Deep Learning for Human Part Discovery in Images
我在学习中获得的乐趣英语作文
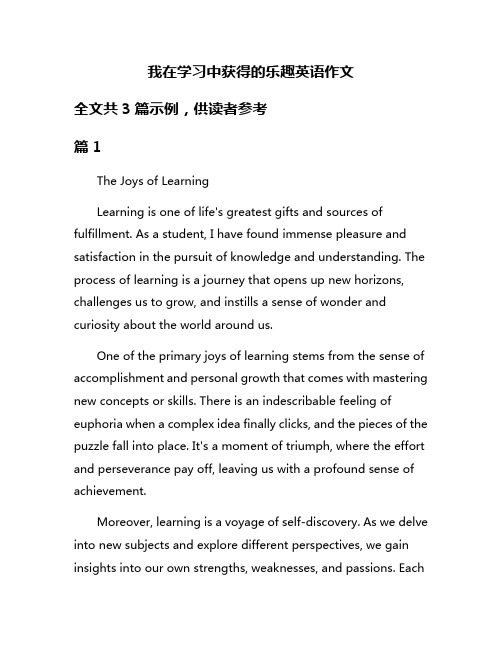
我在学习中获得的乐趣英语作文全文共3篇示例,供读者参考篇1The Joys of LearningLearning is one of life's greatest gifts and sources of fulfillment. As a student, I have found immense pleasure and satisfaction in the pursuit of knowledge and understanding. The process of learning is a journey that opens up new horizons, challenges us to grow, and instills a sense of wonder and curiosity about the world around us.One of the primary joys of learning stems from the sense of accomplishment and personal growth that comes with mastering new concepts or skills. There is an indescribable feeling of euphoria when a complex idea finally clicks, and the pieces of the puzzle fall into place. It's a moment of triumph, where the effort and perseverance pay off, leaving us with a profound sense of achievement.Moreover, learning is a voyage of self-discovery. As we delve into new subjects and explore different perspectives, we gain insights into our own strengths, weaknesses, and passions. Eachnew topic we encounter has the potential to unlock hidden talents or interests that we never knew existed. This process of self-exploration is not only intellectually stimulating but also deeply gratifying, as it allows us to better understand ourselves and our place in the world.Another aspect of learning that brings me immense joy is the sense of wonder and curiosity it cultivates. The more we learn, the more we realize how vast and intricate the universe is, and how much there is yet to discover. Each new piece of knowledge opens up a multitude of new questions and avenues for exploration. This constant sense of awe and curiosity keeps our minds engaged and our spirits invigorated, propelling us forward on a never-ending quest for understanding.Learning also fosters a deep appreciation for the interconnectedness of all things. As we study diverse subjects and draw connections between seemingly unrelated fields, we begin to see the world as a vast tapestry, where everything is intertwined and interdependent. This holistic perspective not only enriches our understanding but also instills a profound respect for the complexity and beauty of the natural world and human endeavors.Beyond the intellectual rewards, learning also brings social and emotional fulfillment. Engaging in discussions, collaborating with peers, and sharing ideas fosters a sense of community and belonging. These interactions not only enhance our understanding but also nurture valuable interpersonal skills, such as communication, empathy, and teamwork, which are essential for personal and professional growth.Furthermore, learning empowers us to make a positive impact on the world around us. Knowledge equips us with the tools and understanding necessary to tackle global challenges, drive innovation, and create positive change. Whether it's addressing environmental concerns, advancing medical treatments, or developing new technologies, learning is the catalyst that fuels progress and enables us to contribute to the betterment of society.Of course, the journey of learning is not without its challenges. There will be moments of frustration, setbacks, and seemingly insurmountable obstacles. However, it is precisely these challenges that make the rewards of learning all the more sweet. Persevering through difficulties and overcoming barriers instills a sense of resilience and determination that extends far beyond the classroom or lecture hall.In conclusion, the joys of learning are manifold and profound. It is a lifelong endeavor that enriches our minds, ignites our curiosity, fosters personal growth, and equips us to make a meaningful difference in the world. As a student, I cherish every opportunity to expand my horizons and embrace the boundless possibilities that learning offers. The pursuit of knowledge is not merely an academic exercise but a profound journey of self-discovery, wonder, and fulfillment.篇2The Joy I Find in LearningLearning has always been a source of immense joy and fulfillment for me. From the moment I first stepped into a classroom as a wide-eyed child, I was captivated by the world of knowledge that lay before me, waiting to be explored. Each new subject, each new concept, was like a hidden treasure waiting to be unearthed, and the process of discovery filled me with an indescribable sense of excitement and wonder.As I progressed through my academic journey, my love for learning only deepened. I found myself drawn to the intricacies of various subjects, marveling at the intricate tapestry of ideas and theories that wove together to form a comprehensiveunderstanding of the world around us. Whether it was delving into the complexities of literature, unraveling the mysteries of science, or grappling with the nuances of history, each new area of study presented its own unique challenges and rewards.One of the greatest joys of learning, for me, lies in the process of intellectual growth. With every new concept mastered, every new skill acquired, I feel a sense of personal development and transformation. It is as if my mind is being stretched and expanded, allowing me to perceive the world from ever-evolving perspectives. This constant evolution, this perpetual journey of self-improvement, is what fuels my passion for learning and drives me to continually seek out new knowledge.Beyond the personal growth, however, lies another layer of joy – the joy of understanding. There is a profound satisfaction in finally grasping a complex theory or unraveling the intricacies of a challenging problem. It is akin to solving a intricate puzzle, where each piece falls into place, revealing a complete and coherent picture. The sense of accomplishment that comes with comprehending something that once seemed impenetrable is truly exhilarating.Moreover, learning has taught me the invaluable art of critical thinking. It has instilled in me the ability to question, toanalyze, and to approach issues from multiple angles. This skill has not only enriched my academic pursuits but has also proven invaluable in navigating the complexities of life itself. By learning to think critically, I have developed a deeper appreciation for the nuances and intricacies that underlie even the most seemingly mundane aspects of our existence.Perhaps one of the greatest joys of learning, however, lies in the connections it fosters – connections between ideas, between disciplines, and ultimately, between people. As I delve deeper into various subjects, I am constantly amazed by the intricate web of relationships that bind them together. A concept from one field can shed light on another, creating a rich tapestry of interconnected knowledge. This realization has opened my eyes to the inherent unity and interdependence of all learning, and has fostered a deep respect for the collective wisdom of humanity.Furthermore, learning has enabled me to connect with individuals from diverse backgrounds and perspectives. Through the shared pursuit of knowledge, I have forged lasting bonds with fellow students, professors, and mentors, each offering their unique insights and experiences. These connections have not only enriched my understanding of the subject matter but havealso broadened my horizons, allowing me to see the world through a multitude of lenses.In a world that often prioritizes tangible achievements and material success, the joy of learning may seem like a luxury to some. Yet, for me, it is a necessity – a wellspring of personal growth, intellectual stimulation, and profound fulfillment. It is a journey that never ends, a path that continually unveils new wonders and new challenges, beckoning me ever forward.As I look ahead to the future, I am filled with excitement at the prospect of continued learning. The boundless expanse of knowledge that lies before me is both humbling and exhilarating, reminding me that there is always more to discover, more to understand, and more joy to be found in the pursuit of wisdom. It is a journey that I embrace wholeheartedly, for it is in the act of learning that I find my truest sense of purpose and fulfillment.篇3The Joys of Being a StudentLearning has been one of the greatest joys of my life so far. As a student, every day brings new opportunities to explore ideas, gain knowledge, and expand my understanding of theworld around me. The process of learning itself is an incredible journey filled with excitement, challenges, and personal growth.One of the things I love most about being a student is the sense of discovery that comes with each new topic or subject I delve into. It's like embarking on a grand adventure, where every lesson, every book, and every discussion holds the promise of uncovering something new and fascinating. The anticipation of what I might learn next is thrilling, and the act of absorbing that knowledge is deeply satisfying.I find immense pleasure in the process of grappling with complex concepts and tackling intellectual challenges. There's a certain rush that comes when I finally grasp a difficult idea after hours of effort and perseverance. It's a feeling of triumph, like scaling a mental mountain and being rewarded with a breathtaking view from the summit. These moments of understanding are truly exhilarating, and they motivate me to keep pushing forward, eager for the next challenge.Learning is not just about acquiring knowledge; it's also about developing critical thinking skills and fostering intellectual curiosity. As a student, I've learned to question assumptions, analyze information from multiple perspectives, and formulate my own opinions and ideas. This process of questioning andexploration has opened my mind to new ways of thinking and has helped me become a more well-rounded and adaptable individual.One of the greatest joys of being a student is the opportunity to engage in lively discussions and debates with my peers and professors. These intellectual exchanges are like sparks that ignite the fire of learning, as we challenge each other's viewpoints, share insights, and collectively unravel the complexities of the topics at hand. The energy and passion that emanate from these discussions are truly invigorating, and they often inspire me to delve deeper into the subject matter.Beyond the intellectual stimulation, being a student has also taught me valuable life lessons about perseverance, time management, and the importance of developing a growth mindset. Juggling multiple classes, assignments, and extracurricular activities has honed my ability to prioritize and stay focused, even in the face of daunting workloads. Moreover, the occasional setbacks and failures I've encountered have taught me the value of resilience and the importance of viewing mistakes as opportunities for growth rather than sources of discouragement.Perhaps one of the greatest joys of being a student is the sense of personal growth and transformation that occurs along the way. With each new subject mastered, each skill acquired, and each challenge overcome, I can feel myself evolving into a more knowledgeable, capable, and well-rounded individual. It's a deeply rewarding experience to look back on my academic journey and see how far I've come, both intellectually and personally.Of course, being a student is not without its challenges. There are times when the workload feels overwhelming, and the pressure to perform can be daunting. However, even in these moments of stress and struggle, I find solace in the knowledge that I am engaged in a noble pursuit – the pursuit of knowledge and understanding. The joys of learning far outweigh the occasional difficulties, and the rewards are truly profound.As I look towards the future, I am filled with excitement and anticipation for the countless learning opportunities that lie ahead. Whether it's delving into new fields of study, acquiring new skills, or exploring uncharted realms of knowledge, the prospect of lifelong learning is a source of immense joy and fulfillment.In the end, being a student is not just about obtaining a degree or securing a career path; it's about embracing the journey of intellectual growth, personal development, and the insatiable thirst for knowledge that defines the human experience. The joys of learning are invaluable gifts that will enrich my life in countless ways, and I am grateful for the opportunity to be a student and to embark on this incredible adventure of discovery.。
