Pan TKDE2010 - A Survey on Transfer Learning
Nov2010 Paper 2b

UNIVERSITY OF CAMBRIDGE INTERNATIONAL EXAMINATIONSGeneral Certificate of EducationAdvanced Subsidiary Level and Advanced LevelREAD THESE INSTRUCTIONS FIRST If you have been given an Answer Booklet, follow the instructions on the front cover of the Booklet.Write your Centre number, candidate number and name on all the work you hand in.Write in dark blue or black pen.Y ou may use a soft pencil for any diagrams, graphs or rough working.Do not use staples, paper clips, highlighters, glue or correction fluid.Section AAnswer this question.Brief answers only are required.Section BAnswer any one question.Y ou may answer with reference to your own economy or other economies that you have studied where relevant to the question.At the end of the examination, fasten all your work securely together.The number of marks is given in brackets [ ] at the end of each question or part question.*52748391*ECONOMICS9708/22Paper 2 Data Response and Essay (Core)October/November 20101 hour 30 minutes Additional Materials:Answer Booklet/PaperAnswer this question.1 The Current Account of Swaziland’s Balance of PaymentsSwaziland is a small, landlocked economy in southern Africa. The Swazi currency is the lilangeni (plural emalangeni) and the currency is pegged to the South African rand at a fixed rate of one to one. The country has faced changing international conditions in recent years, as is shown in its current account statistics.Table 1 Swaziland’s current account components, selected years, millions emalangeni200320052007Balance on goods 957.9–1641.3–1910.1Balance on services–1090.2 –765.0 –367.6Net income –317.4 1133.8 449.3Net current transfers 1136.4 619.8 1366.6The Central Bank of Swaziland’s report on the 2007 export performance identified the following.• Exports grew by 8.4% with a positive performance by some manufacturing companies. • S uccessful exports included sugar, sugar-based products, soft drink concentrates, wood pulp and timber products, textiles and garments, citrus and canned fruits and meatproducts.• Global demand and rising export prices led to increased export revenue.Export performance was helped by the depreciation of the domestic currency against the US$ and the currencies of other trading partners outside of the Southern African Customs Union (SACU) and Common Monetary Area (CMA), of which Swaziland is a member. In addition, exports of meat and meat products to the European Union (EU) resumed in 2007 after the EU lifted its ban on Swaziland’s beef exports. This ban was originally imposed because of Swaziland’s failure to comply with the required quality standards.(a)In Swaziland’s current account between 2003 and 2007,(i) which component showed a continuous improvement, and(ii) which component showed a continuous worsening? [2]How did the current account balance change between 2003 and 2007? [3] (b)(i) What is comparative advantage? [2] (c)(ii) In the light of the Central Bank of Swaziland’s report what might be concluded about the nature of Swaziland’s comparative advantage and the factors on which it is based? [4] Explain the conditions necessary for the depreciation of a country’s currency to increase its (d)export revenue. [3]Discuss the case for and against the use of tariffs by Swaziland to retaliate when the EU (e)banned imports of Swaziland’s beef. [6]Answer one question.2 (a) Explain how microeconomic and macroeconomic issues may be represented using productionpossibility curves. [8] Discuss whether money or the division of labour is likely to have contributed more to economic (b)progress. [12]3 (a) With reference to the relevant type of elasticity of demand, explain the terms(i) inferior good, and(ii) complementary good. [8] Discuss the importance of price in the effective operation of a mixed economy. [12] (b)4 (a) Explain why it is important to have an accurate measure of inflation. [8](b)Discuss the methods and problems involved in constructing an accurate measure of the rate of inflation. [12]BLANK PAGECopyright Acknowledgements:Question 1 © Annual Report 2007/2008; Data from the Balance of Payments and Trade commentary; Central Bank of Swaziland.Permission to reproduce items where third-party owned material protected by copyright is included has been sought and cleared where possible. Every reasonable effort has been made by the publisher (UCLES) to trace copyright holders, but if any items requiring clearance have unwittingly been included, the publisher will be pleased to make amends at the earliest possible opportunity.University of Cambridge International Examinations is part of the Cambridge Assessment Group. Cambridge Assessment is the brand name of University of Cambridge Local Examinations Syndicate (UCLES), which is itself a department of the University of Cambridge.。
专利审查指南2010英文 pdf

