经典功率谱估计
利用经典谱估计法估计信号的功率谱(随机信号)

随机信号利用经典谱估计法估计信号的功率谱作业综述:给出一段信号“asd.wav”,利用经典谱估计法的原理,通过不同的谱估计方法,求出信号的功率谱密度函数。
采用MATLAB语言,利用MATLAB语言强大的数据处理和数据可视化能力,通过GUI的对话框模板,使操作更为简便!在一个GUI界面中,同时呈现出不同方法产生出的功率谱。
这里给出了几种不同的方法:BT法,周期图法,平均法以及Welch法。
把几种不同方法所得到的功率谱都呈现在一个界面中,便于对几种不同方法得到的功率谱作对比。
一.题目要求给出一段信号及采样率,利用经典谱估计法估计出信号的功率谱。
二.基本原理及方法经典谱估计的方法,实质上依赖于传统的傅里叶变换法。
它是将数据工作区外的未知数据假设为零,相当于数据加窗,主要方法有BT法,周期图法,平均法以及Welch法。
1. BT法(Blackman-Tukey)● 理论基础:(1)随机序列的维纳-辛钦定理由于随机序列{X(n)}的自相关函数Rx(m)=E[X(n)X(n+m)]定义在离散点m 上,设取样间隔为,则可将随机序列的自相关函数用连续时间函数表示为等式两边取傅里叶变换,则随机序列的功率谱密度(2)谱估计BT法是先估计自相关函数Rx(m)(|m|=0,1,2…,N-1),然后再经过离散傅里叶变换求的功率谱密度的估值。
即其中可有式得到。
2. 周期图法● 理论基础:周期图法是根据各态历经随机过程功率谱的定义来进行谱估计的。
在前面我们已知,各态历经的连续随机过程的功率谱密度满足式中是连续随机过程第i个样本的截取函数的频谱。
对应在随机序列中则有由于随机序列中观测数据仅在的点上存在,则的N点离散傅里叶变换为:因此有随机信号的观测数据的功率谱估计值(称“周期图”)如下:由于上式中的离散傅里叶变换可以用快速傅里叶变换计算,因此就可以估计出功率谱。
3.平均法:● 理论基础:平均法可视为周期图法的改进。
周期图经过平均后会使它的方差减少,达到一致估计的目的,有一个定理:如果是不相关的随机变量,且都有个均值及其方差,则可以证明它们的算术平均的均值为。
经典功率谱和Burg法的功率谱估计

现代信号处理作业实验题目:设信号)()8.0cos(25.0)47.0cos()35.0cos()(321n v n n n n x ++++++=θπθπθπ,其中321,,θθθ是[]ππ,-内的独立随机变量,v(n)是单位高斯白噪声。
1.利用周期图法对序列进行功率谱估计。
数据窗采用汉明窗。
2.利用BT 法对序列进行功率谱估计,自相关函数的最大相关长度为M=64,128,256,512采用BARTLETT 窗。
3.利用Welch 法对序列进行功率谱估计,50%重叠,采用汉明窗,L=256,128,64。
4.利用Burg 法对序列进行AR 模型功率谱估计,阶数分别为10,13.要求每个实验都取1024个点,fft 作为谱估计,取50个样本序列的算术平均,画出平均的功率谱图。
实验原理:1)。
周期图法:又称间接法,它把随机信号的N 个观察值x N (n)直接进行傅里叶变换,得到X N (e jw ),然后取其幅值的平方,再除以N ,作为对x (n )真实功率谱的估计。
2^)(1)(jw e X Nw P N per =, 其中∑-=-=1)()(N n jwn N jwN e n x e X 2)。
BT 法:对于N 个观察值x(0),x(1),。
,x(N-1),令x N (n)=a(n)x(n)。
计算r x (m )为∑--=-≤+=mN n N Nx N m m n x n xN m r 101),()(1)(,计算其傅里叶变换∑-=--≤=MMm jwm xBT N M e m rm v w P 1 ,)()()(^^,作为观察值的功率谱的估计。
其中v(m)是平滑窗。
3)。
Welch 法:假定观察数据是x(n),n=0,1,2...,N-1,现将其分段,每段长度为M,段与段之间的重叠为M-K,第i 个数据段经加窗后可表示为 1,...,1,0 )()()(-=+=M i iK n x n a n x i M其中K 为一整数,L 为分段数,该数据段的周期图为2)(1)(^w X MU w P i M iper =,其中∑-=-=10)()(M n j w n iM i M e n x w X 。
经典功率谱估计方法实现问题的研究

1 随机信号的经典谱估计方法估计功率谱密度的平滑周期图是一种计算简单的经典方法。
它的主要特点是与任何模型参数无关,是一类非参数化方法[4]。
它的主要问题是:由于假定信号的自相关函数在数据观测区以外等于零,因此估计出来的功率谱很难与信号的真实功率谱相匹配。
在一般情况下,周期图的渐进性能无法给出实际功率谱的一个满意的近似,因而是一种低分辨率的谱估计方法。
本章主要介绍了周期图法、相关法谱估计(BT )、巴特利特(Bartlett)平均周期图的方法和Welch 法这四种方法。
2.1 周期图法周期图法又称直接法。
它是从随机信号x(n)中截取N 长的一段,把它视为能量有限x(n)真实功率谱)(jw x e S 的估计)(jw x e S 的抽样.周期图这一概念早在1899年就提出了,但由于点数N一般比较大,该方法的计算量过大而在当时无法使用。
只是1965年FFT 出现后,此法才变成谱估计的一个常用方法。
周期图法[5]包含了下列两条假设:1.认为随机序列是广义平稳且各态遍历的,可以用其一个样本x(n)中的一段)(n x N 来估计该随机序列的功率谱。
这当然必然带来误差。
2.由于对)(n x N 采用DFT ,就默认)(n x N 在时域是周期的,以及)(k x N 在频域是周期的。
这种方法把随机序列样本x(n)看成是截得一段)(n x N 的周期延拓,这也就是周期图法这个名字的来历。
与相关法相比,相关法在求相关函数)(m R x 时将)(n x N 以外是数据全都看成零,因此相关法认为除)(n x N 外x(n)是全零序列,这种处理方法显然与周期图法不一样。
但是,当相关法被引入基于FFT 的快速相关后,相关法和周期图法开始融合。
通过比较我们发现:如果相关法中M=N ,不加延迟窗,那么就和补充(N-1)个零的周期图法一样了。
简单地可以这样说:周期图法是M=N 时相关法的特例。
因此相关法和周期图法可结合使用。
2.2 相关法谱估计(BT )法这种方法以相关函数为媒介来计算功率谱,所以又叫间接法。
经典功率谱估计

雷达和声呐系统
目标检测
在雷达和声呐系统中,经典功率谱估计常被用于目标检测。通过对接收到的信号进行功率 谱分析,可以判断是否存在目标以及目标的位置和速度等信息。
