Statistical method of recognizing local cohesion in spoken dialogues
Apparatus and method for measuring of absolute val
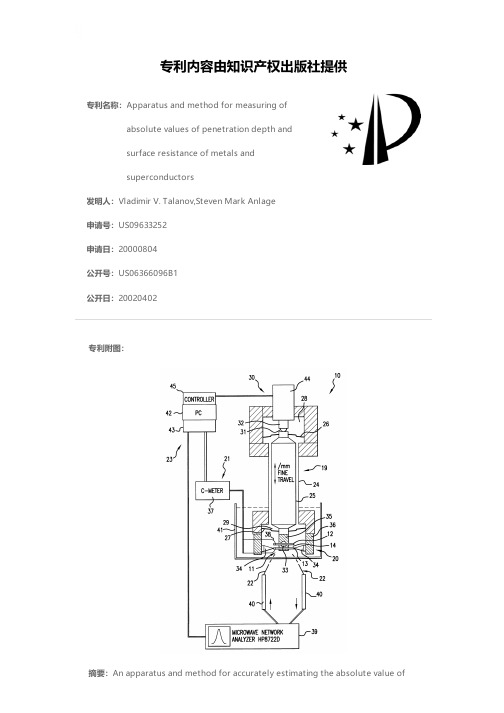
专利名称:Apparatus and method for measuring ofabsolute values of penetration depth andsurface resistance of metals andsuperconductors发明人:Vladimir V. Talanov,Steven Mark Anlage申请号:US09633252申请日:20000804公开号:US06366096B1公开日:20020402专利内容由知识产权出版社提供专利附图:摘要:An apparatus and method for accurately estimating the absolute value ofsurface resistances and penetration depths of metallic films and bulk samples. The apparatus carries out measurements using two nominally identical samples with flat sample surfaces which are brought together with a thin dielectric separation of variable thickness sandwiched between the samples in order to form a two-conductor parallel plate transmission line resonator which carries an electromagnetic wave. A liquid or gas of unknown dielectric properties fills the dielectric spacer. A resonant condition of the microwave signal is established and the resonant frequency and the quality factor Q are measured while the spacing between the sample plates is varied. The variation of the resonant frequency and Q with spacer thickness is then analyzed to yield absolute values of the sample surface resistance and penetration depth which are then further used for determination of absolute complex conductivity and surface impedance of the samples.申请人:UNIVERSITY OF MARYLAND, COLLEGE PARK代理机构:Rosenberg, Klein & Lee更多信息请下载全文后查看。
统计模式识别方法
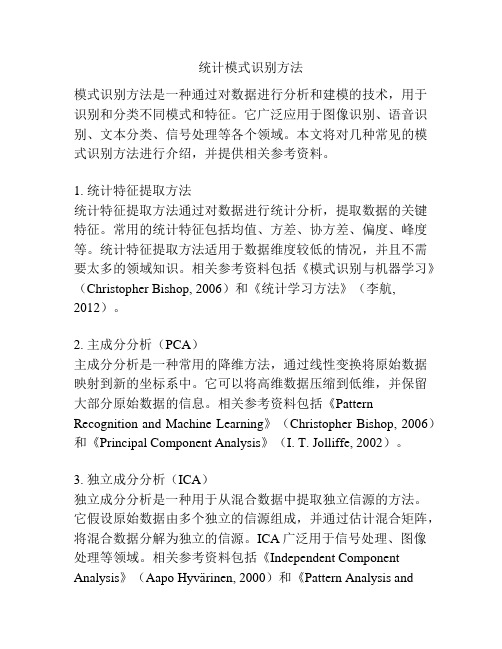
统计模式识别方法模式识别方法是一种通过对数据进行分析和建模的技术,用于识别和分类不同模式和特征。
它广泛应用于图像识别、语音识别、文本分类、信号处理等各个领域。
本文将对几种常见的模式识别方法进行介绍,并提供相关参考资料。
1. 统计特征提取方法统计特征提取方法通过对数据进行统计分析,提取数据的关键特征。
常用的统计特征包括均值、方差、协方差、偏度、峰度等。
统计特征提取方法适用于数据维度较低的情况,并且不需要太多的领域知识。
相关参考资料包括《模式识别与机器学习》(Christopher Bishop, 2006)和《统计学习方法》(李航, 2012)。
2. 主成分分析(PCA)主成分分析是一种常用的降维方法,通过线性变换将原始数据映射到新的坐标系中。
它可以将高维数据压缩到低维,并保留大部分原始数据的信息。
相关参考资料包括《Pattern Recognition and Machine Learning》(Christopher Bishop, 2006)和《Principal Component Analysis》(I. T. Jolliffe, 2002)。
3. 独立成分分析(ICA)独立成分分析是一种用于从混合数据中提取独立信源的方法。
它假设原始数据由多个独立的信源组成,并通过估计混合矩阵,将混合数据分解为独立的信源。
