RANGE-EFFICIENT COUNTING OF DISTINCT ELEMENTS IN A MASSIVE DATA STREAM
用数字介绍自己的物品数量英语作文
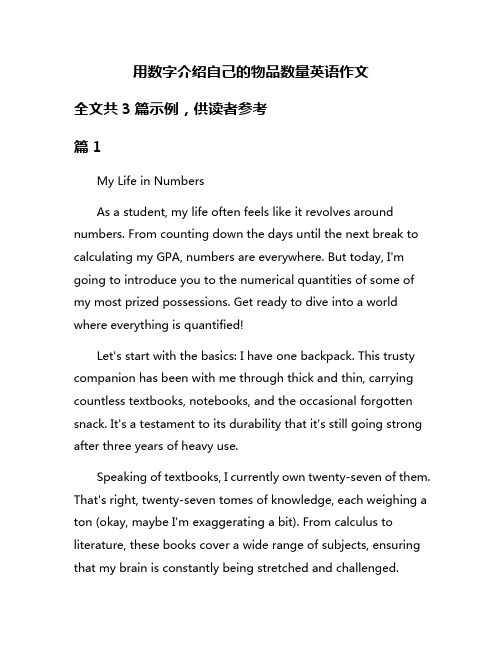
用数字介绍自己的物品数量英语作文全文共3篇示例,供读者参考篇1My Life in NumbersAs a student, my life often feels like it revolves around numbers. From counting down the days until the next break to calculating my GPA, numbers are everywhere. But today, I'm going to introduce you to the numerical quantities of some of my most prized possessions. Get ready to dive into a world where everything is quantified!Let's start with the basics: I have one backpack. This trusty companion has been with me through thick and thin, carrying countless textbooks, notebooks, and the occasional forgotten snack. It's a testament to its durability that it's still going strong after three years of heavy use.Speaking of textbooks, I currently own twenty-seven of them. That's right, twenty-seven tomes of knowledge, each weighing a ton (okay, maybe I'm exaggerating a bit). From calculus to literature, these books cover a wide range of subjects, ensuring that my brain is constantly being stretched and challenged.Now, let's talk about something a little more fun: my collection of video games. As of this moment, I have thirty-two games spanning various consoles and genres. Fromheart-pounding first-person shooters to immersive role-playing adventures, my gaming library caters to every mood and whim. It's a good thing I have two controllers, or my friends and I would be constantly fighting over who gets to play next.But what's a gamer without a reliable computer? My trusty laptop has been my constant companion for four years now. It's seen me through countless all-nighters, fueled by an unhealthy amount of coffee (but more on that later). With its high-speed processor and ample storage space, it's the perfect tool for tackling assignments, streaming movies, and, of course, playing the occasional game or two.Speaking of caffeine, let's talk about my coffee mug collection. I'll admit, it's a bit excessive, but when you have fifteen different mugs, each with its own unique design or quirky slogan, it's hard to resist adding to the collection. From the classic "World's Best Student" mug to the one that says "I'll sleep when I'm dead," these mugs are more than just vessels for my daily dose of caffeine – they're a reflection of my personality.But what's a student without a trusty pen or pencil? I have forty-two pens and twenty-six pencils scattered throughout my room, backpack, and desk drawers. You never know when inspiration (or a pop quiz) might strike, so it's always best to be prepared. Plus, there's something deeply satisfying about the way a brand-new pen glides across the page, leaving a trail of crisp, legible words in its wake.Of course, no student's life would be complete without a healthy dose of stress relief. That's where my collection offifty-three stress balls comes in. From the classic foam variety to the more unconventional ones shaped like animals or food items, these little squishable wonders have been my saviors during many a tense study session or exam.Speaking of exams, let's not forget about the countless sheets of paper I've gone through over the years. I'd estimate that I've used approximately three thousand pages of lined notebook paper and two thousand sheets of printer paper for assignments, notes, and rough drafts. It's a good thing trees are a renewable resource, or I might feel a little guilty about my paper consumption.But what's a student without a little fun and relaxation? That's where my collection of one hundred and twelve moviescomes in. From classic comedies to thought-provoking dramas, my movie library is a veritable cinematic buffet, ready to whisk me away from the stresses of academic life whenever I need a break.And let's not forget about my music collection. With over two thousand songs spanning genres from rock to hip-hop to classical, my playlists are as diverse as they are extensive. Whether I'm studying, working out, or just in need of a sonic escape, my tunes are always there to keep me company.Of course, no student's life would be complete without a little bit of fashion. I have twenty-eight shirts, sixteen pairs of jeans, and thirty-seven pairs of socks (because you can never have too many socks). From the classic t-shirt and jeans combo to the occasional dressier outfit for presentations or formal events, my wardrobe is a reflection of my ever-changing moods and styles.And let's not forget about the little things that make life a little more comfortable. I have six pillows on my bed (because you can never have too many pillows), ten blankets (for those chilly study sessions), and four different types of snacks stashed away for late-night cravings.As you can see, my life as a student is a numerical wonderland, filled with countless items and possessions, each with its own unique quantity and purpose. From the practical to the whimsical, these numbers represent not just the things I own, but the experiences, memories, and chapters of my life.So, the next time you see a student hunched over a textbook or furiously typing away at a laptop, remember: behind those seemingly mundane actions lies a world of numbers, each one telling a story, quantifying a life lived to the fullest.篇2My Life by the NumbersHi there! I'm an average college student trying to make my way through the chaos of classes, activities, socializing, and everything else that comes with university life. Instead of just telling you about myself though, I thought I'd give you a fun little numerical introduction with the numbers representing the quantities of different items and aspects of my life. Here goes!0 - The number of funky colored socks I own since somehow all my socks seem to be plain white or black these days. I really need to jazz up my sock game.1 - The single, oversized college branded hoodie that has become my beloved security blanket over the past few years. I wear it everywhere - to classes, the library, even fancy campus events because hey, it's basically a trendy fashion statement at this point, right?2 - The two energy drinks I seem to survive on each day to power through my overloaded schedule of lectures, study sessions, club meetings, and the occasional party. I know, I know...not the healthiest fuel, but they've become an essential vice.3 - The magical number of hours of sleep I tend to get each night as the endless cycle of work, activities, and socializing rages on around me. The insomnia struggle is real!4 - The four different painfully complicated academic subjects I'm somehow juggling this semester between math, science, language, and philosophy courses. What was I thinking?5 - The number of different aimless, stress-induced doodles you can find covering the margins of all my class notebooks as my mind inevitably starts to wander during intense lectures. Lots of stars, squiggles, and indecipherable figures.6 - The seemingly endless count of random papers, handouts, and misc items currently stuffed into my backpack, creating a constantly cluttered abyss to dig through when I need to find anything specific. I really need to just empty and reorganize the whole thing.7 - The approximate number of times per class that I have to resist the urge to pull out my phone and scroll through social media feeds when the topic gets dry or I start to lose focus. The constant temptation!8 - The ungodly number of cups of coffee I tend to consume over the course of each day to try and cope with my lack of sleep and get through quantities of work, reading assignments, and late night study sessions. Caffeine is my closest friend/enemy.9 - The number of differentBuffCourses (our online course portal) tabs I have open at any given time across my laptop and phone for accessing materials, uploading assignments, and the like for all my current classes. Organizational overload!10 - The shocking number of times I seem to lose or misplace something important like my student ID, dorm key, wallet, etc. over the span of each semester due to a combination of chaos and lack of sleep making me a certified scatterbrain. It'sgotten to the point where my friends just shake their heads knowingly whenever I'm frantically looking for something.11 - The minimum number of times per day I check my email inbox, constantly sifting through a wide array of messages from professors, campus organizations, promotions, and more. It never stops!12 - The number of random pens I seem to have scattered across all my bags, notebooks, room, etc. at any given time. Yet whenever I need one, none of them have ink and I'm left scrawling away with a sad useless pen like a crazy person.13 - The count of different textbooks I'm currently lugging around with me at all times in my backpack and constantly referencing for classes and assignments. My back may be slowly disintegrating under the weight.14 - The number of times per week I tend to have at least a partial mental breakdown, meltdown, or existential crisis about my life, workload, future, or just life in general. The stress is real, folks!15 - The collection of distinct binders and notebooks I use for separately organizing materials for each of my courses to