距离和速度测量
在雷达和声呐系统中,经典功率谱估计还可以用于距离和速度测量。通过对接收到的信号 进行功率谱分析,可以估计出目标与系统之间的距离和相对速度。
信号分类
在雷达和声呐系统中,经典功率谱估计还可以用于信号分类。通过对接收到的信号进行功 率谱分析,可以判断目标的类型,例如区分飞机、船舶或车辆等不同类型目标。
05 经典功率谱估计的改进方 法
基于小波变换的功率谱估计
1
小波变换能够将信号分解成不同频率和时间尺度 的分量,从而更好地揭示信号的内在结构和特征。
然而,这些方法通常需要较长 的数据长度和较为复杂的计算 过程,对于短数据和实时处理 的应用场景具有一定的局限性 。
研究展望
01
随着信号处理技术的发展,经典功率谱估计方法仍有进一步优化的空 间。
02
针对短数据和实时处理的应用场景,研究更为快速、准确的功率谱估 计方法具有重要的实际意义。
03
结合机器学习和人工智能技术,探索基于数据驱动的功率谱估计方法 是一个值得关注的方向。
优点
能够提供较高的频率分辨率和较低的估计误差。
原理
格莱姆-梅尔谱估计利用了信号的模型参数,通过 构造一个模型函数来描述信号的频率响应特性, 并求解该函数的极值问题得到信号的功率谱。
缺点
需要预先设定模型函数的形式和参数,且计算复 杂度较高。
03 经典功率谱估计的优缺点
优点
01
02
03
算法成熟
经典功率谱估计方法经过 多年的研究和发展,已经 相当成熟,具有较高的稳 定性和可靠性。
功率谱估计的方法

功率谱估计的方法
功率谱估计是信号处理中常用的一种方法,用于分析信号在频域内的特点,通常可以分为以下几种方法:
一、经典方法
1.傅里叶变换法:将时域信号通过傅里叶变换变换到频域,然后计算功率谱密度。
2.自相关法:通过自相关函数反映信号的统计平稳性,然后通过傅里叶变换计算功率谱密度。
3.周期图法:将信号分解为若干个周期波形,然后对每个周期波形进行傅里叶变换计算周期功率谱,最后汇总得到整个信号的功率谱。
二、非经典方法
1. 时-频分析法:如短时傅里叶变换(STFT)、小波变换等,将信号分解为时域和频域两个维度的分量,从而可以分析信号在时间和频率上的变化。
2. 基于协方差矩阵的特征值分解法:通过建立协方差矩阵,在张成空
间中求解特征向量,从而达到计算信号功率谱的目的。
3. 基于频率锁定法:如MUSIC法、ESPRIT法等,是一种利用特定信号空间中的特定模式进行处理的方法。
以上方法各有特点,根据实际需求选择不同的方法可以得到相应的功率谱估计结果。
生医信号处理课件6经典功率谱

(2)方差 Var[S (e j )] Var[S (e j )] S 2 (e j ) M per x 频率分辨率及旁瓣泄漏
Re s[SM (e j )] ()3dB
二、平均法(Bartlett法)
Bartlett提出,将 x(n)分为长L,互不重叠的k段子序列,N=kL,
3、讨论
m M 2 w ( m) M
M↑偏差↓
M↑方差↑ 一般M=N/5
五、谱估计技术的性能指标
1、变异性γ (归一化方差)
2、品质因数μ
4 2 x
j
j
结论:非一致估计
例
三、周期图的随机起伏
Cov[S per (e
j1
), S per (e
j2
sin[(k l ) ] 2 )] [ ] (k l ) N sin[ ] N
4 x
一、数据加窗(修正周期图)
数据窗
6.4.3 功率谱估计的改进
E[ S per (e )]
j
j
1 E[ S BT (e )] S x ( e j ) W ( e j ) 2
j
N ,
W (e j ) ()
是渐近无偏
2、方差
if 1 M N
j
1 2 Var[ S BT (e )] S x (e j ) W 2 (e j ( u ) )du 2N 1 j S x (e ) N
Bartlett法和Welch法分别对周期图和修正周期图进行平均, 从而达到减少方差的目的。 Blackman-Tukey法为了减少周
期图的方差,对自相关序列的估计进行加窗处理,从而减
少自相关序列的估计中那些不可靠的估计值对周期图的贡 献。
经典功率谱估计实验报告(3篇)
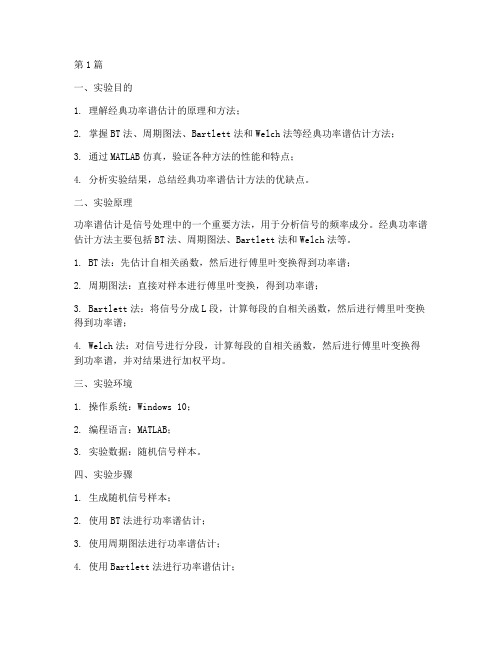
第1篇一、实验目的1. 理解经典功率谱估计的原理和方法;2. 掌握BT法、周期图法、Bartlett法和Welch法等经典功率谱估计方法;3. 通过MATLAB仿真,验证各种方法的性能和特点;4. 分析实验结果,总结经典功率谱估计方法的优缺点。
二、实验原理功率谱估计是信号处理中的一个重要方法,用于分析信号的频率成分。
经典功率谱估计方法主要包括BT法、周期图法、Bartlett法和Welch法等。
1. BT法:先估计自相关函数,然后进行傅里叶变换得到功率谱;2. 周期图法:直接对样本进行傅里叶变换,得到功率谱;3. Bartlett法:将信号分成L段,计算每段的自相关函数,然后进行傅里叶变换得到功率谱;4. Welch法:对信号进行分段,计算每段的自相关函数,然后进行傅里叶变换得到功率谱,并对结果进行加权平均。
三、实验环境1. 操作系统:Windows 10;2. 编程语言:MATLAB;3. 实验数据:随机信号样本。
四、实验步骤1. 生成随机信号样本;2. 使用BT法进行功率谱估计;3. 使用周期图法进行功率谱估计;4. 使用Bartlett法进行功率谱估计;5. 使用Welch法进行功率谱估计;6. 对比分析各种方法的估计结果。
五、实验结果与分析1. BT法:BT法是一种较为精确的功率谱估计方法,其估计结果与真实功率谱较为接近。
但是,BT法需要计算样本的自相关函数,计算量较大。
2. 周期图法:周期图法是一种简单易行的功率谱估计方法,但其估计结果存在较大误差。
当样本长度N较大时,周期图法的估计结果逐渐接近真实功率谱。
3. Bartlett法:Bartlett法在Bartlett窗口的宽度较大时,估计结果较为准确。
但是,当Bartlett窗口的宽度较小时,估计结果误差较大。
4. Welch法:Welch法是一种改进的周期图法,通过分段和加权平均,提高了估计精度。
Welch法在估计精度和计算量之间取得了较好的平衡。
功率谱估计的经典方法PPT课件

吉林大学通信工程学院信息科学实验室
6
时间平均
(11)一个平稳随机过程的一个取样序列的时间平均等于它的集合平
均,则称它是遍历性随机过程。时间平均记为 x(n) ,则取样序列的算术
平均值和时间取样自相关序列定义为
x(n) lim 1
功率谱估计的经典方法
版权所有
吉林大学通信工程学院信息科学实验室
1
离散随机过程
