图像检测外文翻译参考文献
外文参考文献翻译-中文
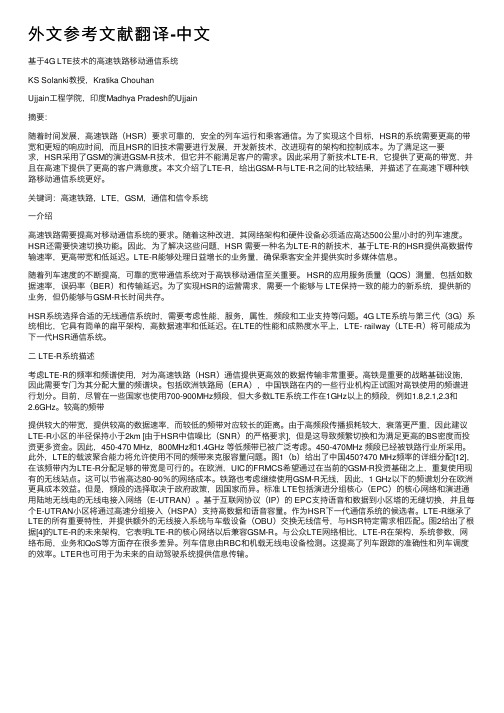
外⽂参考⽂献翻译-中⽂基于4G LTE技术的⾼速铁路移动通信系统KS Solanki教授,Kratika ChouhanUjjain⼯程学院,印度Madhya Pradesh的Ujjain摘要:随着时间发展,⾼速铁路(HSR)要求可靠的,安全的列车运⾏和乘客通信。
为了实现这个⽬标,HSR的系统需要更⾼的带宽和更短的响应时间,⽽且HSR的旧技术需要进⾏发展,开发新技术,改进现有的架构和控制成本。
为了满⾜这⼀要求,HSR采⽤了GSM的演进GSM-R技术,但它并不能满⾜客户的需求。
因此采⽤了新技术LTE-R,它提供了更⾼的带宽,并且在⾼速下提供了更⾼的客户满意度。
本⽂介绍了LTE-R,给出GSM-R与LTE-R之间的⽐较结果,并描述了在⾼速下哪种铁路移动通信系统更好。
关键词:⾼速铁路,LTE,GSM,通信和信令系统⼀介绍⾼速铁路需要提⾼对移动通信系统的要求。
随着这种改进,其⽹络架构和硬件设备必须适应⾼达500公⾥/⼩时的列车速度。
HSR还需要快速切换功能。
因此,为了解决这些问题,HSR 需要⼀种名为LTE-R的新技术,基于LTE-R的HSR提供⾼数据传输速率,更⾼带宽和低延迟。
LTE-R能够处理⽇益增长的业务量,确保乘客安全并提供实时多媒体信息。
随着列车速度的不断提⾼,可靠的宽带通信系统对于⾼铁移动通信⾄关重要。
HSR的应⽤服务质量(QOS)测量,包括如数据速率,误码率(BER)和传输延迟。
为了实现HSR的运营需求,需要⼀个能够与 LTE保持⼀致的能⼒的新系统,提供新的业务,但仍能够与GSM-R长时间共存。
HSR系统选择合适的⽆线通信系统时,需要考虑性能,服务,属性,频段和⼯业⽀持等问题。
4G LTE系统与第三代(3G)系统相⽐,它具有简单的扁平架构,⾼数据速率和低延迟。
在LTE的性能和成熟度⽔平上,LTE- railway(LTE-R)将可能成为下⼀代HSR通信系统。
⼆ LTE-R系统描述考虑LTE-R的频率和频谱使⽤,对为⾼速铁路(HSR)通信提供更⾼效的数据传输⾮常重要。
数字图像处理外文翻译参考文献
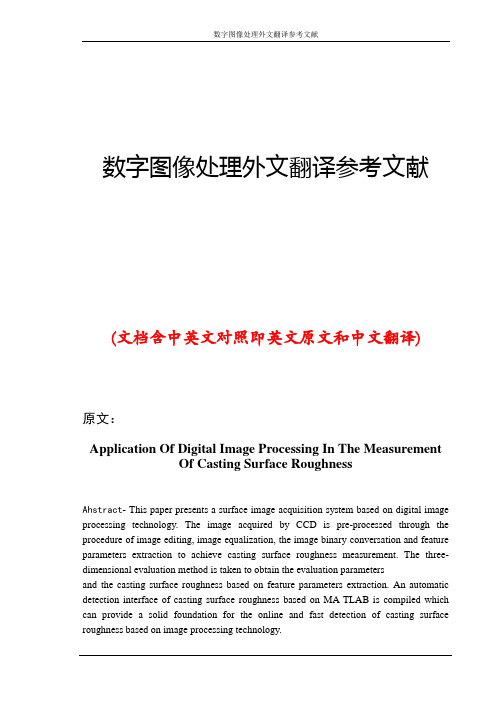
数字图像处理外文翻译参考文献(文档含中英文对照即英文原文和中文翻译)原文:Application Of Digital Image Processing In The MeasurementOf Casting Surface RoughnessAhstract- This paper presents a surface image acquisition system based on digital image processing technology. The image acquired by CCD is pre-processed through the procedure of image editing, image equalization, the image binary conversation and feature parameters extraction to achieve casting surface roughness measurement. The three-dimensional evaluation method is taken to obtain the evaluation parametersand the casting surface roughness based on feature parameters extraction. An automatic detection interface of casting surface roughness based on MA TLAB is compiled which can provide a solid foundation for the online and fast detection of casting surface roughness based on image processing technology.Keywords-casting surface; roughness measurement; image processing; feature parametersⅠ.INTRODUCTIONNowadays the demand for the quality and surface roughness of machining is highly increased, and the machine vision inspection based on image processing has become one of the hotspot of measuring technology in mechanical industry due to their advantages such as non-contact, fast speed, suitable precision, strong ability of anti-interference, etc [1,2]. As there is no laws about the casting surface and the range of roughness is wide, detection parameters just related to highly direction can not meet the current requirements of the development of the photoelectric technology, horizontal spacing or roughness also requires a quantitative representation. Therefore, the three-dimensional evaluation system of the casting surface roughness is established as the goal [3,4], surface roughness measurement based on image processing technology is presented. Image preprocessing is deduced through the image enhancement processing, the image binary conversation. The three-dimensional roughness evaluation based on the feature parameters is performed . An automatic detection interface of casting surface roughness based on MA TLAB is compiled which provides a solid foundation for the online and fast detection of casting surface roughness.II. CASTING SURFACE IMAGE ACQUISITION SYSTEMThe acquisition system is composed of the sample carrier, microscope, CCD camera, image acquisition card and the computer. Sample carrier is used to place tested castings. According to the experimental requirements, we can select a fixed carrier and the sample location can be manually transformed, or select curing specimens and the position of the sampling stage can be changed. Figure 1 shows the whole processing procedure.,Firstly,the detected castings should be placed in the illuminated backgrounds as far as possible, and then through regulating optical lens, setting the CCD camera resolution and exposure time, the pictures collected by CCD are saved to computer memory through the acquisition card. The image preprocessing and feature value extraction on casting surface based on corresponding software are followed. Finally the detecting result is output.III. CASTING SURFACE IMAGE PROCESSINGCasting surface image processing includes image editing, equalization processing, image enhancement and the image binary conversation,etc. The original and clipped images of the measured casting is given in Figure 2. In which a) presents the original image and b) shows the clipped image.A.Image EnhancementImage enhancement is a kind of processing method which can highlight certain image information according to some specific needs and weaken or remove some unwanted informations at the same time[5].In order to obtain more clearly contour of the casting surface equalization processing of the image namely the correction of the image histogram should be pre-processed before image segmentation processing. Figure 3 shows the original grayscale image and equalization processing image and their histograms. As shown in the figure, each gray level of the histogram has substantially the same pixel point and becomes more flat after gray equalization processing. The image appears more clearly after the correction and the contrast of the image is enhanced.Fig.2 Casting surface imageFig.3 Equalization processing imageB. Image SegmentationImage segmentation is the process of pixel classification in essence. It is a very important technology by threshold classification. The optimal threshold is attained through the instmction thresh = graythresh (II). Figure 4 shows the image of the binary conversation. The gray value of the black areas of the Image displays the portion of the contour less than the threshold (0.43137), while the white area shows the gray value greater than the threshold. The shadows and shading emerge in the bright region may be caused by noise or surface depression.Fig4 Binary conversationIV. ROUGHNESS PARAMETER EXTRACTIONIn order to detect the surface roughness, it is necessary to extract feature parameters of roughness. The average histogram and variance are parameters used to characterize the texture size of surface contour. While unit surface's peak area is parameter that can reflect the roughness of horizontal workpiece.And kurtosis parameter can both characterize the roughness of vertical direction and horizontal direction. Therefore, this paper establisheshistogram of the mean and variance, the unit surface's peak area and the steepness as the roughness evaluating parameters of the castings 3D assessment. Image preprocessing and feature extraction interface is compiled based on MATLAB. Figure 5 shows the detection interface of surface roughness. Image preprocessing of the clipped casting can be successfully achieved by this software, which includes image filtering, image enhancement, image segmentation and histogram equalization, and it can also display the extracted evaluation parameters of surface roughness.Fig.5 Automatic roughness measurement interfaceV. CONCLUSIONSThis paper investigates the casting surface roughness measuring method based on digital Image processing technology. The method is composed of image acquisition, image enhancement, the image binary conversation and the extraction of characteristic parameters of roughness casting surface. The interface of image preprocessing and the extraction of roughness evaluation parameters is compiled by MA TLAB which can provide a solid foundation for the online and fast detection of casting surface roughness.REFERENCE[1] Xu Deyan, Lin Zunqi. The optical surface roughness research pro gress and direction[1]. Optical instruments 1996, 18 (1): 32-37.