基于matlab的车牌定位源程序及运行结果 (1)
基于MATLAB的车牌定位算法设计 电子信息工程毕业设计论文
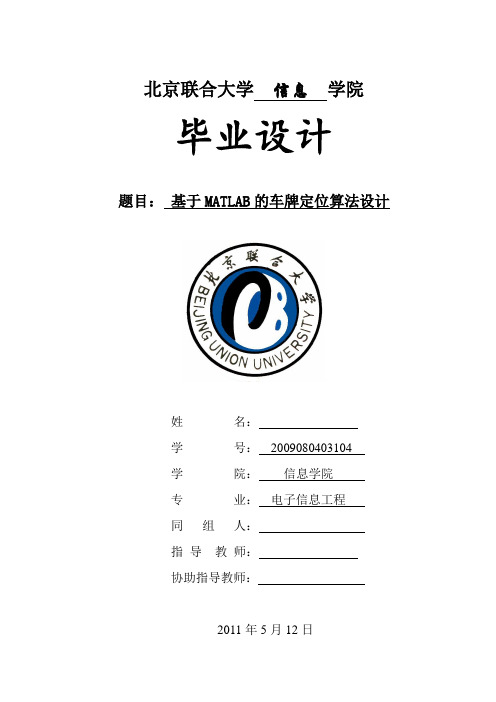
北京联合大学信息学院毕业设计题目:基于MATLAB的车牌定位算法设计姓名:学号:2009080403104学院:信息学院专业:电子信息工程同组人:指导教师:协助指导教师:2011年5月12日摘要车牌自动识别系统是现代智能交通管理的重要组成部分,可用于各级各类车辆管理场所。
与传统的车辆管理方法相比,它大大地提高了管理效率与水平,节省了人力、物力,实现了车辆管理的科学化、规范化,对交通治安起到了一定的保障作用,因此有着广泛的应用前景。
车牌自动识别系统一般包括车牌定位、字符分割和字符识别三个模块。
它的研究主要涉及到模式识别、人工智能、计算机视觉、数字图像处理等众多学科领域。
车牌的定位、分割更是该系统的关键,由于图像场景的复杂性以及车牌位置和图像质量的不可预知性,牌照定位分割系统一直都未做到令人满意,所以有必要对其进行进一步的研究。
本文通过对大量资料的搜集、整理,总结了近年来国内外在车牌定位分割领域的最新研究成果和进展,对车牌区域的固有特征和目前的车牌定位、分割技术进行了分析和比较,提出了自己的观点并设计了一个车牌定位、分割系统。
本文利用MATLAB工具实现车牌定位算法研究。
利用灰度修正.滤波和图像增强等处理方法.较好地消除了图像的噪音,提高了图像质量。
通过对车牌特征的研究,利用边缘扫描方法实现车牌定位。
关键词:车牌定位;倾斜矫正;图像预处理;图像分割AbstractVehicles License Plate Recognition System(LPRS),which is all important part of the contemporary Intelligent Transportation System(ITS),can be applied to vehicle management situations of all levels and all kinds.Compared with traditional vehicles managements,LPRS has greatly improved the efficiency and level of management and saved manpower and material resources,laying a good foundation for the realization of standardized management.We Call safely come to the conclusion that LPRS has already improved the order of the traffic system, illustrating a good prospect of application for us.Generally, the LPR system consists of three modules:license plate location、character segmentation and character recognition.Its study concerns various disciplines including Pattern Recognition、Artificial Intelligence,Computer Vision、Digital Image Processing and SO 011.It is the location and segmentation of license plates standing at the heart of LPR system.Considering that the complexity of image background and the uncertainly of plate position and image quality,it is necessary to do further research into it.By summarizing the latest research achievements and development in the area of license plate location and segmentation both here and abroad,this paper, after making a deep comparison between the intrinsic characteristics of license plate and the current location and segmentation technologies on it,proposes its own understanding and designs a new LP location and segmentation system.The paper introduces a method of car license plate location and realizes a system of car license plate location based on MATLAB.The pre--processing methods including gray level modification,filter and image enhancement,are used to improve image quality and cut image noise.Car license plate location is realized by the method of edge detection and according to the car plate feature.key words:License plate location;Slant correction;Image pre--process ;car Image Segmentation.