Gene快速使用指南
如何使用Gene
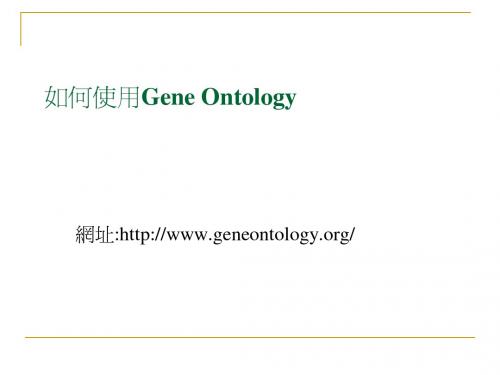
網址:/
在首頁的GO website 底下的 在首頁的 ontology files 點進去
在GO format 底下四個資料夾 function 、process、comnpent、defs 、 、 按右鍵另存新檔下載
程式使用順序
GO_格式化
Step1:run GO的格式化.cpp(把<字元取代成%字元) Input file: 下載下來的process.ontology.txt檔 output file:定為000 Step2 runGO_id 中的go.cpp(擷取2個欄位) Input file: 下載下來的gene_association.goa_human output file: 定為001 Step3:run 刪除多餘的GO.cpp(刪除兩列或多列相同的字串) Input file: 上步驟的 001 output file: 定為002
二、下載GO 對應的Swiss-Prot
在首頁的GO website 底下的 在首頁的 annotations點進去 點進去
找到Homo sapiens 找到 GO Annotations 下 載 按 右 鍵 另 存 新 檔
以下是程式使用說明 依照step逐一執行 紅色 表示功能及目的 棕色 表示要執行的程式檔 藍色 表示是輸入的程式檔 藍色 表示是輸出的程式檔
-
Step4:run GO_term中的GOid的F&P&C的term.cpp (擷取出term term關鍵字和goid關鍵字 ) tmponent.ontology.txt 裡面的GOID先截取 出來,再加上 下載下來的GO.defs檔 output file: 定為003 Step5:run GO_statement中的: GO的功能.cpp (印出Swissprot 和GO的id關鍵字以及%開頭的字串) Swissprot Input file:用step3的002 以及下載下來的function.ontology output file: 定為004
GeneSnap操作指南
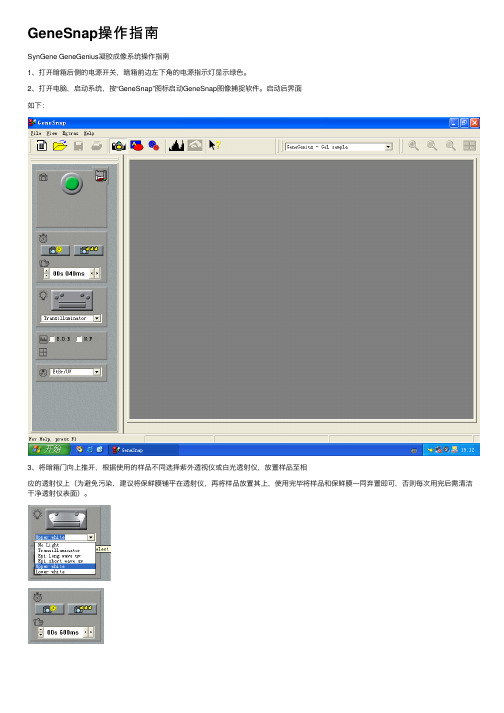
GeneSnap操作指南SynGene GeneGenius凝胶成像系统操作指南1、打开暗箱后侧的电源开关,暗箱前边左下⾓的电源指⽰灯显⽰绿⾊。
2、打开电脑,启动系统,按“GeneSnap”图标启动GeneSnap图像捕捉软件。
启动后界⾯如下:3、将暗箱门向上推开,根据使⽤的样品不同选择紫外透视仪或⽩光透射仪,放置样品⾄相应的透射仪上(为避免污染,建议将保鲜膜铺平在透射仪,再将样品放置其上,使⽤完毕将样品和保鲜膜⼀同弃置即可,否则每次⽤完后需清洁⼲净透射仪表⾯)。
4、根据机器配置不同,选择相应的光源(上左图,“No Light”⽆光源;“Transilluminator”紫外光源;“Epi long wave uv”365nm紫外投射光源(选配件),“Epi short wave uv”254nm 紫外投射光源(选配件),“Upper white”投射背景光源,仅⽤于调整样品位置;“Lower white”⽩光透射仪,需将透射仪放下);相应的滤光⽚(仅适⽤于配置滤光⽚轮的机器,上中图,随机器配置不同可能有不同滤光⽚,不带滤光⽚的机型该项为灰⾊,不需选择);根据样品不同,在上左图中选择相应曝光时间,蛋⽩样品时间较短,凝胶则需提⾼(⼀般在1秒左右)。
5、选定完毕,按上左图的绿⾊按钮开始实时预览,控制⾯板变成如上中图所⽰,同时屏幕将会显⽰暗箱中的图像,三个按钮可分别控制光圈、图像缩放、焦距调整。
在实际⼯作中,先适当调整光圈及曝光时间使样品可见(建议适当减少光圈,增⼤曝光时间,⼀般在1-2秒左右,在做凝胶时),使⽤缩放按钮使样品尽量占满屏幕,最后调整焦距使样品清晰可见,做综合调整即可获得适合图像,若效果合适,按下红⾊按钮即可将图像摄取下来。
6、摄取下来后在“File”菜单下的“Save”项保存⽂件(只能以“sgd”格式保存),假如要以其他格式(如BMP、TIF、JPG等格式保存),可以选择“File”下的“Export”项保存。
GenAlgo包使用指南说明书
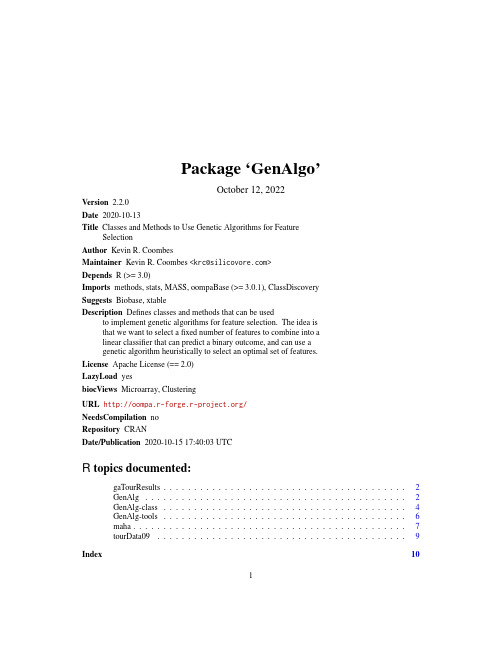
Package‘GenAlgo’October12,2022Version2.2.0Date2020-10-13Title Classes and Methods to Use Genetic Algorithms for FeatureSelectionAuthor Kevin R.CoombesMaintainer Kevin R.Coombes<******************>Depends R(>=3.0)Imports methods,stats,MASS,oompaBase(>=3.0.1),ClassDiscoverySuggests Biobase,xtableDescription Defines classes and methods that can be usedto implement genetic algorithms for feature selection.The idea isthat we want to select afixed number of features to combine into alinear classifier that can predict a binary outcome,and can use agenetic algorithm heuristically to select an optimal set of