2011 Modality Focus Training Flowsensorik Chinese (NXPowerLite)
英语
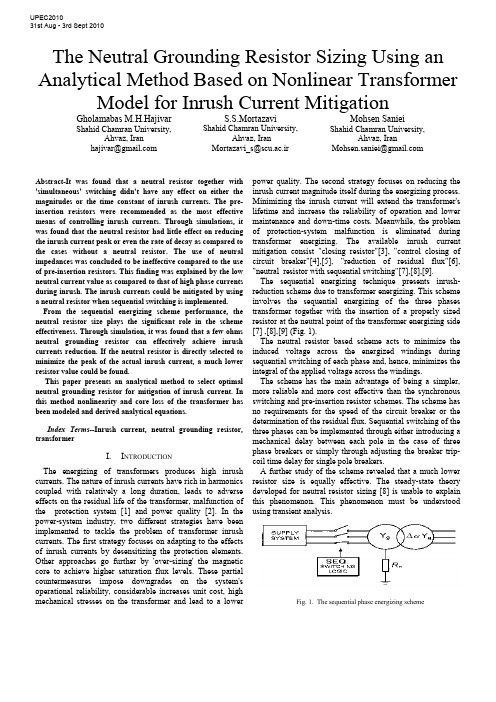
The Neutral Grounding Resistor Sizing Using an Analytical Method Based on Nonlinear Transformer Model for Inrush Current MitigationGholamabas M.H.Hajivar Shahid Chamran University,Ahvaz, Iranhajivar@S.S.MortazaviShahid Chamran University,Ahvaz, IranMortazavi_s@scu.ac.irMohsen SanieiShahid Chamran University,Ahvaz, IranMohsen.saniei@Abstract-It was found that a neutral resistor together with 'simultaneous' switching didn't have any effect on either the magnitudes or the time constant of inrush currents. The pre-insertion resistors were recommended as the most effective means of controlling inrush currents. Through simulations, it was found that the neutral resistor had little effect on reducing the inrush current peak or even the rate of decay as compared to the cases without a neutral resistor. The use of neutral impedances was concluded to be ineffective compared to the use of pre-insertion resistors. This finding was explained by the low neutral current value as compared to that of high phase currents during inrush. The inrush currents could be mitigated by using a neutral resistor when sequential switching is implemented. From the sequential energizing scheme performance, the neutral resistor size plays the significant role in the scheme effectiveness. Through simulation, it was found that a few ohms neutral grounding resistor can effectively achieve inrush currents reduction. If the neutral resistor is directly selected to minimize the peak of the actual inrush current, a much lower resistor value could be found.This paper presents an analytical method to select optimal neutral grounding resistor for mitigation of inrush current. In this method nonlinearity and core loss of the transformer has been modeled and derived analytical equations.Index Terms--Inrush current, neutral grounding resistor, transformerI.I NTRODUCTIONThe energizing of transformers produces high inrush currents. The nature of inrush currents have rich in harmonics coupled with relatively a long duration, leads to adverse effects on the residual life of the transformer, malfunction of the protection system [1] and power quality [2]. In the power-system industry, two different strategies have been implemented to tackle the problem of transformer inrush currents. The first strategy focuses on adapting to the effects of inrush currents by desensitizing the protection elements. Other approaches go further by 'over-sizing' the magnetic core to achieve higher saturation flux levels. These partial countermeasures impose downgrades on the system's operational reliability, considerable increases unit cost, high mechanical stresses on the transformer and lead to a lower power quality. The second strategy focuses on reducing the inrush current magnitude itself during the energizing process. Minimizing the inrush current will extend the transformer's lifetime and increase the reliability of operation and lower maintenance and down-time costs. Meanwhile, the problem of protection-system malfunction is eliminated during transformer energizing. The available inrush current mitigation consist "closing resistor"[3], "control closing of circuit breaker"[4],[5], "reduction of residual flux"[6], "neutral resistor with sequential switching"[7],[8],[9].The sequential energizing technique presents inrush-reduction scheme due to transformer energizing. This scheme involves the sequential energizing of the three phases transformer together with the insertion of a properly sized resistor at the neutral point of the transformer energizing side [7] ,[8],[9] (Fig. 1).The neutral resistor based scheme acts to minimize the induced voltage across the energized windings during sequential switching of each phase and, hence, minimizes the integral of the applied voltage across the windings.The scheme has the main advantage of being a simpler, more reliable and more cost effective than the synchronous switching and pre-insertion resistor schemes. The scheme has no requirements for the speed of the circuit breaker or the determination of the residual flux. Sequential switching of the three phases can be implemented through either introducing a mechanical delay between each pole in the case of three phase breakers or simply through adjusting the breaker trip-coil time delay for single pole breakers.A further study of the scheme revealed that a much lower resistor size is equally effective. The steady-state theory developed for neutral resistor sizing [8] is unable to explain this phenomenon. This phenomenon must be understood using transient analysis.Fig. 1. The sequential phase energizing schemeUPEC201031st Aug - 3rd Sept 2010The rise of neutral voltage is the main limitation of the scheme. Two methods present to control the neutral voltage rise: the use of surge arrestors and saturated reactors connected to the neutral point. The use of surge arresters was found to be more effective in overcoming the neutral voltage rise limitation [9].The main objective of this paper is to derive an analytical relationship between the peak of the inrush current and the size of the resistor. This paper presents a robust analytical study of the transformer energizing phenomenon. The results reveal a good deal of information on inrush currents and the characteristics of the sequential energizing scheme.II. SCHEME PERFORMANCESince the scheme adopts sequential switching, each switching stage can be investigated separately. For first-phase switching, the scheme's performance is straightforward. The neutral resistor is in series with the energized phase and this resistor's effect is similar to a pre-insertion resistor.The second- phase energizing is one of the most difficult to analyze. Fortunately, from simulation studies, it was found that the inrush current due to second-phase energizing is lower than that due to first-phase energizing for the same value of n R [9]. This result is true for the region where the inrush current of the first-phase is decreasing rapidly as n R increases. As a result, when developing a neutral-resistor-sizing criterion, the focus should be directed towards the analysis of the first-phase energizing.III. A NALYSIS OF F IRST -P HASE E NERGIZING The following analysis focuses on deriving an inrush current waveform expression covering both the unsaturatedand saturated modes of operation respectively. The presented analysis is based on a single saturated core element, but is suitable for analytical modelling of the single-phase transformers and for the single-phase switching of three-phase transformers. As shown in Fig. 2, the transformer's energized phase was modeled as a two segmented saturated magnetizing inductance in series with the transformer's winding resistance, leakage inductance and neutral resistance. The iron core non-l inear inductance as function of the operating flux linkages is represented as a linear inductor inunsaturated ‘‘m l ’’ and saturated ‘‘s l ’’ modes of operation respectively. (a)(b)Fig. 2. (a) Transformer electrical equivalent circuit (per-phase) referred to the primary side. (b) Simplified, two slope saturation curve.For the first-phase switching stage, the equivalent circuit represented in Fig. 2(a) can accurately represent behaviour of the transformer for any connection or core type by using only the positive sequence Flux-Current characteristics. Based on the transformer connection and core structure type, the phases are coupled either through the electrical circuit (3 single phase units in Yg-D connection) or through the Magnetic circuit (Core type transformers with Yg-Y connection) or through both, (the condition of Yg-D connection in an E-Core or a multi limb transformer). The coupling introduced between the windings will result in flux flowing through the limbs or magnetic circuits of un-energized phases. For the sequential switching application, the magnetic coupling will result in an increased reluctance (decreased reactance) for zero sequence flux path if present. The approach presented here is based on deriving an analytical expression relating the amount of inrush current reduction directly to the neutral resistor size. Investigation in this field has been done and some formulas were given to predict the general wave shape or the maximum peak current.A. Expression for magnitude of inrush currentIn Fig. 2(a), p r and p l present the total primary side resistance and leakage reactance. c R shows the total transformer core loss. Secondary side resistance sp r and leakage reactance sp l as referred to primary side are also shown. P V and s V represent the primary and secondary phase to ground terminal voltages, respectively.During first phase energizing, the differential equation describing behaviour of the transformer with saturated ironcore can be written as follows:()())sin((2) (1)φω+⋅⋅=⋅+⋅+⋅+=+⋅+⋅+=t V (t)V dtdi di d λdt di l (t)i R r (t)V dt d λdt di l (t)i R r (t)V m P ll p pp n p P p p p n p PAs the rate of change of the flux linkages with magnetizing current dt d /λcan be represented as an inductance equal to the slope of the i −λcurve, (2) can be re-written as follows;()(3) )()()(dtdi L dt di l t i R r t V lcore p p P n p P ⋅+⋅+⋅+=λ (4) )()(L core l p c l i i R dtdi−⋅=⋅λ⎩⎨⎧==sml core L L di d L λλ)(s s λλλλ>≤The general solution of the differential equations (3),(4) has the following form;⎪⎩⎪⎨⎧>−⋅⋅+−⋅+−−⋅+≤−⋅⋅+−⋅+−⋅=(5) )sin(//)()( )sin(//)(s s 22222221211112121111λλψωττλλψωττt B t e A t t e i A t B t e A t e A t i s s pSubscripts 11,12 and 21,22 denote un-saturated and saturated operation respectively. The parameters given in the equation (5) are given by;() )(/12221σ⋅++⎟⎟⎠⎞⎜⎜⎝⎛⋅−++⋅=m p c p m n p c m m x x R x x R r R x V B()2222)(/1σ⋅++⎟⎟⎠⎞⎜⎜⎝⎛⋅−++⋅=s p c p s n p c s m x x R x x R r R x V B⎟⎟⎟⎟⎟⎠⎞⎜⎜⎜⎜⎜⎝⎛⋅−+++=⋅−−⎟⎟⎟⎠⎞⎜⎜⎜⎝⎛−c p m n p m p c m R x x R r x x R x σφψ111tan tan ⎟⎟⎟⎟⎟⎠⎞⎜⎜⎜⎜⎜⎝⎛⋅−+++=⋅−−⎟⎟⎟⎠⎞⎜⎜⎜⎝⎛−c p s n p s p c m R R r x x R x σφψ112tan tan )sin(111211ψ⋅=+B A A )sin(222221s t B A A ⋅−⋅=+ωψ mp n p m p m p m p c xx R r x x x x x x R ⋅⋅+⋅−⋅+−⋅+⋅⋅⋅=)(4)()(21211σστm p n p m p m p m p c xx R r x x x x x x R ⋅⋅+⋅−⋅++⋅+⋅⋅⋅=)(4)()(21212σστ s p n p s p s p s p xx R r x x x x x x c R ⋅⋅+⋅−⋅+−⋅+⋅⋅⋅=)(4)()(21221σστ sp n p s p s p sp c xx R r x x x x x x R ⋅⋅+⋅−⋅++⋅+⋅⋅⋅=)(4)()(21222σστ ⎟⎟⎠⎞⎜⎜⎝⎛−⋅==s rs s ri i λλλ10 cnp R R r ++=1σ21221112 , ττττ>>>>⇒>>c R , 012≈A , 022≈A According to equation (5), the required inrush waveform assuming two-part segmented i −λcurve can be calculated for two separate un-saturated and saturated regions. For thefirst unsaturated mode, the current can be directly calculated from the first equation for all flux linkage values below the saturation level. After saturation is reached, the current waveform will follow the second given expression for fluxlinkage values above the saturation level. The saturation time s t can be found at the time when the current reaches the saturation current level s i .Where m λ,r λ,m V and ωare the nominal peak flux linkage, residual flux linkage, peak supply voltage and angular frequency, respectivelyThe inrush current waveform peak will essentially exist during saturation mode of operation. The focus should be concentrated on the second current waveform equation describing saturated operation mode, equation (5). The expression of inrush current peak could be directly evaluated when both saturation time s t and peak time of the inrush current waveform peak t t =are known [9].(10))( (9) )(2/)(222222121//)()(2B eA t e i A peak peak t s t s n peak n n peak R I R R t +−⋅+−−⋅+=+=ττωψπThe peak time peak t at which the inrush current will reachits peak can be numerically found through setting the derivative of equation (10) with respect to time equal to zero at peak t t =.