外文翻译----数字图像处理与边缘检测
数字图像处理外文翻译参考文献
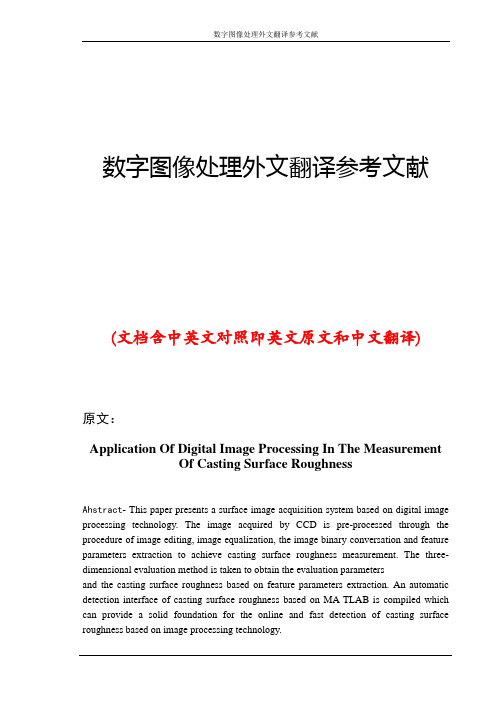
数字图像处理外文翻译参考文献(文档含中英文对照即英文原文和中文翻译)原文:Application Of Digital Image Processing In The MeasurementOf Casting Surface RoughnessAhstract- This paper presents a surface image acquisition system based on digital image processing technology. The image acquired by CCD is pre-processed through the procedure of image editing, image equalization, the image binary conversation and feature parameters extraction to achieve casting surface roughness measurement. The three-dimensional evaluation method is taken to obtain the evaluation parametersand the casting surface roughness based on feature parameters extraction. An automatic detection interface of casting surface roughness based on MA TLAB is compiled which can provide a solid foundation for the online and fast detection of casting surface roughness based on image processing technology.Keywords-casting surface; roughness measurement; image processing; feature parametersⅠ.INTRODUCTIONNowadays the demand for the quality and surface roughness of machining is highly increased, and the machine vision inspection based on image processing has become one of the hotspot of measuring technology in mechanical industry due to their advantages such as non-contact, fast speed, suitable precision, strong ability of anti-interference, etc [1,2]. As there is no laws about the casting surface and the range of roughness is wide, detection parameters just related to highly direction can not meet the current requirements of the development of the photoelectric technology, horizontal spacing or roughness also requires a quantitative representation. Therefore, the three-dimensional evaluation system of the casting surface roughness is established as the goal [3,4], surface roughness measurement based on image processing technology is presented. Image preprocessing is deduced through the image enhancement processing, the image binary conversation. The three-dimensional roughness evaluation based on the feature parameters is performed . An automatic detection interface of casting surface roughness based on MA TLAB is compiled which provides a solid foundation for the online and fast detection of casting surface roughness.II. CASTING SURFACE IMAGE ACQUISITION SYSTEMThe acquisition system is composed of the sample carrier, microscope, CCD camera, image acquisition card and the computer. Sample carrier is used to place tested castings. According to the experimental requirements, we can select a fixed carrier and the sample location can be manually transformed, or select curing specimens and the position of the sampling stage can be changed. Figure 1 shows the whole processing procedure.,Firstly,the detected castings should be placed in the illuminated backgrounds as far as possible, and then through regulating optical lens, setting the CCD camera resolution and exposure time, the pictures collected by CCD are saved to computer memory through the acquisition card. The image preprocessing and feature value extraction on casting surface based on corresponding software are followed. Finally the detecting result is output.III. CASTING SURFACE IMAGE PROCESSINGCasting surface image processing includes image editing, equalization processing, image enhancement and the image binary conversation,etc. The original and clipped images of the measured casting is given in Figure 2. In which a) presents the original image and b) shows the clipped image.A.Image EnhancementImage enhancement is a kind of processing method which can highlight certain image information according to some specific needs and weaken or remove some unwanted informations at the same time[5].In order to obtain more clearly contour of the casting surface equalization processing of the image namely the correction of the image histogram should be pre-processed before image segmentation processing. Figure 3 shows the original grayscale image and equalization processing image and their histograms. As shown in the figure, each gray level of the histogram has substantially the same pixel point and becomes more flat after gray equalization processing. The image appears more clearly after the correction and the contrast of the image is enhanced.Fig.2 Casting surface imageFig.3 Equalization processing imageB. Image SegmentationImage segmentation is the process of pixel classification in essence. It is a very important technology by threshold classification. The optimal threshold is attained through the instmction thresh = graythresh (II). Figure 4 shows the image of the binary conversation. The gray value of the black areas of the Image displays the portion of the contour less than the threshold (0.43137), while the white area shows the gray value greater than the threshold. The shadows and shading emerge in the bright region may be caused by noise or surface depression.Fig4 Binary conversationIV. ROUGHNESS PARAMETER EXTRACTIONIn order to detect the surface roughness, it is necessary to extract feature parameters of roughness. The average histogram and variance are parameters used to characterize the texture size of surface contour. While unit surface's peak area is parameter that can reflect the roughness of horizontal workpiece.And kurtosis parameter can both characterize the roughness of vertical direction and horizontal direction. Therefore, this paper establisheshistogram of the mean and variance, the unit surface's peak area and the steepness as the roughness evaluating parameters of the castings 3D assessment. Image preprocessing and feature extraction interface is compiled based on MATLAB. Figure 5 shows the detection interface of surface roughness. Image preprocessing of the clipped casting can be successfully achieved by this software, which includes image filtering, image enhancement, image segmentation and histogram equalization, and it can also display the extracted evaluation parameters of surface roughness.Fig.5 Automatic roughness measurement interfaceV. CONCLUSIONSThis paper investigates the casting surface roughness measuring method based on digital Image processing technology. The method is composed of image acquisition, image enhancement, the image binary conversation and the extraction of characteristic parameters of roughness casting surface. The interface of image preprocessing and the extraction of roughness evaluation parameters is compiled by MA TLAB which can provide a solid foundation for the online and fast detection of casting surface roughness.REFERENCE[1] Xu Deyan, Lin Zunqi. The optical surface roughness research pro gress and direction[1]. Optical instruments 1996, 18 (1): 32-37.[2] Wang Yujing. Turning surface roughness based on image measurement [D]. Harbin:Harbin University of Science and Technology[3] BRADLEY C. Automated surface roughness measurement[1]. The InternationalJournal of Advanced Manufacturing Technology ,2000,16(9) :668-674.[4] Li Chenggui, Li xing-shan, Qiang XI-FU 3D surface topography measurement method[J]. Aerospace measurement technology, 2000, 20(4): 2-10.[5] Liu He. Digital image processing and application [ M]. China Electric Power Press,2005译文:数字图像处理在铸件表面粗糙度测量中的应用摘要—本文提出了一种表面图像采集基于数字图像处理技术的系统。
图像处理-毕设论文外文翻译(翻译+原文)
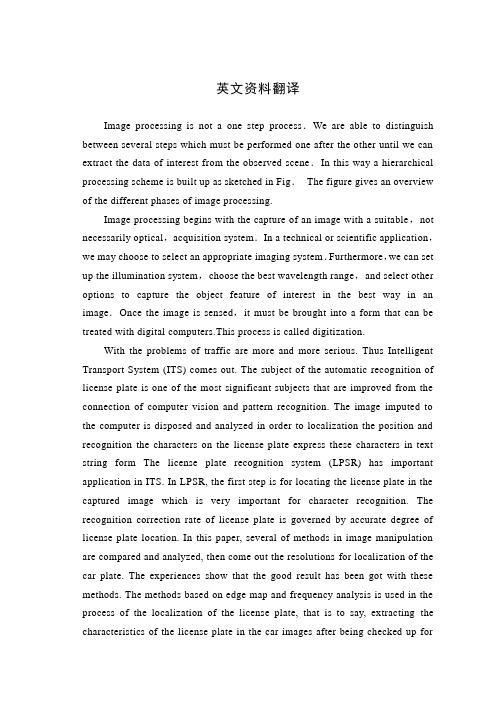
