计量经济学4答案
计量经济学精要习题参考答案(第四版)
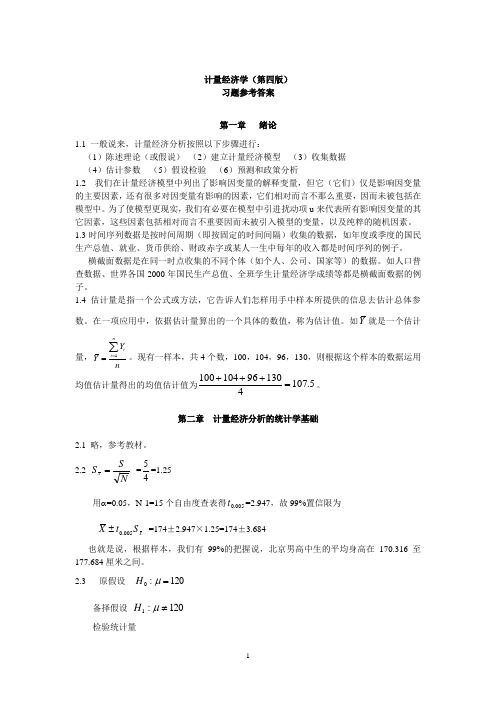
计量经济学(第四版)习题参考答案第一章 绪论1.1 一般说来,计量经济分析按照以下步骤进行:(1)陈述理论(或假说) (2)建立计量经济模型 (3)收集数据 (4)估计参数 (5)假设检验 (6)预测和政策分析1.2 我们在计量经济模型中列出了影响因变量的解释变量,但它(它们)仅是影响因变量的主要因素,还有很多对因变量有影响的因素,它们相对而言不那么重要,因而未被包括在模型中。
为了使模型更现实,我们有必要在模型中引进扰动项u 来代表所有影响因变量的其它因素,这些因素包括相对而言不重要因而未被引入模型的变量,以及纯粹的随机因素。
1.3时间序列数据是按时间周期(即按固定的时间间隔)收集的数据,如年度或季度的国民生产总值、就业、货币供给、财政赤字或某人一生中每年的收入都是时间序列的例子。
横截面数据是在同一时点收集的不同个体(如个人、公司、国家等)的数据。
如人口普查数据、世界各国2000年国民生产总值、全班学生计量经济学成绩等都是横截面数据的例子。
1.4 估计量是指一个公式或方法,它告诉人们怎样用手中样本所提供的信息去估计总体参数。
在一项应用中,依据估计量算出的一个具体的数值,称为估计值。
如Y 就是一个估计量,1nii YY n==∑。
现有一样本,共4个数,100,104,96,130,则根据这个样本的数据运用均值估计量得出的均值估计值为5.107413096104100=+++。
第二章 计量经济分析的统计学基础2.1 略,参考教材。
2.2 NS S x ==45=1.25 用α=0.05,N-1=15个自由度查表得005.0t =2.947,故99%置信限为 x S t X 005.0± =174±2.947×1.25=174±3.684也就是说,根据样本,我们有99%的把握说,北京男高中生的平均身高在170.316至177.684厘米之间。
2.3 原假设 120:0=μH备择假设 120:1≠μH 检验统计量()10/25XX μσ-Z ====查表96.1025.0=Z 因为Z= 5 >96.1025.0=Z ,故拒绝原假设, 即此样本不是取自一个均值为120元、标准差为10元的正态总体。
伍德里奇-计量经济学(第4版)答案
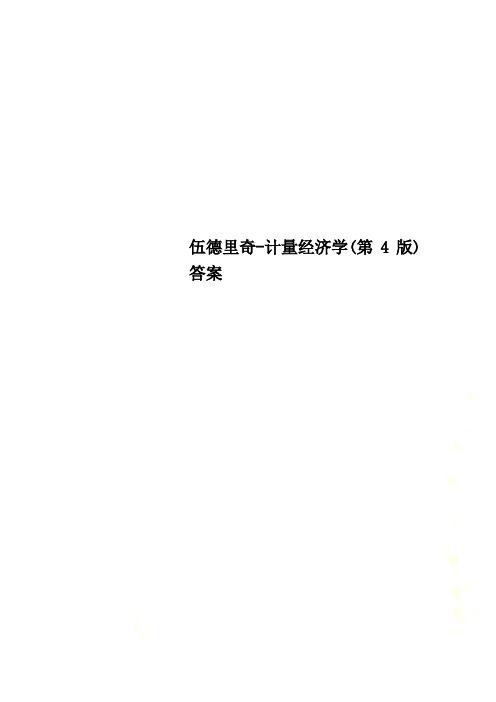
伍德里奇-计量经济学(第4版)答案计量经济学答案第二章2.4 (1)在实验的准备过程中,我们要随机安排小时数,这样小时数(hours )可以独立于其它影响SAT 成绩的因素。
然后,我们收集实验中每个学生SAT 成绩的相关信息,产生一个数据集{}n i hours sat i i ,...2,1:),(=,n 是实验中学生的数量。
从式(2.7)中,我们应尽量获得较多可行的i hours 变量。
(2)因素:与生俱来的能力(天赋)、家庭收入、考试当天的健康状况①如果我们认为天赋高的学生不需要准备SAT 考试,那天赋(ability )与小时数(hours )之间是负相关。
②家庭收入与小时数之间可能是正相关,因为收入水平高的家庭更容易支付起备考课程的费用。
③排除慢性健康问题,考试当天的健康问题与SAT 备考课程上的小时数(hours )大致不相关。
(3)如果备考课程有效,1β应该是正的:其他因素不变情况下,增加备考课程时间会提高SAT 成绩。
(4)0β在这个例子中有一个很有用的解释:因为E (u )=0,0β是那些在备考课程上花费小时数为0的学生的SAT平均成绩。
2.7(1)是的。
如果住房离垃圾焚化炉很近会压低房屋的价格,如果住房离垃圾焚化炉距离远则房屋的价格会高。
(2)如果城市选择将垃圾焚化炉放置在距离昂贵的街区较远的地方,那么log(dist)与房屋价格就是正相关的。
也就是说方程中u包含的因素(例如焚化炉的地理位置等)和距离(dist)相关,则E(u︱log(dist))≠0。