目录摘要 (1)Abstract (2)引言 (4)一、绪论 (5)1 . 1 、课题的背景和意义 (5)1 . 2、国内外研究状况 (5)1 . 3、车牌识别系统的应用范围 (6)二、系统概述 (9)三、硬件系统设计 (10)3.1、硬件系统设计 (10)3.2、各模块功能 (10)3.3、各模块与DSP的接口设计 (10)3.4.系统原理图和生成的PCB板 (15)四、在MATLAB环境下实现车牌定位的功能 (17)4.1、车牌定位系统介绍 (17)4.2、图像预处理 (17)4.3、灰度化 (18)4.4、图像边缘检测 (20)4.5、形态学处理 (21)4.6、车牌提取 (23)五、结论 (25)问题和不足: (26)不足之处: (27)六、主要参考资料如下: (28)七、致谢 (29)引言随着我国交通运输的不断发展,智能交通系统(Intelligent Traffic System,简称ITS)的推广变的越来越重要,而作为ITS的一个重要组成部分,车辆牌照识别系统(vehicle license plate recognition system,简称LPR)对于交通管理、治安处罚等工作的智能化起着十分重要的作用。
基于某matlab车牌地定位与分割识别程序
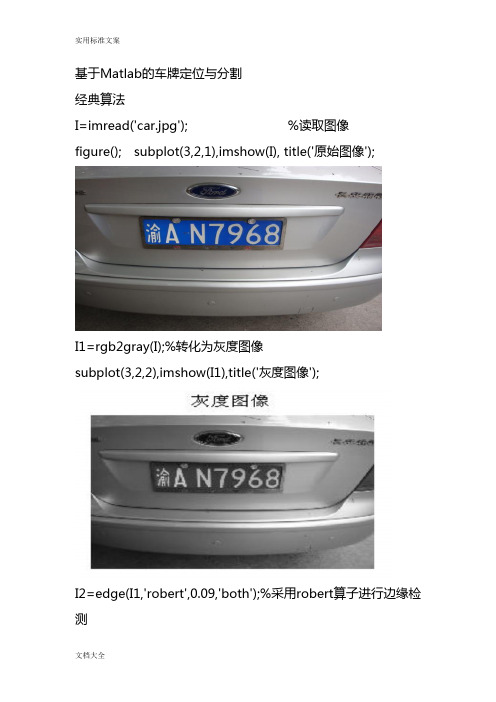
基于Matlab的车牌定位与分割经典算法I=imread('car.jpg'); %读取图像figure(); subplot(3,2,1),imshow(I), title('原始图像');I1=rgb2gray(I);%转化为灰度图像subplot(3,2,2),imshow(I1),title('灰度图像');I2=edge(I1,'robert',0.09,'both');%采用robert算子进行边缘检测subplot(3,2,3),imshow(I2),title('边缘检测后图像');se=[1;1;1]; %线型结构元素I3=imerode(I2,se); %腐蚀图像subplot(3,2,4),imshow(I3),title('腐蚀后边缘图像');se=strel('rectangle',[25,25]); 矩形结构元素I4=imclose(I3,se);%图像聚类、填充图像subplot(3,2,5),imshow(I4),title('填充后图像');I5=bwareaopen(I4,2000);%去除聚团灰度值小于2000的部分subplot(3,2,6),imshow(I5),title('形态滤波后图像');[y,x,z]=size(I5);I6=double(I5);Y1=zeros(y,1);for i=1:yfor j=1:xif(I6(i,j,1)==1)Y1(i,1)= Y1(i,1)+1;endendend[temp MaxY]=max(Y1);figure();subplot(3,2,1),plot(0:y-1,Y1),title('行方向像素点灰度值累计和'),xlabel('行值'),ylabel('像素');%求的车牌的行起始位置和终止位置PY1=MaxY;while ((Y1(PY1,1)>=50)&&(PY1>1))PY1=PY1-1;endPY2=MaxY;while ((Y1(PY2,1)>=50)&&(PY2<y))PY2=PY2+1;endIY=I(PY1:PY2,:,:);X1=zeros(1,x);for j=1:xfor i=PY1:PY2if(I6(i,j,1)==1)X1(1,j)= X1(1,j)+1;endend endsubplot(3,2,2),plot(0:x-1,X1),title('列方向像素点灰度值累计和'),xlabel('列值'),ylabel('像数');%求的车牌的列起始位置和终止位置PX1=1;while ((X1(1,PX1)<3)&&(PX1<x))PX1=PX1+1;endPX2=x;while ((X1(1,PX2)<3)&&(PX2>PX1))PX2=PX2-1;endPX1=PX1-1;PX2=PX2+1;%分割出车牌图像%dw=I(PY1:PY2,PX1:PX2,:);subplot(3,2,3),imshow(dw),title('定位剪切后的彩色车牌图像')4.2 车牌字符分割确定车牌位置后下一步的任务就是进行字符切分分离出车牌号码的全部字符图像。
基于MATLAB的车牌定位系统(含全套CAD图纸)
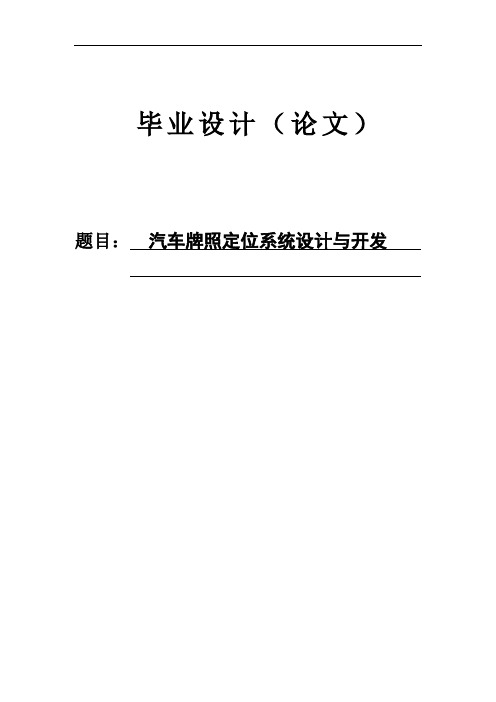
毕业设计(论文)题目:汽车牌照定位系统设计与开发诚信承诺书本人郑重声明:所呈交的毕业设计(论文)汽车牌照定位系统设计与开发是本人在导师的指导下独立进行研究所取得的成果,其内容除了在毕业设计(论文)中特别加以标注引用,表示致谢的内容外,本毕业设计(论文)不包含任何其他个人、集体已发表或撰写的成果作品。