ICA广泛用于信号处理、图像处理等领域。
相关参考资料包括《Independent Component Analysis》(Aapo Hyvärinen, 2000)和《Pattern Analysis andMachine Intelligence》(Simon Haykin, 1999)。
4. 支持向量机(SVM)支持向量机是一种二分类和多分类的模式识别方法。
它通过找到一个最优的超平面,将样本分成不同的类别。
SVM可以灵活地处理线性可分和线性不可分的问题,并具有很好的泛化能力。
蒙特卡罗方法概述7
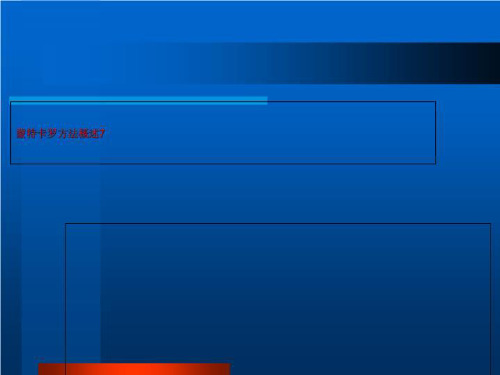
表示击中r处相应的得分数(环数),f(r)为该运动员的 弹着点的分布密度函数,它反映运动员的射击水平。 该运动员的射击成绩为
g0 g(r)f(r)dr
用概率语言来说,<g>是随机变量g(r)的数学期
望,即
gE g(r)
现假设该运动员进行了N次射击,每次射击的弹 着g(r点2),依…次,为g(rrN1),的算r2 术,平…均,值rN , 则 N 次 得 分 g(r1) ,
➢ 计算机模拟试验过程
计算机模拟试验过程,就是将试验过程(如投针, 射击)化为数学问题,在计算机上实现。以上述两个 问题为例,分别加以说明。
例1. 蒲丰氏问题 例2. 射击问题(打靶游戏)
由上面两个例题看出,蒙特卡罗方法常以一个 “概率模型”为基础,按照它所描述的过程,使用由 已知分布抽样的方法,得到部分试验结果的观察值, 求得问题的近似解。
一些人进行了实验,其结果列于下表 :
实验者
年份 投计次数 π的实验值
沃尔弗(Wolf) 1850 5000
3.1596
斯密思(Smith) 1855 3204
3.1553
福克斯(Fox)
1894 1120
3.1419
拉查里尼 (Lazzarini)
1901 3408
3.1415929
例2. 射击问题(打靶游戏)
1 N
gN N i1 g(ri )
作为积分的估计值(近似值)。
为了得到具有一定精确度的近似解,所需试验的
次数是很多的,通过人工方法作大量的试验相当困难, 甚至是不可能的。因此,蒙特卡罗方法的基本思想虽 然早已被人们提出,却很少被使用。本世纪四十年代 以来,由于电子计算机的出现,使得人们可以通过电 子计算机来模拟随机试验过程,把巨大数目的随机试 验交由计算机完成,使得蒙特卡罗方法得以广泛地应 用,在现代化的科学技术中发挥应有的作用。
评估算法的重要指标

评估算法的重要指标评估算法的重要指标在机器学习和数据挖掘领域中,评估算法的性能是非常关键的。
以下是一些重要指标,可用于评估算法的性能。
1. 精度(Accuracy):精度是指分类器正确分类的样本数占总样本数的比例。
它是最常用的分类器性能指标。
2. 灵敏度(Sensitivity):灵敏度也称为真阳性率,它是指真实正例中分类器正确分类的比例。
灵敏度越高,表示分类器对正例的识别能力越强。
3. 特异度(Specificity):特异度也称为真阴性率,它是指真实负例中分类器正确分类的比例。
特异度越高,表示分类器对负例的识别能力越强。
4. 精确率(Precision):精确率是指被分类为正例中实际为正例的比例。
精确率越高,表示分类器对正例判定正确性越高。
5. 召回率(Recall):召回率也称为查全率或灵敏度,它是指实际为正例中被正确识别为正例的比例。
召回率越高,表示分类器对正例判定漏报率越低。
6. F1值(F1 Score):F1值是精确率和召回率的加权平均值,它综合了精确率和召回率的优缺点。
F1值越高,表示分类器的性能越好。
7. ROC曲线(Receiver Operating Characteristic Curve):ROC 曲线是真阳性率与假阳性率之间的关系曲线。
ROC曲线下面积越大,表示分类器的性能越好。
8. AUC(Area Under Curve):AUC是ROC曲线下面积,它是评估分类器性能的重要指标。
AUC越大,表示分类器的性能越好。
以上是评估算法常用的一些指标,不同任务需要选择不同的指标进行评估。
除了这些指标外,还有其他一些指标可以用于评估算法性能,如错误率、Kappa系数等。
机器学习中的迁移学习算法评估指标
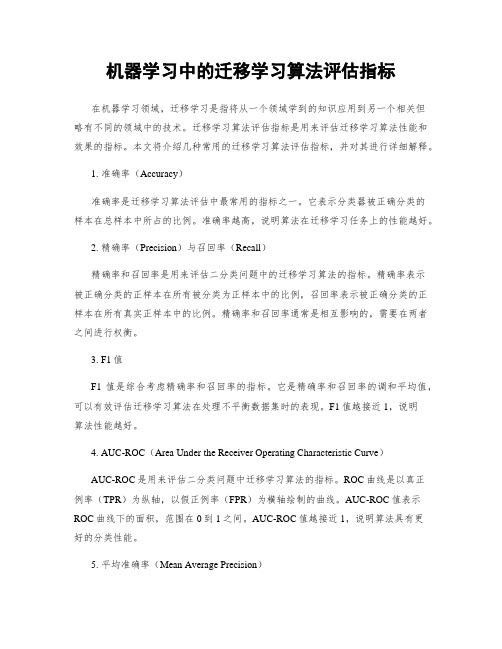
机器学习中的迁移学习算法评估指标在机器学习领域,迁移学习是指将从一个领域学到的知识应用到另一个相关但略有不同的领域中的技术。
迁移学习算法评估指标是用来评估迁移学习算法性能和效果的指标。
本文将介绍几种常用的迁移学习算法评估指标,并对其进行详细解释。
1. 准确率(Accuracy)准确率是迁移学习算法评估中最常用的指标之一。
它表示分类器被正确分类的样本在总样本中所占的比例。
准确率越高,说明算法在迁移学习任务上的性能越好。
2. 精确率(Precision)与召回率(Recall)精确率和召回率是用来评估二分类问题中的迁移学习算法的指标。
