ABSTRACT Learning to Trade With Insider Information
国际经济学作业答案第三章

Home has a comparative advantage in both products.
The opportunity cost of cloth in terms of widgets in Foreign is if it is ascertained that Foreign uses
neither country will want to export the good in which it enjoys comparative advantage.
both countries will want to specialize in cloth.
Given the following information:
both countries could benefit from trade with each other.
neither country could benefit from trade with each other.
each country will want to export the good in which it enjoys comparative advantage.
each country enjoys superior terms of trade.
each country has a more elastic demand for the imported goods.
each country has a more elastic supply for the supplied goods.
语言学名词解释
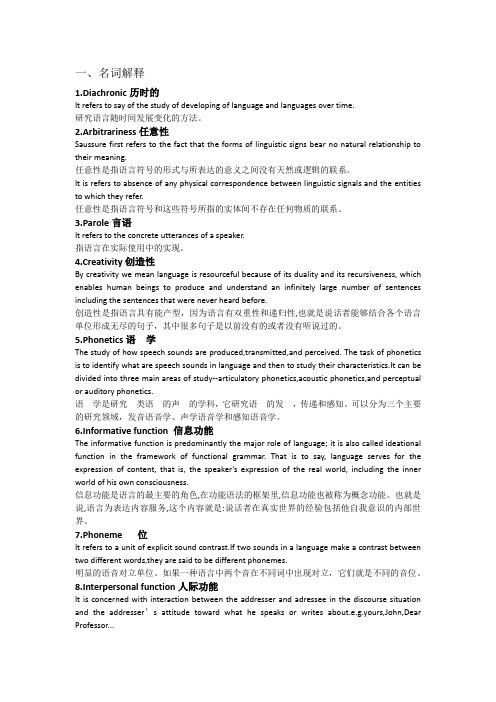
一、名词解释1.Diachronic历时的It refers to say of the study of developing of language and languages over time.研究语言随时间发展变化的方法。
2.Arbitrariness任意性Saussure first refers to the fact that the forms of linguistic signs bear no natural relationship to their meaning.任意性是指语言符号的形式与所表达的意义之间没有天然或逻辑的联系。
It is refers to absence of any physical correspondence between linguistic signals and the entities to which they refer.任意性是指语言符号和这些符号所指的实体间不存在任何物质的联系。
3.Parole言语It refers to the concrete utterances of a speaker.指语言在实际使用中的实现。
4.Creativity创造性By creativity we mean language is resourceful because of its duality and its recursiveness, which enables human beings to produce and understand an infinitely large number of sentences including the sentences that were never heard before.创造性是指语言具有能产型,因为语言有双重性和递归性,也就是说话者能够结合各个语言单位形成无尽的句子,其中很多句子是以前没有的或者没有听说过的。
计算机专业英语chapter
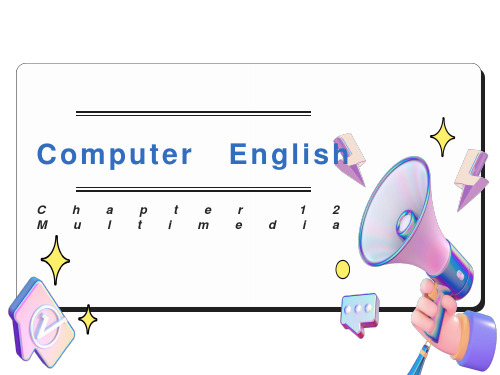
Abbreviations : MIDI (Musical Instrument Digital Interface) 乐器数字接口
12-2 ELEMENTS OF MULTIMEDIA
We break the word multimedia into its component parts, we get multi -meaning more than one ,and media-meaning form of communication. Those types of media include: . Text . Audio Sound . Static Graphics Images . Animation . Full-Motion Video 如果我们把multimedia这个词分开,我们便得到multi——多,和media——媒体。媒体类型包括: · 文本 · 声音 · 静态图像 · 动画 · 动态视频 Logical Structures. Identifying these logical relationships is a job of the data administrator. A data definition language is used for this purpose. The DBMS may then employ one of the following logical structuring techniques during storage access, and retrieval operations [1]: 逻辑结构。确定这些逻辑关系是数据管理者的任务,由数据定义语言完成。DBMS在存储、访问和检索操作过程中可选用以下逻辑构造技术:
trade的用法及短语
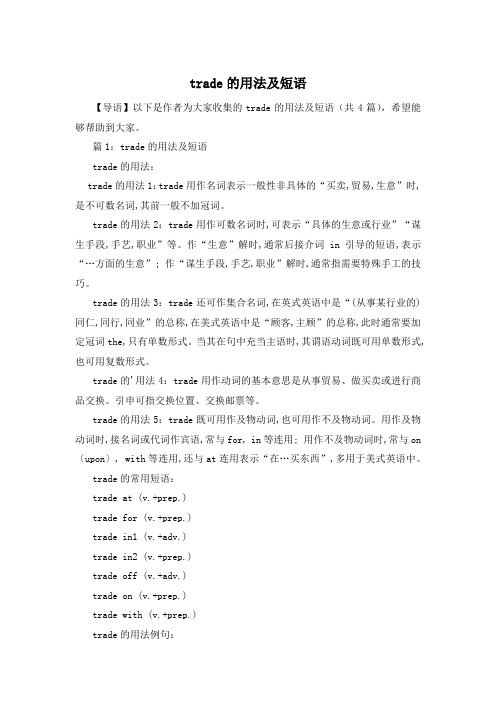
trade的用法及短语【导语】以下是作者为大家收集的trade的用法及短语(共4篇),希望能够帮助到大家。
篇1:trade的用法及短语trade的用法:trade的用法1:trade用作名词表示一般性非具体的“买卖,贸易,生意”时,是不可数名词,其前一般不加冠词。
trade的用法2:trade用作可数名词时,可表示“具体的生意或行业”“谋生手段,手艺,职业”等。
作“生意”解时,通常后接介词in引导的短语,表示“…方面的生意”; 作“谋生手段,手艺,职业”解时,通常指需要特殊手工的技巧。
trade的用法3:trade还可作集合名词,在英式英语中是“(从事某行业的)同仁,同行,同业”的总称,在美式英语中是“顾客,主顾”的总称,此时通常要加定冠词the,只有单数形式。
当其在句中充当主语时,其谓语动词既可用单数形式,也可用复数形式。
trade的'用法4:trade用作动词的基本意思是从事贸易、做买卖或进行商品交换。
引申可指交换位置、交换邮票等。
trade的用法5:trade既可用作及物动词,也可用作不及物动词。
用作及物动词时,接名词或代词作宾语,常与for, in等连用; 用作不及物动词时,常与on 〔upon〕, with等连用,还与at连用表示“在…买东西”,多用于美式英语中。
trade的常用短语:trade at (v.+prep.)trade for (v.+prep.)trade in1 (v.+adv.)trade in2 (v.+prep.)trade off (v.+adv.)trade on (v.+prep.)trade with (v.+prep.)trade的用法例句:1. The nature of the polymer is currently a trade secret.这一聚合物的性质目前是个商业机密。
2. They issue a fixed number of shares that trade publicly.他们发行一定数量的可公开交易的股票。
基于近远程视角分析黄河中下游五省区虚拟水贸易
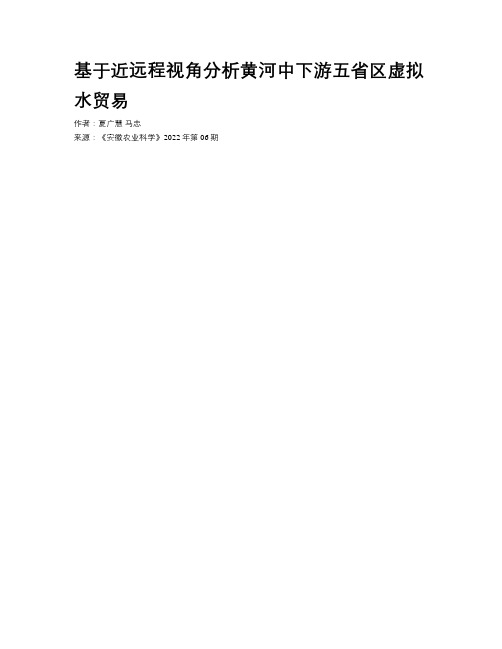
基于近远程视角分析黄河中下游五省区虚拟水贸易作者:夏广慧马忠来源:《安徽农业科学》2022年第06期摘要以區域间投入产出表为基础,量化黄河中下游五省区(山西省、内蒙古、山东省、河南省和陕西省)基于消费的水足迹的规模和结构以及与省级的虚拟水贸易,通过近远程视角分析黄河中下游五省区依靠内外部水资源的程度,并通过实例分析虚拟水贸易的空间流动特点。
结果表明,黄河中下游五省区各部门的用水系数差别较大,其中内蒙古的农业用水系数最高,均高于700 m3/万元;黄河中下游五省区近远程水足迹之比为99∶1,即对内部水资源依赖性较强,对外部水资源依赖性较弱,近程水资源极大地促进了黄河中下游发展;黄河中下游的水足迹与距离呈现出一定的负相关关系,即距离越大,水足迹流动量越小。
五省区的水足迹分别距流出地3.21、68.42、75.18、77.32、19.53 km,其中山西的水足迹距离流出地的平均距离最短,河南的水足迹距离流出地的平均距离最长;在虚拟水近远程贸易中,黄河中下游是虚拟水净流入区,有3 976.6 万m3的虚拟水净流入量;山西省和陕西省是虚拟水净流入区,内蒙古、山东和河南三省区是虚拟水净流出区。
我国蓬勃发展的经济给水资源带来了巨大压力,黄河中下游五省区的水资源压力也在不断增加,提高综合用水效率,调整进出口策略,实施虚拟水贸易策略是黄河中下游高质量发展的重要举措。
关键词近远程视角;黄河中下游;虚拟水贸易中图分类号 F 124.5 文献标识码 A文章编号 0517-6611(2022)06-0178-08doi:10.3969/j.issn.0517-6611.2022.06.042开放科学(资源服务)标识码(OSID):Analysis of Virtual Water Trade in Five Provinces in the Middle and Lower Reaches of the Yellow River Based on Near-distance PerspectiveXIA Guang-hui,MA Zhong (School of Geography and Environmental Sciences,Northwest Normal University,Lanzhou,Gansu 730070)Abstract Based on the inter-regional input-output table,the scale and structure of the consumption-based water footprint of five provinces (Shanxi Province,Inner Mongolia,Shandong Province,Henan Province and Shaanxi Province) and regions in the middle and lower reaches of the Yellow River and their virtual water trade with the provincial level were quantified.This paper analyzed the degree of dependence on internal and external water resources in the five provinces and regions of the middle and lower reaches of the Yellow River from a near and long distance perspective,and analyzed the spatial flow characteristics of virtual water trade through examples.The results showed that the water use coefficien of five provinces and regions in the middle and lower reaches of the Yellow River varied greatly,Inner Mongolia had the highest agricultural water use coefficient,which was higher than 700 m3/104 yuan.The ratio of short-range and long-range water footprints in five provinces in the middle and lower reaches of the Yellow River was 99∶1,which meant that the dependence on internal water resources was strong and the dependen-ce on external water resources was weak.The short-distance water resources greatly promoted the development of the middle and lower reaches of the Yellow River.The water footprint in the middle and lower reaches of the Yellow River presented a negative correlation with distance,that was,the larger the distance,the smaller the water footprint flow.The water footprint of the five provinces was 3.21,68.42,75.18,77.32 and 19.53 km from the outflow place,respectively.The average distance between the water footprint of Shanxi and the outflow place was the shortest,and the average distance between Henan and the outflow place was the longest .In the near and long distance virtual water trade,the middle and lower reaches of the Yellow River were the net inflow areas of virtual water,with a net inflow of 39.766 million m3.Shanxi Province and Shaanxi Province were the areas of virtual water net inflow,and Inner Mongolia,Shandong and Henan were the areas of virtual water netoutflow.China's booming economy has brought great pressure on water resources,and the pressure on water resources in the five provinces and regions in the middle and lower reaches of the Yellow River is also increasing.Improving the comprehensive water use efficiency,adjusting import and export strategies,and implementing the virtual water trade strategy are important measures for high-quality development in the middle and lower reaches of the Yellow River.Key words Near-long distance perspective;Middle and lower reaches of Yellow River;Virtual water trade基金项目国家自然科学基金项目(41461115,41061050)。
tpo53三篇托福阅读TOEFL原文译文题目答案译文背景知识

tpo53三篇托福阅读TOEFL原文译文题目答案译文背景知识阅读-1 (2)原文 (2)译文 (5)题目 (8)答案 (16)背景知识 (18)阅读-2 (21)原文 (21)译文 (24)题目 (27)答案 (34)背景知识 (36)阅读-1原文Evidence of the Earliest Writing①Although literacy appeared independently in several parts of the prehistoric world,the earliest evidence of writing is the cuneiform Sumerian script on the clay tablets of ancient Mesopotamia,which, archaeological detective work has revealed,had its origins in the accounting practices of commercial activity.Researchers demonstrated that preliterate people,to keep track of the goods they produced and exchanged,created a system of accounting using clay tokens as symbolic representations of their products.Over many thousands of years,the symbols evolved through several stages of abstraction until they became wedge-shaped(cuneiform)signs on clay tablets, recognizable as writing.②The original tokens(circa8500B.C.E.)were three-dimensional solid shapes—tiny spheres,cones,disks,and cylinders.A debt of six units of grain and eight head of livestock,for example might have been represented by six conical and eight cylindrical tokens.To keep batches of tokens together,an innovation was introduced(circa3250B.C.E.) whereby they were sealed inside clay envelopes that could be brokenopen and counted when it came time for a debt to be repaid.But because the contents of the envelopes could easily be forgotten, two-dimensional representations of the three-dimensional tokens were impressed into the surface of the envelopes before they were sealed.Eventually,having two sets of equivalent symbols—the internal tokens and external markings—came to seem redundant,so the tokens were eliminated(circa3250-3100B.C.E.),and only solid clay tablets with two-dimensional symbols were retained.Over time,the symbols became more numerous,varied,and abstract and came to represent more than trade commodities,evolving eventually into cuneiform writing.③The evolution of the symbolism is reflected in the archaeological record first of all by the increasing complexity of the tokens themselves. The earliest tokens,dating from about10,000to6,000years ago,were of only the simplest geometric shapes.But about3500B.C.E.,more complex tokens came into common usage,including many naturalistic forms shaped like miniature tools,furniture,fruit,and humans.The earlier,plain tokens were counters for agricultural products,whereas the complex ones stood for finished products,such as bread,oil, perfume,wool,and rope,and for items produced in workshops,such as metal,bracelets,types of cloth,garments,mats,pieces of furniture, tools,and a variety of stone and pottery vessels.The signs marked onclay tablets likewise evolved from simple wedges,circles,ovals,and triangles based on the plain tokens to pictographs derived from the complex tokens.④Before this evidence came to light,the inventors of writing were assumed by researchers to have been an intellectual elite.Some,for example,hypothesized that writing emerged when members of the priestly caste agreed among themselves on written signs.But the association of the plain tokens with the first farmers and of the complex tokens with the first artisans—and the fact that the token-and-envelope accounting system invariably represented only small-scale transactions—testifies to the relatively modest social status of the creators of writing.⑤And not only of literacy,but numeracy(the representation of quantitative concepts)as well.The evidence of the tokens provides further confirmation that mathematics originated in people’s desire to keep records of flocks and other goods.Another immensely significant step occurred around3100 B.C.E.,when Sumerian accountants extended the token-based signs to include the first real numerals. Previously,units of grain had been represented by direct one-to-one correspondence―by repeating the token or symbol for a unit of grain the required number of times.The accountants,however,devisednumeral signs distinct from commodity signs,so that eighteen units of grain could be indicated by preceding a single grain symbol with a symbol denoting“18.”Their invention of abstract numerals and abstract counting was one of the most revolutionary advances in the history of mathematics.⑥What was the social status of the anonymous accountants who produced this breakthrough?The immense volume of clay tablets unearthed in the ruins of the Sumerian temples where the accounts were kept suggests a social differentiation within the scribal class,with a virtual army of lower-ranking tabulators performing the monotonous job of tallying commodities.We can only speculate as to how high or low the inventors of true numerals were in the scribal hierarchy,but it stands to reason that this laborsaving innovation would have been the brainchild of the lower-ranking types whose drudgery is eased.译文最早文字的证据①虽然读写能力是在史前世界的几个地方分别出现的,但书写的最早证据是古代美索不达米亚泥板上的苏美尔楔形文字,根据考古探查工作揭示,它起源于商业活动的会计实践。
小学上册I卷英语第4单元测验卷