[2] Wang Yujing. Turning surface roughness based on image measurement [D]. Harbin:Harbin University of Science and Technology[3] BRADLEY C. Automated surface roughness measurement[1]. The InternationalJournal of Advanced Manufacturing Technology ,2000,16(9) :668-674.[4] Li Chenggui, Li xing-shan, Qiang XI-FU 3D surface topography measurement method[J]. Aerospace measurement technology, 2000, 20(4): 2-10.[5] Liu He. Digital image processing and application [ M]. China Electric Power Press,2005译文:数字图像处理在铸件表面粗糙度测量中的应用摘要—本文提出了一种表面图像采集基于数字图像处理技术的系统。
图像识别中英文对照外文翻译文献
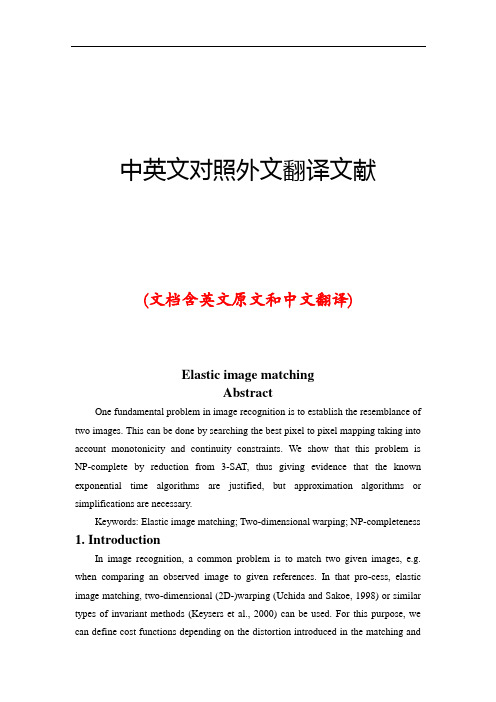
中英文对照外文翻译文献(文档含英文原文和中文翻译)Elastic image matchingAbstractOne fundamental problem in image recognition is to establish the resemblance of two images. This can be done by searching the best pixel to pixel mapping taking into account monotonicity and continuity constraints. We show that this problem is NP-complete by reduction from 3-SAT, thus giving evidence that the known exponential time algorithms are justified, but approximation algorithms or simplifications are necessary.Keywords: Elastic image matching; Two-dimensional warping; NP-completeness 1. IntroductionIn image recognition, a common problem is to match two given images, e.g. when comparing an observed image to given references. In that pro-cess, elastic image matching, two-dimensional (2D-)warping (Uchida and Sakoe, 1998) or similar types of invariant methods (Keysers et al., 2000) can be used. For this purpose, we can define cost functions depending on the distortion introduced in the matching andsearch for the best matching with respect to a given cost function. In this paper, we show that it is an algorithmically hard problem to decide whether a matching between two images exists with costs below a given threshold. We show that the problem image matching is NP-complete by means of a reduction from 3-SAT, which is a common method of demonstrating a problem to be intrinsically hard (Garey and Johnson, 1979). This result shows the inherent computational difficulties in this type of image comparison, while interestingly the same problem is solvable for 1D sequences in polynomial time, e.g. the dynamic time warping problem in speech recognition (see e.g. Ney et al., 1992). This has the following implications: researchers who are interested in an exact solution to this problem cannot hope to find a polynomial time algorithm, unless P=NP. Furthermore, one can conclude that exponential time algorithms as presented and extended by Uchida and Sakoe (1998, 1999a,b, 2000a,b) may be justified for some image matching applications. On the other hand this shows that those interested in faster algorithms––e.g. for pattern recognition purposes––are right in searching for sub-optimal solutions. One method to do this is the restriction to local optimizations or linear approximations of global transformations as presented in (Keysers et al., 2000). Another possibility is to use heuristic approaches like simulated annealing or genetic algorithms to find an approximate solution. Furthermore, methods like beam search are promising candidates, as these are used successfully in speech recognition, although linguistic decoding is also an NP-complete problem (Casacuberta and de la Higuera, 1999). 2. Image matchingAmong the varieties of matching algorithms,we choose the one presented by Uchida and Sakoe(1998) as a starting point to formalize the problem image matching. Let the images be given as(without loss of generality) square grids of size M×M with gray values (respectively node labels)from a finite alphabet &={1,…,G}. To define thed:&×&→N , problem, two distance functions are needed,one acting on gray valuesg measuring the match in gray values, and one acting on displacement differences :Z×Z→N , measuring the distortion introduced by t he matching. For these distance ddfunctions we assume that they are monotonous functions (computable in polynomial time) of the commonly used squared Euclid-ean distance, i.ed g (g 1,g 2)=f 1(||g 1-g 2||²)and d d (z)=f 2(||z||²) monotonously increasing. Now we call the following optimization problem the image matching problem (let µ={1,…M} ).Instance: The pair( A ; B ) of two images A and B of size M×M .Solution: A mapping function f :µ×µ→µ×µ.Measure:c (A,B,f )=),(),(j i f ij g B Ad ∑μμ⨯∈),(j i+∑⨯-⋅⋅⋅∈+-+μ}1,{1,),()))0,1(),(())0,1(),(((M j i d j i f j i f dμ⨯-⋅⋅⋅∈}1,{1,),(M j i +∑⋅⋅⋅⨯∈+-+1}-M ,{1,),()))1,0(),(())1,0(),(((μj i d j i f j i f d 1}-M ,{1,),(⋅⋅⋅⨯∈μj iGoal:min f c(A,B,f).In other words, the problem is to find the mapping from A onto B that minimizes the distance between the mapped gray values together with a measure for the distortion introduced by the mapping. Here, the distortion is measured by the deviation from the identity mapping in the two dimensions. The identity mapping fulfills f(i,j)=(i,j),and therefore ,f((i,j)+(x,y))=f(i,j)+(x,y)The corresponding decision problem is fixed by the followingQuestion:Given an instance of image matching and a cost c′, does there exist a ma pping f such that c(A,B,f)≤c′?In the definition of the problem some care must be taken concerning the distance functions. For example, if either one of the distance functions is a constant function, the problem is clearly in P (for d g constant, the minimum is given by the identity mapping and for d d constant, the minimum can be determined by sorting all possible matching for each pixel by gray value cost and mapping to one of the pixels with minimum cost). But these special cases are not those we are concerned with in image matching in general.We choose the matching problem of Uchida and Sakoe (1998) to complete the definition of the problem. Here, the mapping functions are restricted by continuity and monotonicity constraints: the deviations from the identity mapping may locally be at most one pixel (i.e. limited to the eight-neighborhood with squared Euclidean distance less than or equal to 2). This can be formalized in this approach bychoosing the functions f1,f2as e.g.f 1=id,f2(x)=step(x):=⎩⎨⎧.2,)10(,2,0>≤⋅xGxMM3. Reduction from 3-SAT3-SAT is a very well-known NP-complete problem (Garey and Johnson, 1979), where 3-SAT is defined as follows:Instance: Collection of clauses C={C1,···,CK} on a set of variables X={x1, (x)L}such that each ckconsists of 3 literals for k=1,···K .Each literal is a variable or the negation of a variable.Question:Is there a truth assignment for X which satisfies each clause ck, k=1,···K ?The dependency graph D(Ф)corresponding to an instance Ф of 3-SAT is defined to be the bipartite graph whose independent sets are formed by the set of clauses Cand the set of variables X .Two vert ices ck and x1are adjacent iff ckinvolvesx 1or-xL.Given any 3-SAT formula U, we show how to construct in polynomial time anequivalent image matching problem l(Ф)=(A(Ф),B(Ф)); . The two images of l (Ф)are similar according to the cost function (i.e.f:c(A(Ф),B(Ф),f)≤0) iff the formulaФ is satisfiable. We perform the reduction from 3-SAT using the following steps:• From the formula Ф we construct the dependency graph D(Ф).