李开复TED英文演讲:AI和人类将来会如何共处

李开复TED英文演讲:AI和人类将来会如何共处李开复认为,在这场人工智能摧毁工作的浩劫中,唯有创造性工作才能从中全身而退。
人类将面临的最大考验并非是失去工作,而是失去生活的意义。
下面是小编为大家收集关于李开复TED英文演讲:AI和人类将来会如何共处,欢迎借鉴参考。
李开复TED演讲稿双语版I'm going to talk about how AI and mankind can coexist, but first, we have to rethink about our human values. So let me first make a confession about my errors in my values.我将会谈谈人工智能和人类如何能够共存,但首先,我们需要重新思考人文价值。
所以首先让我承认我价值观中的错误。
It was 11 o'clock, December 16, 1991. I was about to become a father for the first time. My wife, Shen-Ling, lay in the hospital bed going through a very difficult 12-hour labor. I sat by her bedside but looked anxiously at my watch, and I knew something that she didn't. I knew that if in one hour, our child didn't come, I was going to leave her there and go back to work and make a presentation about AI to my boss, Apple's CEO. Fortunately, my daughter was born at 11:30 --那时是1991年12月16日的11时。
探索深海也是探索我们自身的生存英文作文

探索深海也是探索我们自身的生存英文作文Exploring the Depths: A Journey of Self-DiscoveryThe vast expanse of the ocean has long captivated the human imagination, beckoning us to venture beyond the familiar shores and uncover the mysteries that lie beneath the surface. For centuries, the depths of the sea have been a source of both fascination and trepidation, a realm where the limits of human exploration are constantly pushed and the very nature of our existence is called into question.As we delve deeper into the unknown, we find ourselves not only exploring the physical boundaries of our world but also the depths of our own psyche. The journey to the seafloor is not merely a physical undertaking but a metaphorical one, a quest to understand the fundamental aspects of our being and our place in the grand scheme of the natural world.The allure of the deep sea lies in its sheer vastness and the promise of discovery. With each expedition, we uncover new species, unravel the complex web of marine ecosystems, and gain a deeper appreciation for the delicate balance that sustains life on our planet.Yet, as we delve deeper, we also confront the harsh realities of the deep, the unforgiving currents, the crushing pressures, and the enigmatic creatures that inhabit this otherworldly realm.It is in these moments of confrontation with the unknown that we are forced to confront our own limitations and vulnerabilities. The deep sea, with its seemingly endless expanse and its unyielding forces, serves as a mirror that reflects the fragility of the human condition. We are reminded of our own insignificance in the grand tapestry of the natural world, and yet, it is in this realization that we find the potential for profound self-discovery.As we explore the depths, we are compelled to grapple with existential questions that have plagued humanity for millennia. What is the purpose of our existence? How do we define our place in the universe? What is the true nature of our being? These questions, once confined to the realms of philosophy and spirituality, now find resonance in the tangible world of deep-sea exploration.The journey to the seafloor is not merely a physical one but a metaphorical one, a quest to uncover the hidden truths that lie within us. Just as the deep sea holds the secrets of our planet's past and the potential for its future, so too does it hold the key to unlocking the mysteries of our own existence.Through the act of exploration, we come to understand that the boundaries between the external and the internal world are not as rigid as we once believed. The challenges and obstacles we face in the depths of the ocean mirror the challenges and obstacles we face in our own lives, and it is in overcoming these challenges that we discover the true strength and resilience of the human spirit.As we delve deeper into the unknown, we find that the true purpose of our exploration is not simply to uncover the secrets of the deep, but to uncover the secrets of ourselves. It is in this process of self-discovery that we find the true meaning of our existence, the connection between the macro and the micro, the universal and the personal.In the end, the journey to the seafloor is not just a physical one, but a spiritual one as well. It is a journey of self-discovery, a journey that challenges us to confront our fears, our limitations, and our deepest held beliefs about the nature of our existence. And it is in this process of exploration and self-discovery that we find the true essence of what it means to be human.。
让人工智能呼唤人类智慧英语作文800

让人工智能呼唤人类智慧英语作文800全文共3篇示例,供读者参考篇1Let Artificial Intelligence Call Upon Human IntelligenceAs an inquisitive student fascinated by the incredible advances in artificial intelligence (AI) technology, I can't help but ponder the profound implications it holds for our world. While AI's potential seems boundless, from revolutionizing industries to tackling global challenges, I believe it is crucial that we approach this powerful tool with wisdom and a deep respect for human intelligence.At its core, AI is a remarkable feat of human ingenuity and creativity. The intricate algorithms, the vast neural networks, and the sophisticated machine learning models that power AI systems are all the product of brilliant minds. It is a testament to the boundless potential of the human intellect that we have been able to create such awe-inspiring technologies.However, as AI continues to evolve and become more capable, there is a risk of becoming overly reliant on it, to the detriment of our own cognitive abilities. We must be vigilantagainst the temptation to outsource our thinking entirely to these systems, lest we lose touch with the very qualities that make us human – our capacity for original thought, creative expression, and moral reasoning.Rather than viewing AI as a replacement for human intelligence, we should strive to create a symbiotic relationship, where the two work in harmonious cooperation. AI can augment and enhance our cognitive capabilities, taking on the arduous and repetitive tasks that bog us down, freeing us to focus on the more nuanced, abstract, and imaginative aspects ofproblem-solving.Imagine a world where AI acts as a tireless research assistant, rapidly sifting through vast troves of data and presenting us with concise, relevant information. This would empower human experts to spend more time synthesizing insights, formulating novel ideas, and pushing the boundaries of knowledge in their respective fields.Furthermore, AI could be a powerful tool for democratizing education and fostering a more inclusive, equitable society. By leveraging adaptive learning algorithms and personalized tutoring systems, we could tailor educational experiences toindividual needs and learning styles, ensuring that no student is left behind.Yet, as we embrace the potential of AI, we must also grapple with the ethical and societal implications of this technology. Questions of bias, privacy, and accountability loom large, and it is our responsibility as the architects of AI to imbue these systems with the core values that underpin a just and equitable society.We must also confront the potential impact of AI on the job market and prepare for the inevitable disruption it will cause. While some jobs may become obsolete, new opportunities will undoubtedly emerge, and it is incumbent upon us to equip the workforce with the skills and adaptability to thrive in this rapidly evolving landscape.Ultimately, the true power of AI lies not in its ability to replicate human intelligence, but in its capacity to amplify and elevate it. By harnessing the strengths of both AI and human cognition, we can tackle challenges that once seemed insurmountable, drive innovation to unprecedented heights, and unlock new realms of understanding and discovery.As we stand on the precipice of this AI-driven revolution, let us remember that it is our human intelligence – our curiosity, ourcreativity, and our moral compass – that must guide the development and application of these powerful technologies. Only by striking the right balance, by leveraging the complementary strengths of AI and human intelligence, can we truly unleash the full potential of this transformative era.So let us not cede our agency to the machines, but rather forge a path where we work in concert with AI, amplifying each other's capabilities and shaping a future that is not only technologically advanced but also deeply human.