小学上册英语第4单元测验卷英语试题一、综合题(本题有100小题,每小题1分,共100分.每小题不选、错误,均不给分)1.What do you call a young female hawk?A. EyasB. NestlingC. ChickD. Cub2.The city of Nuku'alofa is the capital of _______.3.Planting trees is a great way to combat ______ (全球变暖).4.Which one is a type of bird?A. DogB. EagleC. FishD. Cat5.What is the common name for the respiratory organ in humans?A. HeartB. LungsC. LiverD. KidneysB6.What do we call a series of events in a story?A. PlotB. ThemeC. SettingD. CharacterA7.He is a _____ (评论家) who reviews films.8.What is the main source of vitamin D?A. SunlightB. MilkC. MeatD. VegetablesA9.What is the name of the famous artist known for his abstract expressionism?A. Jackson PollockB. Mark RothkoC. Willem de KooningD. Barnett NewmanA10.The __________ (历史的传记) tell individual stories within the larger narrative.11.My ___ (小狗) is very loyal and protective.12.What is the name of the famous American author known for "The Grapes of Wrath"?A. John SteinbeckB. F. Scott FitzgeraldC. Ernest HemingwayD. Mark TwainA13.What do we call the act of taking care of someone?A. CaringB. NurturingC. Looking afterD. All of the AboveD14. A ____ is a small mammal that enjoys digging in the ground.15. A mixture of two or more metals is called _______.16.The _____ (植物知识) can be passed down through generations.17.The author writes _____ (小说) about adventure.18.Do you know my _____ (同学)?19.I enjoy exploring new possibilities with my toy ________ (玩具名称).20. A lion is a type of ______.21. A ____ is a small, colorful bird that sings sweetly.22. A ______ (蜜蜂) works hard to make honey.23.The __________ is the layer of skin that helps to protect against injury.24.I can create a magical adventure with my toy ________ (玩具名称).25.Which animal lives in water?A. CatB. FishC. DogD. BirdB26.What is the term for the study of weather?A. BiologyB. GeologyC. MeteorologyD. AstronomyC27.What is the capital of Saudi Arabia?A. RiyadhB. JeddahC. MeccaD. Medina28.Chemical reactions often involve ______ changes.29.How many bones are in the adult human body?A. 206B. 256C. 306D. 156答案:A30.What is the largest land animal?A. RhinoB. GiraffeC. ElephantD. HippoC31.What is the name of the famous waterfall located on the border of the United States and Canada?A. Niagara FallsB. Victoria FallsC. Angel FallsD. Iguazu FallsA32.Which instrument is a string instrument?A. PianoB. FluteC. GuitarD. TrumpetC33.The _______ can provide food for people and animals.34.What do we call a device used for making calls?A. TelevisionB. ComputerC. PhoneD. RadioC35.I like to eat _____. (pizza/quickly/fast)36.What do you call a young horse?A. CalfB. FoalC. KidD. Puppy37.What is the name of the largest mammal?A. ElephantB. Blue WhaleC. GiraffeD. Hippopotamus38.The capital of Panama is __________.39.What is the time at noon?A. 6 AMB. 12 PMC. 3 PMD. 6 PMB40.The _______ (Vatican City) is the smallest independent state in the world.41.The _______ (小田鼠) scurries quickly through the grass.42.The capital of Dominica is ________ (罗索).43. A chemical reaction can be represented by a ______ equation.44. A compound that can donate protons is called an ______.45.What color is the sky?A. GreenB. BlueC. RedD. YellowB46.Herbs like _____ (罗勒) are used in cooking.47.I want to create a ________ to celebrate love.48. A ______ contains minerals that can be economically valuable.49.What is the name of the famous ancient city in Greece?A. AthensB. MycenaeC. DelphiD. All of the above50.We have a pet ___. (chicken)51.What is the capital of Hungary?A. BudapestB. DebrecenC. SzegedD. MiskolcA52.I enjoy listening to podcasts about __________.53.What do we call the process of studying the ocean?A. OceanographyB. Marine biologyC. Aquatic scienceD. HydrogeologyA Oceanography54.The _______ (The Age of Exploration) led to the discovery of new lands and trade routes.55.I often eat dinner with my ____.56.I think it’s essential to stay _______ (形容词) in tough times. It helps us become stronger.57.What is the smallest unit of life?A. CellB. TissueC. OrganD. OrganismA58._____ (植物的用途) range from food to decoration.59.My sister has a pet ______ (鹦鹉) that talks.60.What is the name of the fairy in Peter Pan?A. TinkerbellB. CinderellaC. AuroraD. Belle61. A ____(sedimentary rock) forms from compressed sediments.62.My dad loves to play ____ (poker) with friends.63.The __________ is a natural area with many trees.64.What do you call the person who repairs cars?A. MechanicB. EngineerC. ArchitectD. DoctorA65.My friend is a great __________ (听众) when I talk.66.The symbol for iodine is _____.67.Owls are known for being ______.68.What do we call the time when the sun rises?A. MorningB. AfternoonC. EveningD. Night69. A ________ (冰岛) is formed by volcanic activity.70.What is the capital of Malawi?A. LilongweB. BlantyreC. MzuzuD. ZombaA71.She has __________ (长长的) hair.72.Metals are generally _______ conductors of electricity.73.Many plants have medicinal properties that can be used for ______ purposes. (许多植物具有药用特性,可以用于治疗。
怎么提高交易能力英语作文

Improving ones trading skills is a multifaceted endeavor that requires a combination of theoretical knowledge,practical experience,and continuous learning.Here are several strategies to enhance your trading capabilities:cation and Training:Start by gaining a solid understanding of the financial markets, trading instruments,and economic principles.This can be achieved through formal education,online courses,or by reading books and articles on trading.2.Technical Analysis:Learn the basics of technical analysis,which involves using charts and various indicators to predict future market movements.Understanding patterns, trends,and price action is crucial for making informed trading decisions.3.Fundamental Analysis:Complement technical analysis with fundamental analysis, which assesses the financial health and performance of companies or economies.This includes analyzing financial statements,economic reports,and news events that can affect market prices.4.Risk Management:Develop a strong risk management strategy to protect your capital. This includes setting stoploss orders,position sizing,and diversifying your portfolio to minimize the impact of any single trade.5.Practice with Demo Accounts:Before risking real money,use demo accounts to practice trading in a simulated environment.This allows you to test strategies and learn from mistakes without financial consequences.6.Stay Informed:Keep up with market news and developments.Subscribe to financial news outlets,follow market analysts,and