计算机视觉课件4
合集下载
计算机视觉课件课件1210v14

W代表权值的总数,Pi代表第i层的感知器数量: 当网络层数不多的时候,随着感知器单元数量的增加,权值数目是平方增加的趋势
、
4.3 前向传播与反向传播算法
随着神经网络的崛起而名声大噪的方法
前向传播算法
假设上一层结点i, j, k,…与本层的结点w有连接,结点w的计算方法就是通过上一层的i, j, k等结点以及对应的连接权值进行加权和运算, 最终结果再加上一个偏置项(图中为了简单省略了),最后通过一个非线性函数(即激活函数),如ReLU,sigmoid等函数,得到的 结果就是本层结点w的输出。最终通过逐层运算的方式,得到输出层结果。
正向传播后: 反向传播:
链式推导:
最终结果:
更新权值:
、
4.4 卷积神经网络概述
计算机视觉核心网络。
卷积神经网络概述
卷积神经网络是一种前馈神经网络,它的人工神经元可以响应一部分覆盖范围内的周围单元,对于大型图像处理有出色表现。 卷积神经网络由一个或多个卷积层和顶端的全连通层(对应经典的神经网络)组成,同时也包括关联权重和池化层。这一结构使得卷 积神经网络能够利用输入数据的二维结构,其中最早比较有名的卷积神经网络为LeNet-5。与其他深度学习结构相比,卷积神经网络在 图像和语音识别方面能够给出更好的结果。这一模型也可以使用反向传播算法进行训练。相比较其他深度、前馈神经网络,卷积神经 网络需要考量的参数更少,使之成为一种颇具吸引力的深度学习结构。
卷积神经网络架构回顾
输入层 -> [[卷积层 -> 线性整流层]*N -> 池化层?]*M -> [全连接层 -> 线性整流层]*K -> 全连接层 堆叠几个卷积和整流层,再加一个池化层,然后再用全连接层控制输出。 上述表达式中,问号符号代表0次或1次,符号N和M则代表具体的数值。通常情况下,取N >= 0 && N <= 3,M >= 0,K >= 0 && K < 3。
、
4.3 前向传播与反向传播算法
随着神经网络的崛起而名声大噪的方法
前向传播算法
假设上一层结点i, j, k,…与本层的结点w有连接,结点w的计算方法就是通过上一层的i, j, k等结点以及对应的连接权值进行加权和运算, 最终结果再加上一个偏置项(图中为了简单省略了),最后通过一个非线性函数(即激活函数),如ReLU,sigmoid等函数,得到的 结果就是本层结点w的输出。最终通过逐层运算的方式,得到输出层结果。
正向传播后: 反向传播:
链式推导:
最终结果:
更新权值:
、
4.4 卷积神经网络概述
计算机视觉核心网络。
卷积神经网络概述
卷积神经网络是一种前馈神经网络,它的人工神经元可以响应一部分覆盖范围内的周围单元,对于大型图像处理有出色表现。 卷积神经网络由一个或多个卷积层和顶端的全连通层(对应经典的神经网络)组成,同时也包括关联权重和池化层。这一结构使得卷 积神经网络能够利用输入数据的二维结构,其中最早比较有名的卷积神经网络为LeNet-5。与其他深度学习结构相比,卷积神经网络在 图像和语音识别方面能够给出更好的结果。这一模型也可以使用反向传播算法进行训练。相比较其他深度、前馈神经网络,卷积神经 网络需要考量的参数更少,使之成为一种颇具吸引力的深度学习结构。
卷积神经网络架构回顾
输入层 -> [[卷积层 -> 线性整流层]*N -> 池化层?]*M -> [全连接层 -> 线性整流层]*K -> 全连接层 堆叠几个卷积和整流层,再加一个池化层,然后再用全连接层控制输出。 上述表达式中,问号符号代表0次或1次,符号N和M则代表具体的数值。通常情况下,取N >= 0 && N <= 3,M >= 0,K >= 0 && K < 3。
人工智能导论课件4 计算机视觉_2019