try and maintain some semblance of order amidst theoverwhelming chaos and information overload. Colors and labels are my friends.16 - The seemingly infinite number of random crumpled up wrappers, granola bar boxes, and snack bags that always end up accumulating in the depths of my backpack and covering my desk during intense study binges. I'm a ravenous snacker.17 - The stash of miscellaneous medications like pain relievers, cold pills, etc. that I horded at the start of the year and somehow still have hanging around after all this time. You never know when you'll need them!18 - The extensive array of електронний записник apps and organizational tools I have downloaded onto my devices to try and structure all the madness, only to end up a useless tangle of half-used planners, reminders, notes, and more. I'm a lost cause when it comes to organization.19 - The ungodly number of times I hit the snooze button each morning, constantly struggling to pull myself out of bed and leave my precious sleep behind to face another intense day. I'm in a perpetual battle with my alarm clock.20 - Finally, the approximate number of times throughout each week that I realize I'm in way over my head, completelyovercommitted, and question why I even put myself through all this stress and exhaustion of college life in the first place. Yet despite it all, I keep pushing forward step-by-step!So there you have it - a fully numerical introduction into this crazy, chaotic, overstuffed life I lead as a university student. Somehow among all those uncountable cups of coffee, piles of papers, amalgamations of pens and binders, constant laments about lack of sleep, and stretches of stress and overwhelm, I'm making it through semester by semester. Wish me luck as I currently forge on into the deep abyss of final exams and the sudden realization that yet another year of this delightfully insane experience has nearly come to a close! Thanks for reading my number-filled little narrative - I'll catch you on the flip side!篇3My Life in Numbers: An Inventory of a Student's PossessionsAs a student, my life can often feel like a jumble of books, papers, pens, and an ever-growing collection of random items I've accumulated over the years. But have you ever stopped to really take stock of all the things you own? I decided to do just that, and the results were quite eye-opening. So, join me on thisnumerical journey as I break down and quantify the physical possessions that make up my life as a student.Let's start with the essentials: books. Being a voracious reader, I have amassed a library of 127 books, ranging from classic literature to modern best-sellers. These tomes are my constant companions, providing me with knowledge, entertainment, and a much-needed escape from the stresses of student life.Moving on to the academic necessities, I have 9 notebooks, each filled with meticulously taken notes from various classes. These notebooks are the repositories of my academic journey, holding the key to acing exams and cementing my understanding of complex concepts.Of course, what would a student's life be without pens? I currently own 27 pens of various colors and styles. From sleek ballpoint pens to vibrant gel pens, each one serves a specific purpose, whether it's taking notes, highlighting important passages, or adding a touch of creativity to my doodles.But let's not forget about the digital realm. As a modern student, I rely heavily on technology to aid in my studies. I have 3 laptops, each serving a different purpose: one for classes, one for personal use, and a trusty backup in case of emergencies.To complement my laptops, I have 5 external hard drives, each containing a wealth of digital resources, from research papers to multimedia files. These hard drives are my digital safety nets, ensuring that my precious data is always backed up and accessible.Now, let's talk about the fun stuff. As a student, it's important to maintain a balance between work and play, and my collection of 18 board games and 32 video games helps me do just that. From intense strategy games to lighthearted party games, these diversions provide much-needed breaks from the rigors of academic life.But what's a student's life without a touch of nostalgia? I have a cherished collection of 45 stuffed animals, each one holding a special memory from my childhood. These plush companions have been with me through thick and thin, offering comfort and companionship during the most challenging times.Of course, no student's life would be complete without the obligatory college memorabilia. I have 12 t-shirts emblazoned with the logo of my university, proudly displaying my affiliation and school spirit.As I delve deeper into this inventory, I realize that my possessions are more than just inanimate objects; they arephysical manifestations of my experiences, interests, and aspirations. Each item tells a story, a chapter in the ever-evolving narrative of my life as a student.Take, for instance, the 7 potted plants adorning my living space. These green companions not only add a touch of nature to my surroundings but also serve as reminders of the importance of nurturing and growth, both in academics and in life.And let's not forget the 23 art prints and posters that adorn my walls. These vibrant displays of creativity not only add visual interest to my living space but also serve as constant sources of inspiration, reminding me to think outside the box and embrace my artistic side.But wait, there's more! I have a collection of 37 mugs, each one with a unique design or quirky phrase. These mugs are more than just vessels for my daily caffeine fix; they are conversation starters, icebreakers, and reflections of my personality.As I continue to take stock of my possessions, I'm struck by the sheer diversity and quantity of items that surround me. From the 68 pairs of socks in my drawer (because let's face it, no student can have too many socks) to the 14 different types of snacks in my pantry (a testament to my love for late-night studysessions), each item serves a purpose and contributes to the tapestry of my student life.And let's not forget about the miscellaneous items that defy categorization. I have 21 random knick-knacks, each with its own unique story and significance. From a quirky paperweight gifted by a friend to a tiny figurine picked up on a memorable trip, these items serve as tangible reminders of the experiences that have shaped me as a person.As I reflect on this numerical inventory, I can't help but feel a sense of gratitude for the abundance of possessions that surround me. Each item, no matter how seemingly insignificant, plays a role in my life as a student, contributing to my growth, comfort, and overall well-being.But more importantly, this exercise has taught me that true wealth lies not in the accumulation of possessions but in the experiences and memories they represent. The items that hold the most value are those that have accompanied me on my journey, serving as constant reminders of the lessons learned, the challenges overcome, and the friendships forged along the way.So, while this inventory may have started as a mere exercise in quantifying my possessions, it has evolved into a poignantreflection on the rich tapestry of my student life. Each item, from the well-worn notebooks to the cherished stuffed animals, tells a story – a story of growth, perseverance, and the relentless pursuit of knowledge.As I embark on the next chapter of my academic journey, I carry with me not just the physical possessions but the lessons and memories they represent. And who knows, perhaps one day, I'll look back on this inventory and smile, remembering the days when my life could be neatly quantified in numbers, before it blossomed into a rich tapestry of experiences and adventures yet to come.。
tpo32三篇托福阅读TOEFL原文译文题目答案译文背景知识