participate in trading forums to stay current with market trends and sentiment.7.Develop a Trading Plan:Create a detailed trading plan that outlines your entry and exit strategies,risk tolerance,and profit targets.Having a plan helps to maintain discipline and avoid emotional decisionmaking.8.Backtesting:Use historical data to test your trading strategies and refine them based on performance.Backtesting can help you understand how your strategy would have performed in different market conditions.9.Emotional Control:Trading can be stressful,and emotions can lead to poor decisions. Practice mindfulness and develop techniques to manage stress and maintain a clear head during trading sessions.10.Continuous Learning:The financial markets are constantly evolving,and successful traders are always learning.Attend seminars,workshops,and webinars to stay updated on new trading techniques and technologies.working:Connect with other traders and professionals in the working can provide valuable insights,new perspectives,and potential collaboration opportunities.e of Trading Tools and Software:Utilize advanced trading tools and software that can help in analyzing data more efficiently,automating trades,and managing your portfolio effectively.13.Evaluate and Adapt:Regularly evaluate your trading performance and adapt your strategies as needed.Be open to change and willing to refine your approach based on feedback and results.14.Patience and Persistence:Becoming a proficient trader takes time.Be patient with your progress and persistent in your efforts to improve.By implementing these strategies,you can gradually enhance your trading skills and increase your chances of success in the dynamic world of financial markets.。
- 1、下载文档前请自行甄别文档内容的完整性,平台不提供额外的编辑、内容补充、找答案等附加服务。
- 2、"仅部分预览"的文档,不可在线预览部分如存在完整性等问题,可反馈申请退款(可完整预览的文档不适用该条件!)。
- 3、如文档侵犯您的权益,请联系客服反馈,我们会尽快为您处理(人工客服工作时间:9:00-18:30)。
Learning to Trade With Insider InformationSanmay DasDept.of Computer Science and EngineeringUniversity of California,San DiegoLa Jolla,CA92093-0404sanmay@ABSTRACTThis paper introduces algorithms for learning how to trade using insider(superior)information in Kyle’s model offi-nancial markets.Prior results infinance theory relied on the insider having perfect knowledge of the structure and parameters of the market.I show here that it is possible to learn the equilibrium trading strategy when its form is known even without knowledge of the parameters govern-ing trading in the model.However,the rate of convergence to equilibrium is slow,and an approximate algorithm that does not converge to the equilibrium strategy achieves bet-ter utility when the horizon is limited.I analyze this ap-proximate algorithm from the perspective of reinforcement learning and discuss the importance of domain knowledge in designing a successful learning algorithm.Categories and Subject DescriptorsJ.4[Social and Behavioral Sciences]:EconomicsGeneral TermsEconomicsKeywordsComputational Finance,Market Microstructure1.INTRODUCTIONInfinancial markets,information is revealed by trading. Once private information is fully disseminated to the pub-lic,prices reflect all available information and reach market equilibrium.Before prices reach equilibrium,agents with superior information have opportunities to gain profits by trading.This paper focuses on the design of a general algo-rithm that allows an agent to learn how to exploit superior or “insider”information(while the term“insider”information has negative connotations in popular belief.I use the term solely to refer to superior information,however it may be Permission to make digital or hard copies of all or part of this work for personal or classroom use is granted without fee provided that copies are not made or distributed for profit or commercial advantage and that copies bear this notice and the full citation on thefirst page.To copy otherwise,to republish,to post on servers or to redistribute to lists,requires prior specific permission and/or a fee.ICEC’07,August19–22,2007,Minneapolis,Minnesota,USA. Copyright2007ACM978-1-59593-700-1/07/0008...$5.00.obtained–for example,paying for an analyst’s report on a firm can be viewed as a way of obtaining insider information about a stock).Suppose a trading agent receives a signal of what price a stock will trade at n trading periods from now.What is the best way to exploit this information in terms of placing trades in each of the intermediate periods? The agent has to make a tradeoffbetween the profit made from an immediate trade and the amount of information that trade reveals to the market.If the stock is undervalued it makes sense to buy some stock,but buying too much may reveal the insider’s information too early and drive the price up,relatively disadvantaging the insider.This problem has been studied extensively in thefinance literature,initially in the context of a trader with monopo-listic insider information[6],and later in the context of com-peting insiders with homogeneous[4]and heterogeneous[3] information.1All these models derive equilibria under the assumption that traders are perfectly informed about the structure and parameters of the world in which they trade. For example,in Kyle’s model,the informed trader knows two important distributions—the ex ante distribution of the liquidation value and the distribution of other(“noise”) trades that occur in each period.In this paper,I start from Kyle’s original model[6],in which the trading process is structured as a sequential auc-tion at the end of which the stock is liquidated.An informed trader or“insider”is told the liquidation value some num-ber of periods before the liquidation date,and must decide how to allocate trades in each of the intervening periods. There is also some amount of uninformed trading(modeled as white noise)at each period.The clearing price at each auction is set by a market-maker who sees only the combined orderflow(from both the insider and the noise traders)and seeks to set a zero-profit price.In the next section I dis-cuss the importance of this problem from the perspectives of research both infinance and in reinforcement learning. In sections3and4I introduce