人工神经网络外文翻译
外文翻译---人工神经网络
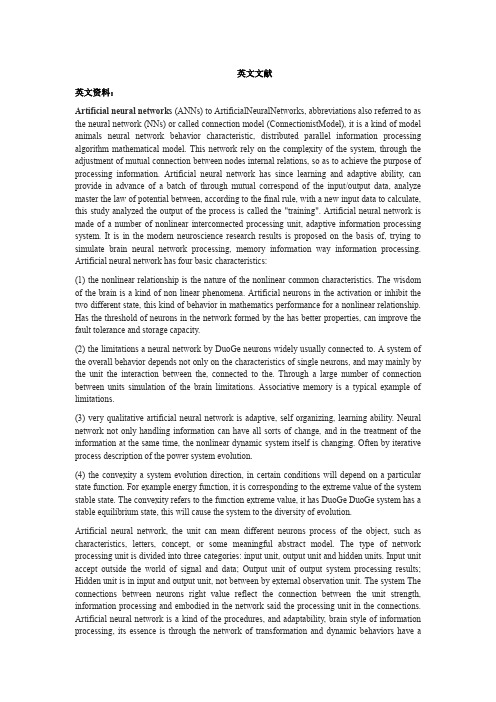
英文文献英文资料:Artificial neural networks (ANNs) to ArtificialNeuralNetworks, abbreviations also referred to as the neural network (NNs) or called connection model (ConnectionistModel), it is a kind of model animals neural network behavior characteristic, distributed parallel information processing algorithm mathematical model. This network rely on the complexity of the system, through the adjustment of mutual connection between nodes internal relations, so as to achieve the purpose of processing information. Artificial neural network has since learning and adaptive ability, can provide in advance of a batch of through mutual correspond of the input/output data, analyze master the law of potential between, according to the final rule, with a new input data to calculate, this study analyzed the output of the process is called the "training". Artificial neural network is made of a number of nonlinear interconnected processing unit, adaptive information processing system. It is in the modern neuroscience research results is proposed on the basis of, trying to simulate brain neural network processing, memory information way information processing. Artificial neural network has four basic characteristics:(1) the nonlinear relationship is the nature of the nonlinear common characteristics. The wisdom of the brain is a kind of non-linear phenomena. Artificial neurons in the activation or inhibit the two different state, this kind of behavior in mathematics performance for a nonlinear relationship. Has the threshold of neurons in the network formed by the has better properties, can improve the fault tolerance and storage capacity.(2) the limitations a neural network by DuoGe neurons widely usually connected to. A system of the overall behavior depends not only on the characteristics of single neurons, and may mainly by the unit the interaction between the, connected to the. Through a large number of connection between units simulation of the brain limitations. Associative memory is a typical example of limitations.(3) very qualitative artificial neural network is adaptive, self-organizing, learning ability. Neural network not only handling information can have all sorts of change, and in the treatment of the information at the same time, the nonlinear dynamic system itself is changing. Often by iterative process description of the power system evolution.(4) the convexity a system evolution direction, in certain conditions will depend on a particular state function. For example energy function, it is corresponding to the extreme value of the system stable state. The convexity refers to the function extreme value, it has DuoGe DuoGe system has a stable equilibrium state, this will cause the system to the diversity of evolution.Artificial neural network, the unit can mean different neurons process of the object, such as characteristics, letters, concept, or some meaningful abstract model. The type of network processing unit is divided into three categories: input unit, output unit and hidden units. Input unit accept outside the world of signal and data; Output unit of output system processing results; Hidden unit is in input and output unit, not between by external observation unit. The system The connections between neurons right value reflect the connection between the unit strength, information processing and embodied in the network said the processing unit in the connections. Artificial neural network is a kind of the procedures, and adaptability, brain style of information processing, its essence is through the network of transformation and dynamic behaviors have akind of parallel distributed information processing function, and in different levels and imitate people cranial nerve system level of information processing function. It is involved in neuroscience, thinking science, artificial intelligence, computer science, etc DuoGe field cross discipline.Artificial neural network is used the parallel distributed system, with the traditional artificial intelligence and information processing technology completely different mechanism, overcome traditional based on logic of the symbols of the artificial intelligence in the processing of intuition and unstructured information of defects, with the adaptive, self-organization and real-time characteristic of the study.Development historyIn 1943, psychologists W.S.M cCulloch and mathematical logic W.P home its established the neural network and the math model, called MP model. They put forward by MP model of the neuron network structure and formal mathematical description method, and prove the individual neurons can perform the logic function, so as to create artificial neural network research era. In 1949, the psychologist put forward the idea of synaptic contact strength variable. In the s, the artificial neural network to further development, a more perfect neural network model was put forward, including perceptron and adaptive linear elements etc. M.M insky, analyzed carefully to Perceptron as a representative of the neural network system function and limitations in 1969 after the publication of the book "Perceptron, and points out that the sensor can't solve problems high order predicate. Their arguments greatly influenced the research into the neural network, and at that time serial computer and the achievement of the artificial intelligence, covering up development new computer and new ways of artificial intelligence and the necessity and urgency, make artificial neural network of research at a low. During this time, some of the artificial neural network of the researchers remains committed to this study, presented to meet resonance theory (ART nets), self-organizing mapping, cognitive machine network, but the neural network theory study mathematics. The research for neural network of research and development has laid a foundation. In 1982, the California institute of J.J.H physicists opfield Hopfield neural grid model proposed, and introduces "calculation energy" concept, gives the network stability judgment. In 1984, he again put forward the continuous time Hopfield neural network model for the neural computers, the study of the pioneering work, creating a neural network for associative memory and optimization calculation, the new way of a powerful impetus to the research into the neural network, in 1985, and scholars have proposed a wave ears, the study boltzmann model using statistical thermodynamics simulated annealing technology, guaranteed that the whole system tends to the stability of the points. In 1986 the cognitive microstructure study, puts forward the parallel distributed processing theory. Artificial neural network of research by each developed country, the congress of the United States to the attention of the resolution will be on jan. 5, 1990 started ten years as the decade of the brain, the international research organization called on its members will the decade of the brain into global behavior. In Japan's "real world computing (springboks claiming)" project, artificial intelligence research into an important component.Network modelArtificial neural network model of the main consideration network connection topological structure, the characteristics, the learning rule neurons. At present, nearly 40 kinds of neural network model, with back propagation network, sensor, self-organizing mapping, the Hopfieldnetwork.the computer, wave boltzmann machine, adapt to the ear resonance theory. According to the topology of the connection, the neural network model can be divided into:(1) prior to the network before each neuron accept input and output level to the next level, the network without feedback, can use a loop to no graph. This network realization from the input space to the output signal of the space transformation, it information processing power comes from simple nonlinear function of DuoCi compound. The network structure is simple, easy to realize. Against the network is a kind of typical prior to the network.(2) the feedback network between neurons in the network has feedback, can use a no to complete the graph. This neural network information processing is state of transformations, can use the dynamics system theory processing. The stability of the system with associative memory function has close relationship. The Hopfield network.the computer, wave ear boltzmann machine all belong to this type.Learning typeNeural network learning is an important content, it is through the adaptability of the realization of learning. According to the change of environment, adjust to weights, improve the behavior of the system. The proposed by the Hebb Hebb learning rules for neural network learning algorithm to lay the foundation. Hebb rules say that learning process finally happened between neurons in the synapse, the contact strength synapses parts with before and after the activity and synaptic neuron changes. Based on this, people put forward various learning rules and algorithm, in order to adapt to the needs of different network model. Effective learning algorithm, and makes the godThe network can through the weights between adjustment, the structure of the objective world, said the formation of inner characteristics of information processing method, information storage and processing reflected in the network connection. According to the learning environment is different, the study method of the neural network can be divided into learning supervision and unsupervised learning. In the supervision and study, will the training sample data added to the network input, and the corresponding expected output and network output, in comparison to get error signal control value connection strength adjustment, the DuoCi after training to a certain convergence weights. While the sample conditions change, the study can modify weights to adapt to the new environment. Use of neural network learning supervision model is the network, the sensor etc. The learning supervision, in a given sample, in the environment of the network directly, learning and working stages become one. At this time, the change of the rules of learning to obey the weights between evolution equation of. Unsupervised learning the most simple example is Hebb learning rules. Competition rules is a learning more complex than learning supervision example, it is according to established clustering on weights adjustment. Self-organizing