature 376,236–238(1995).9.Hopfield,J.J.&Herz,A.V.M.Rapid local synchronization of action potentials:Toward computation with coupled integrate-and-fire neurons.Proc.Natl A 92,6655–6662(1995).10.Abbott,L.F.&van Vreeswijk,C.Asynchronous states in neural networks of pulse-coupled oscillators.Phys.Rev.E 48(2),1483–1490(1993).11.Nowak,M.A.&May,R.M.Evolutionary games and spatial chaos.Nature 359,826–829(1992).12.Kauffman,S.A.Metabolic stability and epigenesis in randomly constructed genetic nets.J.Theor.Biol.22,437–467(1969).gram,S.The small world problem.Psychol.Today 2,60–67(1967).14.Kochen,M.(ed.)The Small World (Ablex,Norwood,NJ,1989).15.Guare,J.Six Degrees of Separation:A Play (Vintage Books,New Y ork,1990).16.Bollaba´s,B.Random Graphs (Academic,London,1985).17.Achacoso,T.B.&Yamamoto,W.S.AY’s Neuroanatomy of C.elegans for Computation (CRC Press,BocaRaton,FL,1992).18.Wasserman,S.&Faust,K.Social Network Analysis:Methods and Applications (Cambridge Univ.Press,1994).19.Phadke,A.G.&Thorp,puter Relaying for Power Systems (Wiley,New Y ork,1988).20.Sattenspiel,L.&Simon,C.P .The spread and persistence of infectious diseases in structured populations.Math.Biosci.90,341–366(1988).21.Longini,I.M.Jr A mathematical model for predicting the geographic spread of new infectious agents.Math.Biosci.90,367–383(1988).22.Hess,G.Disease in metapopulation models:implications for conservation.Ecology 77,1617–1632(1996).23.Blythe,S.P .,Castillo-Chavez,C.&Palmer,J.S.T oward a unified theory of sexual mixing and pair formation.Math.Biosci.107,379–405(1991).24.Kretschmar,M.&Morris,M.Measures of concurrency in networks and the spread of infectious disease.Math.Biosci.133,165–195(1996).25.Das,R.,Mitchell,M.&Crutchfield,J.P .in Parallel Problem Solving from Nature (eds Davido,Y.,Schwefel,H.-P.&Ma¨nner,R.)344–353(Lecture Notes in Computer Science 866,Springer,Berlin,1994).26.Axelrod,R.The Evolution of Cooperation (Basic Books,New Y ork,1984).27.Gray,C.M.,Ko¨nig,P .,Engel,A.K.&Singer,W.Oscillatory responses in cat visual cortex exhibit inter-columnar synchronization which reflects global stimulus properties.Nature 338,334–337(1989).Acknowledgements.We thank B.Tjaden for providing the film actor data,and J.Thorp and K.Bae for the Western States Power Grid data.This work was supported by the US National Science Foundation (Division of Mathematical Sciences).Correspondence and requests for materials should be addressed to D.J.W.