• The dependency graph D(Ф)is drawn in the plane.• The drawing of D(Ф)is refined to depict the logical behaviour of Ф , yielding two images(A(Ф),B(Ф)).For this, we use three types of components: one component to represent variables of Ф , one component to represent clauses of Ф, and components which act as interfaces between the former two types. Before we give the formal reduction, we introduce these components.3.1. Basic componentsFor the reduction from 3-SAT we need five components from which we will construct the in-stances for image matching , given a Boolean formula in 3-DNF,respectively its graph. The five components are the building blocks needed for the graph drawing and will be introduced in the following, namely the representations of connectors,crossings, variables, and clauses. The connectors represent the edges and have two varieties, straight connectors and corner connectors. Each of the components consists of two parts, one for image A and one for image B , where blank pixels are considered to be of the‘background ’color.We will depict possible mappings in the following using arrows indicating the direction of displacement (where displacements within the eight-neighborhood of a pixel are the only cases considered). Blank squares represent mapping to the respective counterpart in the second image.For example, the following displacements of neighboring pixels can be used with zero cost:On the other hand, the following displacements result in costs greater than zero:Fig. 1 shows the first component, the straight connector component, which consists of a line of two different interchanging colors,here denoted by the two symbols◇and□. Given that the outside pixels are mapped to their respe ctive counterparts and the connector is continued infinitely, there are two possible ways in which the colored pixels can be mapped, namely to the left (i.e. f(2,j)=(2,j-1)) or to the right (i.e. f(2,j)=(2,j+1)),where the background pixels have different possibilities for the mapping, not influencing the main property of the connector. This property, which justifies the name ‘connector ’, is the following: It is not possible to find a mapping, which yields zero cost where the relative displacements of the connector pixels are not equal, i.e. one always has f(2,j)-(2,j)=f(2,j')-(2,j'),which can easily be observed by induction over j'.That is, given an initial displacement of one pixel (which will be ±1 in this context), the remaining end of the connector has the same displacement if overall costs of the mapping are zero. Given this property and the direction of a connector, which we define to be directed from variable to clause, wecan define the state of the connector as carrying the‘true’truth value, if the displacement is 1 pixel in the direction of the connector and as carrying the‘false’ truth value, if the displacement is -1 pixel in the direction of the connector. This property then ensures that the truth value transmitted by the connector cannot change at mappings of zero cost.Image A image Bmapping 1 mapping 2Fig. 1. The straight connector component with two possible zero cost mappings.For drawing of arbitrary graphs, clearly one also needs corners,which are represented in Fig. 2.By considering all possible displacements which guarantee overall cost zero, one can observe that the corner component also ensures the basic connector property. For example, consider the first depicted mapping, which has zero cost. On the other hand, the second mapping shows, that it is not possible to construct a zero cost mapping with both connectors‘leaving’the component. In that case, the pixel at the position marked‘? ’either has a conflict (that i s, introduces a cost greater than zero in the criterion function because of mapping mismatch) with the pixel above or to the right of it,if the same color is to be met and otherwise, a cost in the gray value mismatch term is introduced.image A image Bmapping 1 mapping 2Fig. 2. The corner connector component and two example mappings.Fig. 3 shows the variable component, in this case with two positive (to the left) and one negated output (to the right) leaving the component as connectors. Here, a fourth color is used, denoted by ·.This component has two possible mappings for thecolored pixels with zero cost, which map the vertical component of the source image to the left or the right vertical component in the target image, respectively. (In both cases the second vertical element in the target image is not a target of the mapping.) This ensures±1 pixel relative displacements at the entry to the connectors. This property again can be deducted by regarding all possible mappings of the two images.The property that follows (which is necessary for the use as variable) is that all zero cost mappings ensure that all positive connectors carry the same truth value,which is the opposite of the truth value for all the negated connectors. It is easy to see from this example how variable components for arbitrary numbers of positive and negated outputs can be constructed.image A image BImage C image DFig. 3. The variable component with two positive and one negated output and two possible mappings (for true and false truth value).Fig. 4 shows the most complex of the components, the clause component. This component consists of two parts. The first part is the horizontal connector with a 'bend' in it to the right.This part has the property that cost zero mappings are possible for all truth values of x and y with the exception of two 'false' values. This two input disjunction,can be extended to a three input dis-junction using the part in the lower left. If the z connector carries a 'false' truth value, this part can only be mapped one pixel downwards at zero cost.In that case the junction pixel (the fourth pixel in the third row) cannot be mapped upwards at zero cost and the 'two input clause' behaves as de-scribed above. On the other hand, if the z connector carries a 'true' truth value, this part can only be mapped one pixel upwards at zero cost,and the junction pixel can be mapped upwards,thus allowing both x and y to carry a 'false' truth value in a zero cost mapping. Thus there exists a zero cost mapping of the clause component iff at least one of the input connectors carries a truth value.image Aimage B mapping 1(true,true,false)mapping 2 (false,false,true,)Fig. 4. The clause component with three incoming connectors x, y , z and zero cost mappings forthe two cases(true,true,false)and (false, false, true).The described components are already sufficient to prove NP-completeness by reduction from planar 3-SAT (which is an NP-complete sub-problem of 3-SAT where the additional constraints on the instances is that the dependency graph is planar),but in order to derive a reduction from 3-SAT, we also include the possibility of crossing connectors.Fig. 5 shows the connector crossing, whose basic property is to allow zero cost mappings if the truth–values are consistently propagated. This is assured by a color change of the vertical connector and a 'flexible' middle part, which can be mapped to four different positions depending on the truth value distribution.image Aimage Bzero cost mappingFig. 