mapping, adapt to the resonance theory is the network and competitive learning about the typical model.Analysis methodStudy of the neural network nonlinear dynamic properties, mainly USES the dynamics system theory and nonlinear programming theory and statistical theory to analysis of the evolution process of the neural network and the nature of the attractor, explore the synergy of neural network behavior and collective computing functions, understand neural information processing mechanism. In order to discuss the neural network and fuzzy comprehensive deal of information may, the concept of chaos theory and method will play a role. The chaos is a rather difficult toprecise definition of the math concepts. In general, "chaos" it is to point to by the dynamic system of equations describe deterministic performance of the uncertain behavior, or call it sure the randomness. "Authenticity" because it by the intrinsic reason and not outside noise or interference produced, and "random" refers to the irregular, unpredictable behavior, can only use statistics method description. Chaotic dynamics of the main features of the system is the state of the sensitive dependence on the initial conditions, the chaos reflected its inherent randomness. Chaos theory is to point to describe the nonlinear dynamic behavior with chaos theory, the system of basic concept, methods, it dynamics system complex behavior understanding for his own with the outside world and for material, energy and information exchange process of the internal structure of behavior, not foreign and accidental behavior, chaos is a stationary. Chaotic dynamics system of stationary including: still, stable quantity, the periodicity, with sex and chaos of accurate solution... Chaos rail line is overall stability and local unstable combination of results, call it strange attractor.A strange attractor has the following features: (1) some strange attractor is a attractor, but it is not a fixed point, also not periodic solution; (2) strange attractor is indivisible, and that is not divided into two and two or more to attract children. (3) it to the initial value is very sensitive, different initial value can lead to very different behavior.superiorityThe artificial neural network of characteristics and advantages, mainly in three aspects: first, self-learning. For example, only to realize image recognition that the many different image model and the corresponding should be the result of identification input artificial neural network, the network will through the self-learning function, slowly to learn to distinguish similar images. The self-learning function for the forecast has special meaning. The prospect of artificial neural network computer will provide mankind economic forecasts, market forecast, benefit forecast, the application outlook is very great. The second, with lenovo storage function. With the artificial neural network of feedback network can implement this association. Third, with high-speed looking for the optimal solution ability. Looking for a complex problem of the optimal solution, often require a lot of calculation, the use of a problem in some of the design of feedback type and artificial neural network, use the computer high-speed operation ability, may soon find the optimal solution.Research directionThe research into the neural network can be divided into the theory research and application of the two aspects of research. Theory study can be divided into the following two categories:1, neural physiological and cognitive science research on human thinking and intelligent mechanism.2, by using the neural basis theory of research results, with mathematical method to explore more functional perfect, performance more superior neural network model, the thorough research network algorithm and performance, such as: stability and convergence, fault tolerance, robustness, etc.; The development of new network mathematical theory, such as: neural network dynamics, nonlinear neural field, etc.Application study can be divided into the following two categories:1, neural network software simulation and hardware realization of research.2, the neural network in various applications in the field of research. These areas include: pattern recognition, signal processing, knowledge engineering, expert system, optimize the combination, robot control, etc. Along with the neural network theory itself and related theory, related to the development of technology, the application of neural network will further.Development trend and research hot spotArtificial neural network characteristic of nonlinear adaptive information processing power, overcome traditional artificial intelligence method for intuitive, such as mode, speech recognition, unstructured information processing of the defects in the nerve of expert system, pattern recognition and intelligent control, combinatorial optimization, and forecast areas to be successful application. Artificial neural network and other traditional method unifies, will promote the artificial intelligence and information processing technology development. In recent years, the artificial neural network is on the path of human cognitive simulation further development, and fuzzy system, genetic algorithm, evolution mechanism combined to form a computational intelligence, artificial intelligence is an important direction in practical application, will be developed. Information geometry will used in artificial neural network of research, to the study of the theory of the artificial neural network opens a new way. The development of the study neural computers soon, existing product to enter the market. With electronics neural computers for the development of artificial neural network to provide good conditions.Neural network in many fields has got a very good application, but the need to research is a lot. Among them, are distributed storage, parallel processing, since learning, the organization and nonlinear mapping the advantages of neural network and other technology and the integration of it follows that the hybrid method and hybrid systems, has become a hotspot. Since the other way have their respective advantages, so will the neural network with other method, and the combination of strong points, and then can get better application effect. At present this in a neural network and fuzzy logic, expert system, genetic algorithm, wavelet analysis, chaos, the rough set theory, fractal theory, theory of evidence and grey system and fusion.汉语翻译人工神经网络(ArtificialNeuralNetworks,简写为ANNs)也简称为神经网络(NNs)或称作连接模型(ConnectionistModel),它是一种模范动物神经网络行为特征,进行分布式并行信息处理的算法数学模型。
Artificial Neural Networks(人工神经网络)
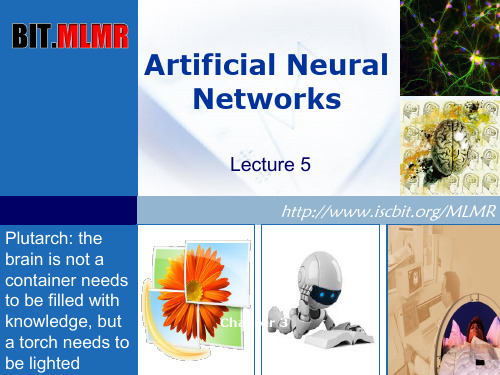
Linear
f ( )
Saturating Linear
f ( )
+1
0
Logistic Sigmoid
Hyperbolic tangent Sigmoid
11
Gaussian
2016/1/12
AI:ANN
Two main problems in ANN
Architectures How to interconnect individual units? Learning Approaches How to automatically determine the connection weights or even structure of ANN?
- B-P Learning Objective:
Solution:
1 K ω arg min eDk ,Yk ω K k 1
*
ω ω ω ω
2016/1/12
17
AI:ANN
Learning Strategies: Competitive Learning Winner-take-all (Unsupervised) How to compete? - Hard competition Only one neuron is activated - Soft competition Neurons neighboring the true winner are activated.
Weight values for a hidden unit:
From T. M. Mitchell, Machine Learning, 2006
人工神经网络
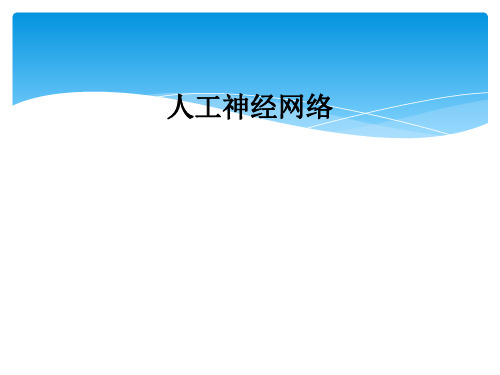
3、阈值函数(Threshold Function)阶跃函数
o
β
0
θ
net
-γ
4、S形函数
压缩函数(Squashing Function)和逻辑斯特 函数(Logistic Function)。
f(net)=a+b/(1+exp(-d*net)) a,b,d为常数。它的饱和值为a和a+b。 最简单形式为: f(net)= 1/(1+exp(-d*net))
用的; – 5)一个神经元接受的信号的累积效果决定该神经
元的状态; – 6) 每个神经元可以有一个“阈值”。
人工神经元
人工神经元
• 人工神经元模型应该具有生物神经元的六 个基本特性。
x1 w1
x2 w2 … xn wn
∑ net=XW
人工神经元的基本构成
x1 w1
x2 w2 … xn wn
∑ net=XW
• 2、 循环联接 –反馈信号。
联接模式
• 3、层(级)间联接 –层间(Inter-field)联接指不同层中的神 经元之间的联接。这种联接用来实现层 间的信号传递
ANN的网络结构
网络的分层结构
• 简单单级网
x1 x2 … xn
w11 w1m
w2m … wn1
wnm 输入层
o1
o2
… om
输出层
x1 x2 … xn
w11 w1m w2m …wn1
输入层
o1
o2
… 输出层
V
om
单级横向反馈网
• V=(vij) • NET=XW+OV • O=F(NET) • 时间参数——神经元的状态在主时钟的控制下同
王勇 -人工神经网络

人工神经网络人工神经网络(Artificial Neural Networks,简写为ANNs)也简称为神经网络(NNs)或称作连接模型(Connection Model),它是一种模仿动物神经网络行为特征,进行分布式并行信息处理的算法数学模型。
这种网络依靠系统的复杂程度,通过调整内部大量节点之间相互连接的关系,从而达到处理信息的目的。
本文将通过对神经网络分析阐述神经网络的概念以及使用方法,以及用实例来更加图书神经网络的使用价值.关键词:人工神经网络,模型一概述神经元网络是机器学习学科中的一个重要部分,用来classification或者regression。
思维学普遍认为,人类大脑的思维分为抽象(逻辑)思维、形象(直观)思维和灵感(顿悟)思维三种基本方式。
逻辑性的思维是指根据逻辑规则进行推理的过程;它先将信息化成概念,并用符号表示,然后,根据符号运算按串行模式进行逻辑推理;这一过程可以写成串行的指令,让计算机执行。
然而,直观性的思维是将分布式存储的信息综合起来,结果是忽然间产生想法或解决问题的办法。
这种思维方式的根本之点在于以下两点:1.信息是通过神经元上的兴奋模式分布存储在网络上;2.信息处理是通过神经元之间同时相互作用的动态过程来完成的。
人工神经网络就是模拟人思维的第二种方式。
这是一个非线性动力学系统,其特色在于信息的分布式存储和并行协同处理。
虽然单个神经元的结构极其简单,功能有限,但大量神经元构成的网络系统所能实现的行为却是极其丰富多彩的。
神经网络(Neural Networks,NN)是由大量的、简单的处理单元(称为神经元)广泛地互相连接而形成的复杂网络系统,它反映了人脑功能的许多基本特征,是一个高度复杂的非线性动力学习系统。
神经网络具有大规模并行、分布式存储和处理、自组织、自适应和自学能力,特别适合处理需要同时考虑许多因素和条件的、不精确和模糊的信息处理问题。
神经网络的发展与神经科学、数理科学、认知科学、计算机科学、人工智能、信息科学、控制论、机器人学、微电子学、心理学、光计算、分子生物学等有关,是一门新兴的边缘交叉学科。
人工神经网络:基本特征外文文献翻译

