Estimation of safety distances in the vicinity of fuel gas pipelines
Triangulation

TriangulationTriangulation Prior to the emergence of electronic distance measuring equipment, triangulation was the preferred and principal method for horizontal control surveys, especially if extensive areas were to be covered. Angles could be more easily measured compared with distances, particularly where long lines over rugged and forested terrain were involved, by erecting the very versatile Bilby towers. The method possesses a large number of inherent checks and closure conditions which help detect blunders and errors in field data and increase the possibility of meeting a high standard of accuracy.As implied by its name, triangulation utilizes geometric figures composed of triangles. Horizontal angles and a limited number of sides called base lines are measured. Using the angles and baseline lengths, triangles are solved trigonometrically and positions of stations (vertices) calculated.Different geometric figures have been employed for control extension by triangulation, but chains of quadrilaterals called arcs (Fig. 20-1) are most common. They are the simplest geometric figures permitting rigorous closure checks and adjustments of field observational errors, and they enable point positions to be calculated by two independent routes for computational checks. More complicated figures like that illustrated in Fig. 20-2 are frequently used to establish horizontal control by triangulation in a metropolitan area.Arcs of triangulation originate from one or more stations of known or fixed position and require the azimuth of line. If two or more stations are fixed, azimuth orientation of the network is automatically determined. Today, fixed starting stations and initial azimuths are normally available from other previous higher-order control surveys. The NGS established beginning positions and azimuths for the national network from astronomical observations which also are made at various intervals throughout extensive arcs to check and supplement angle and baseline measurements and help maintain true azimuth orientation. In Fig. 20-1 the arc of triangulation originates from fixed station A and employs the known azimuth of line AB and IJ have been measured. From this information, positions of stations of stations B through IJ were calculated.In executing triangulation surveys, it is general practice to locate a number of intersection stations as part of the project. They can be tall prominent objects in the area, such as church spires, smokestacks, or water towers visible from several triangulation stations.Triangulation Reconnaissance One of the most important aspects of any triangulation survey is the reconnaissance and selection of stations. Factors to be considered are (1) strength of figure, (2) station intervisibility, (3) station accessibility for the original triangulation observing part and surveyors who will subsequently use the stations, and (4) overall project efficiency. Careful attention must be given to each factor in planning and designing the optimum triangulation network; for a given project.Strength of figure deals with the relative accuracies of computed station positions that result from use of angles of various sizes in calculations. Triangulation computations are based on the trigonometric law of sines. The sine function changes significantly for angles near 0°and 180°so a small observational error in an angle close to these values produces a comparatively large difference in position calculations. Conversely, sines of angles near 90 change very slowly; thus,a small observational error in that region causes little change in the computed position since similar observational errors are expected for each angle, design of triangulation figures having favorable angle sizes increases overall triangulation accuracy.Rigorous procedures beyond the scope of this book have been developed for evaluating relative strengths of geometric figures used in triangulation. In general, angles approximately 90°are optimum, and if no angles smaller than 30°or larger than 150°are included in calculations, the figure should have sufficient strength. Locations of triangulation stations fix the angle sizzes so they must be planned carefully for maximum strength of figure. If local terrain or other conditions preclude use of figures having strong angles. More frequent base-line measurements are necessary.Station intervisibility is vital in triangulation because lines of sight to all stations within each figure must be clear for measuring angles. Preliminary decisions on station placement an be resolved from available topographic maps. Intervening ridges that might obstruct sight lines are checked by plotting profiles of the lines between stations. Trees on line and, for long lengths, the combined effects of earth curvature and refraction are additional factors affecting station intervisibility. After making a preliminary decision on station locations, a visual test should be made by visiting each proposed site. Stations are normally placed on the highest points in an area and,if necessary, towers erected to elevate the theodolite, observer, and targets above the ground stations. Because of the uncertainty of refraction near the ground, lines of sight should be kept at least 10 ft above it and not graze intervening ridges.Field Measurements for Triangulation As previously stated, the basic field measurements for triangulation are horizontal angles and base-line lengths. Angles can be measured suing repeating instruments, or more likely directional theodolite such as the Kern DKM-3 having a plate bubble sensitivity of 10-sec/2-mm division, or the Wild T-3 with a 7-sec bubble sensitivity. Both theodolite are suitable for first-order work and permit angles to be read by estimation to the nearest 0.1 sec.To reduce effects of atmospheric refraction on high-order triangulation, observations are made at night with lights for targets. At each station, several “positions”are read; a position consists of angles or directions distributed around the horizontal circle of the instrument in both the direct and plunged modes. With directional theodolite, to compensate for possible circle graduation errors, the circle is advanced by approximately 180°/n for each successive position, where n is the number of positions at the station. Angles should be computed in the field from the directions, checked for acceptable misclosure, and any rejected ones repeated before leaving the station. The average of all satisfactory values for each angle is used in the triangulation calculations.Base lines now preferably are measured by electronic methods, which produce excellent accuracies. Precise Invar tapes may also be used. Several measurements should be made in both directions. Slope distances must be reduced to horizontal and mean sea level lengths calculated, sea level distances are converted to grid lengths by applying scale factors.Triangulation Adjustment Errors that occur in angle and distance measurements require and adjustment. The most rigorous method utilizes least squares. In that procedure, all angle measurements plus distance or azimuth observations can be simultaneously included in the adjustment, and any configuration of quadrilaterals or more complicated figures handled to get station positions having maximum probability. The theory is beyond the scope of this text.Other approximate methods for triangulation adjustments, easily applied to standard figures such as quadrilaterals, also give satisfactory results and are described in advanced surveying books.。
矿井目标定位中移动信标辅助的距离估计新方法

矿井目标定位中移动信标辅助的距离估计新方法胡青松;耿飞;曹灿;张申【摘要】为了降低测距不准对矿井目标定位精度的影响,提出一种移动信标辅助的距离估计方法MBDisEst.该方法由安装有惯导设备或/和激光定位装置的瓦检员或矿车充当移动信标,它们通过与矿山物联网中的其他设备交换信息校准自身坐标.MBDisEst以移动信标和目标节点之间的相对运动和几何约束为基础,利用加权最小二乘法计算目标节点与虚拟信标的距离,可将静止和运动目标的距离估计统一在同一框架.仿真结果表明:MBDisEst的测距精度比TOA的测距精度高,其测距误差随移动信标速度的增大而增大,随移动信标通信半径的增加而减小,基于MBDisEst的定位方法具有较高的定位精度.%To mitigate the affection of the inaccurate distance measurement on the accuracy of the localization system in coal mines, an improved distance estimation method assisted by mobile beacons called MBDisEst was proposed. Some gas inspectors and mining cars equipped with inertial navigation equipment or/and laser positioning devices were selected as mobile anchors, which communicated with other devices for Internet of mine things to calibrate their own coordinates. MBDisEst computed the distances between target nodes and virtual anchors using weighted least square method based on their relative motion and geometrical restriction, and combined static and mobile target scenarios into a unified framework. The simulations show that the distance measurement accuracy of MBDisEst is larger than TOA's, and the measurement error grows up with the speed of mobile anchors and goes down with the communication range of mobile anchors. And thelocalization methods based on distance measurement of MBDisEst has larger accuracy.【期刊名称】《中南大学学报(自然科学版)》【年(卷),期】2017(048)005【总页数】7页(P1227-1233)【关键词】移动信标辅助;矿井目标定位;距离估计;定位精度【作者】胡青松;耿飞;曹灿;张申【作者单位】中国矿业大学信息与控制工程学院,江苏徐州,221008;矿山互联网应用技术国家地方联合工程实验室,江苏徐州,221008;国网北京经济技术研究院徐州勘测设计中心,江苏徐州,221005;中国矿业大学信息与控制工程学院,江苏徐州,221008;矿山互联网应用技术国家地方联合工程实验室,江苏徐州,221008;中国矿业大学信息与控制工程学院,江苏徐州,221008;矿山互联网应用技术国家地方联合工程实验室,江苏徐州,221008【正文语种】中文【中图分类】TD676矿井目标定位系统有助于煤矿企业合理地调配资源,在矿难发生时快速确定受困人员位置[1−2],是煤矿必须配备的安全避险设施之一。
油田生产设施环境安全距离理论与估算方法初探
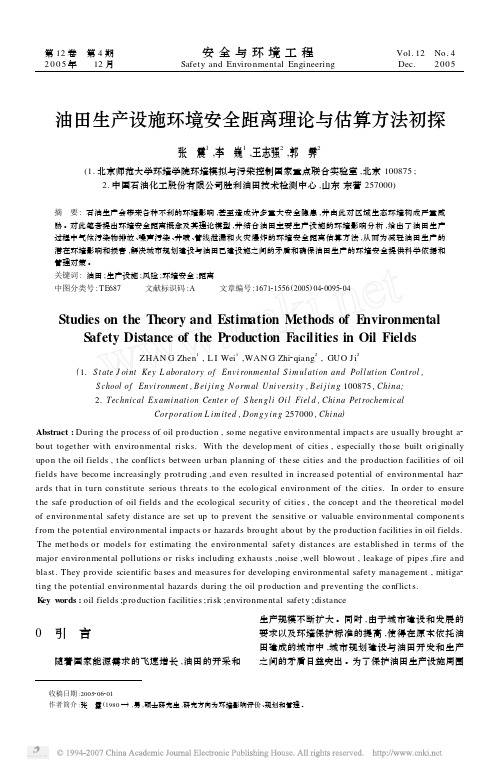
油田生产设施环境安全距离理论与估算方法初探张 震1,李 巍1,王志强2,郭 霁2(1.北京师范大学环境学院环境模拟与污染控制国家重点联合实验室,北京100875;2.中国石油化工股份有限公司胜利油田技术检测中心,山东东营257000)摘 要:石油生产会带来各种不利的环境影响,甚至造成许多重大安全隐患,并由此对区域生态环境构成严重威胁。
对此笔者提出环境安全距离概念及其理论模型,并结合油田主要生产设施的环境影响分析,给出了油田生产过程中气体污染物排放、噪声污染、井喷、管线泄漏和火灾爆炸的环境安全距离估算方法,从而为减轻油田生产的潜在环境影响和损害,解决城市规划建设与油田已建设施之间的矛盾和确保油田生产的环境安全提供科学依据和管理对策。
