Categories and Categorization类别与分类
文本分类方法总结
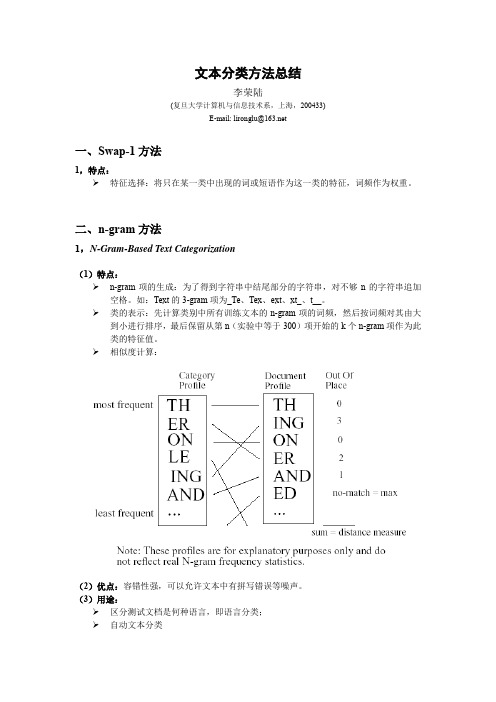
文本分类方法总结李荣陆(复旦大学计算机与信息技术系,上海,200433)E-mail: lironglu@一、Swap-1方法1,特点:特征选择:将只在某一类中出现的词或短语作为这一类的特征,词频作为权重。
二、n-gram方法1,N-Gram-Based Text Categorization(1)特点:n-gram项的生成:为了得到字符串中结尾部分的字符串,对不够n的字符串追加空格。
如:Text的3-gram项为_Te、Tex、ext、xt_、t__。
类的表示:先计算类别中所有训练文本的n-gram项的词频,然后按词频对其由大到小进行排序,最后保留从第n(实验中等于300)项开始的k个n-gram项作为此类的特征值。
相似度计算:(2)优点:容错性强,可以允许文本中有拼写错误等噪声。
(3)用途:区分测试文档是何种语言,即语言分类;自动文本分类2,CAN Bayes(Chain Augmented Naive Bayes)Bayes 分类器是一个性能很好的线性分类器,但是它假设文档的每个分类特征属性间是相互独立的,这显然是不成立的。
假设d i ={w i1,w i2,…,w in }为一任意文档,它属于文档类C ={c 1, c 2,…, c k }中的某一类c j 。
根据Bayes 分类器有:)()|()()()|()|(j j i i j j i i j c P c d P d P c P c d P d c P ∝=,其中∏==rk j ik j i c w P c d P 1)|()|(。
如果使用Bayes 网络来描述特征属性间的联系,则失去了Bayes 模型的简单性和线性特征。
我们使用了统计语言学中的N-Gram 模型,它假设一个词在文档中某个位置出现的概率仅与它之前的n-1个词有关,即:)|()|(11121--+--=i n i n i i i i w w w w P w w w w P 。
英语词典的分类与微观结构
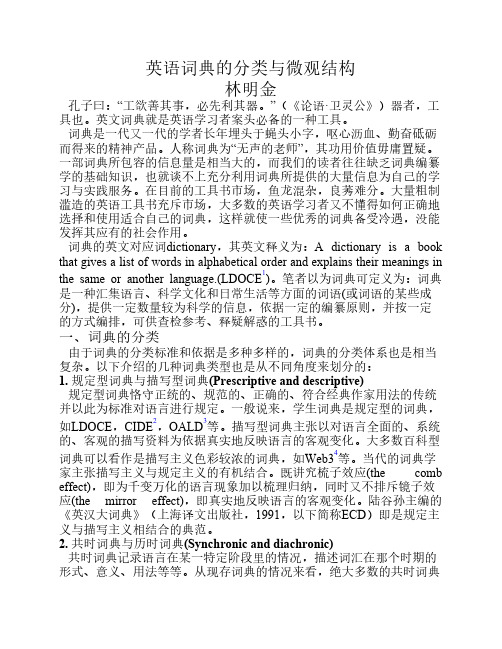
英语词典的分类与微观结构林明金孔子曰:“工欲善其事,必先利其器。
”(《论语·卫灵公》)器者,工具也。
英文词典就是英语学习者案头必备的一种工具。
词典是一代又一代的学者长年埋头于蝇头小字,呕心沥血、勤奋砥砺而得来的精神产品。
人称词典为“无声的老师”,其功用价值毋庸置疑。
一部词典所包容的信息量是相当大的,而我们的读者往往缺乏词典编纂学的基础知识,也就谈不上充分利用词典所提供的大量信息为自己的学习与实践服务。
在目前的工具书市场,鱼龙混杂,良莠难分。