the market model and two learning algorithms,and in Section5I present experimen-tal results.Finally,Section6concludes and discusses future research directions.1My discussion offinance models in this paper draws di-rectly from these original papers and from the survey by O’Hara[8].2.MOTIV ATION:BOUNDEDRATIONALITY AND REINFORCEMENT LEARNINGOne of the arguments for the standard economic model of a decision-making agent as an unboundedly rational op-timizer is the argument from learning.In a survey of the bounded rationality literature,John Conlisk lists this as the second among eight arguments typically used to make the case for unbounded rationality[2].To paraphrase his description of the argument,it is all right to assume un-bounded rationality because agents learn optima through menting on this argument,Conlisk says“learn-ing is promoted by favorable conditions such as rewards,re-peated opportunities for practice,small deliberation cost at each repetition,good feedback,unchanging circumstances, and a simple context.”The learning process must be an-alyzed in terms of these issues to see if it will indeed lead to agent behavior that is optimal and to see how differences in the environment can affect the learning process.The de-sign of a successful learning algorithm for agents who are not necessarily aware of who else has inside information or what the price formation process is could elucidate the con-ditions that are necessary for agents to arrive at equilibrium, and could potentially lead to characterizations of alternative equilibria in these models.One way of approaching the problem of learning how to trade in the framework developed here is to apply a standard reinforcement learning algorithm with function approxima-tion.Fundamentally,the problem posed here has infinite (continuous)state and action spaces(prices and quantities are treated as real numbers),which pose hard challenges for reinforcement learning algorithms.However,reinforcement learning has worked in various complex domains,perhaps most famously in backgammon[11](see Sutton and Barto for a summary of some of the work on value function ap-proximation[10]).There are two key differences between these successes and the problem studied here that make it difficult for the standard methodology to be successful with-out properly tailoring the learning algorithm to incorporate important domain knowledge.First,successful applications of reinforcement learning with continuous state and action spaces usually require the pres-ence of an offline simulator that can give the algorithm ac-cess to many examples in a costless manner.The environ-ment envisioned here is intrinsically online—the agent in-teracts with the environment by making potentially costly trading decisions which actually affect the payoffit receives. In addition to this,the agent wants to minimize exploration cost because it is an active participant in the economic envi-ronment.Achieving a high utility from early on in the learn-ing process is important to agents in such environments. Second,the sequential nature of the auctions complicates the learning problem.If we were to try and model the pro-cess in terms of a Markov decision problem(MDP),each state would have to be characterized not just by traditional state variables(in this case,for example,last traded price and liquidation value of a stock)but by how many auctions in total there are,and which of these auctions is the cur-rent one.The optimal behavior of a trader at the fourth auction out offive is different from the optimal behavior at the second auction out of ten,or even the ninth auc-tion out of ten.While including the current auction and total number of auctions as part of the state would allow us to represent the problem as an MDP,it would not be particularly helpful because the generalization ability from one state to another would be poor.This problem might be mitigated in circumstances where the optimal behavior does not change much from auction to auction,and characterizing these circumstances is important.In fact,I describe an al-gorithm below that uses a representation where the current auction and the total number of auctions do not factor into the decision.This approach is very similar to model based reinforcement learning with value function approximation, but the main reason why it works very well in this case is that we understand the form of the optimal strategy,so the representations of the value function,state space,and tran-sition model can be tailored so that the algorithm performs close to optimally.I discuss this in more detail in Section5. An alternative approach to the standard reinforcement learning methodology is to use explicit knowledge of the do-main and learn separate functions for each auction.The learning process receives feedback in terms of actual prof-its received for each auction from the current one onwards, so this is a form of direct utility estimation[12].While this approach is related to the direct-reinforcement learning method of Moody and Saffell[7],the problem studied here involves more consideration of delayed rewards,so it is nec-essary to learn something equivalent to a value function in order to optimize the total reward.The important domain facts that help in the development of a learning algorithm are based on Kyle’s results.Kyle proves that in equilibrium,the expected future profits from auction i onwards are a linear function of the square dif-ference between the liquidation value and the last traded price(the actual linear function is different for each i).He also proves that the next traded price is a linear function of the amount traded.These two results are the key to the learning algorithm.I will show in later sections that the algorithm can learn from a small amount of randomized training data and then select the optimal actions according to the trader’s beliefs at every time period.With a small number of auctions,the learning rule enables the trader to converge to the optimal strategy.With a larger number of auctions the number of episodes required to reach the optimal strategy becomes impractical and an approximate mechanism achieves better