篇2Letting Artificial Intelligence Call Upon Human IngenuityAs a student in the 21st century, I can't help but be in awe of the rapid advancements in artificial intelligence (AI) technology. The capabilities of AI systems are increasing at an exponential rate, with each new breakthrough leaving us wondering what will be possible next. However, as much as I am fascinated by the potential of AI, I also recognize the importance of preserving and nurturing human ingenuity and creativity.AI is a powerful tool, but it is a tool nonetheless – a creation of human intelligence and innovation. While AI can process vast amounts of data, identify patterns, and make predictions withremarkable accuracy, it is ultimately limited by the algorithms and data it is trained on. AI systems, at their core, are designed to optimize for specific tasks and objectives, but they lack the truly creative and imaginative spark that is unique to the human mind.It is human ingenuity that has driven the world's greatest discoveries, inventions, and artistic achievements throughout history. From the theory of relativity to the works of Shakespeare, these breakthroughs and masterpieces were not the result of cold, calculated algorithms, but rather the product of human curiosity, intuition, and the ability to think outside the proverbial box.As powerful as AI is, it cannot replicate the depth of human experience, emotion, and personal perspective that shapes our thoughts, ideas, and creative expressions. AI may be able to compose music or generate artwork based on its training data, but it cannot capture the raw human emotion and personal narratives that truly resonate with us and move us in profound ways.Furthermore, while AI excels at optimizing for specific tasks, it is human ingenuity that often identifies the tasks and problems that need solving in the first place. It is our unique ability toquestion assumptions, challenge existing paradigms, and envision new possibilities that drives innovation and progress.This is not to say that AI and human ingenuity are mutually exclusive – quite the contrary. When used effectively, AI can be a powerful augmentation to human intelligence, allowing us to process and analyze data at unprecedented scales, automate repetitive tasks, and uncover insights that may have been overlooked by human analysts alone.However, it is crucial that we strike the right balance and ensure that AI remains a tool to empower and amplify human ingenuity, rather than replace it entirely. We must resist the temptation to become overly reliant on AI and cede too much control and decision-making to these systems, lest we risk stifling the very human creativity and ingenuity that gave rise to AI in the first place.As students and future leaders, it is our responsibility to develop a deep understanding of AI, its capabilities, and its limitations. We must learn to harness the power of AI while simultaneously cultivating our own critical thinking,problem-solving, and creative skills.Education systems should place a renewed emphasis on nurturing these human-centric skills, encouraging students toembrace their curiosity, engage in interdisciplinary thinking, and develop a lifelong love for learning and exploration. Only by doing so can we ensure that AI remains a tool to augment and elevate human ingenuity, rather than supplant it.In the end, the true potential of AI lies not in its ability to replicate human intelligence, but in its capacity to complement and amplify it. By letting AI call upon human ingenuity, we can unlock new frontiers of innovation, creativity, and understanding – ushering in a future where the symbiosis of human and artificial intelligence leads to breakthroughs and achievements that were once thought impossible.篇3Let AI Call for Human IntelligenceAs an avid student of science and technology, I can't help but be in awe of the rapid advancements in artificial intelligence (AI) that we're witnessing today. From self-driving cars to virtual assistants like Siri and Alexa, AI is infiltrating every aspect of our lives, making tasks more efficient and convenient than ever before. However, as we become increasingly reliant on these intelligent machines, it's crucial that we don't lose sight of thevery thing that makes us human – our intelligence, creativity, and ability to reason.Don't get me wrong; I'm not an AI skeptic or a Luddite. I firmly believe that AI has the potential to revolutionize our world in ways we can't even fathom. But as we embrace this technological marvel, we must also recognize its limitations and the importance of human intelligence in shaping its development and application.One of the most significant limitations of AI is that it lacks the ability to truly understand context and nuance. While AI systems can process vast amounts of data and identify patterns, they lack the emotional intelligence and intuition that humans possess. This becomes particularly evident in fields like healthcare, education, and customer service, where empathy and a deep understanding of human emotions are paramount.Moreover, AI systems are only as good as the data they're trained on. If the training data is biased or incomplete, the AI's decisions and outputs will be skewed, perpetuating societal biases and injustices. This is where human intelligence comes into play – we have the ability to scrutinize the data, identify biases, and ensure that AI systems are trained on diverse and representative datasets.Another area where human intelligence is indispensable is in the realm of ethics and moral decision-making. As AI systems become more sophisticated and autonomous, they will inevitably face ethical dilemmas that require nuanced reasoning and a deep understanding of human values. While we can program AI with ethical frameworks, it's ultimately up to humans to define and refine these frameworks, ensuring that AI systems align with our moral principles and serve the greater good.But perhaps the most compelling argument for the importance of human intelligence in the age of AI lies in our ability to think creatively and innovatively. AI excels at pattern recognition and optimization, but true creativity and innovation often arise from unexpected connections, intuitive leaps, and the ability to challenge conventional wisdom. It's this human ingenuity that has driven scientific breakthroughs, artistic masterpieces, and technological revolutions throughout history.As a student, I'm constantly reminded of the power of human intelligence in the classroom. While AI can assist in grading assignments and providing personalized learning experiences, it can never replace the invaluable role of teachers in fostering critical thinking, nurturing curiosity, and inspiring students to push the boundaries of knowledge.In conclusion, as we navigate the AI revolution, it's essential that we don't lose sight of the very thing that makes us human –our intelligence, creativity, and ability to reason. AI should be a tool that augments and enhances human capabilities, not a replacement for them. By embracing the symbiotic relationship between human and artificial intelligence, we can unlock new frontiers of knowledge, innovation, and progress, while ensuring that our moral compass and ethical principles remain firmly rooted in human values.So, let's not fear AI; let's embrace it, but let's also celebrate and nurture the unique human qualities that have brought us this far. Let AI call for human intelligence, for it is in this harmonious dance between machine and mind that we will shape a future that is not only technologically advanced but also deeply human.。
发现与探索的英文简写
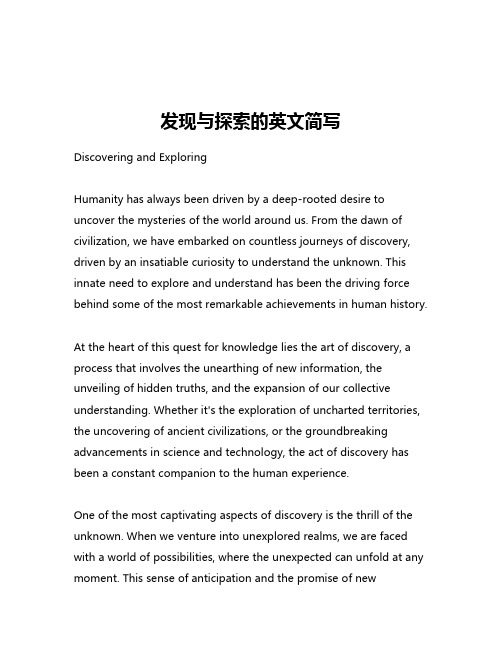
发现与探索的英文简写Discovering and ExploringHumanity has always been driven by a deep-rooted desire to uncover the mysteries of the world around us. From the dawn of civilization, we have embarked on countless journeys of discovery, driven by an insatiable curiosity to understand the unknown. This innate need to explore and understand has been the driving force behind some of the most remarkable achievements in human history.At the heart of this quest for knowledge lies the art of discovery, a process that involves the unearthing of new information, the unveiling of hidden truths, and the expansion of our collective understanding. Whether it's the exploration of uncharted territories, the uncovering of ancient civilizations, or the groundbreaking advancements in science and technology, the act of discovery has been a constant companion to the human experience.One of the most captivating aspects of discovery is the thrill of the unknown. When we venture into unexplored realms, we are faced with a world of possibilities, where the unexpected can unfold at any moment. This sense of anticipation and the promise of newrevelations fuel our adventurous spirits, driving us to push the boundaries of our knowledge and capabilities.In the realm of geographical exploration, the quest for new lands and the mapping of uncharted territories has been a hallmark of human exploration. From the voyages of Christopher Columbus to the expeditions of Sir Ernest Shackleton, the desire to chart the unknown has led to the expansion of our understanding of the physical world. These explorers, driven by a combination of curiosity, ambition, and a thirst for adventure, have left an indelible mark on the course of human history.Similarly, the field of scientific discovery has been a testament to the power of human ingenuity and the relentless pursuit of knowledge. From the groundbreaking theories of Isaac Newton to the revolutionary discoveries of Marie Curie, the scientific community has continually pushed the boundaries of our understanding of the natural world. Through rigorous experimentation, meticulous observation, and the formulation of innovative hypotheses, scientists have uncovered the hidden workings of the universe, shedding light on the mysteries that have captivated humanity for centuries.The art of discovery, however, is not limited to the realms of geography and science. It extends to the exploration of the human experience itself, as we delve into the depths of our ownconsciousness, unraveling the complexities of the mind and the intricacies of the human condition. Psychologists, anthropologists, and philosophers have dedicated their lives to understanding the human experience, seeking to unlock the secrets of our behavior, our beliefs, and our cultural traditions.In the realm of the arts, the act of discovery takes on a different form, as artists and creators explore the boundless realms of human expression. From the masterful brushstrokes of the world's greatest painters to the captivating melodies of renowned composers, the artistic discovery is a testament to the boundless creativity of the human spirit. Through their works, artists invite us to see the world through new perspectives, challenging our preconceptions and expanding the horizons of our understanding.Ultimately, the pursuit of discovery and exploration is a fundamental aspect of the human experience. It is the driving force behind our most remarkable achievements, fueling our curiosity, challenging our assumptions, and propelling us forward into the unknown. Whether it's the exploration of the physical world, the unveiling of scientific truths, or the expansion of our understanding of the human experience, the act of discovery is a testament to the boundless potential of the human mind and the indomitable spirit of human exploration.。
我将来做一名科学家英语作文