这就违背SLR4(零条件均值假设),而且最小二乘法估计可能有偏。
(3)房屋面积,浴室的数量,地段大小,屋龄,社区的质量(包括学校的质量)等因素,正如第(2)问所提到的,这些因素都与距离焚化炉的远近(dist,log(dist))相关2.11(1)当cigs(孕妇每天抽烟根数)=0时,预计婴儿出生体重=110.77盎司;当cigs(孕妇每天抽烟根数)=20时,预计婴儿出生体重(bwght)=109.49盎司。
计量经济学精要习题参考答案(第四版)
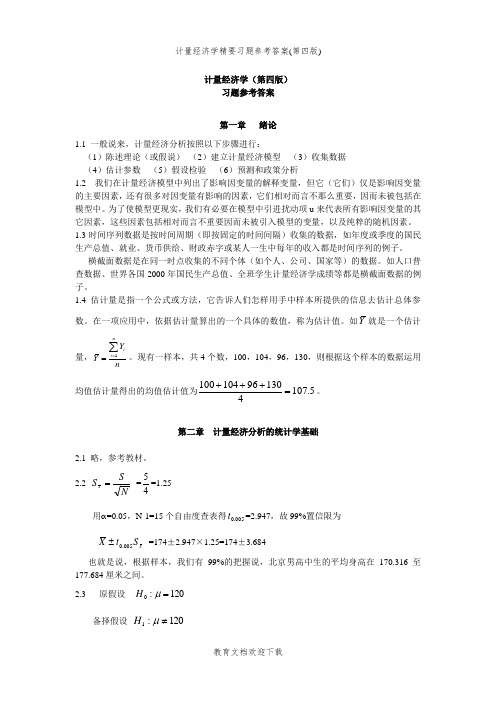
计量经济学(第四版)习题参考答案第一章 绪论1.1 一般说来,计量经济分析按照以下步骤进行:(1)陈述理论(或假说) (2)建立计量经济模型 (3)收集数据 (4)估计参数 (5)假设检验 (6)预测和政策分析1.2 我们在计量经济模型中列出了影响因变量的解释变量,但它(它们)仅是影响因变量的主要因素,还有很多对因变量有影响的因素,它们相对而言不那么重要,因而未被包括在模型中。
为了使模型更现实,我们有必要在模型中引进扰动项u 来代表所有影响因变量的其它因素,这些因素包括相对而言不重要因而未被引入模型的变量,以及纯粹的随机因素。
1.3时间序列数据是按时间周期(即按固定的时间间隔)收集的数据,如年度或季度的国民生产总值、就业、货币供给、财政赤字或某人一生中每年的收入都是时间序列的例子。
横截面数据是在同一时点收集的不同个体(如个人、公司、国家等)的数据。
如人口普查数据、世界各国2000年国民生产总值、全班学生计量经济学成绩等都是横截面数据的例子。
1.4 估计量是指一个公式或方法,它告诉人们怎样用手中样本所提供的信息去估计总体参数。
在一项应用中,依据估计量算出的一个具体的数值,称为估计值。
如Y 就是一个估计量,1nii YY n==∑。
现有一样本,共4个数,100,104,96,130,则根据这个样本的数据运用均值估计量得出的均值估计值为5.107413096104100=+++。
第二章 计量经济分析的统计学基础2.1 略,参考教材。
2.2 NS S x ==45=1.25 用α=0.05,N-1=15个自由度查表得005.0t =2.947,故99%置信限为 x S t X 005.0± =174±2.947×1.25=174±3.684也就是说,根据样本,我们有99%的把握说,北京男高中生的平均身高在170.316至177.684厘米之间。
2.3 原假设 120:0=μH备择假设 120:1≠μH检验统计量()10/25XX μσ-Z ====查表96.1025.0=Z 因为Z= 5 >96.1025.0=Z ,故拒绝原假设, 即此样本不是取自一个均值为120元、标准差为10元的正态总体。
计量经济学4-7章单选、多选题带答案

D
)
5、在以下选项中,正确表达了序列自相关的是(
A.Cov(ui , u j ) 0, i j C.Cov( xi , x j ) 0, i j
A.无偏的,非有效的. C.无偏的,有效的
) A B.Cov(u i , u j ) 0, i j
D.Cov( xi , u j ) 0, i j
自相关性 产生的后果:
Cov(ut , u s ) 0
ts
1、参数估计量无偏,但不满足有效性;
ˆ 2、 2严重低估计总体方差 2
3、参数的显著性检验(t 检验)失效; 4、区间估计和预测区间的精度降低
检验方法: 补救措施: 1、广义差分法
1、图示法;
2、D-W检验
2、一阶差分估计法
3、科克兰内-奥克特法(Cochrane-Orcutt)迭方法
X j f ( X 2 ,, X j 1 , X j 1 ,, X k )
R2 j
补救措施:
1、增加样本容量; 3、数据的结合; 2、利用先验信息改变参数的约束条件 4、利用差分方程(变换模型的形式)
5、逐步回归法
异方差(方差非齐性). 产生的后果:
Var (ui ) i2 2 f ( X )