results.In all cases the trader continues to receive a highflow utility from early episodes onwards.3.MARKET MODELThe model is based on Kyle’s original model[6].There is a single security which is traded in N sequential auc-tions.The liquidation value v of the security is realized af-ter the N th auction,and all holdings are liquidated at that time.v is drawn from a Gaussian distribution with mean p0and varianceΣ0,which are common knowledge.Here we assume that the N auctions are identical and distributed evenly in time.An informed trader or insider observes v in advance and chooses an amount to trade∆x i at each auc-tion i∈{1,...,N}.There is also an uninformed orderflow amount∆u i at each period,sampled from a Gaussian distri-bution with mean0and varianceσ2u∆t i where∆t i=1/N for our purposes(more generally,it represents the time intervalbetween two auctions).2The trading process is mediated by a market-maker who absorbs the order flow while earn-ing zero expected profits.The market-maker only sees the combined order flow ∆x i +∆u i at each auction and sets the clearing price p i .The zero expected profit condition can be expected to arise from competition between market-makers.Equilibrium in the monopolistic insider case is defined by a profit maximization condition on the insider which says that the insider optimizes overall profit given available in-formation,and a market efficiency condition on the (zero-profit)market-maker saying that the market-maker sets the price at each auction to the expected liquidation value of the stock given the combined order flow.Formally,let πi denote the profits made by the insider on positions acquired from the i th auction onwards.Then πi =P N k =i (v −p k )∆x k .Suppose that X is the insider’s trading strategy and is a function of all information avail-able to her,and P is the market-maker’s pricing rule and is again a function of available information.X i is a map-ping from (p 1,p 2,...,p i −1,v )to x i where x i represents the insider’s total holdings after auction i (from which ∆x i can be calculated).P i is a mapping from (x 1+u 1,...,x i +u i )to p i .X and P consist of all the component X i and P i .Kyle defines the sequential auction equilibrium as a pair X and P such that the following two conditions hold:1.Profit maximization :For all i =1,...,N and all X :E [πi (X,P )|p 1,...,p i −1,v ]≥E [πi (X ,P )|p 1,...,p i −1,v ]2.Market efficiency :For all i =1,...,N ,p i =E [v |x 1+u 1,...,x i +u i ]The first condition ensures that the insider’s strategy is optimal,while the second ensures that the market-maker plays the competitive equilibrium (zero-profit)strategy.Kyle also shows that there is a unique linear equilibrium [6].Theorem 1(Kyle,1985).There exists a unique lin-ear (recursive)equilibrium in which there are constantsβn ,λn ,αn ,δn ,Σnsuch that for:∆x n =βn (v −p n −1)∆t n ∆p n =λn (∆x n +∆u n )Σn =var (v |∆x 1+∆u 1,...,∆x n +∆u n )E [πn |p 1,...,p n −1,v ]=αn −1(v −p n −1)2+δn −1Given Σ0the constants βn ,λn ,αn ,δn ,Σn are the unique solution to the difference equation system:αn −1=14λn (1−αn λn )δn −1=δn +αn λ2n σ2u ∆t nβn ∆t n =1−2αn λnn n n λn =βn Σn /σ2uΣn =(1−βn λn ∆t n )Σn −12The motivation for this formulation is to allow the represen-tative uninformed trader’s holdings over time to be a Brow-nian motion with instantaneous variance σ2u .The amount traded represents the change in holdings over the interval.subject to αN =δN =0and the second order condition λn (1−αn λn )=0.3The two facts about the linear equilibrium that will be es-pecially important for learning are that there exist constants λi ,αi ,δi such that:∆p i =λi (∆x i +∆u i )(1)E [πi |p 1,...,p i −1,v ]=αi −1(v −p i −1)2+δi −1(2)Perhaps the most important result of Kyle’s character-ization of equilibrium is that the insider’s information is incorporated into prices gradually,and the optimal action for the informed trader is not to trade particularly aggres-sively at earlier dates,but instead to hold on to some of the information.In the limit as N →∞the rate of reve-lation of information actually becomes constant.Also note that the market-maker imputes a strategy to the informed trader without actually observing her behavior,only the or-der flow.4.A LEARNING MODEL 4.1The Learning ProblemI am interested in examining a scenario in which the in-formed trader knows very little about the structure of the world,but must learn how to trade using the superior in-formation she possesses.I assume that the price-setting market-maker follows the strategy defined by the Kyle equi-librium.This is justifiable because the market-maker (as a specialist in the New York Stock Exchange sense [9])is typically in an institutionally privileged situation with re-spect to the market and has also observed the order-flow over a long period of time.It is reasonable to conclude that the market-maker will have developed a good domain theory over time.The problem faced by the insider is similar to the stan-dard reinforcement learning model [5,1,10]in which an agent does not have complete domain knowledge,but is in-stead placed in an environment in which it must interact by taking actions in order to gain reinforcement.In this model the actions an agent takes are the trades it places,and the reinforcement corresponds to the profits it receives.The informed trader makes no assumptions about the market-maker’s pricing function or the distribution of noise trad-ing,but instead tries to maximize profit over the course of each sequential auction while also learning the appropriate functions.4.2A Learning AlgorithmAt each auction i the goal of the insider is to maximizeπi =∆x i (v −p i )+πi +1(3)The insider must learn both p i and πi +1as functions of the available information.We know that in equilibrium p i is a linear function of p i −1and ∆x i ,while πi +1is a linear function of (v −p i )2.This suggests that an insider could learn a good representation of next price and future profit 3The second order condition rules out a situation in which the insider can make unbounded profits by first destabilizing prices with unprofitable trades.based on these parameters.In this model,the insider tries to