外文文献翻译(含:英文原文及中文译文)英文原文Artificial Neural Networks - Basic FeaturesComposed of a large number of processing units connected by a nonlinear, adaptive information processing system. It is the basis for modern neuroscience research findings presented, trying to simulate a large neural network processing, memory, information processing way of information. Artificial neural network has four basic characteristics:(1) non-linear non-linear relationship is the general characteristics of the natural world. The wisdom of the brain is a nonlinear phenomenon. Artificial neural activation or inhibition in two different states, this behavior mathematically expressed as a linear relationship. Threshold neurons have a network with better performance, can improve fault tolerance and storage capacity.(2) non-limitation of a neural network is usually more extensive neuronal connections made. The overall behavior of a system depends not only on the characteristics of single neurons, and may primarily by interaction between units, connected by the decision. By a large number of connections between the cells of non-simulated brain limitations. Associative memory limitations of a typical example of non-(3) characterization of artificial neural network is adaptive,self-organizing, self-learning ability. Neural networks can not only deal with the changes of information, but also process information the same time, nonlinear dynamic system itself is also changing. Iterative process is frequently used in describing the evolution of dynamical systems.(4) Non-convexity of the direction of the evolution of a system, under certain conditions, will depend on a particular state function. Such as energy function, and its extreme value corresponding to the state of the system more stable. Non-convexity of this function is more than one extremum, this system has multiple stable equilibrium, which will cause the system to the evolution of diversity.Artificial neural network, neural processing unit can be expressed in different objects, such as features, letters, concepts, or some interesting abstract patterns. The type of network processing unit is divided into three categories: input units, output units and hidden units. Input unit receiving the signal and data outside world; output unit for processing the results to achieve the output; hidden unit is in between the input and output units can not be observed from outside the system unit. Neurons and the connection weights reflect the strength of the connections between elements of information representation and processing reflected in the network processing unit connected relationships. Artificial neural network is a non-procedural, adaptability, the brain's information processing style, its essence is transformation through the network anddynamic behavior is a parallel distributed information processing, and to varying degrees and levels mimic brain information processing system. It is involved in neural science, thinking, science and artificial intelligence, computer science and other interdisciplinary fields. Artificial neural networks are parallel distributed systems, using traditional artificial intelligence and information processing technology is completely different mechanism to overcome the traditional symbol of artificial intelligence-based logic in dealing with intuition, unstructured information deficiencies, adaptive, Self-organization and the characteristics of real-time learning.Artificial Neural Network – HistoryIn 1943, psychologist WSMcCulloch mathematical logician W. Pitts neural network and the establishment of a mathematical model, called the MP model. They put forward by MP model neurons and network structure of formal mathematical description of methods, that a single neuron can perform logic functions, thus creating the era of artificial neural network. In 1949, psychologists proposed the idea of synaptic strength variable. 60 years, artificial neural network to the further development of improved neural network models have been proposed, including the sensors and the adaptive linear element, etc.. M. Minsky and so careful analysis of the sensor represented by the neural network system capabilities and limitations, the in 1969 published a "Perceptron" book, pointed out thatthe sensor can not solve the issue of higher order predicate. Their argument has greatly influenced research in neural networks, combined with serial computers and artificial intelligence at the achievements made to cover up the development of new computer and artificial intelligence, new ways of necessity and urgency to the research of artificial neural networks at a low ebb . In the meantime, some artificial neural network remains committed to the study, researchers proposed to adapt resonance theory (ART Wang), Zi Zuzhiyingshe, Ren Zhi machine network, while for the neural network Shuxue research. More research and development of neural network research foundation. In 1982, California Institute of Technology physicist JJHopfield proposed Hopfield neural grid model, the concept of "computational energy" concept gives the network stability of the judge. In 1984, he made continuous time Hopfield neural network model for the neural computer research done pioneering work to create a neural network for associative memory and optimization of computing new ways to effectively promote the study of neural networks, In 1985, there are scholars of the Boltzmann model, the use of statistical thermodynamics in the study simulated annealing technology to ensure the overall stability of the whole system tends to point. 1986 to study the microstructure of cognition, proposed the theory of parallel distributed processing. Artificial neural networks in various countries of the importance attached by the U.S. Congress passed a resolution to January5, 1990 began with a decade as the "brain decade", the international research organization called on its members to "brain of the 10 in "to the global behavior. In Japan's "real-world computing (RWC)" project, the artificial intelligence research has become an important part.The main consideration of artificial neural network model topology of the network connection, the characteristics of neurons, learning rules. Currently, nearly 40 kinds of neural network model, including back propagation network, perceptron, self organizing maps, Hopfield networks, Boltzmann machines, meet the resonance theory. According to the connection topology, neural network model can be divided into: (1) before the network to the network each neuron for the former level of input and output to the next level, network, no feedback, you can use a directed loop-free graph. This network signal from the input space and output space transform its information processing capabilities from a simple nonlinear function of several complex. Network structure is simple, easy to implement. Back-propagation network is a typical feedforward network.(2) feedback network network neurons have feedback, you can use an undirected complete graph. This neural network information processing is the state of transformation, you can deal with dynamic systems theory. Stability of the system is closely related with the associative memory function. Hopfield networks, Boltzmann machinesbelong to this category.Neural network learning is an important part of its adaptability is achieved through learning. According to the environmental changes in the value of the right to make adjustments to improve the system's behavior. Hebb proposed by the Hebb learning rule neural network learning algorithm for the foundation. Hebb learning rule that eventually occurred in the synapses between neurons, synaptic contacts with the synaptic strength of neuronal activity before and after the change. On this basis, it proposed a variety of learning rules and algorithms to meet the needs of different network models. Effective learning algorithm, making neural network connection weights through the adjustment, construction of the objective world of the intrinsic representation, forming a unique information processing method, information Cunchu and processing reflected in the network connection.According to different learning environment, neural network learning methods can be divided into supervised learning and unsupervised learning. In monitoring the study, the training sample data added to the network input side, while the corresponding desired output and network output compared Dedao error signal, thereby Kongzhiquanzhi connection strength adjustments, Jing Hou several training convergence to a determine the weights. When the sample situation changes, the weights can be modified by learning to adapt to thenew environment. Use supervised learning back propagation neural network model has the network, and HC. Non-supervised learning, the prior is not a given standard sample placed directly into the network environment, learning stage and become one session. At this point, learn to obey the law of change in the evolution equation of connection weights. Non-supervised learning the most simple example is the Hebb learning rule. Competitive learning rule is a more complex example of non-supervised learning, which is based on the established clustering weight adjustment. Self-organizing map, resonance theory network to adapt to competition learn about all the typical model. Study of nonlinear dynamics of neural networks, the main use of dynamic systems theory, nonlinear programming theory and statistical theory to analyze the evolutionary neural network attractor nature of the process and to explore the neural network of collaborative behavior and collective computing to understand neural information processing mechanism. To investigate the neural networks and fuzzy in the integrity of information in terms of dealing with the possibility of chaos theory concepts and methods could be useful. Chaos is a very difficult to precisely defined mathematical concept. Generally speaking, the "chaos" refers to the dynamic system described by deterministic equations demonstrated the non-deterministic behavior, or call to determine the randomness. "Certainty" because it causes from within and not outside noise or interference generated, and"random" refers to the irregular, unpredictable behavior, can only be described using statistical methods. The main features of chaotic dynamic system is its state of sensitive dependence on initial conditions, reflect the inherent randomness of chaos. Chaos theory is the description of chaotic behavior with the basic theory of nonlinear dynamical systems, concepts, methods, and it is to understand the dynamic complexity of the system for their own actions with the outside world and its material, energy and information exchange process in the inner structured, and not external and accidental behavior, chaotic state is a steady state. Steady state chaotic dynamical systems, including: static, steady volume, periodicity, quasi-period and chaotic solution. Lorenz is the overall stability and local instability in the results of the combination, called the strange attractor. A strange attractor has the following characteristics: (1) strange attractor is an attractor, but it is neither fixed points nor periodic solutions; (2) strange attractor is indivisible, that can not be divided into two and two or more attractors; (3) is very sensitive to its initial value, different initial values will lead to very different behavior.Artificial neural network - advantagesArtificial neural network features and advantages mainly in three aspects:First, self-learning function. For example, in pattern recognition, only the first of many different images in the model and thecorresponding results should be identified input of artificial neural network, the network will be through self-learning function, and slowly learn to identify similar images. Self-learning function for the forecasts of particular importance. Expected future human artificial neural network computer will provide economic forecast, market forecasting, prediction efficiency, the application of the future is very bright.Second, with the association storage. Artificial neural network feedback network can achieve this association.Third, find the optimal solution with high capacity. Find the optimal solution of a complex problem, often requires a great amount of computation, the use of a problem for the design of a feedback type artificial neural network, play a computer's high-speed computing power, may soon find the optimal solution.Artificial Neural NetworksNeural network research can be divided into theoretical and applied research on two areas.Theory can be divided into the following categories:1, using neuropsychological and cognitive science of human thinking and intelligence mechanism.2, the neural basis of theoretical research, using mathematical methods to explore a more complete functional performance, superior of the neural network model, in-depth study and performance of networkalgorithms, such as: stability, convergence, fault tolerance, robustness, etc.; Development mathematical theory of the new network, such as: neural network dynamics, nonlinear neural farms.Applied research can be divided into the following categories:1, neural network software simulation and hardware implementation of the study. 