班级:计科94学号:0921144作者姓名:2013 年5 月25 日无锡太湖学院信机系计算机科学与技术专业毕业设计论文任务书一、题目及专题:1、题目汽车牌照定位系统设计与开发2、专题二、课题来源及选题依据课题来源:导师指定选题依据:汽车车牌识别系统是近几年发展起来的计算机视觉和模式识别技术在智能交通领域应用的重要研究课题之一。
在车牌自动识别系统中,首先要将车牌从所获取的图像中分割出来实现车牌定位。
这是进行车牌字符识别的重要步骤,定位的准确与否直接影响车牌识别率。
车辆牌照定位与识别是计算机视觉与模式识别技术在智能交通领域应用的重要研究课题之一,该技术应用范围非常广泛,其中包括:(1)交通流量检测;(2)交通控制与诱导;(3)机场、港口等出入口车辆管理;(4)小区车辆管理;(5)闯红灯等违章车辆监控;(6)不停车自动收费;(7)道口检查站车辆监控;(8)公共停车场安全防盗管理;(9)计算出行时间等。
其潜在在市场应用价值极大,有能力产生巨大的社会效益和经济效益。
三、本设计(论文或其他)应达到的要求:软件、技术要求:在基于图像处理的车牌识别技术的基础上设计并开发了一个基于MATLAB的车牌定位系统通过编写MATLAB文件对各种车辆图像处理方法进行分析、比较,最终确定了车牌预处理、车牌粗定位和精定位的方法。
四、接受任务学生:计科94 班姓名宋开拓五、开始及完成日期:自2012 年11 月12 日至2013年5月25日六、设计(论文)指导(或顾问):指导教师签名签名签名教研室主任〔学科组组长〕签名研究所所长系主任签名2012年11月12日车辆牌照识别系统(vehicle license plate recognition system,简称LPR)是现代智能交通系统中的一项重要研究课题,是实现智能交通的重要环节,涉及领域异常广阔。
基于MATLAB的车牌识别系统的源代码(可以实现)
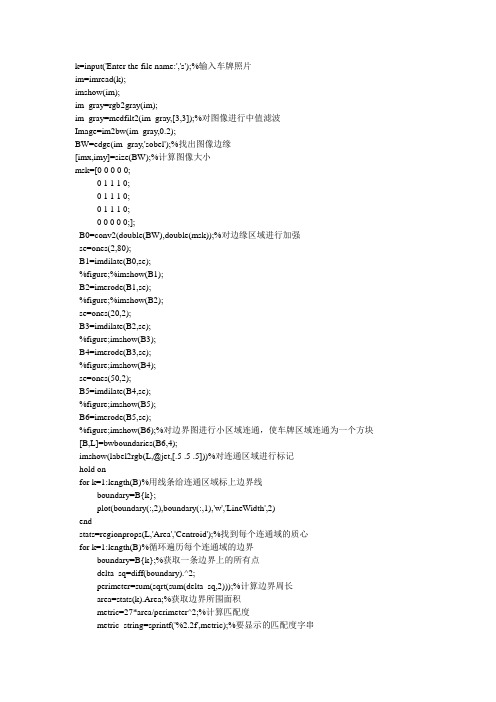
k=input('Enter the file name:','s');%输入车牌照片im=imread(k);imshow(im);im_gray=rgb2gray(im);im_gray=medfilt2(im_gray,[3,3]);%对图像进行中值滤波Image=im2bw(im_gray,0.2);BW=edge(im_gray,'sobel');%找出图像边缘[imx,imy]=size(BW);%计算图像大小msk=[0 0 0 0 0;0 1 1 1 0;0 1 1 1 0;0 1 1 1 0;0 0 0 0 0;];B0=conv2(double(BW),double(msk));%对边缘区域进行加强se=ones(2,80);B1=imdilate(B0,se);%figure;%imshow(B1);B2=imerode(B1,se);%figure;%imshow(B2);se=ones(20,2);B3=imdilate(B2,se);%figure;imshow(B3);B4=imerode(B3,se);%figure;imshow(B4);se=ones(50,2);B5=imdilate(B4,se);%figure;imshow(B5);B6=imerode(B5,se);%figure;imshow(B6);%对边界图进行小区域连通,使车牌区域连通为一个方块[B,L]=bwboundaries(B6,4);imshow(label2rgb(L,@jet,[.5 .5 .5]))%对连通区域进行标记hold onfor k=1:length(B)%用线条给连通区域标上边界线boundary=B{k};plot(boundary(:,2),boundary(:,1),'w','LineWidth',2)endstats=regionprops(L,'Area','Centroid');%找到每个连通域的质心for k=1:length(B)%循环遍历每个连通域的边界boundary=B{k};%获取一条边界上的所有点delta_sq=diff(boundary).^2;perimeter=sum(sqrt(sum(delta_sq,2)));%计算边界周长area=stats(k).Area;%获取边界所围面积metric=27*area/perimeter^2;%计算匹配度metric_string=sprintf('%2.2f',metric);%要显示的匹配度字串if metric>=0.85&&metric<=1.15&&area>1000%截取出匹配度接近1且面积大于1000像素的连通域centroid=stats(k).Centroid;plot(centroid(1),centroid(2),'ko');%提取该连通域所对应在二值图像中的矩形区域goalboundary=boundary;s=min(goalboundary,[],1);e=max(goalboundary,[],1);goal=imcrop(Image,[s(2) s(1) e(2)-s(2) e(1)-s(1)]);endtext(boundary(1,2)-35,boundary(1,1)+13,metric_string,'Color','g','FontSize',14,'FontWeight','bold') ;%显示匹配度字串endgoal=~goal;%对截取图像进行反色处理figure;imshow(goal);[a,b]=size(goal);for