features.License Apache License(==2.0)LazyLoad yesbiocViews Microarray,ClusteringURL /NeedsCompilation noRepository CRANDate/Publication2020-10-1517:40:03UTCR topics documented:gaTourResults (2)GenAlg (2)GenAlg-class (4)GenAlg-tools (6)maha (7)tourData09 (9)Index101gaTourResults Results of a Genetic AlgorithmDescriptionWe ran a genetic algorithm tofind the optimal’fantasy’team for the competition run by the Versus broadcasting network for the2009Tour de France.In order to make the vignette run in a timely fashion,we saved the results in this data object.Usagedata(gaTourResults)FormatThere are four objects in the datafile.Thefirst is recurse,which is an object of the GenAlg-class representing thefinal generation.The other three objects are all numeric vector of length1100: diversity contains the average population diversity at each generation,fitter contains the max-imumfitness,and meanfit contains the meanfitness.SourceKevin R.CoombesGenAlg A generic Genetic Algorithm for feature selectionDescriptionThese functions allow you to initialize(GenAlg)and iterate(newGeneration)a genetic algorithm to perform feature selection for binary class prediction in the context of gene expression microarrays or other high-throughput technologies.UsageGenAlg(data,fitfun,mutfun,context,pm=0.001,pc=0.5,gen=1)newGeneration(ga)popDiversity(ga)Argumentsdata The initial population of potential solutions,in the form of a data matrix with one individual per row.fitfun A function to compute thefitness of an individual solution.Must take two input arguments:a vector of indices into rows of the population matrix,and a contextlist within which any other items required by the function can be resolved.Mustreturn a real number;higher values indicate betterfitness,with the maximumfitness occurring at the optimal solution to the underlying numerical problem.mutfun A function to mutate individual alleles in the population.Must take two argu-ments:the starting allele and a context list as in thefitness function.context A list of additional data required to perform mutation or to computefitness.This list is passed along as the second argument when fitfun and mutfun are called.pm A real value between0and1,representing the probability that an individual allele will be mutated.pc A real value between0and1,representing the probability that crossover will occur during reproduction.gen An integer identifying the current generation.ga An object of class GenAlgValueBoth the