英文资料翻译Image processing is not a one step process.We are able to distinguish between several steps which must be performed one after the other until we can extract the data of interest from the observed scene.In this way a hierarchical processing scheme is built up as sketched in Fig.The figure gives an overview of the different phases of image processing.Image processing begins with the capture of an image with a suitable,not necessarily optical,acquisition system.In a technical or scientific application,we may choose to select an appropriate imaging system.Furthermore,we can set up the illumination system,choose the best wavelength range,and select other options to capture the object feature of interest in the best way in an image.Once the image is sensed,it must be brought into a form that can be treated with digital computers.This process is called digitization.With the problems of traffic are more and more serious. Thus Intelligent Transport System (ITS) comes out. The subject of the automatic recognition of license plate is one of the most significant subjects that are improved from the connection of computer vision and pattern recognition. The image imputed to the computer is disposed and analyzed in order to localization the position and recognition the characters on the license plate express these characters in text string form The license plate recognition system (LPSR) has important application in ITS. In LPSR, the first step is for locating the license plate in the captured image which is very important for character recognition. The recognition correction rate of license plate is governed by accurate degree of license plate location. In this paper, several of methods in image manipulation are compared and analyzed, then come out the resolutions for localization of the car plate. The experiences show that the good result has been got with these methods. The methods based on edge map and frequency analysis is used in the process of the localization of the license plate, that is to say, extracting the characteristics of the license plate in the car images after being checked up forthe edge, and then analyzing and processing until the probably area of license plate is extracted.The automated license plate location is a part of the image processing ,it’s also an important part in the intelligent traffic system.It is the key step in the Vehicle License Plate Recognition(LPR).A method for the recognition of images of different backgrounds and different illuminations is proposed in the paper.the upper and lower borders are determined through the gray variation regulation of the character distribution.The left and right borders are determined through the black-white variation of the pixels in every row.The first steps of digital processing may include a number of different operations and are known as image processing.If the sensor has nonlinear characteristics, these need to be corrected.Likewise,brightness and contrast of the image may require improvement.Commonly,too,coordinate transformations are needed to restore geometrical distortions introduced during image formation.Radiometric and geometric corrections are elementary pixel processing operations.It may be necessary to correct known disturbances in the image,for instance caused by a defocused optics,motion blur,errors in the sensor,or errors in the transmission of image signals.We also deal with reconstruction techniques which are required with many indirect imaging techniques such as tomography that deliver no direct image.A whole chain of processing steps is necessary to analyze and identify objects.First,adequate filtering procedures must be applied in order to distinguish the objects of interest from other objects and the background.Essentially,from an image(or several images),one or more feature images are extracted.The basic tools for this task are averaging and edge detection and the analysis of simple neighborhoods and complex patterns known as texture in image processing.An important feature of an object is also its motion.Techniques to detect and determine motion are necessary.Then the object has to be separated from the background.This means that regions of constant features and discontinuities must be identified.This process leads to alabel image.Now that we know the exact geometrical shape of the object,we can extract further information such as the mean gray value,the area,perimeter,and other parameters for the form of the object[3].These parameters can be used to classify objects.This is an important step in many applications of image processing,as the following examples show:In a satellite image showing an agricultural area,we would like to distinguish fields with different fruits and obtain parameters to estimate their ripeness or to detect damage by parasites.There are many medical applications where the essential problem is to detect pathologi-al changes.A classic example is the analysis of aberrations in chromosomes.Character recognition in printed and handwritten text is another example which has been studied since image processing began and still poses significant difficulties.You hopefully do more,namely try to understand the meaning of what you are reading.This is also the final step of image processing,where one aims to understand the observed scene.We perform this task more or less unconsciously whenever we use our visual system.We recognize people,we can easily distinguish between the image of a scientific lab and that of a living room,and we watch the traffic to cross a street safely.We all do this without knowing how the visual system works.For some times now,image processing and computer-graphics have been treated as two different areas.Knowledge in both areas has increased considerably and more complex problems can now be treated.Computer graphics is striving to achieve photorealistic computer-generated images of three-dimensional scenes,while image processing is trying to reconstruct one from an image actually taken with a camera.In this sense,image processing performs the inverse procedure to that of computer graphics.We start with knowledge of the shape and features of an object—at the bottom of Fig. and work upwards until we get a two-dimensional image.To handle image processing or computer graphics,we basically have to work from the same knowledge.We need to know the interaction between illumination and objects,how a three-dimensional scene is projected onto an image plane,etc.There are still quite a few differences between an image processing and a graphics workstation.But we can envisage that,when the similarities and interrelations between computergraphics and image processing are better understood and the proper hardware is developed,we will see some kind of general-purpose workstation in the future which can handle computer graphics as well as image processing tasks[5].The advent of multimedia,i. e. ,the integration of text,images,sound,and movies,will further accelerate the unification of computer graphics and image processing.In January 1980 Scientific American published a remarkable image called Plume2,the second of eight volcanic eruptions detected on the Jovian moon by the spacecraft Voyager 1 on 5 March 1979.The picture was a landmark image in interplanetary exploration—the first time an erupting volcano had been seen in space.It was also a triumph for image processing.Satellite imagery and images from interplanetary explorers have until fairly recently been the major users of image processing techniques,where a computer image is numerically manipulated to produce some desired effect-such as making a particular aspect or feature in the image more visible.Image processing has its roots in photo reconnaissance in the Second World War where processing operations were optical and interpretation operations were performed by humans who undertook such tasks as quantifying the effect of bombing raids.With the advent of satellite