()(11) )sin(/)(022222221212221/ψωωττττ−⋅⋅⋅−−−⋅+−=+−⋅peak t s t B A t te A i peak s peakeThe inrush waveform consists of exponentially decaying'DC' term and a sinusoidal 'AC' term. Both DC and AC amplitudes are significantly reduced with the increase of the available series impedance. The inrush waveform, neglecting the relatively small saturating current s i ,12A and 22A when extremely high could be normalized with respect to theamplitude of the sinusoidal term as follows; (12) )sin(/)()(2221221⎥⎦⎤⎢⎣⎡−⋅+−−⋅⋅=ψωτt t t e B A B t i s p(13) )sin(/)()sin()( 22221⎥⎦⎤⎢⎣⎡−⋅+−−⋅⋅−⋅=ψωτωψt t t e t B t i s s p ))(sin()( 2s n n t R R K ⋅−=ωψ (14) ωλλλφλφωλλφωmm m r s s t r m s mV t dt t V dtd t V V s=⎪⎭⎪⎬⎫⎪⎩⎪⎨⎧⎥⎥⎦⎤⎢⎢⎣⎡⎟⎟⎠⎞⎜⎜⎝⎛−−+−⋅=+⋅+⋅⋅==+⋅⋅=−∫(8) 1cos 1(7))sin((6))sin(10The factor )(n R K depends on transformer saturation characteristics (s λand r λ) and other parameters during saturation.Typical saturation and residual flux magnitudes for power transformers are in the range[9]; .).(35.1.).(2.1u p u p s <<λ and .).(9.0.).(7.0u p r u p <<λIt can be easily shown that with increased damping 'resistance' in the circuit, where the circuit phase angle 2ψhas lower values than the saturation angle s t ⋅ω, the exponential term is negative resulting in an inrush magnitude that is lowerthan the sinusoidal term amplitude.B. Neutral Grounding Resistor SizingBased on (10), the inrush current peak expression, it is now possible to select a neutral resistor size that can achieve a specific inrush current reduction ratio )(n R α given by:(15) )0(/)()(==n peak n peak n R I R I R α For the maximum inrush current condition (0=n R ), the total energized phase system impedance ratio X/R is high and accordingly, the damping of the exponential term in equation (10) during the first cycle can be neglected; [][](16))0(1)0()0(2212=⋅++⎥⎦⎤⎢⎣⎡⋅−+===⎟⎟⎠⎞⎜⎜⎝⎛+⋅⋅n s p c p s pR x n m n peak R x x R x x r R K V R I c s σ High n R values leading to considerable inrush current reduction will result in low X / R ratios. It is clear from (14) that X / R ratios equal to or less than 1 ensure negative DC component factor ')(n R K ' and hence the exponential term shown in (10) can be conservatively neglected. Accordingly, (10) can be re-written as follows;()[](17) )()(22122n s p c p s n p R x m n n peak R x x R x x R r V R B R I c s σ⋅++⎥⎦⎤⎢⎣⎡⋅−+=≈⎟⎟⎠⎞⎜⎜⎝⎛+⋅Using (16) and (17) to evaluate (15), the neutral resistorsize which corresponds to a specific reduction ratio can be given by;[][][](18) )0()(1)0( 12222=⋅++⋅−⋅++⋅−+⋅+=⎥⎥⎦⎤⎢⎢⎣⎡⎥⎥⎦⎤⎢⎢⎣⎡=n s p c p s p n s p c p s n p n R x x R x x r R x x R x x R r R K σσα Very high c R values leading to low transformer core loss, it can be re-written equation (18) as follows [9]; [][][][](19) 1)0(12222s p p s p n p n x x r x x R r R K +++++⋅+==α Equations (18) and (19) reveal that transformers require higher neutral resistor value to achieve the desired inrush current reduction rate. IV. A NALYSIS OF SECOND-P HASE E NERGIZING It is obvious that the analysis of the electric and magnetic circuit behavior during second phase switching will be sufficiently more complex than that for first phase switching.Transformer behaviour during second phase switching was served to vary with respect to connection and core structure type. However, a general behaviour trend exists within lowneutral resistor values where the scheme can effectively limitinrush current magnitude. For cases with delta winding or multi-limb core structure, the second phase inrush current is lower than that during first phase switching. Single phase units connected in star/star have a different performance as both first and second stage inrush currents has almost the same magnitude until a maximum reduction rate of about80% is achieved. V. NEUTRAL VOLTAGE RISEThe peak neutral voltage will reach values up to peak phasevoltage where the neutral resistor value is increased. Typicalneutral voltage peak profile against neutral resistor size is shown in Fig. 6- Fig. 8, for the 225 KVA transformer during 1st and 2nd phase switching. A del ay of 40 (ms) between each switching stage has been considered. VI. S IMULATION A 225 KVA, 2400V/600V, 50 Hz three phase transformer connected in star-star are used for the simulation study. The number of turns per phase primary (2400V) winding is 128=P N and )(01.0pu R R s P ==, )(05.0pu X X s P ==,active power losses in iron core=4.5 KW, average length and section of core limbs (L1=1.3462(m), A1=0.01155192)(2m ), average length and section of yokes (L2=0.5334(m),A2=0.01155192)(2m ), average length and section of air pathfor zero sequence flux return (L0=0.0127(m),A0=0.01155192)(2m ), three phase voltage for fluxinitialization=1 (pu) and B-H characteristic of iron core is inaccordance with Fig.3. A MATLAB program was prepared for the simulation study. Simulation results are shown in Fig.4-Fig.8.Fig. 3.B-H characteristic iron coreFig.4. Inrush current )(0Ω=n RFig.5. Inrush current )(5Ω=n RFig.6. Inrush current )(50Ω=n RFig.7. Maximum neutral voltage )(50Ω=n RFig.8. Maximum neutral voltage ).(5Ω=n RFig.9. Maximum inrush current in (pu), Maximum neutral voltage in (pu), Duration of the inrush current in (s)VII. ConclusionsIn this paper, Based on the sequential switching, presents an analytical method to select optimal neutral grounding resistor for transformer inrush current mitigation. In this method, complete transformer model, including core loss and nonlinearity core specification, has been used. It was shown that high reduction in inrush currents among the three phases can be achieved by using a neutral resistor .Other work presented in this paper also addressed the scheme's main practical limitation: the permissible rise of neutral voltage.VIII.R EFERENCES[1] Hanli Weng, Xiangning Lin "Studies on the UnusualMaloperation of Transformer Differential Protection During the Nonlinear Load Switch-In",IEEE Transaction on Power Delivery, vol. 24, no.4, october 2009.[2] Westinghouse Electric Corporation, Electric Transmissionand Distribution Reference Book, 4th ed. East Pittsburgh, PA, 1964.[3] K.P.Basu, Stella Morris"Reduction of Magnetizing inrushcurrent in traction transformer", DRPT2008 6-9 April 2008 Nanjing China.[4] J.H.Brunke, K.J.Frohlich “Elimination of TransformerInrush Currents by Controlled Switching-Part I: Theoretical Considerations” IEEE Trans. On Power Delivery, Vol.16,No.2,2001. [5] R. Apolonio,J.C.de Oliveira,H.S.Bronzeado,A.B.deVasconcellos,"Transformer Controlled Switching:a strategy proposal and laboratory validation",IEEE 2004, 11th International Conference on Harmonics and Quality of Power.[6] E. Andersen, S. Bereneryd and S. Lindahl, "SynchronousEnergizing of Shunt Reactors and Shunt Capacitors," OGRE paper 13-12, pp 1-6, September 1988.[7] Y. Cui, S. G. Abdulsalam, S. Chen, and W. Xu, “Asequential phase energizing method for transformer inrush current reduction—part I: Simulation and experimental results,” IEEE Trans. Power Del., vol. 20, no. 2, pt. 1, pp. 943–949, Apr. 2005.[8] W. Xu, S. G. Abdulsalam, Y. Cui, S. Liu, and X. Liu, “Asequential phase energizing method for transformer inrush current reduction—part II: Theoretical analysis and design guide,” IEEE Trans. Power Del., vol. 20, no. 2, pt. 1, pp. 950–957, Apr. 2005.[9] S.G. Abdulsalam and W. Xu "A Sequential PhaseEnergization Method for Transformer Inrush current Reduction-Transient Performance and Practical considerations", IEEE Transactions on Power Delivery,vol. 22, No.1, pp. 208-216,Jan. 2007.。
最近鲁棒优化进展Recent Advances in Robust Optimization and Robustness An Overview

Recent Advances in Robust Optimization and Robustness:An OverviewVirginie Gabrel∗and C´e cile Murat†and Aur´e lie Thiele‡July2012AbstractThis paper provides an overview of developments in robust optimization and robustness published in the aca-demic literature over the pastfive years.1IntroductionThis review focuses on papers identified by Web of Science as having been published since2007(included),be-longing to the area of Operations Research and Management Science,and having‘robust’and‘optimization’in their title.There were exactly100such papers as of June20,2012.We have completed this list by considering 726works indexed by Web of Science that had either robustness(for80of them)or robust(for646)in their title and belonged to the Operations Research and Management Science topic area.We also identified34PhD disserta-tions dated from the lastfive years with‘robust’in their title and belonging to the areas of operations research or management.Among those we have chosen to focus on the works with a primary focus on management science rather than system design or optimal control,which are broadfields that would deserve a review paper of their own, and papers that could be of interest to a large segment of the robust optimization research community.We feel it is important to include PhD dissertations to identify these recent graduates as the new generation trained in robust optimization and robustness analysis,whether they have remained in academia or joined industry.We have also added a few not-yet-published preprints to capture ongoing research efforts.While many additional works would have deserved inclusion,we feel that the works selected give an informative and comprehensive view of the state of robustness and robust optimization to date in the context of operations research and management science.∗Universit´e Paris-Dauphine,LAMSADE,Place du Mar´e chal de Lattre de Tassigny,F-75775Paris Cedex16,France gabrel@lamsade.dauphine.fr Corresponding author†Universit´e Paris-Dauphine,LAMSADE,Place du Mar´e chal de Lattre de Tassigny,F-75775Paris Cedex16,France mu-rat@lamsade.dauphine.fr‡Lehigh University,Industrial and Systems Engineering Department,200W Packer Ave Bethlehem PA18015,USA aure-lie.thiele@2Theory of Robust Optimization and Robustness2.1Definitions and BasicsThe term“robust optimization”has come to encompass several approaches to protecting the decision-maker against parameter ambiguity and stochastic uncertainty.At a high level,the manager must determine what it means for him to have a robust solution:is it a solution whose feasibility must be guaranteed for any realization of the uncertain parameters?or whose objective value must be guaranteed?or whose distance to optimality must be guaranteed? The main paradigm relies on worst-case analysis:a solution is evaluated using the realization of the uncertainty that is most unfavorable.The way to compute the worst case is also open to debate:should it use afinite number of scenarios,such as historical data,or continuous,convex uncertainty sets,such as polyhedra or ellipsoids?The answers to these questions will determine the formulation and the type of the robust counterpart.Issues of over-conservatism are paramount in robust optimization,where the uncertain parameter set over which the worst case is computed should be chosen to achieve a trade-off between system performance and protection against uncertainty,i.e.,neither too small nor too large.2.2Static Robust OptimizationIn this framework,the manager must take a decision in the presence of uncertainty and no recourse action will be possible once uncertainty has been realized.It is then necessary to distinguish between two types of uncertainty: uncertainty on the feasibility of the solution and uncertainty on its objective value.Indeed,the decision maker generally has different attitudes with respect to infeasibility and sub-optimality,which justifies analyzing these two settings separately.2.2.1Uncertainty on feasibilityWhen uncertainty affects the feasibility of a solution,robust optimization seeks to obtain a solution that will be feasible for any realization taken by the unknown coefficients;however,complete protection from adverse realiza-tions often comes at the expense of a severe deterioration in the objective.This extreme approach can be justified in some engineering applications of robustness,such as robust control theory,but is less advisable in operations research,where adverse events such as low customer demand do not produce the high-profile repercussions that engineering failures–such as a doomed satellite launch or a destroyed unmanned robot–can have.To make the robust methodology appealing to business practitioners,robust optimization thus focuses on obtaining a solution that will be feasible for any realization taken by the unknown coefficients within a smaller,“realistic”set,called the uncertainty set,which is centered around the nominal values of the uncertain parameters.The goal becomes to optimize the objective,over the set of solutions that are feasible for all coefficient values in the uncertainty set.The specific choice of the set plays an important role in ensuring computational tractability of the robust problem and limiting deterioration of the objective at optimality,and must be thought through carefully by the decision maker.A large branch of robust optimization focuses on worst-case optimization over a convex uncertainty set.The reader is referred to Bertsimas et al.