A. 经济变量的惯性作用 C. 设定偏误 B. 经济行为的滞后作用 D. 解释变量的共线性.
44、
yi 1 2 xi ui ,Var(ui ) f ( xi )
2 i 2
则对原模型变换的正确形式为(
B ) P99
xi ui 1 B. 2 f ( xi ) f ( xi ) f ( xi ) f ( xi ) yi D. yi f ( xi ) 1 f ( xi ) 2 xi f ( xi ) ui f ( xi )
计量经济学(第四版)习题及参考答案详细版知识讲解
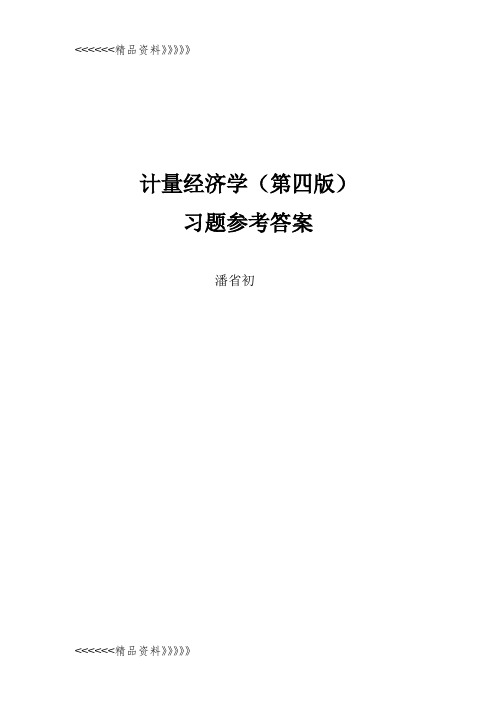
计量经济学(第四版)习题参考答案潘省初第一章 绪论1.1 试列出计量经济分析的主要步骤。
一般说来,计量经济分析按照以下步骤进行:(1)陈述理论(或假说) (2)建立计量经济模型 (3)收集数据 (4)估计参数 (5)假设检验 (6)预测和政策分析 1.2 计量经济模型中为何要包括扰动项?为了使模型更现实,我们有必要在模型中引进扰动项u 来代表所有影响因变量的其它因素,这些因素包括相对而言不重要因而未被引入模型的变量,以及纯粹的随机因素。
1.3什么是时间序列和横截面数据? 试举例说明二者的区别。
时间序列数据是按时间周期(即按固定的时间间隔)收集的数据,如年度或季度的国民生产总值、就业、货币供给、财政赤字或某人一生中每年的收入都是时间序列的例子。
横截面数据是在同一时点收集的不同个体(如个人、公司、国家等)的数据。
如人口普查数据、世界各国2000年国民生产总值、全班学生计量经济学成绩等都是横截面数据的例子。
1.4估计量和估计值有何区别?估计量是指一个公式或方法,它告诉人们怎样用手中样本所提供的信息去估计总体参数。
在一项应用中,依据估计量算出的一个具体的数值,称为估计值。
如Y就是一个估计量,1nii YY n==∑。
现有一样本,共4个数,100,104,96,130,则根据这个样本的数据运用均值估计量得出的均值估计值为5.107413096104100=+++。
第二章 计量经济分析的统计学基础2.1 略,参考教材。
2.2请用例2.2中的数据求北京男生平均身高的99%置信区间NSS x ==45=1.25 用α=0.05,N-1=15个自由度查表得005.0t =2.947,故99%置信限为 x S t X 005.0± =174±2.947×1.25=174±3.684也就是说,根据样本,我们有99%的把握说,北京男高中生的平均身高在170.316至177.684厘米之间。
计量经济学课后答案第四、五章(内容参考)
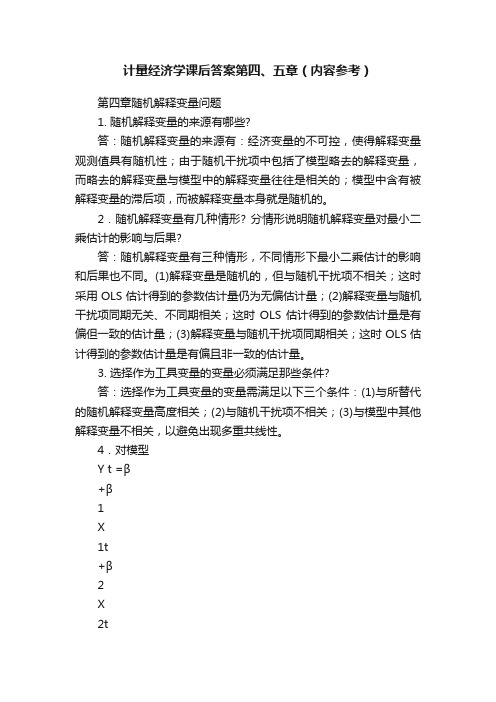
计量经济学课后答案第四、五章(内容参考)第四章随机解释变量问题1. 随机解释变量的来源有哪些?答:随机解释变量的来源有:经济变量的不可控,使得解释变量观测值具有随机性;由于随机干扰项中包括了模型略去的解释变量,而略去的解释变量与模型中的解释变量往往是相关的;模型中含有被解释变量的滞后项,而被解释变量本身就是随机的。
2.随机解释变量有几种情形? 分情形说明随机解释变量对最小二乘估计的影响与后果?答:随机解释变量有三种情形,不同情形下最小二乘估计的影响和后果也不同。
(1)解释变量是随机的,但与随机干扰项不相关;这时采用OLS估计得到的参数估计量仍为无偏估计量;(2)解释变量与随机干扰项同期无关、不同期相关;这时OLS估计得到的参数估计量是有偏但一致的估计量;(3)解释变量与随机干扰项同期相关;这时OLS估计得到的参数估计量是有偏且非一致的估计量。
3. 选择作为工具变量的变量必须满足那些条件?答:选择作为工具变量的变量需满足以下三个条件:(1)与所替代的随机解释变量高度相关;(2)与随机干扰项不相关;(3)与模型中其他解释变量不相关,以避免出现多重共线性。
4.对模型Y t =β+β1X1t+β2X2t+β3Yt-1+μt假设Yt-1与μt相关。
为了消除该相关性,采用工具变量法:先求Y t关于X1t与 X2t回归,得到Yt,再做如下回归:Y t =β+β1X1t+β2X2t+β3Y t?1-+μt试问:这一方法能否消除原模型中Yt的相关性? 为什么?解答:能消除。
在基本假设下,X1t,X2t与μt应是不相关的,由此知,由X1t 与X2t估计出的Yt应与μt不相关。
5.对于一元回归模型Y t =β+β1Xt*+μt假设解释变量Xt *的实测值Xt与之有偏误:Xt= Xt*+et,其中et是具有零均值、无序列相关,且与Xt不相关的随机变量。
试问:(1) 能否将X t= X t*+e t代入原模型,使之变换成Y t=β0+β1X t+νt后进行估计? 其中,νt为变换后模型的随机干扰项。
计量经济学第4章课后答案

17CHAPTER 4SOLUTIONS TO PROBLEMS4.2 (i) and (iii) generally cause the t statistics not to have a t distribution under H 0.Homoskedasticity is one of the CLM assumptions. An important omitted variable violates Assumption MLR.3. The CLM assumptions contain no mention of the sample correlations among independent variables, except to rule out the case where the correlation is one.4.3 (i) While the standard error on hrsemp has not changed, the magnitude of the coefficient has increased by half. The t statistic on hrsemp has gone from about –1.47 to –2.21, so now the coefficient is statistically less than zero at the 5% level. (From Table G.2 the 5% critical value with 40 df is –1.684. The 1% critical value is –2.423, so the p -value is between .01 and .05.)(ii) If we add and subtract 2βlog(employ ) from the right-hand-side and collect terms, we havelog(scrap ) = 0β + 1βhrsemp + [2βlog(sales) – 2βlog(employ )] + [2βlog(employ ) + 3βlog(employ )] + u = 0β + 1βhrsemp + 2βlog(sales /employ ) + (2β + 3β)log(employ ) + u ,where the second equality follows from the fact that log(sales /employ ) = log(sales ) – log(employ ). Defining 3θ ≡ 2β + 3β gives the result.