tpo32三篇托福阅读TOEFL原文译文题目答案译文背景知识阅读-1 (2)原文 (2)译文 (5)题目 (7)答案 (16)背景知识 (16)阅读-2 (25)原文 (25)译文 (28)题目 (31)答案 (40)背景知识 (41)阅读-3 (49)原文 (49)译文 (53)题目 (55)答案 (63)背景知识 (64)阅读-1原文Plant Colonization①Colonization is one way in which plants can change the ecology of a site. Colonization is a process with two components: invasion and survival. The rate at which a site is colonized by plants depends on both the rate at which individual organisms (seeds, spores, immature or mature individuals) arrive at the site and their success at becoming established and surviving. Success in colonization depends to a great extent on there being a site available for colonization – a safe site where disturbance by fire or by cutting down of trees has either removed competing species or reduced levels of competition and other negative interactions to a level at which the invading species can become established. For a given rate of invasion, colonization of a moist, fertile site is likely to be much more rapid than that of a dry, infertile site because of poor survival on the latter. A fertile, plowed field is rapidly invaded by a large variety of weeds, whereas a neighboring construction site from which the soil has been compacted or removed to expose a coarse, infertile parent material may remain virtually free of vegetation for many months or even years despite receiving the same input of seeds as the plowed field.②Both the rate of invasion and the rate of extinction vary greatly among different plant species. Pioneer species - those that occur only in the earliest stages of colonization -tend to have high rates of invasion because they produce very large numbers of reproductive propagules (seeds, spores, and so on) and because they have an efficient means of dispersal (normally, wind).③If colonizers produce short-lived reproductive propagules, they must produce very large numbers unless they have an efficient means of dispersal to suitable new habitats. Many plants depend on wind for dispersal and produce abundant quantities of small, relatively short-lived seeds to compensate for the fact that wind is not always a reliable means If reaching the appropriate type of habitat. Alternative strategies have evolved in some plants, such as those that produce fewer but larger seeds that are dispersed to suitable sites by birds or small mammals or those that produce long-lived seeds. Many forest plants seem to exhibit the latter adaptation, and viable seeds of pioneer species can be found in large numbers on some forest floors. For example, as many as 1,125 viable seeds per square meter were found in a 100-year-old Douglas fir/western hemlock forest in coastal British Columbia. Nearly all the seeds that had germinated from this seed bank were from pioneer species. The rapid colonization of such sites after disturbance is undoubtedly in part a reflection of the largeseed band on the forest floor.④An adaptation that is well developed in colonizing species is a high degree of variation in germination (the beginning of a seed’s growth). Seeds of a given species exhibit a wide range of germination dates, increasing the probability that at least some of the seeds will germinate during a period of favorable environmental conditions. This is particularly important for species that colonize an environment where there is no existing vegetation to ameliorate climatic extremes and in which there may be great climatic diversity.⑤Species succession in plant communities, i.e., the temporal sequence of appearance and disappearance of species is dependent on events occurring at different stages in the life history of a species. Variation in rates of invasion and growth plays an important role in determining patterns of succession, especially secondary succession. The species that are first to colonize a site are those that produce abundant seed that is distributed successfully to new sites. Such species generally grow rapidly and quickly dominate new sites, excluding other species with lower invasion and growth rates. The first community that occupies a disturbed area therefore may be composed of specie with the highest rate of invasion, whereas the community of the subsequent stage may consist of plants with similar survival ratesbut lower invasion rates.译文植物定居①定居是植物改变一个地点生态环境的一种方式。
物流英语题库
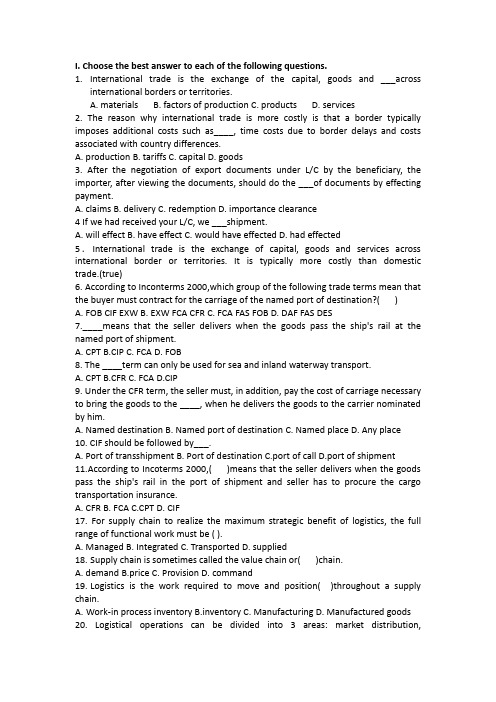
I. Choose the best answer to each of the following questions.1.International trade is the exchange of the capital, goods and ___acrossinternational borders or territories.A. materialsB. factors of productionC. productsD. services2. The reason why international trade is more costly is that a border typically imposes additional costs such as____, time costs due to border delays and costs associated with country differences.A. productionB. tariffsC. capitalD. goods3. After the negotiation of export documents under L/C by the beneficiary, the importer, after viewing the documents, should do the ___of documents by effecting payment.A. claimsB. deliveryC. redemptionD. importance clearance4 If we had received your L/C, we ___shipment.A. will effectB. have effectC. would have effectedD. had effected 5.International trade is the exchange of capital, goods and services across international border or territories. It is typically more costly than domestic trade.(true)6. According to Inconterms 2000,which group of the following trade terms mean that the buyer must contract for the carriage of the named port of destination?( )A. FOB CIF EXWB. EXW FCA CFRC. FCA FAS FOBD. DAF FAS DES7.____means that the seller delivers when the goods pass the ship's rail at the named port of shipment.A. CPTB.CIPC. FCAD. FOB8. The ____term can only be used for sea and inland waterway transport.A. CPTB.CFRC. FCAD.CIP9. Under the CFR term, the seller must, in addition, pay the cost of carriage necessary to bring the goods to the ____, when he delivers the goods to the carrier nominated by him.A. Named destinationB. Named port of destinationC. Named placeD. Any place10. CIF should be followed by___.A. Port of transshipmentB. Port of destinationC.port of callD.port of shipment11.According to Incoterms 2000,( )means that the seller delivers when the goods pass the ship's rail in the port of shipment and seller has to procure the cargo transportation insurance.A. CFRB. FCAC.CPTD. CIF17. For supply chain to realize the maximum strategic benefit of logistics, the full range of functional work must be ( ).A. ManagedB. IntegratedC. TransportedD. supplied18.Supply chain is sometimes called the value chain or( )chain.A. demandB.priceC. ProvisionD. command19.Logistics is the work required to move and position( )throughout a supply chain.A.Work-in process inventoryB.inventoryC. ManufacturingD. Manufactured goods20. Logistical operations can be divided into 3 areas: market distribution,manufacturing support, and ( ).A.ProcurementB. AppointmentC.serviceD. Order21.In most supply chains,customer requirements are transmitted in the form of____.A.MaterialsB.OrdersC..valuseD.Inventories22. Finding and managing the desired( )mix across the supply chain is a primary responsibilities of logistics.A.ValueB.ServiceC.TransportationD.Cost23. Transportation requirements can be satisfied in ( )basic ways.A.2B.3C.4D.524. For a successful logistics system, a delicate balance should be maintained between transportation cost and ( ).A.inventoryB.Market shareC.Market serviceD.Service quality25. Logistical strategies should be designed to maintain the ( )possible financial investment in inventory.A.lowestB.MaximumC.ProperD.Highest26. An enterprise operating transportation in logistics may engage the service of a wide variety of( )that provide different transportation services on a per shipment basis.A.CarriersB. ConsigneesC.Customers27. within individual logistics areas,different movement requirements exist with respect to ( ),availability of inventory,and urgency of movement.A.manufacturing supportB.ProcurementC.Size of orderD.Market distribution28.()is concerned with purchasing and arranging inbound movement ofmaterials,parts,and /or finished inventory from suppliers to manufacturing or assembly plants,warehouses,or retail stores.A.market distributionB.ProcurementC.Manufacturing supportD.Inventory。
一些a range of
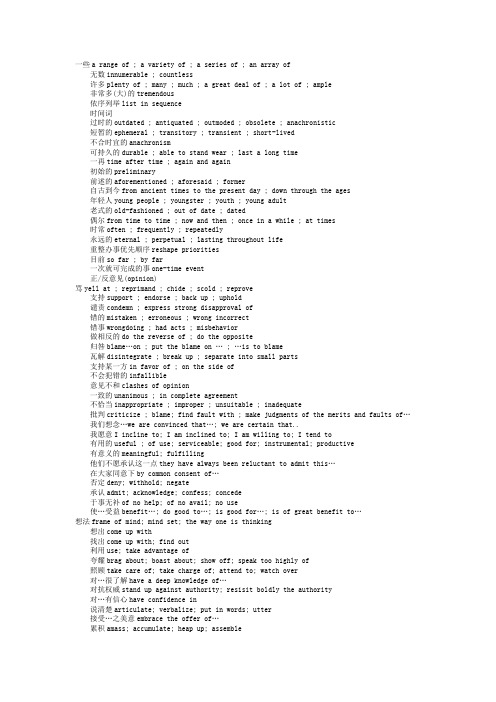
一些a range of ; a variety of ; a series of ; an array of无数innumerable ; countless许多plenty of ; many ; much ; a great deal of ; a lot of ; ample非常多(大)的tremendous依序列举list in sequence时间词过时的outdated ; antiquated ; outmoded ; obsolete ; anachronistic短暂的ephemeral ; transitory ; transient ; short-lived不合时宜的anachronism可持久的durable ; able to stand wear ; last a long time一再time after time ; again and again初始的preliminary前述的aforementioned ; aforesaid ; former自古到今from ancient times to the present day ; down through the ages年轻人young people ; youngster ; youth ; young adult老式的old-fashioned ; out of date ; dated偶尔from time to time ; now and then ; once in a while ; at times时常often ; frequently ; repeatedly永远的eternal ; perpetual ; lasting throughout life重整办事优先顺序reshape priorities目前so far ; by far一次就可完成的事one-time event正/反意见(opinion)骂yell at ; reprimand ; chide ; scold ; reprove支持support ; endorse ; back up ; uphold谴责condemn ; express strong disapproval of错的mistaken ; erroneous ; wrong incorrect错事wrongdoing ; had acts ; misbehavior做相反的do the reverse of ; do the opposite归咎blame…on ; put the blame on … ; …is to blame瓦解disintegrate ; break up ; separate into small parts支持某一方in favor of ; on the side of不会犯错的infallible意见不和clashes of opinion一致的unanimous ; in complete agreement不恰当inappropriate ; improper ; unsuitable ; inadequate批判criticize ; blame; find fault with ; make judgments of the merits and faults of…我们想念…we are convinced that…; we are certain that..