learn parameters a1,a2,b1,b2,b3such that:p i=b1p i−1+b2∆x i+b3(4)πi+1=a1(v−p i)2+a2(5) These equations are applicable for all periods except the last,since p N+1is undefined,but we know thatπN+1=0. From this we get:πi=∆x i(v−b1p i−1−b2∆x i−b3)+a1(v−b1p i−1−b2∆x i−b3)2+a2(6) The profit is maximized when the partial derivative with respect to the amount traded is0.Setting∂πi∂(∆x i)=0:∆x i=−v+b1p i−1+b3+2a1b2(v−b1p i−1−b3)122(7)Now consider a repeated sequential auction game where each episode consists of N auctions.Initially the trader trades randomly for a particular number of episodes,gath-ering data as she does so,and then performs a linear re-gression on the stored data to estimate thefive parameters above for each auction.The trader then updates the pa-rameters periodically by considering all the observed data (see Algorithm1for pseudocode).The trader trades op-timally according to her beliefs at each point in time,and any trade provides information on the parameters,since the price change is a noisy linear function of the amount traded. There may be benefits to sometimes not trading optimally in order to learn more.This becomes a problem of both active learning(choosing a good∆x to learn more,and a problem of balancing exploration and exploitation.Data:T:total number of episodes,N:number of auctions, K:number of initialization episodes,D[i][j]:datafrom episode i,auction j,F j:estimated parametersfor auction jfor i=1:K dofor j=1:N doChoose random trading amount,save data in D[i][j]endfor j=1:N doEstimate F j by regressing on D[1][j]...D[K][j] for i=K+1:T dofor j=1:N doChoose trading amount based on F j,save data inD[i][j]if i mod5=0thenfor j=1:N doEstimate F j by regressing on D[1][j]...D[i][j]endendAlgorithm1:The equilibrium learning algorithm 4.3An Approximate AlgorithmAn alternative algorithm would be to use the same pa-rameters for each auction,instead of estimating separate a’s and b’s for each auction(see Algorithm2).Essentially,this algorithm is a learning algorithm which characterizes the state entirely by the last traded price and the liquidation price,irrespective of the particular auction number or even the total number of auctions.The value function of a state is given by the expected profit,which we know from equation 6.We can solve for the optimal action based on our knowl-edge of the system.In the last auction before liquidation, the insider trades knowing that this is the last auction,and does not take future expected profit into account,simply maximizing the expected value of that trade.Stating this more explicitly in terms of standard rein-forcement learning terminology,the insider assumes that the world is characterized by the following.•A continuous state space where the state is v−p,where p is the last traded price.•A continuous action space where actions are given by ∆x,the amount the insider chooses to trade.•A stochastic transition model mapping p and∆x to p (v is assumed constant during an episode).The model is that p is a(noisy)linear function of∆x and p.•A(linear)value function mapping(v−p)2toπ,the expected profit.In addition,the agent knows at the last auction of an episode that the expected future profit from the next stage onwards is0.Of course,the world does not really conform exactly to the agent’s model.One important problem that arises be-cause of this is that the agent does not take into account the difference between the optimal way of trading at differ-ent auctions.The great advantage is that the agent should be able to learn with considerably less data and perhaps do a better job of maximizingfinite-horizon utility.Fur-ther,if the parameters are not very different from auction to auction this algorithm should be able tofind a good ap-proximation of the optimal strategy.Even if the parameters are considerably different for some auctions,if the expected difference between the liquidation value and the last traded price is not high at those auctions,the algorithm might learn a close-to-optimal strategy.The next section discusses the performance of these algorithms,and analyzes the condi-tions for their success.I will refer to thefirst algorithm as the equilibrium learning algorithm and to the second as the approximate learning algorithm in what follows.Data:T:total number of episodes,N:number of auctions, K:number of initialization episodes,D[i][j]:datafrom episode i,auction j,F:estimated parameters for i=1:K dofor j=1:N doChoose random trading amount,save data in D[i][j]endEstimate F by regressing on D[1][]...D[K][]fori=K+1:T dofor j=1:N doChoose trading amount based on F,save data inD[i][j]endif i mod5=0thenEstimate F by regressing on D[1][]...D[i][]endAlgorithm2:The approximate learning algorithm5.EXPERIMENTAL RESULTS5.1Experimental SetupTo determine the behavior of the two learning algorithms, it is important to compare their behavior with the behavior of the optimal strategy under perfect information.In order to elucidate the general properties of these algorithms,this section reports experimental results when there are4auc-tions per episode.For the equilibrium learning algorithmthe insider trades randomly for50episodes,while for the approximate algorithm the insider trades randomly for10 episodes,since it needs less data to form a somewhat rea-sonable initial estimate of the parameters.4In both cases, the amount traded at auction i is randomly sampled from a Gaussian distribution with mean0and variance100/N (where N is the number of auctions per episode).Each simu-lation trial runs for40,000episodes in total,and all reported experiments are averaged over100trials.The actual param-eter values,unless otherwise specified,are p0=75,Σ0= 25,σ2u=25(the units are arbitrary).The market-maker and the optimal insider(used for comparison purposes)are assumed to know these values and solve the Kyle difference equation system tofind out the parameter values they use in making price-setting and trading decisions respectively.5.2Main ResultsFigure1shows the average absolute value of the quantity traded by an insider as a function of the number of episodes that have passed.The graphs show that a learning agent us-ing the equilibrium learning algorithm