5. The connector crossing component and one zero cost mapping.3.2. ReductionUsing the previously introduced components, we can now perform the reduction from 3-SAT to image matching .Proof of the claim that the image matching problem is NP-complete:Clearly, the image matching problem is in NP since, given a mapping f and two images A and B ,the computation of c(A,B,f)can be done in polynomial time. To prove NP-hardness, we construct a reduction from the 3-SAT problem. Given an instance of 3-SAT we construct two images A and B , for which a mapping of cost zero exists iff all the clauses can be satisfied.Given the dependency graph D ,we construct an embedding of the graph into a 2D pixel grid, placing the vertices on a large enough distance from each other (say100(K+L)² ).This can be done using well-known methods from graph drawing (see e.g.di Battista et al.,1999).From this image of the graph D we construct the two images A and B , using the components described above.Each vertex belonging to a variable is replaced with the respective parts of the variable component, having a number of leaving connectors equal to the number of incident edges under consideration of the positive or negative use in the respective clause. Each vertex belonging to a clause is replaced by the respective clause component,and each crossing of edges is replaced by the respective crossing component. Finally, all the edges are replaced with connectors and corner connectors, and the remaining pixels inside the rectangular hull of the construction are set to the background gray value. Clearly, the placement of the components can be done in such a way that all the components are at a large enough distance from each other, where the background pixels act as an 'insulation' against mapping of pixels, which do not belong to the same component. It can be easily seen, that the size of the constructed images is polynomial with respect to the number of vertices and edges of D and thus polynomial in the size of the instance of 3-SAT, at most in the order (K+L)².Furthermore, it can obviously be constructed in polynomial time, as the corresponding graph drawing algorithms are polynomial.Let there exist a truth assignment to the variables x1,…,xL, which satisfies allthe clauses c1,…,cK. We construct a mapping f , that satisfies c(f,A,B)=0 asfollows.For all pixels (i, j ) belonging to variable component l with A(i,j)not of the background color,set f(i,j)=(i,j-1)if xlis assigned the truth value 'true' , set f(i,j)=(i,j+1), otherwise. For the remaining pixels of the variable component set A(i,j)=B(i,j),if f(i,j)=(i,j), otherwise choose f(i,j)from{(i,j+1),(i+1,j+1),(i-1,j+1)}for xl'false' respectively from {(i,j-1),(i+1,j-1),(i-1,j-1)}for xl'true ',such that A(i,j)=B(f(i,j)). This assignment is always possible and has zero cost, as can be easily verified.For the pixels(i,j)belonging to (corner) connector components,the mapping function can only be extended in one way without the introduction of nonzero cost,starting from the connection with the variable component. This is ensured by thebasic connector property. By choosing f (i ,j )=(i,j )for all pixels of background color, we obtain a valid extension for the connectors. For the connector crossing components the extension is straight forward, although here ––as in the variable mapping ––some care must be taken with the assign ment of the background value pixels, but a zero cost assignment is always possible using the same scheme as presented for the variable mapping.It remains to be shown that the clause components can be mapped at zero cost, if at least one of the input connectors x , y , z carries a ' true' truth value.For a proof we regard alls even possibilities and construct a mapping for each case. In thedescription of the clause component it was already argued that this is possible,and due to space limitations we omit the formalization of the argument here.Finally, for all the pixels (i ,j )not belonging to any of the components, we set f (i ,j )=(i ,j )thus arriving at a mapping function which has c (f ,A ,B )=0。
外文文献及翻译
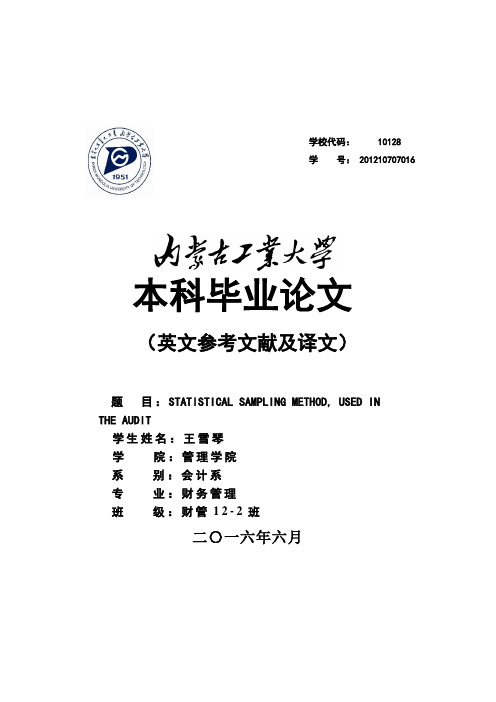
((英文参考文献及译文)二〇一六年六月本科毕业论文 题 目:STATISTICAL SAMPLING METHOD, USED INTHE AUDIT学生姓名:王雪琴学 院:管理学院系 别:会计系专 业:财务管理班 级:财管12-2班 学校代码: 10128 学 号: 201210707016Statistics and AuditRomanian Statistical Review nr. 5 / 2010STATISTICAL SAMPLING METHOD, USED IN THE AUDIT - views, recommendations, fi ndingsPhD Candidate Gabriela-Felicia UNGUREANUAbstractThe rapid increase in the size of U.S. companies from the earlytwentieth century created the need for audit procedures based on the selectionof a part of the total population audited to obtain reliable audit evidence, tocharacterize the entire population consists of account balances or classes oftransactions. Sampling is not used only in audit – is used in sampling surveys,market analysis and medical research in which someone wants to reach aconclusion about a large number of data by examining only a part of thesedata. The difference is the “population” from which the sample is selected, iethat set of data which is intended to draw a conclusion. Audit sampling appliesonly to certain types of audit procedures.Key words: sampling, sample risk, population, sampling unit, tests ofcontrols, substantive procedures.Statistical samplingCommittee statistical sampling of American Institute of CertifiedPublic Accountants of (AICPA) issued in 1962 a special report, titled“Statistical sampling and independent auditors’ which allowed the use ofstatistical sampling method, in accordance with Generally Accepted AuditingStandards (GAAS). During 1962-1974, the AICPA published a series of paperson statistical sampling, “Auditor’s Approach to Statistical Sampling”, foruse in continuing professional education of accountants. During 1962-1974,the AICPA published a series of papers on statistical sampling, “Auditor’sApproach to Statistical Sampling”, for use in continuing professional educationof accountants. In 1981, AICPA issued the