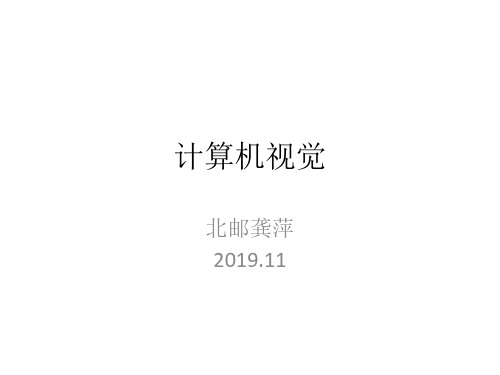
计算机视觉解决的主要问题是: 给出一张二维图像,计算机视觉系统必
须识别出图像中的对象及其特征,如形状、 纹理、颜色、大小、空间排列等,从而尽可 能完整地描述该图像。
计算机视觉典型任务
计算机视觉典型任务
区分计算机视觉与其相关领域 • 图像处理
图像处理旨在处理原始图像以应用某种变换。其目标 通常是改进图像或将其作为某项特定任务的输入,而计算 机视觉的目标是描述和解释图像。例如,降噪、对比度或 旋转操作这些典型的图像处理组件可以在像素层面执行, 无需对图像整体具备全面的了解。
• 还要标出每个对象的边界。因此,与分类目的不同,相关模型要具有像素级的密集预 测能力
计算机视觉
• 计算机视觉典型任务 -图像分类 -定位 -目标检测 -语义分割 • 图像处理基本原理 • 计算机视觉基本原理
-通用策略 -现有数据集
• 目标检测原理及模型
-边界框、锚框 -F-RCNN -YOLO系列 -SSD
目标矩形框 – 例如,人脸检测(人脸为目标、 背景为非目标)、汽
车检测(汽车为目标、 背景为非目标)
计算机视觉典型任务
• 语义分割(semantic segmentation)
– 需要对图像的每一个像素点进行分类 – 这里的类别为:多个目标类别和多个非目标类别
• 左边为输入图像,右边为经过语义分割后的输出图像,该模型不仅要识别出摩托车和 驾驶者
• 语义分割
-目标识别 -目标追踪
图像处理基本原理
图像
• 什么是图像?
光学图像 Lena
IKONOS卫星 光学图像 423mile 高 16000miles/h 1m分辨率 EP-3, 海南陵水,01-4-4
大脑断层图 像
25km x 75km
须识别出图像中的对象及其特征,如形状、 纹理、颜色、大小、空间排列等,从而尽可 能完整地描述该图像。
计算机视觉典型任务
计算机视觉典型任务
区分计算机视觉与其相关领域 • 图像处理
图像处理旨在处理原始图像以应用某种变换。其目标 通常是改进图像或将其作为某项特定任务的输入,而计算 机视觉的目标是描述和解释图像。例如,降噪、对比度或 旋转操作这些典型的图像处理组件可以在像素层面执行, 无需对图像整体具备全面的了解。
• 还要标出每个对象的边界。因此,与分类目的不同,相关模型要具有像素级的密集预 测能力
计算机视觉
• 计算机视觉典型任务 -图像分类 -定位 -目标检测 -语义分割 • 图像处理基本原理 • 计算机视觉基本原理
-通用策略 -现有数据集
• 目标检测原理及模型
-边界框、锚框 -F-RCNN -YOLO系列 -SSD
目标矩形框 – 例如,人脸检测(人脸为目标、 背景为非目标)、汽
车检测(汽车为目标、 背景为非目标)
计算机视觉典型任务
• 语义分割(semantic segmentation)
– 需要对图像的每一个像素点进行分类 – 这里的类别为:多个目标类别和多个非目标类别
• 左边为输入图像,右边为经过语义分割后的输出图像,该模型不仅要识别出摩托车和 驾驶者
• 语义分割
-目标识别 -目标追踪
图像处理基本原理
图像
• 什么是图像?
光学图像 Lena
IKONOS卫星 光学图像 423mile 高 16000miles/h 1m分辨率 EP-3, 海南陵水,01-4-4
大脑断层图 像
25km x 75km
计算机视觉(4)PPT
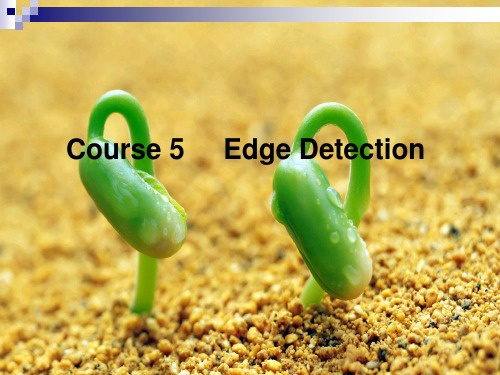
1) Goal: 2) Given an image corrupted by acquisition noise,
locate the edges most likely to be generated by scene elements not by noise.