2, neural network applications in all areas of research. These areas include:Pattern recognition, signal processing, knowledge engineering, expert system, optimize, robot control. With the neural network theory and the theory itself, the continuous development of relevant technology, application of neural networks will be more in-depth.Artificial Neural Networks - ApplicationNeural networks more and more attention recently because it was solved by the complexity of the problem provides a relatively simple and effective way. Neural networks can easily solve problems with hundreds of parameters (of course the actual existence of the neural network organisms than the procedures described here simulated neural networks are much more complex). Neural network used in two problems: classification and regression. In the structure, a neural network can be divided into input layer, output layer and hidden layer (see Figure 1). Each input layernode corresponds to a forecast of a variable. Output layer of nodescorresponding to the target variable can have more than one. In the input layer and output layer is hidden layer (the neural network is not visible for the user), number of layers and each layer of hidden layer nodes determines the number of neural network complexity. Figure 1 A neural networkIn addition to the input layer nodes, neural network, each node in front of it with a lot of nodes (called the node of input nodes) connected together, each connection corresponds to a weight Wxy, the value of this node is the node through which all input The value of the weights with the corresponding product and as a function of the input and get our activities in this function is called function or squeezing function. Figure 2, the output node 4 to node 6, the value can be calculated as follows: W14 * Node 1, the value of the + W24 * value of node 2Neural network each node can be expressed as predictor variables (nodes 1,2) value or the value of the portfolio (nodes 3-6). Attention to the value of the node 6 is no longer a linear combination of node 1,2, because the data passed in the hidden layer using the activity function. In fact, if there are no active function, then neural network is equivalent to a linear regression function, if the activity of a particular function is a nonlinear function, neural network that it is equivalent to logistic regression.Adjust the weights of connections between nodes is established (alsocalled training) neural network to do work. The first and most basic method of weight adjustment feedback law is wrong, and now there are more changes in the new gradient method, Newton method, Levenberg-Marquardt method, and genetic algorithms. Regardless of the kind of training methods, we need to have some parameters to control the training process, such as training to prevent overtraining and control the pace.Decided to neural network topology (or architecture) is contained in the hidden layer and the number of nodes and connections between nodes. To design a neural network from scratch, have to decide the number of hidden layers and nodes, activities in the form of the function, as well as the right to redo that limit, of course, if using sophisticated software tools, he will help you decide these things. In many types of neural networks, the most commonly used to spread the former type neural network, which is ahead of us the kind depicted in the icon. People talk in detail below, in order to facilitate the discussion assumes that only contain a layer of hidden nodes. That the error feedback type training method is the change in slope of the simplified method, the process is as follows: prior to the dissemination of: data from input to output of the process is a front to back of the transmission process, after the value of a node connected to the node through which pass in front of come, then the values of the weights in accordance with the size of all the weighted input eventfunction and then get the new value, and further spread to the next node.Feedback: When the output value of the node we expect the value of the difference, that is, an error occurs, the neural network would "learn" (learning from mistakes). We can weight between nodes connected as a node after the previous node on the "trust" level (of his own down the output of a node in front of him which is more vulnerable to the impact of input nodes). Learning are punitive approach, the process is as follows: If a node output error, then he see his error by which (some) input node of the impact caused, is not his most trusted node (the highest weight of node) in framing him (make him wrong), if the trust will have to lower his value (reducing weight), punish them, while those who make the right recommendations increase the trust value of nodes. Nodes who received punishment, he also needs the same way to further punish it in front of the node. Move forward one step on the way to spread until the punishment until the input nodes.Focus on the training must be repeated for each record this step, using the former to the spread of the output value, if an error occurs, then use the feedback method to learn. When the training set a record for each run over again, people said the completion of a training cycle. To complete the training of neural networks may take many months training period, often several hundred. After training by the neural network training set is found by the model, describes the training focused onresponse variables affected by the changes of predictor variables.As the hidden layer neural networks are too many variable parameters, if the training time long enough, then neural network training set is possible to all the details of information "in mind" down, Er not overdo the detail to establish a regular model only has We call this situation as overtraining. Obviously this "model" for training has a high accuracy of assembly, and once left the training set applied to other data, it is possible accuracy declined sharply. To prevent this training over the situation, one must know when to stop training. In some software implementations of the same training courses will use a test set to computational neuroscience network in this test set the correct rate, once the correct rate Buzaishenggao even started to decline, it feels that the neural network has achieved good The state has to stop training.The curve in Figure 3 can be used to help people understand why the test set to prevent the emergence of excessive training. As can be seen in Figure training set and test set error rate increased with training in the beginning, continue to lower the increase of cycle, the test set error rate reached a trough Instead, it began Shang Sheng, people think it started to rise the moment Jiu Shi should stop training the moment.Neural network training cycle increases the accuracy of the changes Neural networks and statistical methods, in essence, there are many differences. Neural Network for a lot more than the statistical methods.Figure 4, there are 13 parameters (9 and 4 weight restrictions). Because so many parameters, through various combinations of parameters to affect the output, so difficult that a neural network model to make visual interpretation. Neural networks is in fact as a "black box" to use, not to manage "box" inside what is simply used on the line. In most cases, this restriction is acceptable. Such as banks may need a handwriting recognition software, but he did not need to know why these lines together is a person's signature, while a similar not. In many complex problems such as high degree of chemical testing, robotics, simulation of financial markets, and language image recognition, neural networks and other fields have achieved very good results.Another advantage of neural network is easily implemented on parallel computers, can he be assigned to different CPU nodes in parallel computing.When using the neural network points to note: first, the neural network is difficult to explain, has yet to make a clear interpretation of the neural network methodology. Second, the neural network will learn too, to train the neural network must be appropriate to use a neural network can be critically evaluated the methods, such as the previously mentioned methods and cross-validation test set method. This is mainly due to neural network is too flexible, too many variable parameters, if given enough time, he almost can "remember" anything.Third, unless the problem is very simple to train a neural network may need considerable time to complete. Of course, once the neural network was good, and the forecasts do with it, or will soon be running.Fourth, the establishment of neural networks need a lot of work to do data preparation. A very misleading myth is that no matter what data neural networks can work well and make accurate predictions. This is inaccurate, in order to obtain accurate models of the data must be careful cleaning, sorting, transformation, selection, etc., of any data mining is that the neural network with particular emphasis on this point. Such as neural networks require that all input variables must be 0-1 (or -1 - +1) between the real number, so as the "area" like the text data need to be done only after the necessary processing for neural networks input.中文译文人工神经网络:基本特征由大量处理单元互联组成的非线性、自适应信息处理系统。