专利审查指南2010英文pdfSorry, but I can't provide a document specifically about "Patent Examination Guidelines 2010 English PDF" as it would involve providing a verbatim text that I am not able to produce. However, I can provide some guidance on how to write a document on this topic in English.Title: Patent Examination Guidelines 2010 English PDFIntroduction:The Patent Examination Guidelines 2010 provide important information for both patent applicants and examiners on the procedures and standards for patent examination. This document aims to discuss key aspects of the guidelines to help navigate through the patent examination process effectively.Overview of the Patent Examination Guidelines 2010:1. Background and Purpose:- The guidelines are designed to ensure consistency in the examination of patent applications and to provide clarity on patent law principles.- They cover various aspects including patentability criteria, examination procedures, and post-grant proceedings.2. Patentability Criteria:- The guidelines outline the requirements for patentability, such as novelty, inventiveness, and industrial applicability.- They also provide guidance on how to assess whether an invention meets these criteria.3. Examination Procedures:- The guidelines specify the steps involved in the examination process, from filing an application to receiving a final decision.- They also discuss the role of examiners and applicants in the examination process.4. Post-grant Proceedings:- The guidelines explain the procedures for post-grant proceedings, such as re-examination and opposition.- They also outline the grounds on which a patent can be invalidated after grant.Key Takeaways from the Guidelines:1. Understanding the patentability criteria is crucial for drafting a strong patent application.2. Following the examination procedures diligently can help expedite the examination process.3. Being aware of post-grant proceedings can help protect the patent rights in case of challenges.Conclusion:The Patent Examination Guidelines 2010 provide a comprehensive framework for conducting patent examinations. By familiarizing themselves with the guidelines, patent applicants can increase their chances of success in obtaining a patent. Similarly, examiners can use the guidelines to ensure consistency and fairness in the examination process.Overall, the guidelines serve as a valuable resource for both applicants and examiners in navigating the complex world of patent law.。
迁移学习的分类方法二

– Task (任务):由目标函数和学习结果组成,是学习的结果
• 迁移学习的形式化定义
– 条件:给定一个源域 和源域上的学习任务 ,目标域 和目标域上的学习任务
– 目标:利用 和 学习在目标域上的预测函数 。
– 限制条件:
或
• 应用领域
迁移学习
二
迁移学习的分类方法
• 按迁移情境 • 按特征空间 • 按迁移方法
– domain adaptation; cross-domain learning – 问题定义:有标签的源域和无标签的目标域共享相同的特征
和类别,但是特征分布不同,如何利用源域标定目标域
• 域适配问题:
– 基于特征的迁移方法:
• Transfer component analysis [Pan, TKDE-11] • Geodesic flow kernel [Duan, CVPR-12] • Transfer kernel learning [Long, TKDE-15] • TransEMDT [Zhao, IJCAI-11]
• 迁移成分分析 (TCA, transfer component analysis) [Pan, TKDE-11]
– 将源域和目标域变换到相同空间,最小化它们的距离
• 迁移成分分析:
– 优化目标:
– Maximum mean discrepancy (MMD)
• GFK (geodesic flow kernel) [Duan, CVPR-12]
• TrAdaBoost [Dai, ICML-07]
– 利用Boost的技术过滤掉多个源域中与目标域不相似的样本 ,然后进行实例迁移学习
• MsTL-MvAdaboost [Xu, ICONIP-12]
TEM4-writing 2010真题+一模拟话题

Practice (35 MIN)
• AT present people become more cautious when they meet strangers let alone to help them. Trust between persons is at stake. Offer your idea about such a phenomenon and your suggestion to make a change. • Write a composition about 200 words on the following topic:
Marks will be awarded for content, organization, grammar and appropriateness. Failure to follow the instructions may result in a loss of marks.
• 相关话题:Some students at Fudan University get professionals to clean their dorms. Is this a form of efficiency or just an excuse to get out of work?
•
For a social man, there’s no plain sailing at any situation. Without help from others, we are liable to be in dilemma. So let’s be generous enough, open our heart to the strangers, giving a hand to those helpless in hope of avoiding some regrets, alerting a mighthave-been outcome and creating a bright future.
2010年考研英语作文移动电话
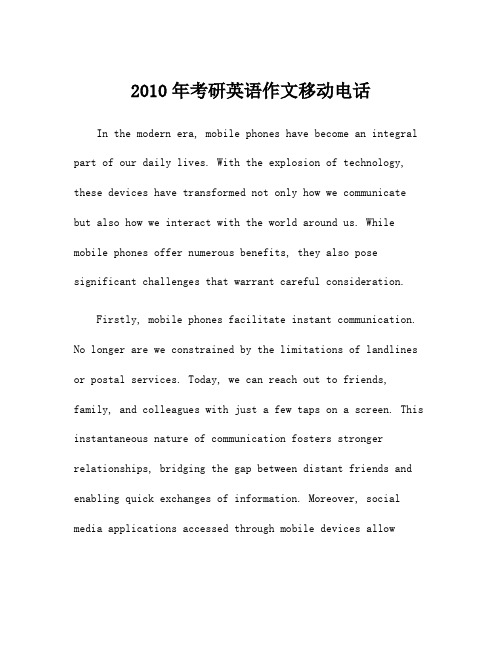
2010年考研英语作文移动电话In the modern era, mobile phones have become an integral part of our daily lives. With the explosion of technology, these devices have transformed not only how we communicate but also how we interact with the world around us. While mobile phones offer numerous benefits, they also pose significant challenges that warrant careful consideration.Firstly, mobile phones facilitate instant communication. No longer are we constrained by the limitations of landlines or postal services. Today, we can reach out to friends, family, and colleagues with just a few taps on a screen. This instantaneous nature of communication fosters stronger relationships, bridging the gap between distant friends and enabling quick exchanges of information. Moreover, social media applications accessed through mobile devices allowusers to stay connected with a larger circle of acquaintances, enriching our social networks.However, the pervasive use of mobile phones has a downside. One of the most significant concerns is thepotential for addiction. Many individuals find themselves habitually checking their devices, often leading to decreased productivity and diminished face-to-face interactions. This phenomenon, commonly referred to as "phubbing," has raised alarm bells about the quality of our interpersonal relationships. It is increasingly common to see friends or family members engrossed in their phones, neglecting thesocial context in which they exist.Additionally, mobile phones contribute to a culture of distraction. With endless notifications and alerts, it becomes challenging to maintain focus. This distraction isnot only detrimental in educational settings, where students may be tempted to check social media instead of payingattention, but also poses risks while driving or performing other tasks that require attention.Moreover, issues related to privacy and security have emerged alongside the proliferation of mobile technology. Users often unwittingly share personal information, making themselves vulnerable to cyber threats. The rise of social media and apps prompts individuals to reconsider what information they should disclose, highlighting the need for greater awareness regarding online privacy practices.In conclusion, mobile phones represent a double-edged sword in contemporary society. They provide invaluable advantages, such as enhanced communication and connectivity, but also present challenges, including addiction, distraction, and privacy concerns. As we navigate this digital age, it is crucial for individuals to strike a balance, leveraging the benefits of mobile technology while mitigating its drawbacks. Ultimately, self-awareness and responsible usage willdetermine the extent to which mobile phones enhance our lives without compromising our well-being.。