我愿意I incline to; I am inclined to; I am willing to; I tend to有用的useful ; of use; serviceable; good for; instrumental; productive有意义的meaningful; fulfilling他们不愿承认这一点they have always been reluctant to admit this…在大家同意下by common consent of…否定deny; withhold; negate承认admit; acknowledge; confess; concede于事无补of no help; of no avail; no use使…受益benefit…; do good to…; is good for…; is of great benefit to…想法frame of mind; mind set; the way one is thinking想出come up with找出come up with; find out利用use; take advantage of夸耀brag about; boast about; show off; speak too highly of照顾take care of; take charge of; attend to; watch over对…很了解have a deep knowledge of…对抗权威stand up against authority; resisit boldly the authority对…有信心have confidence in说清楚articulate; verbalize; put in words; utter接受…之美意embrace the offer of…累积amass; accumulate; heap up; assemble连系tact; get in touch with; contact with排除这可能性rule out the possibility等于is equivalent to; equal选择choose; elect; opt for; pick; single out发出deliver; give out; hand over绕路detour; take a detour; take a roundabout way禁止进入is kept out; is barred from小看make little of坏了out of order; on the blink; is not working分别distinguish bet ween; make a distinction between; tell…from依靠count on; depend on忽视neglect; give too little care to存在come to be; come into existence; come to birth; come into being考虑consider; take into consideration; take into account考虑到in consideration of用尽力气exhaust one’s strength; use up one’s strength开动initiate; set going准备…brace for; prepare for在于lie in; rest on; rest with主动take the initiative不算exclusive of; not counting; leaving out应该得到deserve; have right to; is worthy of避免avoid; shun; get around; circumvent幻想fantasy; play of the mind以此标准来算by this criterion; by this standard乍看之下at first glance面对in the face of; in the presence of以by means of; by virtue of; by the use of不惜代价at all costs每况愈下from bad to worse承受错误造成的后果in reaping the harvest of his mistakes取得同意…get the go-ahead to不择手段unscrupulously; by hook or by crook想法与作法beliefs and practices内情ins and outs; turns and twists关键时刻the critical moment虽然although; notwithstanding; albeit; though根据according to; on the basis of; on the ground of (that); in the light of; in line with; in accordance with逃避问题evade the question增大enlarge; extend; aggrandize澄清clarify; make clear赔偿compensate for; give…as compensation for实现carry out; implement; real ize; make…come true假定suppose; assume; postulate; hypothesize极端的radical; extreme极端的措施drastic measures剩下的the rest; the remainder; what is left换言之in other words; put another way结果result; aftermath; consequence优点advantage; strength; strong point; merit; benefit简言之put simply; in short; in brief; in a nutshell举例而言for instance; for example; to illustrate; let us cite 特别是an illustration; to cite a concrete case特别是especially; more than others; particularly; in particular既然…now that…;seeing that…迹象inkling; hint; clue; a slight suggestion缺点disadvantage; demerit; shortcoming; drawback; weakness除去do away with; eliminate; remove; get rid of缺少for lack of; for a deficiency of毕竟after all; all in all范围scope; field; realm潜力potential;行为conduct; behavior; doings隔绝isolate; insulate分辨出identify; recognize不易懂的elusive; hard to understand展开unfold回馈feedback主导的人物a dominant figure; a controlling man; the most influential person 观点viewpoint; point of view; perspective; standpoint正在进行中is underway只是一种姿态is merely a gesture立场position; stand; stance意向inclination; leaning; intention特权privilege; a special right来自stem from; come from一件事的不同说法alternative statements of fact交织intertwine; interweave好奇心the eager desire to know; curiosity尊敬respect; esteem; think highly of顽固的headstrong; obstinate; stubborn暗淡的gloomy; dark; dim巨大的huge; gigantic; colossal; vast; enormous; tremendous探索explore; fathom执行carry out; execute; do现代modern times; modern age; contemporary age偏见prejudice; bias; partiality; predilection混乱chaos; commotion; confusion; disturbance; tumult无弹性(僵硬)rigid无缺点的flawless; airtight无药可救incurable无法避免的unavoidable; inevitable细密的计划elaborate plan取消cancel; annul; abolish解药a cure for…; a remedy for;谜puzzle; riddle; enigma机会平等equality of opportunity较有影响力的国家a predominant country遵守abide by; conform to; observe; comply with热情的passionate; ardent; zealous模糊的ambiguous; vague; obscure影响长远的far-reaching失望despair; loss of hope; without hope幼稚childish; childlike; na?e挑剔的picky; choosy; fastidious破坏destroy; ruin; break to pieces; devasate技巧的skillful; adept; dexterous警觉的alert; watchful; on guard; wary of忍受bear; put up with; endure; stand证据evidence; facts; proof; grounds; testimony很容易地easily; with little problem; with little hindrance令人惊讶的amazing; astonishing; astounding生动的报导vivid description争取compete for; try hard to win遗产heritage; legacy; inheritance保护protect; safeguard; preserve; shelter了解understand; comprehend; catch the meaning of; catch on汇露reveal; make known; disclose放大amplify; magnify; enlarge动力impetus; driving force; momentum自满的complacent第一流的first-rate; excellent安全处refuge; asylum; haven; sanctuary强调emphasize; stress; highlight短视的决定short-sighted decision真正的genuine; authentic; real怪异的eccentric; peculiar; odd明显的distinct; clear; explicit; obvious得到…的注意capture one’s attention事事干涉的meddlesome; interfering背景setting; background假的fake; false; counterfeit夸大报导dramatize退步setback古人the ancients古老的old; ancient; archaeic逃犯infringe (on); violate使害怕intimidate; frighten带来生气enliven对手rival吸引人的intriguing旁观者onlooker准确地说to be exact; to be precise; precisely突然醒悟it dawned on me that仔细思考之后after long deliberation; after careful thought对比及其相关用词可互换的interchangeable可与…相比is comparable with (to)普遍的prevailing; common; prevalent是一个对比is a sharp contrast to比作is likened to; is compared to多样化的heterogeneous单一性的homogeneous一般而言in general; generally speaking; by and large满于现状be happy with what you are预测未来project into the future另一个观点是… another way of looking at the matter is…不宜取笑… it is not decent to make fun of…评估社会文化因素 assess (evaluate) sociocultural factors那并非说… that does not mean that…那有这回事 there is no such thing as一个有待克服的困难是… a major hurdle for us to overcome is…由…造成 caused by; attributable to; due to; resulting from由…组成is made up of…; is comprised of; consist必须从两方面考虑此问题this problem needs to be considered on two dimensions:限制limit; restrict; refrain; restrain; keep within limits; confine; keep in check 一般人认为… conventional wisdom suggests that…这方法有陷阱the method had pitfalls:说服convince; persuade; cause to believe具体的specific; concrete; tangible刻意的intentional; on purpose; intended费时间去了解…take time to acquaint oneself with……是此问题的核心…is at the root of the issue无法估计is beyond calculation; incalculable无资格的disqualified一、用形容词“very”,“single”等表示强调Red Army fought a battle on this very spot. 红军就在此地打过一仗。
一个简单的去除重复字段的SQL查询语句
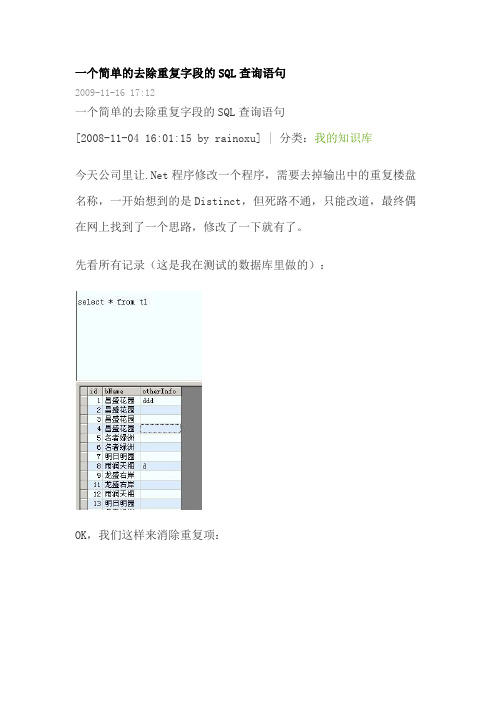
一个简单的去除重复字段的SQL查询语句2009-11-16 17:12一个简单的去除重复字段的SQL查询语句[2008-11-04 16:01:15 by rainoxu] | 分类:我的知识库今天公司里让.Net程序修改一个程序,需要去掉输出中的重复楼盘名称,一开始想到的是Distinct,但死路不通,只能改道,最终偶在网上找到了一个思路,修改了一下就有了。
先看所有记录(这是我在测试的数据库里做的):OK,我们这样来消除重复项:1.select * from table1 as awhere not exists(select 1 from table1 where logID=a.LogID and ID>a.ID)2.最近做一个数据库的数据导入功能,发现联合主键约束导致不能导入,原因是源表中有重复数据,但是源表中又没有主键,很是麻烦。
经过努力终于解决了,现在就来和大家分享一下,有更好的办法的可以相互交流。
有重复数据主要有一下几种情况:1.存在两条完全相同的纪录这是最简单的一种情况,用关键字distinct就可以去掉example:select distinct * from table(表名) where (条件)2.存在部分字段相同的纪录(有主键id即唯一键)如果是这种情况的话用distinct是过滤不了的,这就要用到主键id的唯一性特点及group by分组example:select * from table where id in (select max(id) from table group by [去除重复的字段名列表,....])3.没有唯一键ID这种情况我觉得最复杂,目前我只会一种方法,有那位知道其他方法的可以留言,交流一下:example:select identity(int1,1) as id,* into newtable(临时表) from table select * from newtable where id in (select max(id) from newtable group by [去除重复的字段名列表,....])drop table newtable关于一个去除重复记录的sql语句2009-8-24 16:33提问者:lichuanbao1234|悬赏分:30 |浏览次数:1075次我要查询一个表中content字段相同的记录的详细信息。
Agent Vi Vi-Search产品介绍说明书
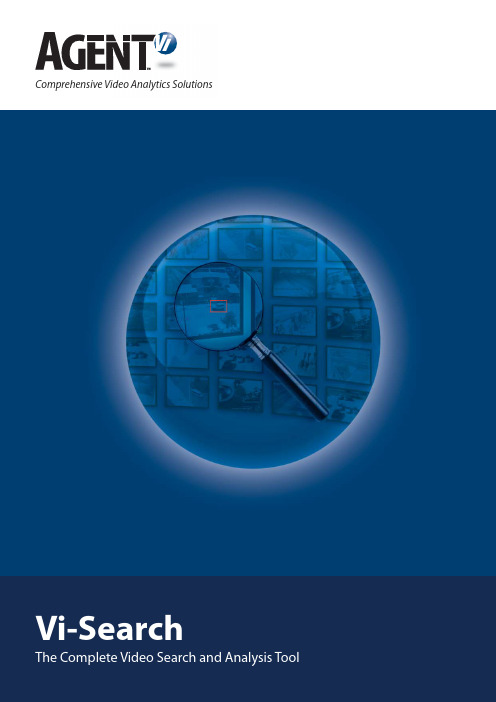
Comprehensive Video Analytics SolutionsVi-SearchThe Complete Video Search and Analysis ToolVi-Search is an innovative video search software that enables rapid and effective retrieval and presentation of specific video segments, events and data from vast amounts of recorded video. While video recording is a basic component of most surveillance networks, the recorded video is rarely utilized due to the lack of an automated and time-efficient solution that enables search and analysis of such stored video. In response, V i-Search automates the search and analysis process and allows for true leveraging of the stored video.The software analyzes the video stream, generates metadata describing the scene content, and allows for later retrieval and analysis of the video through an automatic search within the stored metadata.Based on Agent Vi’s open architecture, pure software approach, Vi-Search seamlessly integrates with a wide range of edge devices and video management systems, in both new and existing surveillance networks.Vi-Search HighlightsAutomatic and Time-Efficient• Scans days of stored video in seconds to display precise results• Eliminates the need for time-consuming manual search and review of stored videoSearch Flexibility• Enables search by event or search by target parameters, including type, size and color• Scene metadata is continuously collected and logged, with no need to pre-define search parametersHighly Scalable• Can be easily applied and expanded to surveillance networks of any size• Enables simultaneous search on multiple cameras Open Architecture• Integrated with a wide range of edge devices and video management systems• Search can be conducted through Vi-Search’s GUI or an integrated third party video management system Hardware-Efficient• Single server can generate detailed and accurate scene metadata for hundreds of cameras simultaneously User-Friendly• No complicated set-up or training required• Operated by simple search queries• Enables easy export of specific video segments and reports as stand-alone filesApplicationsVi-Search enables utilization of stored video for a wide variety of security, safety, law enforcement and business intelligence applications:Business Intelligence and Operational