数学建模寒假作业答案
数学建模课后习题答案
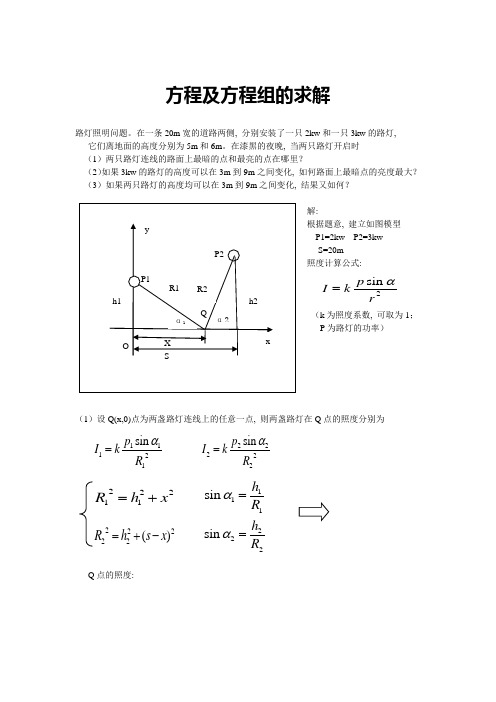
方程及方程组的求解路灯照明问题。
在一条20m 宽的道路两侧, 分别安装了一只2kw 和一只3kw 的路灯, 它们离地面的高度分别为5m 和6m 。
在漆黑的夜晚, 当两只路灯开启时 (1)两只路灯连线的路面上最暗的点和最亮的点在哪里? (2)如果3kw 的路灯的高度可以在3m 到9m 之间变化, 如何路面上最暗点的亮度最大? (3)如果两只路灯的高度均可以在3m 到9m 之间变化, 结果又如何?解:根据题意, 建立如图模型P1=2kw P2=3kw S=20m 照度计算公式:2sin r p k I α= (k 为照度系数, 可取为1;P 为路灯的功率)(1)设Q(x,0)点为两盏路灯连线上的任意一点, 则两盏路灯在Q 点的照度分别为21111sin R p k I α= 22222sin R p k I α=22121x h R += 111sin R h =α22222)(x s h R -+= 222sin R h =αQ 点的照度:3232322222322111))20(36(18)25(10))((()(()(x x x s h h P x h h P x I -+++=-+++=要求最暗点和最亮点, 即为求函数I(x)的最大值和最小值, 所以应先求出函数的极值点5252522222522111'))20(36()20(54)25(30))(()(3)(3)(x x x x x s h x s h P x h x h P x I -+-++-=-+-++-=利用MATLAB 求得0)('=x I 时x 的值代码:s=solve('(-30*x)/((25+x^2)^(5/2))+(54*(20-x))/((36+(20-x)^2)^(5/2))'); s1=vpa(s,8); s1运行结果: s1 =19.97669581 9.338299136 8.538304309-11.61579012*i .2848997038e-1综上, x=9.33m 时, 为最暗点;x=19.97m 时, 为最亮点。
数学建模题目及答案
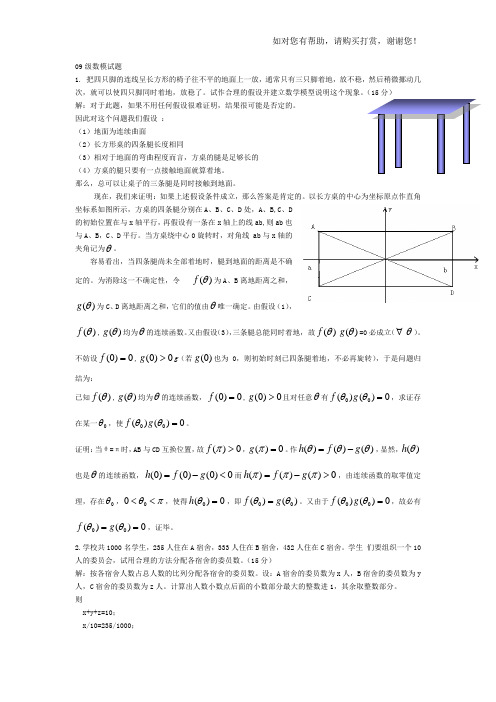
09级数模试题1. 把四只脚的连线呈长方形的椅子往不平的地面上一放,通常只有三只脚着地,放不稳,然后稍微挪动几次,就可以使四只脚同时着地,放稳了。
试作合理的假设并建立数学模型说明这个现象。
(15分)解:对于此题,如果不用任何假设很难证明,结果很可能是否定的。
因此对这个问题我们假设 :(1)地面为连续曲面(2)长方形桌的四条腿长度相同(3)相对于地面的弯曲程度而言,方桌的腿是足够长的(4)方桌的腿只要有一点接触地面就算着地。
那么,总可以让桌子的三条腿是同时接触到地面。
现在,我们来证明:如果上述假设条件成立,那么答案是肯定的。
以长方桌的中心为坐标原点作直角坐标系如图所示,方桌的四条腿分别在A 、B 、C 、D 处,A 、B,C 、D的初始位置在与x 轴平行,再假设有一条在x 轴上的线ab,则ab 也与A 、B ,C 、D 平行。
当方桌绕中心0旋转时,对角线 ab 与x 轴的夹角记为θ。
容易看出,当四条腿尚未全部着地时,腿到地面的距离是不确定的。
为消除这一不确定性,令 ()f θ为A 、B 离地距离之和,()g θ为C 、D 离地距离之和,它们的值由θ唯一确定。
由假设(1),()f θ,()g θ均为θ的连续函数。
又由假设(3),三条腿总能同时着地, 故()f θ()g θ=0必成立(∀θ)。
不妨设(0)0f =,(0)0g >g (若(0)g 也为0,则初始时刻已四条腿着地,不必再旋转),于是问题归结为:已知()f θ,()g θ均为θ的连续函数,(0)0f =,(0)0g >且对任意θ有00()()0f g θθ=,求证存在某一0θ,使00()()0f g θθ=。
证明:当θ=π时,AB 与CD 互换位置,故()0f π>,()0g π=。
作()()()h f g θθθ=-,显然,()h θ也是θ的连续函数,(0)(0)(0)0h f g =-<而()()()0h f g πππ=->,由连续函数的取零值定理,存在0θ,00θπ<<,使得0()0h θ=,即00()()f g θθ=。
数学建模习题集及标准答案
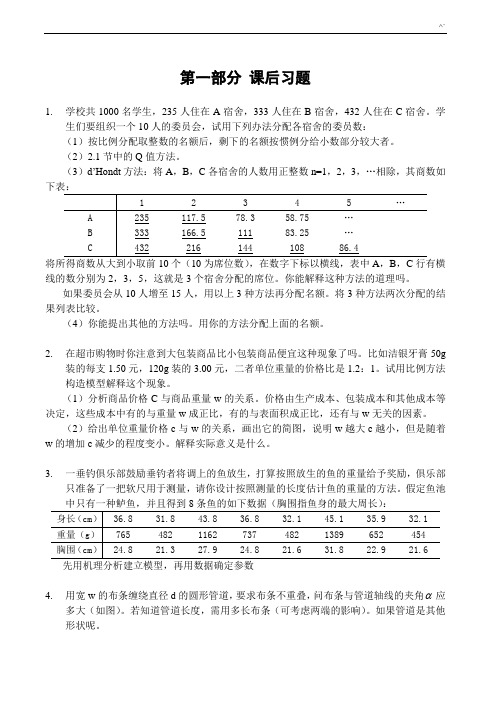
3.动态模型:描述对象特征随时间(空间)的演变过程,分析对象特征的变化规律,预报对象特征的未来性态,研究控制对象特征的手段;微分方程建模:模根据函数及其变化率之间的关系确定函数,根据建模目的和问题分析作出简化假设,按照内在规律或用类比法建立微分方程。
4.按照你的观点应从那几个方面来建立传染病模型。
5.叙述Leslie人口模型的特点。并讨论稳定状况下种群的增长规律。
6.试比较连续形式的阻滞增长模型(Logistic模型)和离散形式阻滞增长模型,并讨论离散形式阻滞增长模型平衡点及其稳定性。
第二部分
1.优点:短期预报比较准确;缺点:不适合中长期预报;原因:预报时假设人口增长率为常数,没有考虑环境对人口增长的制约作用。
(4)你能提出其他的方法吗。用你的方法分配上面的名额。
2.在超市购物时你注意到大包装商品比小包装商品便宜这种现象了吗。比如洁银牙膏50g装的每支1.50元,120g装的3.00元,二者单位重量的价格比是1.2:1。试用比例方法构造模型解释这个现象。
(1)分析商品价格C与商品重量w的关系。价格由生产成本、包装成本和其他成本等决定,这些成本中有的与重量w成正比,有的与表面积成正比,还有与w无关的因素。
根据上述分析我们可以看出,该博弈比较明确可以预测的结果有这样几种情况:
(1) ,此时本博弈的结果是乙在第一阶段不愿意借给对方,结束博弈,双方得益
(1,0),不管这时候b的值是多少;(2) ,此时博弈的结果仍然是乙在第一阶段选择不借,结束博弈,双方得益(1,0);(3) ,此时博弈的结果是乙在第一阶段选择借,甲在第二阶段选择不分,乙在第三阶段选择打,最后结果是双方得益
数学建模答案(完整版)
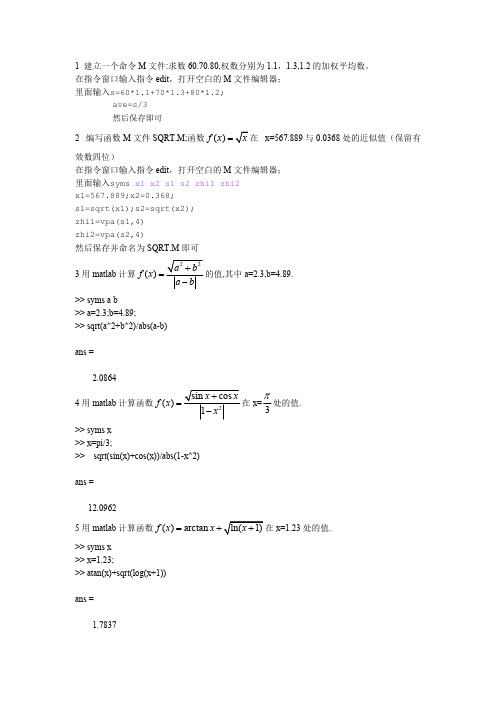
1 建立一个命令M 文件:求数60.70.80,权数分别为1.1,1.3,1.2的加权平均数。
在指令窗口输入指令edit ,打开空白的M 文件编辑器;里面输入s=60*1.1+70*1.3+80*1.2;ave=s/3然后保存即可2 编写函数M 文件SQRT.M;函数 x=567.889与0.0368处的近似值(保留有()f x =效数四位)在指令窗口输入指令edit ,打开空白的M 文件编辑器;里面输入syms x1 x2 s1 s2 zhi1 zhi2 x1=567.889;x2=0.368;s1=sqrt(x1);s2=sqrt(x2);zhi1=vpa(s1,4)zhi2=vpa(s2,4)然后保存并命名为SQRT.M 即可3用matlab 计算的值,其中a=2.3,b=4.89.()f x >> syms a b >> a=2.3;b=4.89;>> sqrt(a^2+b^2)/abs(a-b)ans = 2.08644用matlab 计算函数在x=处的值.()f x =3π>> syms x >> x=pi/3;>> sqrt(sin(x)+cos(x))/abs(1-x^2)ans = 12.09625用matlab 计算函数在x=1.23处的值.()arctan f x x =+>> syms x >> x=1.23;>> atan(x)+sqrt(log(x+1))ans = 1.78376 用matlab 计算函数在x=-2.1处的值.()()f x f x ==>> syms x >> x=-2.1;>> 2-3^x*log(abs(x))ans =1.92617 用蓝色.点连线.叉号绘制函数在[0,2]上步长为0.1的图像.>> syms x y>> x=0:0.2:2;y=2*sqrt(x);>> plot(x,y,'b.-')8 用紫色.叉号.实连线绘制函数在上步长为0.2的图像.ln 10y x =+[20,15]-->> syms x y>> x=-20:0.2:-15;y=log(abs(x+10));>> plot(x,y,'mx-')ln 10[20,y x =+--9 用红色.加号连线 虚线绘制函数在[-10,10]上步长为0.2的图像.sin(22x y π=->> syms x y;>> x=-10:0.2:10;y=sin(x/2-pi/2);>> plot(x,y,'r+--')10用紫红色.圆圈.点连线绘制函数在上步长为0.2的图像.sin(2)3y x π=+[0,4]πsin(2)sin()[0,4]322x y x y πππ=+=->> syms x y >> x=0:0.2:4*pi;y=sin(2*x+pi/3);>> plot(x,y,'mo-.')11 在同一坐标中,用分别青色.叉号.实连线与红色.星色.虚连线绘制y=与.y =>> syms x y1 y2>> x=0:pi/50:2*pi;y1=cos(3*sqrt(x));y2=3*cos(sqrt(x));>> plot(x,y1,'cx-',x,y2,'r*--')12 在同一坐标系中绘制函数这三条曲线的图标,并要求用两种方法加234,,y x y x y x ===各种标注.234,,y x y x y x ===>> syms x y1 y2 y3;>> x=-2:0.1:2;y1=x.^2;y2=x.^3;y3=x.^4;plot(x,y1,x,y2,x,y3);13 作曲线的3维图像2sin x t y t z t ⎧=⎪=⎨⎪=⎩>> syms x y t z >> t=0:1/50:2*pi;>> x=t.^2;y=sin(t);z=t;>> stem3(x,y,z)14 作环面在上的3维图像(1cos )cos (1cos )sin sin x u v y u v z u =+⎧⎪=+⎨⎪=⎩(0,2)(0,2)ππ⨯>> syms x y u v z>> u=0:pi/50:2*pi;v=0:pi/50:2*pi;>>x=(1+cos(u)).*cos(v);y=(1+cos(u)).