关键词:油田;生产设施;风险;环境安全;距离中图分类号:TE687 文献标识码:A 文章编号:167121556(2005)0420095204Studies on the Theory and Estim ation Methods of E nvironmental S afety Distance of the Production F acilities in Oil FieldsZHAN G Zhen1,L I Wei1,WAN G Zhi2qiang2,GUO Ji2(1.S t ate J oi nt Key L aboratory of Envi ronment al S i m ul ation and Poll ution Cont rol,S chool of Envi ronment,B ei j i ng N orm al U ni versit y,B ei j i n g100875,Chi na;2.Technical Ex ami nation Center of S hengli Oil Fiel d,Chi na Pet rochemicalCor poration L imited,Dong y i n g257000,Chi na)Abstract:During t he p rocess of oil production,some negative environmental impact s are usually brought a2 bout toget her wit h environmental risks.Wit h t he develop ment of cities,especially t hose built originally upon t he oil fields,t he conflict s between urban planning of t hese cities and t he p roduction facilities of oil fields have become increasingly prot ruding,and even resulted in increased potential of environmental haz2 ards t hat in t urn constit ute serious t hreat s to t he ecological environment of t he cities.In order to ensure t he safe p roduction of oil fields and t he ecological security of cities,t he concept and t he t heoretical model of environmental safety distance are set up to prevent t he sensitive or valuable environmental component s f rom t he potential environmental impact s or hazards bro ught abo ut by t he p roduction facilities in oil fields. The met hods or models for estimating t he environmental safety distances are established in terms of t he major environmental pollutions or risks including exhaust s,noise,well blowout,leakage of pipes,fire and blast.They p rovide scientific bases and measures for developing environmental safety management,mitiga2 ting t he potential environmental hazards during t he oil p roduction and preventing t he conflict s.K ey w ords:oil fields;production facilities;risk;environmental safety;distance0 引 言随着国家能源需求的飞速增长,油田的开采和生产规模不断扩大。
双目视觉自动检测香蕉植株假茎茎高茎宽
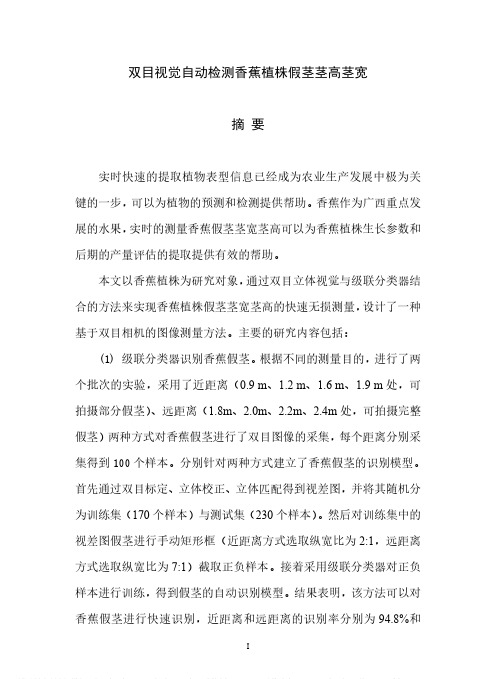
affect the detection accuracy. In the short-distance measurement mode, the measurement accuracy at the 1.2 m distrom the true value reaches 0.8538, and the relative error is only 2.0%.
双目视觉自动检测香蕉植株假茎茎高茎宽
摘要
实时快速的提取植物表型信息已经成为农业生产发展中极为关 键的一步,可以为植物的预测和检测提供帮助。香蕉作为广西重点发 展的水果,实时的测量香蕉假茎茎宽茎高可以为香蕉植株生长参数和 后期的产量评估的提取提供有效的帮助。
本文以香蕉植株为研究对象,通过双目立体视觉与级联分类器结 合的方法来实现香蕉植株假茎茎宽茎高的快速无损测量,设计了一种 基于双目相机的图像测量方法。主要的研究内容包括:
(3) 设计了一种香蕉假茎茎高茎宽联合估算方法。该方法主要针 对远距离测量方式下已获取得到完整的假茎主体的情况。在上一步同 样方法测量得到茎宽参数的同时,也通过两点的间的距离公式计算假 茎的的根部到最低叶片分支下最细的假茎处的置距离的方法得到茎 高的测量结果。结果表明,不同的测量距离会影响检测精度,1.8 m 距离处的香蕉植株假茎茎高茎宽测量精度最高,茎高的识别模型与真 实值的 R2 达到了 0.9717,相对误差仅为 3.1%;茎宽的识别模型与真 实值的 R2 达到了 0.9653,相对误差仅为 1.2%。
(1) The cascade classifier recognizes the pseudo-stem of bananas. According to different measurement purposes, two batches of experiments were carried out, using close distances (0.9 m, 1.2 m, 1.6 m, 1.9 m, some pseudo-stem can be photographed), and long distances (1.8
leg的英语俚语

leg的英语俚语The term "leg" in English slang can refer to a variety of different meanings and contexts. While the literal definition of a leg is one of the two lower limbs of the human body, the slang usage of this word has evolved to encompass all sorts of additional connotations. In this essay, we'll explore the many different ways the word "leg" is used in colloquial English speech and writing.One of the most common slang uses of "leg" is to describe a unit of distance or measurement. For example, someone might say they had to "walk a few legs" to get somewhere, meaning they had to walk a fair distance. This usage stems from the idea that the length of one's leg can be used as a rough estimation of measurement. In the same vein, a person could refer to the "legs" of a journey, meaning the different segments or stages of travel from one place to another.The word "leg" is also often used in sports and athletic contexts. In baseball, for instance, a "leg" can refer to a single base that a runner reaches safely. Getting a "pair of legs" would mean successfully advancing to two bases. Similarly, in horse racing, the differentsections of a race are sometimes called the "legs" of the competition.A horse that wins multiple "legs" of a race series is considered particularly impressive. Even non-sporting activities like hiking can involve "legs" - the different trail sections or converted distances covered during a hike.When it comes to slang related to the human body, "leg" takes on some more colorful connotations. Someone with "good legs" is often considered physically attractive, particularly in reference to a woman's legs. Complimenting a person's "gams" is a more old-fashioned way of commenting on their nice legs. On the flip side, a person who is perceived as unattractive or lacking in physical appeal might be described as having "bum legs."The word "leg" can also be used idiomatically to express other ideas. If someone is said to be "pulling your leg," they are joking or fibbing, trying to trick the listener. Asking someone to "leg it" is a way of telling them to hurry up and get moving. In gambling or gaming, a "leg up" refers to an advantage or head start. And if a plan or scheme is described as having "legs," it means it has longevity and staying power.Interestingly, "leg" has taken on some sexual connotations in slang as well. In this context, it can be used as a verb meaning to engage in sexual activity or flirtation with someone. A person might "leg"another individual at a party, for instance. The term "leg over" is an even more explicit sexual reference. While these usages tend to be considered quite crass, they illustrate how versatile and multifaceted the word "leg" can be in colloquial speech.Beyond just the human body, "leg" has also become a slang term in certain industries and subcultures. In aviation, for example, a "leg" refers to a single flight segment of a longer journey. Pilots and airline staff will often speak of the "legs" of a trip. In the world of crime and law enforcement, a "leg" is used to describe a lead or clue in an investigation. Detectives may pursue different "legs" of a case in search of answers.The slang use of "leg" is not limited to English either. Many other languages have adopted similar colloquial usages of this word. In Spanish, for instance, "la pata" (the leg) can be used informally to refer to a person's foot or lower limb. In French, "une jambe" (a leg) might be used to signify a portion or section of something. These linguistic parallels demonstrate how the slang application of "leg" has become a widespread phenomenon.Ultimately, the versatility of the word "leg" in English slang reflects the central importance of this body part in human experience and culture. From measuring distance to expressing sexuality, legs play a crucial role in our physical, spatial, and metaphorical understandingof the world. The many slang usages of this term highlight just how deeply embedded our conception of "legs" is in the fabric of everyday language and communication. Whether used literally or figuratively, the word "leg" continues to be a rich source of linguistic creativity and expression.。
理工英语3形考答案
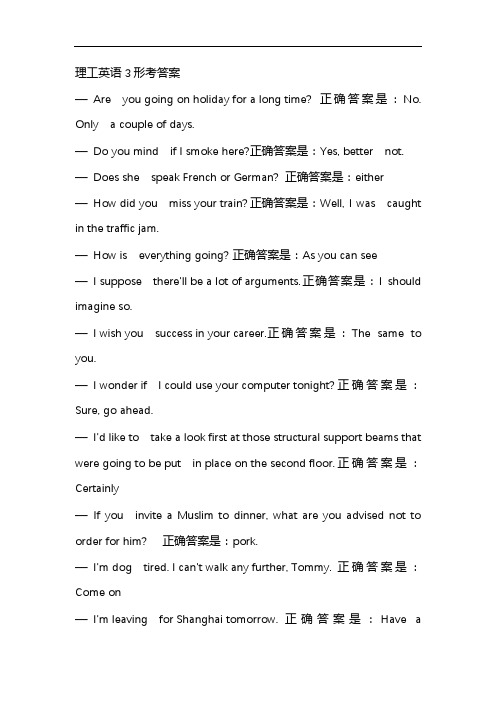
理工英语3形考答案—Are you going on holiday for a long time? 正确答案是:No. Only a couple of days.—Do you mind if I smoke here? 正确答案是:Yes, better not. —Does she speak French or German? 正确答案是:either —How did you miss your train? 正确答案是:Well, I was caught in the traffic jam.—How is everything going? 正确答案是:As you can see—I suppose there'll be a lot of arguments. 正确答案是:I should imagine so.—I wish you success in your career. 正确答案是:The same to you.—I wonder if I could use your computer tonight? 正确答案是:Sure, go ahead.—I'd like to take a look first at those structural support beams that were going to be put in place on the second floor. 正确答案是:Certainly—If you invite a Muslim to dinner, what are you advised not to order for him? 正确答案是:pork.—I'm dog tired. I can't walk any further, Tommy. 正确答案是:Come on—I'm leaving for Shanghai tomorrow. 正确答案是:Have apleasant trip!—In what form will you take the investment? 正确答案是:We'll contribute a site and the required premises.—Is it more advisable to upgrade our present facilities than taking the risk of opening a new park? 正确答案是:I don't think so. —Is it possible for you to expand business there?正确答案是:Yes, I think so.—It's getting dark. I'm afraid I must be off now. 正确答案是:See you.—I've started my own software company. 正确答案是:No kidding! Congratulations!—Jack won't like the film, you know. 正确答案是:So what? —Sorry, I made a mistake again. 正确答案是:Never mind. —What vegetables are in season now? 正确答案是:I think —When do we have to pay the bill? 正确答案是:By—Who should be responsible for the accident? 正确答案是:as told—Would you like some more beer? 正确答案是:Just a little ______ these potential problems, two-way radios are preferable as they are extremely reliable for short distances and can broadcast to several people at once. 正确答案是:Given–__________ father took part in the charity activity in theneighbourhood yesterday? 正确答案是:Whose__________ important it is for kids to imagine freely! 正确答案是:How—________________ about it now? 正确答案是:What's being done A budget is an estimation of the _______ and _______ over a specified future period of time. 正确答案是:revenue; expensesA bus driver _______________ the safety of his passengers. 正确答案是:is responsible forA campus emergency ______ occur at any time of the day or night, weekend, or holiday, with little or no warning. 正确答案是:may。
古代没有尺子时人们测量长度英文作文
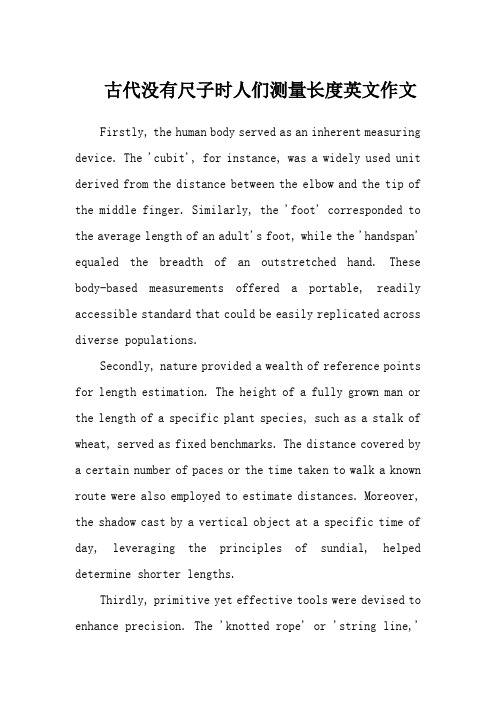