imagery in the late 1960s,much computer-based work began and the color composite satellite images,sometimes startlingly beautiful, have become part of our visual culture and the perception of our planet.Like computer graphics,it was until recently confined to research laboratories which could afford the expensive image processing computers that could cope with the substantial processing overheads required to process large numbers of high-resolution images.With the advent of cheap powerful computers and image collection devices like digital cameras and scanners,we have seen a migration of image processing techniques into the public domain.Classical image processing techniques are routinely employed bygraphic designers to manipulate photographic and generated imagery,either to correct defects,change color and so on or creatively to transform the entire look of an image by subjecting it to some operation such as edge enhancement.A recent mainstream application of image processing is the compression of images—either for transmission across the Internet or the compression of moving video images in video telephony and video conferencing.Video telephony is one of the current crossover areas that employ both computer graphics and classical image processing techniques to try to achieve very high compression rates.All this is part of an inexorable trend towards the digital representation of images.Indeed that most powerful image form of the twentieth century—the TV image—is also about to be taken into the digital domain.Image processing is characterized by a large number of algorithms that are specific solutions to specific problems.Some are mathematical or context-independent operations that are applied to each and every pixel.For example,we can use Fourier transforms to perform image filtering operations.Others are“algorithmic”—we may use a complicated recursive strategy to find those pixels that constitute the edges in an image.Image processing operations often form part of a computer vision system.The input image may be filtered to highlight or reveal edges prior to a shape detection usually known as low-level operations.In computer graphics filtering operations are used extensively to avoid abasing or sampling artifacts.中文翻译图像处理不是一步就能完成的过程。
外文翻译---基于模糊逻辑技术图像上边缘检测
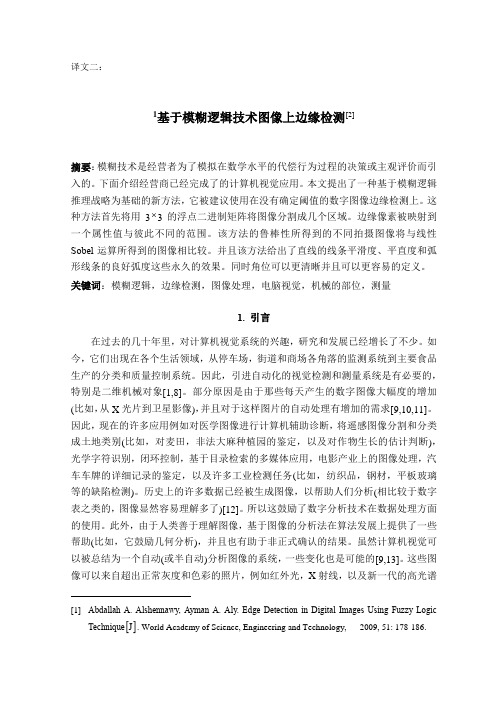
译文二:1基于模糊逻辑技术图像上边缘检测[2]摘要:模糊技术是经营者为了模拟在数学水平的代偿行为过程的决策或主观评价而引入的。
下面介绍经营商已经完成了的计算机视觉应用。
本文提出了一种基于模糊逻辑推理战略为基础的新方法,它被建议使用在没有确定阈值的数字图像边缘检测上。
这种方法首先将用3⨯3的浮点二进制矩阵将图像分割成几个区域。
边缘像素被映射到一个属性值与彼此不同的范围。
该方法的鲁棒性所得到的不同拍摄图像将与线性Sobel运算所得到的图像相比较。
并且该方法给出了直线的线条平滑度、平直度和弧形线条的良好弧度这些永久的效果。
同时角位可以更清晰并且可以更容易的定义。
关键词:模糊逻辑,边缘检测,图像处理,电脑视觉,机械的部位,测量1.引言在过去的几十年里,对计算机视觉系统的兴趣,研究和发展已经增长了不少。
如今,它们出现在各个生活领域,从停车场,街道和商场各角落的监测系统到主要食品生产的分类和质量控制系统。
因此,引进自动化的视觉检测和测量系统是有必要的,特别是二维机械对象[1,8]。
部分原因是由于那些每天产生的数字图像大幅度的增加(比如,从X光片到卫星影像),并且对于这样图片的自动处理有增加的需求[9,10,11]。
因此,现在的许多应用例如对医学图像进行计算机辅助诊断,将遥感图像分割和分类成土地类别(比如,对麦田,非法大麻种植园的鉴定,以及对作物生长的估计判断),光学字符识别,闭环控制,基于目录检索的多媒体应用,电影产业上的图像处理,汽车车牌的详细记录的鉴定,以及许多工业检测任务(比如,纺织品,钢材,平板玻璃等的缺陷检测)。
历史上的许多数据已经被生成图像,以帮助人们分析(相比较于数字表之类的,图像显然容易理解多了)[12]。
所以这鼓励了数字分析技术在数据处理方面的使用。
此外,由于人类善于理解图像,基于图像的分析法在算法发展上提供了一些帮助(比如,它鼓励几何分析),并且也有助于非正式确认的结果。
虽然计算机视觉可以被总结为一个自动(或半自动)分析图像的系统,一些变化也是可能的[9,13]。
数字图像处理常用词汇表
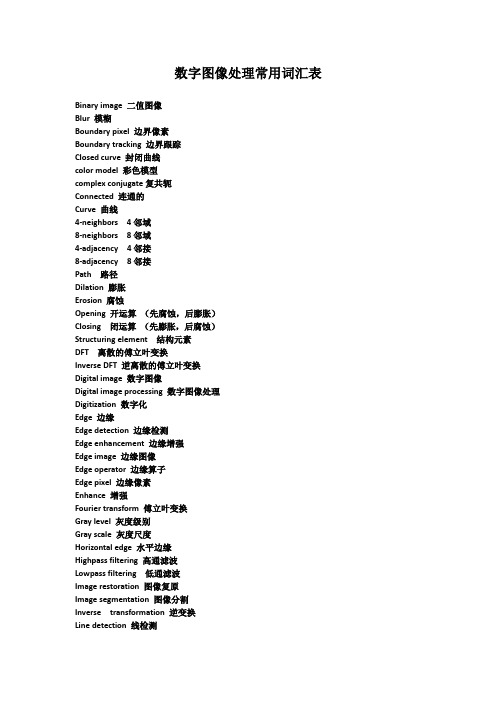
数字图像处理常用词汇表Binary image 二值图像Blur 模糊Boundary pixel 边界像素Boundary tracking 边界跟踪Closed curve 封闭曲线color model 彩色模型complex conjugate复共轭Connected 连通的Curve 曲线4-neighbors 4邻域8-neighbors 8邻域4-adjacency 4邻接8-adjacency 8邻接Path 路径Dilation 膨胀Erosion 腐蚀Opening 开运算(先腐蚀,后膨胀)Closing 闭运算(先膨胀,后腐蚀)Structuring element 结构元素DFT 离散的傅立叶变换Inverse DFT 逆离散的傅立叶变换Digital image 数字图像Digital image processing 数字图像处理Digitization 数字化Edge 边缘Edge detection 边缘检测Edge enhancement 边缘增强Edge image 边缘图像Edge operator 边缘算子Edge pixel 边缘像素Enhance 增强Fourier transform 傅立叶变换Gray level 灰度级别Gray scale 灰度尺度Horizontal edge 水平边缘Highpass filtering 高通滤波Lowpass filtering 低通滤波Image restoration 图像复原Image segmentation 图像分割Inverse transformation 逆变换Line detection 线检测Line pixel 直线像素Linear filter线性滤波Median filter中值滤波Mask 掩模Neighborhood 邻域Neighborhood operation 邻域运算Noise 噪音Noise reduction 噪音消减Pixel 像素Point operation 点运算Region 区域Region averaging 区域平均Weighted region averaging加权区域平均Resolution 分辨率Sharpening 锐化Shape number 形状数Smoothing 平滑Threshold 阈值Thresholding 二值化Transfer function 传递函数Vertical edge 垂直边缘Horizontal edge 水平边缘RGB color cube RGB色彩立方体HSI color model HSI 色彩模型Circular color plane 圆形彩色平面Triangular color plane 三角形彩色平面。
数字图像处理英文文献翻译参考
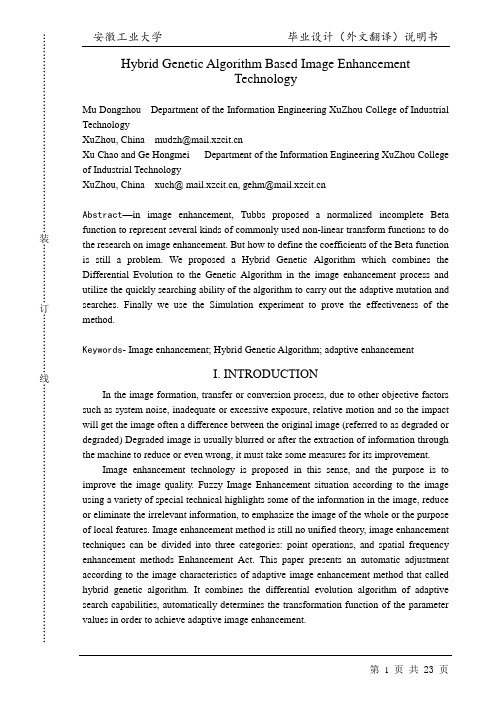
…………………………………………………装………………订………………线…………………………………………………………………Hybrid Genetic Algorithm Based Image EnhancementTechnologyMu Dongzhou Department of the Information Engineering XuZhou College of Industrial TechnologyXuZhou, China ****************.cnXu Chao and Ge Hongmei Department of the Information Engineering XuZhou College of Industrial TechnologyXuZhou, China ***************.cn,***************.cnAbstract—in image enhancement, Tubbs proposed a normalized incomplete Beta function to represent several kinds of commonly used non-linear transform functions to do the research on image enhancement. But how to define the coefficients of the Beta function is still a problem. We proposed a Hybrid Genetic Algorithm which combines the Differential Evolution to the Genetic Algorithm in the image enhancement process and utilize the quickly searching ability of the algorithm to carry out the adaptive mutation and searches. Finally we use the Simulation experiment to prove the effectiveness of the method.Keywords- Image enhancement; Hybrid Genetic Algorithm; adaptive enhancementI. INTRODUCTIONIn the image formation, transfer or conversion process, due to other objective factors such as system noise, inadequate or excessive exposure, relative motion and so the impact will get the image often a difference between the original image (referred to as degraded or degraded) Degraded image is usually blurred or after the extraction of information through the machine to reduce or even wrong, it must take some measures for its improvement.Image enhancement technology is proposed in this sense, and the purpose is to improve the image quality. Fuzzy Image Enhancement situation according to the image using a variety of special technical highlights some of the information in the image, reduce or eliminate the irrelevant information, to emphasize the image of the whole or the purpose of local features. Image enhancement method is still no unified theory, image enhancement techniques can be divided into three categories: point operations, and spatial frequency enhancement methods Enhancement Act. This paper presents an automatic adjustment according to the image characteristics of adaptive image enhancement method that called hybrid genetic algorithm. It combines the differential evolution algorithm of adaptive search capabilities, automatically determines the transformation function of the parameter values in order to achieve adaptive image enhancement.