(2011a)and Ben-Tal and Nemirovski(2008)for comprehensive surveys of robust optimization and to Ben-Tal et al.(2009)for a book treatment of the topic.2.2.2Uncertainty on objective valueWhen uncertainty affects the optimality of a solution,robust optimization seeks to obtain a solution that performs well for any realization taken by the unknown coefficients.While a common criterion is to optimize the worst-case objective,some studies have investigated other robustness measures.Roy(2010)proposes a new robustness criterion that holds great appeal for the manager due to its simplicity of use and practical relevance.This framework,called bw-robustness,allows the decision-maker to identify a solution which guarantees an objective value,in a maximization problem,of at least w in all scenarios,and maximizes the probability of reaching a target value of b(b>w).Gabrel et al.(2011)extend this criterion from afinite set of scenarios to the case of an uncertainty set modeled using intervals.Kalai et al.(2012)suggest another criterion called lexicographicα-robustness,also defined over afinite set of scenarios for the uncertain parameters,which mitigates the primary role of the worst-case scenario in defining the solution.Thiele(2010)discusses over-conservatism in robust linear optimization with cost uncertainty.Gancarova and Todd(2012)studies the loss in objective value when an inaccurate objective is optimized instead of the true one, and shows that on average this loss is very small,for an arbitrary compact feasible region.In combinatorial optimization,Morrison(2010)develops a framework of robustness based on persistence(of decisions)using the Dempster-Shafer theory as an evidence of robustness and applies it to portfolio tracking and sensor placement.2.2.3DualitySince duality has been shown to play a key role in the tractability of robust optimization(see for instance Bertsimas et al.(2011a)),it is natural to ask how duality and robust optimization are connected.Beck and Ben-Tal(2009) shows that primal worst is equal to dual best.The relationship between robustness and duality is also explored in Gabrel and Murat(2010)when the right-hand sides of the constraints are uncertain and the uncertainty sets are represented using intervals,with a focus on establishing the relationships between linear programs with uncertain right hand sides and linear programs with uncertain objective coefficients using duality theory.This avenue of research is further explored in Gabrel et al.(2010)and Remli(2011).2.3Multi-Stage Decision-MakingMost early work on robust optimization focused on static decision-making:the manager decided at once of the values taken by all decision variables and,if the problem allowed for multiple decision stages as uncertainty was realized,the stages were incorporated by re-solving the multi-stage problem as time went by and implementing only the decisions related to the current stage.As thefield of static robust optimization matured,incorporating–ina tractable manner–the information revealed over time directly into the modeling framework became a major area of research.2.3.1Optimal and Approximate PoliciesA work going in that direction is Bertsimas et al.(2010a),which establishes the optimality of policies affine in the uncertainty for one-dimensional robust optimization problems with convex state costs and linear control costs.Chen et al.(2007)also suggests a tractable approximation for a class of multistage chance-constrained linear program-ming problems,which converts the original formulation into a second-order cone programming problem.Chen and Zhang(2009)propose an extension of the Affinely Adjustable Robust Counterpart framework described in Ben-Tal et al.(2009)and argue that its potential is well beyond what has been in the literature so far.2.3.2Two stagesBecause of the difficulty in incorporating multiple stages in robust optimization,many theoretical works have focused on two stages.Regarding two-stage problems,Thiele et al.(2009)presents a cutting-plane method based on Kelley’s algorithm for solving convex adjustable robust optimization problems,while Terry(2009)provides in addition preliminary results on the conditioning of a robust linear program and of an equivalent second-order cone program.Assavapokee et al.(2008a)and Assavapokee et al.(2008b)develop tractable algorithms in the case of robust two-stage problems where the worst-case regret is minimized,in the case of interval-based uncertainty and scenario-based uncertainty,respectively,while Minoux(2011)provides complexity results for the two-stage robust linear problem with right-hand-side uncertainty.2.4Connection with Stochastic OptimizationAn early stream in robust optimization modeled stochastic variables as uncertain parameters belonging to a known uncertainty set,to which robust optimization techniques were then applied.An advantage of this method was to yield approaches to decision-making under uncertainty that were of a level of complexity similar to that of their deterministic counterparts,and did not suffer from the curse of dimensionality that afflicts stochastic and dynamic programming.Researchers are now making renewed efforts to connect the robust optimization and stochastic opti-mization paradigms,for instance quantifying the performance of the robust optimization solution in the stochastic world.The topic of robust optimization in the context of uncertain probability distributions,i.e.,in the stochastic framework itself,is also being revisited.2.4.1Bridging the Robust and Stochastic WorldsBertsimas and Goyal(2010)investigates the performance of static robust solutions in two-stage stochastic and adaptive optimization problems.The authors show that static robust solutions are good-quality solutions to the adaptive problem under a broad set of assumptions.They provide bounds on the ratio of the cost of the optimal static robust solution to the optimal expected cost in the stochastic problem,called the stochasticity gap,and onthe ratio of the cost of the optimal static robust solution to the optimal cost in the two-stage adaptable problem, called the adaptability gap.Chen et al.(2007),mentioned earlier,also provides a robust optimization perspective to stochastic programming.Bertsimas et al.(2011a)investigates the role of geometric properties of uncertainty sets, such as symmetry,in the power offinite adaptability in multistage stochastic and adaptive optimization.Duzgun(2012)bridges descriptions of uncertainty based on stochastic and robust optimization by considering multiple ranges for each uncertain parameter and setting the maximum number of parameters that can fall within each range.The corresponding optimization problem can be reformulated in a tractable manner using the total unimodularity of the feasible set and allows for afiner description of uncertainty while preserving tractability.It also studies the formulations that arise in robust binary optimization with uncertain objective coefficients using the Bernstein approximation to chance constraints described in Ben-Tal et al.(2009),and shows that the robust optimization problems are deterministic problems for modified values of the coefficients.While many results bridging the robust and stochastic worlds focus on giving probabilistic guarantees for the solutions generated by the robust optimization models,Manuja(2008)proposes a formulation for robust linear programming problems that allows the decision-maker to control both the probability and the expected value of constraint violation.Bandi and Bertsimas(2012)propose a new approach to analyze stochastic systems based on robust optimiza-tion.The key idea is to replace the Kolmogorov axioms and the concept of random variables as primitives of probability theory,with uncertainty sets that are derived from some of the asymptotic implications of probability theory like the central limit theorem.The authors show that the performance analysis questions become highly structured optimization problems for which there exist efficient algorithms that are capable of solving problems in high dimensions.They also demonstrate that the proposed approach achieves computationally tractable methods for(a)analyzing queueing networks,(b)designing multi-item,multi-bidder auctions with budget constraints,and (c)pricing multi-dimensional options.2.4.2Distributionally Robust OptimizationBen-Tal et al.(2010)considers the optimization of a worst-case expected-value criterion,where the worst case is computed over all probability distributions within a set.The contribution of the work is to define a notion of robustness that allows for different guarantees for different subsets of probability measures.The concept of distributional robustness is also explored in Goh and Sim(2010),with an emphasis on linear and piecewise-linear decision rules to reformulate the original problem in aflexible manner using expected-value terms.Xu et al.(2012) also investigates probabilistic interpretations of robust optimization.A related area of study is worst-case optimization with partial information on the moments of distributions.In particular,Popescu(2007)analyzes robust solutions to a certain class of stochastic optimization problems,using mean-covariance information about the distributions underlying the uncertain parameters.The author connects the problem for a broad class of objective functions to a univariate mean-variance robust objective and,subsequently, to a(deterministic)parametric quadratic programming problem.The reader is referred to Doan(2010)for a moment-based uncertainty model for stochastic optimization prob-lems,which addresses the ambiguity of probability distributions of random parameters with a minimax decision rule,and a comparison with data-driven approaches.Distributionally robust optimization in the context of data-driven problems is the focus of Delage(2009),which uses observed data to define a”well structured”set of dis-tributions that is guaranteed with high probability to contain the distribution from which the samples were drawn. Zymler et al.(2012a)develop tractable semidefinite programming(SDP)based approximations for distributionally robust individual and joint chance constraints,assuming that only thefirst-and second-order moments as well as the support of the uncertain parameters are given.Becker(2011)studies the distributionally robust optimization problem with known mean,covariance and support and develops a decomposition method for this family of prob-lems which recursively derives sub-policies along projected dimensions of uncertainty while providing a sequence of bounds on the value of the derived policy.Robust linear optimization using distributional information is further studied in Kang(2008).Further,Delage and Ye(2010)investigates distributional robustness with moment uncertainty.Specifically,uncertainty affects the problem both in terms of the distribution and of its moments.The authors show that the resulting problems can be solved efficiently and prove that the solutions exhibit,with high probability,best worst-case performance over a set of distributions.Bertsimas et al.(2010)proposes a semidefinite optimization model to address minimax two-stage stochastic linear problems with risk aversion,when the distribution of the second-stage random variables belongs to a set of multivariate distributions with knownfirst and second moments.The minimax solutions provide a natural distribu-tion to stress-test stochastic optimization problems under distributional ambiguity.Cromvik and Patriksson(2010a) show that,under certain assumptions,global optima and stationary solutions of stochastic mathematical programs with equilibrium constraints are robust with respect to changes in the underlying probability distribution.Works such as Zhu and Fukushima(2009)and Zymler(2010)also study distributional robustness in the context of specific applications,such as portfolio management.2.5Connection with Risk TheoryBertsimas and Brown(2009)describe how to connect uncertainty sets in robust linear optimization to coherent risk measures,an example of which is Conditional Value-at-Risk.In particular,the authors show the link between polyhedral uncertainty sets of a special structure and a subclass of coherent risk measures called distortion risk measures.Independently,Chen et al.