2) Operations required in edge detection
2 f
2 f
2 f
x2 y2
In discrete computation:
f 2 f[i, j 1]2f[i, j] f[i, j 1]
x2 f 2 f[i 1, j]2f[i, j] f[i 1, j] y2
Or, by template,
010
2 1 -4 1
010
4. LapLacian of Gaussian (LOG)
LOG combines Guassian filter with the LapLasian for edge detection:
iii) Edge Location: Decide which local maximum in the filter’s output are edges and which are just caused by noise.
3) Edge descriptions:
edge normal: the direction (unit vector) of the maximum intensity variation at edge pixels. Edge normal is perpendicular to the edge.
Course 5 Edge Detection
Course 5 Edge Detection
locate the edges most likely to be generated by scene elements not by noise.
2) Operations required in edge detection
2 f
2 f
2 f
x2 y2
In discrete computation:
f 2 f[i, j 1]2f[i, j] f[i, j 1]
x2 f 2 f[i 1, j]2f[i, j] f[i 1, j] y2
Or, by template,
010
2 1 -4 1
010
4. LapLacian of Gaussian (LOG)
LOG combines Guassian filter with the LapLasian for edge detection:
iii) Edge Location: Decide which local maximum in the filter’s output are edges and which are just caused by noise.
3) Edge descriptions:
edge normal: the direction (unit vector) of the maximum intensity variation at edge pixels. Edge normal is perpendicular to the edge.
Course 5 Edge Detection
Course 5 Edge Detection
《计算机视觉》PPT课件
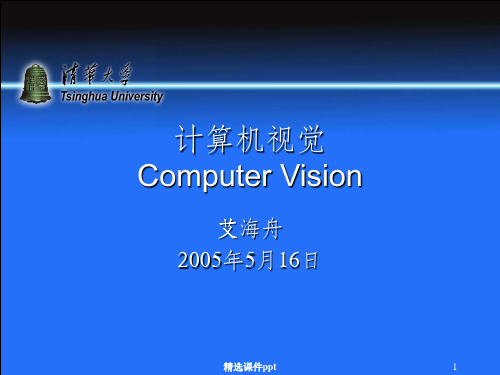
fucntion)
精选课件ppt
11
Overview (3)
计算机视觉的图像模型基础
✓ 摄像机模型及其校准
▪ 内参数、外参数
✓ 图像特征
▪ 边缘、角点、轮廓、纹理、形状…
✓ 图像序列特征 (运动)
▪ 对应点、光流
精选课件ppt
12
Overview (4)
计算机视觉的信号处理层次
低层视觉处理
✓ 单图像:滤波/边缘检测/纹理
计算机视觉的基本的分析工具和数学模型 Signal processing approach: FFT, filtering, wavelets, … Subspace approach: PCA, LDA, ICA, … Bayesian inference approach: EM, Condensation/SIS/…, MCMC, …. Machine learning approach: SVM/Kernel machine, Boosting/Adaboost, NN/Regression, … HMM, BN/DBN, … Gibbs, MRF, …
✓ 多图像:几何/立体/从运动恢复仿射或透视结构 affine/perspective structure from motion
中层视觉处理
✓ 聚类分割/拟合线条、曲线、轮廓 clustering for segmentation, fitting line…
✓ 基于概率方法的聚类分割/拟合
✓ 跟踪 tracking
精选课件ppt
6
Tools
Intel OpenCV, IPL