(e-mail:djw24@).0.150.20.250.30.350.00010.0010.010.11rhalfpaFigure 3Simulation results for a simple model of disease spreading.The community structure is given by one realization of the family of randomly rewired graphs used in Fig.1.a ,Critical infectiousness r half ,at which the disease infects half the population,decreases with p .b ,The time T (p )required for a maximally infectious disease (r ¼1)to spread throughout the entire population has essen-tially the same functional form as the characteristic path length L (p ).Even if only a few per cent of the edges in the original lattice are randomly rewired,the time to global infection is nearly as short as for a random graph.0.20.40.60.810.00010.0010.010.11pb。
GE_Smallworld产品功能特性及优势

1.1GE Smallworld产品功能特性及优势GE-Smallworld 的创新性解决方案是基于Smallworld 核心空间技术(Smallworld Core Spatial Technology)。
这一革命性的、面向对象以及以数据库驱动的核心产品在每个GE Smallworld 的软件产品的中心都提供了强有力的始终如一的构造。
这种强有力的软件引擎是当今最具可延伸性、可扩展性、以及开放性的开发环境,并由此给组织带来了多方面的收益,比如:低风险投资、低成本的拥有、快速执行、投资的快速回报以及经得起时间考验的结构。
Smallworld 核心空间技术是给当今全球近千个大型GIS 应用系统提供支持的基础。
通过按客户具体要求来开发软件,Smallworld 核心比传统的系统开发项目节省几个月甚至几年的时间,并由此带来低风险和更快的投资回报。
Smallworld 核心之所以成为如此强有力的软件引擎是因为它具有以下创新性特点:∙强有力以面向对象的语言∙高度可扩展方案∙层级递升结构∙管理版本以及长事务处理能力∙无缝的数据存取和集成大量数据格式∙分布式缓存管理对于电力用户来说,Smallworld 最大的优点在于它是为管线类应用而设计的,这使得它尤其适用于电网公司用户的应用。
先进的系统架构Smallworld 核心空间技术采用引领未来的系统架构,能够以前所未有的方式运用空间信息。
Smallworld 核心是我们所有产品的基础并且是为客户以及合作伙伴进行软件和应用开发的强大平台。
Smallworld 软件被用来开发大型电力、天然气、给排水、电信等行业的GIS 网络资产管理和运营系统,以及评估战略性市场契机等目的。
该软件还与需要空间信息的其他产品融合,包括客户关系管理系统,市场分析系统,网络管理,以及工作管理等。
无缝及连续的数据库GE Smallworld 的核心空间技术在单一环境下具有单独的连续不断的数据结构,用来储存和表示所有的空间和非空间数据。
复杂网络的同步与控制

Complex Network Synchronization and Topology
indentification 第5讲:复杂网络的动力学同步与控制
• Network Synchronization
Synchronization Theorem
• Let
0 1 2 N x1(t) x2(t) xN (t) s(t)
c2 d
be the eigenvalues of the coupling matrix A.
The synchronization state is exponentially stable,
N
xi f (xi ) aij (t)(t)x j , i 1, 2, , N j 1
如果A是常数矩阵,内联是自治的,则动力网络 是非时变的,否则是时变动力网络。
网络同步定义: 首先定义同步流形为线性子空间
M= x : xi xj ,i, j
如果当 t 时,x趋近于M,则称网络同 步. 即 对于所有的节点,在任意初始条件下
Chaos Communications
Francis C M Lau, Michael C K Tse, PolyU Centre for Chaos Control and Synchronization
Network Synchronization
同步是复杂网络的集体行为.