(iii) No. We are interested in the coefficient on log(employ ), which has a t statistic of .2, which is very small. Therefore, we conclude that the size of the firm, as measured by employees, does not matter, once we control for training and sales per employee (in a logarithmic functional form).(iv) The null hypothesis in the model from part (ii) is H 0:2β = –1. The t statistic is [–.951 – (–1)]/.37 = (1 – .951)/.37 ≈ .132; this is very small, and we fail to reject whether we specify a one- or two-sided alternative.4.4 (i) In columns (2) and (3), the coefficient on profmarg is actually negative, although its t statistic is only about –1. It appears that, once firm sales and market value have been controlled for, profit margin has no effect on CEO salary.(ii) We use column (3), which controls for the most factors affecting salary. The t statistic on log(mktval ) is about 2.05, which is just significant at the 5% level against a two-sided alternative.18(We can use the standard normal critical value, 1.96.) So log(mktval ) is statistically significant. Because the coefficient is an elasticity, a ceteris paribus 10% increase in market value is predicted to increase salary by 1%. This is not a huge effect, but it is not negligible, either.(iii) These variables are individually significant at low significance levels, with t ceoten ≈ 3.11 and t comten ≈ –2.79. Other factors fixed, another year as CEO with the company increases salary by about 1.71%. On the other hand, another year with the company, but not as CEO, lowers salary by about .92%. This second finding at first seems surprising, but could be related to the “superstar” effect: firms that hire CEOs from outside the company often go after a small pool of highly regarded candidates, and salaries of these people are bid up. More non-CEO years with a company makes it less likely the person was hired as an outside superstar.4.7 (i) .412 ± 1.96(.094), or about .228 to .596.(ii) No, because the value .4 is well inside the 95% CI.(iii) Yes, because 1 is well outside the 95% CI.4.8 (i) With df = 706 – 4 = 702, we use the standard normal critical value (df = ∞ in Table G.2), which is 1.96 for a two-tailed test at the 5% level. Now t educ = −11.13/5.88 ≈ −1.89, so |t educ | = 1.89 < 1.96, and we fail to reject H 0: educ β = 0 at the 5% level. Also, t age ≈ 1.52, so age is also statistically insignificant at the 5% level.(ii) We need to compute the R -squared form of the F statistic for joint significance. But F = [(.113 − .103)/(1 − .113)](702/2) ≈ 3.96. The 5% critical value in the F 2,702 distribution can be obtained from Table G.3b with denominator df = ∞: cv = 3.00. Therefore, educ and age are jointly significant at the 5% level (3.96 > 3.00). In fact, the p -value is about .019, and so educ and age are jointly significant at the 2% level.