精确率表示被正确分类的正样本在所有被分类为正样本中的比例,召回率表示被正确分类的正样本在所有真实正样本中的比例。
精确率和召回率通常是相互影响的,需要在两者之间进行权衡。
3. F1值F1值是综合考虑精确率和召回率的指标。
它是精确率和召回率的调和平均值,可以有效评估迁移学习算法在处理不平衡数据集时的表现。
F1值越接近1,说明算法性能越好。
4. AUC-ROC(Area Under the Receiver Operating Characteristic Curve)AUC-ROC是用来评估二分类问题中迁移学习算法的指标。
ROC曲线是以真正例率(TPR)为纵轴,以假正例率(FPR)为横轴绘制的曲线。
AUC-ROC值表示ROC曲线下的面积,范围在0到1之间。
AUC-ROC值越接近1,说明算法具有更好的分类性能。
5. 平均准确率(Mean Average Precision)平均准确率是用来评估迁移学习算法在多类别问题中的指标。
它综合了每个类别的准确率,并计算出一个平均值。
平均准确率越高,说明算法对多个类别的分类性能越好。
6. 均方误差(Mean Squared Error)均方误差是用来评估回归问题中的迁移学习算法的指标。
它表示预测值与真实值之间的差异程度。
均方误差越小,说明算法对实际值的预测越准确。
靶标识别 相机姿态估计算法
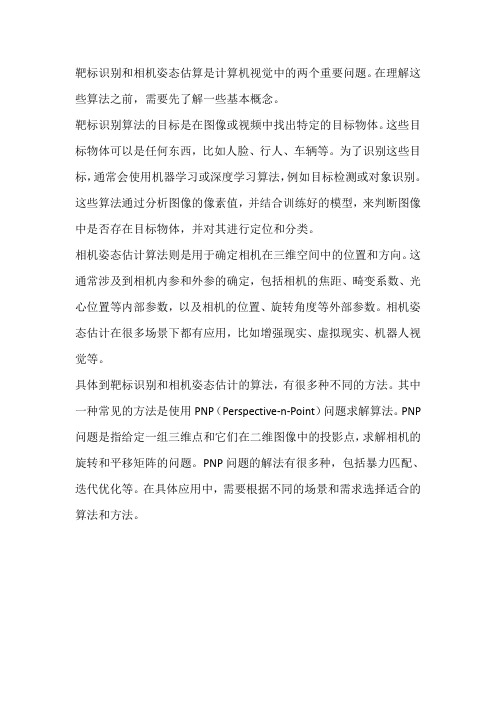
靶标识别和相机姿态估算是计算机视觉中的两个重要问题。
在理解这些算法之前,需要先了解一些基本概念。
靶标识别算法的目标是在图像或视频中找出特定的目标物体。
这些目标物体可以是任何东西,比如人脸、行人、车辆等。
为了识别这些目标,通常会使用机器学习或深度学习算法,例如目标检测或对象识别。
这些算法通过分析图像的像素值,并结合训练好的模型,来判断图像中是否存在目标物体,并对其进行定位和分类。
相机姿态估计算法则是用于确定相机在三维空间中的位置和方向。
这通常涉及到相机内参和外参的确定,包括相机的焦距、畸变系数、光心位置等内部参数,以及相机的位置、旋转角度等外部参数。
相机姿态估计在很多场景下都有应用,比如增强现实、虚拟现实、机器人视觉等。
具体到靶标识别和相机姿态估计的算法,有很多种不同的方法。
其中一种常见的方法是使用PNP(Perspective-n-Point)问题求解算法。
PNP 问题是指给定一组三维点和它们在二维图像中的投影点,求解相机的旋转和平移矩阵的问题。
PNP问题的解法有很多种,包括暴力匹配、迭代优化等。
在具体应用中,需要根据不同的场景和需求选择适合的算法和方法。
描述统计学与推断统计学名词解释

描述统计学与推断统计学名词解释描述统计学(Descriptive Statistics)是统计学的一个分支,主要研究如何通过数据收集、处理、分析和解释,来描述和总结所观察到的现象的基本统计信息。
它包括统计数据的收集方法、数据的加工处理方法、数据的显示方法、数据分布特征的概括与分析方法等。
描述统计学通过数理统计方法来反映数据的特点,并通过图表形式对所收集的数据进行必要的可视化,进一步综合、概括和分析得出数据的客观规律。
推断统计学(Inferential Statistics)也是统计学的一个分支,主要研究如何根据样本数据去推断总体数量特征的方法。
它是在对样本数据进行描述的基础上,对统计总体的未知数量特征做出以概率形式表述的推断。
推断统计学通常用于对总体参数的估计和假设检验,其结果通常是为了得到下一步的行动策略。
描述统计学和推断统计学是统计学的两个重要分支,二者相辅相成。
描述统计学是推断统计学的基础,而推断统计学则是描述统计学的进一步发展。
在实际应用中,需要根据具体的研究目的和数据情况来选择合适的统计方法。
机器学习算法评估准确度分析方法整理
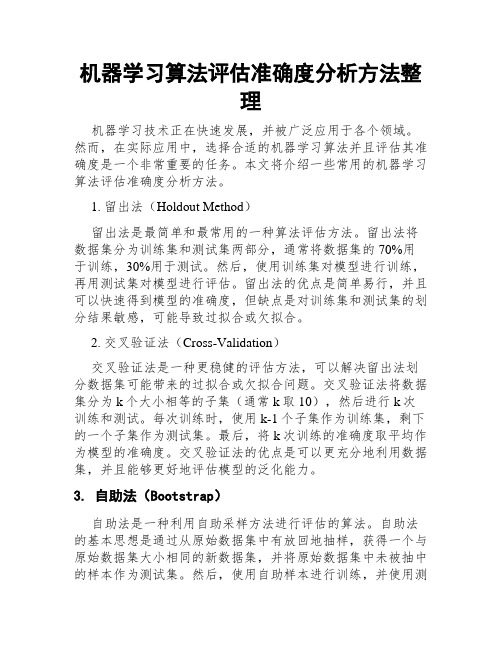
机器学习算法评估准确度分析方法整理机器学习技术正在快速发展,并被广泛应用于各个领域。
然而,在实际应用中,选择合适的机器学习算法并且评估其准确度是一个非常重要的任务。
本文将介绍一些常用的机器学习算法评估准确度分析方法。
1. 留出法(Holdout Method)留出法是最简单和最常用的一种算法评估方法。
留出法将数据集分为训练集和测试集两部分,通常将数据集的70%用于训练,30%用于测试。
然后,使用训练集对模型进行训练,再用测试集对模型进行评估。
留出法的优点是简单易行,并且可以快速得到模型的准确度,但缺点是对训练集和测试集的划分结果敏感,可能导致过拟合或欠拟合。
2. 交叉验证法(Cross-Validation)交叉验证法是一种更稳健的评估方法,可以解决留出法划分数据集可能带来的过拟合或欠拟合问题。
交叉验证法将数据集分为k个大小相等的子集(通常k取10),然后进行k次训练和测试。
每次训练时,使用k-1个子集作为训练集,剩下的一个子集作为测试集。
最后,将k次训练的准确度取平均作为模型的准确度。