GenAlg generator and the newGeneration functions return a GenAlg-class object.The popDiversity function returns a real number representing the average diversity of the population.Here diversity is defined by the number of alleles(selected features)that differ in two individuals. Author(s)Kevin R.Coombes<******************>,P.Roebuck<***********************>See AlsoGenAlg-class,GenAlg-tools,maha.Examples#generate some fake datanFeatures<-1000nSamples<-50fakeData<-matrix(rnorm(nFeatures*nSamples),nrow=nFeatures,ncol=nSamples)fakeGroups<-sample(c(0,1),nSamples,replace=TRUE)myContext<-list(dataset=fakeData,gps=fakeGroups)#initialize populationn.individuals<-200n.features<-9y<-matrix(0,n.individuals,n.features)for(i in1:n.individuals){y[i,]<-sample(1:nrow(fakeData),n.features)}#set up the genetic algorithmmy.ga<-GenAlg(y,selectionFitness,selectionMutate,myContext,0.001,0.75)#advance one generationmy.ga<-newGeneration(my.ga)GenAlg-class Class"GenAlg"DescriptionObjects of the GenAlg class represent one step(population)in the evolution of a genetic algorithm.This algorithm has been customized to perform feature selection for the class prediction problem.Usage##S4method for signature GenAlgas.data.frame(x,s=NULL,optional=FALSE,...)##S4method for signature GenAlgas.matrix(x,...)##S4method for signature GenAlgsummary(object,...)Argumentsobject object of class GenAlgx object of class GenAlgs character vector giving the row names for the data frame,or NULLoptional logical scalar.If TRUE,setting row names and converting column names to syn-tactic names is optional....extra arguments for generic routinesObjects from the ClassObjects should be created by calls to the GenAlg generator;they will also be created automatically as a result of applying the function newGeneration to an existing GenAlg object.Slotsdata:The initial population of potential solutions,in the form of a data matrix with one individual per row.fitfun:A function to compute thefitness of an individual solution.Must take two input argu-ments:a vector of indices into the rows of the population matrix,and a context list within which any other items required by the function can be resolved.Must return a real num-ber;higher values indicate betterfitness,with the maximumfitness occurring at the optimal solution to the underlying numerical problem.mutfun:A function to mutate individual alleles in the population.Must take two arguments:the starting allele and a context list as in