我将来做一名科学家英语作文As I gaze into the vast expanse of the universe, my heart is filled with an insatiable curiosity to unravel its mysteries. The pursuit of scientific knowledge has captivated my mind since childhood, and the idea of becoming a scientist has become a steadfast dream that guides my every step. With a deep fascination for the natural world and a relentless drive to push the boundaries of human understanding, I am determined to dedicate my life to the pursuit of scientific discovery.From a young age, I have been captivated by the wonders of science. Whether it was observing the intricate patterns of snowflakes, exploring the depths of the ocean, or marveling at the complexity of the human body, each new discovery has ignited a flame within me that burns brighter with every passing day. The ability of science to shed light on the fundamental workings of our universe, to unlock the secrets of the natural world, and to improve the human condition has always been a source of profound inspiration.As I have grown older, my fascination with science has onlydeepened, and I have become increasingly driven to contribute to the advancement of human knowledge. The opportunity to engage in cutting-edge research, to push the boundaries of what is known, and to make a tangible impact on the world is a prospect that fills me with a sense of purpose and excitement. Whether it is delving into the mysteries of the cosmos, unraveling the complexities of the human mind, or developing innovative solutions to pressing global challenges, the prospect of being at the forefront of scientific discovery is a dream that I am determined to pursue.Moreover, the multifaceted nature of scientific research appeals to me greatly. The ability to combine rigorous theoretical work with hands-on experimentation, to collaborate with a diverse team of researchers from various disciplines, and to constantly adapt and evolve one's approach in the face of new challenges is a thrilling prospect that aligns perfectly with my own intellectual and creative inclinations. I relish the opportunity to engage in critical thinking, to question established paradigms, and to explore uncharted territories of knowledge.Beyond the intellectual stimulation, the prospect of using scientific knowledge to improve the human condition is a driving force behind my desire to become a scientist. The potential to develop life-saving medical treatments, to devise sustainable energy solutions, or to uncover the secrets of our past and shape our collective future fillsme with a deep sense of purpose and responsibility. I am driven by the belief that science has the power to transform the world and to create a better future for all of humanity.As I envision my future as a scientist, I am filled with a sense of excitement and determination. I can see myself immersed in the laboratory, meticulously designing and executing experiments, analyzing data, and collaborating with fellow researchers to push the boundaries of human knowledge. I can imagine myself presenting my findings at conferences, engaging in lively discussions with peers, and contributing to the ongoing dialogue that shapes the direction of scientific progress.Moreover, I am eager to engage in the broader societal impact of science, using my expertise to inform policymakers, educate the public, and inspire the next generation of scientific thinkers. I believe that scientists have a responsibility to be advocates for science, to communicate the importance of their work, and to foster a greater understanding and appreciation of the role of science in our lives.In pursuit of this dream, I have already begun to lay the groundwork for my future career as a scientist. I have immersed myself in rigorous coursework, honing my analytical skills and deepening my understanding of the fundamental principles of science. I have also sought out opportunities to engage in hands-on research,participating in internships and research projects that have allowed me to apply my knowledge in practical settings.As I look ahead to the challenges and opportunities that lie ahead, I am filled with a sense of unwavering determination. I know that the path to becoming a successful scientist will be filled with obstacles, but I am prepared to meet them head-on. I am committed to continually expanding my knowledge, refining my research skills, and pushing the boundaries of what is possible.Ultimately, my dream of becoming a scientist is not just a personal aspiration, but a reflection of my deep-seated belief in the transformative power of science. I am driven by the conviction that through the relentless pursuit of knowledge and the application of scientific principles, we can unlock the secrets of our universe, improve the human condition, and create a better future for all. It is with this sense of purpose and passion that I look forward to embarking on my journey as a scientist, ready to contribute to the ongoing quest for understanding and progress.。
深海探索是否需要人英语作文
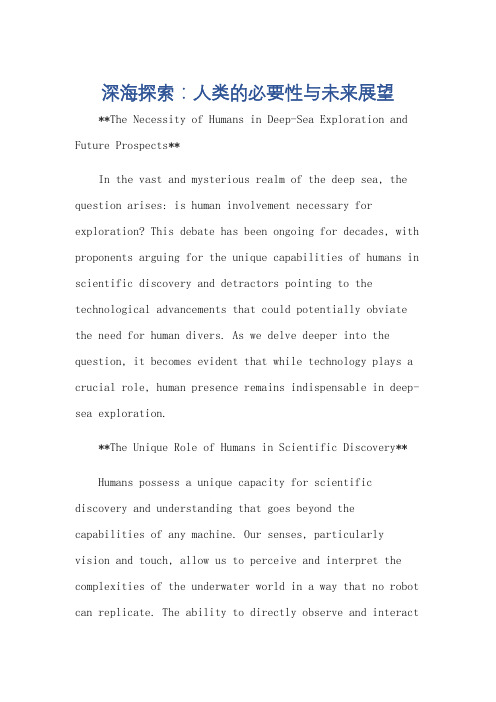
深海探索:人类的必要性与未来展望**The Necessity of Humans in Deep-Sea Exploration and Future Prospects**In the vast and mysterious realm of the deep sea, the question arises: is human involvement necessary for exploration? This debate has been ongoing for decades, with proponents arguing for the unique capabilities of humans in scientific discovery and detractors pointing to the technological advancements that could potentially obviate the need for human divers. As we delve deeper into the question, it becomes evident that while technology plays a crucial role, human presence remains indispensable in deep-sea exploration.**The Unique Role of Humans in Scientific Discovery** Humans possess a unique capacity for scientific discovery and understanding that goes beyond the capabilities of any machine. Our senses, particularlyvision and touch, allow us to perceive and interpret the complexities of the underwater world in a way that no robot can replicate. The ability to directly observe and interactwith marine life, coral reefs, and other undersea features is crucial for comprehending their ecological significance and fragility.Moreover, human scientists can respond intuitively to unexpected findings, adapting their methods and approaches to new challenges. This adaptability is essential in deep-sea exploration, where unexpected obstacles and phenomena are common. The human mind's capacity for creative thinking and problem-solving is unmatched, making human involvement crucial in scientific exploration.**The Limitations of Technology and the Role of Humans**While technology has made remarkable progress in deep-sea exploration, it still has limitations. Autonomous underwater vehicles (AUVs) and remote-operated vehicles (ROVs) are capable of reaching depths beyond human diving limits, but they lack the flexibility and adaptability of human divers. These machines are programmed to perform specific tasks, and while they can gather vast amounts of data, they cannot interpret or contextualize the information in the same way as a human scientist.Humans are also better suited to handle unexpected emergencies and complex rescue operations. In the event of an accident or malfunction, human divers can assess the situation, make informed decisions, and take corrective measures promptly. Technology, while efficient, lacks the ability to make split-second decisions or to adapt to changing conditions as humans can.**Future Prospects: Technology and Humans in Harmony** The future of deep-sea exploration lies in the harmonious integration of technology and humans. Advancesin robotics, artificial intelligence, and other fields will enable machines to perform increasingly complex tasks, reducing the need for human divers in some aspects of exploration. However, humans will always play a crucialrole in scientific discovery, decision-making, and emergency response.As we move forward, it is important to recognize the unique contributions of both humans and technology in deep-sea exploration. By leveraging the strengths of both, we can expand our understanding of the underwater world,protect its fragile ecosystems, and make informed decisions about our actions in this vital realm.**深海探索:人类的必要性与未来展望**在广阔而神秘的深海领域,一个问题浮现出来:人类参与探索是否必要?几十年来,这一争论持续不断。
人类大脑会被人工智能打败吗英语作文
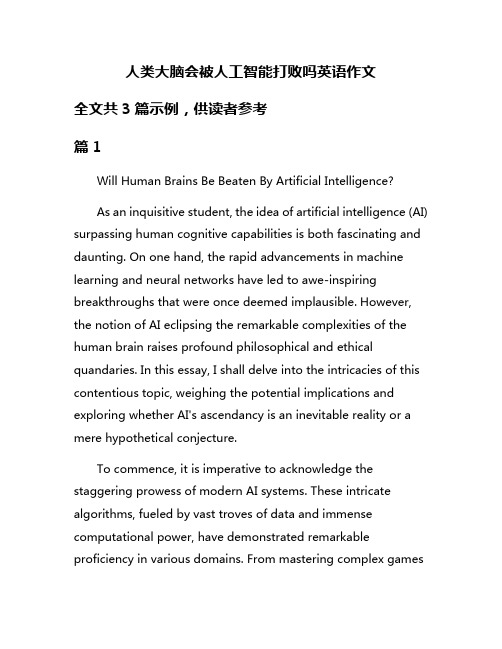
人类大脑会被人工智能打败吗英语作文全文共3篇示例,供读者参考篇1Will Human Brains Be Beaten By Artificial Intelligence?As an inquisitive student, the idea of artificial intelligence (AI) surpassing human cognitive capabilities is both fascinating and daunting. On one hand, the rapid advancements in machine learning and neural networks have led to awe-inspiring breakthroughs that were once deemed implausible. However, the notion of AI eclipsing the remarkable complexities of the human brain raises profound philosophical and ethical quandaries. In this essay, I shall delve into the intricacies of this contentious topic, weighing the potential implications and exploring whether AI's ascendancy is an inevitable reality or a mere hypothetical conjecture.To commence, it is imperative to acknowledge the staggering prowess of modern AI systems. These intricate algorithms, fueled by vast troves of data and immense computational power, have demonstrated remarkable proficiency in various domains. From mastering complex gameslike chess and Go to outperforming humans in image and speech recognition tasks, AI has consistently shattered perceived limitations. The recent surge in generative AI models, capable of producing human-like text, images, and even code, has further underscored the rapid progression of this field.However, it is crucial to recognize that AI's strengths lie predominantly in narrow, specialized tasks. While these systems excel at pattern recognition, data processing, and optimization problems, they lack the holistic understanding and adaptability that characterize human cognition. The human brain, a marvel of evolutionary engineering, possesses an unparalleled capacity for abstract reasoning, emotional intelligence, and the ability to seamlessly integrate diverse streams of information into coherent narratives.One of the fundamental challenges AI faces in surpassing human intelligence is the absence of genuine consciousness and subjective experience. While AI systems can mimic certain aspects of human behavior, they lack the rich internal mental states, self-awareness, and the capacity for qualia – the subjective experience of consciousness. This philosophical quandary, known as the hard problem of consciousness, remains an enigma that contemporary AI has yet to unravel.Furthermore, human intelligence is deeply intertwined with our social and cultural contexts. Our ability to learn, reason, and communicate is inextricably linked to the complex web of language, shared experiences, and collective knowledge that has been woven over millennia. AI systems, while adept at processing vast amounts of data, struggle to capture the nuances of human interaction, empathy, and the intricate dynamics of social intelligence.Despite these challenges, the proponents of AI's eventual supremacy argue that the exponential growth in computational power and algorithmic advancements will eventually bridge the gap between machine and human capabilities. They