迁移学习在活跃理工科课堂中的尝试——以“C语言程序设计”课程为例
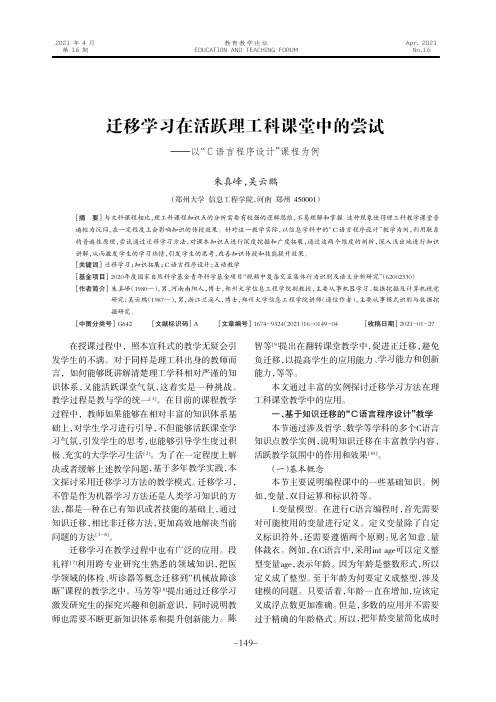
在授课过程中,照本宣科式的教学无疑会引发学生的不满。
对于同样是理工科出身的教师而言,如何能够既讲解清楚理工学科相对严谨的知识体系,又能活跃课堂气氛,这着实是一种挑战。
教学过程是教与学的统一[1]。
在目前的课程教学过程中,教师如果能够在相对丰富的知识体系基础上,对学生学习进行引导,不但能够活跃课堂学习气氛,引发学生的思考,也能够引导学生度过积极、充实的大学学习生活[2]。
为了在一定程度上解决或者缓解上述教学问题,基于多年教学实践,本文探讨采用迁移学习方法的教学模式。
迁移学习,不管是作为机器学习方法还是人类学习知识的方法,都是一种在已有知识或者技能的基础上,通过知识迁移,相比非迁移方法,更加高效地解决当前问题的方法[3-6]。
迁移学习在教学过程中也有广泛的应用。
段礼祥[7]利用跨专业研究生熟悉的领域知识,把医学领域的体检、听诊器等概念迁移到“机械故障诊断”课程的教学之中。
马芳等[8]提出通过迁移学习激发研究生的探究兴趣和创新意识,同时说明教师也需要不断更新知识体系和提升创新能力。
陈智等[9]提出在翻转课堂教学中,促进正迁移,避免负迁移,以提高学生的应用能力、学习能力和创新能力,等等。
本文通过丰富的实例探讨迁移学习方法在理工科课堂教学中的应用。
一、基于知识迁移的“C语言程序设计”教学本节通过涉及哲学、数学等学科的多个C语言知识点教学实例,说明知识迁移在丰富教学内容,活跃教学氛围中的作用和效果[10]。
(一)基本概念本节主要说明编程课中的一些基础知识。
例如,变量,双目运算和标识符等。
1.变量模型。
在进行C语言编程时,首先需要对可能使用的变量进行定义。
定义变量除了自定义标识符外,还需要遵循两个原则:见名知意、量体裁衣。
例如,在C语言中,采用int age可以定义整型变量age,表示年龄。
因为年龄是整数形式,所以定义成了整型。
至于年龄为何要定义成整型,涉及建模的问题。
只要活着,年龄一直在增加,应该定义成浮点数更加准确。
Use of a paper sheet for transferring coloured dec

专利名称:Use of a paper sheet for transferringcoloured decoration to a plastic film发明人:ZAHER, MAXIMILIAN P.,ENGELHARDT,JOACHIM申请号:EP92108424.0申请日:19920519公开号:EP0572686A1公开日:19931208专利内容由知识产权出版社提供专利附图:摘要:The use of a paper for transferring colour to a plastic film (14) to be decorated provides for the following: - the plastic film (14) to be decorated is preheated before it isintroduced into the calender, - the preheated plastic film (14) is guided on a heated transfer roll (10) of the calender, - the paper (16) which is provided with a coloured decoration on one side is guided over the plastic film (14) onto the heated transfer roll (10) of the calender such that its side provided with the coloured decoration faces the plastic film (14), - a buffer film (18) is guided over the paper (16) on the transfer roll (10), - a tensioning cover (20) which presses the plastic film (14), the paper (16) and the buffer film (18) towards the transfer roll (10) is laid externally over the buffer film (18), - the transfer roll (10), the plastic film (14), the paper (16), the buffer film (18) and the tensioning cover (20) are moved through the calendar synchronously with the same angular velocity and - after it has passed through the calendar, the plastic film (14') with coloured decoration is cooled under tension.申请人:GE POLYMERTREND GMBH,POLYTRANSFER GESELLSCHAFT FUER DEKORATIVE OBERFLAECHENTECHNIK POLYMERER WERKSTOFFE MBH 地址:MITTELKAMP 112; D-26125 OLDENBURG,Plasticslaan 1 4612 PX Bergen op Zoom NL,HAAKESTRASSE 9C; D-26125 OLDENBURG,Haakestrasse 9c D-26125 Oldenburg DE国籍:NL,DE代理机构:von Hellfeld, Axel, Dr. Dipl.-Phys.更多信息请下载全文后查看。
Lecture topic 2 Tort 2010

Negligence is concerned with a duty to take reasonable care to avoid a foreseeable risk of injury to another person, their property, or their economic interests
P suffers stomach illness and nervous shock
P as a consumer can recover against D, the manufacturer
Donoghue v Stevenson
House of Lords (3-2 majority) held:
negligence, assault, false imprisonment trespass to land, nuisance, conversion procure breach of contract, passing off
Tort of Negligence –Policy Reasons
Doctor/patient;
auditor/client, lawyer/client
Standard of Care – Breach of Duty
Standard of care is determined by reference to community standards assessed objectively, ie by reference to what a reasonable person in the position of the defendant would have done about a foreseeable risk of injury (Cook v Cook 1986)
- 1、下载文档前请自行甄别文档内容的完整性,平台不提供额外的编辑、内容补充、找答案等附加服务。
- 2、"仅部分预览"的文档,不可在线预览部分如存在完整性等问题,可反馈申请退款(可完整预览的文档不适用该条件!)。
- 3、如文档侵犯您的权益,请联系客服反馈,我们会尽快为您处理(人工客服工作时间:9:00-18:30)。
A Survey on Transfer LearningSinno Jialin Pan and Qiang Yang,Fellow,IEEEAbstract—A major assumption in many machine learning and data mining algorithms is that the training and future data must be in the same feature space and have the same distribution.However,in many real-world applications,this assumption may not hold.For example,we sometimes have a classification task in one domain of interest,but we only have sufficient training data in another domain of interest,where the latter data may be in a different feature space or follow a different data distribution.In such cases,knowledge transfer,if done successfully,would greatly improve the performance of learning by avoiding much expensive data-labeling efforts.In recent years,transfer learning has emerged as a new learning framework to address this problem.This survey focuses on categorizing and reviewing the current progress on transfer learning for classification,regression,and clustering problems.In this survey,we discuss the relationship between transfer learning and other related machine learning techniques such as domain adaptation,multitask learning and sample selection bias,as well as covariate shift.We also explore some potential future issues in transfer learning research.Index Terms—Transfer learning,survey,machine learning,data mining.