大量粗制滥造的英语工具书充斥市场,大多数的英语学习者又不懂得如何正确地选择和使用适合自己的词典,这样就使一些优秀的词典备受冷遇,没能发挥其应有的社会作用。
词典的英文对应词dictionary,其英文释义为:A dictionary is a book that gives a list of words in alphabetical order and explains their meanings in the same or another language.(LDOCE1)。
笔者以为词典可定义为:词典是一种汇集语言、科学文化和日常生活等方面的词语(或词语的某些成分),提供一定数量较为科学的信息,依据一定的编纂原则,并按一定的方式编排,可供查检参考、释疑解惑的工具书。
一、词典的分类由于词典的分类标准和依据是多种多样的,词典的分类体系也是相当复杂。
以下介绍的几种词典类型也是从不同角度来划分的:1. 规定型词典与描写型词典(Prescriptive and descriptive)规定型词典恪守正统的、规范的、正确的、符合经典作家用法的传统并以此为标准对语言进行规定。
一般说来,学生词典是规定型的词典,如LDOCE,CIDE2,OALD3等。
描写型词典主张以对语言全面的、系统的、客观的描写资料为依据真实地反映语言的客观变化。
大多数百科型词典可以看作是描写主义色彩较浓的词典,如Web34等。
CategoriesorClassification类别或分类
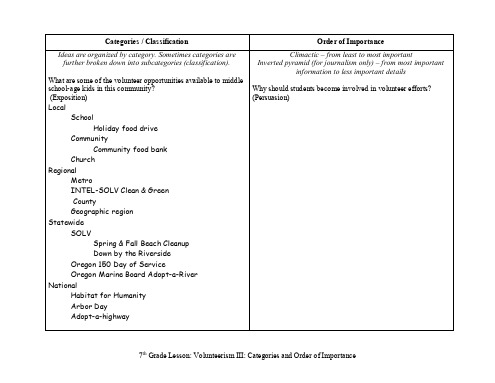
Climactic – from least to most important Inverted pyramid (for journalism only) – from most important
information to less important details
Why should students become involved in volunteer efforts? (Persuasion)
7th Grade Lesson: Volunteerism III: Categories and Order of Importance
information to less important details
Why should students become involved in volunteer efforts? (Persuasion)
Winter School food drive School adopt-a-family
Categories / Classification
Ideas are organized by category. Sometimes categories are further broken down into subcategories (classification).