✓ Camera calibration (Zhang Zhengyou’s method) ✓ Face detection (a variation of Viola’s) ✓ Motion analysis and object tracking
精选课件ppt
11
Overview (3)
计算机视觉的图像模型基础
✓ 摄像机模型及其校准
▪ 内参数、外参数
✓ 图像特征
▪ 边缘、角点、轮廓、纹理、形状…
✓ 图像序列特征 (运动)
▪ 对应点、光流
精选课件ppt
12
Overview (4)
计算机视觉的信号处理层次
低层视觉处理
✓ 单图像:滤波/边缘检测/纹理
计算机视觉的基本的分析工具和数学模型 Signal processing approach: FFT, filtering, wavelets, … Subspace approach: PCA, LDA, ICA, … Bayesian inference approach: EM, Condensation/SIS/…, MCMC, …. Machine learning approach: SVM/Kernel machine, Boosting/Adaboost, NN/Regression, … HMM, BN/DBN, … Gibbs, MRF, …
✓ 多图像:几何/立体/从运动恢复仿射或透视结构 affine/perspective structure from motion
中层视觉处理
✓ 聚类分割/拟合线条、曲线、轮廓 clustering for segmentation, fitting line…
✓ 基于概率方法的聚类分割/拟合
✓ 跟踪 tracking
精选课件ppt
6
Tools
Intel OpenCV, IPL
✓ Camera calibration (Zhang Zhengyou’s method) ✓ Face detection (a variation of Viola’s) ✓ Motion analysis and object tracking
计算机视觉技术 ppt课件
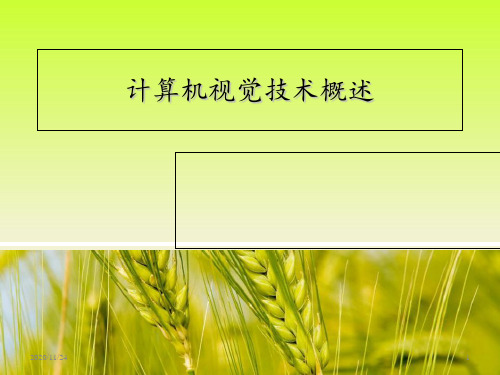
2020/11/24
13
计算机视觉的发展趋势
目前,过去由于CPU处理能力强大,可以进行较为 复杂的图像处理,并且一个Pc可支持多个相机进行多 方位的检测,因此PC Based方案受到了广大厂商的青 睐。目前国内多数厂商对计算机视觉的认识,已不仅 仅停留在PC Based方案层面。嵌入式方案越来越引起 厂商们的重视,其具有更大的灵活性,成本又低于PC Based方案,就抗干扰能力来说,嵌入式方案也更能适 应工业环境的电子干扰、温度变化、供电电压波动等 多种干扰,因此,目前计算机视觉正在向嵌入式的方 向发展。
2020/11/24
12
5. 交通: 汽车车牌识别、高速公路收费、违章闯红灯检 测、交通管制系统等。采用智能交通管理系统,通过在 交通要道放置摄像头,当有违章车辆(如闯红灯) 时,摄像 头将车辆的牌照拍摄下来,传输给中央管理系统,系统利 用图像处理技术,对拍摄的图片进行分析,提取出车牌号, 存储在数据库中,可以供管理人员进行检索。 6. 商标管理:可以建立商标图像库,利用图像检索技术, 对新申请的商标与图像库里的注册商标进行分析,检查 是否设计相似或雷同。
计算机视觉技术概述
2020/11/24
1
学习内容:
★计算机视觉技术的定义 ★计算机视觉技术的发展 ★计算机视觉技术的应用 ★计算机视觉技术的图像处理方法 ★计算机视觉技术的发展趋势
2020/11/24
2
精品资料
• 你怎么称呼老师?
• 如果老师最后没有总结一节课的重点的难点,你 是否会认为老师的教学方法需要改进?
• 你所经历的课堂,是讲座式还是讨论式? • 教师的教鞭
• “不怕太阳晒,也不怕那风雨狂,只怕先生骂我 笨,没有学问无颜见爹娘 ……”
计算机视觉预备知识PPT课件
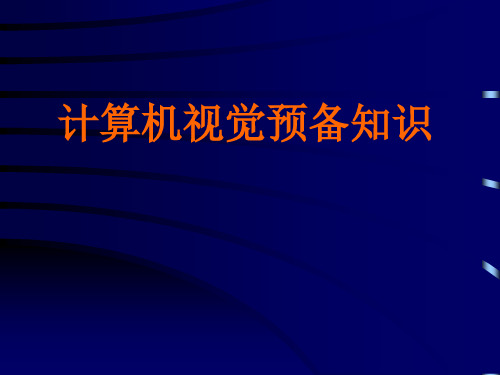
计算机视觉基本特点
• 分阶段信息处理,多层次信息表示 • 绝大部分问题为病态问题 • 原始信息被污染,信息处理量巨大 • 一种知识导引与管理系统 • 多学科交叉与结合
实用图像处理技术
灰度图像 彩色图像 二值化图像
图像的增强
1.直方图的均衡化
2.平滑滤波Βιβλιοθήκη 原图噪声污染后的图像
均值滤波后的图像
中值滤波后的图像
I
1RGB
3
Ri,Gi,Bi
RG BHSI
Hi,Si,Ii
H S I
Ri,Gi,Bi
H i H ,S i S ,Ii I
RG BHSI
颜色模型的转换 图5.30划分HSI空间的方法
计算机视觉预备知识
暂时到此
返回
返回
图像处理