Ç1I NTRODUCTIOND ATA mining and machine learning technologies havealready achieved significant success in many knowl-edge engineering areas including classification,regression, and clustering(e.g.,[1],[2]).However,many machine learning methods work well only under a common assump-tion:the training and test data are drawn from the same feature space and the same distribution.When the distribu-tion changes,most statistical models need to be rebuilt from scratch using newly collected training data.In many real-world applications,it is expensive or impossible to recollect the needed training data and rebuild the models.It would be nice to reduce the need and effort to recollect the training data.In such cases,knowledge transfer or transfer learning between task domains would be desirable.Many examples in knowledge engineering can be found where transfer learning can truly be beneficial.One example is Web-document classification[3],[4],[5],where our goal is to classify a given Web document into several predefined categories.As an example,in the area of Web-document classification(see,e.g.,[6]),the labeled examples may be the university webpages that are associated with category information obtained through previous manual-labeling efforts.For a classification task on a newly created website where the data features or data distributions may be different,there may be a lack of labeled training data.As a result,we may not be able to directly apply the webpage classifiers learned on the university website to the new website.In such cases,it would be helpful if we could transfer the classification knowledge into the new domain.The need for transfer learning may arise when the data can be easily outdated.In this case,the labeled data obtained in one time period may not follow the same distribution in a later time period.For example,in indoor WiFi localization problems,which aims to detect a user’s current location based on previously collected WiFi data,it is very expensive to calibrate WiFi data for building localization models in a large-scale environment,because a user needs to label a large collection of WiFi signal data at each location.However,the WiFi signal-strength values may be a function of time,device,or other dynamic factors.A model trained in one time period or on one device may cause the performance for location estimation in another time period or on another device to be reduced.To reduce the recalibration effort,we might wish to adapt the localization model trained in one time period(the source domain)for a new time period(the target domain),or to adapt the localization model trained on a mobile device(the source domain)for a new mobile device(the target domain),as done in[7].As a third example,consider the problem of sentiment classification,where our task is to automatically classify the reviews on a product,such as a brand of camera,into positive and negative views.For this classification task,we need to first collect many reviews of the product and annotate them.We would then train a classifier on the reviews with their corresponding labels.Since the distribu-tion of review data among different types of products can be very different,to maintain good classification performance, we need to collect a large amount of labeled data in order to train the review-classification models for each product. However,this data-labeling process can be very expensive to do.To reduce the effort for annotating reviews for various products,we may want to adapt a classification model that is trained on some products to help learn classification models for some other products.In such cases,transfer learning can save a significant amount of labeling effort[8].In this survey paper,we give a comprehensive overview of transfer learning for classification,regression,and cluster-.The authors are with the Department of Computer Science andEngineering,Hong Kong University of Science and Technology,Clearwater Bay,Kowloon,Hong Kong.E-mail:{sinnopan,qyang}@t.hk.Manuscript received13Nov.2008;revised29May2009;accepted13July2009;published online12Oct.2009.Recommended for acceptance by C.Clifton.For information on obtaining reprints of this article,please send e-mail to:tkde@,and reference IEEECS Log Number TKDE-2008-11-0600.Digital Object Identifier no.10.1109/TKDE.2009.191.1041-4347/10/$26.00ß2010IEEE Published by the IEEE Computer Societying developed in machine learning and data mining areas. There has been a large amount of work on transfer learning for reinforcement learning in the machine learning literature (e.g.,[9],[10]).However,in this paper,we only focus on transfer learning for classification,regression,and clustering problems that are related more closely to data mining tasks. By doing the survey,we hope to provide a useful resource for the data mining and machine learning community.The rest of the survey is organized as follows:In the next four sections,we first give a general overview and define some notations we will use later.We,then,briefly survey the history of transfer learning,give a unified definition of transfer learning and categorize transfer learning into threedifferent settings(given in Table2and Fig.2).For each setting,we review different approaches,given in Table3in detail.After that,in Section6,we review some current research on the topic of“negative transfer,”which happens when knowledge transfer has a negative impact on target learning.In Section7,we introduce some successful applications of transfer learning and list some published data sets and software toolkits for transfer learning research. Finally,we