What are some of the volunteer opportunities available to middle school-age kids in this community? (Exposition) Local
Mentoring Reading buddies for the elementary school
主要分类方法介绍【VIP专享】

分类(Categorization or Classification)就是按照某种标准给对象贴标签(label),再根据标签来区分归类。
分类是事先定义好类别,类别数不变。
分类器需要由人工标注的分类训练语料训练得到,属于有指导学习范畴。
最常用的分类算法就是贝叶斯分类算法,(贝叶斯分类器)用到的知识就是概率的东西不准确的说,就是给它一些已经知道分类的例子作为样本,让它自己“找到”分类标准。
说概念就太抽象,我给你举一些例子吧比如程序是区分大豆和绿豆的。
我们输入的数据是比如颜色值、半径大小,属于黄豆还是绿豆等等(当然这是个简单的例子)。
首先我们需要拿出一些“豆子”的数据给程序,并告诉它是黄豆还是绿豆,然后通过自己的算法,让程序“计算”出区分两种东西的“边界条件”,或者简单说就是提取特征(一般用的比较多的就是距离)。
这就相当于训练/学习等概念。
主要分类方法介绍主要分类方法介绍解决分类问题的方法很多[40-42],单一的分类方法主要包括:决策树、贝叶斯、人工神经网络、K-近邻、支持向量机和基于关联规则的分类等;另外还有用于组合单一分类方法的集成学习算法,如Bagging和Boosting等。
(1)决策树决策树是用于分类和预测的主要技术之一,决策树学习是以实例为基础的归纳学习算法,它着眼于从一组无次序、无规则的实例中推理出以决策树表示的分类规则。
构造决策树的目的是找出属性和类别间的关系,用它来预测将来未知类别的记录的类别。
它采用自顶向下的递归方式,在决策树的内部节点进行属性的比较,并根据不同属性值判断从该节点向下的分支,在决策树的叶节点得到结论。
主要的决策树算法有ID3、C4.5(C5.0)、CART、PUBLIC、SLIQ和SPRINT算法等。
它们在选择测试属性采用的技术、生成的决策树的结构、剪枝的方法以及时刻,能否处理大数据集等方面都有各自的不同之处。
(2)贝叶斯贝叶斯(Bayes)分类算法是一类利用概率统计知识进行分类的算法,如朴素贝叶斯(Naive Bayes)算法。
categorization语言学定义
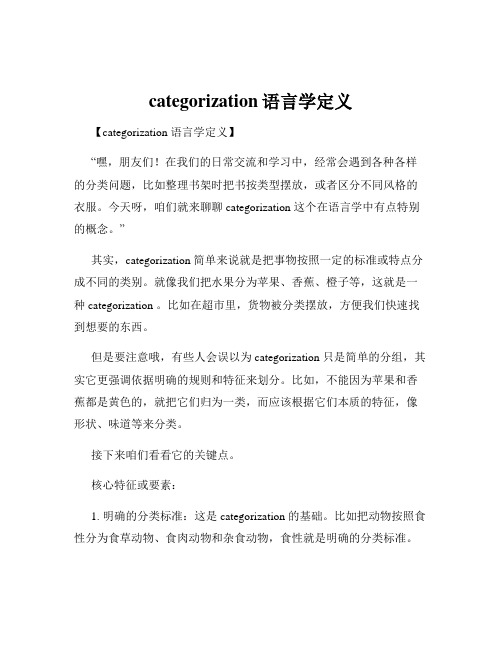
categorization语言学定义【categorization 语言学定义】“嘿,朋友们!在我们的日常交流和学习中,经常会遇到各种各样的分类问题,比如整理书架时把书按类型摆放,或者区分不同风格的衣服。
今天呀,咱们就来聊聊 categorization 这个在语言学中有点特别的概念。
”其实,categorization 简单来说就是把事物按照一定的标准或特点分成不同的类别。
就像我们把水果分为苹果、香蕉、橙子等,这就是一种 categorization 。
比如在超市里,货物被分类摆放,方便我们快速找到想要的东西。
但是要注意哦,有些人会误以为 categorization 只是简单的分组,其实它更强调依据明确的规则和特征来划分。
比如,不能因为苹果和香蕉都是黄色的,就把它们归为一类,而应该根据它们本质的特征,像形状、味道等来分类。
接下来咱们看看它的关键点。
核心特征或要素:1. 明确的分类标准:这是 categorization 的基础。
比如把动物按照食性分为食草动物、食肉动物和杂食动物,食性就是明确的分类标准。
2. 系统性:分类要有条理和逻辑。
像把书籍按照文学、历史、科学等大类,然后再细分,这就是系统性的体现。
3. 可变性:分类不是一成不变的。
随着新事物的出现或者我们对事物认识的深入,分类可能会改变。
比如说以前对于音乐的分类比较简单,现在随着音乐风格的多样化,分类也更加细致和丰富了。
容易混淆的概念:Categorization 和 classification 这两个词很相似,但也有区别。
Categorization 更侧重于基于事物内在的本质特征进行分类,比较细致和深入。
而 classification 则更侧重于从宏观的角度进行大致的分类,相对来说没有那么细致。
比如说在生物学中,对物种的 categorization 会非常详细,考虑到基因、生态等多个方面;而 classification 可能只是简单地分为动物、植物、微生物等大类别。
SCI分类中英文名称翻译

CATEGORIES分类Acoustics[ə'kustɪks]音响学Agricultural Economics & Policy农业经济与政策Agricultural Engineering农业工程Agriculture, Dairy & Animal Science农业、乳制品和动物科学Agriculture,Multidisciplinary[,mʌltɪ'dɪsəplənɛri]农业及相关科学Agronomy[ə'grɑnəmi]农业科学Allergy[ˈælədʒi]变态反应Anatomy & Morphology[ə'nætəmi][mɔr'fɑlədʒi]解剖与形态学Andrology[æn'drɔlədʒi]男科学Anesthesiology[θiː'ɒlədʒɪ]麻醉学Astronomy & Astrophysics[ə'strɑnəmi][ˌæstroʊ'fɪzɪks]天文学和天体物理学Audiology & Speech-LanguagePathology[ˌɔdiˈɑlədʒi][pæˈθɑlədʒi]听力学与言语病理学Automation & Control Systems自动化及控制系统Behavioral