*sin(v);z=sin(u);>> plot3(x,y,z)15 求极限0lim x +→0lim x +→>> syms x y >> y=sin(2^0.5*x)/sqrt(1-cos(x));>> limit(y,x,0,'right') ans = 216 求极限1201lim (3x x +→>> syms y x >> y=(1/3)^(1/(2*x));>> limit(y,x,0,'right') ans = 017求极限lim x >> syms x y >> y=(x*cos(x))/sqrt(1+x^3);>> limit(y,x,+inf) ans = 018 求极限21lim (1x x x x →+∞+->> syms x y >> y=((x+1)/(x-1))^(2*x);>> limit(y,x,+inf) ans = exp(4)19 求极限01cos 2lim sin x xx x →->> syms x y >> y=(1-cos(2*x))/(x*sin(x));>> limit(y,x,0) ans = 220 求极限 x →>> syms x y >> y=(sqrt(1+x)-sqrt(1-x))/x;>> limit(y,x,0) ans = 121 求极限2221lim 2x x x x x →+∞++-+>> syms x y >> y=(x^2+2*x+1)/(x^2-x+2);>> limit(y,x,+inf) ans = 122 求函数y=的导数5(21)arctan x x -+>> syms x y >> y=(2*x-1)^5+atan(x);>> diff(y) ans = 10*(2*x - 1)^4 + 1/(x^2 + 1)23 求函数y=的导数2tan 1x x y x=+>> syms y x>> y=(x*tan(x))/(1+x^2);>> diff(y)ans =tan(x)/(x^2 + 1) + (x*(tan(x)^2 + 1))/(x^2 + 1) - (2*x^2*tan(x))/(x^2 + 1)^224 求函数的导数3tan x y e x -=>> syms y x >> y=exp^(-3*x)*tan(x)>> y=exp(-3*x)*tan(x) y = exp(-3*x)*tan(x) >> diff(y) ans = exp(-3*x)*(tan(x)^2 + 1) - 3*exp(-3*x)*tan(x)25 求函数y=在x=1的导数22ln sin 2x x π+>> syms x y >> y=(1-x)/(1+x);>> diff(y,x,2) ans = 2/(x + 1)^2 - (2*(x - 1))/(x + 1)^3 >> syms x y >> y=2*log(x)+sin(pi*x/2)^2;>> dxdy=diff(y) dxdy = 2/x + pi*cos((pi*x)/2)*sin((pi*x)/2)zhi=subs(dxdy,1)zhi = 226 求函数y=的二阶导数01cos 2lim sin x x x x →-11x x-+>> syms x y>> y=(1-x)/(1+x);>> diff(y,x,2) ans = 2/(x + 1)^2 - (2*(x - 1))/(x + 1)^327 求函数的导数;>> syms x y >> y=((x-1)^3*(3+2*x)^2/(1+x)^4)^0.2;>> diff(y) ans = (((8*x + 12)*(x - 1)^3)/(x + 1)^4 + (3*(2*x + 3)^2*(x - 1)^2)/(x + 1)^4 - (4*(2*x + 3)^2*(x - 1)^3)/(x + 1)^5)/(5*(((2*x + 3)^2*(x - 1)^3)/(x + 1)^4)^(4/5))28在区间()内求函数的最值.,-∞+∞43()341f x x x =-+>> f='-3*x^4+4*x^3-1';>> [x,y]=fminbnd(f,-inf,inf)x =NaN y = NaN >> f='3*x^4-4*x^3+1';>> [x,y]=fminbnd(f,-inf,inf)x = NaN y = NaN29在区间(-1,5)内求函数发的最值.()(f x x =->> f='(x-1)*x^0.6';>> [x,y]=fminbnd(f,-1,5)x =0.3750y = -0.3470>> >> f='-(x-1)*x^0.6';>> [x,y]=fminbnd(f,-1,5)x = 4.9999y = -10.505930 求不定积分(ln 32sin )x x dx -⎰(ln 32sin )x x dx -⎰>> syms x y >> y=log(3*x)-2*sin(x);>> int(y) ans = 2*cos(x) - x + x*log(3) + x*log(x)31求不定积分2sin x e xdx ⎰>> syms x y>> y=exp(x)*sin(x)^2;>> int(y)ans =-(exp(x)*(cos(2*x) + 2*sin(2*x) - 5))/1032. 求不定积分 >> syms x y >> y=x*atan(x)/(1+x)^0.5;>> int(y)Warning: Explicit integral could not be found. ans = int((x*atan(x))/(x + 1)^(1/2), x)33.计算不定积分2(2cos )x x x e dx --⎰>> syms x y >> y=1/exp(x^2)*(2*x-cos(x));>> int(y)Warning: Explicit integral could not be found. ans = int(exp(-x^2)*(2*x - cos(x)), x)34.计算定积分10(32)xe x dx -+⎰>> syms x y >> y=exp(-x)*(3*x+2);>> int(y,0,1) ans = 5 - 8*exp(-1)10(32)x e x dx -+⎰35.计算定积分0x →120(1)cos x arc xdx+⎰>> syms y x>> y=(x^2+1)*acos(x);>> int(y,0,1)ans =11/936.计算定积分10cos ln(1)x x dx +⎰>> syms x y >> y=(cos(x)*log(x+1));>> int(y,0,1)Warning: Explicit integral could not be found. ans = int(log(x + 1)*cos(x), x == 0..1)37计算广义积分;2122x x dx +∞++-∞⎰>> syms y x >> y=(1/(x^2+2*x+2));>> int(y,-inf,inf) ans = pi 38.计算广义积分;20x dx x e +∞-⎰>> syms x y>> y=x^2*exp(-x);>> int(y,0,+inf)ans =2。
数学建模答案与解析
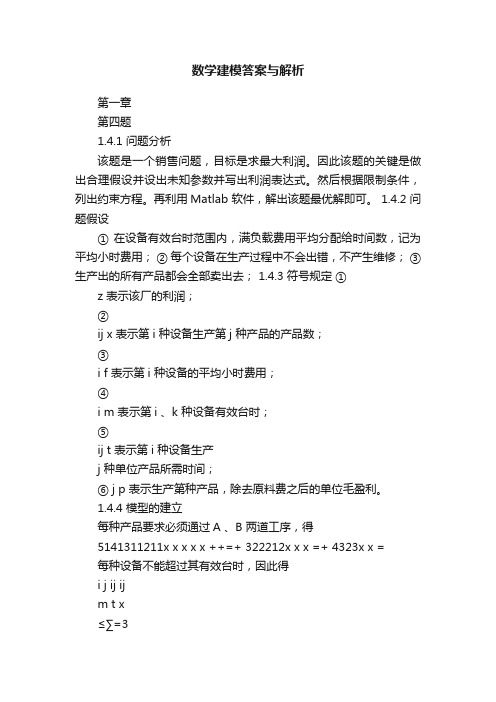
数学建模答案与解析第一章第四题1.4.1 问题分析该题是一个销售问题,目标是求最大利润。
因此该题的关键是做出合理假设并设出未知参数并写出利润表达式。
然后根据限制条件,列出约束方程。
再利用Matlab 软件,解出该题最优解即可。
1.4.2 问题假设① 在设备有效台时范围内,满负载费用平均分配给时间数,记为平均小时费用;② 每个设备在生产过程中不会出错,不产生维修;③ 生产出的所有产品都会全部卖出去; 1.4.3 符号规定①z 表示该厂的利润;②ij x 表示第i 种设备生产第j 种产品的产品数;③i f 表示第i 种设备的平均小时费用;④i m 表示第i 、k 种设备有效台时;⑤ij t 表示第i 种设备生产j 种单位产品所需时间;⑥ j p 表示生产第种产品,除去原料费之后的单位毛盈利。
1.4.4 模型的建立每种产品要求必须通过A 、B 两道工序,得5141311211x x x x x ++=+ 322212x x x =+ 4323x x =每种设备不能超过其有效台时,因此得i j ij ijm t x≤∑=3*( i =1、2、3、4、5)由于每个产品必须由A 、B 两道工序才能完成,因此经过任一工序的所有产品数与总的产品数相同。
因此,在计算总收入时,就用某一工序加工产品总数即可。
这里选用A 工序。
故所得的最大利润为max j i j ijp xz *2131∑∑===-ii i j ij ijf t x∑∑==5131**因此,模型的简化如下:5143413231232221121165.00696.15526.015.1625.09148.13611.17753.0 15.175.0max x x x x x x x x x x z +++++++++=5141311211x x x x x ++=+ 322212x x x =+ 4323x x =i j ij ijm t x≤∑=31* ( i =1 2 3 4 5)0≥ij x1.4.5 利用Matlab 解得结果如下,源程序见附件一..t s 51732458850003245002300120051434132312322211211=== =======x x x x x x x x x x总的利润为1147元 1.4.6 问题改进在该题做的过程中,超负荷费用安排的不合理。
数学建模 建模答案.docx
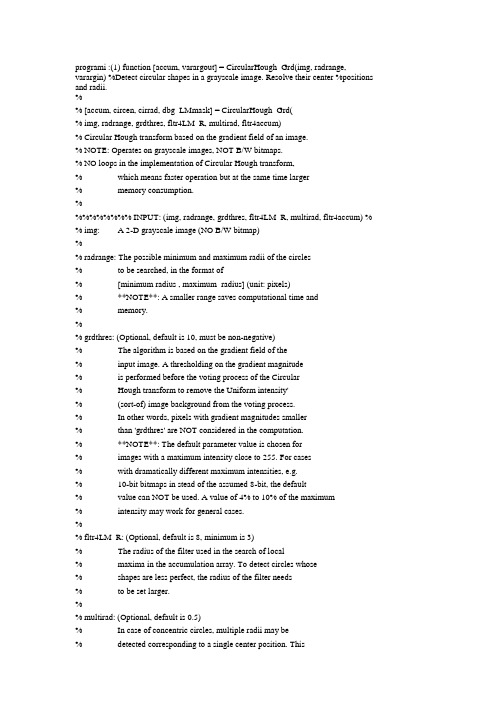
programi :(1) function [accum, varargout] = CircularHough_Grd(img, radrange, varargin) %Detect circular shapes in a grayscale image. Resolve their center %positions and radii.%% [accum, circen, cirrad, dbg_LMmask] = CircularHough_Grd(% img, radrange, grdthres, fltr4LM_R, multirad, fltr4accum)% Circular Hough transform based on the gradient field of an image.% NOTE: Operates on grayscale images, NOT B/W bitmaps.% NO loops in the implementation of Circular Hough transform,% which means faster operation but at the same time larger% memory consumption.%%%%%%%%% INPUT: (img, radrange, grdthres, fltr4LM_R, multirad, fltr4accum) % % img: A 2-D grayscale image (NO B/W bitmap)%% radrange: The possible minimum and maximum radii of the circles% to be searched, in the format of% [minimum radius , maximum_radius] (unit: pixels)% **NOTE**: A smaller range saves computational time and% memory.