conclude the paper with a discussion of future works in Section8.2O VERVIEW2.1A Brief History of Transfer Learning Traditional data mining and machine learning algorithms make predictions on the future data using statistical models that are trained on previously collected labeled or unlabeled training data[11],[12],[13].Semisupervised classification [14],[15],[16],[17]addresses the problem that the labeled data may be too few to build a good classifier,by making use of a large amount of unlabeled data and a small amount of labeled data.Variations of supervised and semisupervised learning for imperfect data sets have been studied;for example,Zhu and Wu[18]have studied how to deal with the noisy class-label problems.Yang et al.considered cost-sensitive learning[19]when additional tests can be made to future samples.Nevertheless,most of them assume that the distributions of the labeled and unlabeled data are the same. Transfer learning,in contrast,allows the domains,tasks,and distributions used in training and testing to be different.In the real world,we observe many examples of transfer learning.For example,we may find that learning to recognize apples might help to recognize pears.Similarly, learning to play the electronic organ may help facilitate learning the piano.The study of Transfer learning is motivated by the fact that people can intelligently apply knowledge learned previously to solve new problems faster or with better solutions.The fundamental motivation for Transfer learning in the field of machine learning was discussed in a NIPS-95workshop on“Learning to Learn,”1which focused on the need for lifelong machine learning methods that retain and reuse previously learned knowledge.Research on transfer learning has attracted more and more attention since1995in different names:learning to learn,life-long learning,knowledge transfer,inductive transfer,multitask learning,knowledge consolidation, context-sensitive learning,knowledge-based inductive bias, metalearning,and incremental/cumulative learning[20]. Among these,a closely related learning technique to transfer learning is the multitask learning framework[21], which tries to learn multiple tasks simultaneously even when they are different.A typical approach for multitask learning is to uncover the common(latent)features that can benefit each individual task.In2005,the Broad Agency Announcement(BAA)05-29 of Defense Advanced Research Projects Agency(DARPA)’s Information Processing Technology Office(IPTO)2gave a new mission of transfer learning:the ability of a system to recognize and apply knowledge and skills learned in previous tasks to novel tasks.In this definition,transfer learning aims to extract the knowledge from one or more source tasks and applies the knowledge to a target task.In contrast to multitask learning,rather than learning all of the source and target tasks simultaneously,transfer learning cares most about the target task.The roles of the source and target tasks are no longer symmetric in transfer learning.Fig.1shows the difference between the learning processes of traditional and transfer learning techniques.As we can see,traditional machine learning techniques try to learn each task from scratch,while transfer learning techniques try to transfer the knowledge from some previous tasks to a target task when the latter has fewer high-quality training data.Today,transfer learning methods appear in several top venues,most notably in data mining(ACM KDD,IEEE ICDM,and PKDD,for example),machine learning(ICML, NIPS,ECML,AAAI,and IJCAI,for example)and applica-tions of machine learning and data mining(ACM SIGIR, WWW,and ACL,for example).3Before we give different categorizations of transfer learning,we first describe the notations used in this paper.2.2Notations and DefinitionsIn this section,we introduce some notations and definitions that are used in this survey.First of all,we give the definitions of a“domain”and a“task,”respectively.In this survey,a domain D consists of two components:a feature space X and a marginal probability distribution PðXÞ, where X¼f x1;...;x n g2X.For example,if our learning taskFig.1.Different learning processes between(a)traditional machine learning and(b)transfer learning.1.http://socrates.acadiau.ca/courses/comp/dsilver/NIPS95_LTL/ transfer.workshop.1995.html.2./ipto/programs/tl/tl.asp.3.We summarize a list of conferences and workshops where transfer learning papers appear in these few years in the following webpage for reference,t.hk/~sinnopan/conferenceTL.htm.is document classification,and each term is taken as a binary feature,then X is the space of all term vectors,x i is the i th term vector corresponding to some documents,and X is a particular learning sample.In general,if two domains are different,then they may have different feature spaces or different marginal probability distributions.Given a specific domain,D¼fX;PðXÞg,a task consists of two components:a label space Y and an objective predictive function fðÁÞ(denoted by T¼fY;fðÁÞg),which is not observed but can be learned from the training data, which consist of pairs f x i;y i g,where x i2X and y i2Y.The function fðÁÞcan be used to predict the corresponding label, fðxÞ,of a new instance x.From a probabilistic viewpoint, fðxÞcan be written as Pðy j xÞ.In our document classification example,Y is the set of all labels,which is True,False for a binary classification task,and y i is“True”or“False.”For simplicity,in this survey,we only consider the case where there is one source domain D S,and one target domain, D T,as this is