• 由于实际景物转换为图像信号时,总会引入各种噪声 或畸变失真,一般需要先进行图像处理。
• 4.视觉的恒常性 1).亮度恒常性
例如:一匹黑布和一匹白布
原因:先验知识 ;本体反射率。 2).大小恒常性
例如:远处的牛比近处的狗要大
3).形状恒常性 4).颜色恒常性
5.错视现象 (a)高度错视; (b)长度错视; (c)平行线错视; (d)大小错视; (e)主从错视;
计算机视觉
计算机视觉是指用计算机实现人的视 觉功能——对客观世界的三维场景的感知、 识别和理解。
计算机视觉系统的组成及原理
图像 输入
数字化
图像 处理
图像 分析
图像 理解
结果 输出
人机交互处理
计算机数字图象处理系统
摄像头 A/D
监视器
D/A
帧存
微 机 接 口
LUT
《计算机视觉》PPT课件
实例:雷达测距系统
computer vision
3
7.1 立体视觉基础
被动测距方法
双目视觉系统:使用两个相隔一定距离的 摄像机同时获取场景图像来生成深度图。
单目运动视觉:一个摄像机在不同空间位 置上获取两幅或两幅以上图像,通过多幅 图像的灰度信息和成像几何来生成深度图
特征深度测量:使用灰度图象的明暗特征、 纹理特征、运动特征间接的估算深度信息。
14
7.2 立体成像
依据双目立体视觉几何关系的深度计算
结合以下公式:
x
x
l
zF
x B x r zF
可以得到: z B F x l x r
其中F是焦距,B是基线距离, xl 是xr 视差。
各种场景中的点的深度就可以通过计算视差来实 现。视差一般是整数。
对于一组给定的摄像机参数,提高场景点深度计
即使两个摄像机处于一般的位置和方向时, 对应场景点的两个图像点仍然位于图像平 面和外极平面的交线(外极线)上。
computer vision
20
7.3 立体成像
从原理上讲根据“立体图象对”抽取深度信息的 处理应包括以下四部分:
在图象中寻找在两幅图象中都便于区分的特征或 用于匹配的基元(primitive)。
立体匹配的匹配规则约束
立体匹配:立体成像的深度信息测量的一个重要 步骤就是寻找立体成像对中的共轭对,即求解对 应问题。
问题:实际中求解对应问题是非常困难的,一是 计算量大,二是匹配的准确度要求高。
解决:为了求解对应,建立了许多约束来减少对 应点误匹配,并最终得到正确的匹配特征点的对 应。
computer vision
computer vision
4
计算机视觉课件精品文档
(7) Perimeter
Def.1: The total length of lines that separate pixels
of S from S .
Def.2: The number of boundary pixels.
(8) Compactness
P2
c
A
Where P— perimeter, A— area. The smallest value of
Horizontal projection:
H[i]B[i, j]
j
Vertical projection:
V[j]B[i, j]
j
For a well alined text binary image, the projection has comb shape, image can be easily partitioned.
b. 8-neighbors: the 4-neighbors pixels plus the diagonal neighbors.
[i-1,j]
[i-1,j-1] [i-1,j] [i-1,j+1]
[i,j-1] [i,j] [i,j+1]
[i,j-1]
[i,j]
[i,j+1]
[i+1,j]
[i+1,j-1] [i-1,j] [i+1,j+1]
(3) Boundary-following:
Track boundary pixels of a region in a particular order (chain sequence)
Algorithm:
a) Use systematic scan (from left to right, and from top
计算机视觉 ppt课件
绪论
(2.27,3.2)
(5 lectures) 视觉基本特性I 生物特性
Week 2
视觉基本特性II
(3.6,3.9) 物理特性
(5 lectures) 视觉基本特性III 几何特性
Week 3
图像处理基础I
(3.13,3.16) 空域处理
(5 lectures) 图像处理基础II 频域处理
Week 4
图像多义性: 三维场景被投影为二维图像,深度和不可 见部分的信息被丢失,因而会出现不同形状的三维物体投 影在图像平面上产生相同图像的问题.另外,在不同角度 获取同一物体的图像会有很大的差异.