professional standard, “AuditSampling”, which provides general guidelines for both sampling methods,statistical and non-statistical.Earlier audits included checks of all transactions in the period coveredby the audited financial statements. At that time, the literature has not givenparticular attention to this subject. Only in 1971, an audit procedures programprinted in the “Federal Reserve Bulletin (Federal Bulletin Stocks)” includedseveral references to sampling such as selecting the “few items” of inventory.Statistics and Audit The program was developed by a special committee, which later became the AICPA, that of Certified Public Accountants American Institute.In the first decades of last century, the auditors often applied sampling, but sample size was not in related to the efficiency of internal control of the entity. In 1955, American Institute of Accountants has published a study case of extending the audit sampling, summarizing audit program developed by certified public accountants, to show why sampling is necessary to extend the audit. The study was important because is one of the leading journal on sampling which recognize a relationship of dependency between detail and reliability testing of internal control.In 1964, the AICPA’s Auditing Standards Board has issued a report entitled “The relationship between statistical sampling and Generally Accepted Auditing Standards (GAAS)” which illustrated the relationship between the accuracy and reliability in sampling and provisions of GAAS.In 1978, the AICPA published the work of Donald M. Roberts,“Statistical Auditing”which explains the underlying theory of statistical sampling in auditing.In 1981, AICPA issued the professional standard, named “Audit Sampling”, which provides guidelines for both sampling methods, statistical and non-statistical.An auditor does not rely solely on the results of a single procedure to reach a conclusion on an account balance, class of transactions or operational effectiveness of the controls. Rather, the audit findings are based on combined evidence from several sources, as a consequence of a number of different audit procedures. When an auditor selects a sample of a population, his objective is to obtain a representative sample, ie sample whose characteristics are identical with the population’s characteristics. This means that selected items are identical with those remaining outside the sample.In practice, auditors do not know for sure if a sample is representative, even after completion the test, but they “may increase the probability that a sample is representative by accuracy of activities made related to design, sample selection and evaluation” [1]. Lack of specificity of the sample results may be given by observation errors and sampling errors. Risks to produce these errors can be controlled.Observation error (risk of observation) appears when the audit test did not identify existing deviations in the sample or using an inadequate audit technique or by negligence of the auditor.Sampling error (sampling risk) is an inherent characteristic of the survey, which results from the fact that they tested only a fraction of the total population. Sampling error occurs due to the fact that it is possible for Revista Română de Statistică nr. 5 / 2010Statistics and Auditthe auditor to reach a conclusion, based on a sample that is different from the conclusion which would be reached if the entire population would have been subject to audit procedures identical. Sampling risk can be reduced by adjusting the sample size, depending on the size and population characteristics and using an appropriate method of selection. Increasing sample size will reduce the risk of sampling; a sample of the all population will present a null risk of sampling.Audit Sampling is a method of testing for gather sufficient and appropriate audit evidence, for the purposes of audit. The auditor may decide to apply audit sampling on an account balance or class of transactions. Sampling audit includes audit procedures to less than 100% of the items within an account balance or class of transactions, so all the sample able to be selected. Auditor is required to determine appropriate ways of selecting items for testing. Audit sampling can be used as a statistical approach and a non- statistical.Statistical sampling is a method by which the sample is made so that each unit consists of the total population has an equal probability of being included in the sample, method of sample selection is random, allowed to assess the results based on probability theory and risk quantification of sampling. Choosing the appropriate population make that auditor’ findings can be extended to the entire population.Non-statistical sampling is a method of sampling, when the auditor uses professional judgment to select elements of a sample. Since the purpose of sampling is to draw conclusions about the entire population, the auditor should select a representative sample by choosing sample units which have characteristics typical of that population. Results will not extrapolate the entire population as the sample selected is representative.Audit tests can be applied on the all elements of the population, where is a small population or on an unrepresentative sample, where the auditor knows the particularities of the population to be tested and is able to identify a small number of items of interest to audit. If the sample has not similar characteristics for the elements of the entire population, the errors found in the tested sample can not extrapolate.Decision of statistical or non-statistical approach depends on the auditor’s professional judgment which seeking sufficient appropriate audits evidence on which to completion its findings about the audit opinion.As a statistical sampling method refer to the random selection that any possible combination of elements of the community is equally likely to enter the sample. Simple random sampling is used when stratification was not to audit. Using random selection involves using random numbers generated byRomanian Statistical Review nr. 5 / 2010Statistics and Audit a computer. After selecting a random starting point, the auditor found the first random number that falls within the test document numbers. Only when the approach has the characteristics of statistical sampling, statistical assessments of risk are valid sampling.In another variant of the sampling probability, namely the systematic selection (also called random mechanical) elements naturally succeed in office space or time; the auditor has a preliminary listing of the population and made the decision on sample size. “The auditor calculated a counting step, and selects the sample element method based on step size. Step counting is determined by dividing the volume of the community to sample the number of units desired. Advantages of systematic screening are its usability. In most cases, a systematic sample can be extracted quickly and method automatically arranges numbers in successive series.”