人工智能9人工神经网络基础
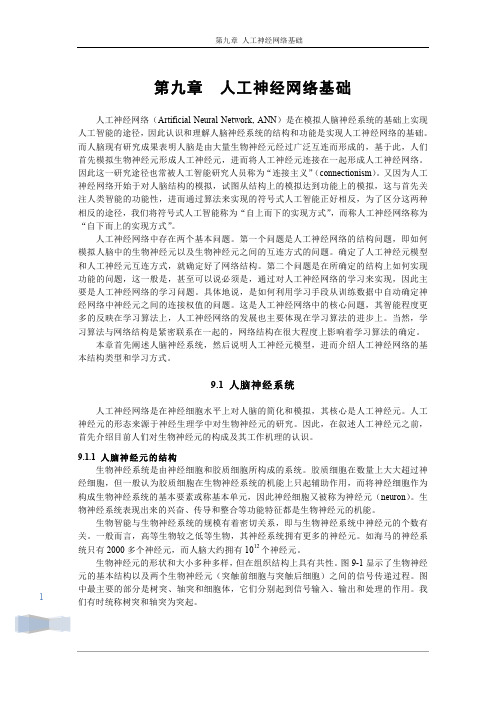
第九章人工神经网络基础人工神经网络(Artificial Neural Network, ANN)是在模拟人脑神经系统的基础上实现人工智能的途径,因此认识和理解人脑神经系统的结构和功能是实现人工神经网络的基础。
而人脑现有研究成果表明人脑是由大量生物神经元经过广泛互连而形成的,基于此,人们首先模拟生物神经元形成人工神经元,进而将人工神经元连接在一起形成人工神经网络。
因此这一研究途径也常被人工智能研究人员称为“连接主义”(connectionism)。
又因为人工神经网络开始于对人脑结构的模拟,试图从结构上的模拟达到功能上的模拟,这与首先关注人类智能的功能性,进而通过算法来实现的符号式人工智能正好相反,为了区分这两种相反的途径,我们将符号式人工智能称为“自上而下的实现方式”,而称人工神经网络称为“自下而上的实现方式”。
人工神经网络中存在两个基本问题。
第一个问题是人工神经网络的结构问题,即如何模拟人脑中的生物神经元以及生物神经元之间的互连方式的问题。
确定了人工神经元模型和人工神经元互连方式,就确定好了网络结构。
第二个问题是在所确定的结构上如何实现功能的问题,这一般是,甚至可以说必须是,通过对人工神经网络的学习来实现,因此主要是人工神经网络的学习问题。
具体地说,是如何利用学习手段从训练数据中自动确定神经网络中神经元之间的连接权值的问题。
这是人工神经网络中的核心问题,其智能程度更多的反映在学习算法上,人工神经网络的发展也主要体现在学习算法的进步上。
当然,学习算法与网络结构是紧密联系在一起的,网络结构在很大程度上影响着学习算法的确定。
本章首先阐述人脑神经系统,然后说明人工神经元模型,进而介绍人工神经网络的基本结构类型和学习方式。
9.1 人脑神经系统人工神经网络是在神经细胞水平上对人脑的简化和模拟,其核心是人工神经元。
人工神经元的形态来源于神经生理学中对生物神经元的研究。
因此,在叙述人工神经元之前,首先介绍目前人们对生物神经元的构成及其工作机理的认识。
外文翻译---人工神经网络
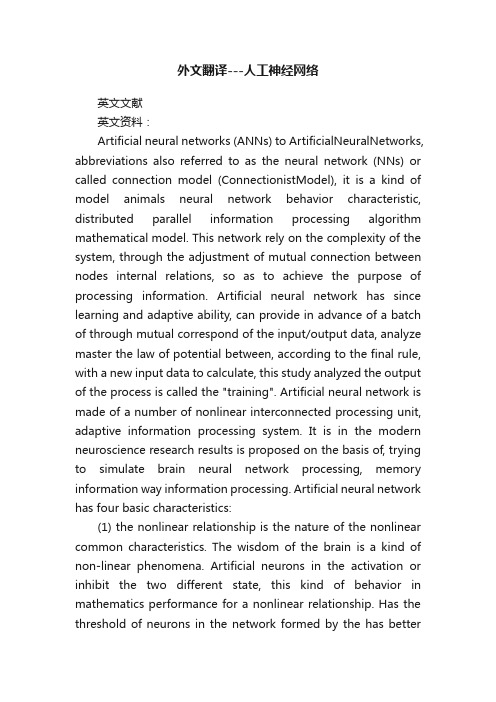
外文翻译---人工神经网络英文文献英文资料:Artificial neural networks (ANNs) to ArtificialNeuralNetworks, abbreviations also referred to as the neural network (NNs) or called connection model (ConnectionistModel), it is a kind of model animals neural network behavior characteristic, distributed parallel information processing algorithm mathematical model. This network rely on the complexity of the system, through the adjustment of mutual connection between nodes internal relations, so as to achieve the purpose of processing information. Artificial neural network has since learning and adaptive ability, can provide in advance of a batch of through mutual correspond of the input/output data, analyze master the law of potential between, according to the final rule, with a new input data to calculate, this study analyzed the output of the process is called the "training". Artificial neural network is made of a number of nonlinear interconnected processing unit, adaptive information processing system. It is in the modern neuroscience research results is proposed on the basis of, trying to simulate brain neural network processing, memory information way information processing. Artificial neural network has four basic characteristics:(1) the nonlinear relationship is the nature of the nonlinear common characteristics. The wisdom of the brain is a kind of non-linear phenomena. Artificial neurons in the activation or inhibit the two different state, this kind of behavior in mathematics performance for a nonlinear relationship. Has the threshold of neurons in the network formed by the has betterproperties, can improve the fault tolerance and storage capacity.(2) the limitations a neural network by DuoGe neurons widely usually connected to. A system of the overall behavior depends not only on the characteristics of single neurons, and may mainly by the unit the interaction between the, connected to the. Through a large number of connection between units simulation of the brain limitations. Associative memory is a typical example of limitations.(3) very qualitative artificial neural network is adaptive, self-organizing, learning ability. Neural network not only handling information can have all sorts of change, and in the treatment of the information at the same time, the nonlinear dynamic system itself is changing. Often by iterative process description of the power system evolution.(4) the convexity a system evolution direction, in certain conditions will depend on a particular state function. For example energy function, it is corresponding to the extreme value of the system stable state. The convexity refers to the function extreme value, it has DuoGe DuoGe system has a stable equilibrium state, this will cause the system to the diversity of evolution.Artificial neural network, the unit can mean different neurons process of the object, such as characteristics, letters, concept, or some meaningful abstract model. The type of network processing unit is divided into three categories: input unit, output unit and hidden units. Input unit accept outside the world of signal and data; Output unit of output system processing results; Hidden unit is in input and output unit, not between by external observation unit. The system The connections between neurons right value reflect the connection between the unit strength, information processing and embodied in the network said theprocessing unit in the connections. Artificial neural network is a kind of the procedures, and adaptability, brain style of information processing, its essence is through the network of transformation and dynamic behaviors have akind of parallel distributed information processing function, and in different levels and imitate people cranial nerve system level of information processing function. It is involved in neuroscience, thinking science, artificial intelligence, computer science, etc DuoGe field cross discipline.Artificial neural network is used the parallel distributed system, with the traditional artificial intelligence and information processing technology completely different mechanism, overcome traditional based on logic of the symbols of the artificial intelligence in the processing of intuition and unstructured information of defects, with the adaptive, self-organization and real-time characteristic of the study.Development historyIn 1943, psychologists W.S.M cCulloch and mathematical logic W.P home its established the neural network and the math model, called MP model. They put forward by MP model of the neuron network structure and formal mathematical description method, and prove the individual neurons can perform the logic function, so as to create artificial neural network research era. In 1949, the psychologist put forward the idea of synaptic contact strength variable. In the s, the artificial neural network to further development, a more perfect neural network model was put forward, including perceptron and adaptive linear elements etc. M.M insky, analyzed carefully to Perceptron as a representative of the neural network system function and limitations in 1969 after the publication of the book "Perceptron, and points out thatthe sensor can't solve problems high order predicate. Their arguments greatly influenced the research into the neural network, and at that time serial computer and the achievement of the artificial intelligence, covering up development new computer and new ways of artificial intelligence and the necessity and urgency, make artificial neural network of research at a low. During this time, some of the artificial neural network of the researchers remains committed to this study, presented to meet resonance theory (ART nets), self-organizing mapping, cognitive machine network, but the neural network theory study mathematics. The research for neural network of research and development has laid a foundation. In 1982, the California institute of J.J.H physicists opfield Hopfield neural grid model proposed, and introduces "calculation energy" concept, gives the network stability judgment. In 1984, he again put forward the continuous time Hopfield neural network model for the neural computers, the study of the pioneering work, creating a neural network for associative memory and optimization calculation, the new way of a powerful impetus to the research into the neural network, in 1985, and scholars have proposed a wave ears, the study boltzmann model using statistical thermodynamics simulated annealing technology, guaranteed that the whole system tends to the stability of the points. In 1986 the cognitive microstructure study, puts forward the parallel distributed processing theory. Artificial neural network of research by each developed country, the congress of the United States to the attention of the resolution will be on jan. 5, 1990 started ten years as the decade of the brain, the international research organization called on its members will the decade of the brain into global behavior. In Japan's "real world computing(springboks claiming)" project, artificial intelligence research into an important component.Network modelArtificial neural network model of the main consideration network connection topological structure, the characteristics, the learning rule neurons. At present, nearly 40 kinds of neural network model, with back propagation network, sensor, self-organizing mapping, the Hopfieldnetwork.the computer, wave boltzmann machine, adapt to the ear resonance theory. According to the topology of the connection, the neural network model can be divided into:(1) prior to the network before each neuron accept input and output level to the next level, the network without feedback, can use a loop to no graph. This network realization from the input space to the output signal of the space transformation, it information processing power comes from simple nonlinear function of DuoCi compound. The network structure is simple, easy to realize. Against the network is a kind of typical prior to the network.