为了描述随机变量,引入了概率分布函数、概率密度函数以及随机变 量的数字特征。这些函数或参数都是针对一维随机变量定义的。统称一 维统计特征。
但对于离散随机过程,因为它是由无限多个随机变量构成的时间序列
xn, n ,因此为完整地描述它,仅知道随机变量的特征是不
Syy(z)
Ryy(m) zm
Rxx(m
p)Rhh (
p)
zm
m
m p
Rxx(n)Rhh ( p)
z n z p
Sxx(z)Shh (z)
m n
S
xx
(
z
)H
(
z
)
H
(
z
1
)
协方差序列的z变换
Sxx(z) Cxx(m) zm , m
称为平稳随机过程的功率谱。在今后的讨论中总假设随机信号的均值为
零,所以有
Sxx(z) Rxx(m) zm , m
由于 Rxx(m) Rxx(m) ,则有 Sxx (z) Sxx (z 1) 。
- 1、下载文档前请自行甄别文档内容的完整性,平台不提供额外的编辑、内容补充、找答案等附加服务。
- 2、"仅部分预览"的文档,不可在线预览部分如存在完整性等问题,可反馈申请退款(可完整预览的文档不适用该条件!)。
- 3、如文档侵犯您的权益,请联系客服反馈,我们会尽快为您处理(人工客服工作时间:9:00-18:30)。
Classical Power Spectrum EstimationAbstract With the increasing need of spectrum, various computational methods and algorithms have been proposed in the literature. Keeping these views and facts of spectrum shaping capability by FRFT based windows we have proposed a closed form solution for Bartlett window in fractional domain. This may be useful for analysis of different upcoming generations of mobile communication in a better way which are based on OFDM technique. Moreover, it is useful for real-time processing of non-stationary signals. As per our best knowledge the closed form solution mentioned in this paper have not been reported in the literature till date.This paper focuses on classical period spectral estimation and moderu spectral estimation based on Burg algorithm. By comparing various algorithms in computational complexity and resolution, Burg algorithm was used to signal processing finally. Experimental and simulation results indicated that digital signal processing system would meet system requirements for measurement accuracy.Keywords periodogram spectral estimation ; Burg algorithmI. INTRODUCTIONWhen we expand the frequency response of any digital filter by means of Fourier series, we get impulse response of the digital filter in the form of coefficients of the Fourier series. But the resultant filter is unrealizable and also its impulse response in infinite in duration. If we directly truncate this series to a finite number of points we have to face with well known Gibbs phenomenon, so we modify the Fourier coefficients bymultiplying it with some finite weighing sequence called window functions, w(n). One desirable characteristics of the Fourier transform of most of the window functions comprises of a central or main lobe of small width containing most of its energy.. Also its side lobes should decay rapidly as the frequency tends to π.1. PERIODOGRAM SPECTRAL ESTIMATIONIF signal by A/u conversion obtain a group of sample data x(1), x(2) ... , through the power spectrum estimates, give the energy of analyzed signal with the frequency distribution and analyze the signal frequency components. Classical power spectrum estimation has two main methods, namely, direct method and auto-correlation method. They are kind of non-parametric methods whose features