%% grdthres: (Optional, default is 10, must be non-negative)% The algorithm is based on the gradient field of the% input image. A thresholding on the gradient magnitude% is performed before the voting process of the Circular% Hough transform to remove the Uniform intensity'% (sort-of) image background from the voting process.% In other words, pixels with gradient magnitudes smaller% than 'grdthres' are NOT considered in the computation.% **NOTE**: The default parameter value is chosen for% images with a maximum intensity close to 255. For cases% with dramatically different maximum intensities, e.g.% 10-bit bitmaps in stead of the assumed 8-bit, the default% value can NOT be used. A value of 4% to 10% of the maximum% intensity may work for general cases.%% fltr4LM_R: (Optional, default is 8, minimum is 3)% The radius of the filter used in the search of local% maxima in the accumulation array. To detect circles whose% shapes are less perfect, the radius of the filter needs% to be set larger.%% multirad: (Optional, default is 0.5)% In case of concentric circles, multiple radii may be% detected corresponding to a single center position. This% argument sets the tolerance of picking up the likely% radii values. It ranges from 0.1 to 1, where 0.1% corresponds to the largest tolerance, meaning more radii % values will be detected, and 1 corresponds to the smallest % tolerance, in which case only the "principal" radius will% be picked up.%% fltr4accum: (Optional. A default filter will be used if not given)% Filter used to smooth the accumulation array. Depending % on the image and the parameter settings, the accumulation % array built has different noise level and noise pattern% (e.g. noise frequencies). The filter should be set to an% appropriately size such that ifs able to suppress the% dominant noise frequency.%%%%%%%%% OUTPUT: [accum, circen, cirrad, dbg_LMmask]%% accum: The result accumulation array from the Circular Hough% transform. The accumulation array has the same dimension % as the input image.%% circen: (Optional)% Center positions of the circles detected. Is a N-by-2% matrix with each row contains the (x, y) positions% of a circle. For concentric circles (with the same center% position), say k of them, the same center position will% appear k times in the matrix.%% cirrad: (Optional)% Estimated radii of the circles detected. Is a N-by-1% column vector with a one-to-one correspondance to the% output tircen*. A value 0 for the radius indicates a% failed detection of the circle's radius.%% dbg_LMmask: (Optional, for debugging purpose)% Mask from the search of local maxima in the accumulation % array.%%%%%%%%%% EXAMPLE #0:% rawimg = imread('TestImg_CHT_a2.bmp');% tic;% [accum, circen, cirrad] = CircularHough_Grd(rawimg, [15 60]);% toe;% figure(l); imagesc(accum); axis image;% title(,Accumulation Array from Circular Hough Transfbrm,);% figure(2); imagesc(rawimg); colormap(,gray,); axis image;% hold on;% plot(circen(:,l), circen(:,2), *r+');% for k = 1 : size(circen, 1),% DrawCircle(circen(k, 1), circen(k,2), cirrad(k), 32,,b」);% end% hold off;% title([*Raw Image with Circles Detected% '(center positions and radii marked)*]);% figure(3); surf(accum, 'EdgeColoF, hone'); axis ij;% title('3-D View of the Accumulation Array*);%% COMMENTS ON EXAMPLE #0:% Kind of an easy case to handle. To detect circles in the image whose% radii range from 15 to 60. Default values for arguments 'grdthres',% 'fltr4LM_R', 'multirad* and ,fltr4accum, are used.%%%%%%%%%% EXAMPLE #1:% rawimg = imread('TestImg_CHT_a3.bmp');% tic;% [accum, circen, cirrad] = CircularHough_Grd(rawimg, [15 60], 10, 20);% toe;% figure(l); imagesc(accum); axis image;% title(,Accumulation Array from Circular Hough Transfbrm,);% figure(2); imagesc(rawimg); colormap('gray'); axis image;% hold on;% plot(circen(:,l), circen(:,2), T+');% for k = 1 : size(circen, 1),% DrawCircle(circen(k, 1), circen(k,2), cirrad(k), 32, 'b-');% end% hold off;% title([*Raw Image with Circles Detected% '(center positions and radii marked)*]);% figure(3); surf(accum, 'EdgeColoF, hone'); axis ij;% title(*3-D View of the Accumulation Array*);%% COMMENTS ON EXAMPLE #1:% The shapes in the raw image are not very good circles. As a result,% the profile of the peaks in the accumulation array are kind of% 'stumpy', which can be seen clearly from the 3-D view of the% accumulation array, (As a comparison, please see the sharp peaks in % the accumulation array in example #0) To extract the peak positions % nicely, a value of 20 (default is 8) is used for argument 'fltr4LM_R', % which is the radius of the filter used in the search of peaks.