(2007)present an approach for constructing uncertainty sets for robust opti-mization using new deviation measures that capture the asymmetry of the distributions.These deviation measures lead to improved approximations of chance constraints.Dentcheva and Ruszczynski(2010)proposes the concept of robust stochastic dominance and shows its applica-tion to risk-averse optimization.They consider stochastic optimization problems where risk-aversion is expressed by a robust stochastic dominance constraint and develop necessary and sufficient conditions of optimality for such optimization problems in the convex case.In the nonconvex case,they derive necessary conditions of optimality under additional smoothness assumptions of some mappings involved in the problem.2.6Nonlinear OptimizationRobust nonlinear optimization remains much less widely studied to date than its linear counterpart.Bertsimas et al.(2010c)presents a robust optimization approach for unconstrained non-convex problems and problems based on simulations.Such problems arise for instance in the partial differential equations literature and in engineering applications such as nanophotonic design.An appealing feature of the approach is that it does not assume any specific structure for the problem.The case of robust nonlinear optimization with constraints is investigated in Bertsimas et al.(2010b)with an application to radiation therapy for cancer treatment.Bertsimas and Nohadani (2010)further explore robust nonconvex optimization in contexts where solutions are not known explicitly,e.g., have to be found using simulation.They present a robust simulated annealing algorithm that improves performance and robustness of the solution.Further,Boni et al.(2008)analyzes problems with uncertain conic quadratic constraints,formulating an approx-imate robust counterpart,and Zhang(2007)provide formulations to nonlinear programming problems that are valid in the neighborhood of the nominal parameters and robust to thefirst order.Hsiung et al.(2008)present tractable approximations to robust geometric programming,by using piecewise-linear convex approximations of each non-linear constraint.Geometric programming is also investigated in Shen et al.(2008),where the robustness is injected at the level of the algorithm and seeks to avoid obtaining infeasible solutions because of the approximations used in the traditional approach.Interval uncertainty-based robust optimization for convex and non-convex quadratic programs are considered in Li et al.(2011).Takeda et al.(2010)studies robustness for uncertain convex quadratic programming problems with ellipsoidal uncertainties and proposes a relaxation technique based on random sampling for robust deviation optimization sserre(2011)considers minimax and robust models of polynomial optimization.A special case of nonlinear problems that are linear in the decision variables but convex in the uncertainty when the worst-case objective is to be maximized is investigated in Kawas and Thiele(2011a).In that setting,exact and tractable robust counterparts can be derived.A special class of nonconvex robust optimization is examined in Kawas and Thiele(2011b).Robust nonconvex optimization is examined in detail in Teo(2007),which presents a method that is applicable to arbitrary objective functions by iteratively moving along descent directions and terminates at a robust local minimum.3Applications of Robust OptimizationWe describe below examples to which robust optimization has been applied.While an appealing feature of robust optimization is that it leads to models that can be solved using off-the-shelf software,it is worth pointing the existence of algebraic modeling tools that facilitate the formulation and subsequent analysis of robust optimization problems on the computer(Goh and Sim,2011).3.1Production,Inventory and Logistics3.1.1Classical logistics problemsThe capacitated vehicle routing problem with demand uncertainty is studied in Sungur et al.(2008),with a more extensive treatment in Sungur(2007),and the robust traveling salesman problem with interval data in Montemanni et al.(2007).Remli and Rekik(2012)considers the problem of combinatorial auctions in transportation services when shipment volumes are uncertain and proposes a two-stage robust formulation solved using a constraint gener-ation algorithm.Zhang(2011)investigates two-stage minimax regret robust uncapacitated lot-sizing problems with demand uncertainty,in particular showing that it is polynomially solvable under the interval uncertain demand set.3.1.2SchedulingGoren and Sabuncuoglu(2008)analyzes robustness and stability measures for scheduling in a single-machine environment subject to machine breakdowns and embeds them in a tabu-search-based scheduling algorithm.Mittal (2011)investigates efficient algorithms that give optimal or near-optimal solutions for problems with non-linear objective functions,with a focus on robust scheduling and service operations.Examples considered include parallel machine scheduling problems with the makespan objective,appointment scheduling and assortment optimization problems with logit choice models.Hazir et al.(2010)considers robust scheduling and robustness measures for the discrete time/cost trade-off problem.3.1.3Facility locationAn important question in logistics is not only how to operate a system most efficiently but also how to design it. Baron et al.(2011)applies robust optimization to the problem of locating facilities in a network facing uncertain demand over multiple periods.They consider a multi-periodfixed-charge network location problem for which they find the number of facilities,their location and capacities,the production in each period,and allocation of demand to facilities.The authors show that different models of uncertainty lead to very different solution network topologies, with the model with box uncertainty set opening fewer,larger facilities.?investigate a robust version of the location transportation problem with an uncertain demand using a2-stage formulation.The resulting robust formulation is a convex(nonlinear)program,and the authors apply a cutting plane algorithm to solve the problem exactly.Atamt¨u rk and Zhang(2007)study the networkflow and design problem under uncertainty from a complexity standpoint,with applications to lot-sizing and location-transportation problems,while Bardossy(2011)presents a dual-based local search approach for deterministic,stochastic,and robust variants of the connected facility location problem.The robust capacity expansion problem of networkflows is investigated in Ordonez and Zhao(2007),which provides tractable reformulations under a broad set of assumptions.Mudchanatongsuk et al.(2008)analyze the network design problem under transportation cost and demand uncertainty.They present a tractable approximation when each commodity only has a single origin and destination,and an efficient column generation for networks with path constraints.Atamt¨u rk and Zhang(2007)provides complexity results for the two-stage networkflow anddesign plexity results for the robust networkflow and network design problem are also provided in Minoux(2009)and Minoux(2010).The problem of designing an uncapacitated network in the presence of link failures and a competing mode is investigated in Laporte et al.(2010)in a railway application using a game theoretic perspective.Torres Soto(2009)also takes a comprehensive view of the facility location problem by determining not only the optimal location but also the optimal time for establishing capacitated facilities when demand and cost parameters are time varying.The models are solved using Benders’decomposition or heuristics such as local search and simulated annealing.In addition,the robust networkflow problem is also analyzed in Boyko(2010),which proposes a stochastic formulation of minimum costflow problem aimed atfinding network design andflow assignments subject to uncertain factors,such as network component disruptions/failures when the risk measure is Conditional Value at Risk.Nagurney and Qiang(2009)suggests a relative total cost index for the evaluation of transportation network robustness in the presence of degradable links and alternative travel behavior.Further,the problem of locating a competitive facility in the plane is studied in Blanquero et al.(2011)with a robustness criterion.Supply chain design problems are also studied in Pan and Nagi(2010)and Poojari et al.(2008).3.1.4Inventory managementThe topic of robust multi-stage inventory management has been investigated in detail in Bienstock and Ozbay (2008)through the computation of robust basestock levels and Ben-Tal et al.(2009)through an extension of the Affinely Adjustable Robust Counterpart framework to control inventories under demand uncertainty.See and Sim (2010)studies a multi-period inventory control problem under ambiguous demand for which only mean,support and some measures of deviations are known,using a factor-based model.The parameters of the replenishment policies are obtained using a second-order conic programming problem.Song(2010)considers stochastic inventory control in robust supply chain systems.The work proposes an inte-grated approach that combines in a single step datafitting and inventory optimization–using histograms directly as the inputs for the optimization model–for the single-item multi-period periodic-review stochastic lot-sizing problem.Operation and planning issues for dynamic supply chain and transportation networks in uncertain envi-ronments are considered in Chung(2010),with examples drawn from emergency logistics planning,network design and congestion pricing problems.3.1.5Industry-specific applicationsAng et al.(2012)proposes a robust storage assignment approach in unit-load warehouses facing variable supply and uncertain demand in a multi-period setting.The authors assume a factor-based demand model and minimize the worst-case expected total travel in the warehouse with distributional ambiguity of demand.A related problem is considered in Werners and Wuelfing(2010),which optimizes internal transports at a parcel sorting center.Galli(2011)describes the models and algorithms that arise from implementing recoverable robust optimization to train platforming and rolling stock planning,where the concept of recoverable robustness has been defined in。
基于多任务多模态学习的谣言检测框架
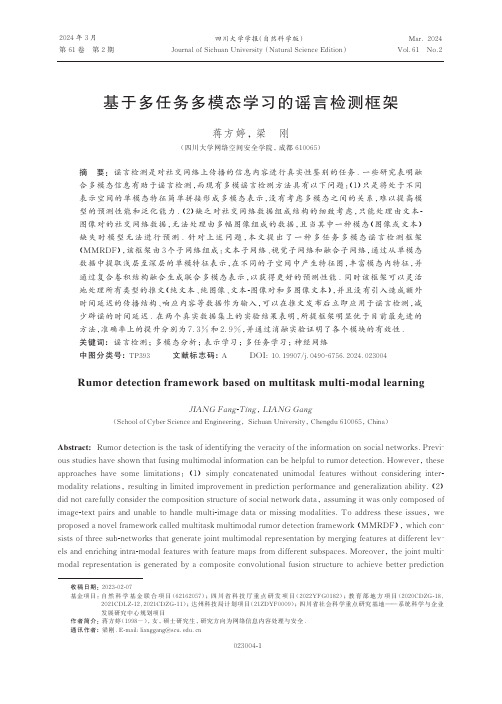