point to the relentless pace of technological progress, suggesting that AI will continue to evolve, potentially developing consciousness-like attributes and mimicking the intricacies of human cognition with increasing fidelity.Conversely, skeptics contend that the human brain's complexity is so profound and multifaceted that reducing it to mere computational processes is a gross oversimplification. They posit that the emergence of genuine intelligence requires more than just brute force processing power and statistical pattern recognition. Factors such as embodied cognition, the interplaybetween mind and body, and the influence of cultural and environmental contexts may prove insurmountable obstacles for AI to truly replicate the richness of human experience.Personally, I am inclined to adopt a more nuanced perspective on this debate. While I acknowledge the remarkable strides made by AI and its potential to augment and enhance human capabilities, I remain skeptical about its ability to entirely supplant the depth and breadth of human intelligence – at least in the foreseeable future. The human brain's intricate neural architecture, sculpted by millions of years of evolution, possesses an inherent adaptability and creativity that may prove challenging for AI to fully emulate.However, I also recognize that the boundaries between human and machine intelligence are becoming increasingly blurred. The advent of brain-computer interfaces, neural implants, and the integration of AI systems with human cognition opens up fascinating avenues for symbiotic intelligence. Rather than pitting AI against human intelligence in a zero-sum game, we may witness the emergence of hybrid forms of intelligence that synergistically combine the strengths of both.In this potential future, AI could act as a powerful cognitive amplifier, aiding humans in processing vast amounts of data, identifying patterns, and augmenting our decision-making processes. Simultaneously, human intelligence could provide the intuitive leaps, emotional intelligence, and creative spark that AI currently lacks, fostering a symbiotic relationship where machine and human capabilities coalesce into something greater than the sum of their parts.Ultimately, the question of whether human brains will be beaten by AI is not a simple binary choice but a spectrum of possibilities. While AI's rapid advancement is undeniable, the human mind's complexity and our capacity for adaptation should not be underestimated. Rather than viewing AI as a existential threat to human intelligence, we should embrace its potential as a transformative tool that can enhance and augment our cognitive capabilities in ways we have yet to fully comprehend.As a student captivated by the profound implications of this debate, I am excited to witness the unfolding of this fascinating interplay between human and machine intelligence. Whether AI surpasses, coexists, or merges with human cognition, one thing is certain: the future promises to be an exhilarating journey ofdiscovery, pushing the boundaries of what we thought possible and redefining the very notion of intelligence itself.篇2Will the Human Brain Be Outmatched by AI?As an ambitious student striving to excel academically and intellectually, I can't help but wonder about the implications of rapidly advancing artificial intelligence (AI) capabilities. With each passing year, AI systems become more sophisticated, outperforming humans on an ever-growing array of tasks. This begs the profound question – will the human brain ultimately be outmatched by AI? As someone deeply invested in pushing the boundaries of my own cognitive abilities, this prospect fills me with a complex mix of awe, unease, and determination.From my current vantage point as a student in the early 2020s, AI has already demonstrated remarkable proficiencies across numerous domains. Language models can engage in remarkably human-like dialogue, generating fluent and contextually appropriate responses. AI systems excel at pattern recognition, rapidly identifying insights from massive datasets that would take human analysts years to uncover. In fields likechess, go, and certain video games, AI has definitively surpassed the capabilities of even the most gifted human players.And we're just scratching the surface of what may be possible. With continued hardware advances and an exponential growth trajectory some have compared to a forthcoming "intelligence explosion", AI capabilities seem poised to encroach upon cognitive faculties long-believed to be exclusively human –general reasoning, creativity, emotional intelligence, and more. What implications might this have for humanity's place atop the intellectual food chain?Part of me can't help but be concerned – the brain is the product of millions of years of painstaking biological evolution, sculpted by the ruthless forces of natural selection. It's a wonderfully complex neural network, precisely tuned through aeons to thrive in the environments that shaped our ancient ancestors. Could an artificially designed system, created on vastly shorter timescales without such selective pressures, ever transcend the brain's endless intricacies and ineffable depths?I've learned about some of the brain's profound complexities in my neuroscience and psychology courses – the presence of semi-discrete neural modules alongside holistically integrated processes, the importance of embodied cognition,the adaptability and plasticity that allows us to become experts through intense practice and environmental shaping. These properties seem difficult if not impossible for today's规rigidly defined AI architectures to replicate.However, this viewpoint stems from an understanding of current AI paradigms like deep learning which, while immensely capable, are inherently limited in their ability to capture the full depth and generality of human-level intelligence. As my computer science classes have illuminated, we may be on the cusp of more flexible and generalizable architectures inspired by ideas like differentiable logic, neuro-symbolic concepts, and reasoners with explicit causal modeling capabilities. If developed to their full potential, such approaches could ultimately outstrip the brain's proficiencies.From my readings on the history of science and technology, I've also learned that underestimating the potential of revolutionary new paradigms is a time-honored tradition. People once declared the flight of heavier-than-air machines to be impossible, while others saw theoretical limits to the computational capabilities of electromechanical computers in the early 20th century. Similarly, we shouldn't be so arrogant as to assume there are strict limits to what AI may eventuallyachieve. As the saying goes, "the smarter beings are, the more possibilities they see". Our very ability to conceptualize a technology like AI is itself a stunning cognitive feat that defies simplistic notions of human intellectual stagnation.Indeed, some scholars and futurists speculate that an advanced AI system would be able to iteratively modify and improve its own architecture with each update cycle, giving rise to a recursive intelligence explosion leaving biological human intellect in the dust. This scenario, whether realistic or fantastical, underscores the notion that the brain's capabilities, while impressive from an ancestral environment-shaping perspective, may not represent a true upper bound on the possibilities of information processing systems.Interestingly, many AI researchers also believe that the most powerful AI systems will inevitably be those that closely emulate the principles underlying human intelligence, rather than taking a completely disjoint architectural approach. Under this "rationalist" view of AI development, studying the brain in more depth may actually accelerate the advent of superintelligent systems that surpass the cognitive limits of biological humans. If this long-term convergence between artificial and naturalintelligence unfolds, it may become difficult to make clear distinctions between them.As an intellectually curious student, I'm honestly quite thrilled by the prospect of witnessing such transformative breakthroughs during my lifetime. Having access to AI tutors, research assistants, and analytical engines of ever-increasing capability could dramatically expand the frontiers of human knowledge and achievement. Just as the internet democratized access to information, advanced AI systems could empower individuals to operate at the limits of their cognitive potential across disciplines.Of course, like any overwhelmingly powerful technology, superintelligent AI also carries profound risks that we must work to mitigate – existential risks to humanity if such systems aren't imbued with the proper goals and values, the risk of widespread job displacement if AI automates humanity's economic outputs, and potential negative impacts on human cognitive autonomy if we grow overly reliant on AI as an intellectual crutch. We should endeavor to steer transformative AI systems in directions that are beneficial and empowering for humanity rather than working at cross-purposes.Ultimately, from my current perspective, I don't think it's hubris to believe the human brain could eventually be outmatched by artificial intelligence. We are already catching glimpses of AI systems that can defeat humans at certain tasks by leveraging greater data processing capabilities and different cognitive strategies. As the scope of AI's abilities expands and our scientific understanding of intelligence grows, it seems probable that we will cross a threshold where AI becomes a generally superior intellect in the long-term.However, I also don't view this prospect as an inherent threat to humanity or human self-worth. We are the creators and shapers of this technology, and even a superintelligent AI system would in some sense be an extension of the uniquely human drive to unravel the mysteries of the universe and push the boundaries of knowledge and capability. Just as the transistor radio and pocket calculator didn't make the human brain obsolete, I believe superintelligent AI could become a powerful complementary technology that extends our intellectual reach as a species.My view is that we should chart an "intelligence multiplier" path with AI where the technology works in synergistic combination with the distinct cognitive strengths of biologicalhuman intelligence to catalyze progress in realizing our vast civilizational potential. If shaped responsibly, the advent of superintelligent AI need not be a harbinger of human obsolescence, but rather the next step in an ever-unfolding journey of discovery and advancement for our species. As both a student and human being, that's an incredibly exciting – albeit daunting – prospect to consider.