%%%%%%%%%% EXAMPLE #2:% rawimg = imread(,TestImg_CHT_b3 .bmp1);% fltr4img = [1 1 1 1 1; 1 2 2 2 1; 1 2 4 2 1; 1 2 2 2 1; 1 1 1 1 1];% fltr4img = fltr4img / sum(fltr4img(:));% imgfltrd = filter2( fltr4img , rawimg );% tic;% [accum, circen, cirrad] = CircularHough_Grd(imgfltrd, [15 80], 8, 10); % toe;% figure(l); imagesc(accum); axis image;% title(,Accumulation Array from Circular Hough Transfbrm,);% figure(2); imagesc(rawimg); colormap('gray'); axis image;% hold on;% plot(circen(:,l), circen(:,2), T+');% for k = 1 : size(circen, 1),% DrawCircle(circen(k, 1), circen(k,2), cirrad(k), 32, 'b-');% end% hold off;% title([*Raw Image with Circles Detected% '(center positions and radii marked)*]);%% COMMENTS ON EXAMPLE #2:% The circles in the raw image have small scale irregularities along % the edges, which could lead to an accumulation array that is bad for % local maxima detection. A 5-by-5 filter is used to smooth out the % small scale irregularities. A blurred image is actually good for the % algorithm implemented here which is based on the image's gradient % field.%%%%%%%%%% EXAMPLE #3:% rawimg = imread('TestImg_CHT_c3.bmp');% fltr4img = [1 1 1 1 1; 1 2 2 2 1; 1 2 4 2 1; 1 2 2 2 1; 1 1 1 1 1];% fltr4img = fltr4img / sum(fltr4img(:));% imgfltrd = filter2( fltr4img , rawimg );% tic;% [accum, circen, cirrad]=...% CircularHough_Grd(imgfltrd, [15 105], 8, 10, 0.7);% toe;% figure(l); imagesc(accum); axis image;% figure(2); imagesc(rawimg); colormap(,gray,); axis image;% hold on;% plot(circen(:,l), circen(:,2), *r+');% for k = 1 : size(circen, 1),% DrawCircle(circen(k, 1), circen(k,2), cirrad(k), 32,,b」);% end% hold off;% title([*Raw Image with Circles Detected% '(center positions and radii marked)*]);%% COMMENTS ON EXAMPLE #3:% Similar to example #2, a filtering before circle detection works for% noisy image too. 'multirad* is set to 0.7 to eliminate the false% detections of the circles* radii.%%%%%%%%%% BUG REPORT:% This is a beta version. Please send your bug reports, comments and% suggestions to pengtao@ . Thanks.%%%%%%%%%%% INTERNAL PARAMETERS:% The INPUT arguments are just part of the parameters that are used by% the circle detection algorithm implemented here. Variables in the code% with a prefix ,prm_, in the name are the parameters that control the% judging criteria and the behavior of the algorithm. Default values for% these parameters can hardly work for all circumstances. Therefore, at% occasions, the values of these INTERNAL PARAMETERS (parameters that% are NOT exposed as input arguments) need to be fine-tuned to make% the circle detection work as expected.% The following example shows how changing an internal parameter could% influence the detection result.% 1. Change the value of the internal parameter 'prm LM LoBndRa* to 0.4% (default is 0.2)% 2. Run the following matlab code:% fltr4accum = [1 2 1; 2 6 2; 1 2 1];% fltr4accum = fltr4accum / sum(fltr4accum(:));% rawimg = imread(,Frame_0_0022jportion.jpg,);% tic;% [accum, circen] = CircularHough_Grd(rawimg,...% [4 14], 10, 4, 0.5, fltr4accum);% toe;% figure(l); imagesc(accum); axis image;% title(*Accumulation Array from Circular Hough Transform*);% figure(2); imagesc(rawimg); colormap(,gray,); axis image;% hold on; plot(circen(:,l), circen(:,2), "); hold off;% title('Raw Image with Circles Detected (center positions marked)*);% 3. See how different values of the parameter 'prm LM LoBndRa* could % influence the result.% Author: Tao Peng% Department of Mechanical Engineering% University of Maryland, College Park, Maryland 20742, USA% pengtao@% Version: Beta Revision: Mar. 07, 2007%%%%%%%% Arguments and parameters %%%%%%%%%%%%%%%%%%%%%%%%%%%%%%%%%%%% Validation of argumentsif ndims(img)〜=2 || 〜isnumeric(img),error(*CircularHough_Grd: "img" has to be 2 dimensionaf);endif 〜all(size(img) >= 32),erro^'CircularHough Grd: "img" has to be larger than 32-by-32');endif numel(radrange)〜=2 || -isnumeric(radrange),error([*CircularHough_Grd: "radrange" has to be \ ...'a two-element vector1]);endprm_r_range = sort(max( [0,0;radrange( 1 ),radrange(2)]));% Parameters (default values)prmgrdthres = 10;prmfltrLMR = 8;prmmultirad = 0.5;funccompucen = true;funccompuradii = true;% Validation of argumentsvapgrdthres = 1;if nargin > (1 + vap_grdthres),if isnumeric(varargin{vap grdthres}) && ...varargin(vap grdthres} (1) >= 0,prm_grdthres = varargin {vapgrdthres} (1);elseerror(['CircularHough_Grd: "grdthres" has to be'a non-negative number1]);endendvap_fltr4LM = 2; % filter for the search of local maximaif nargin > (1 + vap_fltr4LM),if isnumeric(varargin{vap_fltr4LM}) && varargin{vap_fltr4LM}(1) >= 3, prmfltrLMR = varargin{vap_fltr4LM} (1);elseerror([,CircularHough_Grd: n fltr4LM_R n has to belarger than or equal to 3']);endendvap_multirad = 3;if nargin > (1 + vap multirad),if isnumeric(varargin{vap_multirad}) && ...varargin{vap multirad}(1) >= 0.1 && ...varargin {vap multirad} (1) <= 1,prmmultirad = varargin {vap_mul tirad} (1);elseerror(['CircularHough_Grd: "multirad" has to be'within the range [0.1, 1]*]);endendvap_fltr4accum = 4; % filter for smoothing the accumulation arrayif nargin > (1 + vap_fltr4accum),if isnumeric(varargin{vap_fltr4accum}) && ...ndims(varargin{vap_fltr4accum}) == 2 && ...all(size(varargin {vap_fltr4accum}) >= 3),fltr4accum = varargin {vap_fltr4accum};elseerror(['CircularHough_Grd: n fltr4accum n has to be \ ...