(iii) Not really. These variables are jointly significant, but including them only changes the coefficient on totwrk from –.151 to –.148.(iv) The standard t and F statistics that we used assume homoskedasticity, in addition to the other CLM assumptions. If there is heteroskedasticity in the equation, the tests are no longer valid.4.11 (i) Holding profmarg fixed, n rdintensΔ = .321 Δlog(sales ) = (.321/100)[100log()sales ⋅Δ] ≈ .00321(%Δsales ). Therefore, if %Δsales = 10, n rdintens Δ ≈ .032, or only about 3/100 of a percentage point. For such a large percentage increase in sales,this seems like a practically small effect.(ii) H 0:1β = 0 versus H 1:1β > 0, where 1β is the population slope on log(sales ). The t statistic is .321/.216 ≈ 1.486. The 5% critical value for a one-tailed test, with df = 32 – 3 = 29, is obtained from Table G.2 as 1.699; so we cannot reject H 0 at the 5% level. But the 10% criticalvalue is 1.311; since the t statistic is above this value, we reject H0 in favor of H1 at the 10% level.(iii) Not really. Its t statistic is only 1.087, which is well below even the 10% critical value for a one-tailed test.1920SOLUTIONS TO COMPUTER EXERCISESC4.1 (i) Holding other factors fixed,111log()(/100)[100log()](/100)(%),voteA expendA expendA expendA βββΔ=Δ=⋅Δ≈Δwhere we use the fact that 100log()expendA ⋅Δ ≈ %expendA Δ. So 1β/100 is the (ceteris paribus) percentage point change in voteA when expendA increases by one percent.(ii) The null hypothesis is H 0: 2β = –1β, which means a z% increase in expenditure by A and a z% increase in expenditure by B leaves voteA unchanged. We can equivalently write H 0: 1β + 2β = 0.(iii) The estimated equation (with standard errors in parentheses below estimates) isn voteA = 45.08 + 6.083 log(expendA ) – 6.615 log(expendB ) + .152 prtystrA(3.93) (0.382) (0.379) (.062) n = 173, R 2 = .793.The coefficient on log(expendA ) is very significant (t statistic ≈ 15.92), as is the coefficient on log(expendB ) (t statistic ≈ –17.45). The estimates imply that a 10% ceteris paribus increase in spending by candidate A increases the predicted share of the vote going to A by about .61percentage points. [Recall that, holding other factors fixed, n voteAΔ≈(6.083/100)%ΔexpendA ).] Similarly, a 10% ceteris paribus increase in spending by B reduces n voteAby about .66 percentage points. These effects certainly cannot be ignored.While the coefficients on log(expendA ) and log(expendB ) are of similar magnitudes (andopposite in sign, as we expect), we do not have the standard error of 1ˆβ + 2ˆβ, which is what we would need to test the hypothesis from part (ii).(iv) Write 1θ = 1β +2β, or 1β = 1θ– 2β. Plugging this into the original equation, and rearranging, givesn voteA = 0β + 1θlog(expendA ) + 2β[log(expendB ) – log(expendA )] +3βprtystrA + u ,When we estimate this equation we obtain 1θ≈ –.532 and se( 1θ)≈ .533. The t statistic for the hypothesis in part (ii) is –.532/.533 ≈ –1. Therefore, we fail to reject H 0: 2β = –1β.21C4.3 (i) The estimated model isn log()price = 11.67 + .000379 sqrft + .0289 bdrms (0.10) (.000043) (.0296)n = 88, R 2 = .588.Therefore, 1ˆθ= 150(.000379) + .0289 = .0858, which means that an additional 150 square foot bedroom increases the predicted price by about 8.6%.