i=a/2:-1:1 %从图像水平中轴开始向上扫描,当白点数少于每行总点数的1/10时,停止扫描,并将该行定义为车牌字符区域的上限num=0;for j=1:bif goal(i,j)==1num=num+1;endendif num<(b*0.1)line_up=i;break;endendfor i=a/2:a %从图像水平中轴开始向下扫描,当白点数少于每行总点数的1/10时,停止扫描,并将该行定义为车牌字符区域的下限num=0;for j=1:bif goal(i,j)==1num=num+1;endendif num<(b*0.1)line_down=i;break;endendgoal=goal(line_up:line_down,1:b);%根据之前定义的上下限截取车牌字符区域figure;imshow(goal);%显示车牌字符区域[a,b]=size(goal);row=zeros(18);now=1;flag=0;for j=1:b %对截取出的字符区域进行竖列扫描,并取每列总点数的1/10作为阈值点,当每列的白点数从阈值以上掉落到阈值以下或从阈值以下上升到阈值以上时,记录该列的横坐标num=0;for i=1:aif goal(i,j)==1num=num+1;endendif flag==0if num<0.1*arow(now)=j;now=now+1;flag=1;endelseif num>0.1*arow(now)=j;now=now+1;flag=0;endendendif row(3)-row(2)>10 %判断扫描出的第二块区域(扫描到的第二列与第三列之间)是否包含有效字符,如包含,则将扫描到的第二列定义为字符分割的起始列;否则,则定义第一列为起始列now=2;elsenow=1;endfigure;l1=0;l2=0;for k=1:8m=row(now);n=row(now+1);temp=goal(1:a,m:n);point=0;%扫描每一个字符图片的白点数for i=1:afor j=1:n-mif temp(i,j)==1point=point+1;endendendif point>0.4*a*(n-m)&&n>m %当扫描到的白点数小于总点数的2/5时放弃输出(有可能是车牌上的点状分隔符)l2=l2+1;%l2用来记录识别出的字符数subplot(1,7,l2);x(k)=code(temp);%调用子程序进行字符扫描,并返回字符的ASCII码x(k)=uint8(x(k));if x(k)>0 %当所选区域不为空时进行输出l1=l1+1;%l1用来记录输出的字符数s(l1)=char(x(k));endtemp(32,32)=0;imshow(temp);endnow=now+2;endy=char(s);%将得到的ASCII码重新转换为字符并在屏幕上输出fprintf('\r\n该车辆的车牌号为:\r\n');disp(y);fprintf('\r\n输出的字符数为:%4d\r\n',l1);fprintf('识别出的字符数为:%4d\r\n',l2);。
基于MATLAB的车牌识别程序详解..精要

(1)车牌区域内字符的纹理特征。 (2)车牌的格式标准和字符标准信息。 (3)车牌区域水平或者垂直投影特征。 (4)车牌区域的位置特点。 (5)车牌的彩色信息。 (6)频谱特征。
处理方法主要分为两大块:行定位与列定位。具体方法如下: 获取特征图像 要想从一整幅包括车身,背景等其他相对于车牌来说属于干扰因素
相关的MATLAB 指令: h=fspecial(‘average’,3); ;%平均值的设定 d=im2bw(round(filter2(h,d)));%平均滤波
(四)、图像的边缘检测:
边缘是图像的最本特征,边缘检测在计算机视觉、图像分析等应 用中起着重要的作用,使图像识别与分析的重要环节。边缘存在于 目标与背景、目标与目标、区域与区域之间因此它是图像分割、形 状特征、纹理特征的基础。缘具有方向与幅度两个特征。沿边缘走 向,像素变化比较平缓。而垂直于边缘走向,则像素变化剧烈。这 种剧烈可能呈现阶跃状,也可能呈现斜坡状。边缘的上的像素的一 阶导数较大; 二阶导数在边缘检测处值为零,呈现零交叉。这种特 点使得边缘检测可以利用数学方法获得,经典的边缘检测算子有 Roberts、Sobel、Prewitt、LoG、Canny算子。
(一)图像预处理 收集到的图片一般为彩色图片,由于彩色图片占用存储容
量大,处理时间长,因此需要对图像进行灰度转换,将彩色图像转 换为灰度图像,灰度图像只保留亮度信息,方便使用,也为后面的 对图像进行二值化处理提供方便。
(完整word版)基于Matlab的车牌识别实现源码

(完整word版)基于Matlab的车牌识别实现源码function[]=main(jpg)close allclctic %测定算法执行的时间[fn,pn]=uigetfile('timg1,jpg','选择图片') %读入图片I=imread([pn,fn]);figure,imshow(I);title('原始图像'); %显示原始图像Im1=rgb2gray(I);figure(2),subplot(1,2,1),imshow(Im1);title('灰度图');figure(2),subplot(1,2,2),imhist(Im1);title('灰度图的直方图'); %显示图像的直方图Tiao=imadjust(Im1,[0.19,0.78],[0,1]); %调整图片figure(3),subplot(1,2,1),imshow(Tiao);title('增强灰度图');figure(3),subplot(1,2,2),imhist(Tiao);title('增强灰度图的直方图');Im2=edge(Tiao,'Roberts','both'); %使用sobel算子进行边缘检测figure(4),imshow(Im2);title('sobel算子实现边缘检测')se=[1;1;1];Im3=imerode(Im2,se);figure(5),imshow(Im3);se=strel('square',40);%'rectangle',[25,25]/'diamond',25/Im4=imclose(Im3,se);figure(6),imshow(Im4);title('平滑图像的轮廓');Im5=bwareaopen(Im4,1500);figure(7),imshow(Im5);title('移除小对象');[y,x,z]=size(Im5); %返回Im5各维的尺寸,并存储在变量y、x、z中Im6=double(Im5); %将Im5换成双精度数值%开始横向扫描tic %tic计时开始,toc结束,计算tic与toc之间程序的运行时间Blue_y=zeros(y,1); %产生y*1的全0矩阵for i=1:y %逐行扫描for j=1:xif(Im6(i,j,1)==1)%如果Im6图像中坐标为(i,j)的点值为1,即为移除小对象的白色区域,Blue_y(i,1)= Blue_y(i,1)+1;%则y*1列矩阵的相应像素点的元素值加1,endendend[temp MaxY]=max(Blue_y);%temp为向量Blue_y的矩阵中的最大值,MaxY为该值的索引(最大值在向量中的位置)%返回包含最大元素的列,即白色区域最宽的列%Y方向车牌区域确定figure(8),subplot(1,2,1),plot(0:y-1,Blue_y),title('行方向白色像素点累计'),xlabel('行数'),ylabel('个数');PY1=MaxY;while ((Blue_y(PY1,1)>=120)&&(PY1>1))PY1=PY1-1;endPY2=MaxY;while ((Blue_y(PY2,1)>=40)&&(PY2<y))< p="">PY2=PY2+1;IY=I(PY1:PY2,:,:);%IY为原始图像I中截取的纵坐标在PY1:PY2之间的部分%横向扫描完成,开始纵向扫描Blue_x=zeros(1,x);%进一步确定x方向的车牌区域for j=1:x %逐列扫描for i=PY1:PY2if(Im6(i,j,1)==1)Blue_x(1,j)= Blue_x(1,j)+1; %根据Im5的x值确定endendendfigure(8),subplot(1,2,2),plot(0:x-1,Blue_x),title('列方向白色像素点累计'),xlabel('列数'),ylabel('个数'); PX1=1;while ((Blue_x(1,PX1)<3)&&(PX1<x))< p="">PX1=PX1+1;endPX2=x;while ((Blue_x(1,PX2)<3)&&(PX2>PX1))PX2=PX2-1;end%end纵向扫描PX1=PX1-2;%对车牌区域的校正PX2=PX2+2;dw=I(PY1:PY2,PX1:PX2,:);t=toc;figure(9),subplot(1,2,1),imshow(IY),title('垂直方向合理区域');figure(9),subplot(1,2,2),imshow(dw),title('定位剪切后的彩色车牌图像') imwrite(dw,'dw.jpg'); %将图像写入图形文件中a=imread('dw.jpg');b=rgb2gray(a);imwrite(b,'车牌灰度图像.jpg');figure(10);subplot(3,2,1),imshow(b),title('1.车牌灰度图像')g_max=double(max(max(b)));g_min=double(min(min(b)));T=round(g_max-(g_max-g_min)/3); %T为设定的二值化的阈值,返回一个四舍五入的整数值[m,n]=size(b);d=(double(b)>=T); %d为二值图像imwrite(d,'车牌二值图像.jpg');figure(10);subplot(3,2,2),imshow(d),title('2.车牌二值图像')figure(10),subplot(3,2,3),imshow(d),title('3.均值滤波前')h=fspecial('average',3);%建立预定义的滤波算子,average为均值滤波,模板尺寸为3*3d=imbinarize(round(filter2(h,d))); %im2bw,使用指定的滤波器h对h进行d即均值滤波imwrite(d,'均值滤波后.jpg');figure(10),subplot(3,2,4),imshow(d),title('4.均值滤波后')se=eye(2);%单位矩阵[m,n]=size(d); %d为二值图像,返回信息矩阵if bwarea(d)/m/n>=0.365%二值图像中对象的总面积与整个面积的比大于0.365d=imerode(d,se);%进行腐蚀elseif bwarea(d)/m/n<=0.235%二值图像中对象的总面积与整个面积的比值小于0.235 d=imdilate(d,se);%进行膨胀endimwrite(d,'膨胀或腐蚀处理后.jpg');figure(10),subplot(3,2,5),imshow(d),title('5.