古代没有尺子时人们测量长度英文作文Firstly, the human body served as an inherent measuring device. The 'cubit', for instance, was a widely used unit derived from the distance between the elbow and the tip of the middle finger. Similarly, the 'foot' corresponded to the average length of an adult's foot, while the 'handspan' equaled the breadth of an outstretched hand. These body-based measurements offered a portable, readily accessible standard that could be easily replicated across diverse populations.Secondly, nature provided a wealth of reference points for length estimation. The height of a fully grown man or the length of a specific plant species, such as a stalk of wheat, served as fixed benchmarks. The distance covered by a certain number of paces or the time taken to walk a known route were also employed to estimate distances. Moreover, the shadow cast by a vertical object at a specific time of day, leveraging the principles of sundial, helped determine shorter lengths.Thirdly, primitive yet effective tools were devised to enhance precision. The 'knotted rope' or 'string line,'marked with uniform intervals, allowed for linear measurements. The 'groma,' an early surveying instrument, facilitated the alignment and measurement of right angles in construction projects. Additionally, the 'water level,' utilizing the principle of communicating vessels, enabled the leveling and measurement of horizontal distances.These methods, though seemingly rudimentary, were grounded in keen observation, empirical knowledge, and a deep understanding of the natural world. They fostered a communal sense of standardization, as individuals within a community would have similar bodily proportions and shared familiarity with local flora and environmental cues. Moreover, they were adaptable to various contexts and scalable through repetition or multiplication, ensuring a degree of reliability in construction, trade, and land division.In conclusion, the absence of modern-day rulers did not deter ancient civilizations from measuring lengths accurately. Instead, it prompted them to harness their innate physical attributes, tap into the timeless rhythms of nature, and devise innovative tools, thereby exemplifying human ingenuity and adaptability in the faceof adversity. These early measurement practices laid the groundwork for the development of standardized units and sophisticated instruments that we rely on today, serving as a testament to mankind's ceaseless pursuit of quantification and precision.。
Am. J. Epidemiol.-2006-Yasui-697-705

Practice of EpidemiologyFamilial Relative Risk Estimates for Use in Epidemiologic AnalysesYutaka Yasui 1,Polly A.Newcomb 2,3,Amy Trentham-Dietz 3,and Kathleen M.Egan 41Department of Public Health Sciences,School of Public Health,University of Alberta,Edmonton,Alberta,Canada.2Cancer Prevention Program,Division of Public Health Sciences,Fred Hutchinson Cancer Research Center,Seattle,WA.3University of Wisconsin Comprehensive Cancer Center,Madison,WI.4Vanderbilt University School of Medicine and Vanderbilt-Ingram Cancer Center,Nashville,TN.Received for publication August 7,2005;accepted for publication March 21,2006.Commonly used crude measures of disease risk or relative risk in a family,such as the presence/absence of disease or the number of affected relatives,do not take into account family structures and ages at disease oc-currence.The Family History Score incorporates these factors and has been used widely in epidemiology.How-ever,the Family History Score is not an estimate of familial relative risk;rather,it corresponds to a measure of statistical significance against a null hypothesis that the family’s disease risk is equal to that expected from reference rates.In this paper,the authors consider an estimate of familial relative risk using the empirical Bayes framework.The approach uses a two-level hierarchical model in which the first level models familial relative risk and the second considers a Poisson count of the number of affected relatives given the familial relative risk from the first level.The authors illustrate the utility of this methodology in a large,population-based case-control study of breast cancer,showing that,compared with commonly used summaries of family history including the Family History Score,the new estimates are more strongly associated with case-control status and more clearly detect effect modification of an environmental risk factor by familial relative risk.Bayes theorem;family;Poisson distribution;regression analysis;riskAbbreviations:AFB,age at first birth;CBCS II,Collaborative Breast Cancer Study II;FSIR,Familial Standardized Incidence Ratio;MLE,maximum likelihood estimator.Estimates of disease relative risk in families have impor-tant utilities in investigations of disease etiology.They are used to examine whether the disease of interest clusters in certain families and whether its etiology has a familial component.They are also used to adjust for familial ag-gregations when evaluating the effects of other nonfamilial etiologic factors in epidemiologic studies.Furthermore,familial relative risk estimates are used to examine effect modification of an etiologic factor according to levels of disease relative risk in families.Finally,a valid assessment of familial relative risk may have important clinical utility in triaging persons for more involved genetic screening and informing family members about potential risks.In spite of the important utilities,family history informa-tion is often handled rather crudely in epidemiologic analy-ses.A commonly used summary of family history is a binary indicator (yes/no)of whether study participants have af-fected family members,often gender specific,in first-or second-degree relatives.Another summary that carries a little more information is the number of affected family members.These crude summaries have two critical deficien-cies in view of their use as familial relative risk estimates.First,they do not account for family size,structure,or ages of family rger families and families with older members are naturally more likely to have members who have developed chronic diseases such as cancer.Second,theCorrespondence to Dr.Yutaka Yasui,Department of Public Health Sciences,University of Alberta,13-106J Clinical Sciences Building,Edmonton,Alberta T6G 2G3,Canada (e-mail:yyasui@ualberta.ca).697Am J Epidemiol2006;164:697–705American Journal of EpidemiologyCopyright ª2006by the Johns Hopkins Bloomberg School of Public Health All rights reserved;printed in U.S.A.Vol.164,No.7DOI:10.1093/aje/kwj256Advance Access publication August 21,2006at :: on November 11, 2014/Downloaded fromcrude summaries do not take chance into account:families with identical familial relative risk levels,sizes,structures,and ages can yield different numbers of affected members by chance alone.Kerber (1)proposed the Familial Standardized Incidence Ratio (FSIR)as a measure of familial relative risk that ac-counts for family size,structure,or ages of family members.Boucher and Kerber (2)applied a linear empirical Bayes approach to log f 1þlog(1þFSIR)g with a normality as-sumption to its underlying true values.In this paper,we extend Kerber’s method for estimating familial relative risk levels,applying empirical Bayes estimation methods with a nonparametric discrete prior distribution to overcome the deficiencies of the crude summaries.Following a brief re-view of the Family History Score (3),which was proposed for the same reasons as described above,we explain why it is actually not an estimate of familial relative risk.The utility of the new method proposed here is shown in a large,population-based case-control study of breast cancer.Two main points are illustrated.First,the empirical Bayes esti-mates of familial relative risk are associated with case-control status more strongly than other summary measures of family history,including Family History Scores.Second,they detect an effect modification of an environmental risk factor according to the level of familial relative risk more clearly than do other summary measures.In the Discussion section of this paper,we outline the potential use of the empirical Bayes familial relative risk estimates in other areas of public health and clinical research.FAMILY HISTORY SCOREA method previously proposed to overcome the defi-ciencies of the crude summaries and used widely in epide-miologic analyses is the Family History Score (3).In thisapproach,an expected risk of the disease of interest is com-puted for each family member by using a set of external reference rates for the disease.For i th family’s j th member,the expected risk E ij is given by the cumulative risk of the disease under observation (4):E ij ¼1ÿexp ÿXkk k t ijk !;where k k is the external reference rate for the k th stratum(e.g.,age-sex-race–defined stratum)and t ijk is the length of time that i th family’s j th member spent under observation in the k th stratum.Ages of family members are accounted for in the computation of the expected risks.The Family His-tory Score Z i for i th family is defined byZ i ¼P j O ij ÿP j E ijPj E ij ð1ÿE ij Þn o ;where O ij is the disease indicator of i th family’s j th mem-ber.If the disease is rare,then E ij is approximately equal to Pk k k t ijk and E ij ð1ÿE ij Þ E ij ;resulting in a simpler formula:Z i ¼P j O ij ÿPj E ij Pj E ij1=2:The Family History Score Z i is in the form of a test sta-tistic,which suggests a measure of statistical significance against a null hypothesis that the disease risk for each family member is equal to the expected risk computed from the external reference rates.A Bernoulli random variable O ij has the ‘‘success probability’’E ij under the null hypothesis and,accordingly,we haveE XjO ij 2435¼X jE ij Var XjO ij 2435¼X jE ij ð1ÿE ij Þ;where the variance formula assumes that O ij ’s within each family are uncorrelated.The Family History Score Z i can then be seen as a test statistic in the form of ðX ÿE ½X Þ=ðVar ½X Þ1=2that usually leads to a standard normal large-sample distribution,where the large sample refers to the size of each family being large.A Family History Score is actually not an estimate of the familial relative risk level.It is a test statistic for a null hypothesis that the disease risk for each family member is equal to the expected risk computed from the external ref-erence rates.Statistical significance determined by the ob-served value of a test statistic is a function of a sample size (i.e.,family size,structure,and ages)as well as the degree of departure from the null hypothesis (i.e.,familial relative risk levels).Data for larger families tend to give higher statistical significance and therefore larger absolute values of Family History Scores given the same level of familial risk.Note also that the numeric values of Family History