*a 2-D matrix with a minimum size of 3-by-3']);endelse% Default filter (5-by-5)fltr4accum = ones(5,5);fltr4accum(2:4,2:4) = 2;fltr4accum(3,3) = 6;end func_compu_cen = (nargout > 1 );func_compu_radii = (nargout > 2 );% Reserved parametersdbg on = false; % debug information dbgbfigno = 4;if nargout > 3, dbg on = true; end%%%%%%%% Buildingaccumulation array %%%%%%%%%%%%%%%%%%%%%%%%%%%%%%%%% Convert the image to single if it is not of% class float (single or double) img_is_double = isa(img, double');if ~(img_is_double || isa(img, 'single')),imgf = single(img);end% Compute the gradient and the magnitude of gradientif img_is_double,[grdx, grdy] = gradient(img);else[grdx, grdy] = gradient(imgf);endgrdmag = sqrt(grdx.A2 + grdy.A2);% Get the linear indices, as well as the subscripts, of the pixels% whose gradient magnitudes are larger than the given threshold grdmasklin = find(grdmag > prm_grdthres);[grdmask_ldxl, grdmask_IdxJ] = ind2sub(size(grdmag), grdmasklin);% Compute the linear indices (as well as the subscripts) of% all the votings to the accumulation array.% The Matlab function 'accumarray* accepts only double variable, % so all indices are forced into double at this point.% A row in matrix ,lin2accum_aJ, contains the J indices (into the % accumulation array) of all the votings that are introduced by a % same pixel in the image. Similarly with matrix linZaccum aP. rr_41inaccum = double( prm_r_range );linaccum_dr = [ (-rr_41inaccum(2) + 0.5) : -rr_41inaccum(l),... (rr_41inaccum(l) + 0.5) : rr_41inaccum(2)];lin2accum_aJ = floor(...double(grdx(grdmasklin)./grdmag(grdmasklin)) * linaccum_dr + ...repmat( double(grdmask_IdxJ)+0.5 , [ 1 ,length(linaccum_dr)])...);lin2accum_al = floor(...double(grdy(grdmasklin)./grdmag(grdmasklin)) * linaccum dr + ...repmat( double(grdmask_IdxI)+0.5 , [1 ,length(linaccum_dr)])...);% Clip the votings that are out of the accumulation arraymask_valid_a J al =...lin2accum_aJ > 0 & lin2accum_aJ < (size(grdmag,2) + 1) & ...Iin2accum_al > 0 & lin2accum_al < (size(grdmag,l) + 1);mask_valid_aJaI_reverse =〜mask_valid_aJaI;lin2accum_aJ = lin2accum_aJ .* maskvalida J al + maskvalidaJ alreverse;lin2accum_al = lin2accum_al .* mask_valid_aJaI + mask_valid_aJaI_reverse;clear mask_valid_aJ alre verse;% Linear indices (of the votings) into the accumulation arraylin2accum = sub2ind( size(grdmag), lin2accum_al, lin2accum_aJ );lin2accum_size = size( lin2accum );lin2accum = reshape( lin2accum, [numel(lin2accum),l]);clear lin2accum_al lin2accum_aJ;% Weights of the votings, currently using the gradient maginitudes% but in fact any scheme can be used (application dependent)weight4accum =...repmat( double(grdmag(grdmasklin)) , [lin2accum_size(2), 1 ]) .* ...mask_valid_aJ al(:);clear mask_valid_aJaI;% Build the accumulation array using Matlab function 'accumarray'accum = accumarray( lin2accum , weight4accum );accum = [ accum ; zeros( numel(grdmag) - numel(accum), 1 )];accum = reshape( accum, size(grdmag));%%%%%%%% Locating local maxima in the accumulation array %%%%%%%%%%%%% Stop if no need to locate the center positions of circlesif ~func_compu_cen,return;endclear lin2accum weight4accum;% Parameters to locate the local maxima in the accumulation array% — Segmentation of 'accum' before locating LM prmuseaoi = true;prm_aoithres_s = 2;prm aoiminsize = floor(min([ min(size(accum)) * 0.25,... prm_r_range(2) * 1.5 ]));% — Filter for searching for local maxima prmfltrLMs = 1.35;prm fltrLM r = ceil( prm fltrLM R * 0.6 );prm fltrLM npix = max([ 6, ceil((prm_fltrLM_R/2)A 1.8)]);% — Lower bound of the intensity of local maximaprm LM LoBndRa = 0.2; % minimum ratio of LM to the max of'accum'% Smooth the accumulation arrayfltr4accum = fltr4accum / sum(fltr4accum(:));accum = filter2( fltr4accum, accum );% Select a number of Areas-Of^Interest from the accumulation array if prmuseaoi, % Threshold value for 'accum1prm_llm_thresl = prm_grdthres * prm_aoithres_s;% Thresholding over the accumulation array accummask = ( accum > prm llm thres 1 );% Segmentation over the mask[accumlabel, accum nRgn] = bwlabel( accummask, 8 );% Select AOIs from segmented regionsaccumAOI = ones(0,4);for k = 1 : accum nRgn,accumrgn lin = find( accumlabel = k);[accumrgn_ldxl, accumrgn_IdxJ]=...ind2sub( size(accumlabel), accumrgn lin);rgn top = min( accumrgn ldxl);rgn bottom = max( accumrgn_ldxl);rgn left = min( accumrgn ldxJ );rgn_right = max( accumrgn ldxJ );% The AOIs selected must satisfy a minimum sizeif ((rgn_right - rgn_left + 1) >= prm_aoiminsize && ...