膨胀或腐蚀处理后.jpg')d=qiege(d); %切割,寻找连续有文字的块,若长度大于某阈值,则认为该块有两个字符组成,需要分割k1=1;k2=1;s=sum(d);j=1;while j~=nwhile s(j)==0j=j+1;endk1=j;while s(j)~=0 && j<=n-1j=j+1;endk2=j-1;if k2-k1>=round(n/6.5)[val,num]=min(sum(d(:,[k1+5:k2-5])));d(:,k1+num+5)=0;endendd=qiege(d);y1=10;y2=0.25;flag=0;word1=[];while flag==0[m,n]=size(d);wide=0;while sum(d(:,wide+1))~=0 %扫过的地方不全为黑色,向右移动,直到不是停止wide=wide+1;endif wide<="" p="">d(:,[1:wide])=0;%将此片区域全部转化为黑色,d=qiege(d);%重新切割elsetemp=qiege(imcrop(d,[1 1 wide m]));%返回已经切割好的区域[m,n]=size(temp);all=sum(sum(temp));%统计切割区域所有元素two_thirds=sum(sum(temp([round(m/3):2*round(m/3)],:)));%统计切割区域1/3至2/3行所有元素if two_thirds/all>y2 flag=1;word1=temp;endd(:,[1:wide])=0;d=qiege(d);end[word2,d]=getword(d); %分割出第二个字符[word3,d]=getword(d); %分割出第三个字符[word4,d]=getword(d); %分割出第四个字符[word5,d]=getword(d); %分割出第五个字符[word6,d]=getword(d); %分割出第六个字符[word7,d]=getword(d); %分割出第七个字符word1=imresize(word1,[40 20]);%模板字符大小统一为40*20,为字符辨认做准备word2=imresize(word2,[40 20]);word3=imresize(word3,[40 20]);word4=imresize(word4,[40 20]);word5=imresize(word5,[40 20]);word6=imresize(word6,[40 20]);word7=imresize(word7,[40 20]);figure(11);subplot(2,7,1),imshow(word1),title('1');subplot(2,7,2),imshow(word2),title('2');subplot(2,7,3),imshow(word3),title('3');subplot(2,7,4),imshow(word4),title('4');subplot(2,7,5),imshow(word5),title('5');subplot(2,7,6),imshow(word6),title('6');subplot(2,7,7),imshow(word7),title('7');imwrite(word1,'1.jpg');imwrite(word2,'2.jpg');imwrite(word3,'3.jpg');imwrite(word4,'4.jpg');imwrite(word5,'5.jpg');imwrite(word6,'6.jpg');imwrite(word7,'7.jpg');liccode=char(['0':'9' 'A':'Z' '辽粤豫鄂鲁陕京津苏浙']);%建立自动识别字符代码表,将t'0':'9' 'A':'Z' '鲁陕苏豫'多个字符串组成一个字符数组,每行对应一个字符串,字符数不足的自动补空格SubBw2=zeros(40,20);%40*20的零矩阵l=1;ii=int2str(I);%整型转换字符串t=imread([ii,'.jpg']);SegBw2=imresize(t,[40 20],'nearest');%改变图片的大小SegBw2=double(SegBw2)>50;%将灰度图转化为二值图像,double产生0-255的灰度值if l==1 %第一位汉字识别kmin=37;kmax=46;%模板中汉字所在的位置elseif l==2 %第二位字母识别kmin=11;kmax=36;%A-Z字母位置elsel>=3 %第三位后字母或数字识别kmin=1;kmax=36;endfor k2=kmin:kmaxfname=strcat('字符模板\',liccode(k2),'.jpg');SamBw2=imread(fname);SamBw2=double(SamBw2)>50;%将模板转换为二值图,double产生0-255的灰度值for i=1:40for j=1:20SubBw2(i,j)=SegBw2(i,j)-SamBw2(i,j);endend%相当于两幅图相减得第三幅图Dmax=0;for k1=1:40for l1=1:20if