Scores cannot be interpreted directly.They suggest statis-tical significance levels determined according to a known probability distribution of the test statistic.In other words,Family History Scores order families by statistical signifi-cance against the null hypotheses,but their numeric values require a metric,the known probability distribution of the test statistic,in order to have interpretable numeric distances between them.These considerations have led us to a dif-ferent approach to estimating familial relative risk levels,which shares similarities with the methods of Kerber (1)and of Boucher and Kerber (2).EMPIRICAL BAYES ESTIMATES OF FAMILIAL RELATIVE RISKWe define the familial relative risk of the disease for i th family as the relative risk of the disease shared by the mem-bers of i th family relative to the external reference.Our model isE ½O ij ¼h i E ij ;698Yasui et al.Am J Epidemiol 2006;164:697–705at :: on November 11, 2014/Downloaded fromwhere O ij ’s are Bernoulli random variables conditionally independent given h i ’s.We may estimate h i by maximiz-ing the sum of the Bernoulli log-likelihood for i th family.The score equation that the maximum likelihood estimator(MLE)ˆhi satisfies is Pj ðO ij ÿˆh i E ij ÞP j ˆh ið1ÿˆh i E ij Þ¼0and the MLE can be simplified to ˆh i ¼P j O ij =P jE ij ;the standardized mortality (or incidence)ratio,under the rare disease assumption.The precision of the MLEs variesacross families,however,because ˆhi is based solely on i th family’s data,and family sizes,structures,and ages differ across families.Small families with Pj O ij 1could yieldextremely high values of ˆhi ’s just by chance alone.Similar difficulties with the MLEs can occur in other bio-statistical applications such as estimation of small-area dis-ease risks (5)and comparison of risk across hospitals for a given medical procedure (6).A common feature shared by these problems is that there are many parameters to be es-timated,each of which is indexed by one of the units of var-ious sizes (e.g.,families,small areas,and hospitals),and the data available from each unit are limited.As a consequence,extreme values of MLEs occur for small units corresponding to very large variances of MLEs.Such difficulties with MLEs can be alleviated by theuse of hierarchical models in which ˆhi ’s are considered random quantities and are modeled in an additional hier-archical layer.Specifically,the hierarchical model takes the formO ij ’s given h i ’s,are independent Bernoulli randomvariables with E ½O ij j h i ¼h i E ij h i ’s are independent following a common distribution G ;8<:where G denotes a probability distribution over positive real numbers.Let us call the layers for O ij j h i and h i the ‘‘observ-able level’’and the ‘‘latent level’’of the hierarchical model,respectively.The latent level assumes common stochastic features for h i ’s,which provide additional information on shared characteristics of h i ’s that are not used to compute MLEs.By adding the latent level,estimators of h i ’s can ‘‘borrow strength’’from other units (e.g.,families)by com-bining the information on each individual unit with that on the common characteristics of h i ’s.For the distribution G of h i ’s,we propose the use of a (nonparametric)discrete distribution with K levels of fa-milial relative risk f /k ;k ¼1;2;...;K g and their associ-ated probabilities f p k g .While G can be a (parametric)continuous distribution such as gamma or lognormal distri-butions,the nonparametric G has an advantage in its flex-ible shape,determined by the data.Maximum likelihood estimation of the nonparametric G has been discussed by a number of authors (7–9).To compute the MLE of G ,we used the C.A.MAN program (Computer Assisted Mixture ANalysis)of Bo ¨hning et al.(10)and their freeware (11).Once the MLE of G is computed,the empirical Bayes esti-mate of h i is given by the posterior mean of h i with the MLE f ˆ/kg ;f p ˆk g ;and K ˆ:ˆh i ¼P K ˆk ¼1ˆ/k p ˆk L ðo i ;ˆ/k ÞP K ˆk ¼1pˆk L ðo i ;ˆ/k Þ;where L ðo i ;ˆ/kÞis the probability of observing the realiza-tion vector o i ¼ðo i 1;o i 2;...Þgiven /k ¼ˆ/k :Note that ˆh i is of the form of a weighted average of f ˆ/kg :APPLICATION TO AN EPIDEMIOLOGICINVESTIGATION OF BREAST CANCER ETIOLOGYAs an example,we apply the proposed familial risk es-timates to a large,population-based case-control study ofbreast cancer.Two main points are illustrated.First,the empirical Bayes estimates of familial relative risk are asso-ciated with case-control status more strongly than other summary measures of family history,including Family His-tory Scores.Second,these estimates detect an effect modi-fication of an environmental risk factor according to the level of familial relative risk more clearly than do other summary measures.Collaborative Breast Cancer Study IIThe data used in this illustration were derived from the Collaborative Breast Cancer Study II (CBCS II);the CBCS II study protocol was approved by the institutional review boards of the participating institutions (12,13).Briefly,CBCS II was a case-control study of breast cancer in which cases were female residents of Wisconsin,Massachusetts (excluding metropolitan Boston),and New Hampshire with a new diagnosis of invasive breast cancer reported to each state’s cancer registry from January 1992through December 1994and aged 50–79years at the time of diagnosis.Of the 6,839eligible cases,5,685completed the standardized telephone interview (83percent).Community controls were randomly selected in each state by using two sampling frames:those 50–64years of age were selected from lists of licensed drivers,and those 65–79years of age were chosen from rosters of Medicare beneficiaries.The controls were selected at random within age strata to yield an age distri-bution similar to that of the cases within each state.Of the 7,655potential controls,5,951completed the telephone interview (78percent).A 40-minute telephone interview elicited information on the number of sisters and daughters for each participant,their current ages,and the age of their mother.If these fe-male relatives were deceased,the interview inquired about their age at death.Participants were asked whether these first-degree female relatives were ever diagnosed with can-cer (including breast cancer)and,if so,the type of cancer and age at diagnosis.The interview also covered reproduc-tive history,physical activity,selected dietary items,alcohol consumption and tobacco use,use of exogenous hormones,body height and weight,personal medical history,and de-mographic factors.Familial Relative Risk Estimates 699Am J Epidemiol 2006;164:697–705at :: on November 11, 2014/Downloaded fromEmpirical Bayes estimates of familial relative risk of breast cancerUsing the first-degree female family history data col-lected in CBCS II,we estimated familial relative risk lev-els of breast cancer by using the empirical Bayes method.For each first-degree female family member,we calculated her person-years at risk of breast cancer incidence stratify-ing by 5-year age segments from birth to the earlier occur-rence of death or the reference date of her family’s enrolled subject.Reference dates for study subjects were defined as the date of diagnosis for breast cancer cases and,for con-trols,the date randomly sampled from the dates of diagnosis among cases within the same 5-year age stratum (on aver-age,1year prior to interview).We then multiplied each person-time segment by the corresponding age-specific ref-erence rate of breast cancer incidence among White females taken from the data of the Surveillance,Epidemiology,and End Results Program registry (14).Summing the products of the above multiplication for each family member yielded each participant’s expected risk E ij of developing breast cancer.Since it is reasonable to assume the rare disease condition for breast cancer,we were able to approximate the model byO i ð¼Pj O ij Þ’s given h i ’s,are independent Poisson random variables with E ½O i j h i ¼h i P j E ijh i’s are independent following a common nonparametric discrete distribution G :8>><>>:We fitted this model by using the vertex exchange algo-rithm with the Newton-Raphson full-optimization step-length procedure in the C.A.MAN program (UNIX version)(10,11).The initial parameter grid was chosen as 10equally spaced points between a relative risk of 0.1and 5.0.The algorithm was stopped based on the maximum directional derivative with an accuracy level of 0.00001.The C.A.MANprogram identified seven grid points (Kˆ¼7)with positive support,which was then refined with the program’s EM algorithm.The resulting nonparametric MLE of G is shown in figure 1.Three of the seven points were very close to each other around a relative risk of 2.6because the EM algo-rithm was stopped by any practical convergence criterion (10):it stopped at the 806th step.However,this does not have any important consequences,as evident from several examples in the paper that described the C.A.MAN pro-gram in detail (10).Specifically,we can interpret figure 1as showing five relative risk clusters,instead of seven,andFIGURE 1.Nonparametric maximum likelihood estimates of the familial relative risk distribution of breast cancer in the Collaborative Breast Cancer Study II (Wisconsin;Massachusetts,excluding metropolitan Boston;and New Hampshire,1992–1994).700Yasui et al.Am J Epidemiol 2006;164:697–705at :: on November 11, 2014/Downloaded fromFIGURE 2.Empirical Bayes familial relative risk estimates of breast cancer for participants in the Collaborative Breast Cancer Study II (Wisconsin;Massachusetts,excluding metropolitan Boston;and New Hampshire,1992–1994)according to the expected number of affected familymembers.FIGURE 3.Family History Scores of breast cancer for participants in the Collaborative Breast Cancer Study II (Wisconsin;Massachusetts,excluding metropolitan Boston;and New Hampshire,1992–1994)according to the expected number of affected family members.Familial Relative Risk Estimates 701Am J Epidemiol 2006;164:697–705at :: on November 11, 2014/Downloaded