(ii) 2β= 1θ – 1501β, and solog(price ) = 0β+ 1βsqrft + (1θ – 1501β)bdrms + u= 0β+ 1β(sqrft – 150 bdrms ) + 1θbdrms + u .(iii) From part (ii), we run the regressionlog(price ) on (sqrft – 150 bdrms ), bdrms ,and obtain the standard error on bdrms . We already know that 1ˆθ= .0858; now we also getse(1ˆθ) = .0268. The 95% confidence interval reported by my software package is .0326 to .1390(or about 3.3% to 13.9%).C4.5 (i) If we drop rbisyr the estimated equation becomesn log()salary = 11.02 + .0677 years + .0158 gamesyr (0.27) (.0121) (.0016)+ .0014 bavg + .0359 hrunsyr (.0011) (.0072)n = 353, R 2= .625.Now hrunsyr is very statistically significant (t statistic ≈ 4.99), and its coefficient has increased by about two and one-half times.(ii) The equation with runsyr , fldperc , and sbasesyr added is22n log()salary = 10.41 + .0700 years + .0079 gamesyr(2.00) (.0120) (.0027)+ .00053 bavg + .0232 hrunsyr (.00110) (.0086)+ .0174 runsyr + .0010 fldperc – .0064 sbasesyr (.0051) (.0020) (.0052) n = 353, R 2 = .639.Of the three additional independent variables, only runsyr is statistically significant (t statistic = .0174/.0051 ≈ 3.41). The estimate implies that one more run per year, other factors fixed,increases predicted salary by about 1.74%, a substantial increase. The stolen bases variable even has the “wrong” sign with a t statistic of about –1.23, while fldperc has a t statistic of only .5. Most major league baseball players are pretty good fielders; in fact, the smallest fldperc is 800 (which means .800). With relatively little variation in fldperc , it is perhaps not surprising that its effect is hard to estimate.(iii) From their t statistics, bavg , fldperc , and sbasesyr are individually insignificant. The F statistic for their joint significance (with 3 and 345 df ) is about .69 with p -value ≈ .56. Therefore, these variables are jointly very insignificant.C4.7 (i) The minimum value is 0, the maximum is 99, and the average is about 56.16. (ii) When phsrank is added to (4.26), we get the following:n log() wage = 1.459 − .0093 jc + .0755 totcoll + .0049 exper + .00030 phsrank (0.024) (.0070) (.0026) (.0002) (.00024)n = 6,763, R 2 = .223So phsrank has a t statistic equal to only 1.25; it is not statistically significant. If we increase phsrank by 10, log(wage ) is predicted to increase by (.0003)10 = .003. This implies a .3% increase in wage , which seems a modest increase given a 10 percentage point increase in phsrank . (However, the sample standard deviation of phsrank is about 24.)(iii) Adding phsrank makes the t statistic on jc even smaller in absolute value, about 1.33, but the coefficient magnitude is similar to (4.26). Therefore, the base point remains unchanged: the return to a junior college is estimated to be somewhat smaller, but the difference is not significant and standard significant levels.