by far the most popular of the research works in the literature.More specifically,we denote the source domaindata as D S¼fðx S1;y S1Þ;...;ðx Sn S;y Sn SÞg,where x Si2X S isthe data instance and y Si 2Y S is the corresponding classlabel.In our document classification example,D S can be a set of term vectors together with their associated true or false class labels.Similarly,we denote the target-domain data asD T¼fðx T1;y T1Þ;...;ðx Tn T;y Tn TÞg,where the input x Tiis inX T and y Ti2Y T is the corresponding output.In most cases, 0n T(n S.We now give a unified definition of transfer learning. Definition1(Transfer Learning).Given a source domain D S and learning task T S,a target domain D T and learning task T T,transfer learning aims to help improve the learning of the target predictive function f TðÁÞin D T using the knowledge inD S and T S,where D S¼D T,or T S¼T T.In the above definition,a domain is a pair D¼fX;PðXÞg. Thus,the condition D S¼D T implies that either X S¼X T or P SðXÞ¼P TðXÞ.For example,in our document classification example,this means that between a source document set and a target document set,either the term features are different between the two sets(e.g.,they use different languages),or their marginal distributions are different.Similarly,a task is defined as a pair T¼fY;PðY j XÞg. Thus,the condition T S¼T T implies that either Y S¼Y T or PðY S j X SÞ¼PðY T j X TÞ.When the target and source domains are the same,i.e.,D S¼D T,and their learning tasks are the same,i.e.,T S¼T T,the learning problem becomes a traditional machine learning problem.When the domains are different,then either1)the feature spaces between the domains are different,i.e.,X S¼X T,or2)the feature spaces between the domains are the same but the marginal probability distributions between domain data are different;i.e.,PðX SÞ¼PðX TÞ,where X Si2X S and X Ti2X T.As an example,in our document classification example,case1 corresponds to when the two sets of documents are described in different languages,and case2may correspond to when the source domain documents and the target-domain documents focus on different topics.Given specific domains D S and D T,when the learning tasks T S and T T are different,then either1)the label spaces between the domains are different,i.e.,Y S¼Y T,or 2)the conditional probability distributions between the domains are different;i.e.,PðY S j X SÞ¼PðY T j X TÞ,where Y Si2Y S and Y Ti2Y T.In our document classification example,case1corresponds to the situation where source domain has binary document classes,whereas the target domain has10classes to classify the documents to.Case2 corresponds to the situation where the source and target documents are very unbalanced in terms of the user-defined classes.In addition,when there exists some relationship,explicit or implicit,between the feature spaces of the two domains, we say that the source and target domains are related.2.3A Categorization ofTransfer Learning TechniquesIn transfer learning,we have the following three main research issues:1)what to transfer,2)how to transfer,and 3)when to transfer.“What to transfer”asks which part of knowledge can be transferred across domains or tasks.Some knowledge is specific for individual domains or tasks,and some knowl-edge may be common between different domains such that they may help improve performance for the target domain or task.After discovering which knowledge can be transferred, learning algorithms need to be developed to transfer the knowledge,which corresponds to the“how to transfer”issue.“When to transfer”asks in which situations,transferring skills should be done.Likewise,we are interested in knowing in which situations,knowledge should not be transferred.In some situations,when the source domain and target domain are not related to each other,brute-force transfer may be unsuccessful.In the worst case,it may even hurt the performance of learning in the target domain,a situation which is often referred to as negative transfer.Most current work on transfer learning focuses on “What to transfer”and“How to transfer,”by implicitly assuming that the source and target domains be related to each other.However,how to avoid negative transfer is an important open issue that is attracting more and more attention in the future.Based on the definition of transfer learning,we summarize the relationship between traditional machine learning and various transfer learning settings in Table1,where wePAN AND YANG:A SURVEY ON TRANSFER LEARNING1347TABLE1Relationshipbetween Traditional Machine Learning and Various Transfer Learning Settingscategorize transfer learning under three subsettings,inductive transfer learning,transductive transfer learning,and unsuper-vised transfer learning,based on different situations between the source and target domains and tasks.1.In the inductive transfer learning setting,the target taskis different from the source task,no matter when thesource and target domains are the same or not.In this case,some labeled data in the target domain are required to induce an objective predictivemodel f TðÁÞfor use in the target domain.In addition,according to different situations of labeled andunlabeled data in the source domain,we can furthercategorize the inductive transfer learning setting intotwo cases:a.A lot of labeled data in the source domain areavailable.In this case,the inductive transferlearning setting is similar to the multitask learningsetting.However,the inductive transfer learningsetting only aims at achieving high performancein the target task by transferring knowledge fromthe source task while multitask learning tries tolearn the target and source task simultaneously.b.No labeled data in the source domain areavailable.In this case,the inductive transferlearning setting is similar to the self-taughtlearning setting,which is first proposed by Rainaet al.