abs(SubBw2(k1,l1))>0Dmax=Dmax+1;endendendError(k2)=Dmax;endError1=Error(kmin:kmax);%模板对应的字符模板进行匹配选择findc=find(Error1==MinError);Code(l*2-1)=liccode(findc(1)+kmin-1);Code(l*2)=' ';l=l+1;endmsgbox(Code,'识别结果')function [word,result]=getword(d) %定义分割字符用函数(1)word=[];flag=0;y1=8;y2=0.5;while flag==0[m,n]=size(d);wide=0;while sum(d(:,wide+1))~=0 && wide<=n-2wide=wide+1;endtemp=imcrop(d,[1 1 wide m]);%用于返回图像的一个裁剪区域[m1,n1]=size(temp);z=sum(temp,2);count=0;for i=1:m1if z(i)~=0count=count+1;endendif count<="" p="">d(:,[1,wide])=0;d=qiege(d);elsetemp=qiege(imcrop(d,[1 1 wide m]));%用于返回图像的一个裁剪区域[m1,n1]=size(temp);if widey2 %宽度过小,或长大于宽的两倍d(:,[1,wide])=0;if sum(sum(d))~=0 %d中所有元素之和d=qiege(d);%切割出最小范围elseword=[];flag=1;endword=qiege(imcrop(d,[1 1 wide m]));%划分出的temp进行切割d(:,[1:wide])=0;%切割完后该区域变为黑色if sum(sum(d))~=0d=qiege(d);flag=1;elsed=[];endendendendresult=d;function e=qiege(d) %定义分割字符用函数(2)[m,n]=size(d);top=1;bottom=m;left=1;right=n; %intewhile sum(d(top,:))==0 && top<=mtop=top+1;endwhile sum(d(bottom,:))==0 && bottom>=1 bottom=bottom-1;endwhile sum(d(:,left))==0 && left<=nleft=left+1;endwhile sum(d(:,right))==0 && right>=1right=right-1;enddd=right-left;hh=bottom-top;e=imcrop(d,[left top dd hh]);//字符实别需要字符图库,没有对照图库是识别不了的</x))<></y))<>。
基于matlab的车牌识别算法
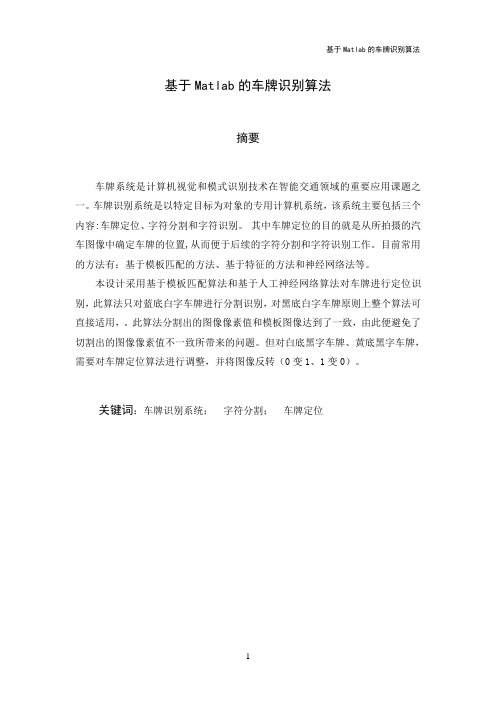
基于Matlab的车牌识别算法摘要车牌系统是计算机视觉和模式识别技术在智能交通领域的重要应用课题之一。
车牌识别系统是以特定目标为对象的专用计算机系统,该系统主要包括三个内容:车牌定位、字符分割和字符识别。
其中车牌定位的目的就是从所拍摄的汽车图像中确定车牌的位置,从而便于后续的字符分割和字符识别工作。
目前常用的方法有:基于模板匹配的方法、基于特征的方法和神经网络法等。
本设计采用基于模板匹配算法和基于人工神经网络算法对车牌进行定位识别,此算法只对蓝底白字车牌进行分割识别,对黑底白字车牌原则上整个算法可直接适用,。
此算法分割出的图像像素值和模板图像达到了一致,由此便避免了切割出的图像像素值不一致所带来的问题。
但对白底黑字车牌、黄底黑字车牌,需要对车牌定位算法进行调整,并将图像反转(0变1、1变0)。
关键词:车牌识别系统;字符分割;车牌定位LICENSE PLATE RECOGNITION ALGORITHM BASEDON MATLABABSTRACTLicense plate system is a computer vision and pattern recognition technology in one of the important application research topic in the field of intelligent transportation. License plate recognition system based on specific goals of a special computer system, the system mainly includes three contents: license plate locating, character segmentation and character recognition. One of the purpose of license plate location is taken from the auto locate the license plate in the image, so as to facilitate the subsequent work character segmentation and character recognition. Now commonly used methods are: based on template matching method, based on the characteristics of the method and neural network, etc.This design