2024 年 3月第 61 卷第 2 期Mar. 2024Vol. 61 No. 2四川大学学报(自然科学版)Journal of Sichuan University (Natural Science Edition)基于多任务多模态学习的谣言检测框架蒋方婷,梁刚(四川大学网络空间安全学院,成都 610065)摘要: 谣言检测是对社交网络上传播的信息内容进行真实性鉴别的任务.一些研究表明融合多模态信息有助于谣言检测,而现有多模谣言检测方法具有以下问题:(1)只是将处于不同表示空间的单模态特征简单拼接形成多模态表示,没有考虑多模态之间的关系,难以提高模型的预测性能和泛化能力.(2)缺乏对社交网络数据组成结构的细致考虑,只能处理由文本-图像对的社交网络数据,无法处理由多幅图像组成的数据,且当其中一种模态(图像或文本)缺失时模型无法进行预测.针对上述问题,本文提出了一种多任务多模态谣言检测框架(MMRDF),该框架由3个子网络组成:文本子网络、视觉子网络和融合子网络,通过从单模态数据中提取浅层至深层的单模特征表示,在不同的子空间中产生特征图,丰富模态内特征,并通过复合卷积结构融合生成联合多模态表示,以获得更好的预测性能.同时该框架可以灵活地处理所有类型的推文(纯文本、纯图像、文本-图像对和多图像文本),并且没有引入造成额外时间延迟的传播结构、响应内容等数据作为输入,可以在推文发布后立即应用于谣言检测,减少辟谣的时间延迟.在两个真实数据集上的实验结果表明,所提框架明显优于目前最先进的方法,准确率上的提升分别为7.3%和2.9%,并通过消融实验证明了各个模块的有效性.关键词: 谣言检测;多模态分析;表示学习;多任务学习;神经网络中图分类号: TP393 文献标志码: A DOI:10.19907/j.0490-6756.2024.023004Rumor detection framework based on multitask multi-modal learningJIANG Fang-Ting, LIANG Gang(School of Cyber Science and Engineering, Sichuan University, Chengdu 610065, China)Abstract: Rumor detection is the task of identifying the veracity of the information on social networks.Previ‑ous studies have shown that fusing multimodal information can be helpful to rumor detection.However, these approaches have some limitations:(1)simply concatenated unimodal features without considering inter-modality relations, resulting in limited improvement in prediction performance and generalization ability.(2)did not carefully consider the composition structure of social network data, assuming it was only composed of image-text pairs and unable to handle multi-image data or missing modalities.To address these issues,we proposed a novel framework called multitask multimodal rumor detection framework (MMRDF), which con‑sists of three sub-networks that generate joint multimodal representation by merging features at different lev‑els and enriching intra-modal features with feature maps from different subspaces.Moreover, the joint multi‑modal representation is generated by a composite convolutional fusion structure to achieve better prediction 收稿日期: 2023-02-07基金项目:自然科学基金联合项目(62162057);四川省科技厅重点研发项目(2022YFG0182);教育部地方项目(2020CDZG-18, 2021CDLZ-12,2021CDZG-11);达州科技局计划项目(21ZDYF0009);四川省社会科学重点研究基地——系统科学与企业发展研究中心规划项目作者简介: 蒋方婷(1998−),女,硕士研究生,研究方向为网络信息内容处理与安全.通讯作者: 梁刚.E-mail: lianggang@第 2 期蒋方婷,等:基于多任务多模态学习的谣言检测框架第 61 卷performance.MMRDF is flexible and capable of handling various types of tweets, including pure text, pure image, image-text pairs, and text with multi-images.Additionally, the MMRDF does not require extra time-delaying data such as propagation structures and response content, allowing for immediate application to ru‑mor detection and reducing the time delay in debunking rumors.Experimental results on two real-world datas‑ets demonstrate that our framework outperforms the state-of-the-art methods, achieving an accuracy improve‑ment of 7.3% and 2.9%.Ablation experiments further validate the effectiveness of each module in the pro‑posed framework.Keywords: Rumor detection;Multi-modal analysis;Representation learning;Multitask learning;Neural Networks1 引言近年来,移动互联网技术的不断更新、媒体内容向移动平台的转变,给当今人们的生活提供了更加便捷的信息交流互动方式[1].根据报告(https:///reports/digital-021-global-overview-report)截止2021年初全球有42.2亿社交媒体用户.随着网络社交媒体的快速发展,社交媒体上的谣言泛滥已经成为一个严重的问题,它可能会对政治、经济和社会稳定造成重大危害.为了实现自动检测谣言并辟谣,人们做了大量的研究工作,早期的文献[2,3]大多基于手工特征构建表示,并训练浅层分类器来判定谣言的真实性.为捕捉文本内容中更多的上下文信息和语义信息的变化,研究者们提出了各种深度学习模型[4-6].但由于文本表示的局限性,基于文本的检测方法对谣言识别的效果贡献受限.为了丰富帖子的表示,一些研究开始将帖子中不同格式的数据引入到谣言检测中,如文献[7,8]结合传播结构和文本内容来捕捉评论内容中的语义演化.然而,这些方法需要长期的观测来获取传播结构和响应信息,导致存在较大的时间延迟,且无法在推文发布后立即揭穿谣言.图像作为推文发布时的另一种数据格式,能够从视觉角度生动地描述事件发生的情况,补充文本描述所缺失的细节,因此引起了研究者的关注.由于社交平台的文本长度限制,越来越多的用户选择将图片与文本一起发布来描述事件.根据文献[9]的数据,新浪微博上有超过一半的推文包含图片.在线社交网络上的以文本为媒介的交流方式逐渐转向以文本、图像、视频为媒介的多媒体交流方式.为吸引并误导读者,谣言制造者故意将虚假图片和欺骗性的图片添加到推文或新闻中[10].由于谣言利用了视觉信号,一些研究[10-12]发现视觉特征是谣言检测的重要指标,有助于提高谣言检测的性能.然而,主流的多模态谣言检测研究大多将单峰模态表示的简单连接作为多模态表示.这种简单的策略可能会导致可能会导致一个模态表示占主导,压制其他模态表示[13,14].此外,来自不同模态的信息处于不同嵌入空间中,例如,文本内容遵循时间逻辑,而视觉内容遵循空间逻辑,这意味着特征表示过程需要为每个模态建立不同的模型,在表示融合过程中需要一个融合模型.表示融合是多模态表示学习中的一个重要问题,旨在联合来自不同模态的表示[15].现有的融合方法可以分为早期融合和后期融合.早期融合在提取单模态特征后立即将其联合为多模态特征表示,而不进行任何学习处理,后期融合试图从单模态特征中单独学习语义信息,并将它们组合成最终的预测结果.早期融合策略将各模态的低层特征进行融合以学习相关性[16],当特征仅出现在高层抽象时,可能会造成语义信息等深层特征的不足.对于后期融合,其通过平均或加权的方式综合考虑子模型的预测结果,而不是多模态表示学习,因此它只能对多模态的线性交互进行建模,而无法学习不同模态特征之间的相关性和互补性.现有谣言检测研究的另一个局限性是所提出的模型未能处理社交网络上所有类型的推文.仅考虑推文中的图像和文本内容,社交网络上的推文有4种类型,分别是:(1)纯文本推文;(2)纯图像推文;(3)文本-图像对(文本推文配一幅图像);(4)文本推文配多幅图像.大多数方法[14]只能处理文本-图像对形式的数据,而忽略了纯文本推文和纯图像推文.此外,以往的研究仅限于处理推文中的单张图像,这种忽略除第一幅图像外的其他图像的数据处理方式,导致视觉信号和图像语义信息不完整.为了应对上述挑战,本文提出了一种多任务多模态谣言检测框架MMRDF.该框架包含两个分别用于处理文本和视觉模态的深度神经子网络,以及一个将文本特征和视觉特征投影到同一第 61 卷四川大学学报(自然科学版)第 2 期多模态空间的融合子网络.每个子网络后附加分类器,通过全局损失反向传播约束以协调每个模态中的表示,从而可以有效地处理各种图文内容.具体而言,我们的工作主要贡献如下:(1)本文提出了一种新的多任务多模态谣言检测框架,该框架由3个子网络组成,即文本模态子网络、视觉模态子网络和融合子网络.为了减少谣言揭穿过程中的时间延迟,该框架没有将传播结构、响应内容等造成额外时间延迟的数据作为输入,以实现在推文发布时即可实现谣言鉴别.(2)针对谣言检测中简单拼接策略导致的联合表示效果不佳的问题,本文提出了一种中间融合方法来融合多模态中不同层次的特征,通过学习单模态不同层次的边缘表示和复杂的跨模态关联特征,进而生成联合表示.从该模型中学习到的联合多模态表示进一步提升了预测性能和泛化能力.(3)由于以往的方法仅限于处理文本-图像对的推文,本文通过从多任务中学习到的三个分类器来处理上述所有类型的推文,并且在其中一种模态缺失时仍然可以运行.此外,为了保持图像语义的完整性、丰富视觉表征,通过数据增强的方法将含有多张图片的推文转换为文本-图像对,而不是默认忽略除第一张图像外的其他图像.(4)在两个真实社交网络数据集上,本文进行了对比实验、定性消融实验、定量消融实验以及减少训练数据实验,结果显示本文模型具有更优的预测性能,比现有基线在准确率上提升了7.3%和2.9%,增强了泛化能力,在少量数据上的训练效果仍能保持领先.2 相关工作2.1 基于单模态的自动谣言检测方法基于文本内容的单模谣言检测方法主要被提出于谣言识别研究的早期,大量研究[2,17,18]根据文本内容中的鉴别特征(如词汇特征,句法特征,主题特征,时序特征等)构建文本表示并训练浅度分类器以鉴别谣言.如2011年,Castillo等[2]从文本内容中提出了基于内容的特征和基于主题的特征,以通过支持向量机、J48决策树、决策规则和贝叶斯网络来评估推文的可信度水平.2015年,Zhao 等[19]提出社交网络中不实信息推文发布后会产生与其主题相关的查询推文与质疑推文,并通过这一特征来发现网络中正在传播的不实信息.在上述基于手工构建特征的方法中,有效特征的提取和选择都是手工的,这是一种代价高昂的劳动密集型方法,并且会给模型带来人为误差,导致模型的性能依赖于手工特征工程的质量.因此,一些研究者考虑使用深度学习方法来克服上述问题.Ma 等[4]提出了一个基于RNN的模型,该模型学习连续的隐藏表示,捕捉上下文信息的变化以识别谣言.Yu等[20]提出了一种基于卷积神经网络的方法,该方法提取分散在输入序列中的局部-全局特征,并形成重要特征之间的深层交互,以助于识别错误信息.由于用户评论中包含了丰富的信息,如人们的立场和观点,一些研究试图分析推文的内容和用户评论之间的潜在关系.Ma等[21]提出了自底向上和自顶向下的树结构来表示推文和用户回复,并利用递归神经网络进行预测.Rao等[22]采用双层粒度的基于注意力掩码的BERT来处理帖子内容和评论,从而发现与推文文本内容相关的评论.Shu等[23]利用新闻内容和部分评论之间的语义相似性实现可解释的谣言检测模型,通过一个共同注意子网络来捕获Top-k个可解释的句子-评论对.然而这个框架需要长文本的新闻和评论,并不适用于社交媒体中的短文本数据.如前所述,由于社交媒体中文本内容的简短性质和非正式表达,基于文本的模型在谣言识别方面的效果有限.相较于基于文本内容的检测方法,仅利用图像的单模谣言检测方法较少.Choudhary等[10]采用5个基于CNN的预训练模型和多数投票策略的分类器来发现多媒体特征,从而进行基于图像的假新闻检测.Qi等[24]提出了一种多域视觉神经网络,将频域的物理信息和像素域的语义信息融合在一起,用于区分虚假新闻图像和真实新闻图像.由于不同的人对同一幅图像可能有不同的解读,很难使用图像单独表示发布者的理解或意图.鉴于文本内容在谣言检测中起着重要的作用,推文内容的表示应同时考虑文本内容和视觉内容.2.2 基于多模态的自动谣言检测方法尽管单模态的谣言检测方法在一定程度上发挥了作用,但在简短、嘈杂、充斥非正式用语的社交网络数据中挖掘谣言仍然是一项艰巨的任务.幸运的是,社交媒体平台上有着大量丰富的多模态信息,如文本内容,传播结构信息,图像内容等.图像作为一种多模态数据,与纯文本相比更具有吸引力,更容易引起人们的关注.为了吸引和误导读者,越来越多的虚假图片和欺骗性图片被故意添加到推文或新闻中[10].深度学习神经网络由于其强大的特征提取能力,可以捕捉到复杂的第 2 期蒋方婷,等: 基于多任务多模态学习的谣言检测框架第 61 卷模式分布,以缓解传统统计特征方法的局限性.一些研究尝试使用深度学习模型提取单峰特征,并将其融合以获得丰富的表征,用于进一步的特定任务.对于文本表示,最近的文献[11,25,26]使用基于Transformer 的模型(例如BERT , Encoder -Decoder 模型)和基于CNN 的模型以更好地捕获语义和上下文信息.对于视觉表示,大多数文献[25,27]使用基于CNN 的模型(例如VGG16,VGG19)来获取视觉特征.然而前向研究很少关注发现模态内的相关性和复杂的跨模态关系.多模态视图下谣言检测的大多数研究[11,25,28]都是采用简单的拼接方式将不同嵌入空间中的多模态表示组合在一起,不同模态的信息在不同的嵌入空间中表示,具有不同的噪声结构,例如,文本内容遵循时间逻辑,而视觉内容则遵循空间逻辑,即在特征表示过程中需要针对每种模态训练不同的模型,再将多模特征融合表示为一个联合表示才能进行更好的预测.但现有的这种简单策略可能会导致一种表示压制另一种表示的表现,并且不能在跨模态中提取更多有效的联合边缘特征[29,30].现有谣言检测研究的另一个局限性是现有模型未能处理社交网络上所有类型的推文.绝大多数关于多模态谣言检测的研究[28,31]只能处理文本-图像对(由文本信息和推文中的一个图像组成),而忽略了单模态的文本/图像推文,当其中一个模态缺失时就无法工作.此外,主流的研究[32-34]难以处理推文中的多个视觉信号,模型只从推文中的第一张图像中提取视觉特征,忽略了剩余图像,破坏了视觉信息的完整性.处理每张图像将有助于生成更丰富的数据表示,并有助于谣言检测任务.3 本文模型3.1 问题定义给定一个帖子P ={T ,V }包括文本内容T 和视觉内容V .文本表示定义为T ={w 1,w 2,..,w n },其中w i ∈R d 是第i 个字向量,n 是总字数,d w 是字嵌入的维数.图像表示定义为V ={v 1,v 2,..,v m },其中v i ∈R d 是图像的第i 个补丁嵌入,m 是图像分割块的个数,d v 是视觉嵌入的维数.本文将谣言检测归结为一个二元分类任务,即P 分为谣言和非谣言.如图1所示,MMRDF 包括三个组成部分:文本子网络、视觉子网络和融合网络.为了减少谣言检测的时间延迟,该框架将推文中包括文本和图像的原始信息作为输入,以便在推文发布后立即高效工作.该框架对一条推文所附的多张图片进行预处理,将图片横向粘贴到一个白色背景中,形成文本-图像对的数据格式.文本和图像内容分别输入至文本子网络和视觉子网络中,提取单模态特征并进行预测.融合网络以文本初始嵌入、图像初始嵌入和两个单峰子网络的隐藏状态作为输入,合并跨模态相关的单模态表示.融合网络从两单模态中提取浅层至深层的单模特征表示,在不同的子空间中产生特征图,丰富模态内特征,并通过复合卷积结构挖掘多模态表征.达到从不同模态中提取的内容相互补充并增强多模表示的目的,从而有助于谣言检测.图1 多任务多模态谣言检测框架的网络结构Fig.1 Network architecture of the proposed Multitask Multimodal Rumor Detection Framework第 61 卷四川大学学报(自然科学版)第 2 期3.2 文本子网络文本子网络处理推文中的文本内容以提取文本表示特征.部分谣言检测研究利用词袋模型和word2vec 嵌入方法来表示文本内容,但这些方法生成的嵌入不能提供单词的位置,并且独立于上下文信息.预训练BERT 模型被提出对大量未标记文本语料库进行预训练,以获得深度双向的语义表示,从而有效地微调各类自然语言处理任务.在文本子网络中,初始嵌入表示E 1是令牌嵌入、位置嵌入和段嵌入的总和,在早期融合阶段将作为浅层特征输入融合网络中,接着将初始嵌入表示传入12层编码器的预训练BERT 模型中,每个编码器层使用多头自注意力机制并行地从不同的表示子空间中提取信息,其中自注意力机制定义为Attention (Q ,K ,V )= softmax (QK Td V)(1)式(1)中,Q 是一个查询向量;K 是一个键向量;V 是一个值向量;d 是向量的维度.多头注意力如下式进行并行计算后拼接L 个特征子空间的词表示,并将多头表示传递给归一化层和含有GELU 激活函数的前馈神经网络,得到当前编码器的输出.MultiHead(Q ,K ,V )=concate (head 1,head 2,...,head L )W O (2)head i =Attention (QW Q i ,KW K i ,VW V i ),i ∈[1,L ](3)式(2)和式(3)中,W Q i ,W K i 和W V i 是查询矩阵、键矩阵和值矩阵的权重;W O 为前馈神经网络的参数.经过12层编码器的处理,每个单词转换为768维的词向量w i .每一层编码器的输出构成模型的隐藏状态H 1={h 1, h 11,…,h111}.为了进一步理解上下文并缓解RNN 中的长程依赖问题,文本子网络采用堆叠的Bi -LSTM 单元来捕获文本特征R 1,将BERT 的最后一个隐藏状态h 111输入到Bi -LSTM 中,从文本序列的前向和后向双方向上提取上下文特征.我们将正向LSTM 单元和反向LSTM 单元的隐藏层输出 s l t 和s l t 连接起来,以得到每个时间点Bi -LSTM 的隐藏层输出s t :s t = s l t ⊕s l t(4)式(4)中,⊕是连接操作符;[s 1, s 2,…,s n ]是文本序列的双向特征表示.在文本推文分类器中,最后一层的双向特征表示s t 经过密集连接层,通过soft‑max 函数计算预测 [y t , y t ,…,y t ],最后采用交叉熵损失函数计算文本子网络的损失Loss 1,如下式.Loss 1=-∑i =1N (y i *log (y t ) +(1-y i )*log (1-y t ))(5)式(5)中,N 为预测推文总数;y i 为第i 个推文的真实标签.3.3 视觉子网络在Transformer 成为自然语言处理领域的基准架构之时,其在计算机视觉领域的应用也被提出:Vision Transformer (ViT )[35].与BERT 类似,ViT也由Transformer 中的编码器组成.ViT 将图像分割为16×16大小的块,然后使用密集层将展平的块映射到具有可训练线性投影的固定块嵌入,并添加位置嵌入,共同组成嵌入向量E 2输入至Transformer 编码器中.在MMRDF 的视觉子网络中,我们使用在1400万张图像上预训练得到的ViT 模型来获取视觉特征,12层编码器的输出组成了模型的隐藏状态H 2={h 20, h 21,…,h 211},为了捕获视觉特征R 2,视觉子网络采用了一个含有激活函数的密集连接层:R 2 =σ(W FC ·h 211)(6)式(6)中,W FC 为全连通层的权值矩阵;h 211为ViT模型的最后一个隐藏状态.与文本子网络相同,σ为激活函数.视觉分类器再通过softmax 函数计算得到视觉子网络的预测 [y v , y v ,…,y v ],计算交叉熵损失Loss 2:Loss 2 =∑i =1N (y i *log (y v ) +()1-y i*log ()1-y v )(7)3.4 融合子网络多模态谣言检测常用方法是分别学习单模态表示,并简单地将它们连接组成多模序列中,即:S =[w 1,w 2,…,w n ,v 1,v 2,…,v m ](8)文本表示是S t =[w 1,w 2,…,w n ]和可视化表示S v =[v 1,v 2,…,v m ].然而这种简单的连接策略可能会导致一个模态表示占主导,压制其他模态表示.同时,由于文本表示和视觉表示属于不同的表示空间,简单的拼接不能将不同的嵌入空间映射到同一个公共空间.卷积神经网络被认为是一种有效的特征提取器,同时它也被用于多模态数据的融合,如人脸、虹膜、指纹、RGB 图像、骨骼数据等.由于BERT 在预训练期间未能关注短文本(如短语),基于CNN 的模型可以通过获取文本的局部信息来解决第 2 期蒋方婷,等: 基于多任务多模态学习的谣言检测框架第 61 卷这个问题.本文构建了一个基于CNN 的融合网络,以获取模态内的相关性(如局部表示)和跨模态表示.该融合网络包含12个融合层,依次融合来自文本子网络和视觉子网络的浅层至深层的单模态特征,以产生多模态表征.如图2所示,每个融合层包含两个主要处理块:多模态表示的模态内融合模块和挖掘跨模态表征的模态间关联模块,第i 个融合层的输入是前一层融合层的输出O i -1、文本模态的隐藏表示h 1i 和视觉模态的隐藏表示h 2i .如图3所示,文本的隐藏表示h 1i 和图像的隐藏表示h 2i 首先通过通道堆叠,得到192x768x2的表示,考虑到后续卷积操作的计算复杂度和计算内存需求,卷积层前加入一个全连接层将768维度的嵌入表示降至128维(D =128).四类具有不同大小的卷积核在输入图上滑动以捕获n -gram 特征,由于在Transformer 预训练模型产生的表示中,每一行代表一个离散的单词或是被分割的图像区域,为获取完整的表示特征,所有卷积核的长度应与词嵌入的维数相同,高度分别为n=[2,3,4,5].每种不同大小的卷积核都设置16个,从而在不同的子空间中产生特征图,进而丰富特征.在输入图A ∈R s ×D 上的卷积计算过程如下:y t n =|| N -n +1i =1σ(w tn ·x i :i +n -1+b t n )(9)Y n =concat (y 1n ,y 2n ,..,y t n ,…,y 16n)(10)其中,w tn 是高度为n 的第t 个卷积核的权重矩阵;x i :i +n -1是输入图A 中第i 行至第i+n -1行的表示;b t n 是高度为n 的第t 个卷积核中的偏置项;σ是ReLU 激活函数.特征映射Y n 表示的n -gram 特征是在具有相同大小(n=[2,3,4,5])同类卷积核的输出上进行特征维度级拼接得到的.如图3中右侧矩阵所示,2-gram 的特征图Y 2尺寸为16×99, 3-gram ,4-gram 和5-gram 的特征图尺寸均为16×100,接着四个特征图通过一个1×3最大池化层,而不是Text -CNN [36]模型中的1-max 池化策略.最大池化层从每个特征映射中提取一个标量,这有助于将每个特征映射到固定长度的向量,但会丢失特征映射中的大部分标量.因此,本文中的池化层包含一个步长为2的1×3核,以下采样得到更多信息的特征,同时将特征图映射到相同的大小.池化后的4个特征图在通道层面上拼接在一起得到局部语义信息作为模态内特征.接着为获取跨模态关联,本文构建复合卷积层(包含1×1卷积层和3×3卷积层)的模态关联模块,沿着通道方向进一步聚合特征.如图4的模态关联模块中,与常见的BN -ReLU -Conv (3×3)网络不同,本文在3×3卷积之前附加1×1卷积构成BN -ReLU -Conv (1×1)-BN -ReLU -Conv (3×3)复合卷积网络结构,1×1卷积层在保留特征相关信息的同时,提供了过滤池化和降维功能,提高了计算效率,使得整个复合卷积网络加强了模态间信息的相互作用.结合图2和图4所示,第i 个模态关联模块的输入是第i -1层融合层的输出O i -1和第i 层融合模块的输出在通道维度上的拼接,经过批标准化(归一化层),ReLU 激活层,1×1卷积层,批标准化(归一化层),ReLU 激活层,3×3卷积层得到当前融合层的隐藏表示h i .图2 第i 个融合层结构Fig.2 The network structure of the i -th fusion layer图3 第i 个融合模块结构Fig.3 The structure of fusion block -i图4 第i 个模态关联模块Fig.4 The structure of fusion correlation block -i。
f-k非视域成像方法的数据集
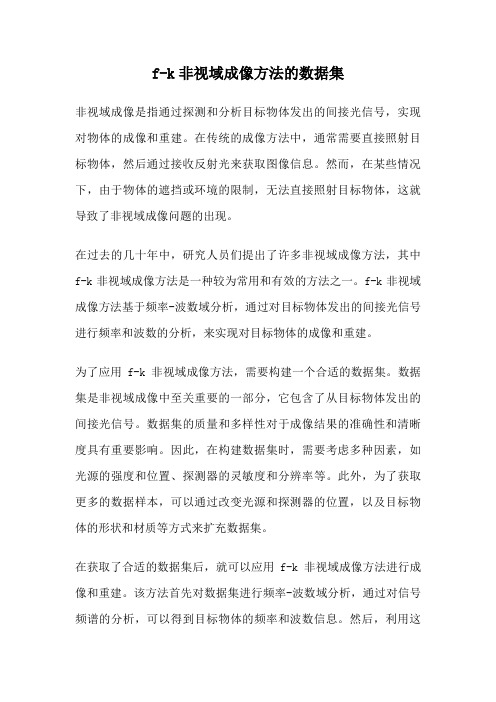
f-k非视域成像方法的数据集非视域成像是指通过探测和分析目标物体发出的间接光信号,实现对物体的成像和重建。
在传统的成像方法中,通常需要直接照射目标物体,然后通过接收反射光来获取图像信息。
然而,在某些情况下,由于物体的遮挡或环境的限制,无法直接照射目标物体,这就导致了非视域成像问题的出现。
在过去的几十年中,研究人员们提出了许多非视域成像方法,其中f-k非视域成像方法是一种较为常用和有效的方法之一。
f-k非视域成像方法基于频率-波数域分析,通过对目标物体发出的间接光信号进行频率和波数的分析,来实现对目标物体的成像和重建。
为了应用f-k非视域成像方法,需要构建一个合适的数据集。
数据集是非视域成像中至关重要的一部分,它包含了从目标物体发出的间接光信号。
数据集的质量和多样性对于成像结果的准确性和清晰度具有重要影响。
因此,在构建数据集时,需要考虑多种因素,如光源的强度和位置、探测器的灵敏度和分辨率等。
此外,为了获取更多的数据样本,可以通过改变光源和探测器的位置,以及目标物体的形状和材质等方式来扩充数据集。
在获取了合适的数据集后,就可以应用f-k非视域成像方法进行成像和重建。
该方法首先对数据集进行频率-波数域分析,通过对信号频谱的分析,可以得到目标物体的频率和波数信息。
然后,利用这些信息,可以重建出目标物体的形状和结构。
需要注意的是,由于数据集中包含了大量的噪声和干扰信号,因此在进行频率-波数域分析时,需要采用一些滤波和降噪技术,以提高重建结果的质量。
f-k非视域成像方法在实际应用中具有广泛的意义和潜力。
首先,该方法可以突破传统成像方法的限制,实现对遮挡物体的成像和重建。
这对于一些特殊领域的研究和应用具有重要意义,如无人驾驶车辆的感知和避障、地质勘探中的隐蔽矿藏探测等。
其次,f-k非视域成像方法可以提供更多的信息和细节,从而提高成像结果的质量和分辨率。
这对于一些精细结构的分析和研究非常重要,如生物医学领域中的组织结构分析和病变检测等。
刺激-反应联结学习在项目特异性比例一致效应中的作用
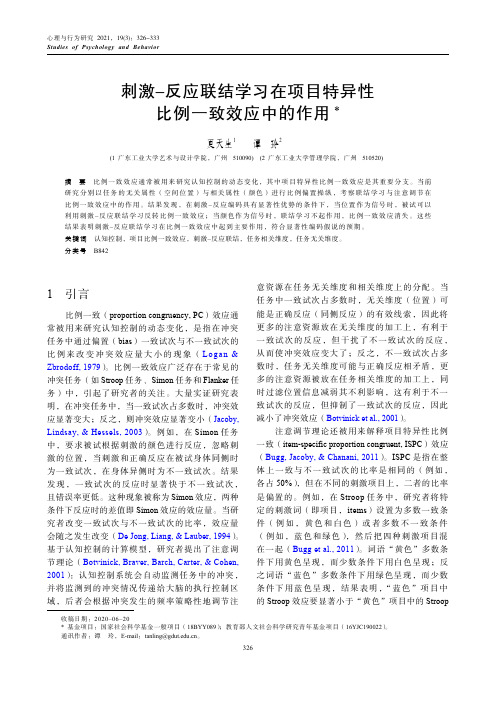
327
效应。研究者认为,可能存在局部的、项目特异 性的认知控制机制,可以根据单个项目的比例偏 置情况选择性地进行注意资源分配从而影响不同 项目的反应(Jacoby et al., 2003)。
然而,注意调节理论近来受到了其他研究的 挑战(Schmidt & Besner, 2008; Schmidt & Lemercier, 2019)。Schmidt 和 Besner 认为,ISPC 效应中比例 一致性与刺激–反应的可能性混合在一起,可以 用更经济的可能性学习来解释这一效应。该研究 提出了可能性学习假说(contingency learning hypothesis), 表 示 认 知 控 制 不 是 必 要 的 , 被 试 完 全可以通过学习发现刺激无关维度与正确反应的 可能性关系,并基于此预测正确的反应。在冲突 任务中,一致试次占多数时,刺激常常伴随着一 致的任务无关的刺激属性,这一属性有很大可能 性可以去预测正确的反应,而多数试次为不一致 时,被试可能根据伴随的不一致的任务无关刺激 属性去预测反应(Schmidt & Lemercier, 2019)。这 一策略可以加快一致或不一致试次的反应,从而 产生 ISPC 效应。可能性学习假说基于刺激–反应 (stimulus-response, S-R)之间的联结解释了 ISPC 效应,是 S-R 联结学习的一种方式(Schmidt, 2013)。
心理与行为研究 2021,19(3):326~333 Studies of Psychology and Behavior
刺激–反应联结学习在项目特异性 比例一致效应中的作用 *
夏天生1 谭 玲2
(1 广东工业大学艺术与设计学院,广州 510090) (2 广东工业大学管理学院,广州 510520)
2011国际眼科药物临床试验培训研讨会在上海召开
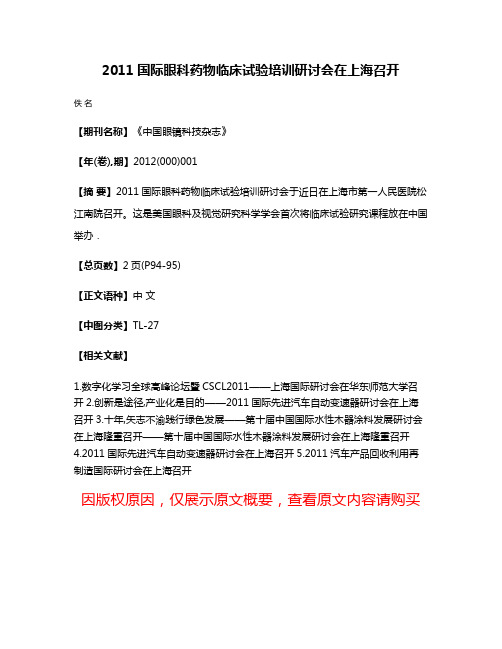
2011国际眼科药物临床试验培训研讨会在上海召开
佚名
【期刊名称】《中国眼镜科技杂志》
【年(卷),期】2012(000)001
【摘要】2011国际眼科药物临床试验培训研讨会于近日在上海市第一人民医院松江南院召开。
这是美国眼科及视觉研究科学学会首次将临床试验研究课程放在中国举办.