fromthe numerical values of the empirical Bayes estimates f ˆhi g would have changed negligibly if the algorithm had run for a longer time.With this nonparametric MLE of G ,the empirical Bayesestimate ˆhi of the familial relative risk level for the i th participant was calculated by the posterior-mean equation.Figure 2displays the empirical Bayes familial relative risk estimates f ˆh i g according to the expected counts f P j E ij g of breast cancer cases in the families.The empirical Bayes familial relative risk estimates are lower for families with larger expected counts for a given observed count of affected family members,P j O ij :This is sensible because,for a given observed count of affected family members,P j O ij ;true familial relative risk should tend to be lower with a larger expected count of affected family members.For CBCS II participants with no family history of breast cancer ðP j O ij ¼0Þ;the empirical Bayes estimates are all less than 1.0.To contrast with the empirical Bayes estimates,the Family History Score values were plotted (figure 3).Recall that Family History Scores are indicators of statistical signifi-cance,not estimates of familial relative risk.Very small dif-ferences in the expected count of affected family members,Pj E ij ;can lead a range of observed counts of affected fam-ily members,P j O ij ;to the same Family History Score;for example,a Family History Score of 6can arise from fam-ilies with ðPj O ij ;P j E ij Þ¼(1,0.03),(2,0.10),(3,0.22),and (4,0.37).Extremely large Family History Score values were observed among the families with the smallest ex-pected counts.These features of Family History Scores are clearly unsuitable for use as estimates of familial relative risk levels.Main effects of family history on disease riskWe examined the degree of association between the case-control status of the CBCS II participants and their familial relative risk estimates to assess the strength of evidence for familial aggregation.We fitted a conditional logistic regres-sion model,conditioned on age group and US state (corre-sponding to the study design),to the case-control data of theTABLE 1.Model deviance and odds ratio estimates with 95%confidence intervals from conditional logistic regression analyses of Collaborative Breast Cancer Study II data (Wisconsin;Massachusetts,excluding metropolitan Boston;and New Hampshire,1992–1994)using various summary measures of familial risk of breast cancer as covariatesSummary measures of familial riskdfUnadjusted *Adjusted y Deviance explainedOdds ratio estimate95%confidenceintervalDeviance explainedOdds ratio estimate95%confidenceintervalFamily historyindicator 1111.5113.6No 1.00 1.00Yes1.731.56,1.92 1.791.60,1.99Observed count(continuous)1116.1 1.591.46,1.73119.8 1.631.49,1.78Observed count 4120.2122.60 1.00 1.001 1.67 1.50,1.87 1.71 1.53,1.922 1.99 1.49,2.66 2.18 1.61,2.963 5.40 2.09,13.93 5.34 2.05,13.9144.260.49,37.07 4.380.50,38.44Family History Score(continuous)187.8 1.141.11,1.1891.5 1.161.12,1.20Family History Score 4118.6118.7<0 1.00 1.00[0,1.305)z 1.42 1.17,1.72 1.54 1.26,1.87[1.305,1.938)z 1.78 1.47,2.16 1.87 1.53,2.28[1.938,2.910)z 1.81 1.49,2.19 1.75 1.43,2.15 2.910z 1.981.63,2.412.071.68,2.55Empirical Bayesestimates (continuous)1123.9 2.50 2.12,2.94122.0 2.58 2.18,3.06*Conditional logistic regression analysis conditional on age and state of residence.y Conditional logistic regression analysis conditional on age and state of residence,adjusting for age at menar-che,parity,age at first birth,age at menopause,body mass index,exogenous hormone use,alcohol consumption,and educational level.z Quartiles of positive Family History Scores.702Yasui et al.Am J Epidemiol 2006;164:697–705at :: on November 11, 2014/Downloaded fromCBCS II with their familial relative risk estimates as a sole covariate(unadjusted analysis)and with a set of adjustment variables(adjusted analysis).The adjustment variables in-cluded participants’age at menarche,parity,age atfirst birth (AFB),age at menopause,body mass index,exogenous hormone use,alcohol consumption,and educational level. Matching on age and the state of residence in the design of the CBCS II was accounted for in the analysis as strata of the conditional logistic regression.Table1presents the deviance explained and odds ratio estimates by each type of familial relative risk estimate in the unadjusted and adjusted condi-tional logistic regression analyses.The amount of deviance explained was used to measure the strength of association between disease status and familial relative risk estimates. Empirical Bayes estimates explained the largest amount of deviance in the unadjusted analysis and nearly the largest in the adjusted analysis using only1degree of freedom,close to the categorical observed counts that used4degrees of freedom.Family History Scores did not show as strong asso-ciations as empirical Bayes estimates,even when the scores were categorized intofive groups(negative and quartiles of positive scores).Thisfinding was consistent with our de-scription earlier that Family History Scores are not estimates of familial relative risk.The results shown in table1suggest that empirical Bayes estimates of familial relative risk pro-vide higher power in the assessment of the main effects of family history(familial aggregation)on disease risk than either the crude summaries or Family History Scores. Examination of an indication of gene-environmental interactionColditz et al.(15)and Egan et al.(16)reported that the effects of reproductive factors on breast cancer risk were modified by family history.Following this intriguingfind-ing,we assessed the effect modification of parity/AFB ef-fects according to familial relative risk levels.We created a covariate of parity and AFB by forming four categories of reproductive patterns:1)nulliparous,2)AFB before age 20years,3)AFB at age20–29years,and4)AFB at age 30years or ing the same conditional logistic re-gression models as those described above(unadjusted and adjusted analyses),we tested an interaction of the parity-AFB covariate with familial relative risk estimates.Three types of familial relative risk estimates were examined,and the results of the unadjusted analysis are shown in table2 (the adjusted analysis gave very similar odds ratio estimates, which are not shown in the tables).The top third of table2shows the odds ratio estimates and95percent confidence intervals for each category of the parity-AFB covariate by presence/absence of family history. The interaction of the parity-AFB covariate and family his-tory was not clear from the odds ratio estimates and was notstatistically significant:v2¼1.74with3degrees of freedom yielding p¼0.63in the unadjusted analysis(p¼0.78in the adjusted analysis).The middle third of this table shows the interaction of the parity-AFB covariate with whether the number of affectedfirst-degree female relatives was two or more.The odds ratio estimates suggest the presence of an effect modification,but the test for interaction was not statistically significant:v2¼4.60with3degrees of freedom yielding p¼0.20in the unadjusted analysis(p¼0.30in the adjusted analysis).The bottom third of table2shows the interaction of the parity-AFB covariate with whether the empirical Bayes es-timate of familial relative risk was1.75or more(i.e.,top 2percent).The odds ratio estimates suggest a pattern of the TABLE2.Odds ratio estimates with95%confidence intervals for parity/age atfirst birth according to various summary measures of familial risk or breast cancer from conditional logistic regression analyses of Collaborative Breast Cancer Study II data(Wisconsin;Massachusetts,excluding metropolitan Boston;and New Hampshire,1992–1994) Familial risk variable andage atfirst birth(years)Odds ratioestimate95%confidenceinterval Family history¼no<20 1.0020–29 1.51 1.26,1.8030 1.47 1.22,1.79Nulliparous 1.28 1.12,1.45 Family history¼yes<20 1.98 1.43,2.7420–29 2.01 1.42,2.8430 1.690.49,1.91Nulliparous 1.62 1.23,2.15Interaction test v2¼1.74(df¼3),p¼0.63<2affectedfirst-degreefemale relatives<20 1.0020–29 1.31 1.16,1.4730 1.58 1.22,1.88Nulliparous 1.57 1.12,1.842affectedfirst-degreefemale relatives<20 3.16 1.43,6.6220–29 1.80 1.42,2.4830 1.940.49,6.19Nulliparous 5.51 1.23,20.55Interaction test v2¼4.60(df¼3),p¼0.20 Empirical Bayesestimate<1.75<20 1.0020–29 1.31 1.17,1.4830 1.58 1.32,1.88Nulliparous 1.57 1.34,1.85 Empirical Bayesestimate 1.75<20 4.80 1.96,11.7220–29 1.59 1.12,2.2430 3.030.64,14.51Nulliparous 5.04 1.33,19.13Interaction test v2¼8.26(df¼3),p¼0.04Familial Relative Risk Estimates703Am J Epidemiol2006;164:697–705 at :: on November 11, 2014 / Downloaded from。
- 1、下载文档前请自行甄别文档内容的完整性,平台不提供额外的编辑、内容补充、找答案等附加服务。
- 2、"仅部分预览"的文档,不可在线预览部分如存在完整性等问题,可反馈申请退款(可完整预览的文档不适用该条件!)。
- 3、如文档侵犯您的权益,请联系客服反馈,我们会尽快为您处理(人工客服工作时间:9:00-18:30)。