thefitness function.p.mutation:numeric scalar between0and1,representing the probability that an individual allele will be mutated.p.crossover:numeric scalar between0and1,representing the probability that crossover will occur during reproduction.generation:integer scalar identifying the current generation.fitness:numeric vector containing thefitness of all individuals in the population.best.fit:A numeric value;the maximumfitness.best.individual:A matrix(often with one row)containing the individual(s)achieving the max-imumfitness.context:A list of additional data required to perform mutation or to computefitness.This list is passed along as the second argument when fitfun and mutfun are called.Methodsas.data.frame signature(x="GenAlg"):Converts the GenAlg object into a data frame.The first column contains thefitness;remaining columns contain three selected features,given as integer indices into the rows of the original data matrix.as.matrix signature(x="GenAlg"):Converts the GenAlg object into a matrix,following the conventions of as.data.frame.summary signature(object="GenAlg"):Print a summary of the GenAlg object.Author(s)Kevin R.Coombes<******************>,P.Roebuck<***********************>ReferencesDavid Goldberg."Genetic Algorithms in Search,Optimization and Machine Learning."Addison-Wesley,1989.See AlsoGenAlg,GenAlg-tools,maha.ExamplesshowClass("GenAlg")6GenAlg-tools GenAlg-tools Utility functions for selection and mutation in genetic algorithmsDescriptionThese functions implement specific forms of mutation andfitness that can be used in genetic algo-rithms for feature selection.UsagesimpleMutate(allele,context)selectionMutate(allele,context)selectionFitness(arow,context)Argumentsallele In the simpleMutate function,allele is a binary vectorfilled with0’s and 1’s.In the selectionMutate function,allele is an integer(which is silentlyignored;see Details).arow A vector of integer indices identifying the rows(features)to be selected from the context$dataset matrix.context A list or data frame containing auxiliary information that is needed to resolve references from the mutation orfitness code.In both selectionMutate andselectionFitness,context must contain a dataset component that is eithera matrix or a data frame.In selectionFitness,the context must also includea grouping factor(with two levels)called gps.DetailsThese functions represent’callbacks’.They can be used in the function GenAlg,which creates objects.They will then be called