Sciences行为科学Biochemical Research Methods生物化学研究方法Biochemistry & Molecular Biology生物化学与分子生物学Biodiversity Conservation[ˌbaɪodɪˈvɚrsɪti]生物多样性保护Biology生物学Biophysics生物物理学Biotechnology & Applied Microbiology生物技术与应用微生物学Cardiac & CardiovascularSystems[ˈkɑrdiˌæk][ˌkɑrdioˈvæskjəlɚ]心脏及心血管系统Cell & Tissue Engineering细胞与组织工程Cell Biology细胞生物学Chemistry, Analytical[ˌænəˈlɪtɪkl]化学分析Chemistry, Applied应用化学Chemistry, Inorganic & Nuclear[ˌɪnɔrˈɡænɪk]无机化学与核化学Chemistry, Medicinal药物化学Chemistry,Multidisciplinary[ˌmʌltiˈdɪsəpləˌnɛri]交叉化学Chemistry, Organic[ɔrˈɡænɪk]有机化学Chemistry, Physical物理化学Clinical Neurology[nʊˈrɑlədʒi]临床神经病学Computer Science, Artificial Intelligence计算机科学与人工智能Computer Science, Cybernetics[,saɪbɚ'nɛtɪks]计算机科学与控制论Computer Science, Hardware &Architecture['ɑrkə'tɛktʃɚ]计算机科学及硬件Computer Science, Information Systems计算机科学与信息系统Computer Science, InterdisciplinaryApplications计算机科学及交叉科学1Computer Science, Software Engineering计算机科学与软件工程Computer Science, Theory & Methods计算机科学理论与方法Construction & Building Technology结构与建筑技术Critical Care Medicine危重症医学Crystallography晶体学Dentistry, Oral Surgery & Medicine牙科,口腔外科与医学Dermatology[,dɝmə'tɑlədʒi]皮肤病学Developmental Biology发育生物学Ecology生态学Education, Scientific Disciplines教育,科学学科Electrochemistry电化学Emergency Medicine急救医学Endocrinology &Metabolism[,ɛndokrə'nɑlədʒi][mɛ'tæbəlɪzəm]内分泌与代谢Energy & Fuels能源与燃料Engineering, Aerospace航空航天工程Engineering, Biomedical生物医学工程Engineering, Chemical化学工程Engineering, Civil['sɪvl]民用工程Engineering, Electrical & Electronic电气与电子工程Engineering, Environmental环境工程Engineering, Geological地质工程Engineering, Industrial工业工程Engineering, Manufacturing制造业工程Engineering, Marine船舶工程Engineering, Mechanical机械工程Engineering, Multidisciplinary多学科工程Engineering, Ocean海洋工程Engineering, Petroleum[pə'trolɪəm]石油工程Entomology[,ɛntə'mɑlədʒi]昆虫学Environmental Sciences环境科学Evolutionary Biology进化生物学Fisheries渔业Food Science & Technology食品科学与技术Forestry林学Gastroenterology & Hepatolog胃肠病学及肝脏病学Genetics & Heredity基因与遗传学Geochemistry & Geophysics地球化学和地球物理学Geography, Physical地理与物理Geology地质学Geosciences, Multidisciplinary多学科性地球科学Geriatrics & Gerontology[,dʒɛrɪ'ætrɪks][,dʒɛrən'tɑlədʒi] 老年病学Health Care Sciences & Services医学保健科学与服务Hematology[,himə'tɑlədʒi]血液学History & Philosophy Of Science历史和科学哲学Horticulture['hɔrtɪ'kʌltʃɚ] 园艺学Imaging Science & Photographic Technology 成像科学与摄影技术Immunology[,ɪmju'nɑlədʒi] 免疫学Infectious Diseases传染病学Instruments & Instrumentation仪器及仪表学Integrative & Complementary Medicine Limnology[lɪm'nɑlədʒi] 湖沼生物学Logic逻辑学Marine & Freshwater Biology海洋和淡水生物学Materials