appears to be slowly converging to the equilibrium strategy in the game with four auctions per episode,while the approximate learning algo-rithm converges quickly to a strategy that is not the optimal strategy.Figure2shows two important facts.First,the graph on the left shows that the average profit made rises much more sharply for the approximate algorithm,which makes better use of available data.Second,the graph on the right shows that the average total utility being received is higher from episode20,000onwards for the equilibrium learner(all differences between the algorithms in this graph are statistically significant at a95%level).Were the sim-ulations to run long enough,the equilibrium learner would outperform the approximate learner in terms of total utility received,but this would require a huge number of episodes per trial.Clearly,there is a tradeoffbetween achieving a higherflow utility and learning a representation that allows the agent to trade optimally in the limit.This problem is exacerbated as the number of auctions increases.With10auctions per episode,an agent using the equilibrium learning algorithm actually does not learn to trade more heavily in auction 10than she did in early episodes even after40,000total episodes,leading to a comparatively poor average profit over the course of the simulation.This is due to the dynamics of learning in this setting.The opportunity to make profits by trading heavily in the last auction are highly dependent on not having traded heavily earlier,and so an agent cannot learn a policy that allows her to trade heavily at the last auction until she learns to trade less heavily earlier.This takes more time when there are more auctions.It is also 4This setting does not affect the long term outcome signif-icantly unless the agent starts offwith terrible initial esti-mates.x 104EpisodeAbsotulevalueofquantitytradedx 104EpisodeAbsolutevalueofquantitytradedFigure1:Average absolute value of quantities traded at each auction by a trader using the equilib-rium learning algorithm(above)and a trader using the approximate learning algorithm(below)as the number of episodes increases.The thick lines par-allel to the X axis represent the average absolute value of the quantity that an optimal insider with full information would trade.x 104EpisodeP r o f i tx 104EpisodeA v g p r o f i t o v e r r e m a i n i n g l e n g t h o f s i m u l a t i o nFigure 2:Above:Average flow profit recieved by traders using the two learning algorithms (each point is an aggregate of 50episodes over all 100tri-als)as the number of episodes increases.Below:Average profit received until the end of the simu-lation measured as a function of the episode from which we start measuring (for episodes 100,10,000,20,000and 30,000).worth noting that assuming that agents have a large amount of time to learn in real markets is unrealistic.The graphs in Figures 1and 2reveal some interesting dy-namics of the learning process.First,with the equilibrium learning algorithm,the average profit made by the agent slowly increases in a fairly smooth manner with the number of episodes,showing that the agent’s policy is constantly im-proving as she learns more.An agent using the approximate learning algorithm shows much quicker learning,but learns a policy that is not asymptotically optimal.The second in-teresting point is about the dynamics of trader behavior —under both algorithms,an insider initially trades far more heavily in the first period than would be considered optimal,but slowly learns to hide her information like an optimal trader would.For the equilibrium learning algorithm,there is a spike in the amount traded in the second period early on in the learning process.This is also a small spike in the amount traded in the third period before the agent starts converging to the optimal strategy.5.3Analysis of the Approximate AlgorithmThe behavior of the trader using the approximate algo-rithm is interesting in a variety of ways.First,let us con-sider the pattern of trades in Figure 1.As mentioned above,the trader trades more aggressively in period 1than in pe-riod 2,and more aggressively in period 2than in period 3.Let us analyze why this is the case.The agent is learning a strategy that makes the same decisions independent of the particular auction number (except for the last auction).At any auction other than the last,the agent is trying to choose ∆x to maximize:∆x (v −p )+W [S v,p ]where p is the next price (also a function of ∆x ,and also taken to be independent of the particular auction)and W [S v,p ]is the value of being in the state characterized by the liqui-dation value v and (last)price p .The agent also believes that the price p is a linear function of p and ∆x .There are two possibilities for the kind of behavior the agent might exhibit,given that she knows that her action will move the stock price in the direction of her trade (if she buys,the price will go up,and if she sells the price will go down).She could try to trade against her signal,because the model she has learned suggests that the potential for future profit gained by pushing the price away from the direction of the true liquidation value is higher than the loss from the one trade.5The other possibility is that she trades with her sig-nal.In this case,the similarity of auctions in the represen-tation ensures that she trades with an intensity proportional to her signal.Since she is trading in the correct direction,the price will move (in expectation)towards the liquidation value with each trade,and the average amount traded will go down with each successive auction.The difference in the last period,of course,is that the trader is solely trying to maximize ∆x (v −p )because she knows that it is her last opportunity to trade.The success of the algorithm when there are as few as four auctions demonstrates that learning an approximate 5This is not really learnable using linear representations for everything unless there is a different function that takes over at some point (such as the last auction),because otherwise the trader would keep trading in the wrong direction and never receive positive reinforcement.。