(2) the feedback network between neurons in the network has feedback, can use a no to complete the graph. This neural network information processing is state of transformations, can use the dynamics system theory processing. The stability of the system with associative memory function has close relationship. The Hopfield network.the computer, wave ear boltzmann machine all belong to this type.Learning typeNeural network learning is an important content, it is through the adaptability of the realization of learning. According to the change of environment, adjust to weights, improve thebehavior of the system. The proposed by the Hebb Hebb learning rules for neural network learning algorithm to lay the foundation. Hebb rules say that learning process finally happened between neurons in the synapse, the contact strength synapses parts with before and after the activity and synaptic neuron changes. Based on this, people put forward various learning rules and algorithm, in order to adapt to the needs of different network model. Effective learning algorithm, and makes the godThe network can through the weights between adjustment, the structure of the objective world, said the formation of inner characteristics of information processing method, information storage and processing reflected in the network connection. According to the learning environment is different, the study method of the neural network can be divided into learning supervision and unsupervised learning. In the supervision and study, will the training sample data added to the network input, and the corresponding expected output and network output, in comparison to get error signal control value connection strength adjustment, the DuoCi after training to a certain convergence weights. While the sample conditions change, the study can modify weights to adapt to the new environment. Use of neural network learning supervision model is the network, the sensor etc. The learning supervision, in a given sample, in the environment of the network directly, learning and working stages become one. At this time, the change of the rules of learning to obey the weights between evolution equation of. Unsupervised learning the most simple example is Hebb learning rules. Competition rules is a learning more complex than learning supervision example, it is according to established clustering on weights adjustment. Self-organizing mapping, adapt to theresonance theory is the network and competitive learning about the typical model.Analysis methodStudy of the neural network nonlinear dynamic properties, mainly USES the dynamics system theory and nonlinear programming theory and statistical theory to analysis of the evolution process of the neural network and the nature of the attractor, explore the synergy of neural network behavior and collective computing functions, understand neural information processing mechanism. In order to discuss the neural network and fuzzy comprehensive deal of information may, the concept of chaos theory and method will play a role. The chaos is a rather difficult toprecise definition of the math concepts. In general, "chaos" it is to point to by the dynamic system of equations describe deterministic performance of the uncertain behavior, or call it sure the randomness. "Authenticity" because it by the intrinsic reason and not outside noise or interference produced, and "random" refers to the irregular, unpredictable behavior, can only use statistics method description. Chaotic dynamics of the main features of the system is the state of the sensitive dependence on the initial conditions, the chaos reflected its inherent randomness. Chaos theory is to point to describe the nonlinear dynamic behavior with chaos theory, the system of basic concept, methods, it dynamics system complex behavior understanding for his own with the outside world and for material, energy and information exchange process of the internal structure of behavior, not foreign and accidental behavior, chaos is a stationary. Chaotic dynamics system of stationary including: still, stable quantity, the periodicity, with sex and chaos of accuratesolution... Chaos rail line is overall stability and local unstable combination of results, call it strange attractor.A strange attractor has the following features: (1) some strange attractor is a attractor, but it is not a fixed point, also not periodic solution; (2) strange attractor is indivisible, and that is not divided into two and two or more to attract children. (3) it to the initial value is very sensitive, different initial value can lead to very different behavior.superiorityThe artificial neural network of characteristics and advantages, mainly in three aspects: first, self-learning. For example, only to realize image recognition that the many different image model and the corresponding should be the result of identification input artificial neural network, the network will through the self-learning function, slowly to learn to distinguish similar images. The self-learning function for the forecast has special meaning. The prospect of artificial neural network computer will provide mankind economic forecasts, market forecast, benefit forecast, the application outlook is very great. The second, with lenovo storage function. With the artificial neural network of feedback network can implement this association. Third, with high-speed looking for the optimal solution ability. Looking for a complex problem of the optimal solution, often require a lot of calculation, the use of a problem in some of the design of feedback type and artificial neural network, use the computer high-speed operation ability, may soon find the optimal solution.Research directionThe research into the neural network can be divided into the theory research and application of the two aspects of research.Theory study can be divided into the following two categories: 1, neural physiological and cognitive science research on human thinking and intelligent mechanism.2, by using the neural basis theory of research results, with mathematical method to explore more functional perfect, performance more superior neural network model, the thorough research network algorithm and performance, such as: stability and convergence, fault tolerance, robustness, etc.; The development of new network mathematical theory, such as: neural network dynamics, nonlinear neural field, etc.Application study can be divided into the following two categories:1, neural network software simulation and hardware realization of research.2, the neural network in various applications in the field of research. These areas include: pattern recognition, signal processing, knowledge engineering, expert system, optimize the combination, robot control, etc. Along with the neural network theory itself and related theory, related to the development of technology, the application of neural network will further.Development trend and research hot spotArtificial neural network characteristic of nonlinear adaptive information processing power, overcome traditional artificial intelligence method for intuitive, such as mode, speech recognition, unstructured information processing of the defects in the nerve of expert system, pattern recognition and intelligent control, combinatorial optimization, and forecast areas to be successful application. Artificial neural network and other traditional method unifies, will promote the artificial intelligence and information processing technology development. In recentyears, the artificial neural network is on the path of human cognitive simulation further development, and fuzzy system, genetic algorithm, evolution mechanism combined to form a computational intelligence, artificial intelligence is an important direction in practical application, will be developed. Information geometry will used in artificial neural network of research, to the study of the theory of the artificial neural network opens a new way. The development of the study neural computers soon, existing product to enter the market. With electronics neural computers for the development of artificial neural network to provide good conditions.Neural network in many fields has got a very good application, but the need to research is a lot. Among them, are distributed storage, parallel processing, since learning, the organization and nonlinear mapping the advantages of neural network and other technology and the integration of it follows that the hybrid method and hybrid systems, has become a hotspot. Since the other way have their respective advantages, so will the neural network with other method, and the combination of strong points, and then can get better application effect. At present this in a neural network and fuzzy logic, expert system, genetic algorithm, wavelet analysis, chaos, the rough set theory, fractal theory, theory of evidence and grey system and fusion.汉语翻译人工神经网络(ArtificialNeuralNetworks,简写为ANNs)也简称为神经网络(NNs)或称作连接模型(ConnectionistModel),它是一种模范动物神经网络行为特征,进行分布式并行信息处理的算法数学模型。
ArtificialNeuralNetworks人工神经网络

11/08/2018
Artificial Neural Networks - I
16
赫布律
• 当细胞A的一个轴突和细胞B 很近,足以对它产 生影响,并且持久地、不断地参与了对细胞B 的 兴奋,那么在这两个细胞或其中之一会发生某 种生长过程或新陈代谢变化,以致于A作为能使 B 兴奋的细胞之一,它的影响加强了。
11/08/2018 Artificial Neural Networks - I 8
The Artificial Neuron
Stimulus
ui t wij x j t
j
x1(t)
x2(t) x3(t)
wi1 wi2 wi3 wi4 wi5
w
j
ij
x j (t )
13
Spiking Neuron Dynamics
neuron output
2.5 2 1.5 1 0.5 0 0 -0.5 -1 10 20 30 40 50 60 70 80 90 100 t V
y(t) urest+(t-tf)
11/08/2018
Artificial Neural Networks - I
11/08/2018
Artificial Neural Networks - I
17
Hebb’s Postulate: revisited
• • • Stent (1973), and Changeux and Danchin (1976) have expanded Hebb’s rule such that it also models inhibitory synapses:
2/(1+exp(-x))-1
- 1、下载文档前请自行甄别文档内容的完整性,平台不提供额外的编辑、内容补充、找答案等附加服务。
- 2、"仅部分预览"的文档,不可在线预览部分如存在完整性等问题,可反馈申请退款(可完整预览的文档不适用该条件!)。
- 3、如文档侵犯您的权益,请联系客服反馈,我们会尽快为您处理(人工客服工作时间:9:00-18:30)。
附录二英文参考文献原文Artificial Neural NetworksArtificial Neural Networks - Basic FeaturesComposed of a large number of processing units connected by a nonlinear, adaptive information processing system. It is the basis for modern neuroscience research findings presented, trying to simulate a large neural network processing, memory, information processing way of information. Artificial neural network has four basic characteristics:(1) non-linear non-linear relationship is the general characteristics of the natural world. The wisdom of the brain is a nonlinear phenomenon. Artificial neural activation or inhibition in two different states, this behavior mathematically expressed as a linear relationship. Threshold neurons have a network with better performance, can improve fault tolerance and storage capacity.(2) non-limitation of a neural network is usually more extensive neuronal connections made. The overall behavior of a system depends not only on the characteristics of single neurons, and may primarily by interaction between units, connected by the decision. By a large number of connections between the cells of non-simulated brain limitations. Associative memory limitations of a typical example of non-(3) characterization of artificial neural network is adaptive, self-organizing, self-learning ability. Neural networks can not only deal with the changes of information, but also process information the same time, nonlinear dynamic system itself is also changing. Iterative process is frequently used in describing the evolution of dynamical systems.