Efficiency applications can benefit from statistical analysis of human or vehicle traffic in specific areas and in distinct time frames. Retail chains and other businesses or organizations can better understand and predict traffic patterns to efficiently allocate resources, provide better customer care, and improve operational procedures.Forensic Analysis often requires search through vast amounts of stored video to pinpoint certain events, or to trace events back to their origin. Through its wide range of search parameters and capabilities, Vi-Search enables users to locate an event in the stored video with high precision in a matter of seconds. A stand-alone file containing the relevant video segment can be easily extracted and saved as evidence.Time-Critical Security and Law Enforcement Opera-tions require quick and effective responses to events as they occur. Once there is knowledge of a criminal or ter-rorist activity, events can be traced back for immediate investigation, providing law enforcement agencies with a full picture of the event including origin and evolution. In this way, deployment and tactical responses are better informed, improving crisis management.V i-Search allows you to reap the true benefits of your surveillance network by enabling automatic and effortless retrieval and analysis of valuable information contained in your stored video.Search and Analysis Functionality Vi-Search provides the following main search and analysis functionalities:Forensic SearchEnables search for particular events or objects in a speci-fied group of cameras and within a distinct time frameStatistical AnalysisConducts people or vehicle counting and generates statistical reports, for a specified group of cameras and within a distinct time frameMotion Path AnalysisProduces graphical presentation of all motion paths in a scene, with immediate access to the video segment relating to each pathVideo SummaryAllows debriefing of multiple search results through one condensed clipSearch Parameters and CapabilitiesVi-Search offers an extensive set of intuitive search parameters and capabilities, including: • Target Type – People, Vehicles, Objects• Event Type – Moving, Stationary, Crossing a line, Occupancy, Crowding • Search by Color and/or Size • Search within defined time frames• Search on selected cameras or group of cameras • Search for Similar Targets – Once a target is observed, a simple search can be conducted to locate additional appearances of the same or similar target in the recorded videoVi-Search is a complete video search and analysis solution. Its open architecture, hardware-efficient approach makes Vi-Search a feasible and effective solution for all markets and industries.Software & Hardware RequirementsVi-Search Server(for 100 cameras)•CPU - Core2Duo 2.66 GH (or similar), RAM - 2 GB• Hard drive - 200 GB per month of storage• OS - Windows XP Professional, Windows Server 2003, Windows Server 2008Vi-Search Client• CPU - Core2Duo 2.4G Hz (or similar), RAM - 1 GB• Hard drive - 50 GB• OS - Windows XP Professional, Windows VistaServer Scalability Analytics servers can be easily added to support more camerasStronger processors can support more cameras on one serverSystem PerformanceQuery Time Average of 15 seconds per camera per 24 hours of recorded metadataRequired Metadata Storage Average of 50 MB per camera per dayIntegration PartnersSupported Edge Devices Axis, COE, IQInVision, MangoDSP, Sony, Verint, VivotekFor additional devices and specific models contact ****************Supported VideoManagement SystemsMilestone, OnSSI, ViconFor a list of vendors and supported versions contact****************Agent Video Intelligence (Agent Vi) is a leading provider of open architecture, video analytics software deployed in a variety of security, safety and business intelligence applications worldwide. The comprehensive video analytics solutions offered by Agent Vi extend from real-time video analysis and alerts to forensic search and post-event analysis, and are fully integrated with a range of third party edge devices and video management systems. Integrating Agent Vi’s advanced video analytics capabilities into existing or new surveillance networks enables users to benefit from the true potential of their surveillance networks, transforming them into intelligent tools that respond to the practical challenges of the 21st century.Agent Video Intelligence Ltd. USA: +1 303 534 5106 EMEA & APAC: +972 72 220 1500For more information, visit: or email: *****************。
target detection
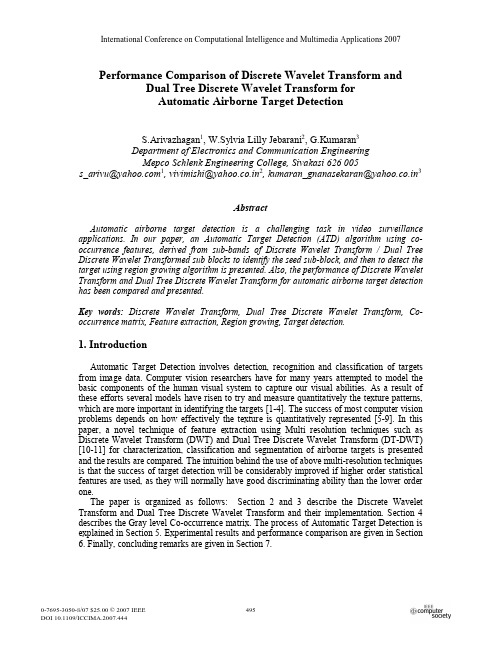
International Conference on Computational Intelligence and Multimedia Applications 2007Performance Comparison of Discrete Wavelet Transform andDual Tree Discrete Wavelet Transform forAutomatic Airborne Target DetectionS.Arivazhagan1, W.Sylvia Lilly Jebarani2, G.Kumaran3Department of Electronics and Communication EngineeringMepco Schlenk Engineering College, Sivakasi 626 005s_arivu@1, vivimishi@yahoo.co.in2, kumaran_gnanasekaran@yahoo.co.in3AbstractAutomatic airborne target detection is a challenging task in video surveillance applications. In our paper, an Automatic Target Detection (ATD) algorithm using co-occurrence features, derived from sub-bands of Discrete Wavelet Transform / Dual Tree Discrete Wavelet Transformed sub blocks to identify the seed sub-block, and then to detect the target using region growing algorithm is presented. Also, the performance of Discrete Wavelet Transform and Dual Tree Discrete Wavelet Transform for automatic airborne target detection has been compared and presented.Key words: Discrete Wavelet Transform, Dual Tree Discrete Wavelet Transform, Co-occurrence matrix, Feature extraction, Region growing, Target detection.1. IntroductionAutomatic Target Detection involves detection, recognition and classification of targets from image data. Computer vision researchers have for many years attempted to model the basic components of the human visual system to capture our visual abilities. As a result of these efforts several models have risen to try and measure quantitatively the texture patterns, which are more important in identifying the targets [1-4]. The success of most computer vision problems depends on how effectively the texture is quantitatively represented [5-9]. In this paper, a novel technique of feature extraction using Multi resolution techniques such as Discrete Wavelet Transform (DWT) and Dual Tree Discrete Wavelet Transform (DT-DWT) [10-11] for characterization, classification and segmentation of airborne targets is presented and the results are compared. The intuition behind the use of above multi-resolution techniques is that the success of target detection will be considerably improved if higher order statistical features are used, as they will normally have good discriminating ability than the lower order one.The paper is organized as follows: Section 2 and 3 describe the Discrete Wavelet Transform and Dual Tree Discrete Wavelet Transform and their implementation. Section 4 describes the Gray level Co-occurrence matrix. The process of Automatic Target Detection is explained in Section 5. Experimental results and performance comparison are given in Section 6. Finally, concluding remarks are given in Section 7.2. Discrete wavelet transformThe Discrete Wavelet Transform (DWT) analyzes the signal at different frequency bands with different resolutions by decomposing the signal into a coarse approximation and detail information. The DWT employs two sets of functions, called scaling functions and wavelet functions, which are associated with low pass and high pass filters, respectively. In order to decompose an image, first, the low pass filter (h) is applied for each row of data and subsequently down sampled by 2, thereby getting the low frequency components of the row. Then, the high pass filter (g) is applied for the same row of data, subsequently down sampled by a factor 2 to get high frequency components, and placed by the side of low pass components. This procedure is done for all rows. Down sampling is done to satisfy Nyquist’s rule, since the signal now has a highest frequency of /2πradians instead of πafter filtering. Next, the filtering is done for each column of the intermediate row decomposed data. The resulting two-dimensional array of coefficients contains four bands of data, each labeled as LL1 (low-low), LH1 (low-high), HL1 (high-low) and HH1 (high-high), corresponding to first level of image decomposition. The LL1 band can be further decomposed in the same manner for second level of decomposition. This can be done up to any level, thereby resulting in a pyramidal decomposition. The one level decomposed image is represented in Figure 1 (a) andFigure 1. Image Decomposition using DWT; (a) One level DWT(b) Filter bank structure for one level of Image decompositionIn our implementation, the target image is subjected to one level of DWT decomposition and the features derived from three detail sub-bands are used for target detection.3. Dual tree discrete wavelet transformThe standard DWT and its extensions suffer from two or more serious limitations. They are: (i) Lack of shift invariance , which