…………………………………………………装………………订………………线…………………………………………………………………II. IMAGE ENHANCEMENT TECHNOLOGYImage enhancement refers to some features of the image, such as contour, contrast, emphasis or highlight edges, etc., in order to facilitate detection or further analysis and processing. Enhancements will not increase the information in the image data, but will choose the appropriate features of the expansion of dynamic range, making these features more easily detected or identified, for the detection and treatment follow-up analysis and lay a good foundation.Image enhancement method consists of point operations, spatial filtering, and frequency domain filtering categories. Point operations, including contrast stretching, histogram modeling, and limiting noise and image subtraction techniques. Spatial filter including low-pass filtering, median filtering, high pass filter (image sharpening). Frequency filter including homomorphism filtering, multi-scale multi-resolution image enhancement applied [1].III. DIFFERENTIAL EVOLUTION ALGORITHMDifferential Evolution (DE) was first proposed by Price and Storn, and with other evolutionary algorithms are compared, DE algorithm has a strong spatial search capability, and easy to implement, easy to understand. DE algorithm is a novel search algorithm, it is first in the search space randomly generates the initial population and then calculate the difference between any two members of the vector, and the difference is added to the third member of the vector, by which Method to form a new individual. If you find that the fitness of new individual members better than the original, then replace the original with the formation of individual self.The operation of DE is the same as genetic algorithm, and it conclude mutation, crossover and selection, but the methods are different. We suppose that the group size is P, the vector dimension is D, and we can express the object vector as (1):xi=[xi1,xi2,…,xiD] (i =1,…,P)(1) And the mutation vector can be expressed as (2):()321rrriXXFXV-⨯+=i=1,...,P (2) 1rX,2rX,3rX are three randomly selected individuals from group, and r1≠r2≠r3≠i.F is a range of [0, 2] between the actual type constant factor difference vector is used to control the influence, commonly referred to as scaling factor. Clearly the difference between the vector and the smaller the disturbance also smaller, which means that if groups close to the optimum value, the disturbance will be automatically reduced.DE algorithm selection operation is a "greedy " selection mode, if and only if the new vector ui the fitness of the individual than the target vector is better when the individual xi, ui will be retained to the next group. Otherwise, the target vector xi individuals remain in the original group, once again as the next generation of the parent vector.…………………………………………………装………………订………………线…………………………………………………………………IV. HYBRID GA FOR IMAGE ENHANCEMENT IMAGEenhancement is the foundation to get the fast object detection, so it is necessary to find real-time and good performance algorithm. For the practical requirements of different systems, many algorithms need to determine the parameters and artificial thresholds. Can use a non-complete Beta function, it can completely cover the typical image enhancement transform type, but to determine the Beta function parameters are still many problems to be solved. This section presents a Beta function, since according to the applicable method for image enhancement, adaptive Hybrid genetic algorithm search capabilities, automatically determines the transformation function of the parameter values in order to achieve adaptive image enhancement.The purpose of image enhancement is to improve image quality, which are more prominent features of the specified restore the degraded image details and so on. In the degraded image in a common feature is the contrast lower side usually presents bright, dim or gray concentrated. Low-contrast degraded image can be stretched to achieve a dynamic histogram enhancement, such as gray level change. We use Ixy to illustrate the gray level of point (x, y) which can be expressed by (3).Ixy=f(x, y) (3) where: “f” is a linear or nonline ar function. In general, gray image have four nonlinear translations [6] [7] that can be shown as Figure 1. We use a normalized incomplete Beta function to automatically fit the 4 categories of image enhancement transformation curve. It defines in (4):()()()()10,01,111<<-=---⎰βαβαβαdtttBufu(4) where:()()⎰---=1111,dtttBβαβα(5) For different value of α and β, we can get response curve from (4) and (5).The hybrid GA can make use of the previous section adaptive differential evolution algorithm to search for the best function to determine a value of Beta, and then each pixel grayscale values into the Beta function, the corresponding transformation of Figure 1, resulting in ideal image enhancement. The detail description is follows:Assuming the original image pixel (x, y) of the pixel gray level by the formula (4),denoted byxyi,()Ω∈yx,, here Ω is the image domain. Enhanced image is denoted by Ixy. Firstly, the image gray value normalized into [0, 1] by (6).minmaxminiiiig xyxy--=(6)where:maxi andm ini express the maximum and minimum of image gray relatively.Define the nonlinear transformation function f(u) (0≤u≤1) to transform source image…………………………………………………装………………订………………线…………………………………………………………………Finally, we use the hybrid genetic algorithm to determine the appropriate Beta function f (u) the optimal parameters αand β. Will enhance the image Gxy transformed antinormalized.V. EXPERIMENT AND ANALYSISIn the simulation, we used two different types of gray-scale images degraded; the program performed 50 times, population sizes of 30, evolved 600 times. The results show that the proposed method can very effectively enhance the different types of degraded image.Figure 2, the size of the original image a 320 × 320, it's the contrast to low, and some details of the more obscure, in particular, scarves and other details of the texture is not obvious, visual effects, poor, using the method proposed in this section, to overcome the above some of the issues and get satisfactory image results, as shown in Figure 5 (b) shows, the visual effects have been well improved. From the histogram view, the scope of the distribution of image intensity is more uniform, and the distribution of light and dark gray area is more reasonable. Hybrid genetic algorithm to automatically identify the nonlinear…………………………………………………装………………订………………线…………………………………………………………………transformation of the function curve, and the values obtained before 9.837,5.7912, from the curve can be drawn, it is consistent with Figure 3, c-class, that stretch across the middle region compression transform the region, which were consistent with the histogram, the overall original image low contrast, compression at both ends of the middle region stretching region is consistent with human visual sense, enhanced the effect of significantly improved.Figure 3, the size of the original image a 320 × 256, the overall intensity is low, the use of the method proposed in this section are the images b, we can see the ground, chairs and clothes and other details of the resolution and contrast than the original image has Improved significantly, the original image gray distribution concentrated in the lower region, and the enhanced image of the gray uniform, gray before and after transformation and nonlinear transformation of basic graph 3 (a) the same class, namely, the image Dim region stretching, and the values were 5.9409,9.5704, nonlinear transformation of images degraded type inference is correct, the enhanced visual effect and good robustness enhancement.Difficult to assess the quality of image enhancement, image is still no common evaluation criteria, common peak signal to noise ratio (PSNR) evaluation in terms of line, but the peak signal to noise ratio does not reflect the human visual system error. Therefore, we use marginal protection index and contrast increase index to evaluate the experimental results.Edgel Protection Index (EPI) is defined as follows:…………………………………………………装………………订………………线…………………………………………………………………(7)Contrast Increase Index (CII) is defined as follows:minmaxminmax,GGGGCCCEOD+-==(8)In figure 