(4) Non-convexity of the direction of the evolution of a system, under certain conditions, will depend on a particular state function. Such as energy function, and its extreme value corresponding to the state of the system more stable. Non-convexity of this function is more than one extremum, this system has multiple stable equilibrium, which will cause the system to the evolution of diversity.Artificial neural network, neural processing unit can be expressed in different objects, such as features, letters, concepts, or some interesting abstract patterns. The type of network processing unit is divided into three categories: input units, output units and hidden units. Input unit receiving the signal and data outside world; output unit for processing the results to achieve the output; hidden unit is in between the input and output units can not be observed from outside the system unit. Neurons and the connection weights reflect the strength of the connections between elements of information representation and processing reflected in the network processing unitconnected relationships. Artificial neural network is a non-procedural, adaptability, the brain's information processing style, its essence is transformation through the network and dynamic behavior is a parallel distributed information processing, and to varying degrees and levels mimic brain information processing system. It is involved in neural science, thinking, science and artificial intelligence, computer science and other interdisciplinary fields. Artificial neural networks are parallel distributed systems, using traditional artificial intelligence and information processing technology is completely different mechanism to overcome the traditional symbol of artificial intelligence-based logic in dealing with intuition, unstructured information deficiencies, adaptive, Self-organization and the characteristics of real-time learning.Artificial Neural Network – HistoryIn 1943, psychologist WSMcCulloch mathematical logician W. Pitts neural network and the establishment of a mathematical model, called the MP model. They put forward by MP model neurons and network structure of formal mathematical description of methods, that a single neuron can perform logic functions, thus creating the era of artificial neural network. In 1949, psychologists proposed the idea of synaptic strength variable. 60 years, artificial neural network to the further development of improved neural network models have been proposed, including the sensors and the adaptive linear element, etc.. M. Minsky and so careful analysis of the sensor represented by the neural network system capabilities and limitations, the in 1969 published a "Perceptron" book, pointed out that the sensor can not solve the issue of higher order predicate. Their argument has greatly influenced research in neural networks, combined with serial computers and artificial intelligence at the achievements made to cover up the development of new computer and artificial intelligence, new ways of necessity and urgency to the research of artificial neural networks at a low ebb . In the meantime, some artificial neural network remains committed to the study, researchers proposed to adapt resonance theory (ART Wang), Zi Zuzhiyingshe, Ren Zhi machine network, while for the neural network Shuxue research. More research and development of neural network research foundation. In 1982, California Institute of Technology physicist JJHopfield proposed Hopfield neural grid model, the concept of "computational energy" concept gives the network stability of the judge. In 1984, he made continuous time Hopfield neural network model for the neural computer research done pioneering work to create a neural network for associative memory and optimization of computing new ways to effectively promote the study of neural networks, In 1985, there are scholars of the Boltzmann model, the use of statistical thermodynamics in the study simulated annealing technology to ensure the overall stability of the whole system tends to point. 1986 to study the microstructure of cognition, proposed the theory of parallel distributed processing. Artificial neural networks in various countries of the importance attached by the U.S. Congress passed a resolution to January 5, 1990 began with a decade as the "brain decade", the international research organization called on its members to "brain of the 10 in "to the global behavior. In Japan's"real-world computing (RWC)" project, the artificial intelligence research has become an important part.The main consideration of artificial neural network model topology of the network connection, the characteristics of neurons, learning rules. Currently, nearly 40 kinds of neural network model, including back propagation network, perceptron, self organizing maps, Hopfield networks, Boltzmann machines, meet the resonance theory. According to the connection topology, neural network model can be divided into:(1) before the network to the network each neuron for the former level of input and output to the next level, network, no feedback, you can use a directed loop-free graph. This network signal from the input space and output space transform its information processing capabilities from a simple nonlinear function of several complex. Network structure is simple, easy to implement. Back-propagation network is a typical feedforward network.(2) feedback network network neurons have feedback, you can use an undirected complete graph. This neural network information processing is the state of transformation, you can deal with dynamic systems theory. Stability of the system is closely related with the associative memory function. Hopfield networks, Boltzmann machines belong to this category.Neural network learning is an important part of its adaptability is achieved through learning. According to the environmental changes in the value of the right to make adjustments to improve the system's behavior. Hebb proposed by the Hebb learning rule neural network learning algorithm for the foundation. Hebb learning rule that eventually occurred in the synapses between neurons, synaptic contacts with the synaptic strength of neuronal activity before and after the change. On this basis, it proposed a variety of learning rules and algorithms to meet the needs of different network models. Effective learning algorithm, making neural network connection weights through the adjustment, construction of the objective world of the intrinsic representation, forming a unique information processing method, information Cunchu and processing reflected in the network connection.According to different learning environment, neural network learning methods can be divided into supervised learning and unsupervised learning. In monitoring the study, the training sample data added to the network input side, while the corresponding desired output and network output compared Dedao error signal, thereby Kongzhiquanzhi connection strength adjustments, Jing Hou several training convergence to a determine the weights. When the sample situation changes, the weights can be modified by learning to adapt to the new environment. Use supervised learning back propagation neural network model has the network, and HC. Non-supervised learning, the prior is not a given standard sample placed directly into the network environment, learning stage and become one session. At this point, learnto obey the law of change in the evolution equation of connection weights. Non-supervised learning the most simple example is the Hebb learning rule. Competitive learning rule is a more complex example of non-supervised learning, which is based on the established clustering weight adjustment. Self-organizing map, resonance theory network to adapt to competition learn about all the typical model.Study of nonlinear dynamics of neural networks, the main use of dynamic systems theory, nonlinear programming theory and statistical theory to analyze the evolutionary neural network attractor nature of the process and to explore the neural network of collaborative behavior and collective computing to understand neural information processing mechanism. To investigate the neural networks and fuzzy in the integrity of information in terms of dealing with the possibility of chaos theory concepts and methods could be useful. Chaos is a very difficult to precisely defined mathematical concept. Generally speaking, the "chaos" refers to the dynamic system described by deterministic equations demonstrated the non-deterministic behavior, or call to determine the randomness. "Certainty" because it causes from within and not outside noise or interference generated, and "random" refers to the irregular, unpredictable behavior, can only be described using statistical methods. The main features of chaotic dynamic system is its state of sensitive dependence on initial conditions, reflect the inherent randomness of chaos. Chaos theory is the description of chaotic behavior with the basic theory of nonlinear dynamical systems, concepts, methods, and it is to understand the dynamic complexity of the system for their own actions with the outside world and its material, energy and information exchange process in the inner structured, and not external and accidental behavior, chaotic state is a steady state. Steady state chaotic dynamical systems, including: static, steady volume, periodicity, quasi-period and chaotic solution. Lorenz is the overall stability and local instability in the results of the combination, called the strange attractor. A strange attractor has the following characteristics: (1) strange attractor is an attractor, but it is neither fixed points nor periodic solutions; (2) strange attractor is indivisible, that can not be divided into two and two or more attractors; (3) is very sensitive to its initial value, different initial values will lead to very different behavior.Artificial neural network - advantagesArtificial neural network features and advantages mainly in three aspects:First, self-learning function. For example, in pattern recognition, only the first of many different images in the model and the corresponding results should be identified input of artificial neural network, the network will be through self-learning function, and slowly learn to identify similar images. Self-learning function for the forecasts of particular importance. Expected future human artificial neural network computer will provide economic forecast, market forecasting, prediction efficiency, the application of the future is very bright.Second, with the association storage. Artificial neural network feedback network can achieve this association.Third, find the optimal solution with high