have nothing to do with any model parameters. Non-parametric spectrum estimation signal extends with N points for the cycle, so it is also known as periodogram. Direct method is regard the N-point observation data X N (n )of the random signal x(n) as an energy limited signal, having direct access to Fourier transform X N (e jw) of X N (n ), and then make the square o f amplitude divided by N as the real power spectrum estimation Of X N (n ).Spectral estimation methods include the following assumptions and steps.The stationary random signal X(n) is regarded as the state traversal , using a sample x(n) instead of X(n), and then use N observations xN(n) to estimate the power spectrum p(w) of x(n). Using the record of acontinuous signal x(t) to estimate P PER(w) , Also including the discretization(A/D), the necessary pretreatment (such as filtering).2. Auto-correlation methodAuto-correlation method (also called the indirect method), A first, X N(n) is estimated from auto-correlation function rem) , then according to Wiener-Khintchine theorem, to get power spectrum of X N(n) by Fourier transform Of r(m) as estimation of p(w) .Computation is not very big when M is small. When M is relatively large, especially near N-l, the estimated deviations of r(m) become larger, which make the estimated quality of power spectrum decline. Therefore, usually take M <<N-l in the auto-correlation method, that is, the maximum length 2N-l of auto-correlation function for truncated, its effect is equivalent to impose a window function, recorded as v( m) .It can be proved that the first window, the mean is equal the trueauto-correlation function r(m) multiplied by the triangular window. The triangular window is generated by data truncation with the width of 2N-1. Where v(m) is the second window of auto-correlation function with the width of 2M-I, M <<N-l. Since the width of v(m) is much smaller than w(m) , the main lobe width of the spectrum v(m) of w(m) will be much greater than spectrum W(w) . Thus, the effect on r(m) imposed by v(m) is equivalent to convolution for P per(w) and v(m) in the frequency domain. Whose role is smoothing periodogram. When M<<N-l, P BT (w) actually smooth periodogram. It can be achieved by directly multiplied by the data window in the x N(n), but this calculation more than the previous[3-4].3. Spectral Estimation of Burg AlgorithmAssumed that the research process x(n) is the output of a linear system H(Z) by stimulated an input sequence u(n), H(Z) is estimated by the known x(n) or r x(m) , and then estimates the power spectrum of x(n) from H(Z) .u(n) is a white noise sequence, the variance is 2, zero of A(Z) and B(Z) should be in the unit circle in order to ensure the stability of H(Z) and the smallest phase system.If known a1,a2.......a p,b1,b2......b q, the power spectrum can be