4, we compared with the Wavelet Transform based algorithm and get the evaluate number in TABLE I.Figure 4 (a, c) show the original image and the differential evolution algorithm for enhanced results can be seen from the enhanced contrast markedly improved, clearer image details, edge feature more prominent. b, c shows the wavelet-based hybrid genetic algorithm-based Comparison of Image Enhancement: wavelet-based enhancement method to enhance image detail out some of the image visual effect is an improvement over the original image, but the enhancement is not obvious; and Hybrid genetic algorithm based on adaptive transform image enhancement effect is very good, image details, texture, clarity is enhanced compared with the results based on wavelet transform has greatly improved the image of the post-analytical processing helpful. Experimental enhancement experiment using wavelet transform "sym4" wavelet, enhanced differential evolution algorithm experiment, the parameters and the values were 5.9409,9.5704. For a 256 × 256 size image transform based on adaptive hybrid genetic algorithm in Matlab 7.0 image enhancement software, the computing time is about 2 seconds, operation is very fast. From TABLE I, objective evaluation criteria can be seen, both the edge of the protection index, or to enhance the contrast index, based on adaptive hybrid genetic algorithm compared to traditional methods based on wavelet transform has a larger increase, which is from This section describes the objective advantages of the method. From above analysis, we can see…………………………………………………装………………订………………线…………………………………………………………………that this method.From above analysis, we can see that this method can be useful and effective.VI. CONCLUSIONIn this paper, to maintain the integrity of the perspective image information, the use of Hybrid genetic algorithm for image enhancement, can be seen from the experimental results, based on the Hybrid genetic algorithm for image enhancement method has obvious effect. Compared with other evolutionary algorithms, hybrid genetic algorithm outstanding performance of the algorithm, it is simple, robust and rapid convergence is almost optimal solution can be found in each run, while the hybrid genetic algorithm is only a few parameters need to be set and the same set of parameters can be used in many different problems. Using the Hybrid genetic algorithm quick search capability for a given test image adaptive mutation, search, to finalize the transformation function from the best parameter values. And the exhaustive method compared to a significant reduction in the time to ask and solve the computing complexity. Therefore, the proposed image enhancement method has some practical value.REFERENCES[1] HE Bin et al., Visual C++ Digital Image Processing [M], Posts & Telecom Press,2001,4:473~477[2] Storn R, Price K. Differential Evolution—a Simple and Efficient Adaptive Scheme forGlobal Optimization over Continuous Space[R]. International Computer Science Institute, Berlaey, 1995.[3] Tubbs J D. A note on parametric image enhancement [J].Pattern Recognition.1997,30(6):617-621.[4] TANG Ming, MA Song De, XIAO Jing. Enhancing Far Infrared Image Sequences withModel Based Adaptive Filtering [J] . CHINESE JOURNAL OF COMPUTERS, 2000, 23(8):893-896.[5] ZHOU Ji Liu, LV Hang, Image Enhancement Based on A New Genetic Algorithm [J].Chinese Journal of Computers, 2001, 24(9):959-964.[6] LI Yun, LIU Xuecheng. On Algorithm of Image Constract Enhancement Based onWavelet Transformation [J]. Computer Applications and Software, 2008,8.[7] XIE Mei-hua, WANG Zheng-ming, The Partial Differential Equation Method for ImageResolution Enhancement [J]. Journal of Remote Sensing, 2005,9(6):673-679.…………………………………………………装………………订………………线…………………………………………………………………基于混合遗传算法的图像增强技术Mu Dongzhou 徐州工业职业技术学院信息工程系 XuZhou, China****************.cnXu Chao and Ge Hongmei 徐州工业职业技术学院信息工程系 XuZhou,********************.cn,***************.cn摘要—在图像增强之中,塔布斯提出了归一化不完全β函数表示常用的几种使用的非线性变换函数对图像进行研究增强。
数字图像检测中英文对照外文翻译文献
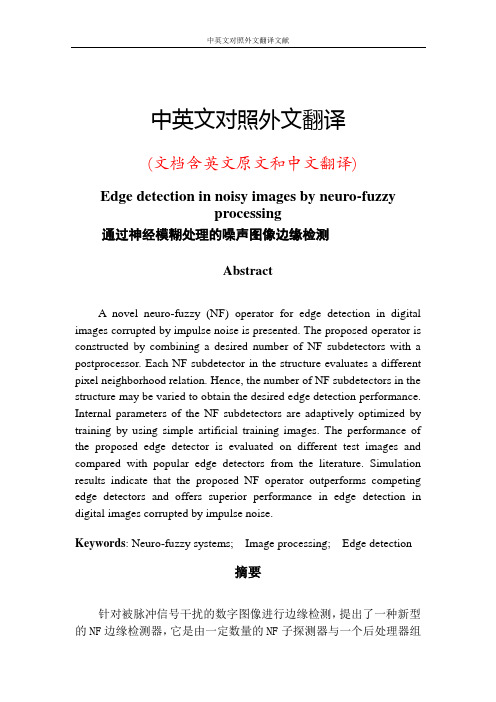
中英文对照外文翻译(文档含英文原文和中文翻译)Edge detection in noisy images by neuro-fuzzyprocessing通过神经模糊处理的噪声图像边缘检测AbstractA novel neuro-fuzzy (NF) operator for edge detection in digital images corrupted by impulse noise is presented. The proposed operator is constructed by combining a desired number of NF subdetectors with a postprocessor. Each NF subdetector in the structure evaluates a different pixel neighborhood relation. Hence, the number of NF subdetectors in the structure may be varied to obtain the desired edge detection performance. Internal parameters of the NF subdetectors are adaptively optimized by training by using simple artificial training images. The performance of the proposed edge detector is evaluated on different test images and compared with popular edge detectors from the literature. Simulation results indicate that the proposed NF operator outperforms competing edge detectors and offers superior performance in edge detection in digital images corrupted by impulse noise.Keywords: Neuro-fuzzy systems; Image processing; Edge detection摘要针对被脉冲信号干扰的数字图像进行边缘检测,提出了一种新型的NF边缘检测器,它是由一定数量的NF子探测器与一个后处理器组成。
外文翻译---图像的边缘检测
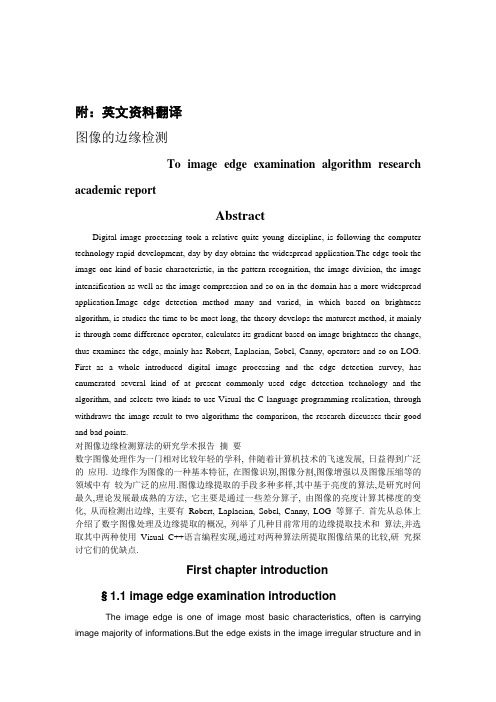
附:英文资料翻译图像的边缘检测To image edge examination algorithm research academic reportAbstractDigital image processing took a relative quite young discipline, is following the computer technology rapid development, day by day obtains the widespread application.The edge took the image one kind of basic characteristic, in the pattern recognition, the image division, the image intensification as well as the image compression and so on in the domain has a more widespread application.Image edge detection method many and varied, in which based on brightness algorithm, is studies the time to be most long, the theory develops the maturest method, it mainly is through some difference operator, calculates its gradient based on image brightness the change, thus examines the edge, mainly has Robert, Laplacian, Sobel, Canny, operators and so on LOG. First as a whole introduced digital image processing and the edge detection survey, has enumerated several kind of at present commonly used edge detection technology and the algorithm, and selects two kinds to use Visual the C language programming realization, through withdraws the image result to two algorithms the comparison, the research discusses their good and bad points.对图像边缘检测算法的研究学术报告摘要数字图像处理作为一门相对比较年轻的学科, 伴随着计算机技术的飞速发展, 日益得到广泛的应用. 边缘作为图像的一种基本特征, 在图像识别,图像分割,图像增强以及图像压缩等的领域中有较为广泛的应用.图像边缘提取的手段多种多样,其中基于亮度的算法,是研究时间最久,理论发展最成熟的方法, 它主要是通过一些差分算子, 由图像的亮度计算其梯度的变化, 从而检测出边缘, 主要有Robert, Laplacian, Sobel, Canny, LOG 等算子. 