交叉验证法的优点是可以更充分地利用数据集,并且能够更好地评估模型的泛化能力。
3. 自助法(Bootstrap)自助法是一种利用自助采样方法进行评估的算法。
自助法的基本思想是通过从原始数据集中有放回地抽样,获得一个与原始数据集大小相同的新数据集,并将原始数据集中未被抽中的样本作为测试集。
然后,使用自助样本进行训练,并使用测试集评估模型。
自助法的优点是可以使用较小的数据集进行训练,并且不需要额外的测试集,但缺点是自助样本可能会包含重复的样本,导致评估结果不准确。
4. ROC曲线(Receiver Operating Characteristic Curve)ROC曲线是一种绘制真正例率(True Positive Rate)和假正例率(False Positive Rate)之间关系的方法。
在机器学习任务中,例如二分类问题,常常需要根据模型的输出进行分类决策,而不仅仅是输出概率。
- 1、下载文档前请自行甄别文档内容的完整性,平台不提供额外的编辑、内容补充、找答案等附加服务。
- 2、"仅部分预览"的文档,不可在线预览部分如存在完整性等问题,可反馈申请退款(可完整预览的文档不适用该条件!)。
- 3、如文档侵犯您的权益,请联系客服反馈,我们会尽快为您处理(人工客服工作时间:9:00-18:30)。
Naoto Katoh and Tsuyoshi Morimoto ATR Interpreting Telecommunications Research Laboratories Seika-cho Soraku-gun Kyoto 619-02 Japan {katonao, morimoto }@ itl.atr.co.jp
Figure 1. An e x a m p l e o f a task-oriented dialogue in Japanese
2 Local Cohesion between Utterances
The discourse structure in task-oriented dialogues has two types of cohesion: global cohesion and local cohesion. Global cohesion is a top-down structured context and is based on a hierarchy of topics led by domain (e.g., hotel reservation or flight cancellation). Using this cohesion, a taskoriented dialogue is segmented into several subdialogues according to the topic. On the other hand, local cohesion is a bottom-up structured context and a coherence relation between utterances, such as question-response or response-confirmation. Different from global cohesion, local cohesion does not have a hierarchy. This paper focuses on local cohesion. Figure 1 shows a Japanese conversation between a person and a hotel staff member, which is an example of a task-oriented dialogue; The person is making a hotel reservation. The first column represents global cohesion and the second column represents local cohesion. For example, the pair of U3 and U4 has local cohesion, because it has a coherence relation for each word in the utterances as follows: c 1) speech act pattern between "onegaiitashimasu (requirement)"in U3 and "desu (response)" in U4
1 Introduction