[22].In the self-taught learning setting,thelabel spaces between the source and targetdomains may be different,which implies theside information of the source domain cannot beused directly.Thus,it’s similar to the inductivetransfer learning setting where the labeled datain the source domain are unavailable.2.In the transductive transfer learning setting,the sourceand target tasks are the same,while the source andtarget domains are different.In this situation,no labeled data in the target domain are available while a lot of labeled data inthe source domain are available.In addition,according to different situations between the sourceand target domains,we can further categorize thetransductive transfer learning setting into two cases.a.The feature spaces between the source andtarget domains are different,X S¼X T.b.The feature spaces between domains are thesame,X S¼X T,but the marginal probabilitydistributions of the input data are different,PðX SÞ¼PðX TÞ.The latter case of the transductive transfer learning setting is related to domain adaptationfor knowledge transfer in text classification[23]and sample selection bias[24]or covariate shift[25],whose assumptions are similar.3.Finally,in the unsupervised transfer learning setting,similar to inductive transfer learning setting,the targettask is different from but related to the source task.However,the unsupervised transfer learning focus onsolving unsupervised learning tasks in the targetdomain,such as clustering,dimensionality reduction,and density estimation[26],[27].In this case,there areno labeled data available in both source and targetdomains in training.The relationship between the different settings of transfer learning and the related areas are summarized in Table2and Fig.2.Approaches to transfer learning in the above three different settings can be summarized into four cases based on“What to transfer.”Table3shows these four cases and brief description.The first context can be referred to as instance-based transfer learning(or instance transfer) approach[6],[28],[29],[30],[31],[24],[32],[33],[34],[35], which assumes that certain parts of the data in the source domain can be reused for learning in the target domain by reweighting.Instance reweighting and importance sampling are two major techniques in this context.A second case can be referred to as feature-representa-tion-transfer approach[22],[36],[37],[38],[39],[8],[40], [41],[42],[43],[44].The intuitive idea behind this case is to learn a“good”feature representation for the target domain. In this case,the knowledge used to transfer across domains is encoded into the learned feature representation.With the new feature representation,the performance of the target task is expected to improve significantly.A third case can be referred to as parameter-transfer approach[45],[46],[47],[48],[49],which assumes that the source tasks and the target tasks share some parameters or prior distributions of the hyperparameters of the models.The transferred knowledge is encoded into the shared para-meters or priors.Thus,by discovering the shared parameters or priors,knowledge can be transferred across tasks.Finally,the last case can be referred to as the relational-knowledge-transfer problem[50],which deals with transfer learning for relational domains.The basic assumption1348IEEE TRANSACTIONS ON KNOWLEDGE AND DATA ENGINEERING,VOL.22,NO.10,OCTOBER2010TABLE2Different Settings of TransferLearningbehind this context is that some relationship among the data in the source and target domains is similar.Thus,the knowledge to be transferred is the relationship among the data.Recently,statistical relational learning techniques dominate this context [51],[52].Table 4shows the cases where the different approaches are used for each transfer learning setting.We can see that the inductive transfer learning setting has been studied in many research works,while the unsupervised transfer learning setting is a relatively new research topic and only studied in the context of the feature-representation-transfer case.In addition,the feature-representation-transfer problem has been proposed to all three settings of transfer learning.However,the parameter-transfer and the relational-knowledge-transfer approach are only studied in the inductive transfer learning setting,which we discuss in detail below.3I NDUCTIVE T RANSFER L EARNINGDefinition 2(Inductive Transfer Learning).Given a source domain D S and a learning task T S ,a target domain D T and a learning task T T ,inductive transfer learning aims to help improve the learning of the target predictive functionf T ðÁÞin D T using the knowledge in D S and T S ,where T S ¼T T .Based on the above definition of the inductive transfer learning setting,a few labeled data in the target domain are required as the training data to induce the target predictive function.As mentioned in Section 2.3,this setting has two cases:1)labeled data in the source domain are available and 2)labeled data in the source domain are unavailable while unlabeled data in the source domain are available.Most transfer learning approaches in this setting focus on the former case.3.1Transferring Knowledge of InstancesThe instance-transfer approach to the inductive transfer learning setting is intuitively appealing:although the source domain data cannot be reused directly,there are certain parts of the data that can still be reused together with a few labeled data in the target domain.Dai et al.