首先从总体上介绍了数字图像处理及边缘提取的概况, 列举了几种目前常用的边缘提取技术和算法,并选取其中两种使用Visual C++语言编程实现,通过对两种算法所提取图像结果的比较,研究探讨它们的优缺点.First chapter introduction§1.1 image edge examination introductionThe image edge is one of image most basic characteristics, often is carrying image majority of informations.But the edge exists in the image irregular structure and innot the steady phenomenon, also namely exists in the signal point of discontinuity place, these spots have given the image outline position, these outlines are frequently we when the imagery processing needs the extremely important some representative condition, this needs us to examine and to withdraw its edge to an image. But the edge examination algorithm is in the imagery processing question one of classical technical difficult problems, its solution carries on the high level regarding us the characteristic description, the recognition and the understanding and so on has the significant influence; Also because the edge examination all has in many aspects the extremely important use value, therefore how the people are devoting continuously in study and solve the structure to leave have the good nature and the good effect edge examination operator question.In the usual situation, we may the signal in singular point and the point of discontinuity thought is in the image peripheral point, its nearby gradation change situation may reflect from its neighboring picture element gradation distribution gradient. According to this characteristic, we proposed many kinds of edge examination operator: If Robert operator, Sobel operator, Prewitt operator, Laplace operator and so on.These methods many are wait for the processing picture element to carry on the gradation analysis for the central neighborhood achievement the foundation, realized and has already obtained the good processing effect to the image edge extraction. . But this kind of method simultaneously also exists has the edge picture element width, the noise jamming is serious and so on the shortcomings, even if uses some auxiliary methods to perform the denoising, also corresponding can bring the flaw which the edge fuzzy and so on overcomes with difficulty.Along with the wavelet analysis appearance, its good time frequency partial characteristic by the widespread application in the imagery processing and in the pattern recognition domain, becomes in the signal processing the commonly used method and the powerful tool.Through the wavelet analysis, may interweave decomposes in the same place each kind of composite signal the different frequency the block signal, but carries on the edge examination through the wavelet transformation, may use its multi-criteria and the multi-resolution nature fully , real effective expresses the image the edge characteristic.When the wavelet transformation criterion reduces, is more sensitive to the image detail; But when the criterion increases, the image detail is filtered out, the examination edge will be only the thick outline.This characteristic is extremely useful in the pattern recognition, we may be called this thick outline the image the main edge.If will be able an image main edge clear integrity extraction, this to the goal division, the recognition and so on following processing to bring the enormous convenience.Generally speaking, the above method all is the work which does based on the image luminance information.In the multitudinous scientific research worker under, has obtained the very good effect diligently.But, because the image edge receives physical condition and so on the illumination influences quite to be big above, often enables many to have a common shortcoming based on brightness edge detection method, that is the edge is not continual, does not seal up.Considered the phase information in the image importance as well as its stable characteristic, causes using the phase information to carry on the imagery processing into new research topic. In this paper soon introduces one kind based on the phase image characteristic examination method - - phase uniform method.It is not uses the image the luminance information, but is its phase characteristic, namely supposition image Fourier component phase most consistent spot achievement characteristic point.Not only it can examine brightness characteristics and so on step characteristic, line characteristic, moreover can examine Mach belt phenomenon which produces as a result of the human vision sensation characteristic.Because the phase uniformity does not need to carry on any supposition to the image characteristic type, therefore it has the very strong versatility.第一章绪论§1.1 图像边缘检测概论图像边缘是图像最基本的特征之一, 往往携带着一幅图像的大部分信息. 而边缘存在于图像的不规则结构和不平稳现象中,也即存在于信号的突变点处,这些点给出了图像轮廓的位置,这些轮廓常常是我们在图像处理时所需要的非常重要的一些特征条件, 这就需要我们对一幅图像检测并提取出它的边缘. 而边缘检测算法则是图像处理问题中经典技术难题之一, 它的解决对于我们进行高层次的特征描述, 识别和理解等有着重大的影响; 又由于边缘检测在许多方面都有着非常重要的使用价值, 所以人们一直在致力于研究和解决如何构造出具有良好性质及好的效果的边缘检测算子的问题.在通常情况下,我们可以将信号中的奇异点和突变点认为是图像中的边缘点,其附近灰度的变化情况可从它相邻像素灰度分布的梯度来反映. 根据这一特点,我们提出了多种边缘检测算子:如Robert 算子,Sobel算子,Prewitt 算子, Laplace 算子等.这些方法多是以待处理像素为中心的邻域作为进行灰度分析的基础,实现对图像边缘的提取并已经取得了较好的处理效果. 但这类方法同时也存在有边缘像素宽, 噪声干扰较严重等缺点,即使采用一些辅助的方法加以去噪,也相应的会带来边缘模糊等难以克服的缺陷.随着小波分析的出现, 其良好的时频局部特性被广泛的应用在图像处理和模式识别领域中, 成为信号处理中常用的手段和有力的工具. 通过小波分析, 可以将交织在一起的各种混合信号分解成不同频率的块信号,而通过小波变换进行边缘检测,可以充分利用其多尺度和多分辨率的性质,真实有效的表达图像的边缘特征.当小波变换的尺度减小时,对图像的细节更加敏感;而当尺度增大时,图像的细节将被滤掉,检测的边缘只是粗轮廓.该特性在模式识别中非常有用,我们可以将此粗轮廓称为图像的主要边缘.如果能将一个图像的主要边缘清晰完整的提取出来,这将对目标分割,识别等后续处理带来极大的便利.总的说来,以上方法都是基于图像的亮度信息来作的工作. 在众多科研工作者的努力下,取得了很好的效果.但是,由于图像边缘受到光照等物理条件的影响比较大, 往往使得以上诸多基于亮度的边缘提取方法有着一个共同的缺点, 那就是边缘不连续, 不封闭. 考虑到相位信息在图像中的重要性以及其稳定的特点, 使得利用相位信息进行图像处理成为新的研究课题. 在本文中即将介绍一种基于相位的图像特征检测方法——相位一致性方法. 它并不是利用图像的亮度信息,而是其相位特点,即假设图像的傅立叶分量相位最一致的点作为特征点.它不但能检测到阶跃特征, 线特征等亮度特征, 而且能够检测到由于人类视觉感知特性而产生的的马赫带现象. 由于相位一致性不需要对图像的特征类型进行任何假设,所以它具有很强的通用性.§1.2 image edge definitionThe image majority main information all exists in the image edge, the main performance for the image partial characteristic discontinuity, is in the image the gradation change quite fierce place, also is the signal which we usually said has the strange change place. The strange signal the gradation change which moves towards along the edge is fierce, usually we divide the edge for the step shape and the roof shape two kind of types (as shown in Figure 1-1).In the step edge two side grey levels have the obvious change; But the roof shape edge is located the gradation increase and the reduced intersection point.May portray the peripheral point in mathematics using the gradation derivative the change, to the step edge, the roof shape edge asks its step, the second time derivative separately. To an edge, has the possibility simultaneously to have the step and the line edge characteristic. For example on a surface, changes from a plane to the normal direction different another plane can produce the step edge; If this surface has the edges and corners which the regular reflection characteristic also two planes form quite to be smooth, then works as when edges and corners smooth surface normal after mirror surface reflection angle, as a result of the regular reflection component, can produce the bright light strip on the edges and corners smooth surface, such edge looked like has likely superimposed a line edge in the step edge. Because edge possible and in scene object important characteristic correspondence, therefore it is the very important image characteristic.Forinstance, an object outline usually produces the step edge, because the object image intensity is different with the background image intensity.§1.3 paper selected topic theory significanceThe paper selected topic originates in holds the important status and the function practical application topic in the image project.The so-called image project discipline is refers foundation discipline and so on mathematics, optics principles, the discipline which in the image application unifies which accumulates the technical background develops.The image project content is extremely rich, and so on divides into three levels differently according to the abstract degree and the research technique: Imagery processing, image analysis and image understanding.As shown in Figure 1-2, in the chart the image division is in between the image analysis and the imagery processing, its meaning is, the image division is from the imagery processing to the image analysis essential step, also is further understands the image the foundation. The image division has the important influence to the characteristic.The image division and based on thedivision goal expression, the characteristic extraction and the parameter survey and so on transforms the primitive image as a more abstract more compact form, causes the high-level image analysis and possibly understands into.But the edge examination is the image division core content, therefore the edge examination holds the important status and the function in the image project.Therefore the edge examination research always is in the image engineering research the hot spot and the focal point, moreover the people enhance unceasingly to its attention and the investment.