环境因素影响:场景中的诸多因素,包括照明、物体形状、 表面颜色、摄像机以及空间关系变化都会对成像有影响.
计算机视觉的任务是用图像创建或恢复现实世界模 型,然后认知现实世界。
具体来说,让计算机具有对周围世界的空间物体进 行传感、抽象、判断的能力,从而达到识别、理解 的目的。
分为三个阶段
◦ 特征提取和区域分割
基于轮廓,纹理,颜色…
◦ 建模与模式表达
基于各种物体的抽象化模 型
◦ 描述和理解
主讲: 曹洋 forrest@ 办公室:科技楼西楼303
课程教材:
使用教材: Richard Szeliski , Computer Vision: Algorithms and Applications,Springer,2010 参考教材:
David A. Forsyth, Jean Ponce著,计算机视觉(一种现 代方法),电子工业出版社 2004。
上世纪60年代,拓展到三维结构,对物体的形状, 物体的空间关系进行描述。通过对积木世界的研 究,引出了边缘、角点等特征提取,图像明暗、 纹理、运动以及成像几何等研究工作。
计算机视觉课件
许多深度学习算法的可解释性较差,难以理解其决策过程和原理,这限制了其在 一些需要解释的场景中的应用。
鲁棒性差
计算机视觉算法在面对复杂环境和噪声干扰时,容易出现误判和失效,鲁棒性有 待提高。
多模态信息融合与跨域问题
多模态信息融合
计算机视觉任务通常涉及多种模态的信息, 如图像、文本、音频等,如何有效地融合这 些信息以提高任务性能是一个挑战。
安全与隐私
随着智能监控的普及,安全与隐私保护也成为了计算机视觉领域的一个重要研究方向,涉及到视频数 据的加密、水印、隐私保护等方面的技术。
医学影像分析
医学影像分析
计算机视觉技术在医学影像分析中发挥着重 要作用,通过对医学影像进行自动分析和识 别,可以辅助医生进行疾病诊断和治疗。
图像分割和识别
在医学影像分析中,图像分割和识别是两个 重要的任务,通过对医学影像进行分割和分 类,可以提取出病变区域和器官等重要信息 ,为医生提供更加准确的诊断依据。
04
计算机视觉技术前沿
深度学习在计算机视觉中的应用
深度学习技术
深度学习在计算机视觉领域的应用已经取得了显著的进展,通过构建深度神经网络,可以 自动提取图像中的特征,实现各种复杂的视觉任务,如目标检测、图像识别、图像生成等 。
卷积神经网络(CNN)
CNN是深度学习在计算机视觉领域中最常用的模型之一,它通过模拟人眼视觉细胞的层 级结构,能够从原始图像中逐层提取越来越抽象的语义信息,从而实现对图像的分类、检 测、分割等任务。
未来趋势
随着深度学习等技术的突破,计 算机视觉将在更多领域得到应用 ,并不断提升其准确性和智能化 水平。
应用领域
工业自动化
计算机视觉在工业自动化领域 应用广泛,如生产线上的质量
鲁棒性差
计算机视觉算法在面对复杂环境和噪声干扰时,容易出现误判和失效,鲁棒性有 待提高。
多模态信息融合与跨域问题
多模态信息融合
计算机视觉任务通常涉及多种模态的信息, 如图像、文本、音频等,如何有效地融合这 些信息以提高任务性能是一个挑战。
安全与隐私
随着智能监控的普及,安全与隐私保护也成为了计算机视觉领域的一个重要研究方向,涉及到视频数 据的加密、水印、隐私保护等方面的技术。
医学影像分析
医学影像分析
计算机视觉技术在医学影像分析中发挥着重 要作用,通过对医学影像进行自动分析和识 别,可以辅助医生进行疾病诊断和治疗。
图像分割和识别
在医学影像分析中,图像分割和识别是两个 重要的任务,通过对医学影像进行分割和分 类,可以提取出病变区域和器官等重要信息 ,为医生提供更加准确的诊断依据。
04
计算机视觉技术前沿
深度学习在计算机视觉中的应用
深度学习技术
深度学习在计算机视觉领域的应用已经取得了显著的进展,通过构建深度神经网络,可以 自动提取图像中的特征,实现各种复杂的视觉任务,如目标检测、图像识别、图像生成等 。
卷积神经网络(CNN)
CNN是深度学习在计算机视觉领域中最常用的模型之一,它通过模拟人眼视觉细胞的层 级结构,能够从原始图像中逐层提取越来越抽象的语义信息,从而实现对图像的分类、检 测、分割等任务。
未来趋势
随着深度学习等技术的突破,计 算机视觉将在更多领域得到应用 ,并不断提升其准确性和智能化 水平。
应用领域
工业自动化
计算机视觉在工业自动化领域 应用广泛,如生产线上的质量
- 1、下载文档前请自行甄别文档内容的完整性,平台不提供额外的编辑、内容补充、找答案等附加服务。
- 2、"仅部分预览"的文档,不可在线预览部分如存在完整性等问题,可反馈申请退款(可完整预览的文档不适用该条件!)。
- 3、如文档侵犯您的权益,请联系客服反馈,我们会尽快为您处理(人工客服工作时间:9:00-18:30)。
——if color is gray, go to step b). d) Stop if all nodes are either black or white.