For ambiguity resolution, processing of a discourse structure is one of the important processes in Natural Language Processing (NLP). Indeed, discourse structures play a useful role in speech recognition, which is an application of NLP. In the case of Japanese, it is very difficult to recognize the end in utterances by using current speech recognition techniques because the sound power of an ending tends to be small. For example, "desu", which represents the speech act type "response", is often misrecognized as "desu-ka (question)" or "desu-ne (confirmation)". On the other hand, Japanese can easily select the adequate expression "desu", when the intention of the previous utterance is concerned with a question. This is because they use the coherence relation (local cohesion)
634Βιβλιοθήκη Local Global cohesion cohesion
I
--Topics
(1) hotel reservation (2) date (3) the number of persons
i u--q (1) I--U1 :Heya wo yoyaku shitai-no-desu-ga L (l would like to make a room reservation.) U2:Kashikomari-mashita (OK.) - U 3 : G o - k i b o u no hinichi wo onegai-itashimasu (Could you tell me when you would like to stay?) U4:Hachi-gatsu to-ka kara ju-ni-nichi desu (From August 10th to 12th.) U5 :Hachi-gatsu to-ka kara ju-niqfichi made ni-haku desu-ne (That's two nights from August 10th to 12th, is that right?) --U6:Hai, sou-desu (Yes, that's correct.) l(3"1V-U7:Nan-mei-sama de shou-ka ~zz_j (How many persons will be staying?) --t t--U8:Futari desu / (Two persons.)
Abstract
This paper presents a method for automatically recognizing local cohesion between utterances, which is one of the discourse structures in task-oriented spoken dialogues. More specifically we can automatically acquire discourse knowledge from an annotated corpus with local cohesion. In this paper we focus on speech act type-based local cohesion. The presented method consists of two steps 1) identifying the speech act expressions in an utterance and 2) calculating the plausibility of local cohesion between the speech act expressions by using the dialogue corpus annotated with local cohesion. We present two methods of interpolating the plausibility of local cohesion based on surface information on utterances. The presented method has obtained a 93% accuracy for closed data and a 78% accuracy for open data in recognizing a pair of utterances with local cohesion.