Synchronization Is one of the most Pervasive phenomena in the Universe
Small-World在对等网络中的应用研究
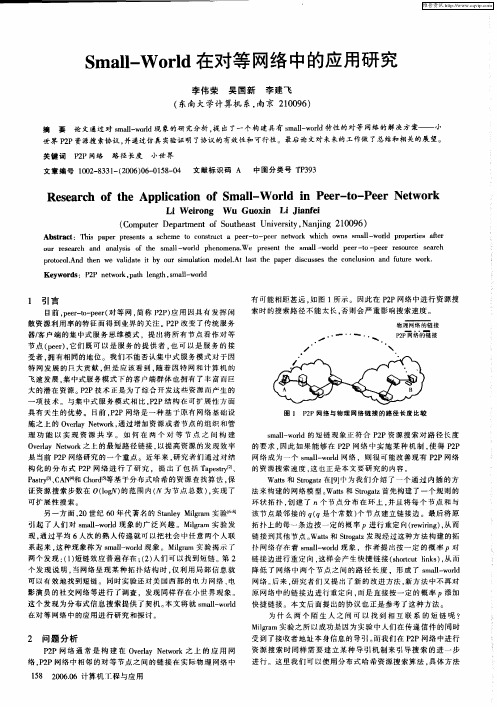
S l W ol mal r — d在对等 网络 中的应用研 究
李 伟荣 吴国新 李建飞 ( 东南大 学计 算机 系, 南京 2 0 9 ) 106
摘 要 论 文通 过 对 s a - ol 象 的研 究 分析 , 出 了一 个 构 建 具 有 s a — ol 性 的 对 等 网 络 的 解 决 方案— — 小 m l wr l d现 提 m l wr l d特
世 界 P P资源搜 索协 议 。 通过 仿 真 实验 证 明 了协 议 的 有效 性 和 可 行性 。最后 论 文对 未 采的 工 作做 了 总结 和相 关 的展 望 。 2 并 关键词 P P网络 2 路 径 长度 小世 界
文 章 编 号 10 — 3 1 (0 60 — 1 8 0 文 献 标 识 码 A 0 2 8 3 一 2 0 )6 0 5 — 4 中 图 分 类号 T 3 3 P 9
R s a c f t e Ap l a i n o m a l W o I n P e - o Pe r Ne wo k e r h o h pi t f S c o l — rd i e r t - e t r
LiW er n W u Gu x n LiJa f i io g o i i n e
一
项 技 术 。 与集 中式 服 务 模 式 相 比 .2 P P结 构 在 可 扩 展 性 方 面
图 1 P P网 络 与物 理 网络 链 接 的 路 径 长 度 E较 2
具 有 天 生 的优 势 。 目前 ,2 P P网络 是 一 种 基 于 原 有 网 络 基 础 设 施 之 上 的 O el e ok 通 过 增 加 资 源 或 者 节 点 的 组 织 和 管 vr yN t r 。 a w 理 功 能 以 实 现 资 源 共 享 。 如 何 在 两 个 对 等 节 点 之 间 构 建 O el e ok之 上 的 最 短 路 径 链接 , 提 高 资 源 的 发 现 效 率 vr y N t r a w 以 是 当前 P P网络 研 究 的一 个 重 点 。近 年 来 , 究 者 们 通 过 对 结 2 研
The Small World Phenomenon小世界现象
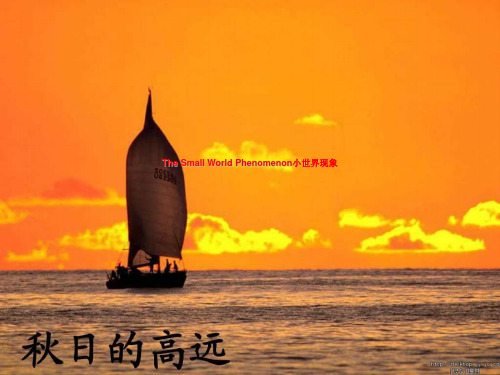
The participants could only forward a letter to a single person that he/she knew on a first name basis
The Small World Phenomenon小世界现象
An Experiment by Milgram (1967)
Chose a target person Asked randomly chosen “starters” to forward a
letter to the target
5
Modeling a social network
Watts – Strogatz (2019)
Created a model for small-world networks
Local contacts Long-range contacts
Effectively incorporated closed triads and short paths into the same model
Very short paths between arbitrary pairs of nodes Individuals operating with purely local information are
very adept at finding these paths
3
What is the “small world” phenomenon?
19
Analysis
六度分离理论在网络深度营销中的价值体现
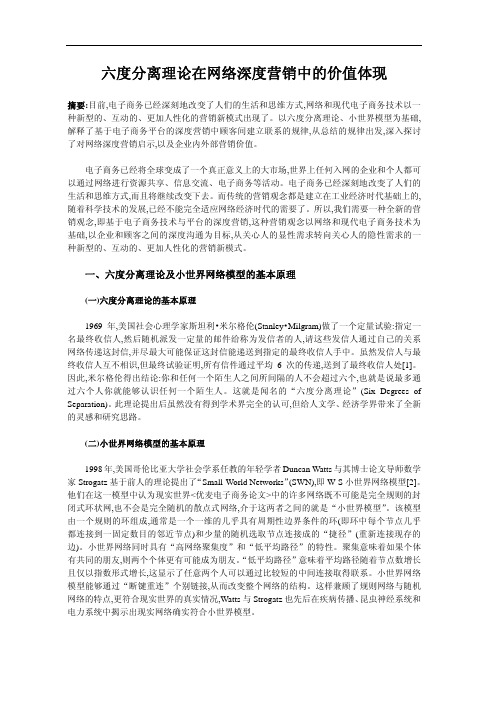
六度分离理论在网络深度营销中的价值体现摘要:目前,电子商务已经深刻地改变了人们的生活和思维方式,网络和现代电子商务技术以一种新型的、互动的、更加人性化的营销新模式出现了。
以六度分离理论、小世界模型为基础,解释了基于电子商务平台的深度营销中顾客间建立联系的规律,从总结的规律出发,深入探讨了对网络深度营销启示,以及企业内外部营销价值。
电子商务已经将全球变成了一个真正意义上的大市场,世界上任何入网的企业和个人都可以通过网络进行资源共享、信息交流、电子商务等活动。
电子商务已经深刻地改变了人们的生活和思维方式,而且将继续改变下去。
而传统的营销观念都是建立在工业经济时代基础上的,随着科学技术的发展,已经不能完全适应网络经济时代的需要了。
所以,我们需要一种全新的营销观念,即基于电子商务技术与平台的深度营销,这种营销观念以网络和现代电子商务技术为基础,以企业和顾客之间的深度沟通为目标,从关心人的显性需求转向关心人的隐性需求的一种新型的、互动的、更加人性化的营销新模式。