repeatedly(for each individual in the population)each time the genetic algorithm is updated to the next generation.The simpleMutate function assumes that chromosomes are binary vectors,so alleles simply take on the value0or1.A mutation of an allele,therefore,flips its state between those two possibilities.The selectionMutate and selectionFitness functions,by contrast,are specialized to perform feature selection assuming afixed number K of features,with a goal of learning how to distinguish between two different groups of samples.We assume that the underlying data consists of a data frame(or matrix),with the rows representing features(such as genes)and the columns representing samples.In addition,there must be a grouping vector(or factor)that assigns all of the sample columns to one of two possible groups.These data are collected into a list,context,containinga dataset matrix and a gps factor.An individual member of the population of potential solutionsis encoded as a length K vector of indices into the rows of the dataset.An individual allele, therefore,is a single index identifying a row of the dataset.When mutating it,we assume that it can be changed into any other possible allele;i.e.,any other row number.To compute thefitness, we use the Mahalanobis distance between the centers of the two groups defined by the gps factor.maha7 ValueBoth selectionMutate and simpleMutate return an integer value;in the simpler case,the value is guaranteed to be a0or1.The selectionFitness function returns a real number.Author(s)Kevin R.Coombes<******************>,P.Roebuck<***********************>See AlsoGenAlg,GenAlg-class,maha.Examples#generate some fake datanFeatures<-1000nSamples<-50fakeData<-matrix(rnorm(nFeatures*nSamples),nrow=nFeatures,ncol=nSamples)fakeGroups<-sample(c(0,1),nSamples,replace=TRUE)myContext<-list(dataset=fakeData,gps=fakeGroups)#initialize populationn.individuals<-200n.features<-9y<-matrix(0,n.individuals,n.features)for(i in1:n.individuals){y[i,]<-sample(1:nrow(fakeData),n.features)}#set up the genetic algorithmmy.ga<-GenAlg(y,selectionFitness,selectionMutate,myContext,0.001,0.75)#advance one generationmy.ga<-newGeneration(my.ga)maha Compute the(squared)Mahalanobis distance between two groups ofvectorsDescriptionThe Mahalanobis distance between two groups of vectorsUsagemaha(data,groups,method="mve")8maha Argumentsdata A matrix with columns representing features(or variables)and rows represent-ing independent samplesgroups