means that small shifts in the input signal can cause major variations in the distribution of energy between DWT coefficients at different scales and (ii) Poor directional selectivity for diagonal features, because the wavelet filters are separable and real.A well-known way of providing shift invariance is to use the undecimated form of the dyadic filter tree. However this still suffers from substantially increased computation requirements compared to the fully decimated DWT and also exhibits high redundancy in the(a) 2 1 2 0 1 0 2 1 1 2 0 1 2 2 0 1 2 2 0 1 2 0 1 0 1(a)(b)output information, making subsequent processing expensive too. A more computationally efficient approach to shift invariance is Dual-Tree Discrete Wavelet Transform (DT-DWT). Furthermore, the DT-DWT also gives much better directional selectivity when filtering multidimensional signals. In summary, it has the properties, such as (i) Approximate shift invariance, (ii) Good directional selectivity in 2-dimensions and (iii) Perfect reconstruction (PR) using short linear-phase filtersDT-DWT use complex-valued filtering (analytic filter) that decomposes the real/complex signals into real and imaginary parts in transform domain. When an image is subjected to one level DT-DWT decomposition, it results in 16 sub bands as shown in Figure 2 (a). These 16 sub bands arise from separable applications of real and imaginary filters on horizontal and vertical directions respectively as shown in Figure 2 (b). Here, each block, identical to standard 2D-DWT results in 4 sub-bands where h is the set of filters {h 0, h 1} and g is the set of filters {g 0, g 1}. The filters h 0, h 1 are real valued low pass and high pass filters respectively for real tree and g 0, g 1 are the corresponding filters for imaginary tree and x and y represent row and column directions respectively for filtering. The overall 2-D dual-tree structure is 4-times redundant than the standard 2-D DWT. In our implementation, features are derived from 12Figure 2. One level 2D DT- DWT;a) Image Decomposition; (b) Filter bank structure4. Gray level co-occurrence matrixWhen a digital image is represented in the form of matrix whose elements indicate the intensity level of the image at that point for the gray level images and the level varies from 0 to 255.Figure 3. Derivation of Co-occurrence matrix(a) A 5x5 image with three gray levels 0, 1 and 2; (b) Co-occurrence matrixorientation (c) The gray level Co-occurrence matrix for d = (1, 1).The gray-level co-occurrence matrix C [i, j] is defined by first specifying a displacement d = (dx, dy) and counting all pairs of pixels separated by d having gray-levels i, j [2]. The dx and dy represent the displacement in x and y directions respectively. For example, consider the simple 55× image having gray levels 0, 1 and 2 as shown in Figure 3 (a). Since, there are only three gray levels, C [i, j] is a 33× matrix. Let the position operator is specified as (1, 1), which has the interpretation: one pixel to the right and one pixel below. For example, there are three pairs of pixels having values [2, 1] which are separated by the specified distance, and hence the entry C[2, 1] has a value of 3. The complete matrix C [i,j] is shown in Figure 3 (c).5. Automatic target detection systemThe various processes involved in the Automatic Target Detection system are shown in Figure 4. The image of size N N × is individually considered as having a number of non-overlapping and adjacent sub-blocks of size n n × where n<N . Starting from the top-left corner5.1 Feature extraction A significant Co-occurrence feature namely contrast is derived from detail sub-bands of DWT / DT-DWT decomposed sub blocks of the original image using the equation (1). This feature is further used in target detection to identify the seed sub block for subsequent region growing process.∑=−=n j i j i C j i contrast 0,),(22)( (1)where C(i, j) is the Co-occurrence matrix elements.5.2 Seed identification and region growingOnce the contrast value of all the sub blocks are computed, the sub block having the maximum contrast value is identified as the ‘seed’ sub block for subsequent region growing. The region growing algorithm merges those neighboring sub blocks which are having a contrast difference less than the threshold. The threshold is computed from the mean of the difference of the contrast values between the ‘seed’ and its eight neighbors. The region growing algorithm is iteratively applied till new sub blocks are no longer merged along with the seed. As the merging process gets completed, a bounded rectangle encompasses the target with a mark on the centroid of the target.6. Experimental results and performance comparisonOur algorithm using DWT and DT-DWT is applied on 6 different airborne target still images of various sizes under both cloudy and clear environment and the experimental results obtained for the same are shown in Table 1. The table shows the original images, Target identified images using DWT and DT-DWT and the corresponding execution times. Though the size of the sub block is dependent on the size of the target, a sub block size of 32 x 32 gave promising results irrespective of the size of the targets in the images chosen for experimentation.Table 1. Performance Comparison of Target Detection using DWT and DT-DWTSl. No Input Image Target Identified image using DWT Target Identified image using DT-DWT Execution time in Seconds for DWT Execution time inSeconds forDT-DWT10.171 0.57820.140 0.45330.125 0.35940.203 0.81250.140 0.48460.156 0.484From the table, it is observed that both DWT and DT-DWT based algorithms performs well on all targets, irrespective of its size and orientation. Also, it is found that the average computation time using C language program for DWT is 0.155 second while for DT-DWT it is 0.528second.7. ConclusionBoth the Multi resolution techniques detect the target irrespective of their size, perspective and background. Compared to DWT, application of DT-DWT results in better spatial localization. This improvement is mainly due to deriving co-occurrence feature namely contrast, from 12 detail sub-bands against three sub-bands in DWT, which results in increased computational time.AcknowledgementThis project is funded by Armament Research Board, Defense Research Development Organization (DRDO), New Delhi. The authors are expressing their sincere thanks to the Management and Principal, Mepco Schlenk Engineering College, Sivakasi for their constant encouragement and support.References[1]. F.Espinal, B.D.Jawerth and T.Kubota, “Wavelet based fractal signature analysis for automatic targetrecognition”, Journal of Society of Photo-Optical Instrumentation Engineers, Vol. 37. No.1, 1998, pp. 166-174.[2].R.M.Haralick, K.Shanmugam and I.Dinstein, “Texture features for image classification”, IEEETransactions on System, Man, Cybernatics, Vol.8, No.6, 1973, pp. 610-621.[3].J.Sklansky, “Image segmentaion and feature extraction”, IEEE Transactions on System, Man, Cybernatics,Vol.8, No.4, 1978, pp. 237-247.[4].L.S.Davis, S.A.Johns and J.K.Aggarwal, “Texture analysis using generalized co-occurrence matrices”,IEEE Transactions on Pattern Analysis and Machine Intelligence, Vol.1, No.3, 1979, pp. 251–259.[5].M.Unser and M.Eden, “Multiresolution feature extraction and selection for texture segmentation”, IEEETransactions on Pattern Analysis and Machine Intelligence, Vol. 11, No.7, 1989, pp. 717 – 728.[6].Tianhorng Chang and C C Jay Kuo, “Texture analysis and classification with tree-structured wavelettransform”, IEEE Transactions on Image processing, Vol.2, No.4, 1993, pp. 429-440.[7].G Van de Wouwer, P Schenders and D Van Dyek, “Statistical texture characterization from discretewavelet representation”, IEEE Transactions on Image processing, Vol.8, No.4, 1999, pp. 592-598.[8].S.Arivazhagan and L.Ganesan, “Texture Classification using Wavelet Transform”, Pattern RecognitionLetters, Vol.24, No. 9-10, 2003, pp. 1513-1521.[9]. A.Howard, C.Padgett and K.Brown, “Real Time Intelligent Target Detection and Analysis with MachineVision”, Proc. of 3rd International Symposium on Intelligent Automation and Control, World Automation Congress, 2000.[10].S erkan Hat.ipoglu, Sanjit K. Mit.ra and Nick Kingsbury, “Texture classification using Dual-Tree ComplexWavelet Transform”, IEE Conference on Image Processing and its Applications, 465, 1999, pp. 344-347.[11].P anchamkumar D Shukla, “Complex Wavelet Transforms and their Applications”, M.Phil.Thesis, SignalProcessing Division, Department of Electronic and Electrical Engineering, University of Strathclyde, Glasgow G11XW, Scotland, United Kingdom, 2003.。
计算机专业英语 考试