一、六度分离理论及小世界网络模型的基本原理(一)六度分离理论的基本原理1969年,美国社会心理学家斯坦利•米尔格伦(Stanley•Milgram)做了一个定量试验:指定一名最终收信人,然后随机派发一定量的邮件给称为发信者的人,请这些发信人通过自己的关系网络传递这封信,并尽最大可能保证这封信能递送到指定的最终收信人手中。
虽然发信人与最终收信人互不相识,但最终试验证明,所有信件通过平均6次的传递,送到了最终收信人处[1]。
因此,米尔格伦得出结论:你和任何一个陌生人之间所间隔的人不会超过六个,也就是说最多通过六个人你就能够认识任何一个陌生人。
这就是闻名的“六度分离理论”(Six Degrees of Separation)。
此理论提出后虽然没有得到学术界完全的认可,但给人文学、经济学界带来了全新的灵感和研究思路。
(二)小世界网络模型的基本原理1998年,美国哥伦比亚大学社会学系任教的年轻学者Duncan Watts与其博士论文导师数学家Strogatz基于前人的理论提出了“Small-World Networks”(SWN),即W-S小世界网络模型[2]。
- 1、下载文档前请自行甄别文档内容的完整性,平台不提供额外的编辑、内容补充、找答案等附加服务。
- 2、"仅部分预览"的文档,不可在线预览部分如存在完整性等问题,可反馈申请退款(可完整预览的文档不适用该条件!)。
- 3、如文档侵犯您的权益,请联系客服反馈,我们会尽快为您处理(人工客服工作时间:9:00-18:30)。
= 2.9 0.1
k 每增加一倍, p(k) 機率變為 1/8.
13
偏好連結(Preferential Attachment) 性質之必要
•假設新結點連接到任何一個節點的機率相等(無 偏好連結) •At time t, the probability that the new node will be connected to any vertex that already has k edges is 1 •p(k) ~ exp(- k) •沒有Scale-free特性
11
Scale-Free Network 特性一: Growth
•Node 的數目隨時間而增加
–在Random Graph或Random Rewiring Graph中,node的數目是固定的 –真實網路中,無論是Internet或WWW, node的數目會隨時間增加而增加
Scale-Free Network 特性二: Preferential Attachment
可使疾病散佈 至一半人口的 疾病傳染機率 Random rewiring 的機率值
16
References
[1] D. J. Watts and S. H. Strogatz, “ Collective dynamics of ‘ small-world’ networks,” Nature, vol. 393, pp. 440-442, June 1998. [2] M. Faloutsos, P. Faloutsos, and C. M. Faloutsos, “ On power-law relationships of the Internet topology,” Proc. SIGCOMM, 1999, pp. 251-262. [3] R. Albert and A.-L. Barabási, “ Statistical mechanics of complex networks,” Review of Modern Physis, to appear. [4] R. Albert, H. Jeong, and A.-L. Barabási, “ Diameter of the World Wide Web,” Nature, vol. 401, pp. 130-131, 1999.
Clustering coefficient = 3 / 6 = 0.5
n = 16 k=4
Degree of Separation(分隔度)
•兩個 node 之間的分隔度為此兩 node 間 最短路徑的長度 •整個網路的分隔度為所有 node pair 分隔 度之平均值
5
Properties of Graphs
如果『富者越富』有極限?
•WWW連結的富者越富現象沒有物理極限 •真實網路的富者越富現象會受物理限制 –飛航網路中,一個機場無法容納太多其它機 場飛來的航機 –電力網路中,變電所不會無限制連結其它電 廠 •如果『富者越富』有極限,最後每個節點的連 結數會差不多 [6]
15
Small-World Network 模型實際應 用:疾病傳播模式
•此處 node 為 web page; link 為之間的連結
Document 有 k 個向外 連結的機率 隨著 k 增加 此機率成指數遞減
Document 有 k 個連結 進來的機率
10
Diameter of WWW [4]
•WWW上約有10億份文件(當時) •WWW的直徑 (Diameter) 約為 19
(k ) m0 t 1
成長(Growth)性質之必要
•Suppose we start with N nodes and no edges •At each time step, we randomly select a node and connect it with probability (ki) to vertex I •After N2 time steps, all nodes are connected
2
Small World 如何解釋?