§1.2 图像边缘的定义图像的大部分主要信息都存在于图像的边缘中, 主要表现为图像局部特征的不连续性, 是图像中灰度变化比较剧烈的地方, 也即我们通常所说的信号发生奇异变化的地方. 奇异信号沿边缘走向的灰度变化剧烈,通常我们将边缘划分为阶跃状和屋顶状两种类型(如图1-1 所示).阶跃边缘中两边的灰度值有明显的变化; 而屋顶状边缘位于灰度增加与减少的交界处. 在数学上可利用灰度的导数来刻画边缘点的变化,对阶跃边缘,屋顶状边缘分别求其一阶,二阶导数. 对一个边缘来说,有可能同时具有阶跃和线条边缘特性.例如在一个表面上,由一个平面变化到法线方向不同的另一个平面就会产生阶跃边缘; 如果这一表面具有镜面反射特性且两平面形成的棱角比较圆滑,则当棱角圆滑表面的法线经过镜面反射角时,由于镜面反射分量,在棱角圆滑表面上会产生明亮光条, 这样的边缘看起来象在阶跃边缘上叠加了一个线条边缘. 由于边缘可能与场景中物体的重要特征对应,所以它是很重要的图像特征.比如,一个物体的轮廓通常产生阶跃边缘, 因为物体的图像强度不同于背景的图像强度.§1.3 论文选题的理论意义论文选题来源于在图像工程中占有重要的地位和作用的实际应用课题.所谓图像工程学科是指将数学,光学等基础学科的原理,结合在图像应用中积累的技术经验而发展起来的学科.图像工程的内容非常丰富,根据抽象程度和研究方法等的不同分为三个层次:图像处理,图像分析和图像理解.如图1-2 所示,在图中,图像分割处于图像分析与图像处理之间,其含义是,图像分割是从图像处理进到图像分析的关键步骤,也是进一步理解图像的基础.图像分割对特征有重要影响. 图像分割及基于分割的目标表达, 特征提取和参数测量等将原始图像转化为更抽象更紧凑的形式, 使得更高层的图像分析和理解成为可能. 而边缘检测是图像分割的核心内容, 所以边缘检测在图像工程中占有重要的地位和作用. 因此边缘检测的研究一直是图像技术研究中热点和焦点,而且人们对其的关注和投入不断提高.。
外文翻译---MATLAB 在图像边缘检测中的应用

英文资料翻译MATLAB application in image edge detection MATLAB of the 1984 countries MathWorks company to market since, after 10 years of development, has become internationally recognized the best technology application software. MATLAB is not only a kind of direct, efficient computer language, and at the same time, a scientific computing platform, it for data analysis and data visualization, algorithm and application development to provide the most core of math and advanced graphics tools. According to provide it with the more than 500 math and engineering function, engineering and technical personnel and scientific workers can integrated environment of developing or programming to complete their calculation.MATLAB software has very strong openness and adapt to sex. Keep the kernel in under the condition of invariable, MATLAB is in view of the different application subject of launch corresponding Toolbox (Toolbox), has now launched image processing Toolbox, signal processing Toolbox, wavelet Toolbox, neural network Toolbox and communication tools box, etc multiple disciplines special kit, which would place of different subjects research work.MATLAB image processing kit is by a series of support image processing function from the composition, the support of the image processing operation: geometric operation area of operation and operation; Linear filter and filter design; Transform (DCT transform); Image analysis and strengthened; Binary image manipulation, etc. Image processing tool kit function, the function can be divided into the following categories: image display; Image file input and output; Geometric operation; Pixels statistics; Image analysis and strengthened; Image filtering; Sex 2 d filter design; Image transformation; Fields and piece of operation; Binary image operation; Color mapping and color space transformation; Image types and type conversion; Kit acquiring parameters and Settings.1.Edge detection thisUse computer image processing has two purposes: produce more suitable for human observation and identification of the images; Hope can by the automatic computer image recognition and understanding.No matter what kind of purpose to, image processing the key step is to contain a variety of scenery of decomposition of image information. Decomposition of the end result is that break down into some has some kind of characteristics of the smallest components, known as the image of the yuan. Relative to the whole image of speaking, this the yuan more easily to be rapid processing.Image characteristics is to point to the image can be used as the sign of the field properties, it can be divided into the statistical features of the image and image visual, two types of levy. The statistical features of the image is to point to some people the characteristics of definition, through the transform to get, such as image histogram, moments, spectrum, etc.; Image visual characteristics is refers to person visual sense can be directly by the natural features, such as the brightness of the area, and texture or outline, etc. The two kinds of characteristics of the image into a series of meaningful goal or regional p rocess called image segmentation.The image is the basic characteristics of edge, the edge is to show its pixel grayscale around a step change order or roof of the collection of those changes pixels. It exists in target and background, goals and objectives, regional and region, the yuan and the yuan between, therefore, it is the image segmentation dependent on the most important characteristic that the texture characteristics of important information sources and shape characteristics of the foundation, and the image of the texture characteristics and the extraction of shape often dependent on image segmentation. Image edge extraction is also the basis of image matching, because it is the sign of position, the change of the original is not sensitive, and can be used for matching the feature points.The edge of the image is reflected by gray not continuity. Classic edge extraction method is investigation of each pixel image in an area of the gray change, use edge first or second order nearby directional derivative change rule,with simple method of edge detection, this method called edge detection method of local operators.The type of edge can be divided into two types: (1) step representation sexual edge, it on both sides of the pixel gray value varies significantly different; (2) the roof edges, it is located in gray value from the change of increased to reduce the turning point. For order jump sexual edge, second order directional derivative in edge is zero cross; For the roof edges, second order directional derivative in edge take extreme value.If a pixel fell in the image a certain object boundary, then its field will become a gray level with the change. The most useful to change two features is the rate of change and the gray direction, they are in the range of the gradient vector and the direction to said. Edge detection operator check every pixel grayscale rate fields and evaluation, and also include to determine the directions of the most use based on directional derivative deconvolution method for masking.Digital image processing technique has been widely applied to the biomedical field, the use of computer image processing and analysis, and complete detection and recognition of cancer cells can help doctors make a diagnosis of tumor cancers. Need to be made in the identification of cancer cells, the quantitative results, the human eye is difficult to accurately complete such work, and the use of computer image processing to complete the analysis and identification of the microscopic images have made great progress. In recent years, domestic and foreign medical images of cancer cells testing to identify the researchers put forward a lot of theory and method for the diagnosis of cancer cells has very important meaning and practical value.Cell edge detection is the cell area of the number of roundness and color, shape and chromaticity calculation and the basis of the analysis their test results directly affect the analysis and diagnosis of the disease. Classical edge detection operators such as Sobel operator, Laplacian operator, each pixel neighborhood of the image gray scale changes to detect the edge. Although these operators is simple, fast, but there are sensitive to noise, get isolated or in short sections of acontinuous edge pixels, overlapping the adjacent cell edge defects, while the optimal threshold segmentation and contour extraction method of combining edge detection, obtained by the iterative algorithm for the optimal threshold for image segmentation, contour extraction algorithm, digging inside the cell pixels, the last remaining part of the image is the edge of the cell, change the processing order of the traditional edge detection algorithm, by MATLAB programming, the experimental results that can effectively suppress the noise impact at the same time be able to objectively and correctly select the edge detection threshold, precision cell edge detection.2.Edge detection of MATLABMATLAB image processing toolkit defines the edge () function is used to test the edge of gray image.