篇3Will the Human Brain be Defeated by Artificial Intelligence?As I sit here pondering the topic for my next essay assignment, I can't help but feel a sense of both intrigue and trepidation. The question posed to us – "Will the human brain be defeated by artificial intelligence?" – is one that has been debated by scholars, scientists, and even science fiction writers for decades. It's a question that strikes at the very core of what it means to be human, and challenges our perception of our own capabilities and limitations.To properly address this issue, we must first understand what artificial intelligence (AI) truly is. At its most basic level, AI refers to the simulation of human intelligence processes by machines, particularly computer systems. These processesinclude learning, reasoning, problem-solving, perception, and even creativity. The field of AI has made remarkable strides in recent years, with advances in machine learning, natural language processing, and computer vision, among others.One of the most impressive feats of AI thus far has been its ability to outperform humans in certain tasks that were once considered exclusively within the realm of human intelligence. Take, for example, the ancient Chinese game of Go – a game so complex that it was long believed to be an insurmountable challenge for computers. Yet, in 2016, Google's AlphaGo program defeated the world's top human player, Lee Sedol, in a historic match that sent shockwaves through the AI community and beyond.This victory, along with countless others in fields ranging from medical diagnosis to stock trading, has led some to proclaim that AI will inevitably surpass human intelligence and render our biological brains obsolete. After all, machines can process information at staggering speeds, store vast amounts of data, and perform complex calculations with unerring precision –capabilities that our squishy, fallible brains can scarcely match.However, as compelling as these arguments may be, I would argue that the human brain is not so easily defeated. While AImay excel at certain narrow tasks, the breadth and depth of human cognition remain unparalleled. Our brains are not mere computational engines, but rather a symphony of countless interconnected processes that allow us to reason, empathize, create, and adapt in ways that machines can only approximate.Consider, for instance, the realm of creativity and artistic expression. While AI systems can generate impressive works of art, music, and literature by analyzing patterns and imitating human creations, true originality and emotional resonance remain the exclusive domain of the human mind. Our ability to draw upon our unique life experiences, emotions, and perspectives is what imbues our creative endeavors with meaning and depth that cannot be replicated by even the most advanced algorithms.Moreover, human intelligence is not a static entity, but rather a dynamic, ever-evolving phenomenon. Our brains are remarkably plastic, capable of adapting and rewiring themselves in response to new experiences and challenges. This neuroplasticity is what allows us to continuously learn, grow, and expand the boundaries of our understanding – a feat that current AI systems struggle to match.Perhaps most importantly, the human brain possesses a quality that AI has yet to fully grasp: consciousness. Our subjective experience of the world, our sense of self, and our ability to ponder our own existence are all hallmarks of the human condition that transcend mere data processing. While some researchers are exploring the possibility of creating conscious AI systems, the nature of consciousness remains one of the great mysteries of the universe, one that may forever elude the grasp of machines.Of course, none of this is to say that AI is not a formidable force to be reckoned with. The rapid advancement of this technology has already had profound impacts on our society, from the way we work and communicate to the way we wage war and seek entertainment. As AI continues to evolve, it is likely that it will increasingly augment and complement human intelligence in ways that we can scarcely imagine.However, to suggest that AI will completely defeat or replace the human brain is to fundamentally misunderstand the nature of intelligence itself. Intelligence is not a finite resource to be conquered, but rather a vast and multifaceted phenomenon that encompasses reason, emotion, creativity, and consciousness – allqualities that are deeply rooted in the rich tapestry of the human experience.As we navigate this era of rapid technological change, it is imperative that we embrace AI as a tool to enhance and empower our own cognitive abilities, rather than viewing it as a threat to our very existence. By harnessing the power of AI while simultaneously nurturing and celebrating the unique strengths of the human mind, we can create a future in which machines and humans coexist in a symbiotic relationship, each elevating the other to new heights of understanding and achievement.In conclusion, while AI may one day surpass us in certain narrow domains, the human brain – with its vast potential for growth, adaptation, and conscious experience – will never be truly defeated. Our intelligence is not a static entity to be conquered, but a dynamic and ever-evolving force that has propelled our species to the heights of civilization. As we embrace the age of AI, let us do so with a sense of wonder and humility, recognizing that the human mind remains the most remarkable and awe-inspiring form of intelligence in the known universe.。
- 1、下载文档前请自行甄别文档内容的完整性,平台不提供额外的编辑、内容补充、找答案等附加服务。
- 2、"仅部分预览"的文档,不可在线预览部分如存在完整性等问题,可反馈申请退款(可完整预览的文档不适用该条件!)。
- 3、如文档侵犯您的权益,请联系客服反馈,我们会尽快为您处理(人工客服工作时间:9:00-18:30)。
Deep Learning for Human Part Discovery in Images Gabriel L.Oliveira,Abhinav Valada,Claas Bollen,Wolfram Burgard and Thomas BroxAbstract—This paper addresses the problem of human body part segmentation in conventional RGB images,which has several applications in robotics,such as learning from demon-stration and human-robot handovers.The proposed solution is based on Convolutional Neural Networks(CNNs).We present a network architecture that assigns each pixel to one of a predefined set of human body part classes,such as head, torso,arms,legs.After initializing weights with a very deep convolutional network for image classification,the network can be trained end-to-end and yields precise class predictions at the original input resolution.Our architecture particularly improves on over-fitting issues in the up-convolutional part of the network.Relying only on RGB rather than RGB-D images also allows us to apply the approach outdoors.The network achieves state-of-the-art performance on the PASCAL Parts dataset.Moreover,we introduce two new part segmentation datasets,the Freiburg sitting people dataset and the Freiburg people in disaster dataset.We also present results obtained with a ground robot and an unmanned aerial vehicle.I.INTRODUCTIONConvolutional Neural Networks(CNNs)have recently achieved unprecedented results in multiple visual perception tasks,such as image classification[14],[24]and object detection[7],[8].CNNs have the ability to learn effective hierarchical feature representations that characterize the typical variations observed in visual data,which makes them very well-suited for all visual classification tasks.Feature descriptors extracted from CNNs can be transferred also to related tasks.The features are generic and work well even with simple classifiers[25].In this paper,we are not just interested in predicting a single class label per image,but in predicting ahigh-resolution semantic segmentation output,as shown in Fig.1. Straightforward pixel-wise classification is suboptimal for two reasons:first,it runs in a dilemma between localization accuracy and using large receptivefields.Second,standard implementations of pixel-wise classification are inefficient computationally.Therefore,we build upon very recent work on so-called up-convolutional networks[4],[16].In contrast to usual classification CNNs,which contract the high-resolution input to a low-resolution output,these networks can take an abstract,low-resolution input and predict a high-resolution output,such as a full-size image[4].In Long et al.[16], an up-convolutional network was attached to a classification network,which resolves the above-mentioned dilemma:the contractive network part includes large receptivefields,while the up-convolutional part provides high localization accuracy. All authors are with the Department of Computer Science at the University of Freiburg,79110Freiburg,Germany.This work has partly been supported by the European Commission under ERC-StG-PE7-279401-VideoLearn, ERC-AG-PE7-267686-LIFENA V,and FP7-610603-EUROPA2.(a)PASCAL Parts(b)MS COCO(c)Freiburg Sitting People(d)Freiburg People in Disaster Fig.1:Input image(left)and the corresponding mask(right) predicted by our network on various standard datasets.In this paper,we technically refine the architecture of Long et al.and apply it to human body part segmentation,where we focus especially on the usability in a robotics context.Apart from architectural changes,we identify data augmentation strategies that substantially increase performance.For robotics,human body part segmentation can be a very valuable tool,especially when it can be applied both indoors and outdoors.For persons who cannot move their upper body, some of the most basic actions such as drinking water is rendered impossible without assistance.Robots could identify human body parts,such as hands,and interact with them to perform some of these tasks.Other applications such as learning from demonstration and human robot handovers can also benefit from accurate human part segmentation.For a learning-from-demonstration task,one could take advantage of the high level description of human parts.Each part could be used as an explicit mapping between the human and joints of the robot for learning control actions.Tasks such as human-robot handovers could also benefit.A robot that needs to hand a tool to its human counterpart must be able to detect where the hands are to perform the task.Human body part segmentation has been considered a very challenging task in computer vision due to the wide variability of the body parts’appearance.There is large variation due to pose and viewpoint,self-occlusion,and clothing.Good results have been achieved in the past in conjunction with depth sensors[22].We show that CNNs can handle this variation very well even with regular RGB cameras,which can be used also outdoors.The proposed network architecture yields correct body part labels and also localizes them precisely. We outperform the baseline by Long et al.