Estimation of safety distances in the vicinity of fuel gas pipelinesSpyros Sklavounos,Fotis Rigas*School of Chemical Engineering,National Technical University of Athens,15700Athens,GreeceReceived2November2004;received in revised form19April2005;accepted10May2005AbstractIn this paper,safety distances around pipelines transmitting liquefied petroleum gas and pressurized natural gas are determined considering the possible outcomes of an accidental event associated with fuel gas release from pressurized transmission systems.Possible outcomes of an accidental fuel gas release were determined by performing the Event Tree Analysis approach.Safety distances were computed for two pipeline transmission systems of pressurized natural gas and liquefied petroleum gas existing in Greece using real data given by Greek Refineries and the Greek Public Gas Enterprise.The software packages CHETAH and BREEZE were used for thermochemical mixture properties estimation and quantitative consequence assessment,respectively.Safety distance determination was performed considering jetfire and gas dispersion to the lowerflammable limit as the worst-case scenarios corresponding to immediate and delayed cloud ignition.The results showed that the jetfire scenario should be considered as the limiter for safety distances determination in the vicinity of natural and petroleum gas pipelines.Based on this conclusion,the obtained results were further treated to yield functional diagrams for prompt safety distance estimation.In addition,qualitative conclusions were made regarding the effect of atmospheric conditions on possible events.Thus,wind velocity was found to dominate during a jetfire event suppressing the thermal radiation effect,whereas gas dispersion was found to be affected mainly by solar radiation that favors the faster dissolution of fuel gas below the lowerflammable limit.q2005Elsevier Ltd.All rights reserved.Keywords:Safety distance;Event Tree Analysis;Consequence modeling;Jetfire;Gas dispersion;Pipeline safety1.IntroductionIn recent years,a great deal of effort has been dedicated not only to accident prevention,but also to the mitigation of accident consequences.In Directive96/61/EEC,article3.e, as a general mitigation principle,the necessary measures are indicated that must be taken to limit accident consequences, while in the Directive96/82/EEC(December2001 Amendment)the compliance with appropriate distances between establishments of dangerous substances and residential areas or areas of public use is imposed(article 12.1),in order to limit the effects of a potential accident on people and human property(EEC,1996a,b).Indeed,USA Federal Office of Pipeline Safety(OPS)is going to issue new regulations for gas pipeline integrity management in high consequence areas,which will identify the vulner-ability zones along the length of pipeline installations (DeWolf,2003).Petroleum gas(PG)was always of great importance, used in chemical processes or for domestic service,while natural gas(NG)use has increased rapidly replacing ordinary fuels and electricity not only for environmental but also for economical reasons.As a result,new storage units are constructed and larger amounts of petroleum and especially natural gas are stored and transported worldwide. In addition to sea transport,fuel gases are transmitted through pipeline systems,which is the point of interest of this work.Pipelines transporting fuel gases for commercial purposes have many times been involved in major accidents (IChemE,2000;Khan&Abbasi,1999).Indeed,pipe failure rates assessed from recent European data range from2.1! 10K4(for small diameters)to7.7!10K4(for large diameters)per km per year,being much greater than the standard acceptable failure probability of10K6(Taylor, 1994).It is worth mentioning that a recent work of Yuhua and Datao(2005)based on Fault Tree AnalysisconcludesJournal of Loss Prevention in the Process Industries19(2006)24–31/locate/jlp0950-4230/$-see front matter q2005Elsevier Ltd.All rights reserved.doi:10.1016/j.jlp.2005.05.002*Corresponding author.Address:School of Chemical Engineering,National Technical University of Athens,15780Athens,Greece.Tel.:C302107723267;fax:C302107723163.E-mail address:rigasf@central.ntua.gr(F.Rigas).that an approximate failure probability of a transmission pipeline is6.25!10K2.Safety distances estimated in this work are based on real data given by Greek Refineries and the Greek Public Gas Enterprise concerning two pipeline systems transmitting liquefied petroleum and natural gas,respectively.In order to indicate the plausible accident outcomes of an accidental gas release,the Event Tree Analysis method(CCPS,1992) was applied.Eventually,jetfire event and gas dispersion to the lowerflammable limit were considered purposeful for further examination.Aiming at the investigation of atmospheric conditions effect on probable outcomes,weather conditions were also taken into account in the computations considering the Pasquill–Gifford stability categories(CCPS,1995c).Since pipe diameter and internal pressure are the major factors that determine the magnitude of a potential release(Bartenev, Gelfand,Makhviladge,&Roberts,1996),these parameters were assumed as independent variables in the construction of diagrams suitable for providing direct safety distance putational results for the thermal radiation resulting from a jetfire,as well as for the Lower Flammable Limit(LFL)distance resulting from gas dispersion,would clarify the role of atmospheric conditions(wind speed and solar radiation)in the accident evolution.2.Historical survey of petroleum and natural gas pipeline accidentsSevere accidents associated with NG and PG trans-mission systems have occurred in the past.The main causes initiating a pipeline accidental event may be classified in five categories(Papadakis,1999):†External interference or third party activity†Corrosion†Construction defect and mechanical or material failure †Ground movement or generally natural hazards†Other or unknown causesIt is worth to mention that recent statistical data(OPS, 2005)reveal that during the last20years,natural pipeline accident rates still remain in the same level,in spite of increased safety measures and advanced safety systems applied in practice.Typical examples of such accidents with catastrophic effects on people and property are quoted below: 2.1.NG pipeline explosion andfireOn March241994,at New Jersey,USA,an explosion of an underground natural gas pipeline was followed by a crater of approximately50m diameter and massiveflames which could be seen more than80km away.The accident resulted in1death and50injuries.Subsequent investi-gations revealed that the pipeline had been damaged by excavation works.Probably,a mechanically induced crack grew to size as a result of enhanced fatigue leading to material failure.2.2.NG pipeline explosionOn September281993,at Las Tejerias,Venezuela,an explosion of a natural gas pipeline occurred underneath a highway.The line ruptured while a state telephone company was installingfiber optic cables.The result was40injured people and50dead.2.3.PG pipeline explosionOne of the most severe chemical accidents that ever happened took place on June41989,at Siberia,Russia.A PG pipeline was commissioned in1985to carry mixed PG (propane,butane,pentane,methane and ethane)to feed an industrial city.Subsequently,it was reported that there had been a leakage for several days and that a heavy smell of gas had been reported a few hours before the explosions and fire.Instead of investigating the complaints,the responsible engineers had responded by increasing the pumping rate in order to maintain the required pressure in the pipeline.The leakage point was found about1/2mile away from the side of a railway.The smell of escaping gas was reported from valley habitants in the area and it is also stated that the escaping liquefied gas formed large pockets in low lying areas along the railway line.The gas cloud is reported to have drifted a distance of5miles away.Some hours later, two passenger trains traveling in opposite directions, approached the area.The imminent turbulence due to their motion mixed up PG mist and vapor with the overlying air to form aflammable cloud mixture.One train sparked off the cloud causing an initial explosion.Two explosions took place in quick succession followed by a wall offire that was about1mile wide.A considerable part of each train was derailed,while trees wereflattened and windows were broken within a radius of2.5and8miles,respectively. Totally462people died and706were injured.3.Methodology3.1.Event Tree AnalysisEvent Tree Analysis(ETA)used in this work is a formal technique and one of the standard approaches when performing industrial incidents investigation as well as pipeline risk assessment(Muhlbauer,1996).ETA is a logic sequence that graphically portrays the combination of events and circumstances in an accident sequence.It is an inductive method,which begins with an initiating undesir-able event and works towards afinal result(outcome);each branch of the Event Tree represents a separate accidentS.Sklavounos,F.Rigas/Journal of Loss Prevention in the Process Industries19(2006)24–3125sequence (CCPS,1992).The general procedure for ETA includes the following steps:1.Determination of the initiating events that can result in certain types of accidents.2.Identification of the critical factors that may affect the initiating event evolution.3.Construction of the Event Tree taking into account the interaction between critical factors and the initiating event.4.Designation and evaluation of resulting accidental events.Generally,the ETA is very useful for providing scenarios on possible failure modes,while the final outcomes can be ranked on the basis of their severity by the use of consequence models.3.2.Worst case scenariosLarge amounts of flammable gases released in the open-air pose a significant hazard for the surroundings,due to their ability to yield disastrous fires and explosions.In this work,in which natural and liquefied petroleum gas pipelines were the point of interest,the purpose of safety distance estimation was achieved following the simplified method of Fig.1.The relevant Event Tree (Fig.2)was constructed to indicate all possible outcomes of an accidental fuel gas release (CCPS,1995a )on the basis of the main critical factors that affect substantially the accident evolution:the time of ignition of the resulting cloud and the degree of confinement provided by the surroundings.The former is related to the mixing of escaping fuel gas with the air.When immediate ignition occurs,gas cloud mixing with atmospheric oxygen is still limited;thus,the ignition takes place on the outer layer,which is between the flammable limits,whereas the inner core of the cloud istooFig.1.Logic diagram for safety distancedetermination.Fig.2.Event Tree Analysis adapted to accidental fuel gas release.S.Sklavounos,F.Rigas /Journal of Loss Prevention in the Process Industries 19(2006)24–3126rich in fuel to ignite.As buoyancy forces of the hot gases begin to dominate,the burning cloud rises and becomes more spherical in shape forming a ball intoflames.That elevation causes gradually further mixing of the gas with oxygen,which brings new volumes of gas intoflammable