(1) BW = edge (I, "method"), returns and I size binary image BW, includingelements of 1 said is on the edge of the point, 0 means the edge points.Method for the following a string of:1) soble: the default value, with derivative Sobel edge detectionapproximate measure, to return to a maximum gradient edge;2) prewitt: with the derivative prewitt approximate edge detection, amaximum gradient to return to edge;3) Roberts: with the derivative Roberts approximate edge detection margins,return to a maximum gradient edge;4) the log: use the Laplace operation gaussian filter to I carry filtering,through the looking for 0 intersecting detection of edge;5) zerocross: use the filter to designated I filter, looking for 0 intersectingdetection of edge.(2) BW = edge (I, "method", thresh) with thresh designated sensitivitythreshold value, rather than the edge of all not thresh are ignored.(3) BW = edge (I, "method" thresh, direction, for soble and prewitt methodspecified direction, direction for string, including horizontal level said direction; Vertical said to hang straight party; Both said the two directions(the default).(4) BW = edge (I, 'log', thresh, log sigma), with sigma specified standarddeviation.(5) [BW, thresh] = edge (...), the return value of a function in fact have multiple(" BW "and" thresh "), but because the brace up with u said as a matrix, and so can be thought a return only parameters, which also shows the introduction of the concept of matrix MATLAB unity and superiority.st wordMATLAB has strong image processing function, provide a simple function calls to realize many classic image processing method. Not only is the image edge detection, in transform domain processing, image enhancement, mathematics morphological processing, and other aspects of the study, MATLAB can greatly improve the efficiency rapidly in the study of new ideas.MATLAB 在图像边缘检测中的应用MATLAB自1984年由国MathWorks公司推向市场以来,历经十几年的发展,现已成为国际公认的最优秀的科技应用软件。
- 1、下载文档前请自行甄别文档内容的完整性,平台不提供额外的编辑、内容补充、找答案等附加服务。
- 2、"仅部分预览"的文档,不可在线预览部分如存在完整性等问题,可反馈申请退款(可完整预览的文档不适用该条件!)。
- 3、如文档侵犯您的权益,请联系客服反馈,我们会尽快为您处理(人工客服工作时间:9:00-18:30)。
附录1 译文数字图像处理与边缘检测数字图像处理数字图像处理方法的研究源于两个主要应用领域:其一是为了便于人们分析而对图像信息进行改进:其二是为使机器自动理解而对图像数据进行存储、传输及显示。
一幅图像可定义为一个二维函数f(x,y),这里x和y是空间坐标,而在任何一对空间坐标(x,y)上的幅值f 称为该点图像的强度或灰度。
当x,y和幅值f 为有限的、离散的数值时,称该图像为数字图像。
数字图像处理是指借用数字计算机处理数字图像,值得提及的是数字图像是由有限的元素组成的,每一个元素都有一个特定的位置和幅值,这些元素称为图像元素、画面元素或像素。
像素是广泛用于表示数字图像元素的词汇。
视觉是人类最高级的感知器官,所以,毫无疑问图像在人类感知中扮演着最重要的角色。
然而,人类感知只限于电磁波谱的视觉波段,成像机器则可覆盖几乎全部电磁波谱,从伽马射线到无线电波。
它们可以对非人类习惯的那些图像源进行加工,这些图像源包括超声波、电子显微镜及计算机产生的图像。
因此,数字图像处理涉及各种各样的应用领域。
图像处理涉及的范畴或其他相关领域(例如,图像分析和计算机视觉)的界定在初创人之间并没有一致的看法。
有时用处理的输入和输出内容都是图像这一特点来界定图像处理的范围。
我们认为这一定义仅是人为界定和限制。
例如,在这个定义下,甚至最普通的计算一幅图像灰度平均值的工作都不能算做是图像处理。
另一方面,有些领域(如计算机视觉)研究的最高目标是用计算机去模拟人类视觉,包括理解和推理并根据视觉输入采取行动等。
这一领域本身是人工智能的分支,其目的是模仿人类智能。
人工智能领域处在其发展过程中的初期阶段,它的发展比预期的要慢的多,图像分析(也称为图像理解)领域则处在图像处理和计算机视觉两个学科之间。
从图像处理到计算机视觉这个连续的统一体内并没有明确的界线。
然而,在这个连续的统一体中可以考虑三种典型的计算处理(即低级、中级和高级处理)来区分其中的各个学科。
低级处理涉及初级操作,如降低噪声的图像预处理,对比度增强和图像尖锐化。
低级处理是以输入、输出都是图像为特点的处理。
中级处理涉及分割(把图像分为不同区域或目标物)以及缩减对目标物的描述,以使其更适合计算机处理及对不同目标的分类(识别)。
中级图像处理是以输入为图像,但输出是从这些图像中提取的特征(如边缘、轮廓及不同物体的标识等)为特点的。
最后,高级处理涉及在图像分析中被识别物体的总体理解,以及执行与视觉相关的识别函数(处在连续统一体边缘)等。
根据上述讨论,我们看到,图像处理和图像分析两个领域合乎逻辑的重叠区域是图像中特定区域或物体的识别这一领域。
这样,在研究中,我们界定数字图像处理包括输入和输出均是图像的处理,同时也包括从图像中提取特征及识别特定物体的处理。
举一个简单的文本自动分析方面的例子来具体说明这一概念。
在自动分析文本时首先获取一幅包含文本的图像,对该图像进行预处理,提取(分割)字符,然后以适合计算机处理的形式描述这些字符,最后识别这些字符,而所有这些操作都在本文界定的数字图像处理的范围内。
理解一页的内容可能要根据理解的复杂度从图像分析或计算机视觉领域考虑问题。
这样,我们定义的数字图像处理的概念将在有特殊社会和经济价值的领域内通用。
数字图像处理的应用领域多种多样,所以文本在内容组织上尽量达到该技术应用领域的广度。
阐述数字图像处理应用范围最简单的一种方法是根据信息源来分类(如可见光、X射线,等等)。
在今天的应用中,最主要的图像源是电磁能谱,其他主要的能源包括声波、超声波和电子(以用于电子显微镜方法的电子束形式)。
建模和可视化应用中的合成图像由计算机产生。
建立在电磁波谱辐射基础上的图像是最熟悉的,特别是X射线和可见光谱图像。
电磁波可定义为以各种波长传播的正弦波,或者认为是一种粒子流,每个粒子包含一定(一束)能量,每束能量成为一个光子。
如果光谱波段根据光谱能量进行分组,我们会得到下图1所示的伽马射线(最高能量)到无线电波(最低能量)的光谱。
如图所示的加底纹的条带表达了这样一个事实,即电磁波谱的各波段间并没有明确的界线,而是由一个波段平滑地过渡到另一个波段。
图像获取是第一步处理。
注意到获取与给出一幅数字形式的图像一样简单。
通常,图像获取包括如设置比例尺等预处理。
图像增强是数字图像处理最简单和最有吸引力的领域。
基本上,增强技术后面的思路是显现那些被模糊了的细节,或简单地突出一幅图像中感兴趣的特征。
一个图像增强的例子是增强图像的对比度,使其看起来好一些。
应记住,增强是图像处理中非常主观的领域,这一点很重要。
图像复原也是改进图像外貌的一个处理领域。
然而,不像增强,图像增强是主观的,而图像复原是客观的。
在某种意义上说,复原技术倾向于以图像退化的数学或概率模型为基础。
另一方面,增强以怎样构成好的增强效果这种人的主观偏爱为基础。
彩色图像处理已经成为一个重要领域,因为基于互联网的图像处理应用在不断增长。
就使得在彩色模型、数字域的彩色处理方面涵盖了大量基本概念。
在后续发展,彩色还是图像中感兴趣特征被提取的基础。
小波是在各种分辨率下描述图像的基础。
特别是在应用中,这些理论被用于图像数据压缩及金字塔描述方法。
在这里,图像被成功地细分为较小的区域。
压缩,正如其名称所指的意思,所涉及的技术是减少图像的存储量,或者在传输图像时降低频带。
虽然存储技术在过去的十年内有了很大改进,但对传输能力我们还不能这样说,尤其在互联网上更是如此,互联网是以大量的图片内容为特征的。
图像压缩技术对应的图像文件扩展名对大多数计算机用户是很熟悉的(也许没注意),如JPG文件扩展名用于JPEG(联合图片专家组)图像压缩标准。
形态学处理设计提取图像元素的工具,它在表现和描述形状方面非常有用。
这一章的材料将从输出图像处理到输出图像特征处理的转换开始。
分割过程将一幅图像划分为组成部分或目标物。
通常,自主分割是数字图像处理中最为困难的任务之一。
复杂的分割过程导致成功解决要求物体被分别识别出来的成像问题需要大量处理工作。
另一方面,不健壮且不稳定的分割算法几乎总是会导致最终失败。
通常,分割越准确,识别越成功。
表示和描述几乎总是跟随在分割步骤的输后边,通常这一输出是未加工的数据,其构成不是区域的边缘(区分一个图像区域和另一个区域的像素集)就是其区域本身的所有点。
无论哪种情况,把数据转换成适合计算机处理的形式都是必要的。
首先,必须确定数据是应该被表现为边界还是整个区域。
当注意的焦点是外部形状特性(如拐角和曲线)时,则边界表示是合适的。
当注意的焦点是内部特性(如纹理或骨骼形状)时,则区域表示是合适的。
则某些应用中,这些表示方法是互补的。
选择一种表现方式仅是解决把原始数据转换为适合计算机后续处理的形式的一部分。
为了描述数据以使感兴趣的特征更明显,还必须确定一种方法。
描述也叫特征选择,涉及提取特征,该特征是某些感兴趣的定量信息或是区分一组目标与其他目标的基础。
识别是基于目标的描述给目标赋以符号的过程。
如上文详细讨论的那样,我们用识别个别目标方法的开发推出数字图像处理的覆盖范围。
到目前为止,还没有谈到上面图2中关于先验知识及知识库与处理模块之间的交互这部分内容。
关于问题域的知识以知识库的形式被编码装入一个图像处理系统。
这一知识可能如图像细节区域那样简单,在这里,感兴趣的信息被定位,这样,限制性的搜索就被引导到寻找的信息处。
知识库也可能相当复杂,如材料检测问题中所有主要缺陷的相关列表或者图像数据库(该库包含变化检测应用相关区域的高分辨率卫星图像)。
除了引导每一个处理模块的操作,知识库还要控制模块间的交互。
这一特性上面图2中的处理模块和知识库间用双箭头表示。
相反单头箭头连接处理模块。
边缘检测边缘检测是图像处理和计算机视觉中的术语,尤其在特征检测和特征抽取领域,是一种用来识别数字图像亮度骤变点即不连续点的算法。
尽管在任何关于分割的讨论中,点和线检测都是很重要的,但是边缘检测对于灰度级间断的检测是最为普遍的检测方法。
虽然某些文献提过理想的边缘检测步骤,但自然界图像的边缘并不总是理想的阶梯边缘。
相反,它们通常受到一个或多个下面所列因素的影响:1.有限场景深度带来的聚焦模糊;2.非零半径光源产生的阴影带来的半影模糊;3.光滑物体边缘的阴影;4.物体边缘附近的局部镜面反射或者漫反射。
一个典型的边界可能是(例如)一块红色和一块黄色之间的边界;与之相反的是边线,可能是在另外一种不变的背景上的少数不同颜色的点。
在边线的每一边都有一个边缘。
在对数字图像的处理中,边缘检测是一项非常重要的工作。
如果将边缘认为是一定数量点亮度发生变化的地方,那么边缘检测大体上就是计算这个亮度变化的导数。
为简化起见,我们可以先在一维空间分析边缘检测。
在这个例子中,我们的数据是一行不同点亮度的数据。
例如,在下面的1维数据中我们可以直观地说在第4与第5个点之间有一个边界:如果光强度差别比第四个和第五个点之间小,或者说相邻的像素点之间光强度差更高,就不能简单地说相应区域存在边缘。
而且,甚至可以认为这个例子中存在多个边缘。
除非场景中的物体非常简单并且照明条件得到了很好的控制,否则确定一个用来判断两个相邻点之间有多大的亮度变化才算是有边界的阈值,并不是一件容易的事。
实际上,这也是为什么边缘检测不是一个简单问题的原因之一。
有许多用于边缘检测的方法,它们大致可分为两类:基于搜索和基于零交叉.基于搜索的边缘检测方法首先计算边缘强度,通常用一阶导数表示,例如梯度模;然后,用计算估计边缘的局部方向,通常采用梯度的方向,并利用此方向找到局部梯度模的最大值。
基于零交叉的方法找到由图像得到的二阶导数的零交叉点来定位边缘。
通常用拉普拉斯算子或非线性微分方程的零交叉点,我们将在后面的小节中描述.滤波做为边缘检测的预处理通常是必要的,通常采用高斯滤波。
已发表的边缘检测方法应用计算边界强度的度量,这与平滑滤波有本质的不同. 正如许多边缘检测方法依赖于图像梯度的计算,他们用不同种类的滤波器来估计x-方向和y-方向的梯度.一旦我们计算出导数之后,下一步要做的就是给出一个阈值来确定哪里是边缘位置。
阈值越低,能够检测出的边线越多,结果也就越容易受到图片噪声的影响,并且越容易从图像中挑出不相关的特性。
与此相反,一个高的阈值将会遗失细的或者短的线段。
如果边缘阈值应用于正确的的梯度幅度图像,生成的边缘一般会较厚,某些形式的边缘变薄处理是必要的。
然而非最大抑制的边缘检测,边缘曲线的定义十分模糊,边缘像素可能成为边缘多边形通过一个边缘连接(边缘跟踪)的过程。
在一个离散矩阵中,非最大抑制阶梯能够通过一种方法来实现,首先预测一阶导数方向、然后把它近似到45度的倍数、最后在预测的梯度方向比较梯度幅度。
一个常用的这种方法是带有滞后作用的阈值选择。