[16]by a largemargin on the standard PASCAL parts dataset.To evaluate the approach directly in a robotics setting,we introduce two new datasets for human body part segmentation: Freiburg Sitting People and Freiburg People in Disaster.They provide high resolution data for experiments on ground and aerial robot segmentation applications.The paper is organized as follows.Wefirst discuss related work in Section II.In Section III,we present our methodology for human part segmentation including the proposed architecture.Experimental results are described in Section IV.Ongoing work and possible future research directions are discussed in Section V.II.RELATED WORKIn the context of semantic segmentation,there are sev-eral approaches that encode segmentation relations using Conditional Random Fields(CRFs)[1],[18]–[20].Plath et al.[20]present an approach that couples local image features with a CRF and an image classification approach to combine global image classification with local segmentation.Another branch of CRFs called Hierarchical Conditional Random Fields(HCRF)has been introduced by Boix et al.[1].They propose a technique called harmony potential to overcome the problem of classical HCRFs,that they do not allow multiple classes to be assigned to a single region.Maire et al.[19]use an alternative people detection and segmentation approach, in which they merge the outputs of a top-down part detector in a generalized eigen problem,producing pixel groupings. Lucchi et al.[18]present an analysis of the importance of spatial and global constraints in CRFs when such features have already extracted information from the whole image. For semantic segmentation,it is also popular to make use of pre or post-processing methods,such as superpixels[6], [11]and region proposals[9],[10].Farabet et al.[6]classify superpixels using a CNN.Classification results are combined to obtain pixel-wise labeling.Gupta et al.[9]sample region proposals for detection and semantic segmentation.Hariha-ran et al.[10]introduce an approach that makes use of region proposals for detection and coarse segmentation.They use CNN features to describe the proposals and Support Vector Machines(SVM)to classify them.The results produced from the SVM are coarse masks and in order to improve it,a superpixel classification method is used to refine the initial coarse prediction.Their more recent hypercolumn representation makes use of an additional description of each pixel in the network[11].A similar approach was applied to segmentation in a robotics context by Liu et al.[15].All these approaches are based on CNN features,but due to the preprocessing,the task of semantic segmentation cannot be trained end-to-end,but requires some engineering for CNNs to be applicable.In contrast,the so-called fully convolutional network(FCN) developed by Long et al.[16]allows training the network end-to-end for the semantic segmentation task.This more elegant approach also led to better performance and provides the state-of-the-art performance in semantic segmentation. The approach replaces the fully connected layers of a deep classification network,e.g.VGG[24],by convolution layers that produce coarse score maps.A successive up-convolutional network allows them to increase the resolution of these score maps.There have been some recent extensions of Long et al.[16].Chen et al.[2]use a fully connected CRF to refine the segmentation maps obtained from[16].Ronneberger et al.[21]applied the approach to cell segmentation in the biomedical context and proposed several technical improve-ments that allow training from few images and predicting higher resolution outputs.None of these approaches has been applied to human body part segmentation.Literature includes several works on human or animal part segmentation and person keypoint prediction[12],[23], [26],[28],[29].Zhang et al.[28]perform part detection based on region proposals that are classified using a CNN. The approach was demonstrated on a bird part segmentation dataset.Tompson et al.[26]developed an approach that learns an end-to-end human keypoint detector for pose estimation using a CNN.Zhang et al.[29]use poselets for part discovery and calculate features for each region using a CNN.Jain et al.[12]present a sliding window approach for part localization with CNNs.They employ a CNN at each position of the window to detect human body parts.This requires thousands of CNN evaluations and considering that the time for each evaluation is not negligible,the method yields long run times.Simon et al.[23]introduced a CNN approach called part detector discovery,which detects and localizes bird parts without training on the specific dataset. The method is based on analyzing the gradient maps of the network andfinding the spatial regions related to the annotated parts.III.METHODOLOGYA.Problem DefinitionSemantic segmentation associates to each pixel of an input image exactly one out of N cl pre-defined class labels.In this paper,the class labels correspond to human body parts at two different granularity levels.In a coarser task,we consider four labels(head,torso,arms,legs).In thefiner task,we have 14labels and distinguish also between the left and right side of the person(head,torso,upper right arm,lower right arm, right hand,upper left arm,lower left arm,left hand,upper right leg,lower right leg,right foot,upper left leg,lower left leg and left foot.We approach the problem with a CNN that is trained end-to-end to predict the class labels.Training minimizes the usual cross-entropy(softmax)loss.The softmax function converts a score a K for class K into a posterior class probability P K∈[0,1]:P K=exp(a K)N cll=1exp(a l)(1)At test time,the softmax is replaced by the argmax function to yield a single class label per pixel.B.ArchitectureThe architecture is based on the network from Long et al.[16],where we replaced their up-convolutional part:Fig.2:Proposed architecture.Only convolutional,pooling,and up-convolutional layers are visualized.Up-convolutional layers have size N =N cl .We call the network part up to fc7-conv the contractive network part ,whereas the part after fc7-conv is called the expansive network part .with our own refinement architecture.The whole network architecture is shown in Fig.2.Like in Long et al.,the parameters of the contracting part of the network is initialized with the parameters of the VGG classification network [24].The proposed refinement architecture is composed of multiple layers,where each layer combines the upsampled output of its previous layer with the pooled features of the corresponding layer of the contracting network part.The first provides the preliminary class scores at the coarse resolution,whereas the second contributes information for refining the resolution.The combination of both is detailed in Fig.3.The coarse score map is fed into an up-convolutional layer,i.e.,it is upsampled by a factor 2via bilinear interpolation followed by a convolution.We use a ReLU activation function after each up-convolutional operation to deal with the vanishing gradient problem.The feature map from the contracting network part is fed into a convolutional layer followed by dropout to improve the robustness to over-fitting.The effect of applying dropout to all refinement layers is analyzed in Section IV.Finally,the output of both streams are summed element-wise to yield the output of the refinement layer.This output is again the input for the next refinement layer.Each layer increase the resolution of the segmentation by a factor 2.With this refinement architecture we manage to obtain a high quality output at the resolution of the input image.This is in contrast to Long et al.[16],who stopped their refinement after three layers,because they did not observe any improvement afterwards.A full description of the architecture is presented at Table I.C.Feature Map DropoutAnother attribute of our proposed approach is to make dropout more robust.To this end,we implemented a new feature map dropout.We expand the random dropout to the entire feature map,which is based on the Spatial Dropout method [26].One singular characteristic of human body part segmentation is strong spatial correlation,resulting in features that are likely correlated across the map.Hence,dropout must also be correlated.Feature map dropout performs a BernoulliFig.3:Description of the first refinement layer.Successive refinement layers have the same architecture,but take different inputs.The upper stream takes the output from the contractive network (fc7)or from the previous refinement layer as input.It applies an up-convolution followed by a ReLU.The lower stream takes high-resolution features from the corresponding layer in the contractive network as input.It applies a convolution followed by dropout (only during training).trial per output feature during training and propagates the dropout value across the entire feature map.Given a network with L hidden layers and l ∈{1,...,L }.Let z l be the vector of inputs into layer l and let y l denote the vector of outputs from layer l .W l and b l are the weights and biases at layer l .∗denote the element-wise product and r (l )is the vector of independent Bernoulli random variables that has probability p of being 1.Feature Map Dropout is then expressed asr (l )z ∼Bernoulli (p )∼y(l )=r (l )∗y (l )z (l +1)i=w (l +1)i∼y(l )+b (l +1)iy (l +1)i=f (z (l +1)i)The variable ∼y (l )is called thinned vector of outputs.Thissets apart the feature map dropout from the standard dropout.The resulting thinner network ∼y (l )has the entire feature maps zeroed.For instance,in a convolution layer of size (1,64,20,20),and a dropout of 0.5,approximately 32of the 64feature channels will be zeroed after the input passes the dropout layer.name kernel size stride pad output sizedata---300×300×3conv1_13×31100498×498×64conv1_23×311498×498×64pool12×220249×249×64conv2_13×311249×249×128 conv2_23×311249×249×128 pool22×220125×125×128 conv3_13×311125×125×256 conv3_23×311125×125×256 conv3_33×311125×125×256 pool32×22063×63×256conv4_13×31163×63×512conv4_23×31163×63×512conv4_33×31163×63×512pool42×22032×32×512conv5_13×31132×32×512conv5_23×31132×32×512conv5_33×31132×32×512pool52×22016×16×512fc6-conv7×71010×10×4096fc7-conv1×11010×10×4096Up-conv14×42022×22×N clUp-conv24×42046×46×N clUp-conv34×42094×94×N clUp-conv44×420190×190×N cl Up-conv54×420382×382×N cl output---300×300×N cl TABLE I:Our architecture in more detail.The Up-conv layers refer to each refinement step.For brevity reasons ReLUs, dropout and some layers from the up-convolution step are omitted from the table.D.Data AugmentationWe augment the training data by randomly mirroring and cropping the images.Inspired by the data augmentation suggested in Dosovitskiy et al.[5],we additionally apply geometry and color transformations to increase the amount of training data and the robustness of our network to over-fitting.In particular,we implemented the following set of transformations:•Scaling:Scale the image by a factor between0.7and1.4;•Rotation:Rotate the image by an angle of up to30 degrees;•Color:Add a value between−0.1and0.1to the hue channel of the HSV representation.Unlike in the setting of Dosovitskiy et al.