4) Region Adjacency Graph: —— Emphasize the adjacency of regions —— node: region —— link: common boundary between regions.
— same as binary image representation
— often be used as a mask over intensity image to select the region for proper processing.
2) Pyramids representation
E (T ) P 2 E 1 (T ) P 1 E 2 (T )
T should be chosen such that
E(T)min
From
E(T) 0, T
Get
P 1p1(T)P 2p2(T)
Applying Gaussian distributions, get:
A2T B TC0
Where:
Course 4 Regions
Course 4. Regions
Def. of Region: A set of connected image pixels,
with similar properties. • similar gray level • similar color • similar texture so on ……
they are similar. —— For the region that are similar, merge them and
modify the adjacency graph. d) Repeat step c) until no region are merged. Criteria for merge:
Where P(.) is homogeneity predicate measurement.
1) Thresholding:
2) (1) P-Tile Thresholing
3) If a pre-knowledge of object size S is given, the
4) area percentage of the object in image A can be got
(3) Adaptive Thresholding
The basic concept is to choose threshold T locally to against uneven distribution of image intensity (caused by uneven illumination in scene). • Partition an image into several regions . • In each region, choose threshold T at major valley of it histograph, and perform threholding in each region.
s— common edge length
T — threshold, e.g. T=0.75
How to measure the weakness of an edge?
I —preset value
i.e., the gradient of image intensity.
3) Region Spliting:
5) Picture Tree: —— Emphasize region inclusion within another region. —— Recursively split an image into regions, and the process stops when no region can be further splitted (homogeneity predicate)
1. Region Representation
1) Array representation:
Given a region R in image S,
Its array representation A is:
A a[i,j]
[i,j]S, a[i,j] 1 0 io[fi,tj]hR e rw
b) Make an adjacency graph for the image .
c) For each region in the image, do the following steps: —— Consider it adjacent regions, and check whether
A12 22
B2(112 222)
C1222
2212
21222
ln(2P1) 1P2
One can solve for T
In the case 12 22 2
We can get
T12 2 lnP(2) 2 12 P1
In most application, we just simply threshold an image at intensity T where histograph has a valley, which can approximate optimal thresholding.
not want.
• Merge operation combines regions that are considered similar.
Algorithm:
a) Form initial regions in the image using thresholding, followed by clustering labeling (find connected components).
Region may be separated adjacent overlapped included
Important principle of region process: ——homogeneity predicate (value similarity) ——special proximity
—— if some property of a region is not constant, the
region should be split —— difficulties:
how to measure “properties” how to find splitting boundary? Algorithm (split and merge): a) Set the whole image as a region R
2. Region Segmentation Suppose an image A is well segmented into n regions Ri, i=1,2,…,n, there must be:
n
Ri A
i 1
P ( R i ) True P ( R i R j ) False
S
1
2
3
4
1
2
3
4
Color of node:
wh.ia tellpixealrse"0" intheregi colo brla. cakllpixealrse"1" intheregi
gr.ayhavbeot"h0" and"1" pixels
Algorithm:
a) Set an image as a region, and represents it as a node in quad tree (0-level)
Pyramid representation of a NxN image (N=2k) contains k layers of reduced image. Each pixel in an upper layer image corresponds to 4 pixels in the lower layer image, i.e.,
Dark region: Bright region:
p1(Z)
1
(Zμ1)2
e 2σ12
2πσ1
p2(Z)
1
e(Z2σμ222)2
2πσ2
1 2
we set partition threshold at T, error probability of object being segmented as background:
5)
by T
P=S/A.