(rgn_bottom - rgn top + 1) >= prm aoiminsize ),accumAOI = [ accumAOI;...rgn top, rgn bottom, rgn left, rgn right ];endendelse% Whole accumulation array as the one AOIaccumAOI = [1, size(accum,l), 1, size(accum,2)];end% Thresholding of 'accum' by a lower boundprm LM LoBnd = max(accum(:)) * prm LM LoBndRa;% Build the filter for searching for local maxima fltr4LM = zeros(2 * prm_fltrLM_R + 1);[mesh4fLM_x, mesh4fLM_y] = meshgrid(-prm_fltrLM_R : prm fltrLM R);mesh4fLM_r = sqrt( mesh4fLM_x.A2 + mesh4fLM_y.A2 );fltr4LM_mask =...(mesh4fLM_r > prm_fltrLM_r & mesh4fLM_r <= prm fltrLM R );fltr4LM = fltr4LMfltr4LM_mask * (prm fltrLM s / sum(fltr4LM_mask(:)));if prm_fltrLM_R >= 4,fltr4LM_mask = ( mesh4fLM_r < (prm_fltrLM_r - 1));elsefltr4LM_mask = ( mesh4fLM_r < prm fltrLM r );endfltr4LM = fltr4LM + fltr4LM mask / sum(fltr4LM_mask(:));% **** Debug code (begin)if dbg_on,dbg_LMmask = zeros(size(accum));end% **** Debug code (end)% For each of the AOIs selected, locate the local maximacircen = zeros(0,2);fbrk = 1 : size(accumAOI, 1),aoi = accumAOI(k,:); % just for referencing convenience% Thresholding of 'accum* by a lower boundaccumaoi_LBMask =...(accum(aoi(l):aoi(2), aoi(3):aoi(4)) > prm LM LoBnd );% Apply the local maxima filtercandLM = conv2( accum(aoi( 1):aoi(2), aoi(3):aoi(4)),...fltr4LM, 'same*);candLM mask = ( candLM > 0 );% Clear the margins of 'candLM mask*candLM_mask([l :prm_fltrLM_R, (end-prm_fltrLM_R+l):end], :) = 0;candLM mask(:, [l:prm_fltrLM_R, (end-prm_fltrLM_R+l):end]) = 0;% **** Debug code (begin)if dbg_on,dbg_LMmask(aoi( 1 ):aoi(2), aoi(3):aoi(4))=...dbg_LMmask(aoi( 1 ):aoi(2), aoi(3):aoi(4)) + ...accumaoi LBMask + 2 * candLM mask;end% **** Debug code (end)% Group the local maxima candidates by adjacency, compute the% centroid position for each group and take that as the center% of one circle detected[candLM label, candLM nRgn] = bwlabel( candLM_mask, 8 );fbr ilabel = 1 : candLM nRgn,% Indices (to current AOI) of the pixels in the groupcandgrp masklin = find( candLM label == ilabel);[candgrp_ldxl, candgrp_IdxJ]=...ind2sub( size(candLM label), candgrp masklin );% Indices (to 'accum') of the pixels in the groupcandgrp_ldxl = candgrp_ldxl + ( aoi(l) - 1 );candgrp IdxJ = candgrp IdxJ + ( aoi(3) - 1 );candgrp_idx2acm =...sub2ind( size(accum) , candgrp ldxl, candgrp IdxJ );% Minimum number of qulified pixels in the groupif sum(accumaoi_LBMask(candgrp_masklin)) < prm_fltrLM_npix, continue;end% Compute the centroid positioncandgrp_acmsum = sum( accum(candgrp_idx2acm));cc_x = sum( candgrp IdxJ .* accum(candgrp_idx2acm) ) / ...candgrpacmsum;cc_y = sum( candgrp_ldxl .* accum(candgrp_idx2acm) ) / ...candgrpacmsum;circen = [circen; cc_x, cc_y];endend% **** Debug code (begin)if dbg_on,figure(dbg bfigno); imagesc(dbg LMmask); axis image;title(*Generated map of local maxima1);if size(accumAOI, 1) == 1,figure(dbg_bfigno+1);surf(candLM, 'EdgeColor1, hone'); axis ij;title(,Accumulation array after local maximum filtering*);endend% **** Debug code (end)%%%%%%%% Estimation of the Radii of Circles %%%%%%%%%%%%% Stop if no need to estimate the radii of circlesif ~func_compu_radii,varargout{l} = circen;return;end% Parameters for the estimation of the radii of circlesfltr4SgnCv=[2 1 1];fltr4SgnCv = fltr4SgnCv / sum(fltr4SgnCv);% Find circle's radius using its signature curve cirrad = zeros( size(circen,l), 1 );for k = 1 : size(circen,l),% Neighborhood region of the circle for building the sgn. curve circen_round = round( circen(k,:));SCvR IO = circen_round(2) - prm_r_range(2) - 1;ifSCvR_IO<l,SCvR_I0= 1;endSCvRIl = circen_round(2) + prm_r_range(2) + 1;if SCvR Il > size(grdx,l),SCvRIl = size(grdx,l);endSCvR JO = circen round(l) - prm_r_range(2) - 1;ifSCvR_JO<l,SCvRJO = 1;endSCvRJ 1 = circenround(l) + prm_r_range(2) + 1;if SCvR Jl > size(grdx,2),SCvRJl = size(grdx,2);end% Build the sgn. curveSgnCvMat_dx = repmat( (SCvR J0:SCvR J 1) - circen(k,l),...[SCvRJl - SCvRJO +1,1]);SgnCvMat_dy = repmat( (SCvR_IO:SCvR_Il)' - circen(k,2),...[1 , SCvRJl - SCvRJO + 1]);SgnCvMat_r = sqrt( SgnCvMat dx .A2 + SgnCvMat_dy .A2 );SgnCvMatrpl = round(SgnCvMatr) + 1;f4SgnCv = abs(...double(grdx(SCvR_IO:SCvR_Il, SCvRJO:SCvRJ 1)) .* SgnCvMat_dx + ...double(grdy(SCvR_IO:SCvR Il, SCvR JO:SCvR J 1)) .* SgnCvMat dy...)./ SgnCvMat r;SgnCv = accumarray( SgnCvMat rp 1(:) , f4SgnCv(:));SgnCv_Cnt = accumarray( SgnCvMat rp 1 (:) , ones(numel(f4SgnCv), 1));SgnCv_Cnt = SgnCv_Cnt + (SgnCv_Cnt == 0);SgnCv = SgnCv ./ SgnCv_Cnt;% Suppress the undesired entries in the sgn. curve% ― Radii that correspond to short arcsSgnCv = SgnCv .