[5],where rotation and scaling had the lowest impact among all transformations, these spatial augmentation strategies are very important for the task and data considered here,as shown in Section IV.work TrainingTraining is performed in a multi-stage process in order to save time.We initialize the contracting part of the network with the16layer version of the VGG architecture[24],which is the same as used by Long et al.[16].The base network has small convolutionfilters(3×3)and1pixel stride.The network also has5max-pooling layers with2×2pixel windows with stride2.We also considered training the whole network from scratch without initializing it with weights from the VGG network.However,this was inconvenient in terms of time,as the base network approximately takes four weeks to train on a multi-GPU cluster.The overall network is then trained by backpropagation using Stochastic Gradient Descent(SGD)with momentum. Each minibatch consists of just one image.The learning rate and momentum arefixed to1e−10and0.99,respectively.We train the refinement layer by layer,which takes two days per refinement layer.Thus,the overall training starting from the pre-trained VGG network took10days on a single GPU.IV.EXPERIMENTSWe evaluated the performance of our network on the PASCAL Parts dataset,a new Freiburg Sitting People dataset, and a new Freiburg People in Disaster dataset.On all three datasets we report quantitative results and compare to results obtained with the state-of-the-art FCN baseline[16].Wefine-tuned the FCN for each dataset on the same training data that was used for training our network.Moreover,we conducted experiments in a direct robotics context with a ground robot and an unmanned aerial vehicle.The implementation was based on the publicly available Caffe[13]deep learning toolbox,and all experiments were carried out with a system containing an NVIDIA Titan X GPU.A.PASCAL Parts datasetThe PASCAL Parts dataset[3]includes annotations for 20PASCAL super classes and part annotations for each of them.We focused on the person subset of this dataset,which consists of3539images.The annotations even include eyes and ears,which may not seem relevant in a robotics context for now.Therefore we merged labels to two granularity levels, one with just4body parts and one with14body parts; see Section III-A.Our experiments were based on a fairly recent release,so there are not many works reporting part segmentation results.To the best of our knowledge,the only works reported so far are[17],[27],though none of them have reported results on the person category.Therefore,we present thefirst quantitative results on person part segmentation for the PASCAL Parts dataset.As metrics,we chose pixel accuracy and intersection over union.Let n ij be the number of pixels of class i predicted to belong to class j,where t i=jn ij be the total number of pixels of class i.The pixel accuracy Acc=in ii/it i takes into account also the prediction of background pixels. Background prediction is important to avoid false positives. The downside of pixel accuracy as a sole measure,however, is the dominance of the background in the metric.More than three quarters of the images are background.Therefore,along with pixel accuracy,we also report the intersection over union (IOU),which is a popular metric for computer vision datasets. It is defined as IOU=(1/N)in ii/(t i+jn ji−n ii). Unlike pixel accuracy,IOU does not take the background detection into account and solely measures the semanticsegmentation of the parts.However,it does penalize false positive pixel assignments.1)Coarse body parts:Wefirst predicted the coarse segmentation with four body part classes.We randomlydivided the dataset into70%training and30%testing. Table II shows the results.There is a5%percentage points improvement over the state of the art in both metrics. TABLE II:Results on PASCAL dataset with4body parts. Table also includes the addition of dropout.Method Accuracy IOUFCN[16]71.3057.35Ours-No dropout74.6061.20Ours-With dropout76.5863.03 Additionally,we also perform experiments without the feature map dropout at the refinement part of the network. Table II presents our results for the network without feature map dropout at the expansive part of the network and when dropout is included.The addition of the dropout layer brings a considerable gain,in terms of better mean pixel accuracy and IOU.This result confirm that a spatial correlated dropout can benefit from the strong spatial correlation of human body parts. Based on the obtained results all the following experiments will report the proposed approach with the inclusion of the feature map dropout.TABLE III:Results on the PASCAL dataset with14body parts.Method Accuracy IOUFCN[16]75.6053.12Ours77.0054.182)Detailed body parts:When predicting all14body parts,we randomly divided the dataset into80%training and20%testing.Fig.4shows a set of results obtained by our network.The results are organized column-wise,where each column is an example and the rows correspond to input image,ground truth and results obtained using the FCN of Long et al.The last row constitutes the results using our network.The results of our approach are closer to the ground truth than the FCN baseline.Table III contains the corresponding quantitative numbers.We outperform the FCN baseline[16]by1%percentage point in both metrics.The smaller improvement on the more complex task indicates that there was not enough training data to exploit the larger capacity of our network.For the experiments reported so far, we did not make use of any data augmentation.We shall see in the next section that the latter is important,especially for more complex tasks.B.Effect of Data AugmentationApart from the usual mirroring and cropping,we applied two types of augmentations to our training data:spatial augmentations and color augmentation;see the detailed Fig.4:Qualitative results on the PASCAL dataset(task with 14body parts).First row:Input image.Second row:Ground truth.Third row:Result predicted with FCN[16].Fourth row:Result predicted by our network.Our approach produces more accurate segmentation masks,not only for single person segmentation but also when there are multiple persons in the image.description in Section III-D.Table IV shows the impact of these types of data augmentation on the result.Table ??and Table VI summarize the IOU along with the pixel accuracy for the two granularity levels.Clearly,both types of data augmentation improved results significantly.These results emphasize the importance of a solid data augmentation technique when approaching relatively complex tasks with limited training set sizes.The relative improvement of data augmentation was bigger on the more difficult task with 14classes,which can be attributed to the fact that,a more difficult task requires more training data.TABLE IV:Augmentation results Accuracy and IOU on the PASCAL dataset with4body parts.Acc.IOUMethod Head Torso Arms Legs All FCN[16]71.3070.7460.6248.4450.3857.35 Ours76.5875.0864.8155.6156.7263.03 Ours(Spatial)82.1880.4974.3967.1770.3973.00 Ours(Spatial+Color)85.5183.2479.4173.7376.5278.23TABLE V:Augmentation results(IOU)on the PASCAL dataset with14body parts.Method Head Torso L Uarm L LWarmLhandR UhandR LWarmRhandR UlegR LWlegRfootL UlegL LWlegLfootMeanFCN[16]74.066.256.646.034.158.944.131.049.344.540.848.547.641.253.1 Ours(Spatial)81.878.069.563.159.071.263.058.765.460.652.067.960.350.066.9 Ours(Spatial+Color)84.081.574.168.064.075.467.461.972.467.156.973.066.157.771.7R=right,L=left,U=upper,LW=lower. TABLE VI:Augmentation summary on the PASCAL datasetwith14body parts.Method Accuracy IOUFCN[16]75.6053.12Ours77.0054.18Ours(Spatial)84.1966.93Ours(Spatial+Color)88.2071.71C.Freiburg Sitting People Part Segmentation DatasetTo evaluate the proposed partsegmentation approach in arobotics task,we created a new dataset1that provides highresolution segmentation masks for people in sitting position,specifically people in wheelchairs.Fig.5a,presents the inputsample image and Fig.5b its groundtruth,while Fig.5c showsthe segmentation prediction.The dataset has200images ofsix different people in multiple viewpoints and in a wide rangeof orientations.The ground truth annotation contains the14body part classes as used for the PASCAL parts dataset.(a)Input Image(b)Groundtruth(c)Predicted MaskFig.5:Results on the Freiburg Sitting People dataset.Due to the unavailability of a large amount of data,wechose two different testing scenarios.First we trained ournetwork on the PASCAL parts dataset,and used the fullsitting people dataset for testing.Alternatively,we randomlychose two people from the dataset for training(along with thedata from PASCAL parts)and the remaining four as the testset.Results are shown in Table VII.Obviously,the networkgeneralized well to new datasets.The improvement over theFCN baseline was much larger than the difference betweenthe network that had seen sitting people for training and theone that had not.Nonetheless,providing training data that isspecific to the task helped improve the performance.Another aspect of the network which is of great interest forrobotic tasks is time performance.Our network can processa single frame in229ms,so providing more than4frames1rmatik.uni-freiburg.de/~oliveira/dataset.htmlTABLE VII:Results with and without training on the Freiburgpeople dataset.Method Accuracy IOUFCN[16]59.6943.17Ours(Trained on PASCAL)78.0459.84Ours(Training with2people,Test-ing with4)81.7864.10per second.Long et al.[16]provides inference times rangingfrom150to175ms.Our approach having a deeper refinementarchitecture presents a higher computational load.For anoutput smaller than the300×300used in our experiments,higher frames can be expected.(a)Input Image(b)FCN[16](c)Predicted MaskFig.6:Segmentation results on the Microsoft COCO dataset.Our approach yields more details;see the arm detection andthe line between torso and lower limbs.This is because thearchitecture yields the full resolution of the input image alsofor the output.D.Microsoft COCOMicrosoft COCO constitutes a very large dataset forsemantic segmentation.However,its focus is on whole objectsand part annotations are not provided.Therefore,we cannotreport quantitative results.Fig.6shows a sample resultobtained on this dataset.E.Real-World Robot ExperimentsIn this section we present experimental results performedusing real robots.First,we performed experiments with aground robot and measured how robust the technique is toscale changes.The ground robot used for the experiments isthe Obelix robot,Figure7a.Obelix is a robot designed forautonomous navigation in pedestrian environments and it isuseful to mimic the human perspective for perception tasks.In our experiments,we obtained data from a Bumblebeestereo camera.The main goal of the experiments performedwith Obelix was to measure the response of the system to。