Science, Biomaterials材料科学,生物材料Materials Science, Ceramics[sə'ræmɪks] 材料科学,制陶业Materials Science, Characterization & Testing 材料科学,表征和测试Materials Science, Coatings & Films材料科学,涂料与薄膜Materials Science, Composites[kəm'pɑzɪt]材料科学,复合材料Materials Science, Multidisciplinary材料科学,多学科Materials Science, Paper & Wood材料科学、纸张和木材Materials Science, Textiles['tekstaɪl] 材料科学、纺织品Mathematical & Computational Biology数学与计算生物学Mathematics数学Mathematics, Applied应用数学Mathematics, Interdisciplinary Applications数学,跨学科的应用Mechanics[mə'kænɪks]力学Medical Ethics[‘ɛθɪks]医学伦理学Medical Informatics 医学信息学Medical Laboratory Technology医学实验室技术Medicine, General & Internal普通内科学Medicine, Legal法医学Medicine, Research & Experimental医学研究与实验Metallurgy & MetallurgicalEngineering['mɛtəlɝdʒi]冶金和冶金工程Meteorology & Atmospheric Sciences[,miːtɪə'rɒlədʒɪ] [,ætməs'fɛrɪk] 气象学和大气科学Microbiology微生物学Microscopy显微镜学Mineralogy[,mɪnə'rælədʒi]矿物学Mining & Mineral Processing矿业和矿物加工Multidisciplinary Sciences多学科科学Mycology[maɪ'kɑlədʒi]真菌学Nanoscience & Nanotechnology纳米科技nano['næno]Neuroimaging神经影像Neurosciences神经科学Nuclear Science & Technology核科学与技术Nursing护理Nutrition & Dietetics[daɪə'tetɪks]营养与饮食学Obstetrics & Gynecology[əb'stɛtrɪks][,gaɪnə'kɑlədʒi] 产科学与妇科学Oceanography海洋学Oncology[ɑn'kɑlədʒi]肿瘤学Operations Research & Management Science运筹与管理科学Ophthalmology [,ɑfθæl'mɑlədʒi]眼科学Optics['ɑptɪks]光学Ornithology[,ɔrnɪ'θɑlədʒi]鸟类学Orthopedics[,ɔrθə'pidɪks]矫形术Otorhinolaryngology[,oto,raɪno,lærɪŋ'ɡɑlədʒi]耳鼻喉科学Paleontology[,pelɪɑn'tɑlədʒi]古生物学Parasitology [,pærəsaɪ'tɑlədʒi] 寄生虫学Pathology [pə'θɑlədʒi] 病理学Pediatrics[,pidi'ætrɪks]小儿科Peripheral Vascular Disease[pə'rɪfərəl]['væskjəlɚ]周围性血管疾病Pharmacology & Pharmacy[,fɑrmə'kɑlədʒədʒi] ['fɑrməsi] 药理学Physics, Applied应用物理学Physics, Atomic, Molecular & Chemical物理、原子、分子和化学Physics, Condensed Matter[kən'dɛnst]凝聚态物理Physics, Fluids & Plasmas['plæzmə]流体和等离子体物理Physics, Mathematical数学物理Physics, Multidisciplinary多学科物理Physics, Nuclear核物理Physics, Particles & Fields物理,粒子和场Physiology生理学Plant Sciences植物学Polymer Science['pɑlɪmɚ]高分子科学Primary Health Care初级保健护理Psychiatry[saɪ'kaɪətri]精神病学Psychology心理学Public, Environmental & Occupational Health 公共卫生、环境和职业健康Radiology, Nuclear Medicine & Medical Imaging放射学、核医学和医学成像Rehabilitation [ˌriːəˌbɪlɪ'teʃn]康复Remote Sensing['sensɪŋ]遥感Reproductive Biology[,riprə'dʌktɪv]生殖生物学Respiratory System呼吸系统Rheumatology[,rumə'tɑlədʒi]风湿病学Robotics[ro'bɑtɪks]机器人学Soil Science土壤科学Spectroscopy [spɛk'trɑskəpi] 光谱学Sport Sciences运动医学Statistics & Probability统计及概率学Substance Abuse物质滥用Surgery外科手术Telecommunications电信学Thermodynamics [,θɝmodaɪ'næmɪks] 热力学Toxicology[,tɑksɪ'kɑlədʒi]毒理学Transplantation移植Transportation Science & Technology运输科学与技术Tropical Medicine热带医学Urology & Nephrology [jʊ'rɑlədʒi][nɪ'frɑlədʒi]泌尿及肾内科学Veterinary Sciences['vɛtərənɛri]兽医学Virology [vaɪ'rɑlədʒi]病毒学Water Resources水资源Zoology动物学。