capacity. Find the optimal solution of a complex problem, often requires a great amount of computation, the use of a problem for the design of a feedback type artificial neural network, play a computer's high-speed computing power, may soon find the optimal solution.Artificial neural network - researchArtificial Neural NetworksNeural network research can be divided into theoretical and applied research on two areas.Theory can be divided into the following categories:1, using neuropsychological and cognitive science of human thinking and intelligence mechanism.2, the neural basis of theoretical research, using mathematical methods to explore a more complete functional performance, superior of the neural network model, in-depth study and performance of network algorithms, such as: stability, convergence, fault tolerance, robustness, etc.; Development mathematical theory of the new network, such as: neural network dynamics, nonlinear neural farms.Applied research can be divided into the following categories:1, neural network software simulation and hardware implementation of the study.2, neural network applications in all areas of research. These areas include:Pattern recognition, signal processing, knowledge engineering, expert system, optimize, robot control. With the neural network theory and the theory itself, the continuous development of relevant technology, application of neural networks will be more in-depth.Artificial Neural Networks - ApplicationNeural networks more and more attention recently because it was solved by the complexity of the problem provides a relatively simple and effective way. Neural networks can easily solve problems with hundreds of parameters (of course the actual existence of the neural network organisms than the procedures described here simulated neural networks are much more complex). Neural network used in two problems: classification and regression. In the structure, a neural network can be divided into input layer, output layer and hidden layer (see Figure 1). Each input layernode corresponds to a forecast of a variable. Output layer of nodes corresponding to the target variable can have more than one. In the input layer and output layer is hidden layer (the neural network is not visible for the user), number of layers and each layer of hidden layer nodes determines the number of neural network complexity.Figure 1 A neural networkIn addition to the input layer nodes, neural network, each node in front of it with a lot of nodes (called the node of input nodes) connected together, each connection corresponds to a weight Wxy, the value of this node is the node through which all input The value of the weights with the corresponding product and as a function of the input and get our activities in this function is called function or squeezing function. Figure 2, the output node 4 to node 6, the value can be calculated as follows:W14 * Node 1, the value of the + W24 * value of node 2Neural network each node can be expressed as predictor variables (nodes 1,2) value or the value of the portfolio (nodes 3-6). Attention to the value of the node 6 is no longer a linear combination of node 1,2, because the data passed in the hidden layer using the activity function. In fact, if there are no active function, then neural network is equivalent to a linear regression function, if the activity of a particular function is a nonlinear function, neural network that it is equivalent to logistic regression.Adjust the weights of connections between nodes is established (also called training) neural network to do work. The first and most basic method of weight adjustment feedback law is wrong, and now there are more changes in the new gradient method, Newton method, Levenberg-Marquardt method, and genetic algorithms. Regardless of the kind of training methods, we need to have some parameters to control the training process, such as training to prevent overtraining and control the pace.Figure 2 weighted Wxy the neural networkDecided to neural network topology (or architecture) is contained in the hidden layer and the number of nodes and connections between nodes. To design a neural network from scratch, have to decide the number of hidden layers and nodes, activities in the form of the function, as well as the right to redo that limit, of course, if using sophisticated software tools, he will help you decide these things. In many types of neural networks, the most commonly used to spread the former type neural network, which is ahead of us the kind depicted in the icon. People talk in detail below, in order to facilitate the discussion assumes that only contain a layer of hidden nodes. That the error feedback type training method is the change in slope of the simplified method, the process is as follows: prior to the dissemination of: data from input to output of the process is a front to back of the transmission process, after the value of a node connected to the node through which pass in front of come, then the values of theweights in accordance with the size of all the weighted input event function and then get the new value, and further spread to the next node.Feedback: When the output value of the node we expect the value of the difference, that is, an error occurs, the neural network would "learn" (learning from mistakes). We can weight between nodes connected as a node after the previous node on the "trust" level (of his own down the output of a node in front of him which is more vulnerable to the impact of input nodes). Learning are punitive approach, the process is as follows: If a node output error, then he see his error by which (some) input node of the impact caused, is not his most trusted node (the highest weight of node) in framing him (make him wrong), if the trust will have to lower his value (reducing weight), punish them, while those who make the right recommendations increase the trust value of nodes. Nodes who received punishment, he also needs the same way to further punish it in front of the node. Move forward one step on the way to spread until the punishment until the input nodes.Focus on the training must be repeated for each record this step, using the former to the spread of the output value, if an error occurs, then use the feedback method to learn. When the training set a record for each run over again, people said the completion of a training cycle. To complete the training of neural networks may take many months training period, often several hundred. After training by the neural network training set is found by the model, describes the training focused on response variables affected by the changes of predictor variables.As the hidden layer neural networks are too many variable parameters, if the training time long enough, then neural network training set is possible to all the details of information "in mind" down, Er not overdo the detail to establish a regular model only has We call this situation as overtraining. Obviously this "model" for training has a high accuracy of assembly, and once left the training set applied to other data, it is possible accuracy declined sharply. To prevent this training over the situation, one must know when to stop training. In some software implementations of the same training courses will use a test set to computational neuroscience network in this test set the correct rate, once the correct rate Buzaishenggao even started to decline, it feels that the neural network has achieved good The state has to stop training.The curve in Figure 3 can be used to help people understand why the test set to prevent the emergence of excessive training. As can be seen in Figure training set and test set error rate increased with training in the beginning, continue to lower the increase of cycle, the test set error rate reached a trough Instead, it began Shang Sheng, people think it started to rise the moment Jiu Shi should stop training the moment.Neural network training cycle increases the accuracy of the changesNeural networks and statistical methods, in essence, there are many differences. Neural Network for a lot more than the statistical methods. Figure 4, there are 13 parameters (9 and 4 weight restrictions). Because so many parameters, through various combinations of parameters to affect the output, so difficult that a neural network model to make visual interpretation. Neural networks is in fact as a "black box" to use, not to manage "box" inside what is simply used on the line. In most cases, this restriction is acceptable. Such as banks may need a handwriting recognition software, but he did not need to know why these lines together is a person's signature, while a similar not. In many complex problems such as high degree of chemical testing, robotics, simulation of financial markets, and language image recognition, neural networks and other fields have achieved very good results.Another advantage of neural network is easily implemented on parallel computers, can he be assigned to different CPU nodes in parallel computing.When using the neural network points to note: first, the neural network is difficult to explain, has yet to make a clear interpretation of the neural network methodology.Second, the neural network will learn too, to train the neural network must be appropriate to use a neural network can be critically evaluated the methods, such as the previously mentioned methods and cross-validation test set method. This is mainly due to neural network is too flexible, too many variable parameters, if given enough time, he almost can "remember" anything.Third, unless the problem is very simple to train a neural network may need considerable time to complete. Of course, once the neural network was good, and the forecasts do with it, or will soon be running.Fourth, the establishment of neural networks need a lot of work to do data preparation.A very misleading myth is that no matter what data neural networks can work well and make accurate predictions. This is inaccurate, in order to obtain accurate models of the data must be careful cleaning, sorting, transformation, selection, etc., of any data mining is that the neural network with particular emphasis on this point. Such as neural networks require that all input variables must be 0-1 (or -1 - +1) between the real number, so as the "area" like the text data need to be done only after the necessary processing for neural networks input.译文人工神经网络人工神经网络-基本特征由大量处理单元互联组成的非线性、自适应信息处理系统。