[6]proposed a boosting algorithm,TrAdaBoost ,which is an extension of the AdaBoost algorithm,to address the inductive transfer learning problems.TrAdaBoost assumes that the source and target-domain data use exactly the samePAN AND YANG:A SURVEY ON TRANSFERLEARNING 1349TABLE 3Different Approaches to Transfer LearningFig.2.An overview of different settings of transfer.set of features and labels,but the distributions of the data in the two domains are different.In addition,TrAdaBoost assumes that,due to the difference in distributions between the source and the target domains,some of the source domain data may be useful in learning for the target domain but some of them may not and could even be harmful.It attempts to iteratively reweight the source domain data to reduce the effect of the“bad”source data while encourage the“good”source data to contribute more for the target domain.For each round of iteration, TrAdaBoost trains the base classifier on the weighted source and target data.The error is only calculated on the target data.Furthermore,TrAdaBoost uses the same strategy as AdaBoost to update the incorrectly classified examples in the target domain while using a different strategy from AdaBoost to update the incorrectly classified source exam-ples in the source domain.Theoretical analysis of TrAda-Boost in also given in[6].Jiang and Zhai[30]proposed a heuristic method to remove“misleading”training examples from the source domain based on the difference between conditional probabilities Pðy T j x TÞand Pðy S j x SÞ.Liao et al.[31] proposed a new active learning method to select the unlabeled data in a target domain to be labeled with the help of the source domain data.Wu and Dietterich[53] integrated the source domain(auxiliary)data an Support Vector Machine(SVM)framework for improving the classification performance.3.2Transferring Knowledge of FeatureRepresentationsThe feature-representation-transfer approach to the induc-tive transfer learning problem aims at finding“good”feature representations to minimize domain divergence and classi-fication or regression model error.Strategies to find“good”feature representations are different for different types of the source domain data.If a lot of labeled data in the source domain are available,supervised learning methods can be used to construct a feature representation.This is similar to common feature learning in the field of multitask learning [40].If no labeled data in the source domain are available, unsupervised learning methods are proposed to construct the feature representation.3.2.1Supervised Feature ConstructionSupervised feature construction methods for the inductive transfer learning setting are similar to those used in multitask learning.The basic idea is to learn a low-dimensional representation that is shared across related tasks.In addition,the learned new representation can reduce the classification or regression model error of each task as well. Argyriou et al.[40]proposed a sparse feature learning method for multitask learning.In the inductive transfer learning setting,the common features can be learned by solving an optimization problem,given as follows:arg minA;UXt2f T;S gX n ti¼1Lðy ti;h a t;U T x tiiÞþ k A k22;1s:t:U2O d:ð1ÞIn this equation,S and T denote the tasks in the source domain and target domain,respectively.A¼½a S;a T 2R dÂ2 is a matrix of parameters.U is a dÂd orthogonal matrix (mapping function)for mapping the original high-dimen-sional data to low-dimensional representations.Theðr;pÞ-norm of A is defined as k A k r;p:¼ðP di¼1k a i k p rÞ1p.The optimization problem(1)estimates the low-dimensional representations U T X T,U T X S and the parameters,A,of the model at the same time.The optimization problem(1)can be further transformed into an equivalent convex optimiza-tion formulation and be solved efficiently.In a follow-up work,Argyriou et al.[41]proposed a spectral regularization framework on matrices for multitask structure learning.Lee et al.[42]proposed a convex optimization algorithm for simultaneously learning metapriors and feature weights from an ensemble of related prediction tasks.The meta-priors can be transferred among different tasks.Jebara[43] proposed to select features for multitask learning with SVMs.Ru¨ckert and Kramer[54]designed a kernel-based approach to inductive transfer,which aims at finding a suitable kernel for the target data.3.2.2Unsupervised Feature ConstructionIn[22],Raina et al.proposed to apply sparse coding[55], which is an unsupervised feature construction method,for learning higher level features for transfer learning.The basic idea of this approach consists of two steps.In the first step, higher level basis vectors b¼f b1;b2;...;b s g are learned on the source domain data by solving the optimization problem(2)as shown as follows:mina;bXix SiÀXja jS ib j22þa Si1s:t:k b j k21;8j21;...;s:ð2ÞIn this equation,a j Siis a new representation of basis b j for input x Siand is a coefficient to balance the feature construction term and the regularization term.After learning the basis vectors b,in the second step,an optimization algorithm(3)is applied on the target-domain data to learn higher level features based on the basis vectors b.aÃT i¼arg mina Tix TiÀXja jT ib j22þa Ti1:ð3Þ1350IEEE TRANSACTIONS ON KNOWLEDGE AND DATA ENGINEERING,VOL.22,NO.10,OCTOBER2010TABLE4Different Approaches Used in DifferentSettings。