【总页数】2页(P94-95)
【正文语种】中文
【中图分类】TL-27
【相关文献】
1.数字化学习全球高峰论坛暨CSCL2011——上海国际研讨会在华东师范大学召开
2.创新是途径,产业化是目的——2011国际先进汽车自动变速器研讨会在上海召开
3.十年,矢志不渝践行绿色发展——第十届中国国际水性木器涂料发展研讨会在上海隆重召开——第十届中国国际水性木器涂料发展研讨会在上海隆重召开
4.2011国际先进汽车自动变速器研讨会在上海召开
5.2011汽车产品回收利用再制造国际研讨会在上海召开
因版权原因,仅展示原文概要,查看原文内容请购买。
基于注意力机制的多模态自动驾驶行为决策模型
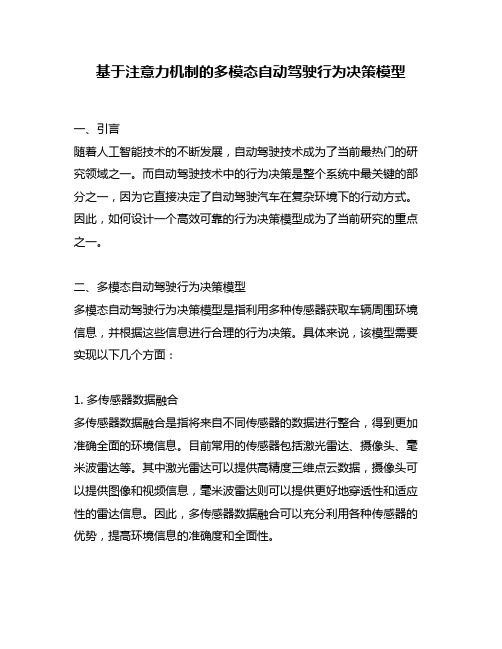
基于注意力机制的多模态自动驾驶行为决策模型一、引言随着人工智能技术的不断发展,自动驾驶技术成为了当前最热门的研究领域之一。
而自动驾驶技术中的行为决策是整个系统中最关键的部分之一,因为它直接决定了自动驾驶汽车在复杂环境下的行动方式。
因此,如何设计一个高效可靠的行为决策模型成为了当前研究的重点之一。
二、多模态自动驾驶行为决策模型多模态自动驾驶行为决策模型是指利用多种传感器获取车辆周围环境信息,并根据这些信息进行合理的行为决策。
具体来说,该模型需要实现以下几个方面:1. 多传感器数据融合多传感器数据融合是指将来自不同传感器的数据进行整合,得到更加准确全面的环境信息。
目前常用的传感器包括激光雷达、摄像头、毫米波雷达等。
其中激光雷达可以提供高精度三维点云数据,摄像头可以提供图像和视频信息,毫米波雷达则可以提供更好地穿透性和适应性的雷达信息。
因此,多传感器数据融合可以充分利用各种传感器的优势,提高环境信息的准确度和全面性。
2. 基于深度学习的特征提取基于深度学习的特征提取是指利用深度学习技术对传感器数据进行处理,提取出有用的特征信息。
目前常用的深度学习模型包括卷积神经网络、循环神经网络等。
其中卷积神经网络可以对图像和视频信息进行处理,提取出图像中的物体信息和运动轨迹等;循环神经网络可以对时间序列数据进行处理,提取出车辆周围环境中物体的运动方向、速度等信息。
因此,基于深度学习的特征提取可以帮助模型更好地理解周围环境,并为后续行为决策做好准备。
3. 注意力机制注意力机制是指在模型中引入注意力机制,让模型能够自动关注重要的信息。
具体来说,在多模态自动驾驶行为决策模型中,引入注意力机制可以使模型更加关注与当前任务相关的信息,并忽略与当前任务无关的信息。
例如,在行驶过程中,模型可以更加关注前方的障碍物和交通信号灯等信息,而忽略路边的景观和建筑等信息。
因此,注意力机制可以提高模型的效率和准确性。
4. 预测未来状态预测未来状态是指利用当前环境信息,预测未来一段时间内车辆周围环境的状态。
自动化外文参考文献(精选120个最新)
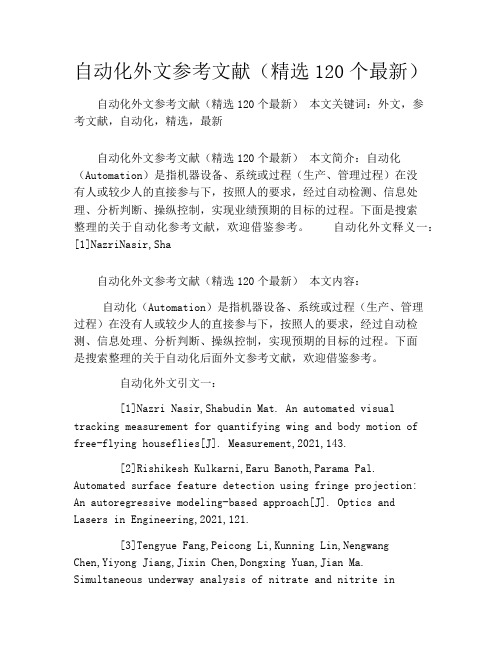
自动化外文参考文献(精选120个最新)自动化外文参考文献(精选120个最新)本文关键词:外文,参考文献,自动化,精选,最新自动化外文参考文献(精选120个最新)本文简介:自动化(Automation)是指机器设备、系统或过程(生产、管理过程)在没有人或较少人的直接参与下,按照人的要求,经过自动检测、信息处理、分析判断、操纵控制,实现业绩预期的目标的过程。
下面是搜索整理的关于自动化参考文献,欢迎借鉴参考。
自动化外文释义一:[1]NazriNasir,Sha自动化外文参考文献(精选120个最新)本文内容:自动化(Automation)是指机器设备、系统或过程(生产、管理过程)在没有人或较少人的直接参与下,按照人的要求,经过自动检测、信息处理、分析判断、操纵控制,实现预期的目标的过程。
下面是搜索整理的关于自动化后面外文参考文献,欢迎借鉴参考。
自动化外文引文一:[1]Nazri Nasir,Shabudin Mat. An automated visual tracking measurement for quantifying wing and body motion of free-flying houseflies[J]. Measurement,2021,143.[2]Rishikesh Kulkarni,Earu Banoth,Parama Pal. Automated surface feature detection using fringe projection: An autoregressive modeling-based approach[J]. Optics and Lasers in Engineering,2021,121.[3]Tengyue Fang,Peicong Li,Kunning Lin,NengwangChen,Yiyong Jiang,Jixin Chen,Dongxing Yuan,Jian Ma. Simultaneous underway analysis of nitrate and nitrite inestuarine and coastal waters using an automated integrated syringe-pump-based environmental-water analyzer[J]. Analytica Chimica Acta,2021,1076.[4]Shengfeng Chen,Jian Liu,Xiaosong Zhang,XinyuSuo,Enhui Lu,Jilong Guo,Jianxun Xi. Development ofpositioning system for Nuclear-fuel rod automated assembly[J]. Robotics and Computer Integrated Manufacturing,2021,61.[5]Cheng-Ta Lee,Yu-Ching Lee,Albert Y. Chen. In-building automated external defibrillator location planning and assessment through building information models[J]. Automation in Construction,2021,106.[6]Torgeir Aleti,Jason I. Pallant,Annamaria Tuan,Tom van Laer. Tweeting with the Stars: Automated Text Analysis of the Effect of Celebrity Social Media ications on ConsumerWord of Mouth[J]. Journal of Interactive Marketing,2021,48.[7]Daniel Bacioiu,Geoff Melton,MayorkinosPapaelias,Rob Shaw. Automated defect classification of SS304 TIG welding process using visible spectrum camera and machine learning[J]. NDT and E International,2021,107.[8]Marcus von der Au,Max Schwinn,KatharinaKuhlmeier,Claudia Büchel,Bj?rn Meermann. Development of an automated on-line purification HPLC single cell-ICP-MS approach for fast diatom analysis[J]. Analytica ChimicaActa,2021,1077.[9]Jitendra Mehar,Ajam Shekh,Nethravathy M. U.,R. Sarada,Vikas Singh Chauhan,Sandeep Mudliar. Automation ofpilot-scale open raceway pond: A case study of CO 2 -fed pHcontrol on Spirulina biomass, protein and phycocyanin production[J]. Journal of CO2 Utilization,2021,33.[10]John T. Sloop,Henry J.B. Bonilla,TinaHarville,Bradley T. Jones,George L. Donati. Automated matrix-matching calibration using standard dilution analysis withtwo internal standards and a simple three-port mixing chamber[J]. Talanta,2021,205.[11]Daniel J. Spade,Cathy Yue Bai,ChristyLambright,Justin M. Conley,Kim Boekelheide,L. Earl Gray. Corrigendum to “Validation of an automated counting procedure for phthalate-induced testicular multinucleated germ cells” [Toxicol. Lett. 290 (2021) 55–61][J]. Toxicology Letters,2021,313.[12]Christian P. Janssen,Shamsi T. Iqbal,Andrew L. Kun,Stella F. Donker. Interrupted by my car? Implications of interruption and interleaving research for automatedvehicles[J]. International Journal of Human - Computer Studies,2021,130.[13]Seunguk Lee,Si Kuan Thio,Sung-Yong Park,Sungwoo Bae. An automated 3D-printed smartphone platform integrated with optoelectrowetting (OEW) microfluidic chip for on-site monitoring of viable algae in water[J]. Harmful Algae,2021,88.[14]Yuxia Duan,Shicai Liu,Caiqi Hu,Junqi Hu,Hai Zhang,Yiqian Yan,Ning Tao,Cunlin Zhang,Xavier Maldague,Qiang Fang,Clemente Ibarra-Castanedo,Dapeng Chen,Xiaoli Li,Jianqiao Meng. Automated defect classification in infrared thermography based on a neural network[J]. NDT and E International,2021,107.[15]Alex M. Pagnozzi,Jurgen Fripp,Stephen E. Rose. Quantifying deep grey matter atrophy using automated segmentation approaches: A systematic review of structural MRI studies[J]. NeuroImage,2021,201.[16]Jin Ye,Zhihong Xuan,Bing Zhang,Yu Wu,LiLi,Songshan Wang,Gang Xie,Songxue Wang. Automated analysis of ochratoxin A in cereals and oil by iaffinity magnetic beads coupled to UPLC-FLD[J]. Food Control,2021,104.[17]Anne Bech Risum,Rasmus Bro. Using deep learning to evaluate peaks in chromatographic data[J].Talanta,2021,204.[18]Faris Elghaish,Sepehr Abrishami,M. Reza Hosseini,Soliman Abu-Samra,Mark Gaterell. Integrated project delivery with BIM: An automated EVM-based approach[J]. Automation in Construction,2021,106.[19]Carl J. Pearson,Michael Geden,Christopher B. Mayhorn. Who's the real expert here? Pedigree's unique bias on trust between human and automated advisers[J]. Applied Ergonomics,2021,81.[20]Vibhas Mishra,Dani?l M.J. Peeters,Mostafa M. Abdalla. Stiffness and buckling analysis of variablestiffness laminates including the effect of automated fibre placement defects[J]. Composite Structures,2021,226.[21]Jenny S. Wesche,Andreas Sonderegger. When computers take the lead: The automation of leadership[J]. Computers in Human Behavior,2021,101.[22]Murat Ayaz,Hüseyin Yüksel. Design of a new cost-efficient automation system for gas leak detection in industrial buildings[J]. Energy &amp; Buildings,2021,200.[23]Stefan A. Mann,Juliane Heide,Thomas Knott,Razvan Airini,Florin Bogdan Epureanu,Alexandru-FlorianDeftu,Antonia-Teona Deftu,Beatrice Mihaela Radu,Bogdan Amuzescu. Recording of multiple ion current components and action potentials in human induced pluripotent stem cell-derived cardiomyocytes via automated patch-clamp[J]. Journal of Pharmacological and Toxicological Methods,2021,100.[24]Rhar? de Almeida Cardoso,Alexandre Cury,Flavio Barbosa. Automated real-time damage detection strategy using raw dynamic measurements[J]. Engineering Structures,2021,196.[25]Mengmeng Zhong,Tielong Wang,Chengdu Qi,Guilong Peng,Meiling Lu,Jun Huang,Lee Blaney,Gang Yu. Automated online solid-phase extraction liquid chromatography tandem mass spectrometry investigation for simultaneous quantification of per- and polyfluoroalkyl substances, pharmaceuticals and personal care products, and organophosphorus flame retardants in environmental waters[J]. Journal of Chromatography A,2021,1602.[26]Pau Climent-Pér ez,Susanna Spinsante,Alex Mihailidis,Francisco Florez-Revuelta. A review on video-based active and assisted living technologies for automated lifelogging[J]. Expert Systems With Applications,2021,139.[27]William Snyder,Marisa Patti,Vanessa Troiani. An evaluation of automated tracing for orbitofrontal cortexsulcogyral pattern typing[J]. Journal of Neuroscience Methods,2021,326.[28]Juan Manuel Davila Delgado,LukumonOyedele,Anuoluwapo Ajayi,Lukman Akanbi,OlugbengaAkinade,Muhammad Bilal,Hakeem Owolabi. Robotics and automated systems in construction: Understanding industry-specific challenges for adoption[J]. Journal of Building Engineering,2021,26.[29]Mohamed Taher Alrefaie,Stever Summerskill,Thomas W Jackon. In a heart beat: Using driver’s physiological changes to determine the quality of a takeover in highly automated vehicles[J]. Accident Analysis andPrevention,2021,131.[30]Tawseef Ayoub Shaikh,Rashid Ali. Automated atrophy assessment for Alzheimer's disease diagnosis from brain MRI images[J]. Magnetic Resonance Imaging,2021,62.自动化外文参考文献二:[31]Vaanathi Sundaresan,Giovanna Zamboni,Campbell Le Heron,Peter M. Rothwell,Masud Husain,Marco Battaglini,Nicola De Stefano,Mark Jenkinson,Ludovica Griffanti. Automatedlesion segmentation with BIANCA: Impact of population-level features, classification algorithm and locally adaptive thresholding[J]. NeuroImage,2021,202.[32]Ho-Jun Suk,Edward S. Boyden,Ingrid van Welie. Advances in the automation of whole-cell patch clamp technology[J]. Journal of Neuroscience Methods,2021,326.[33]Ivana Duznovic,Mathias Diefenbach,Mubarak Ali,Tom Stein,Markus Biesalski,Wolfgang Ensinger. Automated measuring of mass transport through synthetic nanochannels functionalized with polyelectrolyte porous networks[J]. Journal of Membrane Science,2021,591.[34]James A.D. Cameron,Patrick Savoie,Mary E.Kaye,Erik J. Scheme. Design considerations for the processing system of a CNN-based automated surveillance system[J]. Expert Systems With Applications,2021,136.[35]Ebrahim Azadniya,Gertrud E. Morlock. Automated piezoelectric spraying of biological and enzymatic assays for effect-directed analysis of planar chromatograms[J]. Journal of Chromatography A,2021,1602.[36]Lilla Z?llei,Camilo Jaimes,Elie Saliba,P. Ellen Grant,Anastasia Yendiki. TRActs constrained by UnderLying INfant anatomy (TRACULInA): An automated probabilistic tractography tool with anatomical priors for use in the newborn brain[J]. NeuroImage,2021,199.[37]Kate?ina Fikarová,David J. Cocovi-Solberg,María Rosende,Burkhard Horstkotte,Hana Sklená?ová,Manuel Miró. A flow-based platform hyphenated to on-line liquid chromatography for automatic leaching tests of chemical additives from microplastics into seawater[J]. Journal of Chromatography A,2021,1602.[38]Darko ?tern,Christian Payer,Martin Urschler. Automated age estimation from MRI volumes of the hand[J]. Medical Image Analysis,2021,58.[39]Jacques Blum,Holger Heumann,Eric Nardon,Xiao Song. Automating the design of tokamak experiment scenarios[J]. Journal of Computational Physics,2021,394.[40]Elton F. de S. Soares,Carlos Alberto V.Campos,Sidney C. de Lucena. Online travel mode detection method using automated machine learning and feature engineering[J]. Future Generation Computer Systems,2021,101.