Categories and Categorization类别与分类
– Window size – Aspect ratio – Translation/scale step size – Non-maxima suppression
• Rowley-Baluja-Kanade (2019-2019) : ~2700 citations
– “Parts” at fixed position, non-maxima suppression, simple cascade, rotation, pretty good accuracy, fast
P(parr|otbj)ect
r P(parr|ntonobj)ect
• Estimate P(part|object) and P(part | nonobject) by counting over examples
P(pa|ortbj) eccot u (pna& trotbj)ect cou (onbtj)ect
• Dalal-Triggs (2019) : ~400
– Careful feature engineering, excellent results, HOG feature, online code
• Felzenszwalb-McAllester-Ramanan (2019)? 8 citations
• 17 types of parts • Discretize wavelet coefficient to 3 values • E.g., part with 8 coefficients has 3^8 = 6561
values
对事物分类和划分的英语作文
对事物分类和划分的英语作文英文回答:Categorization and Division of Things.Categorization and division are fundamental cognitive processes that humans use to organize and understand the world around them. Categorization involves grouping similar objects or concepts together, while division involves splitting a larger group into smaller, more specific subsets. Both categorization and division play a crucial role in our ability to make sense of the complex world we live in.One of the most important functions of categorization is that it allows us to quickly and efficiently identify and respond to objects in our environment. When we encounter a new object, our brains automatically compare it to existing categories in our memory. If the object matches a category, we can quickly access the information we havestored about that category and use it to guide our behavior. For example, if we see a dog, we can quickly categorize it as a "dog" and access our knowledge about dogs to know howto interact with it.Division, on the other hand, allows us to break down complex concepts or objects into smaller, more manageable parts. This can help us to understand the structure and organization of things in a more detailed way. For example, if we are studying the human body, we can divide it into smaller parts such as the head, torso, limbs, and organs. This division makes it easier for us to understand the function and interrelationship of the different parts ofthe body.Categorization