A factor or logical vector with length equal to the number of rows(samples)in the data matrixmethod A character string determining the method that should be used to estimate the covariance matrix.The default value of"mve"uses the cov.mve function fromthe MASS package.The other valid option is"var",which uses the var functionfrom the standard stats package.DetailsThe Mahalanobis distance between two groups of vectors is the distance between their centers, computed in the equivalent of a principal component space that accounts for different variances.ValueReturns a numeric vector of length1.Author(s)Kevin R.Coombes<******************>,P.Roebuck<***********************>ReferencesMardia,K.V.and Kent,J.T.and Bibby,J.M.Multivariate Analysis.Academic Press,Reading,MA1979,pp.213–254.See Alsocov.mve,varExamplesnFeatures<-40nSamples<-2*10dataset<-matrix(rnorm(nSamples*nFeatures),ncol=nSamples)groups<-factor(rep(c("A","B"),each=10))maha(dataset,groups)tourData099 tourData09Tour de France2009DescriptionEach row represents the performance of a rider in the2009Tour de France;the name and team of the rider are used as the row names.The four columns are the Cost(to include on a team in the Versus fantasy challenge),Scores(based on dailyfinishing position),JerseyBonus(for any days spent in one of the three main leader jerseys),and Total(the sum of Scores and JerseyBonus). Usagedata(tourData09)FormatA data frame with102rows and4columns.SourceThe data were collected in2009from the web site /tdfgames,which appears to no longer exist.Index∗classesGenAlg-class,4∗classifGenAlg-class,4∗datasetsgaTourResults,2tourData09,9∗multivariatemaha,7∗optimizeGenAlg,2GenAlg-class,4GenAlg-tools,6as.data.frame,GenAlg-method(GenAlg-class),4as.matrix,GenAlg-method(GenAlg-class), 4cov.mve,8diversity(gaTourResults),2fitter(gaTourResults),2 gaTourResults,2GenAlg,2,4–7GenAlg-class,4GenAlg-tools,6maha,3,5,7,7meanfit(gaTourResults),2 newGeneration,4newGeneration(GenAlg),2 popDiversity(GenAlg),2recurse(gaTourResults),2 selectionFitness(GenAlg-tools),6selectionMutate(GenAlg-tools),6simpleMutate(GenAlg-tools),6summary,GenAlg-method(GenAlg-class),4tourData09,9var,810。
gene_tool操作指南
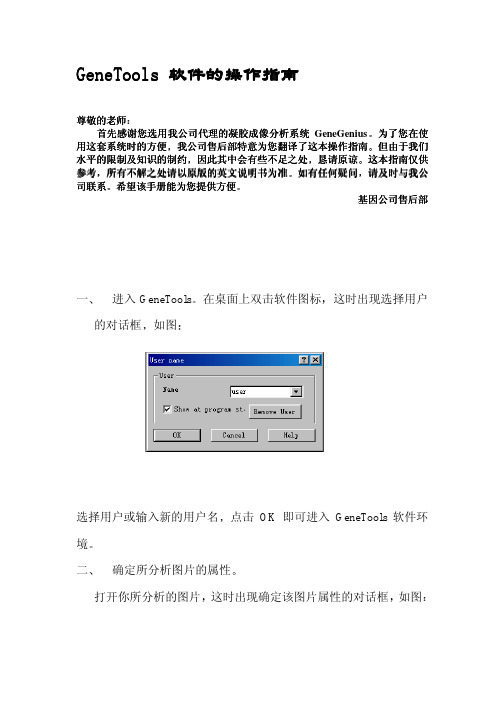
即可自动打开 Excel 程序 显示所有的结果
2 编辑新的 Marker
当 要 编 辑 新 的 Marker 时 在 标 准 分 子 量 对 话 框 Assign
molecular weight 中点击 Edit Standard 如图
再点击 New standard 按钮 出现编辑新 Marker 的对话框 如图
基因公司售后部
一 进入 GeneTools 在桌面上双击软件图标 这时出现选择用户 的对话框 如图
选择用户或输入新的用户名 点击 OK 即可进入 GeneTools 软件环 境 二 确定所分析图片的属性