[41]M. Marouli,S. Pommé. Autom ated optical distance measurements for counting at a defined solid angle[J].Applied Radiation and Isotopes,2021,153.[42]Yi Dai,Zhen-Hua Yu,Jian-Bo Zhan,Bao-Shan Yue,Jiao Xie,Hao Wang,Xin-Sheng Chai. Determination of starch gelatinization temperatures by an automated headspace gas chromatography[J]. Journal of Chromatography A,2021,1602.[43]Marius Tarp?,Tobias Friis,Peter Olsen,MartinJuul,Christos Georgakis,Rune Brincker. Automated reduction of statistical errors in the estimated correlation functionmatrix for operational modal analysis[J]. Mechanical Systems and Signal Processing,2021,132.[44]Wenxia Dai,Bisheng Yang,Xinlian Liang,ZhenDong,Ronggang Huang,Yunsheng Wang,Wuyan Li. Automated fusionof forest airborne and terrestrial point clouds throughcanopy density analysis[J]. ISPRS Journal of Photogrammetry and Remote Sensing,2021,156.[45]Jyh-Haur Woo,Marcus Ang,Hla Myint Htoon,Donald Tan. Descemet Membrane Endothelial Keratoplasty Versus Descemet Stripping Automated Endothelial Keratoplasty andPenetrating Keratoplasty[J]. American Journal of Ophthalmology,2021,207.[46]F. Wilde,S. Marsen,T. Stange,D. Moseev,J.W. Oosterbeek,H.P. Laqua,R.C. Wolf,K. Avramidis,G.Gantenbein,I.Gr. Pagonakis,S. Illy,J. Jelonnek,M.K. Thumm,W7-X team. Automated mode recovery for gyrotrons demonstrated at Wendelstein 7-X[J]. Fusion Engineering and Design,2021,148.[47]Andrew Kozbial,Lekhana Bhandary,Shashi K. Murthy. Effect of yte seeding density on dendritic cell generation in an automated perfusion-based culture system[J]. Biochemical Engineering Journal,2021,150.[48]Wen-Hao Su,Steven A. Fennimore,David C. Slaughter. Fluorescence imaging for rapid monitoring of translocation behaviour of systemic markers in snap beans for automatedcrop/weed discrimination[J]. Biosystems Engineering,2021,186.[49]Ki-Taek Lim,Dinesh K. Patel,Hoon Se,JanghoKim,Jong Hoon Chung. A fully automated bioreactor system for precise control of stem cell proliferation anddifferentiation[J]. Biochemical Engineering Journal,2021,150.[50]Mitchell L. Cunningham,Michael A. Regan,Timothy Horberry,Kamal Weeratunga,Vinayak Dixit. Public opinion about automated vehicles in Australia: Results from a large-scale national survey[J]. Transportation Research Part A,2021,129.[51]Yi Xie,Qiaobei You,Pingyang Dai,Shuyi Wang,Peiyi Hong,Guokun Liu,Jun Yu,Xilong Sun,Yongming Zeng. How to achieve auto-identification in Raman analysis by spectral feature extraction &amp; Adaptive Hypergraph[J].Spectrochimica Acta Part A: Molecular and Biomolecular Spectroscopy,2021,222.[52]Ozal Yildirim,Muhammed Talo,Betul Ay,Ulas Baran Baloglu,Galip Aydin,U. Rajendra Acharya. Automated detection of diabetic subject using pre-trained 2D-CNN models with frequency spectrum images extracted from heart ratesignals[J]. Computers in Biology and Medicine,2021,113.[53]Marius Kern,Laura Tusa,Thomas Lei?ner,Karl Gerald van den Boogaart,Jens Gutzmer. Optimal sensor selection for sensor-based sorting based on automated mineralogy data[J]. Journal of Cleaner Production,2021,234.[54]Karim Keddadouche,Régis Braucher,Didier L.Bourlès,Mélanie Baroni,Valéry Guillou,La?titia Léanni,Georges Auma?tre. Design and performance of an automated chemical extraction bench for the preparation of 10 Be and 26 Al targets to be analyzed by accelerator mass spectrometry[J]. Nuclear Inst. and Methods in Physics Research, B,2021,456.[55]Christian P. Janssen,Stella F. Donker,Duncan P. Brumby,Andrew L. Kun. History and future of human-automation interaction[J]. International Journal of Human - Computer Studies,2021,131.[56]Victoriya Orlovskaya,Olga Fedorova,Michail Nadporojskii,Raisa Krasikova. A fully automated azeotropic drying free synthesis of O -(2-[ 18 F]fluoroethyl)- l -tyrosine ([ 18 F]FET) using tetrabutylammonium tosylate[J]. Applied Radiation and Isotopes,2021,152.[57]Dinesh Krishnamoorthy,Kjetil Fjalestad,Sigurd Skogestad. Optimal operation of oil and gas production usingsimple feedback control structures[J]. Control Engineering Practice,2021,91.[58]Nick Oliver,Thomas Calvard,Kristina Poto?nik. Safe limits, mindful organizing and loss of control in commercial aviation[J]. Safety Science,2021,120.[59]Bo Sui,Nils Lubbe,Jonas B?rgman. A clustering approach to developing car-to-two-wheeler test scenarios for the assessment of Automated Emergency Braking in China using in-depth Chinese crash data[J]. Accident Analysis and Prevention,2021,132.[60]Ji-Seok Yoon,Eun Young Choi,Maliazurina Saad,Tae-Sun Choi. Automated integrated system for stained neuron detection: An end-to-end framework with a high negative predictive rate[J]. Computer Methods and Programs in Biomedicine,2021,180.自动化外文参考文献八:[61]Min Wang,Barbara E. Glick-Wilson,Qi-Huang Zheng. Facile fully automated radiosynthesis and quality control of O -(2-[ 18 F]fluoroethyl)- l -tyrosine ([ 18 F]FET) for human brain tumor imaging[J]. Applied Radiation andIsotopes,2021,154.[62]Fabian Pütz,Finbarr Murphy,Martin Mullins,LisaO'Malley. Connected automated vehicles and insurance: Analysing future market-structure from a business ecosystem perspective[J]. Technology in Society,2021,59.[63]Victoria A. Banks,Neville A. Stanton,Katherine L. Plant. Who is responsible for automated driving? A macro-level insight into automated driving in the United Kingdom using the Risk Management Framework and Social NetworkAnalysis[J]. Applied Ergonomics,2021,81.[64]Yingjun Ye,Xiaohui Zhang,Jian Sun. Automated vehicle’s behavior decision making using deep reinforcement learning and high-fidelity simulation environment[J]. Transportation Research Part C,2021,107.[65]Hasan Alkaf,Jameleddine Hassine,TahaBinalialhag,Daniel Amyot. An automated change impact analysis approach for User Requirements Notation models[J]. TheJournal of Systems &amp; Software,2021,157.[66]Zonghua Luo,Jiwei Gu,Robert C. Dennett,Gregory G. Gaehle,Joel S. Perlmutter,Delphine L. Chen,Tammie L.S. Benzinger,Zhude Tu. Automated production of a sphingosine-1 phosphate receptor 1 (S1P1) PET radiopharmaceutical [ 11C]CS1P1 for human use[J]. Applied Radiation andIsotopes,2021,152.[67]Sarfraz Qureshi,Wu Jiacheng,Jeroen Anton van Kan. Automated alignment and focusing system for nuclear microprobes[J]. Nuclear Inst. and Methods in Physics Research, B,2021,456.[68]Srikanth Sagar Bangaru,Chao Wang,MarwaHassan,Hyun Woo Jeon,Tarun Ayiluri. Estimation of the degreeof hydration of concrete through automated machine learning based microstructure analysis – A study on effect of image magnification[J]. Advanced Engineering Informatics,2021,42.[69]Fang Tengyue,Li Peicong,Lin Kunning,Chen Nengwang,Jiang Yiyong,Chen Jixin,Yuan Dongxing,Ma Jian. Simultaneous underway analysis of nitrate and nitrite in estuarine and coastal waters using an automated integrated syringe-pump-based environmental-water analyzer.[J]. Analytica chimica acta,2021,1076.[70]Ramos Inês I,Carl Peter,Schneider RudolfJ,Segundo Marcela A. Automated lab-on-valve sequential injection ELISA for determination of carbamazepine.[J]. Analytica chimica acta,2021,1076.[71]Au Marcus von der,Schwinn Max,Kuhlmeier Katharina,Büchel Claudia,Meermann Bj?rn. Development of an automated on-line purification HPLC single cell-ICP-MS approach for fast diatom analysis.[J]. Analytica chimica acta,2021,1077.[72]Risum Anne Bech,Bro Rasmus. Using deep learning to evaluate peaks in chromatographic data.[J].Talanta,2021,204.[73]Spade Daniel J,Bai Cathy Yue,LambrightChristy,Conley Justin M,Boekelheide Kim,Gray L Earl. Corrigendum to "Validation of an automated counting procedure for phthalate-induced testicular multinucleated germ cells" [Toxicol. Lett. 290 (2021) 55-61].[J]. Toxicologyletters,2021,313.[74]Zhong Mengmeng,Wang Tielong,Qi Chengdu,Peng Guilong,Lu Meiling,Huang Jun,Blaney Lee,Yu Gang. Automated online solid-phase extraction liquid chromatography tandem mass spectrometry investigation for simultaneousquantification of per- and polyfluoroalkyl substances, pharmaceuticals and personal care products, and organophosphorus flame retardants in environmental waters.[J]. Journal of chromatography. A,2021,1602.[75]Stein Christopher J,Reiher Markus. autoCAS: A Program for Fully Automated MulticonfigurationalCalculations.[J]. Journal of computationalchemistry,2021,40(25).[76]Alrefaie Mohamed Taher,Summerskill Stever,Jackon Thomas W. In a heart beat: Using driver's physiological changes to determine the quality of a takeover in highly automated vehicles.[J]. Accident; analysis andprevention,2021,131.[77]Shaikh Tawseef Ayoub,Ali Rashid. Automatedatrophy assessment for Alzheimer's disease diagnosis frombrain MRI images.[J]. Magnetic resonance imaging,2021,62.[78]Xie Yi,You Qiaobei,Dai Pingyang,Wang Shuyi,Hong Peiyi,Liu Guokun,Yu Jun,Sun Xilong,Zeng Yongming. How to achieve auto-identification in Raman analysis by spectral feature extraction &amp; Adaptive Hypergraph.[J]. Spectrochimica acta. Part A, Molecular and biomolecular spectroscopy,2021,222.[79]Azadniya Ebrahim,Morlock Gertrud E. Automated piezoelectric spraying of biological and enzymatic assays for effect-directed analysis of planar chromatograms.[J]. Journal of chromatography. A,2021,1602.[80]Fikarová Kate?ina,Cocovi-Solberg David J,Rosende María,Horstkotte Burkhard,Sklená?ová Hana,Miró Manuel. Aflow-based platform hyphenated to on-line liquid chromatography for automatic leaching tests of chemical additives from microplastics into seawater.[J]. Journal of chromatography. A,2021,1602.[81]Moitra Dipanjan,Mandal Rakesh Kr. Automated AJCC (7th edition) staging of non-small cell lung cancer (NSCLC) using deep convolutional neural network (CNN) and recurrent neural network (RNN).[J]. Health information science and systems,2021,7(1).[82]Ramos-Payán María. Liquid - Phase microextraction and electromembrane extraction in millifluidic devices:A tutorial.[J]. Analytica chimica acta,2021,1080.[83]Z?llei Lilla,Jaimes Camilo,Saliba Elie,Grant P Ellen,Yendiki Anastasia. TRActs constrained by UnderLying INfant anatomy (TRACULInA): An automated probabilistic tractography tool with anatomical priors for use in the newborn brain.[J]. NeuroImage,2021,199.[84]Sedghi Gamechi Zahra,Bons Lidia R,Giordano Marco,Bos Daniel,Budde Ricardo P J,Kofoed Klaus F,Pedersen Jesper Holst,Roos-Hesselink Jolien W,de Bruijne Marleen. Automated 3D segmentation and diameter measurement of the thoracic aorta on non-contrast enhanced CT.[J]. European radiology,2021,29(9).[85]Smith Claire,Galland Barbara C,de Bruin Willemijn E,Taylor Rachael W. Feasibility of Automated Cameras to Measure Screen Use in Adolescents.[J]. American journal of preventive medicine,2021,57(3).