(iv) The variable id is just a worker identification number, which should be randomly assigned (at least roughly). Therefore, id should not be correlated with any variable in the regression equation. It should be insignificant when added to (4.17) or (4.26). In fact, its t statistic is about .54.23C4.9 (i) The results from the OLS regression, with standard errors in parentheses, aren log() psoda =−1.46 + .073 prpblck + .137 log(income ) + .380 prppov (0.29) (.031) (.027) (.133)n = 401, R 2 = .087The p -value for testing H 0: 10β= against the two-sided alternative is about .018, so that we reject H 0 at the 5% level but not at the 1% level.(ii) The correlation is about −.84, indicating a strong degree of multicollinearity. Yet eachcoefficient is very statistically significant: the t statistic for log()ˆincome β is about 5.1 and that forˆprppovβ is about 2.86 (two-sided p -value = .004).(iii) The OLS regression results when log(hseval ) is added aren log() psoda =−.84 + .098 prpblck − .053 log(income ) (.29) (.029) (.038) + .052 prppov + .121 log(hseval ) (.134) (.018)n = 401, R 2 = .184The coefficient on log(hseval ) is an elasticity: a one percent increase in housing value, holding the other variables fixed, increases the predicted price by about .12 percent. The two-sided p -value is zero to three decimal places.(iv) Adding log(hseval ) makes log(income ) and prppov individually insignificant (at even the 15% significance level against a two-sided alternative for log(income ), and prppov is does not have a t statistic even close to one in absolute value). Nevertheless, they are jointly significant at the 5% level because the outcome of the F 2,396 statistic is about 3.52 with p -value = .030. All of the control variables – log(income ), prppov , and log(hseval ) – are highly correlated, so it is not surprising that some are individually insignificant.(v) Because the regression in (iii) contains the most controls, log(hseval ) is individually significant, and log(income ) and prppov are jointly significant, (iii) seems the most reliable. It holds fixed three measure of income and affluence. Therefore, a reasonable estimate is that if the proportion of blacks increases by .10, psoda is estimated to increase by 1%, other factors held fixed.。
计量经济学第四版习题及参考答案解析
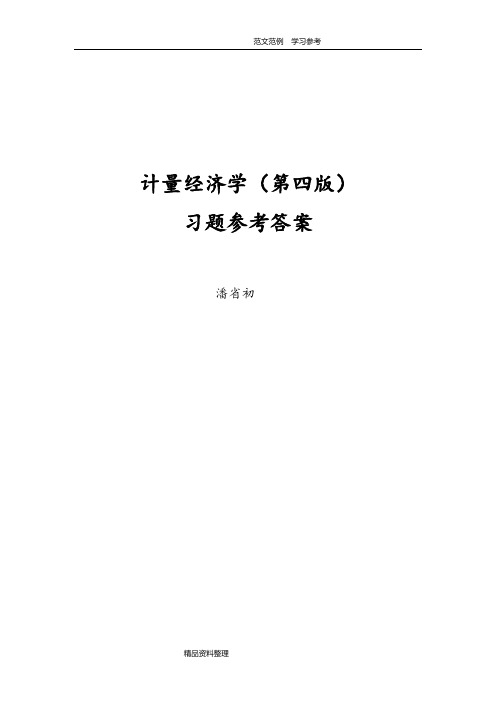
计量经济学(第四版)习题参考答案潘省初第一章 绪论1.1 试列出计量经济分析的主要步骤。
一般说来,计量经济分析按照以下步骤进行:(1)陈述理论(或假说) (2)建立计量经济模型 (3)收集数据 (4)估计参数 (5)假设检验 (6)预测和政策分析 1.2 计量经济模型中为何要包括扰动项?为了使模型更现实,我们有必要在模型中引进扰动项u 来代表所有影响因变量的其它因素,这些因素包括相对而言不重要因而未被引入模型的变量,以及纯粹的随机因素。
1.3什么是时间序列和横截面数据? 试举例说明二者的区别。
时间序列数据是按时间周期(即按固定的时间间隔)收集的数据,如年度或季度的国民生产总值、就业、货币供给、财政赤字或某人一生中每年的收入都是时间序列的例子。
横截面数据是在同一时点收集的不同个体(如个人、公司、国家等)的数据。
如人口普查数据、世界各国2000年国民生产总值、全班学生计量经济学成绩等都是横截面数据的例子。
1.4估计量和估计值有何区别?估计量是指一个公式或方法,它告诉人们怎样用手中样本所提供的信息去估计总体参数。
在一项应用中,依据估计量算出的一个具体的数值,称为估计值。
如Y就是一个估计量,1nii YY n==∑。
现有一样本,共4个数,100,104,96,130,则根据这个样本的数据运用均值估计量得出的均值估计值为5.107413096104100=+++。
第二章 计量经济分析的统计学基础2.1 略,参考教材。