一、选择题1.What is the process of converting a high-level programming language into machine languagecalled?A.Debuggingpilation(正确答案)C.ExecutionD.Interpretation2.Which of the following is a programming paradigm that organizes software design around data,and the operations performed on that data?A.Object-oriented programming(正确答案)B.Procedural programmingC.Functional programmingD.Event-driven programming3.In computer networks, what does the term "protocol" refer to?A. A set of rules governing the exchange of information between devices(正确答案)B.The physical connection between devicesC.The speed of data transmissionD.The type of data being transmitted4.What is the term used to describe the process of dividing a complex problem into smaller, moremanageable parts?A.Modularization(正确答案)B.OptimizationC.EncapsulationD.Polymorphism5.In computer security, what is the term for unauthorized access to or modification of data?A.EncryptionB.DecryptionC.Hacking(正确答案)D.Firewall6.Which of the following is a type of software that allows two or more computers tocommunicate and share resources?A.Operating systemB.Database management systemwork operating system(正确答案)D.Word processing software7.What is the term used to describe the process of identifying and correcting errors in computerprograms?A.Debugging(正确答案)B.TestingC.Codingpilation8.In computer graphics, what is the term for the number of distinct pixels that can be displayedon a screen?A.Resolution(正确答案)B.Color depthC.Refresh rateD.Aspect ratio。
- 1、下载文档前请自行甄别文档内容的完整性,平台不提供额外的编辑、内容补充、找答案等附加服务。
- 2、"仅部分预览"的文档,不可在线预览部分如存在完整性等问题,可反馈申请退款(可完整预览的文档不适用该条件!)。
- 3、如文档侵犯您的权益,请联系客服反馈,我们会尽快为您处理(人工客服工作时间:9:00-18:30)。
SIAM J.C OMPUT.c 2007Society for Industrial and Applied Mathematics Vol.37,No.2,pp.359–379RANGE-EFFICIENT COUNTING OF DISTINCT ELEMENTS IN AMASSIVE DATA STREAM∗A.PAVAN†AND SRIKANTA TIRTHAPURA‡Abstract.Efficient one-pass estimation of F0,the number of distinct elements in a data stream, is a fundamental problem arising in various contexts in databases and networking.We consider range-efficient estimation of F0:estimation of the number of distinct elements in a data stream where each element of the stream is not just a single integer but an interval of integers.We present a randomized algorithm which yields an( ,δ)-approximation of F0,with the following time and space complexi-ties(n is the size of the universe of the items):(1)The amortized processing time per interval isO(log1δlog n).(2)The workspace used is O(12log1δlog n)bits.Our algorithm improves upon a pre-vious algorithm by Bar-Yossef,Kumar and Sivakumar[Proceedings of the13th ACM–SIAM Sympo-sium on Discrete Algorithms(SODA),2002,pp.623–632],which requires O(15log1δlog5n)process-ing time per item.This algorithm can also be used to compute the max-dominance norm of a stream of multiple signals and significantly improves upon the previous best time and space bounds by Cor-mode and Muthukrishnan[Proceedings of the11th European Symposium on Algorithms(ESA),Lec-ture Notes in Comput.Sci.2938,Springer,Berlin,2003,pp.148–160].This algorithm also provides an efficient solution to the distinct summation problem,which arises during data aggregation in sensor networks[Proceedings of the2nd International Conference on Embedded Networked Sensor Systems,ACM Press,New York,2004,pp.250–262,Proceedings of the20th International Conference on Data Engineering(ICDE),2004,pp.449–460].Key words.data streams,range efficiency,distinct elements,reductions,sensor networks AMS subject classifications.68W20,68W25,68W40,68P15DOI.10.1137/0506436721.Introduction.One of the most significant successes of research on data stream processing has been the efficient estimation of the frequency moments of a stream in one-pass using limited space and time per item.An especially important aggregate is the number of distinct elements in a stream,which is often referred to as the zeroth frequency moment and denoted by F0.The counting of distinct elements arises in numerous applications involving a stream.For example,most database query optimization algorithms need an estimate of F0of the data set[HNSS95].In network traffic monitoring,this can be used to compute the number of distinct web pages re-quested from a web site or the number of distinct source addresses among all Internet protocol(IP)packets passing through a router.Further,the computation of many other aggregates of a data stream can be reduced to the computation of F0.In most data stream algorithms in the literature the following model is studied: Each element in the stream is a single item(which can be represented by an integer), and the algorithm needs to process this item“efficiently,”both with respect to time and space;i.e.,the time taken to process each element should be small,and the total ∗Received by the editors October27,2005;accepted for publication(in revised form)November 13,2006;published electronically May16,2007.A preliminary version of this article appeared in Proceedings of the21st International Conference on Data Engineering(ICDE),IEEE Computer Society,2005./journals/sicomp/37-2/64367.html†Department of Computer Science,Iowa State University,Ames,IA50010(pavan@). This author’s research was supported in part by NSF grant CCF-0430807.‡Department of Electrical and Computer Engineering,Iowa State University,Ames,IA50010 (snt@).This author’s research was supported in part by NSF grant CNS-0520102.359360 A.PAVAN AND SRIKANTA TIRTHAPURAworkspace used should be small.Many algorithms have been designed in this model to estimate frequency moments [AMS99,GT01,BYJK +02,CK04]and other aggre-gates [FKSV02,DGIM02,CDIM02,AJKS02,Mut05,BBD +02]of massive data sets.However,in many cases it is advantageous to design algorithms which work on a more general data stream,where each element of the stream is not a single item but a list of items .In a stream of integers,this list often takes the form of an interval of integers.To motivate this requirement for processing intervals of integers,we give some examples below.Bar-Yossef,Kumar,and Sivakumar [BYKS02]formalize the concept of reductions between data stream problems and demonstrate that,in many cases,reductions natu-rally lead to the need for processing a list of items quickly,much faster than processing them one by one.They consider the problem of estimating the number of triangles in a graph G ,where the edges of G arrive as a stream in an arbitrary order.They present an algorithm that uses a reduction from the problem of computing the number of triangles in a graph to the problem of computing the zeroth and second frequency moments (denoted F 0and F 2,respectively)of a stream of integers.However,for each edge e in the stream,the reduction produces a list of integers,and the size of each such list could be as large as n ,the number of vertices in the graph.If one used an algorithm for F 0or F 2which processed these integers one by one,the processing time per edge would be Ω(n ),which is prohibitive.Thus,this reduction needs an algorithm for computing F 0and F 2which can handle a list of integers efficiently,and such an algorithm is called list-efficient .In many cases,including the above,these lists are simply intervals of integers,and an algorithm which can handle such intervals efficiently is called range-efficient .Another application of such list-efficient and range-efficient algorithms is in aggre-gate computation over sensor networks [NGSA04,CLKB04].The goal here is to com-pute the sum (or average)of a stream of sensor observations.However,due to multi-path routing of data,the same observation could be repeated multiple times at a node,and the sum should be computed over only distinct elements of the stream.This leads to the distinct summation problem defined below,which was studied by Considine et al.[CLKB04]and Nath et al.[NGSA04].Given a multiset of items M ={x 1,x 2,...},where x i =(k i ,c i ),compute distinct (k i ,c i )∈M c i .The distinct summation problem can be reduced to F 0computation as follows:For each x i =(k i ,c i ),generate a list l i of c i distinct but consecutive integers such that for distinct x i ’s the lists l i do not overlap,but if element x i reappeared,the same list l i would be generated.An F 0algorithm on this stream of l i ’s will give the distinct summation required,but the algorithm should be able to efficiently process a list of elements.Each list l i is a range of integers,so this needs a range-efficient algorithm for F 0.Yet another application of range-efficient algorithms for F 0is in computing the max-dominance norm of multiple data streams.The concept of the max-dominance norm is useful in financial applications [CM03]and IP network monitoring [CM03].The max-dominance norm problem is as follows:Given k streams of m integers each,leta i,j ,i =1,2,...,k,j =1,2,...,m ,represent the j th element of the i th stream.The max-dominance norm is defined as m j =1max 1≤i ≤k a i,j .Assume for all a i,j ,1≤a i,j ≤n .In section 5we show that the computation of the max-dominance norm can be reduced to range-efficient F 0and derive efficient algorithms using this reduction.The above examples illustrate that range-efficient computation of F 0is a funda-mental problem,useful in diverse scenarios involving data streams.In this paper,weDISTINCT ELEMENTS IN DATA STREAMS 361present a novel algorithm for range-efficient computation of F 0of a data stream that provides the current best time and space bounds.It is well known [AMS99]that exact computation of the F 0of a data stream requires space linear in the size of the input in the worst case.In fact,even deterministically approximating F 0using sublinear space is impossible.For processing massive data streams,it is clearly infeasible to use space linear in the