[86]Lambert Marie-?ve,Arsenault Julie,AudetPascal,Delisle Benjamin,D'Allaire Sylvie. Evaluating an automated clustering approach in a perspective of ongoing surveillance of porcine reproductive and respiratory syndrome virus (PRRSV) field strains.[J]. Infection, genetics and evolution : journal of molecular epidemiology and evolutionary genetics in infectious diseases,2021,73.[87]Slanetz Priscilla J. Does Computer-aided Detection Help in Interpretation of Automated Breast US?[J]. Radiology,2021,292(3).[88]Sander Laura,Pezold Simon,Andermatt Simon,Amann Michael,Meier Dominik,Wendebourg Maria J,Sinnecker Tim,Radue Ernst-Wilhelm,Naegelin Yvonne,Granziera Cristina,Kappos Ludwig,Wuerfel Jens,Cattin Philippe,Schlaeger Regina. Accurate, rapid and reliable, fully automated MRI brainstem segmentation for application in multiple sclerosis and neurodegenerative diseases.[J]. Human brainmapping,2021,40(14).[89]Pajkossy Péter,Sz?ll?si ?gnes,Racsmány Mihály. Retrieval practice decreases processing load of recall: Evidence revealed by pupillometry.[J]. International journal of psychophysiology : official journal of the International Organization of Psychophysiology,2021,143.[90]Kaiser Eric A,Igdalova Aleksandra,Aguirre Geoffrey K,Cucchiara Brett. A web-based, branching logic questionnaire for the automated classification ofmigraine.[J]. Cephalalgia : an international journal of headache,2021,39(10).自动化外文参考文献四:[91]Kim Jin Ju,Park Younhee,Choi Dasom,Kim Hyon Suk. Performance Evaluation of a New Automated Chemiluminescent Ianalyzer-Based Interferon-Gamma Releasing Assay AdvanSure I3 in Comparison With the QuantiFERON-TB Gold In-Tube Assay.[J]. Annals of laboratory medicine,2021,40(1).[92]Yang Shanling,Gao Xican,Liu Liwen,Shu Rui,Yan Jingru,Zhang Ge,Xiao Yao,Ju Yan,Zhao Ni,Song Hongping. Performance and Reading Time of Automated Breast US with or without Computer-aided Detection.[J]. Radiology,2021,292(3).[93]Hung Andrew J,Chen Jian,Ghodoussipour Saum,OhPaul J,Liu Zequn,Nguyen Jessica,Purushotham Sanjay,Gill Inderbir S,Liu Yan. A deep-learning model using automated performance metrics and clinical features to predict urinary continence recovery after robot-assisted radical prostatectomy.[J]. BJU international,2021,124(3).[94]Kim Ryan S,Kim Gene. Double Descemet Stripping Automated Endothelial Keratoplasty (DSAEK): Secondary DSAEK Without Removal of the Failed Primary DSAEK Graft.[J]. Ophthalmology,2021,126(9).[95]Sargent Alexandra,Theofanous Ioannis,Ferris Sarah. Improving laboratory workflow through automated pre-processing of SurePath specimens for human papillomavirus testing with the Abbott RealTime assay.[J]. Cytopathology : official journal of the British Society for Clinical Cytology,2021,30(5).[96]Saba Tanzila. Automated lung nodule detection and classification based on multiple classifiers voting.[J]. Microscopy research and technique,2021,82(9).[97]Ivan D. Welsh,Jane R. Allison. Automated simultaneous assignment of bond orders and formal charges[J]. Journal of Cheminformatics,2021,11(1).[98]Willem Jespers,MauricioEsguerra,Johan ?qvist,Hugo Gutiérrez-de-Terán. QligFEP: an automated workflow for small molecule free energycalculations in Q[J]. Journal of Cheminformatics,2021,11(1).[99]Manav Raj,Robert Seamans. Primer on artificial intelligence and robotics[J]. Journal of OrganizationDesign,2021,8(1).[100]Yvette Pronk,Peter Pilot,Justus M.Brinkman,Ronald J. Heerwaarden,Walter Weegen. Response rate and costs for automated patient-reported outcomes collection alone compared to combined automated and manual collection[J]. Journal of Patient-Reported Outcomes,2021,3(1).[101]Tristan Martin,Ana?s Moyon,Cyril Fersing,Evan Terrier,Aude Gouillet,Fabienne Giraud,BenjaminGuillet,Philippe Garrigue. Have you looked for “stranger things” in your automated PET dose dispensing system? A process and operators qualification scheme[J]. EJNMMI Radiopharmacy and Chemistry,2021,4(1).[102]Manuel Peuster,Michael Marchetti,Ger ardo García de Blas,Holger Karl. Automated testing of NFV orchestrators against carrier-grade multi-PoP scenarios using emulation-based smoke testing[J]. EURASIP Journal on Wireless ications and Networking,2021,2021(1).[103]R. Ferrús,O. Sallent,J. Pérez-Romero,R. Agustí. On the automation of RAN slicing provisioning: solution framework and applicability examples[J]. EURASIP Journal on Wireless ications and Networking,2021,2021(1).[104]Duo Li,Peter Wagner. Impacts of gradual automated vehicle penetration on motorway operation: a comprehensive evaluation[J]. European Transport Research Review,2021,11(1).[105]Abel Gómez,Ricardo J. Rodríguez,María-Emilia Cambronero,Valentín Valero. Profiling the publish/subscribe paradigm for automated analysis using colored Petri nets[J]. Software &amp; Systems Modeling,2021,18(5).[106]Dipanjan Moitra,Rakesh Kr. Mandal. Automated AJCC (7th edition) staging of non-small cell lung cancer (NSCLC) using deep convolutional neural network (CNN) and recurrent neural network (RNN)[J]. Health Information Science and Systems,2021,7(1).[107]Marta D’Alonzo,Laura Martincich,Agnese Fenoglio,Valentina Giannini,Lisa Cellini,ViolaLiberale,Nicoletta Biglia. Nipple-sparing mastectomy: external validation of a three-dimensional automated method to predict nipple occult tumour involvement on preoperative breast MRI[J]. European Radiology Experimental,2021,3(1).[108]N. V. Dozmorov,A. S. Bogomolov,A. V. Baklanov. An Automated Apparatus for Measuring Spectral Dependences ofthe Mass Spectra and Velocity Map Images of Photofragments[J]. Instruments and Experimental Techniques,2021,62(4).[109]Zhiqiang Sun,Bingzhao Gao,Jiaqi Jin,Kazushi Sanada. Modelling, Analysis and Simulation of a Novel Automated Manual Transmission with Gearshift Assistant Mechanism[J]. International Journal of Automotive Technology,2021,20(5).[110]Andrés Vega,Mariano Córdoba,Mauricio Castro-Franco,Mónica Balzarini. Protocol for automating errorremoval from yield maps[J]. Precision Agriculture,2021,20(5).[111]Bethany L. Lussier,DaiWai M. Olson,Venkatesh Aiyagari. Automated Pupillometry in Neurocritical Care: Research and Practice[J]. Current Neurology and Neuroscience Reports,2021,19(10).[112] B. Haskali,Peter D. Roselt,David Binns,Amit Hetsron,Stan Poniger,Craig A. Hutton,Rodney J. Hicks. Automated preparation of clinical grade [ 68 Ga]Ga-DOTA-CP04, a cholecystokinin-2 receptor agonist, using iPHASE MultiSyn synthesis platform[J]. EJNMMI Radiopharmacy andChemistry,2021,4(1).[113]Ju Hyun Ahn,Minho Na,Sungkwan Koo,HyunsooChun,Inhwan Kim,Jong Won Hur,Jae Hyuk Lee,Jong G. Ok. Development of a fully automated desktop chemical vapor deposition system for programmable and controlled carbon nanotube growth[J]. Micro and Nano Systems Letters,2021,7(1).[114]Kamellia Shahi,Brenda Y. McCabe,Arash Shahi. Framework for Automated Model-Based e-Permitting System forMunicipal Jurisdictions[J]. Journal of Management in Engineering,2021,35(6).[115]Ahmed Khalafallah,Yasmin Shalaby. Change Orders: Automating Comparative Data Analysis and Controlling Impacts in Public Projects[J]. Journal of Construction Engineering and Management,2021,145(11).[116]José ?. Martínez-Huertas,OlgaJastrzebska,Ricardo Olmos,José A. León. Automated summary evaluation with inbuilt rubric method: An alternative to constructed responses and multiple-choice testsassessments[J]. Assessment &amp; Evaluation in Higher Education,2021,44(7).[117]Samsonov,Koshel,Walther,Jenny. Automated placement of supplementary contour lines[J]. International Journal of Geographical Information Science,2021,33(10).[118]Veronika V. Odintsova,Peter J. Roetman,Hill F. Ip,René Pool,Camiel M. Van der Laan,Klodiana-DaphneTona,Robert R.J.M. Vermeiren,Dorret I. Boomsma. Genomics of human aggression: current state of genome-wide studies and an automated systematic review tool[J]. PsychiatricGenetics,2021,29(5).[119]Sebastian Eggert,Dietmar W Hutmacher. In vitro disease models 4.0 via automation and high-throughput processing[J]. Biofabrication,2021,11(4).[120]Asad Mahmood,Faizan Ahmad,Zubair Shafiq,Padmini Srinivasan,Fareed Zaffar. A Girl Has No Name: Automated Authorship Obfuscation using Mutant-X[J]. Proceedings on Privacy Enhancing Technologies,2021,2021(4).。
- 1、下载文档前请自行甄别文档内容的完整性,平台不提供额外的编辑、内容补充、找答案等附加服务。
- 2、"仅部分预览"的文档,不可在线预览部分如存在完整性等问题,可反馈申请退款(可完整预览的文档不适用该条件!)。
- 3、如文档侵犯您的权益,请联系客服反馈,我们会尽快为您处理(人工客服工作时间:9:00-18:30)。
8403735 (Set of 5) Acrylonitrile-butadiene-styrene (ABS) No, (only desinfectable) Primus, Fabius GS, Julian, Cato, Cicero, Evita family, Savina etc.
SpiroLife® • Order number: • Material: • Sterilizable: • Compatible Dräger devices:
在60 l/min流速时压降损失< 0.2mbar 其他技术明显偏高 (1 – 2 mbar)
压差技术(Datex) 容易被水汽阻塞
热丝技术的优势
单一技术
在麻醉时不论病人类型都只使用一种传感器 即使儿童或新生儿也无需更换传感器
成熟技术
Dräger 几十年的技术成为核心竞争力 请注意: 经过深入的市场调查,最新技术的比较, Dräger 决定在我们最新开发的麻醉 工作站ZEUS上仍然保持采用这一技术!!!
7
Flow and oxygen sensors | 02.06.2012
The sensors present themselves Product group 266
Spirolog® • Order number: • Material: • Sterilizable: • Compatible Dräger devices:
主要目的:
为客户提供长效选择 保持市场份额,提高流量传感器销售
市场推广 (I)
2004-1-1 开始全球推广
计划销售数量:
in 2004: 6.000 件 in 2005: 10.000 件
订货号
Байду номын сангаас
SpiroLife®
MK01900
试销价
RMB 3740.00/件
兼容性
SpiroLife® 适用于使用Spirolog® 的所有机器
例如 Primus, Cicero, Evita 系列
麻醉工作站Zeus 只使用 SpiroLife® 传感器 !
市场推广(II)
使用寿命
如使用方法正确,大约24个月 由于不能排除人为损坏原因,所以没有保质期
原包装
作为高质量的标志,每个SpiroLife®都有一个金属盒包装. 封面有六种不同的Dräger Medical 历史主题
MK01900 Polyphenylsulphone (PPSU) Yes, in steam (134°C) Zeus, Primus, Fabius GS, Julian, Cato, Cicero, Evita family, Savina etc.
Budget 2010 for the whole Product group 266: ??? 11 Mio Euro !!!
Neonatal flow sensor insert • Order number: • Material: • Sterilizable: • Compatible Dräger devices:
8410179 (Set of 5) Polysulfon (PSU) Yes, in steam (134°C) Basic Babylog flow sensors
8 Flow and oxygen sensors | 02.06.2012
推广SpiroLife®的目的
SpiroLife® 流量传感器
全新/ 目前Spirolog® 传感器的进一步发展 长寿命, 可以高压消毒 寿命: 大约 10 倍 Spirolog® (~ 24 个月) 完全兼容 Spirolog® 与 Spirolog® 的新外形一致
The sensors present themselves Product group 266
Neonatal Y piece flow sensor • Order number: • Material: • Sterilizable: • Compatible Dräger devices: Neonatal ISO 15 flow sensor • Order number: • Material: • Sterilizable: • Compatible Dräger devices:
SpiroLife®
Dräger Medical 全新的流量传感器
流量传感器 不仅仅只是 “某个附件”
容量计 Flowsensor 报警 分钟通气量高/低
容量参数 分钟通气量, 潮气量
提供
流速波形
流速触发 在自主通气模式 如 SIMV
容量环
流量传感器 连接示意图
热丝技术
如何测量流量...... .....…热丝的温度是一定的温度, 算的气流的流量 (.....…增加额外电流恒定 原来的温度。)
SpiroLife®的卖点
使用寿命显著延长 可重复使用 符合呼吸回路整体消毒的要求 Dräger 在热丝技术上的竞争力 100% Dräger 原装附件
高质量,对病人和使用者安全
CE标准
.....…有气流流过时带走热量,温度降低,导致电热丝的阻值变化,通过检测电流变
Kaltes Gas
Warmes Gas
Heißer Draht
热丝技术的优势
响应时间最快
T90 (响应时间) 从 10 ms 降至 1 ms, 明显快于其他技术 (50 - 150 ms)
测量时不会产生压降损失
8410185 Polysulfon (PSU) Yes, in steam (134°C) Babylog 8000, Evita Neo Flow
8411130 Polysulfon (PSU) Yes, in steam (134°C) Babylog 8000, Evita Neo Flow