limits sustaining thefire.Fireballs of this kind have been recorded to travel hundreds of meters until fully burned.On the contrary,when delayed ignition occurs,the fuel cloud can be adequately mixed with air,so that after ignition it flashes back.It differs fromfireball since it proceeds faster and can burn from inner to outerflammable layers provided that a proper ignition source is found there.If sufficiently mixed with air,fast burning occurs initially with a medium increase of pressure.This subsonic burning is known as deflagration and is possible when the fuel–air mixture is withinflammability limits,yet far from stoichiometry.If considerable confinement exists and in addition the oxygen content within the cloud is around the Zero Oxygen Balance(stoichiometric fuel–air mixture),the flame propagation speed increases rapidly producing a blast wave(Phillips,1994).In this case,theflame front propagates at a supersonic velocity and a strong shock wave develops in the cloud,which is characterized by an abrupt high overpressure front.The general term for explosions in which a shockwave develops is called detonation.Especially for gaseous mixtures exploding in confined spaces the term Confined Vapor Cloud Explosion (CVCE)is used,in which either a detonation or a deflagration takes place.In a very poor or very rich fuel mixture,but one that is still withinflammable limits,the flame front travels the cloud in low velocity and insignificant pressure increase,a phenomenon known asflashfire.Consequently,buildings inside the LFL radius could be severely damaged due to the potential of a confined vapor cloud explosion.Moreover,if the escaping gas is not trapped and immediate ignition occurs,then a long-lasting jetfire would pose the most significant hazard,due to the high thermal radiation levels(Jo&Ahn,2002).These two outcomes were considered as the dominant hazards for a pipeline fuel gas release event and reckoned as requiring further investigation.putational toolsHydrocarbons are practically non-toxic;even for chronic occupational exposure,systemic effects have not been reported(Hathaway,Proctor,&Hughes,1996).Therefore, in the subsequent investigation the gas concentration prediction during cloud dispersion is limited in gas concentration above the Lower Flammable Limit(LFL). The distance from the point of incident at which concentration value drops below the LFL(LFL distance) is a limiting distance,beyond whichfire or explosion hazard is eliminated.Consequently,a model is needed for predicting gas dispersion,in combination with a model suitable for performing source-term analysis.Furthermore, a quantitative model for calculating thermal radiation effect of jetfire is required.Petroleum and natural gas are not particular compounds but mixtures constituted from several chemical molecules. On the other hand,consequence modeling computations demand the knowledge of some thermochemical properties of the mixtures.For this purpose,another computer program capable of calculating gas mixtures properties was utilized. Each one of the above models is described below.4.1.CHETAH programCHETAH is a computing tool developed by ASTM for predicting both thermochemical properties and certain reactive substance hazards associated with a pure chemical, a mixture of chemicals,or a chemical reaction.This is accomplished through the knowledge of just the molecular structures of the components and mixture composition. CHETAH is useful for classifying materials for their ability to decompose with violence,estimating heats of combustion (used infire models),calculating LFLs(used in dispersion models)and providing hazard potential classification (CCPS,1995b;Shanley&Melhem,1995).4.2.EXPERT modelDispersion models require data related to the source of the release.Such data are the temperature and density at the orifice area and the release rate with which a gas or aerosol escapes into the atmosphere.The EXPERT model calculates these source-term parameters based on user-specified chemical property data of the escaping substance,type of storage equipment,storage conditions and opening size (Grosch&Miller,1998).4.3.SLAB and AFTOX modelSLAB computer model simulates the dispersion of denser-than-air releases.Therefore,it was used for LFL distance calculation resulting from an LPG release,in addition to high pressure(50bar)natural gas release,in which gas density was calculated to be significantly higher (1.75kg/m3)than that of air(1.19kg/m3).It is a slab model with properties averaged in the horizontal and vertical directions thus being a one-dimensional model.The model is based on a set of six differential equations,for the conservation of total mass,material released mass, momentum and energy(Lees,1996).It does not take into account the presence of obstacles in theflowfield assuming a non-sloping unobstructed terrain.AFTOX model is a Gaussian puff model for uniform terrain and wind conditions.It has been designed to simulate lighter-than-air releases and it assumes a non-sloping unobstructed dispersion terrain,while it was usedS.Sklavounos,F.Rigas/Journal of Loss Prevention in the Process Industries19(2006)24–3127for the LFL distance estimation resulting from intermedi-ate(19bar)and low(4bar)natural gas release.4.4.JET FLAME modelThe JET FLAME radiation model predicts the radiation flux of gaseous jetflames resulting from pressurized pipeline accidents,in which the escapingflammable gas is ignited.Thus,it calculates the distance in which a particular thermal radiation intensity value is developed.The model assumes that theflame shape is cylindrical,while it takes into account the internal pipe conditions(pressure and temperature),in addition to atmospheric conditions(CCPS,1995c).EXPERT,JET FLAME,SLAB and AFTOX models are included in BREEZE HAZARD software package,which has been effectively put in practice in previous consequence analysis procedures(Rigas&Sklavounos,2002),while SLAB and AFTOX have been approved by US EPA’s Risk management program for Offsite Consequence Analysis Guidance.However,all of them are based on simplified assumptions that strongly affect the results.Thus,SLAB model computes mass,momentum and energy balances only in one direction(downwind)and assumesflat terrain with no obstructions or slope.Likewise,JET FLAME model does not take into account obstacles intervening between flames and target that actually decrease the thermal radiation impact.Consequently,the results presented hereinafter should be considered conservative.5.Material properties computationEstimations of thermochemical properties of a gas mixture like heat of combustion and physical quantities such as the lowerflammable limit provide useful input data for the operation of consequence models.The above requirements were obtained by using the CHETAH program described previously.In Table1,typical compositions of petroleum and natural gas are given.Since the ratio of secondary components in NG mixture is very low(approximately2%),NG physical properties may be identified with the properties of methane. In contrast,PG mixture consists of more than one component in considerable concentrations(propane and butane)that cannot be merged.Providing the appropriate data of the mixture molar composition,CHETAH code calculated PG combustion heat and lowerflammable limit as shown in Table2.6.Safety distance estimations6.1.Input variablesNominal and operating conditions,as well as the sizes of LPG and NG pipelines studied in this work are shown in Table3.The primary goal of hazard zones determination and hence safety distances assessment is accomplished using consequence analysis models for the worst case scenarios mentioned in Section3.2.Regarding the input data introduced in these models,it is of vital importance to highlight the following:†The wind and particularly its turbulent nature plus solar radiation are significant factors in gas dispersion.The vigorous whirling molecular motion dominating in the atmospheric environment results in gas agitation that may bring faster the cloud into theflammable range (Deaves,1992).Moreover,the shape and size of the jet fire(and hence the thermal radiation emitted)is strongly affected by the wind speed(Arnaldos,Casal,Montiel, Sanchez-Carricondo,&Vilchez,1998).Therefore, atmospheric conditions were taken into account in the computations in the form of the so-called Pasquill–Gifford stability categories.Three discernible classes were reckoned to be significant:A—unstable conditionsTable1Typical composition of natural and petroleum gasGas mixture Components Content(%v.v.)NG(Russian natural gas)Methane98 Ethane0.6 Propane0.2 Butane0.2 Pentane and heavier hydrocarbons0.1 Nitrogen0.8 Carbon dioxide0.1LPG(refinery product)Ethane0.8Propane20.1Butane78.6Pentane and heavierhydrocarbons0.5Table2Petroleum gas combustion heat and LFL calculated with CHETAH codeLPG property Calculated value Input dataCombustion heat45826kJ/kg JET FLAME(jetfiremodel)Lowerflammablelimit1.78%v/v SLAB and AFTOX(dispersion models)Table3Data on operating conditions and internal diameters of LPG and NGpipelinesFuelgasPipeline system Pressure(bar)Diameter(cm)Nominal OperatingNG Internationaltransportation705025–91Interstate transportation191924Regional distribution449–16LPG Regional distribution312436 S.Sklavounos,F.Rigas/Journal of Loss Prevention in the Process Industries19(2006)24–3128attributed to strong solar radiation and low wind speed;D—neutral conditions identified with moderate to strong winds with significant cloud cover and hence low solar radiation;F—stable conditions,corresponding to rela-tively light winds and nights of clear sky (no solar radiation).