between the two utterances, question-response. In the conventional approach (i.e., rulebased approach) to processing the discourse structure [Hauptmann 88][Kudo 9 0 ] [ Y a m a o k a 91][Young 91], NLP engineers built discourse knowledge by hand-coding. However, the rulebased approach has a bottleneck in that it is a hard job to add discourse knowledge when the employed NLP system deals with a larger domain and more vocabulary. Recently, statistical approaches have been attracting attention for their ability to acquire linguistic knowledge from a corpus. Compared with the above rule-based approach, a statistical approach is easy to apply to larger domains since the linguistic knowledge can be automatically extracted from the corpora concerned with the domain. However, little research has been reported in discourse processing [Nagata 94][Reithinger 95], while in the areas of morphological analysis and syntactic analysis, many research studies have been proposed in recent years. This paper presents a method for automatically recognizing local cohesion between utterances, which is one of the discourse structures in task-oriented spoken dialogues. We can automatically acquire discourse knowledge from an annotated corpus with local cohesion. In this paper we focus on speech act type-based local cohesion. The presented method consists of two steps ~1) identifying the speech act expressions in an utterance and 2) calculating the plausibility of local cohesion between the speech act expressions by using the dialogue corpus annotated with local cohesion. We present two methods of interpolating the plausibility of local cohesion based on surface information on utterances. Our method has obtained a 93% accuracy for closed data and a 78% accuracy for open data in recognizing a pair of utterances with local cohesion. In Section 2, local cohesion in task-oriented dialogues is described. In Section 3, our statistical method is presented. In Section 4, the results of a series of experiments using our method are described.