打开你所分析的图片 这时出现确定该图片属性的对话框 如图
从 Document 中选择图片的种类 Colony Gel PCR Spot blot 从 Electrophoresis direction 中选择电泳的方向 从 Image type 中 选 择 图 片 的 类 型 Fluorescence ( 荧 光 ) Absorption(吸收光) 如果想手动调节选带 则可在 Number of 中输入泳道的数目 若想让软件自动寻道 可设为默认值 0 设置完后 点击 OK 即可进入分析环境
双击 Track1 即可得到第一泳道的波形图 再双击即可取消 该波形图 若依次双击其它泳道则所选泳道的波形同时出现在坐标 中 七 Spot blot 图片的分析
打开一个 Spot blot 图片后 在其属性中选取 Spot blot 然 后点击 OK 打开图片后若软件没有自动寻找到杂交点 可以手 动寻找 在菜单 Spot 中选择 locate 后 软件会自动定义每个杂 交点的位置 同时计算出它们的原始光密度值 如图
三 GeneTools 环境及工具栏的说明
GeneTools 操作指南(中文)

描述栏
描述栏显示当前泳道的一些相关信息,您可以在“Tracks”菜单使用使用“Description” 来编辑当前泳道的描述。
剖面图栏
剖面图栏显示当先选中泳道的峰值剖面图。 如果您选择其中一个泳道作为条带匹配标准,其剖面图会一直显示在该栏,以于其他的 泳道进行对比分析。 在“Extras”菜单 “Configuration”里可以对各个项目的颜色进行更改。 您也可以在剖面图栏对各个条带进行编辑,通过鼠标右键。
5. 使用双向箭头选择于分子量标注的第一峰
GeneTools
入门指南
基因有限公司售后服务部编译
编者言 本手册系原版英文说明书编译而来,希望它能为您操作该软件时 带来帮助。由于译者的水平限制,手册中可能存在错误和遗漏,如与 英文说明书发生冲突,请英文原版说明书为准。本手册只作为您操作 的参考依据,不能作为其他评定标准。
基因公司售后部应用工程师:张传峰 2004-3-23
分子量标准库
当前使用的分子量标准
4. 在分子量标准库里选择所需的分子量标准
运行 GeneTools
1 点击开始按钮、选择程序菜单,进入 Syngene 子菜单,选择 GeneTools,双击运行。 根据程序的设置,用户名称对话框可能会出现在界面,如下图:
2 如果您先前已经输入用户名,您就可以从下拉框中选择您的用户名,否则输入您的用 户名。 用户名将被打印在报告中,保存在文件的历史记录中,以便于您追踪是谁创建和修改 了该文件。 如果您不想显示用户名提示对话框,您可以不选择“Show at program”,这样以来, 最后注册使用用户名就会被默认为当前用户,自动加载。 3 从 Extras 菜单中选择 Configuration,弹出 GeneTools configuration (软件属性 设置)对话框:
基因编辑技术的实验操作指南
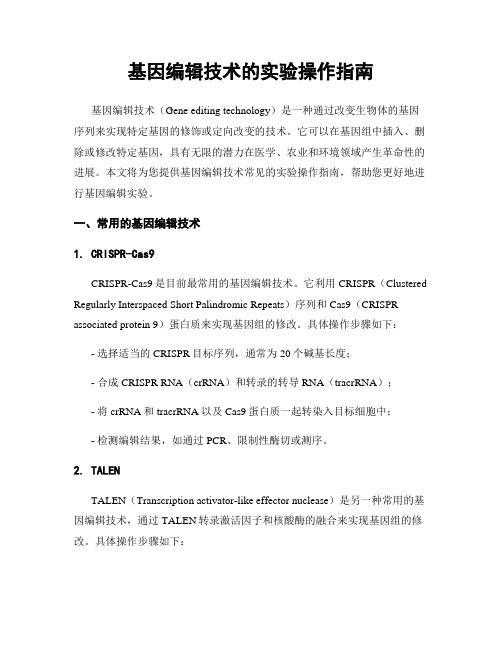
基因编辑技术的实验操作指南基因编辑技术(Gene editing technology)是一种通过改变生物体的基因序列来实现特定基因的修饰或定向改变的技术。
它可以在基因组中插入、删除或修改特定基因,具有无限的潜力在医学、农业和环境领域产生革命性的进展。
本文将为您提供基因编辑技术常见的实验操作指南,帮助您更好地进行基因编辑实验。
一、常用的基因编辑技术1. CRISPR-Cas9CRISPR-Cas9是目前最常用的基因编辑技术。
它利用CRISPR(Clustered Regularly Interspaced Short Palindromic Repeats)序列和Cas9(CRISPR associated protein 9)蛋白质来实现基因组的修改。
具体操作步骤如下:- 选择适当的CRISPR目标序列,通常为20个碱基长度;- 合成CRISPR RNA(crRNA)和转录的转导RNA(tracrRNA);- 将crRNA和tracrRNA以及Cas9蛋白质一起转染入目标细胞中;- 检测编辑结果,如通过PCR、限制性酶切或测序。
2. TALENTALEN(Transcription activator-like effector nuclease)是另一种常用的基因编辑技术,通过TALEN转录激活因子和核酸酶的融合来实现基因组的修改。
具体操作步骤如下:- 设计合成TALEN结构域,根据目标基因的序列选择合适的TALEN结构域;- 将TALEN融合基因导入表达载体中;- 用质粒转染靶细胞,使TALEN蛋白进入细胞;- 监测基因组修饰效果,例如通过PCR、限制性酶切或测序。
二、实验操作指南1. 实验前准备在进行基因编辑实验前,需要准备以下实验材料和设备:- 细胞培养基和细胞培养器具;- CRISPR-Cas9或TALEN相关质粒和实验试剂盒;- 转染试剂;- DNA测序仪或其他用于验证编辑结果的设备。
E.Coil Genepulse Xcell 电穿孔操作指南
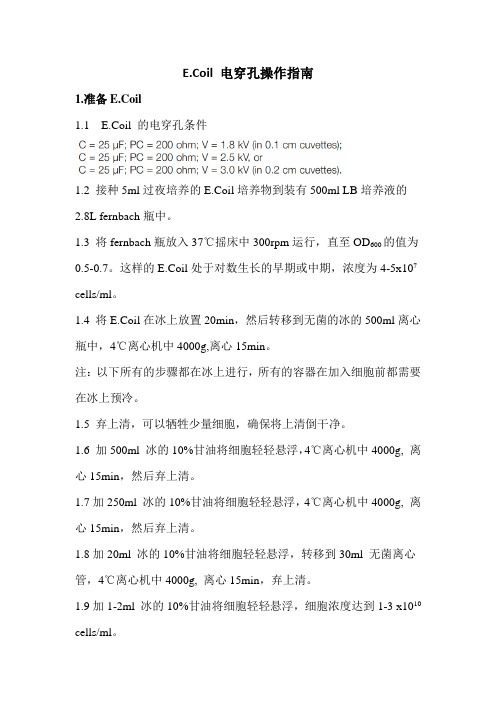
E.Coil 电穿孔操作指南1.准备E.Coil1.1 E.Coil 的电穿孔条件1.2 接种5ml过夜培养的E.Coil培养物到装有500ml LB培养液的2.8L fernbach瓶中。
1.3 将fernbach瓶放入37℃摇床中300rpm运行,直至OD600的值为0.5-0.7。
这样的E.Coil处于对数生长的早期或中期,浓度为4-5x107 cells/ml。
1.4 将E.Coil在冰上放置20min,然后转移到无菌的冰的500ml离心瓶中,4℃离心机中4000g,离心15min。