†For the purpose of safety distance determination concerning the jet fire scenario,maximal permissible levels for thermal radiation intensity must be set.Among relative data (CCPS,1994;Green Book,1989)the more conservative values were adopted (Table 4).With reference to cloud dispersion scenario,the LFL distance was determined.6.2.Results and discussionThe burning of a flammable gas issuing from a pipe or other orifice at the point of exit forms a jet fire.In fact,release sizes range from small leaks to the full loss of contents giving a wide range of gas release rates,which go up to some hundred of kilogram/second with regard to high pressure pipelines (Olorunmaiye &Imide,1993).Initially,release magnitudes were studied for the range of small cracks to full-bore pipe rupture.Typical example demon-strating safety distance change with crack size in different weather conditions (A,F,and D)for the high pressure (50bar)NG pipeline is presented in Fig.3.It is obvious that thermal radiation impact is mainly affected by the wind speed:the lower the wind speed,the greater the radius ofrisk.The conclusion still remains the same for the LPG pipeline (Fig.4),namely the safety distance is maximized for the air stability category A.In contrast,LFL distance is most affected by solar radiation (Fig.5).In particular,the lower the solar radiation,the greater the LFL distance.This conclusion may be qualitatively drawn from Fig.6,in which safety distance has been plotted against wind speed and pipe diameter.It is evident that safety distance is maximized for intermediate wind speed,namely for atmospheric stability category F that corresponds to complete absence of solar radiation.In Fig.7a and b,where jet fire and dispersion scenarios are compared with regard to the resulting safety distances,it is clear that jet fire scenario entails higher safety distances than that of dispersion scenario.Indeed,safety distances are higher when related to human effect rather than to property damage.The latter was expected,since the threshold value for human injury is lower than that of property damage (Table 4).As a result,the jet fire scenario should be considered as the limiter for safety distance determination.The utilization of the results obtained through the jet fire safety distance calculation (considering atmospheric stab-ility category A,full-bore rupture and the pipe diameters and pressures shown in Table 3)yielded the diagramsTable 4Thermal radiation threshold limit values for acceptable damage on people and property Damaged entity Thermal radiation critical value (kW/m 2)Exposure duration Foreseen effectProperty4Not too short(R 30min)Rupture of glassPeople 1.560s1%probability of 1st degreeburnsFig.3.Safety distance for the 0.91m and 50bar NG pipeline related to the jet fire scenario and atmospheric stability categories A,F and D,calculated for small releases to full-borerupture.Fig.4.Safety distance for the LPG pipeline related to the jet fire scenario and atmospheric stability categories A,F and D,calculated for small releases to full-borerupture.Fig.5.Safety distance for the 0.91m and 50bar NG pipeline related to the cloud dispersion scenario and atmospheric stability categories A,F and D,calculated for small releases to full-bore rupture.S.Sklavounos,F.Rigas /Journal of Loss Prevention in the Process Industries 19(2006)24–3129illustrated in Figs.8and 9.Thus,from these Figures,safety distance may be directly determined,when internal pipe pressure and diameter are known.As one can see,safety distance increases when the internal diameter or the operating pressure of the pipeline increases.Nevertheless,safety distance seems to be more sensitive with pipe size rather than operating pressure.As an example,let us consider an internal pipe diameter equal to 60cm (Fig.8).Then for the operating pressure range from 20to 70bar,the safety distance varies between 450and 750m,namely a range of 300m.In contrast,considering a pressure value equal to 50bar,the safety distance variesbetween 325and 925m when the diameter changes from 25to 91cm,namely a range of 600m.This conclusion is to be found in agreement with that of Jo and Ahn (2002).7.ConclusionsFlammable gas transmission pipelines pose major risk for the surroundings,due to the probability of rupture and release of the content to the atmosphere with the potential of a fire or/and explosion.In this work,a methodology for estimating safety distances in the vicinity of petroleum and natural gas pipelines is proposed,with the perspective to find application in emergency response planning.In summary,the following remarks may bereferred:Fig.6.Safety distance plot against wind speed and pipe diameter for the 0.91m and 50bar NG pipeline according to cloud dispersion scenario for full-bore piperupture.Fig.7.Safety distance for both jet fire and dispersion scenarios assuming full-bore rupture of 50bar (a)and 4bar (b)NG pipelines.The stability categories adopted were:A for the thermal radiation safety distance and F for the LFLdistance.Fig.8.Safety distance isopleths (m)against operating pressure and pipe diameter for the 50bar pipeline,assuming atmospheric stability category A,1.5kW/m 2thermal radiation intensity and full-bore piperupture.Fig.9.Safety distance isopleths (m)against operating pressure and pipe diameter for the 4bar pipeline,assuming atmospheric stability category A,1.5kW/m 2thermal radiation intensity and full-bore pipe rupture.S.Sklavounos,F.Rigas /Journal of Loss Prevention in the Process Industries 19(2006)24–3130†The jetfire scenario should be considered as the limiter for safety distances determination in the vicinity of natural and petroleum gas pipelines.†Safety distances are more sensitive to pipeline size than operating pressure.†The thermal effect of a jetfire event,as well as the distance that fuel gas travels from source to its lower flammable limit position,strongly depends on atmos-pheric conditions.Indeed,different weather conditions favor each type of accident:the impact radius thermal radiation is mainly affected by wind speed and increases when wind speed decreases,whereas gas dispersion in mainly affected by solar radiation and LFL distance increases when solar radiation decreases.†Safety distances in the vicinity of fuel gas pipelines may be plotted in diagrams against independent variables.These diagrams could be used in loss prevention applications(i.e.ignition sources elimination within the LFL distance in industrial sites),as well as in safer land-use planning.AcknowledgementsThis work was supported by the Ministry of National Education and Religious Affairs(Community Support Framework2000–2006)under the HERACLITUS research program.ReferencesArnaldos,J.,Casal,J.,Montiel,H.,Sanchez-Carricondo,M.,&Vilchez, J.A.(1998).Design of a computer tool for the evaluation of the consequences of accidental natural gas releases in distribution pipes.Journal of Loss Prevention in the Process Industries,11(2),135–148. Bartenev,A.M.,Gelfand,B.E.,Makhviladge,G.M.,&Roberts,J.P.(1996).Statistical analysis of accidents on the Middle Asia—Centre gas pipelines.Journal of Hazardous Materials,46(1),57–69.CCPS(1992).Guidlines for hazard evaluation procedures.New York: AIChE.CCPS(1994).Guidelines for evaluating the characteristics of vapor cloud explosions,flashfires and BLEVEs.New York:AIChE.CCPS(1995a).Chemical transportation risk analysis.New York:AIChE. CCPS(1995b).Guideliness for safe storage and handling of reactive materials.New York:PS(1995c).Undetstanding atmospheric dispersion of accidental releases.New York:AIChE.Deaves,D.M.(1992).Dense gas dispersion modelling.Journal of Loss Prevention in the Process Industries,5(4),219–227.DeWolf,G.B.(2003).Process safety management in the pipeline industry: Parallels and differences between the pipeline integrity management (IMP)rule of the office of pipeline safety and the PSM/RMP approach for process facilities.Journal of Hazardous Materials,104(1–3),169–192.EEC(1996a).Concerning integrated pollution and control.European Council Directive96/61,Brussels.EEC(1996b).On the control of major-accident hazards involving dangerous substances.European Council Directive96/82,Brussels. Green Book(1989).Methods for the determination of possible damage-CPR16E(2nd ed.).The Netherlands:TNO.Grosch,T.G.,&Miller,M.D.(1998).An expert system for source-term analysis and accidental release modelling.Proceedings of the91st Air and Waste Management Association Annual Meeting,San Diego. Hathaway,G.J.,Proctor,N.H.,&Hughes,P.J.(1996).Chemical hazards for the workplace(4th ed.).New York:Van Nostrand Reinhold. IChemE(2000).The accident database—CD form.Loughborough: IChemE.Jo,Y.D.,&Ahn,B.J.(2002).Analysis of hazard areas associated with high-pressure natural gas pipelines.Journal of Loss Prevention in the Process Industries,15(3),179–188.Khan,F.I.,&Abbasi,S.A.(1999).Major accidents in process industries and an analysis of causes and consequences.Journal of Loss Prevention in the Process Industries,12(5),361–378.Lees,F.P.(1996).Loss prevention in the process industries(2nd ed.).Oxford:Butterworth-Heinemann.Muhlbauer,W.K.(1996).Pipeline risk management manual(2nd ed.).Houston:Gulf.Olorunmaiye,J.A.,&Imide,N.E.(1993).Computation of natural gas pipeline rupture problems using the method of characteristics.Journal of Hazardous Materials,34(1),81–98.OPS(2005).Natural gas incident yearly summaries(1986–2004).US Department of Transportation,Office of Pipeline Safety,Washington. Papadakis,A.G.(1999).Major hazard pipelines:A comparative study of onshore transmission accidents.Journal of Loss Prevention in the Process Industries,12(1),91–107.Phillips,H.(1994).Explosions in the process industries(2nd ed.).Warwickshire:IChemE.Rigas,F.,&Sklavounos,S.(2002).Risk and consequence analyses of hazardous chemicals in marshalling yards and warehouses at Ikonio/Piraeus harbour,Greece.Journal of Loss Prevention in the Process Industries,15(6),531–544.Shanley,E.S.,&Melhem,G.A.(1995).A review of ASTM CHETAH7.0 hazard evaluation criteria.Journal of Loss Prevention in the Process Industries,8(5),261–264.Taylor,J.R.(1994).Risk analysis for process plants,pipelines and transport.London:E&FN Spon.Yuhua,D.,&Datao,Y.(2005).Estimation of failure probability of oil and gas transmission pipelines by fuzzy fault tree analysis.Journal of Loss Prevention in the Process Industries,18(2),83–88.S.Sklavounos,F.Rigas/Journal of Loss Prevention in the Process Industries19(2006)24–3131。