[2].Selection by probability proportional to size - is a method which emphasizes those population units’recorded higher values. The sample is constituted so that the probability of selecting any given element of the population is equal to the recorded value of the item;Stratifi ed selection - is a method of emphasis of units with higher values and is registered in the stratification of the population in subpopulations. Stratification provides a complete picture of the auditor, when population (data table to be analyzed) is not homogeneous. In this case, the auditor stratifies a population by dividing them into distinct subpopulations, which have common characteristics, pre-defined. “The objective of stratification is to reduce the variability of elements in each layer and therefore allow a reduction in sample size without a proportionate increase in the risk of sampling.” [3] If population stratification is done properly, the amount of sample size to come layers will be less than the sample size that would be obtained at the same level of risk given sample with a sample extracted from the entire population. Audit results applied to a layer can be designed only on items that are part of that layer.I appreciated as useful some views on non-statistical sampling methods, which implies that guided the selection of the sample selecting each element according to certain criteria determined by the auditor. The method is subjective; because the auditor selects intentionally items containing set features him.The selection of the series is done by selecting multiple elements series (successive). Using sampling the series is recommended only if a reasonable number of sets used. Using just a few series there is a risk that the sample is not representative. This type of sampling can be used in addition to other samples, where there is a high probability of occurrence of errors. At the arbitrary selection, no items are selected preferably from the auditor, Revista Română de Statistică nr. 5 / 2010Statistics and Auditthat regardless of size or source or characteristics. Is not the recommended method, because is not objective.That sampling is based on the auditor’s professional judgment, which may decide which items can be part or not sampled. Because is not a statistical method, it can not calculate the standard error. Although the sample structure can be constructed to reproduce the population, there is no guarantee that the sample is representative. If omitted a feature that would be relevant in a particular situation, the sample is not representative.Sampling applies when the auditor plans to make conclusions about population, based on a selection. The auditor considers the audit program and determines audit procedures which may apply random research. Sampling is used by auditors an internal control systems testing, and substantive testing of operations. The general objectives of tests of control system and operations substantive tests are to verify the application of pre-defined control procedures, and to determine whether operations contain material errors.Control tests are intended to provide evidence of operational efficiency and controls design or operation of a control system to prevent or detect material misstatements in financial statements. Control tests are necessary if the auditor plans to assess control risk for assertions of management.Controls are generally expected to be similarly applied to all transactions covered by the records, regardless of transaction value. Therefore, if the auditor uses sampling, it is not advisable to select only high value transactions. Samples must be chosen so as to be representative population sample.An auditor must be aware that an entity may change a special control during the course of the audit. If the control is replaced by another, which is designed to achieve the same specific objective, the auditor must decide whether to design a sample of all transactions made during or just a sample of transactions controlled again. Appropriate decision depends on the overall objective of the audit test.Verification of internal control system of an entity is intended to provide guidance on the identification of relevant controls and design evaluation tests of controls.Other tests:In testing internal control system and testing operations, audit sample is used to estimate the proportion of elements of a population containing a characteristic or attribute analysis. This proportion is called the frequency of occurrence or percentage of deviation and is equal to the ratio of elements containing attribute specific and total number of population elements. WeightRomanian Statistical Review nr. 5 / 2010Statistics and Audit deviations in a sample are determined to calculate an estimate of the proportion of the total population deviations.Risk associated with sampling - refers to a sample selection which can not be representative of the population tested. In other words, the sample itself may contain material errors or deviations from the line. However, issuing a conclusion based on a sample may be different from the conclusion which would be reached if the entire population would be subject to audit.Types of risk associated with sampling:Controls are more effective than they actually are or that there are not significant errors when they exist - which means an inappropriate audit opinion. Controls are less effective than they actually are that there are significant errors when in fact they are not - this calls for additional activities to establish that initial conclusions were incorrect.Attributes testing - the auditor should be defining the characteristics to test and conditions for misconduct. Attributes testing will make when required objective statistical projections on various characteristics of the population. The auditor may decide to select items from a population based on its knowledge about the entity and its environment control based on risk analysis and the specific characteristics of the population to be tested.Population is the mass of data on which the auditor wishes to generalize the findings obtained on a sample. Population will be defined compliance audit objectives and will be complete and consistent, because results of the sample can be designed only for the population from which the sample was selected.Sampling unit - a unit of sampling may be, for example, an invoice, an entry or a line item. Each sample unit is an element of the population. The auditor will define the sampling unit based on its compliance with the objectives of audit tests.Sample size - to determine the sample size should be considered whether sampling risk is reduced to an acceptable minimum level. Sample size is affected by the risk associated with sampling that the auditor is willing to accept it. The risk that the auditor is willing to accept lower, the sample will be higher.Error - for detailed testing, the auditor should project monetary errors found in the sample population and should take into account the projected error on the specific objective of the audit and other audit areas. The auditor projects the total error on the population to get a broad perspective on the size of the error and comparing it with tolerable error.For detailed testing, tolerable error is tolerable and misrepresentations Revista Română de Statistică nr. 5 / 2010Statistics and Auditwill be a value less than or equal to materiality used by the auditor for the individual classes of transactions or balances audited. If a class of transactions or account balances has been divided into layers error is designed separately for each layer. Design errors and inconsistent errors for each stratum are then combined when considering the possible effect on the total classes of transactions and account balances.Evaluation of sample results - the auditor should evaluate the sample results to determine whether assessing relevant characteristics of the population is confirmed or needs to be revised.When testing controls, an unexpectedly high rate of sample error may lead to an increase in the risk assessment of significant misrepresentation unless it obtained additional audit evidence to support the initial assessment. For control tests, an error is a deviation from the performance of control procedures prescribed. The auditor should obtain evidence about the nature and extent of any significant changes in internal control system, including the staff establishment.If significant changes occur, the auditor should review the understanding of internal control environment and consider testing the controls changed. Alternatively, the auditor may consider performing substantive analytical procedures or tests of details covering the audit period.In some cases, the auditor might not need to wait until the end audit to form a conclusion about the effectiveness of operational control, to support the control risk assessment. In this case, the auditor might decide to modify the planned substantive tests accordingly.If testing details, an unexpectedly large amount of error in a sample may cause the auditor to believe that a class of transactions or account balances is given significantly wrong in the absence of additional audit evidence to show that there are not material misrepresentations.When the best estimate of error is very close to the tolerable error, the auditor recognizes the risk that another sample have different best estimate that could exceed the tolerable error.ConclusionsFollowing analysis of sampling methods conclude that all methods have advantages and disadvantages. But the auditor is important in choosing the sampling method is based on professional judgment and take into account the cost / benefit ratio. Thus, if a sampling method proves to be costly auditor should seek the most efficient method in view of the main and specific objectives of the audit.Romanian Statistical Review nr. 5 / 2010Statistics and Audit The auditor should evaluate the sample results to determine whether the preliminary assessment of relevant characteristics of the population must be confirmed or revised. If the evaluation sample results indicate that the relevant characteristics of the population needs assessment review, the auditor may: require management to investigate identified errors and likelihood of future errors and make necessary adjustments to change the nature, timing and extent of further procedures to take into account the effect on the audit report.Selective bibliography:[1] Law no. 672/2002 updated, on public internal audit[2] Arens, A şi Loebbecke J - Controve …Audit– An integrate approach”, 8th edition, Arc Publishing House[3] ISA 530 - Financial Audit 2008 - International Standards on Auditing, IRECSON Publishing House, 2009- Dictionary of macroeconomics, Ed C.H. Beck, Bucharest, 2008Revista Română de Statistică nr. 5 / 2010Statistics and Audit摘要美国公司的规模迅速增加,从第二十世纪初创造了必要的审计程序,根据选定的部分总人口的审计,以获得可靠的审计证据,以描述整个人口组成的帐户余额或类别的交易。
医学影像学英文文献

医学影像学英文文献英文回答:Within the realm of medical imaging, sophisticated imaging techniques empower healthcare professionals with the ability to visualize and comprehend anatomical structures and physiological processes in the human body. These techniques are instrumental in diagnosing diseases, guiding therapeutic interventions, and monitoring treatment outcomes.Computed tomography (CT) and magnetic resonance imaging (MRI) are two cornerstone imaging modalities widely employed in medical practice. CT utilizes X-rays and advanced computational algorithms to generate cross-sectional images of the body, providing detailed depictions of bones, soft tissues, and blood vessels. MRI, on the other hand, harnesses the power of powerful magnets and radiofrequency waves to create intricate images that excel in showcasing soft tissue structures, including the brain,spinal cord, and internal organs.Positron emission tomography (PET) and single-photon emission computed tomography (SPECT) are nuclear medicine imaging techniques that involve the administration of radioactive tracers into the body. These tracers accumulate in specific organs or tissues, enabling the visualization and assessment of metabolic processes and disease activity. PET is particularly valuable in oncology, as it can detect the presence and extent of cancerous lesions.Ultrasound, also known as sonography, utilizes high-frequency sound waves to produce images of internal structures. It is a versatile technique commonly employed in obstetrics, cardiology, and abdominal imaging. Ultrasound offers real-time visualization, making it ideal for guiding procedures such as biopsies and injections.Interventional radiology is a specialized field that combines imaging guidance with minimally invasive procedures. Interventional radiologists utilize imaging techniques to precisely navigate catheters and otherinstruments through the body, enabling the diagnosis and treatment of conditions without the need for open surgery. This approach offers reduced invasiveness and faster recovery times compared to traditional surgical interventions.Medical imaging has revolutionized healthcare by providing invaluable insights into the human body. The ability to visualize anatomical structures andphysiological processes in exquisite detail has transformed the practice of medicine, leading to more accurate diagnoses, targeted treatments, and improved patient outcomes.中文回答:医学影像学是现代医学不可或缺的一部分,它利用各种成像技术对人体的解剖结构和生理过程进行可视化和理解,在疾病诊断、治疗方案制定和治疗效果评估中发挥着至关重要的作用。
A Threshold Selection Method from Gray-Level Histograms图像分割经典论文翻译(部分)

A Threshold Selection Method from Gray-Level Histograms[1][1]Otsu N, A threshold selection method from gray-level histogram. IEEE Transactions on System,Man,and Cybemetics,SMC-8,1978:62-66.一种由灰度直方图选取阈值的方法摘要介绍了一种对于画面分割自动阈值选择的非参数和无监督的方法。
最佳阈值由判别标准选择,即最大化通过灰度级所得到的类的方差。
这个过程很简单,是利用了灰度直方图0阶和第1阶的累积。
这是简单的方法扩展到多阈值的问题。