注:以下所有的步骤都在冰上进行,所有的容器在加入细胞前都需要在冰上预冷。
1.5 弃上清,可以牺牲少量细胞,确保将上清倒干净。
1.6 加500ml 冰的10%甘油将细胞轻轻悬浮,4℃离心机中4000g, 离心15min,然后弃上清。
1.7加250ml 冰的10%甘油将细胞轻轻悬浮,4℃离心机中4000g, 离心15min,然后弃上清。
1.8加20ml 冰的10%甘油将细胞轻轻悬浮,转移到30ml 无菌离心管,4℃离心机中4000g, 离心15min,弃上清。
1.9加1-2ml 冰的10%甘油将细胞轻轻悬浮,细胞浓度达到1-3x1010cells/ml。
2.0 将细胞悬液按每管20-50ul分装到1.5mlEP管中,-70℃储存,可保存6个月。
2.电转化2.1 将制备好的细胞悬液从-70℃中拿出来,冰上解冻。
每个样品准备如下:a. 1.5ml 离心管放于冰上;b. 0.1或0.2cm电击杯放于冰上;c. 17x100mm管子加入1ml SOC室温放置。
2.2 a. 选用0.1cm电击杯,在冰上的1.5ml 离心管中加入20ul细胞悬液,加入1-2ul DNA,混匀,冰上孵育1min。
b. 选用0.2cm电击杯,在冰上的1.5ml 离心管中加入20-40ul细胞悬液,加入1-2ul DNA,混匀,冰上孵育1min。
Gene Ontology(GO)使用指南(内部资料)
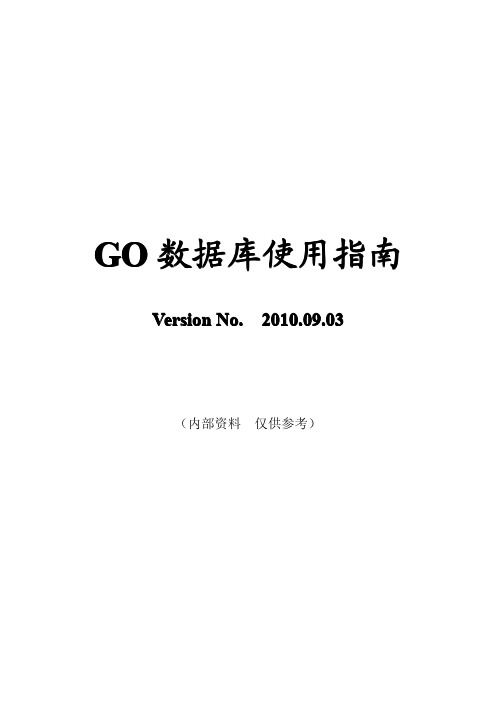
1.3.1 1.3.2 1.3.3 1.3.4 1.4 第二部分 2.1 2.2
语义之间关系的基本理解··················································································· 4 关系之间的推导··································································································· 5 调节控制关系(the regulates relation)及其推导··················································· 6 本体论的组织结构······························································································· 7
注:基因产物和其生物功能常常被我们混淆。例如, “乙醇脱氢酶”既可以指放在 Eppendorf 试管里的 基因产物,也表明了它的功能。但是这之间其实是存在差别的:一个基因产物可以拥有多种分子功能,多 种基因产物也可以行使同一种分子功能。比如还是“乙醇脱氢酶” ,其实多种基因产物都具有这种功能, 而 并不是所有的这些酶都是由乙醇脱氢酶基因编码的。一个基因产物可以同时具有 “乙醇脱氢酶”和“乙醛 歧化酶”两种功能,甚至更多。所以,在 GO 中,很重要的一点在于,当使用“乙醇脱氢酶活性”这种术 语时,所指的是功能,并不是基因产物。
GO 提供了一系列的语义(terms)用来描述基因、基因产物的特性。这些语义分为三种 不同的种类:细胞学组件,用于描述亚细胞结构、位置和大分子复合物,如核仁、端粒和识 别起始的复合物等;分子功能,用于描述基因、基因产物个体的功能,如与碳水化合物结合 或 ATP 水解酶活性等;生物学途径,指分子功能的有序组合,达成更广的生物功能,如有 丝分裂或嘌呤代谢等。 基因产物可能分别具有分子生物学上的功能、 生物学途径和在细胞中的组件作用。 当然, 它们也可能在某一个方面有多种性质。如细胞色素 C,在分子功能上体现为电子传递活性, 在生物学途径中与氧化磷酸化和细胞凋亡有关,在细胞中存在于线粒体质中和线粒体内膜 上。
- 1、下载文档前请自行甄别文档内容的完整性,平台不提供额外的编辑、内容补充、找答案等附加服务。
- 2、"仅部分预览"的文档,不可在线预览部分如存在完整性等问题,可反馈申请退款(可完整预览的文档不适用该条件!)。
- 3、如文档侵犯您的权益,请联系客服反馈,我们会尽快为您处理(人工客服工作时间:9:00-18:30)。
G e n e快速使用指南 The Standardization Office was revised on the afternoon of December 13, 2020
Gene5快速操作指南
初次使用前,请仔细阅读仪器和软件说明书或在有经验人员的指导下操作。
1 打开电脑,酶标仪,酶标仪进行自检,酶标仪样品托盘自动弹出,提示自检通过,可以进行上样检测。
2 点击桌面上Gene5图标打开软件,进入欢迎界面。
3 点击向导精灵图标,进入程序编辑。
4 对程序每一个步骤进行设置
3 全部设定好之后,点击Finish完成程序编辑。
4 选择File > Save 保存程序,并指定程序的名称和保存路径。
5 选择File > New Experiment,选择刚才编辑好的程序,点击OK。
6 选择 Read Plate,编辑板的信息后,点击Read,开始读板。
7 选择File > Save 保存Experiment,并指定Experiment的名称和保存路径。
利用Sample库进行检测
在Gene5欢迎界面中点击absorbance、Fluorescence或
Luminescence,选择需要的的实验类型,打开程序进行检测。
点击欢迎界面,可以用来打开最近一次打开的程序和实验结果
注意:实验结束,关闭软件、仪器。
注意保持仪器清洁,防尘、防潮、放阳光直射,本手册仅供参考,不作为操作标准流程,详细信息请阅读仪器和软件说明书。
基因有限公司市场部二零零七年三
月。