* ( SgnCv_Cnt >= (pi/4 * [O:(numel(SgnCv_Cnt)-1 )]*));% ― Radii that are out of the given rangeSgnCv( 1 : (round(prm_r_range( 1))+1) ) = 0;SgnCv( (round(prm_r_range(2))+1) : end ) = 0;% Get rid of the zero radius entry in the arraySgnCv = SgnCv(2:end);% Smooth the sgn. curveSgnCv = filtfilt( fltr4SgnCv , [1] , SgnCv );% Get the maximum value in the sgn. curveSgnCv_max = max(SgnCv);if SgnCv_max <= 0,cirrad(k) = 0;continue;end% Find the local maxima in sgn. curve by 1st order derivatives% ― Mark the ascending edges in the sgn. curve as Is and% ― descending edges as OsSgnCv AscEdg = ( SgnCv(2:end) - SgnCv(l:(end-l)) ) > 0;% ― Mark the transition (ascending to descending) regionsSgnCv LMmask = [ 0; 0; SgnCv_AscEdg(l:(end-2)) ] & (〜SgnCv_AscEdg);SgnCv LMmask = SgnCvLMmask & [ SgnCv_LMmask(2:end); 0 ];% Incorporate the minimum value requirementSgnCvLMmask = SgnCvLMmask & ...(SgnCv(l:(end-l)) >= (prm_multirad * SgnCv_max));% Get the positions of the peaksSgnCv LMPos = sort( find(SgnCv_LMmask));% Save the detected radiiif isempty(SgnCvLMPos),cirrad(k) = 0;elsecirrad(k) = SgnCvLMPos(end);for i radii = (length(SgnCv LMPos) - 1) : -1 : 1,circen = [ circen; circen(k,:)];cirrad = [ cirrad; SgnCv_LMPos(i_radii)];endendend% Outputvarargout{l} = circen;varargout{2} = cirrad;if nargout > 3,varargout{3} = dbg_LMmask;endprograms:programs:2 function DrawCircle (x, y, r, nseg, S)% Draw a circle on the current figure using ploylines%% DrawCircle (x, y, r, nseg, S)% A simple function for drawing a circle on graph.%% INPUT: (x, y, r, nseg, S)% x, y: Center of the circle% r: Radius of the circle% nseg: Number of segments for the circle% S: Colors, plot symbols and line types%% OUTPUT: None%% BUG REPORT:% Please send your bug reports, comments and suggestions to% pengtao@glue. umd. edu . Thanks.% Author: Tao Peng% Department of Mechanical Engineering% University of Maryland, College Park, Maryland 20742, USA % pengtao@glue. umd. edu% Version: alpha Revision: Jan. 10, 2006theta = 0 : (2 * pi / nseg) : (2 * pi);pline_x 二r * cos(theta) + x;pline_y 二r * sin(theta) + y;plot (pline_x, pline_y, S);3function testiml二imread (' image 1. jpg');% rawimg = imread(,TestImg_CHT_c3. bmp J);rawimg=rgb2gray(iml);tic;[accum, circen, cirrad] = CircularHough_Grd(rawimg, [20 30], 5,50);circentoe;figure(1) ; imagesc(accum); axis image;title (J Accumulation Array from Circular Hough Transform,); figure (2) ; imagesc (rawimg) ; colormap (J gray,) ; axis image; hold on;plot (circen(:, 1), circen(:, 2), ' r+');for k = 1 : size (circen, 1),DrawCircle (circen (k, 1), circen (k, 2), cirrad (k), 32, ' b-'); end hold off; title(f Raw Image with Circles Detected ...'(center positions and radii marked)']);figure (3); surf(accum, ' EdgeColor,, ' none5); axis ij; title (J 3-D View of the Accumulation Array');附带图像image 1. jpg直接运行test.m即可得到上方的结果!当然方法是活的,只要合理即可行。
数学建模作业答案

一、教材76页第1章习题1第7题(来自高中数学课本的数学探究问题,满分10分) 表1.17是某地一年中10天的白昼时间(单位:小时),请选择合适的函数模型,并进行数据拟合.一、解:根据地理常识,某地的白昼时间是以一年为周期而变化的,以日期在一年中的序号为自变量x ,以白昼时间为因变量y ,则根据表1.17的数据可知在一年(一个周期)内,随着x 的增加,y 先增后减,y 大约在6月21日(夏至)达到最大值,在12月21日(冬至)达到最小值,在3月21日(春分)或9月21日(秋分)达到中间值。
选择正弦函数sin(2/365)y A x b πϕ=++作为函数模型。
根据表1.17的数据,推测,A b ϕ和的值,作非线性拟合得26.9022sin( 1.3712)12.385365y x π=-+,预测该地12月21日的白昼时间为5.49小时。
二、教材100页第2章习题2第1题(满分10分)继续考虑第2.2节“汽车刹车距离”案例,请问“两秒准则”和“一车长度准则”一样吗?“两秒准则”是否足够安全?对于安全车距,你有没有更好的建议?二、解:“两秒准则”表明亲厚车距D 与车速v 成正比例关系2D K v =,其中22K s =。
对于小型汽车,“一车长度准则”与“两秒准则”不一致。
由221[()]d D v k v K k -=--可以计算得到当212()/54.428/v K k k km h <-=时有d D <,“两秒准则”足够安全,或者把刹车距离实测数据和“两秒准则”画在同一幅图中,根据图形指出“两秒准则”足够安全的车速范围。
用最大刹车距离除以车速,得到最大刹车距离所需要的尾随时间,并以尾随时间为依据,提出更安全的准则,如“3秒准则”,“4秒准则”或“t 秒准则”(见下图)三、教材100页第2章习题2第3题(满分10分)继续考虑第2.3节“生猪出售时机”案例,做灵敏度分析,分别考虑农场每天投入的资金对最佳出售时机和多赚的纯利润的影响.三、解:(,)2,(,)4dt c dQ x S t c S Q c dc tdcQ =∙=-=∙=-四、教材143页第3章习题3第2题(满分10分)某种山猫在较好、中等及较差的自然环境下,年平均增长率分别为 1.68%、0.55%和-4.5%. 假设开始时有100只山猫,按以下情况分别讨论山猫数量逐年变化的过程及趋势:(1) 三种自然环境下25年的变化过程,结果要列表并图示;(2) 如果每年捕获3只,山猫数量将如何变化?会灭绝吗?如果每年只捕获1只呢? (3) 在较差的自然环境下,如果要使山猫数量稳定在60只左右,每年要人工繁殖多少只?解:(1)设第k 年山猫的数量为k x ,列式得1(1)(0,1,2,k k x r x k +=+=…),用循环语句计算,并列表和作图。
数学建模习题及答案课后习题
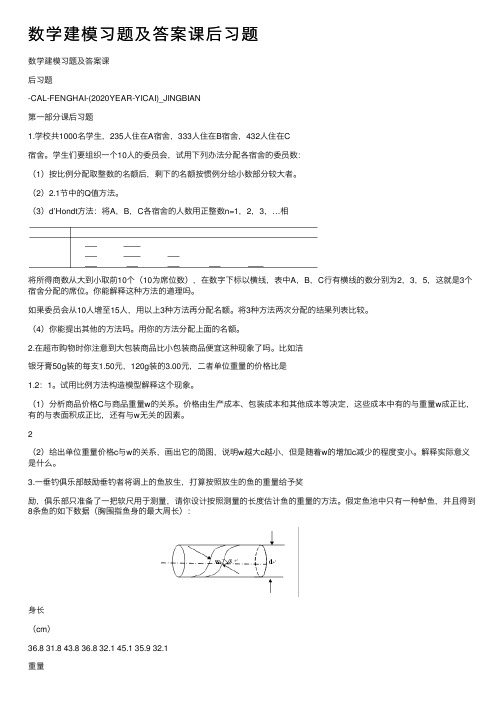
数学建模习题及答案课后习题数学建模习题及答案课后习题-CAL-FENGHAI-(2020YEAR-YICAI)_JINGBIAN第⼀部分课后习题1.学校共1000名学⽣,235⼈住在A宿舍,333⼈住在B宿舍,432⼈住在C宿舍。
学⽣们要组织⼀个10⼈的委员会,试⽤下列办法分配各宿舍的委员数:(1)按⽐例分配取整数的名额后,剩下的名额按惯例分给⼩数部分较⼤者。
(2)2.1节中的Q值⽅法。
(3)d’Hondt⽅法:将A,B,C各宿舍的⼈数⽤正整数n=1,2,3,…相将所得商数从⼤到⼩取前10个(10为席位数),在数字下标以横线,表中A,B,C⾏有横线的数分别为2,3,5,这就是3个宿舍分配的席位。
你能解释这种⽅法的道理吗。
如果委员会从10⼈增⾄15⼈,⽤以上3种⽅法再分配名额。
将3种⽅法两次分配的结果列表⽐较。
(4)你能提出其他的⽅法吗。
⽤你的⽅法分配上⾯的名额。
2.在超市购物时你注意到⼤包装商品⽐⼩包装商品便宜这种现象了吗。
⽐如洁银⽛膏50g装的每⽀1.50元,120g装的3.00元,⼆者单位重量的价格⽐是1.2:1。
试⽤⽐例⽅法构造模型解释这个现象。
(1)分析商品价格C与商品重量w的关系。
价格由⽣产成本、包装成本和其他成本等决定,这些成本中有的与重量w成正⽐,有的与表⾯积成正⽐,还有与w⽆关的因素。
2(2)给出单位重量价格c与w的关系,画出它的简图,说明w越⼤c越⼩,但是随着w的增加c减少的程度变⼩。
解释实际意义是什么。
3.⼀垂钓俱乐部⿎励垂钓者将调上的鱼放⽣,打算按照放⽣的鱼的重量给予奖励,俱乐部只准备了⼀把软尺⽤于测量,请你设计按照测量的长度估计鱼的重量的⽅法。