Thus, wTe
P
set the value
p()d
of
threshold
at
0
6) such that
p( )
L
0 p( ) 1
(2) Optimal thresholding:
Suppose an image contain only two principal brightness regions and both obey Gaussian distribution in intensity.
N N N N N N 1 1 22 44
Pixels value of upper layer image can be obtained by ——averaging the corresponding pixels of lower layer image, or ——subsampling (e.g. choose upper-left pixel value)
4) Region Adjacency Graph: —— Emphasize the adjacency of regions —— node: region —— link: common boundary between regions.
— same as binary image representation
— often be used as a mask over intensity image to select the region for proper processing.
2) Pyramids representation
E (T ) P 2 E 1 (T ) P 1 E 2 (T )
T should be chosen such that
E(T)min
From
E(T) 0, T
Get
P 1p1(T)P 2p2(T)
Applying Gaussian distributions, get:
A2T B TC0
Where:
Course 4 Regions
Course 4. Regions
Def. of Region: A set of connected image pixels,
with similar properties. • similar gray level • similar color • similar texture so on ……
they are similar. —— For the region that are similar, merge them and
modify the adjacency graph. d) Repeat step c) until no region are merged. Criteria for merge:
Where P(.) is homogeneity predicate measurement.
1) Thresholding:
2) (1) P-Tile Thresholing
3) If a pre-knowledge of object size S is given, the
4) area percentage of the object in image A can be got
(3) Adaptive Thresholding
The basic concept is to choose threshold T locally to against uneven distribution of image intensity (caused by uneven illumination in scene). • Partition an image into several regions . • In each region, choose threshold T at major valley of it histograph, and perform threholding in each region.
s— common edge length
T — threshold, e.g. T=0.75
How to measure the weakness of an edge?
I —preset value
i.e., the gradient of image intensity.
3) Region Spliting:
5) Picture Tree: —— Emphasize region inclusion within another region. —— Recursively split an image into regions, and the process stops when no region can be further splitted (homogeneity predicate)
1. Region Representation
1) Array representation:
Given a region R in image S,
Its array representation A is:
A a[i,j]
[i,j]S, a[i,j] 1 0 io[fi,tj]hR e rw
b) Make an adjacency graph for the image .
c) For each region in the image, do the following steps: —— Consider it adjacent regions, and check whether
A12 22
B2(112 222)
C1222
2212
21222
ln(2P1) 1P2
One can solve for T
In the case 12 22 2
We can get
T12 2 lnP(2) 2 12 P1
In most application, we just simply threshold an image at intensity T where histograph has a valley, which can approximate optimal thresholding.
not want.
• Merge operation combines regions that are considered similar.
Algorithm:
a) Form initial regions in the image using thresholding, followed by clustering labeling (find connected components).
Region may be separated adjacent overlapped included
Important principle of region process: ——homogeneity predicate (value similarity) ——special proximity
—— if some property of a region is not constant, the
region should be split —— difficulties:
how to measure “properties” how to find splitting boundary? Algorithm (split and merge): a) Set the whole image as a region R
2. Region Segmentation Suppose an image A is well segmented into n regions Ri, i=1,2,…,n, there must be:
n
Ri A
i 1
P ( R i ) True P ( R i R j ) False
S
1
2
3
4
1
2
3
4
Color of node:
wh.ia tellpixealrse"0" intheregi colo brla. cakllpixealrse"1" intheregi
gr.ayhavbeot"h0" and"1" pixels
Algorithm:
a) Set an image as a region, and represents it as a node in quad tree (0-level)
Pyramid representation of a NxN image (N=2k) contains k layers of reduced image. Each pixel in an upper layer image corresponds to 4 pixels in the lower layer image, i.e.,
Dark region: Bright region:
p1(Z)
1
(Zμ1)2
e 2σ12
2πσ1
p2(Z)
1
e(Z2σμ222)2
2πσ2
1 2
we set partition threshold at T, error probability of object being segmented as background:
5)
by T
P=S/A.
Thus, wTe
P
set the value
p()d
of
threshold
at
0
6) such that
p( )
L
0 p( ) 1
(2) Optimal thresholding:
Suppose an image contain only two principal brightness regions and both obey Gaussian distribution in intensity.
N N N N N N 1 1 22 44
Pixels value of upper layer image can be obtained by ——averaging the corresponding pixels of lower layer image, or ——subsampling (e.g. choose upper-left pixel value)