2.2请用例2.2中的数据求北京男生平均身高的99%置信区间NS S x ==45=1.25 用α=0.05,N-1=15个自由度查表得005.0t =2.947,故99%置信限为 x S t X 005.0± =174±2.947×1.25=174±3.684也就是说,根据样本,我们有99%的把握说,北京男高中生的平均身高在170.316至177.684厘米之间。
- 1、下载文档前请自行甄别文档内容的完整性,平台不提供额外的编辑、内容补充、找答案等附加服务。
- 2、"仅部分预览"的文档,不可在线预览部分如存在完整性等问题,可反馈申请退款(可完整预览的文档不适用该条件!)。
- 3、如文档侵犯您的权益,请联系客服反馈,我们会尽快为您处理(人工客服工作时间:9:00-18:30)。
第四章 多重共线性
一、单项选择题
1、完全的多重共线性是指解释变量的数据矩阵的秩( B )
(A )大于k+1 (B )小于k+1 (C )等于k+1 (D )等于k+1
2、当模型存在严重的多重共线性时,OLS 估计量将不具备( D )
(A )线性 (B )无偏性 (C )有效性 (D )一致性
3、如果每两个解释变量的简单相关系数比较高,大于( D )时则可认为存在着较严重的多重共线性。
(A )0.5 (B )0.6 (C )0.7 (D )0.8
4、方差扩大因子VIF j 可用来度量多重共线性的严重程度,经验表明,VIF j ( A )时,说明解释变量与其余解释变量间有严重的多重共线性。
(A )小于5 (B )大于1 (C )小于1 (D )大于10
5、对于模型01122i i i i y x x u βββ=+++,与r 23等于0相比,当r 23等于0.5时,3
ˆβ的方差将是原来的(C ) (A )2倍 (B )1.5倍 (C )1.33倍 (D )1.25倍
6、无多重共线性是指数据矩阵的秩( D )
(A )小于k (B )等于k (C )大于k (D )等于k+1
7、无多重共线性假定是假定各解释变量之间不存在( A )
(A )线性关系 (B )非线性关系 (C )自相关 (D )异方差
8、经济变量之间具有共同变化的趋势时,由其构建的计量经济模型易产生( C )
(A )异方差 (B )自相关
(C )多重共线性 (D )序列相关
9、完全多重共线性产生的后果包括参数估计量的方差( C )
(A )增大 (B )减小
(C )无穷大 (D )无穷小
10、不完全多重共线性产生的后果包括参数估计量的方差( A )
(A )增大 (B )减小
(C )无穷大 (D )无穷小
11、不完全多重共线性下,对参数区间估计时,置信区间趋于( A )
(A )变大 (B )变小
(C )不变 (D )难以估计
12、较高的简单相关系数是多重共线性存在的( B )
(A )必要条件 (B )充分条件
(C )充要条件 (D )并非条件
13、方差扩大因子VIF j 是由辅助回归的可决系数R j 2计算而得,R j 2越大,方差扩大因子VIF j 就( A )
(A )越大 (B )越小
(C )不变 (D )无关
14、解释变量间的多重共线性越弱,方差扩大因子VIF j 就越接近于( A )
(A )1 (B )2
(C )0 (D )10
15、多重共线性是一个(D )
(A )样本特性 (B )总体特性
(C )模型特性 (D )以上皆不对
二、多项选择题
1、多重共线性包括(ABCD )
(A )完全的多重共线性 (B )不完全的多重共线性
(C)解释变量间精确的线性关系(D)解释变量间近似的线性关系
(E)非线性关系
2、多重共线性产生的经济背景主要由(ABD )
(A)经济变量之间具有共同变化趋势(B)模型中包含滞后变量
(C)采用截面数据(D)样本数据自身的原因
3、多重共线性检验的方法包括(ABCD )
(A)简单相关系数检验法(B)方差扩大因子法
(C)直观判断法(D)逐步回归法
(E)DW检验法
4、修正多重共线性的经验方法包括(ABCDE )
(A)剔除变量法(B)增大样本容量
(C)变换模型形式(D)截面数据与时间序列数据并用
(E)变量变换
5、严重的多重共线性常常会出现下列情形(ABCD )
(A)适用OLS得到的回归参数估计值不稳定
(B)回归系数的方差增大
(C)回归方程高度显著的情况下,有些回归系数通不过显著性检验
(D)回归系数的正负号得不到合理的经济解释
三、名词解释(每题4分)
1、多重共线性
2、完全的多重共线性
3、辅助回归
4、方差扩大因子VIF j
5、逐步回归法
6、不完全的多重共线性
四、简答题(每题5分)
1、多重共线性的实质是什么?
2、为什么会出现多重共线性?
3、多重共线性对回归参数的估计有何影响?
4、判断是否存在多重共线性的方法有那些?
5、针对多重共线性采取的补救措施有那些?
6、具有严重多重共线性的回归方程能否用来进行预测?
五、辨析题
1、在高度多重共线性的情形中,要评价一个或多个偏回归系数的单个显著性是不可能的。
×
2、尽管有完全的多重共线性,OLS估计量仍然是BLUE。
√
3、如果其他条件不变,VIF越高,OLS估计量的方差越大。
√
4、如果在多元回归中,根据通常的t检验,全部偏回归系数都是统计上不显著的,你就不会得到一个高的R2值。
×
5、如果分析的目的仅仅是预测,则多重共线性是无害的。
√
6、如果有某一辅助回归显示出高的R j2值,则高度共线性的存在是肯定无疑的。
×
六、计算分析题
1、克莱因与戈德伯格曾用1921-1950年(1942-1944年战争期间略去)美国国内消费Y和工资收入X1、非工资—非农业收入X
2、农业收入X3的时间序列资料,利用OLSE估计得出了下列回归方程:
37
.
107 95
.0(1.09)
(0.66)
(0.17)
(8.92)
3
121
.0
2
452
.0
1
059
.1
133
.8
ˆ
2=
=+
+
+
=
F
R
X X
X Y
(括号中的数据为相应参数估计量的标准误)。
试对上述模型进行评析,指出其中存在的问题。
从模型拟合结果可知,样本观测个数为27,消费模型的判定系数95.02
=R ,F 统计量为107.37,在0.05置信水平下查分子自由度为3,分母自由度为23的F 临界值为3.028,计算的F 值远大于临界值,表明回归方程是显著的。
模型整体拟合程度较高。
依据参数估计量及其标准误,可计算出各回归系数估计量的t 统计量值: 11.009.1121.0,69.066.0452.0,10.617.0059.1,91.092.8133.83210========
t t t t
除1t 外,其余的j t 值都很小。
工资收入X1的系数的t 检验值虽然显著,但该系数的估计值过大,该值为工资收入对消费边际效应,因为它为1.059,意味着工资收入每增加一美元,消费支出的增长平均将超过一美元,这与经济理论和常识不符。
另外,理论上非工资—非农业收入与农业收入也是消费行为的重要解释变量,但两者的t 检验都没有通过。
这些迹象表明,模型中存在严重的多重共线性,不同收入部分之间的相互关系,掩盖了各个部分对解释消费行为的单独影响。