•如果我們有一張夠大的紙,紙上有60億 個點,每個點代表一個人 •每個點連接最近的50個點(每個人認識 最靠近的50個人) •從紙的一邊走到另一邊需要幾步?
–六千萬步! –六度分隔是錯的嗎?!
Regular Graph
‧有 n 個點,每一個點只與離它最近之 k 個點形成 edge •人際關係網路是一種 Regular graph 嗎?
•連結出現的機率並不一致(富者越富)
–新的node出現時,有較高機率會連結到已有 很多連結的node –新的網頁出現時,有較高機率連到 Yahoo 或 Google 這些「熱門網頁」 –新的演員出道時,有較高機率與已成名演員 合作 (與另一個新演員合作挑大樑的機率較 低)
12
Scale-Free Network Model [5]
•某個node的群聚度定義為其鄰居彼此間也 是鄰居的程度
•假設該node有 k 個鄰居 –此 k 個鄰居間所有可能形成的連結總數為 k (k-1)/2。 –此node的群聚度為此 k 個鄰居間真正形成的 連結總數除以可能形成的連結總數
•整個網路的群聚度為所有node群聚度的平 均值
4
Degree of Clustering in a Regular Graph
n=8 k=4
3
Random Graphs
‧我們來假設另一種模型,其中每一個點 與其它點形成 edge 的機率均相同 ‧如果每個人與其它50個(隨機選取的) 人熟識,則60億人滿足六度分隔性質 ‧此為隨機圖 (Random Graph) •人際關係網路是一種隨機圖嗎?
Degree of Clustering(群聚度)
Edge Rewiring Probability p
•p = 0
–no edges are rewired –regular graph
•p = 1
–All edges are rewired randomly –random graph
•0 < p < 1
–small-world networks
8
Small World Networks 範例二
•美國西部電力網 –Node: 發電機、變壓器、變電所 (n=4941) –Edge: 高壓輸送線 (平均連結數 k=2.67)
‧分隔度18.7 (Random Network = 12.4) ‧群聚度0.080 (Random Network = 0.005)
(ki ) ki k j
j
ki: node i 的連結數
After t Time Steps
•A random network with t + m0 nodes and mt edges •The probability that a node has k edges follows a power law
7
如何解釋人際關係網路?
•每個人和最親近的50個人相連 •Rewiring probability = 2/10000.
–分隔度降為 8
•Rewiring probability = 3/10000.
–分隔度降為 5
•少數幾條捷徑(Short Cuts)扮演關鍵角色 •群聚度幾乎沒變
Small World Networks 範例一
•Starts with a regular network •Choose a node and for each of its edge: With probability p, we reconnect this edge to a node chosen uniformly at random •Repeat this until all edges have been considered
–由一份文件到另外任何一份文件所需點選的 連結次數
•全球資訊網也是 Small-world network •它和以Random Rewiring造出來的 small-world network 一樣嗎?
Small World Networks不只一種?
•Random Rewiring造出來的 small-world network中,每個node的連結數相同 •Internet或WWW中,少數幾個node擁有 非常多的連結。但大多數的node連結數 都不多。 •這種網路稱為Scale-free Network
•以人為Node; 彼此認識的兩個人之間存 在Edge
60 億人
Clustering Effects(群聚效應)
‧在人際關係網路中,你與每個人形成 edge的機率並不相同 ‧你與唸中華大學的人(住台灣的人)熟 識的機率,比你與唸開羅大學的人(住 烏茲別克的人)熟識的機率高 ‧人比較容易熟識住在附近的人!
•電影資料庫(The Internet Movie Database) –/ –225,226 位演員 (nodes) –曾合演過一部電影的演員即有連結 –平均連結數 k = 61 –分隔度3.65 (Random Network = 2.99) –群聚度0.79 (Random Network = 0.00027)
Small World Networks
•高群聚度 (Degree of clustering) •低分隔度數 (Degree of separation) •Question: How to create (model) a small-world network?
ห้องสมุดไป่ตู้
6
Random Rewiring [1]
14
More Details About the Model
•我們假設 (k) ~ k,真實狀況中 (k) ~ k ‧不同網路的 p(k) 值雖皆遵循Power law p(k) = k - ,但 值的範圍從2.1至4。此模 型的 值為3。 •很多網路不只會為新的節點加入連結, 也會在舊的節點中加入連結 •很多網路有時也會移除連結