假定鱼池中只有⼀种鲈鱼,并且得到8条鱼的如下数据(胸围指鱼⾝的最⼤周长):⾝长(cm)36.8 31.8 43.8 36.8 32.1 45.1 35.9 32.1重量(g)765 482 1162 737 482 1389 652 454 胸围(cm)24.8 21.3 27.9 24.8 21.6 31.8 22.9 21.6先⽤机理分析建⽴模型,再⽤数据确定参数4.⽤宽w的布条缠绕直径d的圆形管道,要求布条不重叠,问布条与管道轴线的夹⾓应多⼤(如图)。
- 1、下载文档前请自行甄别文档内容的完整性,平台不提供额外的编辑、内容补充、找答案等附加服务。
- 2、"仅部分预览"的文档,不可在线预览部分如存在完整性等问题,可反馈申请退款(可完整预览的文档不适用该条件!)。
- 3、如文档侵犯您的权益,请联系客服反馈,我们会尽快为您处理(人工客服工作时间:9:00-18:30)。
数学建模协会寒假作业答案【作业一】某市有甲、乙、丙、丁四个居民区,自来水由A 、B 、C 三个水库供应。
四个区每天必须得到保证的基本生活用水分别为30,70,10,10千吨,但由于水源紧张,三个水库每天最多只能分别供应50,60,50千吨自来水。
由于地理位置的差别,自来水公司从各水库向各区送水所需付出的引水管理费不同(见表1-1,其中C 水库与丁区之间没有输水管道),其他管理费用都是450元/千吨。
根据公司规定,各区用户按照统一标准900元/千吨收费。
此外,四个区都向公司申请了额外用水量,分别为每天50,70,20,40千吨。
问题一:该公司应如何分配供水量,才能获利最多?的最大供水量都提高一倍,问那时供水方案应如何改变?公司利润可增加到多少? (灵敏度分析)【答案】分配供水量就是安排从三个水库向四个区送水的方案,目标是获利最多。
而从题目给出的数据看,A 、B 、C 三个水库的供水量160千吨,不超过四个区的基本生活用水量与额外用水量之和300千吨,因而总能全部卖出并获利,于是自来水公司每天的总收人是900×(50+603-50)=144000元,与送水方案无关。
同样,公司每天的其他管理费用为450×(50+60+50)=72000元,也与送水方案无关。
所以,要使利润最大,只需使引水管理费最小即可。
另外,送水方案自然要受三个水库的供应量和四个区的需求量的限制。
很明显,决策变量为A 、B 、C 三个水库(1,2,3i =)分别向甲、乙、丙、丁四个区(1,2,3,4j =)的供水量。
设水库i 向j 区的日供水量为ij x 。
由于C 水库与丁区之间没有输水管道,即340x =,因此只有11个决策变量。
由以上分析,问题的目标可以从获利最多转化为引水费用最少,于是有:1112131421222324313233min 160130220170140130190150190200230x x x x x x x x x x x =++++++++++约束条件有两类:一类是水库的供应量限制,另一类是各区的需求量限制。
1112131421222324313233506050x x x x x x x x x x x +++=+++=++=112131122232132333142430807014010301050x x x x x x x x x x x ≤++≤≤++≤≤++≤≤+≤LINGO 线性规划源程序如下所示:Model:min=160*x11+130*x12+220*x13+170*x14+140*x21+130*x22+190*x23+150* x24+190*x31+200*x32+230*x33;x11+x12+x13+x14=50;x21+x22+x23+x24=60;x31+x32+x33=50x11+x2l+x31>=30;x12+x22+x32>=70;x13+x14+x24>=10;x14+x24>=10;x11+x21+x3l<=80;x12+x22+x32<=140x13+x23+x33<=30:x14+x24<=50:其中第二行为目标函数,首先用max=或者min=声明求解的目标函数最大化或者最小化,然后键入目标函数。
与LINDO语法不同,在LINGO的语法操作中有乘号,一定要乘号,末尾结束出现分号。
而且数值不能出现在左边,键入的表达式应该为最简表达式。
按住Ctrl+S运行,得到运行结果如下:Global optimal solution found。
Objective value:24400.00Total solver iterations:8LINGO得到的结果与LINDO得到的结果类似,但是LINGO不支持灵敏度分析。
上述结果显示:通过2次迭代可以得到全局最优值24400。
工厂A向乙地区供应50千吨自来水;工厂B向乙提供50千吨自来水,向丁提供10千吨自来水;工厂C向甲地区提供40千吨自来水,向丙地区提供10千吨自来水。
结果后一部分为自来水供应的影子价格分析。
需要注意的是:LINDO和LINGO在求解规划问题时,可能存在由多个最优决策都能得到最优值的情况,但是软件只会显示其中一种。
LINDO公司已经将LINDO软件从其产品目录中删除,这意味着以后不会再有LINDO软件的新版本了,而LINGO还在不断地更新。
从上面的编程过程中,大家可以发现一个问题:当决策变量或者约束很多时,一条一条输入约束将是一件非常麻烦的事情。
LINGO语言又称为建模化语言,其优势在于它能够支持数学语言的输入,尤其是能够提供集合支持,给编程带来了极大的便利。
以本题为例,使用建模化语言编程代码如下所示。
LINGO建模化语言解决线性规划问题源代码如下model:sets:chandi/1..3/:a;yonghu/1..4/:b,d;routes(ehandi,yonghu):C,x;endsetsdata:a=50,60,50;b=30,70,10,10;d=80,140,30,50;c=160,130,220,170140,130,190,150,190,200,230,99999999;enddatamin=@sum(routes:c*x);@for(chandi(i):@sum(yonghu(j):x(i,j))=a(i));@for(yonghu(j):@sum(chandi(i):x(i,j))<=d(j));@for(yonghu(j):@sum(chandi(i):x(i,j))>=b(j));End与以前的LINDO、LINGO语法不同,程序首先定义了集合,以sets开始,定义了三种属性的集合:n为1×3的向量,b和d为l×4的向量,c和z为3×4的矩阵,以end-sets结束。
然后对定义的集合进行数据初始化,以data开始:分别对a,b,c,d进行初使化。
其中由于自来水厂C不能给用户丁供水,因此可以定义一个非常大的数,代表非常大的代价,以enddata结束。
接下去的就是目标函数和约束条件,在目标函数中使用了@sum求和函数,在约束中使用了@for循环函数。
可见,如果决策变量或者约束很多,也可以不用一条一条输入了,只需要一条循环便可以解决。
得到结果。
由结果显示:通过8次迭代可以得到全局最优值24400。
z就是所需的决策变量矩阵,工厂A向乙地区供应50千吨自来水;工厂B向乙提供50千吨自来水,向丁提供10千吨自来水;工厂C向甲地区提供40千吨自来水,向丙地区提供10千吨自来水。
结构后一部分为自来水供应的影子价格分析,与以前得到的结果相同。
问题二是关于灵敏度分析,具体可参见优化问题课件,在此就不再赘述。
——摘自邬学军,周凯,宋军全编著,数学建模竞赛辅导教程,浙江大学出版社,2009。
08,第84页某公司出口换汇成本分析对经营同一类产品出口业务的公司进行抽样调查, 被调查的13家公司,其出口换汇成本与商品流转费用率资料如下表。
设出口换汇成本为Y , 商品流转费用x(2)求2σ的无偏估计。
(3) 并估计某家公司商品流转费用率是6.5%的出口换汇成本。
【答案】令商品流转费用率为试验指标(因变量)y ,令出口换汇成本为影响因变量的因素即自变量x ,然后用回归拟合建立起二者之间的关系,且令ˆˆy abx =+,用回归模型解该方程的具体程序如下:x=[1.40,1.20,1.00,1.90,1.30,2.40,1.40,1.60,2.00,1.00,1.60,1.80,1.40]; y=[4.20,5.30,7.10,3.70,6.20,3.50,4.80,5.50,4.10,5.00,4.00,3.40,6.90]; X=[ones(size(x))',x']; %执行回归命令[b,bint,r,rint,stats]=regress(y',X,0.05)rcoplot(r,rint) %画出残差及它们的置信区间的图形 问题一参数估计:ˆˆ8.2333, 2.1667ab ==- 变量y 关于x 的线性回归方程:ˆ8.2333 2.1677yx =- 问题二2σ的无偏估计体现的是回归方程的优化程度,因此:检验:2=0.495810.8168R F =,,2R 和F 都相对较小,说明回归直线对样本数据点的拟合程度低;问题三预测:利用回归线性方程可解得商品流转费用率为 6.5%是对应的出口换汇成本的近似值,具体程序如下:y0=6.5;x0=(8.2333-y0)/2.1667;运行程序结果可得:00.800y =(人民币/美元)1、根据经验,当一种新商品投入市场后,随着人们对它的拥有量的增加,其销售量)(t s 下降的速度与)(t s 成正比。
广告宣传可给销量添加一个增长速度,它与广告费)(t a 成正比,但广告只能影响这种商品在市场上尚未饱和的部分(设饱和量为M )。
建立一个销售)(t s 的模型。
若广告宣传只进行有限时间τ,且广告费为常数a ,问)(t s 如何变化?【答案】根据经验,当一种新商品投入市场后,随着人们对它的拥有量的增加,其销售量)(t s 下降的速度与)(t s 成正比。
广告宣传可给销量添加一个增长速度,它与广告费)(t a 成正比,但广告只能影响这种商品在市场上尚未饱和的部分(设饱和量为M )。
建立一个销售)(t s 的模型。
若广告宣传只进行有限时间τ,且广告费为常数a ,问)(t s 如何变化?解:假设在没有广告宣传的情况下,销售量()s t 的模型为:1()()ds t k s t dt=- 在加入广告宣传后,销售量()s t 随时间变化的情况如下:120()()()(())t ds t k s t k a t M s x dx dt=-+-⎰ 其中0()t s x dx ⎰为0t 时间内的总销售量。
如果广告宣传只进行有限的时间τ,则上述模型变为12000100()()(())()(),t k s t k a t M s x dx t t t ds t dt k s t t t t t ττ⎧-+-≤≤+⎪=⎨-≥≥+⎪⎩⎰2、在鱼塘投放尾鱼苗,随着时间的增长,尾数将减少而每尾的质量将增加。
设尾数的(相对)减少率为常数,由于喂养引起的每尾鱼重量的增加率与鱼的表面积成正比,由于消耗引起的每尾鱼重量的减少率与重量成正比。
分别建立尾数和每尾鱼重的微分方程,并求解。
【答案】在鱼塘投放尾鱼苗,随着时间的增长,尾数将减少而每尾的质量将增加。
设尾数的(相对)减少率为常数,由于喂养引起的每尾鱼重量的增加率与鱼的表面积成正比,由于消耗引起的每尾鱼重量的减少率与重量成正比。