几种实验结果呈现也支持了方法的有效性。
一.简介选择灰度充分的阈值,从图片的背景中提取对象对于图像处理非常重要。
在这方面已经提出了多种技术。
在理想的情况下,直方图具有分别表示对象和背景的能力,两个峰之间有很深的明显的谷,使得阈值可以选择这个谷底。
然而,对于大多数实际图片,它常常难以精确地检测谷底,特别是在这种情况下,当谷是平的和广泛的,具有噪声充满时,或者当两个峰是在高度极其不等,通常不产生可追踪的谷。
已经出现了,为了克服这些困难,提出的一些技术。
它们是,例如,谷锐化技术[2],这个技术限制了直方图与(拉普拉斯或梯度)的衍生物大于绝对值的像素,并且描述了绘制差分直方图方法[3],选择灰度级的阈值与差的最大值。
这些利用在原始图象有关的信息的相邻像素(或边缘),修改直方图以便使其成为阈值是有用的。
另一类方法与参数方法的灰度直方图直接相关。
例如,该直方图在最小二乘意义上与高斯分布的总和近似,应用了统计决策程序 [4]。
然而,这种方法需要相当繁琐,有时不稳定的计算。
此外,在许多情况下,高斯分布与真实模型的近似值较小。
在任何情况下,没有一个阈值的评估标准能够对大多数的迄今所提出的方法进行评价。
这意味着,它可能是派生的最佳阈值方法来建立一个适当的标准,从更全面的角度评估阈值的“好与坏”的正确方法。
图像去噪 英文文献及翻译
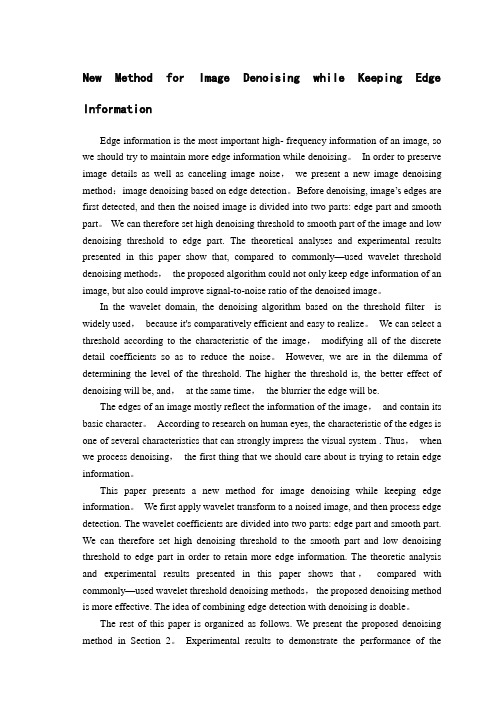
New Method for Image Denoising while Keeping Edge InformationEdge information is the most important high- frequency information of an image, so we should try to maintain more edge information while denoising。
In order to preserve image details as well as canceling image noise,we present a new image denoising method:image denoising based on edge detection。
Before denoising, image’s edges are first detected, and then the noised image is divided into two parts: edge part and smooth part。
We can therefore set high denoising threshold to smooth part of the image and low denoising threshold to edge part. The theoretical analyses and experimental results presented in this paper show that, compared to commonly—used wavelet threshold denoising methods,the proposed algorithm could not only keep edge information of an image, but also could improve signal-to-noise ratio of the denoised image。
目标检测参考文献
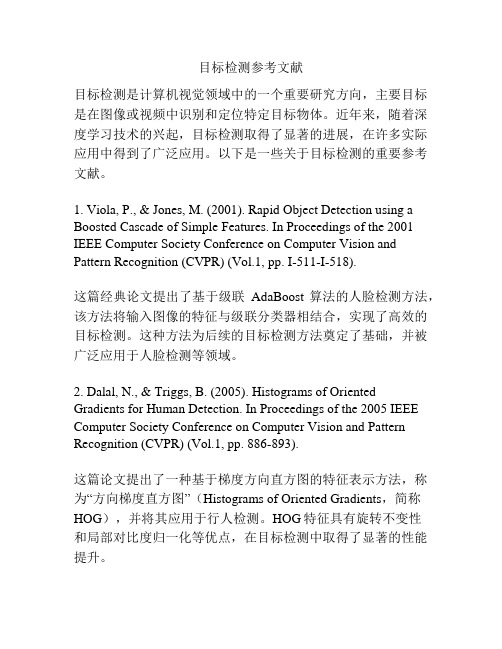
目标检测参考文献目标检测是计算机视觉领域中的一个重要研究方向,主要目标是在图像或视频中识别和定位特定目标物体。
近年来,随着深度学习技术的兴起,目标检测取得了显著的进展,在许多实际应用中得到了广泛应用。
以下是一些关于目标检测的重要参考文献。
1. Viola, P., & Jones, M. (2001). Rapid Object Detection using a Boosted Cascade of Simple Features. In Proceedings of the 2001 IEEE Computer Society Conference on Computer Vision and Pattern Recognition (CVPR) (Vol.1, pp. I-511-I-518).这篇经典论文提出了基于级联AdaBoost算法的人脸检测方法,该方法将输入图像的特征与级联分类器相结合,实现了高效的目标检测。
这种方法为后续的目标检测方法奠定了基础,并被广泛应用于人脸检测等领域。
2. Dalal, N., & Triggs, B. (2005). Histograms of Oriented Gradients for Human Detection. In Proceedings of the 2005 IEEE Computer Society Conference on Computer Vision and Pattern Recognition (CVPR) (Vol.1, pp. 886-893).这篇论文提出了一种基于梯度方向直方图的特征表示方法,称为“方向梯度直方图”(Histograms of Oriented Gradients,简称HOG),并将其应用于行人检测。
HOG特征具有旋转不变性和局部对比度归一化等优点,在目标检测中取得了显著的性能提升。
- 1、下载文档前请自行甄别文档内容的完整性,平台不提供额外的编辑、内容补充、找答案等附加服务。
- 2、"仅部分预览"的文档,不可在线预览部分如存在完整性等问题,可反馈申请退款(可完整预览的文档不适用该条件!)。
- 3、如文档侵犯您的权益,请联系客服反馈,我们会尽快为您处理(人工客服工作时间:9:00-18:30)。
图像检测外文翻译参考文献(文档含中英文对照即英文原文和中文翻译)译文基于半边脸的人脸检测概要:图像中的人脸检测是人脸识别研究中一项非常重要的研究分支。
为了更有效地检测图像中的人脸,此次研究设计提出了基于半边脸的人脸检测方法。
根据图像中人半边脸的容貌或者器官的密度特征,比如眼睛,耳朵,嘴巴,部分脸颊,正面的平均全脸模板就可以被构建出来。
被模拟出来的半张脸是基于人脸的对称性的特点而构建的。
图像中人脸检测的实验运用了模板匹配法和相似性从而确定人脸在图像中的位置。
此原理分析显示了平均全脸模型法能够有效地减少模板的局部密度的不确定性。
基于半边脸的人脸检测能降低人脸模型密度的过度对称性,从而提高人脸检测的速度。
实验结果表明此方法还适用于在大角度拍下的侧脸图像,这大大增加了侧脸检测的准确性。
关键词:人脸模板,半边人脸模板,模板匹配法,相似性,侧脸。
I.介绍近几年,在图像处理和识别以及计算机视觉的研究领域中,人脸识别是一个很热门的话题。
作为人脸识别中一个重要的环节,人脸检测也拥有一个延伸的研究领域。
人脸检测的主要目的是为了确定图像中的信息,比如,图像总是否存在人脸,它的位置,旋转角度以及人脸的姿势。
根据人脸的不同特征,人脸检测的方法也有所变化[1-4]。
而且,根据人脸器官的密度或颜色的固定布局,我们可以判定是否存在人脸。
因此,这种基于肤色模型和模板匹配的方法对于人脸检测具有重要的研究意义[5-7]。
这种基于模板匹配的人脸检测法是选择正面脸部的特征作为匹配的模板,导致人脸搜索的计算量相对较大。
然而,绝大多数的人脸都是对称的。
所以我们可以选择半边正面人脸模板,也就是说,选择左半边脸或者有半边脸作为人脸匹配的模板,这样,大大减少了人脸搜索的计算。
II.人脸模板构建的方法人脸模板的质量直接影响匹配识别的效果。
为了减少模板局部密度的不确定性,构建人脸模板是基于大众脸的信息,例如,平均的眼睛模板,平均的脸型模板。
这种方法很简单。
在模板的仿射变换的实例中,人脸检测的有效性可以被确保。
构建人脸模板的过程如下[8]:步骤一:选择正面人脸图像;步骤二:决定人脸区域的大小和选择人脸区域;步骤三:将选出来的人脸区域格式化成同一种尺寸大小;步骤四:计算人脸区域相对应像素的平均值。
在构建模板之前,挑选些有正面人脸的图片。
首先,决定人脸区域的尺寸大小。
然后,在图像中手动挑选人脸区域。
我们设选定的人脸区域的数量为n。
因为人脸区域的矩阵向量都是被独立分布的,所以在那些人脸图像相同位置的像素值也是独立分布的。
我们设在人脸区域第k(k=1,2,Ă,n)位置的像素值是fk(i,j) (k=1,2,…,n),那些人脸图像的是标准比例系数wk (k=1,2,…,n),由此得出正面人脸模板的表达式:1根据统计学,如果在人脸区域第k个位置,有些像素值fk(i,j)趋于正态分布, 其中u是像素fk(i,j)的平均值,是方差,T(i,j)是正态分布。
所以模板局部密度的不确定性大大降低了。
2如果抽样的人脸图像都是在同一间距下拍摄的,相对应的人脸尺寸是一致的,标准的比例像素wk 就等于1. 那么,大众人脸模板T(i,j)也就变成了III.正面的平均全脸模板的构建在人脸与相机间距相同,鸟瞰图的拍摄角度是15°的情况下,120张人脸图像被取样,包含正面的,左侧倾斜30°和左侧倾斜45°。
每种角度的图像都是40张。
其中20张中的人戴帽子,2张没有戴帽子。
被抽样的图像如图1所示。
正面左侧倾斜30°左侧倾斜45°图1 :各个角度的人脸图像在图像中,正面人脸包括特征器管像眼睛,耳朵,鼻子,部分脸颊等等,如2图2(a)所示。
这些图像的分布特征可以作为检测人脸存在的根据。
所以人的眼睛,耳朵,鼻子,嘴巴和部分脸颊都被选作可以构建整张正面人脸模板的主要区域,如图2(b)所示。
这种方法可以排除异常区域和非人类特有物的影响,比如帽子,胡须等。
图2:人脸特有器官的模型手动取样16张人脸图像。
每张图像都是22 × 26像素。
做为一个比较性的实验,模板不仅要匹配正面图像,还要匹配侧面图像。
所以模板不能太宽。
构建整张正面人脸模板如图3所示。
通过16张正面人脸模板,正面的平均全脸模板就可以被构建出来。
图3:正面全脸的平均模板的构建IV.平均的半脸模板的构建正面的平均全脸模板可以被看做大径相同的左脸模板和有脸模板的结合体。
所以正面的全脸模板可以被中心对称轴分成左脸模板和右脸模板。
所以,半边脸模板的构建如图4所示。
此外,平均半脸模板可以根据平均全脸模板的原理来构建。
这样可以减低在全脸模板中密集度的对称冗余的问题。
方法如图5所示。
图4:构建半脸模板的模型图5:半脸平均模板的构建在一张完美的人脸模板中,左脸和右脸的密集度是对称的,也就是说,两半边脸是相似的一对。
事实上,在一张人脸图像中左脸和右脸存在一些差异,两半边脸的器官密集度的分布也不是完全对称的,所以相似性就降低了。
就拿左半边脸为例,当利用平均的半脸模板搜索人脸图像时,左半边脸会先被识别出来,如图6(a)所示。
图中实线框内是被检测出的左脸,接着根据左脸模板来检测右半边脸的位置。
被检测到的可能是右半边脸的位置如图6(b)所示,这些位置由虚线框标记出来。
图6:被检测出来的可能是半边脸的位置IV. 判别函数在实验中,图像中半边脸被检测出来是运用了模板匹配的方法。
此方法的基本原理解释如下。
被选择的平均半脸模板在被检测的图像上到处搜索。
接着,计算模板与被检测到的半边脸的相似度。
如果在某些位置,相似度方程的值大于阈值,那么我们就认为这班别脸的图像相似于平均半脸模板。
相似度是指图像上局部区域的统计值。
一些不同的子块影像的图像相似度值也许与其他的一样,尽管如此,它们还是属于不同的字块图像。
在实验中,与半脸模板匹配的子块图像所在位置的相似度值应该被保留,而那些未匹配的值应该被剔除。
此方法具体可以描述如下:假设半脸模板T 的长度是I ,宽是J ,如图四所示。
那么全脸模板的长度就是2I ,宽是J 。
假设被检测的图像的长度是L ,宽是W ,当模板为放在(m,n)时,子块图像在图像中相对应的位置为。
于是模板与子块图像S(m,n)的相似度的公式(3) [9]可以表达为上述公式中,判定图像中是否存在半边脸的规则是,给定一个阈值th ,如果S(m,n) th,那么此半边脸相似于子块图像;如果S(m,n)=0,那么此半边脸完全与子块图像一致。
把公式(3)展开后,得到 其中,()[]∑∑==i i j j j i P n m 112,,是子块的能,被位于图像(i, j)位置的半脸模板所覆盖。
在到处搜索图像的时候,它的值变化很慢。
()()j i T j i i i j j n m P,,11,∙∑∑==表示模板T 与子块图像的相关系数。
当模板T 与子块图像完全匹配时,相关系数达到最大值。
()[]∑∑==i i j j j i T 112,是半脸模板T 的能,当半脸模板被构建完后,它的值就被确定了。
它与子块的位置没有任何关系。
所以,模板T 与子块图像的关联系数和子块的能值的比率就是相似度值,如下:简化式子(5),得到式子(6)其中,s(m,n) 是相似度,。
判定半边脸存在的规则如下:给定阈值th ,如果s(m,n) th,那么总结为此半脸模板T 相似于子块图像;如果s(m,n)=1,那么半脸入班T 完全与子块图像匹配。
假设O(T)代表基于半边脸模板检测人脸的时间花费,O(F) 代表基于全脸模板检测人脸的时间花费。
计算方法如下:O(T) = I * J * ( L - I ) * ( W - J ) (7) O(F) = 2I * J * ( L - 2I ) * ( W - J ) (8)O(T) t 与O(F) 的比值如下()()()()()()IL I L J W I L J I J W I L J I F O T O 4222--=-*-**-*-**= (9) 当L 的值大大超过I 值时,方式(9)的值接近1/2,也就是说,基于半边脸模板检测人脸的时间花费是全脸模板方法的1/2。
所以,半边脸模板检测方法可以省一半的时间。
V. 实验结果正如图五显示的平均半脸模板,左脸与左脸,右脸与右脸,左脸与右脸的相似度都是通过式子(6)计算得出。
计算结果分别是1.0000,1.0000 和0.9535。
实验结果是相同半边脸的相似度值很高,不同半边脸是相似的一对。
所以左脸对于右脸的密集度冗余很大。
人脸检测的实验中,利用左半脸模板来检测正面人脸图像,左偏30°和45°的图像。
准确检测的结果如图7显示。
此外,作为对比,同时做了基于平均全脸模板的检测实验,结果如图8所示。
图7:基于半边脸模板的人脸检测实验结果图8:基于平均全脸模板的人脸检测实验结果在人脸检测实验中,准确的人脸检测比率是非常总要的估算准则,描述如下40张正面人脸图像,40张左侧偏离30°的人脸图像,40张左侧偏离45°的人脸图像。
表格1中是被准确检测的人脸的数目和检测比率。
表格1中结果可以总结如下:(1)正面图像的人脸检测准确率很高,因为平均全脸模板是根据一系列的正面人脸图像而构建的,平均半边脸模板是根据平均全脸模板的原理而都建的,这两者都表现了人脸的特征。
(2)当利用平均全脸模板在图像中检测的人脸倾斜,比如左倾斜30°或者45°,检测的准确率就很快地下降了,因为当左脸倾斜时,右脸的很多信息丢失了。
而且在全脸模板里很难找到能够匹配右脸的信息。
(3)当根据平均半边脸模板检测侧脸时,准确率会相对高一点。
主要是因为脸向左侧倾斜时,左脸并没有丢失很多信息,以至于她们可以很好地与平均半脸模板匹配。
然而,人脸偏离的角度过大,检测的准确度也会大大下降,这主要是因为在人脸图像取样的过程中,正脸的成像从三维空间变成了二维空间,这样不仅损失了人脸图像位置的深度信息,而且严重地改变了脸上器官的位置。
所以相似度也随之降低了。
VI.总结平均人脸模板是根据脸部特有的特征这一原理构建的。
人脸位置是根据人脸模板与不同角度的人脸的相似度来判断的。
下面是理论分析和实验的结果:眼睛,耳朵,鼻子,嘴巴和部分脸颊是构建人脸模板的必要部分,它们的密集分布特征是作为人脸检测的依据。
(1)平均人脸模板大大减少了局部特征器官的密集信息的不确定性。
在平均全脸模板的基础上,平均半脸模板能够通过脸上器官位置的对称性构建出来,然后后半边脸就可以直接被检测出来。
人脸模板的密集冗余就大大减少了。
(2)通过基于模板匹配法的人脸检测的时间复杂性的分析,半脸模板相对于全脸模板的价值更适用于实际。
半脸模板能够节省一半的时间,所以检测的数独就可以提高。