and division are closely related processes that work together to help us organize and understand the world around us. Categorization allows us to quickly identify and respond to objects, while division allows us to break down complex concepts into smaller, more manageable parts. Both processes are essential for ourability to make sense of the world and to interact with iteffectively.中文回答:事物的分类和划分。
categorize单词拆分记忆
categorize单词拆分记忆一、单词拆分记忆“categorize”可以拆分为“cate - gor - ize”。
“cate”可能让我们联想到“category”(类别),这是这个单词的核心概念;“gor”部分可以当作一个辅助记忆的部分;“ize”是常见的动词后缀,表示“使……化”,所以“categorize”的意思就是“使……归类,将……分类”。
二、单词释义“categorize”是动词,意思是把……分类,归类(将事物、人等按照特定的标准或特征划分到不同的类别中)。
例如:We need to categorize these books according to their genres.(我们需要根据书籍的类型将这些书分类。
)三、单词用法1. 它通常是及物动词,后面直接跟宾语。
例如:The librarian will categorize all the new arrivals.(图书管理员将对所有新到的书籍进行分类。
)2. 可以用于被动语态。
例如:These products are categorized by their functions.(这些产品按照它们的功能被分类。
)四、近义词1. classify:这是和“categorize”最接近的近义词。
例如:Scientists classify animals into different groups.(科学家将动物分类成不同的群组。
)2. group:更强调将事物聚集在一起形成组,虽然有分类的意味,但没有“categorize”那么明确按照特定标准分类。
例如:We group the students by their ages.(我们按照学生的年龄将他们分组。
)3. sort:和“categorize”用法相似,侧重于按照某种顺序或者性质排列。
例如:Sort these papers alphabetically.(按照字母顺序将这些文件分类。
词块的定义及分类探究
国内对词块的研究自 2000 年开始逐渐增多,研究内容主要强调词块在英语教学中的重要性,一般为 思辨研究和经验介绍(濮建忠,2003 [6];刘晓玲,阳志清 2003 [7];常晨光,2004 [8]);自 2005 年以来,
DOI: 10.12677/ml.2021.91034
244
现代语言学
吴小琴
词块的定义及分类探究
吴小琴
江苏开放大学,外国语学院,江苏 南京
收稿日期:2021年1月11日;录用日期:2021年2月16日;发布日期:2021年2月26日
摘要
本文对国内外二十多年的词块定义及分类进行了系统评述,发现学者对于词块的定义未形成统一的说法, 词块的分类标准不一,分类种类繁多。本研究认为,对词块的定义关键在于多维度(形式、功能、意义、 结构等)描述其特点。当前词块分类体系并没有完全将功能和结构完全分开,而在强调某一单项标准时, 又忽略了其他的标准,应该从多个方面,如结构、功能、成分词数、出现频率、开放程度对词块进行分 类。
Bolinger (1976 [4])认为,语法规则固然可以帮助人们表达新的想法和观点,生成前所未有的话语, 但过分强调了语言的规则性。事实上,语言使用者更多时候是使用大量储存在记忆系统里的词块,大多 数交际涉及的都是可预见的生活平常事,人们所使用的语言大多数是在重复自己或别人曾经使用过的, 并非特别具有创造性。比如我们可以说“anyhow”、“anywhere”,却不能说“anywhen”。在跨文化交 际中,经常会有这样的情况发生:中国英语学习者运用语法规则产出一个英语句子,却不能被来自英语 国家的人所接受。在英语这种语言体系里,存在着大量游离于语法规则之外的语言现象。这正是英语学 习者学习的难点所在。纯粹依靠语法规则生成的句子表达却不一定是地道的表达,如何使自己的书面和 口语表达更接近英语本族语使用者的表达习惯,这正是词块可以发挥的作用。
- 1、下载文档前请自行甄别文档内容的完整性,平台不提供额外的编辑、内容补充、找答案等附加服务。
- 2、"仅部分预览"的文档,不可在线预览部分如存在完整性等问题,可反馈申请退款(可完整预览的文档不适用该条件!)。
- 3、如文档侵犯您的权益,请联系客服反馈,我们会尽快为您处理(人工客服工作时间:9:00-18:30)。
A Statistical Method for 3D Object Detection Applied to Faces and Cars
Henry Schneiderman and Takeo Kanade
Rapid Object Detection using a Boosted Cascade of Simple Features
Back to recognition
Cascade for Fast Detection
Examples
Yes Stage 1 H1(x) > t1?