obtained.The basic idea of Burg algorithm is to average power of forward and backward prediction error minimum. Burg definition of m-order forward and backward error is:The filter output can be obtained by substituting Levision recurrence formula. Defined average power of m-order forward and backward prediction error, if m p∂/m k∂=0, thatThe Burg recursive algorithm step is as follows. Firstly, initial value Po and reflection coefficient k m are calculated according to the above equation, then obtained filtering coefficient of forward prediction and prediction error power Pm . Finally, the filtering output is:Repeat the above step, until the prediction error power is no longer obviously reduced, when calculating the order of p,assumed that 111----<=m m m m m p p k p p α, where α is the controlling parameter. According to actual requirements, if the velocity error <0.3%, it is desirable α = 0.001, then the prediction error power P m is white noise variance σ2 . All the orders of AR parameters, and power spectrum was obtained [5-7] .4.Bartlett windowBartlett windowin time domain is given in Fig. 1 followed by MSLL plots and SLFOR plots in Fig. 2 and Fig. 3 respectively for a particular value of the tunable parameter a . In Fig. 4 and Fig. 5 , three dimensional plots of the Bartlett window taking amplitude and normalized frequency for different values of the tunable parameter a is drawn. Table I comprises of all the tabulated values of MSLL, HMLW, SLFOR, width of the main lobe at -3dB and -6dB down from the peak of the main lobe for different values ofa .The width of the main lobe limits the frequency resolution of the resultant windowed signal. As the width of the main lobe becomes narrow we are able to distinguish clearly between two adjacent placed frequency components. But on the other side narrowing of the main lobe results in spectral leakage i.e. energy of the window spreads into side lobes so a trade off is there and the window which is best suited for a particular application is chosen. We see as thevalue of a decreases from 0.9 to 0.1 the value of MSLL varies from -13 dB to -11.9 dB approximately and -3dB bandwidth and -6dB bandwidth decreases from 0.1441 to 0.02355 and 0.18825 and 0.0294 respectively. Side-lobe fall off-rate is also varied from -14dB/octave to -22dB/octave and then to -17.5 db/octave as a is decreased.Figure 1. (Time domain Bartlett window)Figure 2.(MSLL Plot for a =0.7)Figure 3.