USES based on template matching algorithm and based on artificial neural network algorithm to locate license plate recognition, the algorithm is only for blue white license plate segmentation recognition, the algorithm can be directly applicable in principle to the black white plate,. This algorithm to segment the image pixel values and template image, thus to avoid the cut out in the process of image pixel values are not consistent. But black on white background and black text plate, yellow bottom plate, adjustments need to license plate localization algorithm, and the image inversion of (0, 1, 1, 0).Key words: license plate recognition system; Character segmentation; License plate location目录1 前言 (4)1.1车牌号识别研究背景 (4)1.2 车牌号识别技术研究现状和趋势 (5)1.2.1国内外车牌识别技术情况及我国车牌特点 (5)1.2.2车牌识别技术的应用前景 (6)1.3 车牌识别研究内容 (7)2 车牌识别系统设计原理概述 (9)3 车牌识别系统程序设计 (11)3.1图像读取及车牌区域提取 (11)3.1.1图像灰度图转化 (11)3.1.2图像的边缘检测 (13)3.1.3灰度图腐蚀 (14)3.1.4图像平滑处理 (15)3.1.5移除小对象 (16)3.1.6车牌区域的边界值计算 (17)3.2字符切割 (18)3.2.1字符切割前的图像去噪处理 (18)3.2.2字符切割前的图像膨胀和腐蚀处理 (19)3.2.3字符切割 (19)3.3字符识别 (22)3.3.1字符识别方法选择 (22)3.3.2字符归一化 (22)3.3.3字符匹配识别 (23)4 仿真结果及分析 (26)4.1 车牌定位及图像读取及其图像处理 (26)4.2 车牌字符分割及其图像处理 (26)5 结论 (28)参考文献 (29)致谢............................................... 错误!未定义书签。
基于matlab的蓝色车牌定位与识别---定位

基于matlab的蓝⾊车牌定位与识别---定位接着昨天的⼯作继续。
定位的过程有些是基于车牌的颜⾊进⾏定位的,⾃⼰则根据数字图像⼀些形态学的⽅法进⾏定位的。
合着代码进⾏相关讲解。
1.相对彩⾊图像进⾏灰度化,然后对图像进⾏开运算。
再⽤⼩波变换获取图像的三个分量。
考虑到车牌的竖直分量较为丰富,选⽤竖直分量进⾏后续操作。
注意下,这⾥的⼀些参数可能和你的图⽚⼤⼩有关,所以要根据实际情况调整。
Image=imread('D:\1_2学习\图像处理\车牌识别\matlab_car_plate-recognization\car_example\car0.jpg');%获取图⽚im=double(rgb2gray(Image));im=imresize(im,[1024,2048]);%重新设置图⽚⼤⼩ [1024,2048]% % 灰度拉伸% % im=imadjust(Image,[0.150.9],[]);s=strel('disk',10);%strei函数Bgray=imopen(im,s);%打开sgray s图像% % figure,imshow(Bgray);title('背景图像');%输出背景图像% %⽤原始图像与背景图像作减法,增强图像im=imsubtract(im,Bgray);%两幅图相减% figure,imshow(mat2gray(im));title('增强⿊⽩图像');%输出⿊⽩图像[Lo_D,Hi_D]=wfilters('db2','d'); % d Decomposition filters[C,S]= wavedec2(im,1,Lo_D,Hi_D); %Lo_D is the decomposition low-pass filter% decomposition vector C corresponding bookkeeping matrix Sisize=prod(S(1,:));%元素连乘%cA = C(1:isize);%cA 49152cH = C(isize+(1:isize));cV = C(2*isize+(1:isize));cD = C(3*isize+(1:isize));%cA = reshape(cA,S(1,1),S(1,2));cH = reshape(cH,S(2,1),S(2,2));cV = reshape(cV,S(2,1),S(2,2));cD = reshape(cD,S(2,1),S(2,2));获取结果2. 对上⾯过程中获取的图像进⾏边沿竖直⽅向检测,再利⽤形态学的开闭运算扩⼤每个竖直⽅向⽐较丰富的区域。