Stage 2 …
H2(x) > t2?
Yes
Stage N
HN(x) > tN?
Pห้องสมุดไป่ตู้ss
No
No
No
Reject
Reject
Reject
• Choose threshold for low false negative rate • Fast classifiers early in cascade • Slow classifiers later, but most examples don’t get
Adaboost as feature selection
• Create a large pool of parts (180K) • “Weak learner” = feature + threshold + parity
• Choose weak learner that minimizes error on the weighted training set
• Schneiderman-Kanade (2019-2000,2019) : ~1150
– Careful feature engineering, excellent results, cascade
• Viola-Jones (2019, 2019) : ~4400
– Haar-like features, Adaboost as feature selection, very fast, easy to implement
Integral Images
• “Haar-like features”
– Differences of sums of intensity – Millions, computed at various positions and scales
within detection window
-1 +1
• How to make it fast • How to deal with different viewpoints • Implementation details
– Window size – Aspect ratio – Translation/scale step size – Non-maxima suppression
Training multiple viewpoints
Train new detector for each viewpoint.
Testing
1) Processing:
a) Lighting correction (optional) b) Compute wavelet coefficients, quantize
• Margin maximization (Schapire et al. 2019)
– Ratch and Warmuth 2019 do this more explicitly
Adaboost: Margin Maximizer
Test error Train error
margin
Interpretations of Adaboost
Goal: Detect all instances of objects
Influential Works in Detection
• Sung-Poggio (1994, 2019) : ~1260
– Basic idea of statistical template detection (I think), bootstrapping to get “face-like” negative examples, multiple whole-face prototypes (in 1994)
• 17 types of parts • Discretize wavelet coefficient to 3 values • E.g., part with 8 coefficients has 3^8 = 6561
values
Part Likelihood
• Class-conditional likelihood ratio
• Adaboost tunes weights discriminatively
Training
1) Create training data
a) Get positive and negative patches b) Pre-process (optional), compute wavelet
2) Slide window over each position/scale (2 pixels, 21/4 scale)
a) Compute part values b) Lookup likelihood ratios c) Sum over parts d) Threshold
3) Use faster classifier to prune patches (cascade)… more on this later
• Rosset Zhu Hastie 2019
– Early stopping is form of L1-regularization
– In many cases, converges to “L1-optimal” separating hyperplane
– “An interesting fundamental similarity between boosting and kernel support vector machines emerges, as both can be described as methods for regularized optimization in high-dimensional predictor space, utilizing a computational trick to make the calculation practical, and converging to margin-maximizing solutions.”
• Reweight
Sidebar: Adaboost
Adaboost
Adaboost
“RealBoost”
Important special case: ht partitions input space:
alphat
Figure from Friedman et al. 2019
Adaboost: Immune to Overfitting?
– Excellent template/parts-based blend
Sliding window detection
What the Detector Sees
Statistical Template
• Object model = log linear model of parts at fixed positions
Test error Train error
Interpretations of Adaboost
• Additive logistic regression (Friedman et al. 2000)
– LogitBoost from Collins et al. 2019 does this more explicitly
coefficients, discretize c) Compute parts values
2) Learn statistics
a) Compute ratios of histograms by counting for positive and negative examples
b) Reweight examples using Adaboost, recount, etc. More on this later
there
Viola-Jones details
• 38 stages with 1, 10, 25, 50 … features
Two-rectangle features
Three-rectangle features
Etc.
Integral Images
• ii = cumsum(cumsum(Im, 1), 2)
x, y ii(x,y) = Sum of the values in the grey region
How to compute B-A? How to compute A+D-B-C?
Paul Viola and Michael Jones
Presenter: Derek Hoiem CS 598, Spring 2009 Feb 24, 2009
Some slides/figures from /~efros/courses/AP06/presentations/Schneiderman-Kanade%20Viola-Jones%20presentation.ppt