(SLFOR plot for a =0.7)Figure 4. (3- dim. Continuous values of FRFT Bartlett window)Figure 5. (3- dim. Continuous values of FRFT Bartlett window)II. SIMULATION ANALYSISSimulation results of several power spectrum algorithms are shown in Fig. 1-6. The following conclusions can be drawn through observing data processing results of various methods to different SNR.1) The frequency error is small using classical spectral estimation methods (FFT, periodogram) for different signal to noise ratio data estimation, but the resolution is low, not enough to smooth the power spectrum graphics.2) Modem Spectral Estimation of MUSIC method and the modified covariance method has higher resolution, smaller error in lower order, more smooth power spectrum, but has generally larger calculation.Figure I. Periodogram spectral power estimationFigure 2. Yule-walker power spectrum estimationFigure 3. Burg spectrum estimation(30-order)Figure 4. Burg spectrum estimation(50-order)Figure 5. Modified covariance power spectrum estimationFigure 6. MUSIC power spectrum estimation3)The processing results of Burg spectrum estimation (50 order) for high signal to noise ratio (10dB, 5dB) is good, smoother graphics, the maximum frequency of power spectrum can accurately identify. Peak deviation is small, the resolution can improve by increasing the order of AR model. Therefore, Burg algorithm is a more common method and has a better quality of spectral estimation to reach system requirements .III. CONCLUSIONSignal processing of speed radar generally uses the power spectrum estimation method. Classical method, whether direct or indirect methods, are available to Fast Fourier Transform (FFT) calculation. But the spectral resolution is low, the resolution is proportional to 27r / N . Compared with classical power spectrum estimation method, the parameter model power spectrum estimation has obvious advantages both the variance performance or in the resolution. Simulation result is shown that Burg algorithm has obvious advantages for spectral resolution andmodel order, which can meet system requirements for measurement accuracy.REFERENCES[1] Huaigao Zhou, Analysis of Multi-moving targets spectrum and Design of DSP-Based Radar. Hunan University School of Electrical and InfOimation Engineering.2002.[2] Guangshu HU,Digital signal processing -theOly. algorithm and implementation. Beijing, Tsinghua University Press,1997[3] S.M Kay. Theory and Application of Modem Spectral Estimation. Science Press, Bei jing, 1997,170-200[4] Kay S M.Malple S L Spectnun Analysis-a Modem Perspective 1981(04)[5] Zhenyang Wu.Application of Golay complementary sequences in the spectrU1ll analysis. Journal of Electronic Measurement and Instruments. 1996, (3)14-17[6] Feng Luo. Research on shOit sequence power spectnllll estimates based on the Burg algorithm. Journal of xidian university(natural science) ,2005. 32(5) : 724-728.[7] K. Singh, Performance of discrete fractional Fourier transform classes in signal processing applications, Ph.D. thesis, Thapar University, Patiala, 2006.[8] H.M. Ozaktas, Z. Zalevsky, M.A. Kutay, The Fractional Fourier Transform with Applications in Optics and Signal Processing, first ed., Wiley, New York, 2001.。