input size.Thus,we focus on designing randomized approximation schemes for range-efficient computation of F 0.Definition 1.For parameters 0< <1and 0<δ<1,an ( ,δ)-estimator for a number Y is a random variable X such that Pr[|X −Y |> Y ]<δ.1.1.Our results.We consider the problem of range-efficient computation of F 0,defined as follows.The input stream is R =r 1,r 2,...,r m ,where each stream element r i =[x i ,y i ]⊂[1,n ]is an interval of integers x i ,x i +1,...,y i .The length of an interval r i could be any number between 1and n .Two parameters,0< <1and 0<δ<1,are supplied by the user.The algorithm is allowed to view each element of the stream only once and has a limited workspace.It is required to process each item quickly.Whenever the user desires,it is required to output the F 0of the input stream,i.e.,the total number of distinct integers contained in all the intervals in the input stream R .For example,if the input stream was [1,10],[2,5],[5,12],[41,50],then F 0=|[1,12]∪[41,50]|=22.We present an algorithm with the following time and space complexities:•the amortized processing time per interval is O (log 1δlog n );•the time to answer a query for F 0at anytime is O (1);•the workspace used is O (1 2log 1δlog n )bits.Prior to this work,the most efficient algorithm for range-efficient F 0computation was by Bar-Yossef,Kumar,and Sivakumar [BYKS02]and took O (1 5log 1δlog 5n )processing time per interval and O (1 3log 1δlog n )space.Our algorithm performs significantly better with respect to time and better with respect to space.Extensions to the basic algorithm.The basic algorithm for range-efficient F 0can be extended to the following more general scenarios:•It can process a list of integers that is in an arithmetic progression as efficiently as it can handle an interval of integers.•The algorithm can also be used in the distributed streams model,where the input stream is split across multiple parties and F 0has to be computed on the union of the streams observed by all the parties.•If the input consists of multidimensional ranges,then the algorithm can be made range-efficient in every coordinate [BYKS02].Our algorithm is based on random sampling.The set of distinct elements in the stream is sampled at an appropriate probability,and this sample is used to determine the number of distinct elements.The random sampling algorithm is based on the algorithm of Gibbons and Tirthapura [GT01]for counting the number of distinct elements in a stream of integers;however,their algorithm [GT01]was not range-efficient.A key technical ingredient in our algorithm is a novel range sampling algorithm,which can quickly determine the size of a sample resulting from an interval of ing this range sampling algorithm,an interval of integers can be processed by the algorithm much faster than processing them one by one,and this ultimately leads to a faster range-efficient F 0algorithm.1.2.Applications.As a result of the improved algorithm for range-efficient F 0,we obtain improved space and time bounds for dominance norms and distinct362A.PAVAN AND SRIKANTA TIRTHAPURAsummation.%newpage Dominance ing a reduction to range-efficient F 0,which is elaborated on in section 5,we derive an ( ,δ)-approximation algorithm for the max-dominance norm with the following performance guarantees:•the workspace in bits is O ((log m +log n )1 2log 1δ);•the amortized processing time per item is O (log a log 1δ),where a is the valueof the item being processed;•the worst-case processing time per item is O ((log log n log a )1 2log 1δ).In prior work,Cormode and Muthukrishnan [CM03]gave an ( ,δ)-approxi-mation algorithm for the max-dominance norm.Their algorithm uses space O ((log n +l 1 log m log log m )1 2log 1δ)and spends O ((log a log m )1 4log 1δ)time to pro-cess each item,where a is the value of the current element.Our algorithm performs better spacewise and significantly better timewise.Distinct summation and data aggregation in sensor networks.Our al-gorithm also provides improved space and time bounds for the distinct summation problem,through a reduction to the range-efficient F 0problem.Given a multiset of items M ={x 1,x 2,...},where each x i is a tuple (k i ,c i ),where k i ∈[1,m ]is the “type”and c i ∈[1,n ]is the “value.”The goal is to compute S = distinct (k i ,c i )∈M c i .In distinct summation,it is assumed that a particular type is always associated with the same value.However,the same (type,value)pair can appear multiple times in the stream.This reflects the setup used in sensor data aggregation,where each sensor observation has an identifier and a value,and the same observation may be trans-mitted to the sensor data “sink”through multiple paths.The sink should compute aggregates such as the sum and average over only distinct observations.We provide an ( ,δ)-approximation for the distinct summation problem with the following guarantees:•the amortized processing time per item is O (log 1δlog n );•the time to answer a query is O (1);•the workspace used is O ((log m +log n )1 2log 1δ)bits.Considine et al.[CLKB04]and Nath et al.[NGSA04]present alternative algo-rithms for the distinct summation problem.Their algorithms are based on the al-gorithm of Flajolet and Martin [FM85]and assume the presence of an ideal hash function,which produces completely independent random outputs on different in-puts.However,their analysis does not consider the space required to store such a hash function.Moreover,it is known that hash functions that generate completely independent random values on different inputs cannot be stored in limited space.Our algorithm does not make an assumption about the availability of ideal hash functions.Instead,we use a hash function which can be stored using limited space but generates only pairwise-independent random numbers.We note that Alon,Ma-tias,and Szegedy [AMS99]provide a way to replace the hash functions used in the Flajolet–Martin algorithm [FM85]by pairwise-independent hash functions.However,the F 0algorithm by Alon,Matias,and Szegedy [AMS99]is not range-efficient.If the hash functions introduced by Alon,Matias,and Szegedy [AMS99]were used in the algorithms in [CLKB04,NGSA04],there still is a need for a range sampling function similar to the one we present in this paper.Counting triangles in a data stream.As described above,the problem of counting triangles in a graph that arrives as a stream of edges can be reduced to range-efficient computation of F 0and F 2on a stream of integers.Our range-efficientDISTINCT ELEMENTS IN DATA STREAMS363 F0algorithm provides a faster solution to one of the components of the problem. However,due to the high complexity of the range-efficient algorithm for F2,this is not sufficient to obtain an overall reduction in the runtime of the algorithm for counting triangles in graphs.There is recent progress on the problem of counting triangles by Buriol et al.[BFL+06](see also Jowhari and Ghodsi[JG05]),who give an algorithm through direct random sampling,without using the reduction to F0and F2.The algorithm by Buriol et al.is currently the most efficient solution to this problem and has a space complexity proportional to the ratio between the number of length2paths in the graph and the number of triangles in the graph and an expected update time O(log|V|·(1+s·|V|/|E|)),where s is the space requirement and V andE are the vertices and the edges of the graph,respectively.1.3.Related work.Estimating F0of a data stream is a very well studied prob-lem,because of its importance in various database and networking applications.It is well known that computing F0exactly requires space linear in the number of distinct values,in the worst case.Flajolet and Martin[FM85]gave a randomized algorithm for estimating F0using O(log n)bits.This assumed the existence of certain ideal hash functions,which we do not know how to store in small space.Alon,Matias,and Szegedy[AMS99]describe a simple algorithm for estimating F0to within a constant relative error which worked with pairwise-independent hash functions and also gave many important algorithms for estimating other frequency moments F k,k>1of a data set.Gibbons and Tirthapura[GT01]and Bar-Yossef et al.[BYJK+02]present algo-rithms for estimating F0to within arbitrary relative error.Neither of these algo-rithms is range-efficient.The algorithm by Gibbons and Tirthapura used randomsampling;its space complexity was O(12log1δlog n),and processing time per elementwas O(log1δ).The space complexity of this algorithm was improved by Bar-Yossefet al.[BYJK+02],who gave an algorithm with better space bounds,which are theorder of the sum of O(log n)and poly(1)terms rather than their product,but at thecost of increased processing time per element.Indyk and Woodruff[IW03]present a lower bound ofΩ(log n+12)for the spacecomplexity of approximating F0,thus narrowing the gap between known upper and lower bounds for the space complexity of F0.Since the F0problem is a special case of the range-efficient F0problem,these lower bounds apply to range-efficient F0,too.Thus our space bounds of O((log m+log n)12log1δ)compare favorably with thislower bound,showing that our algorithms are near optimal spacewise.However an important metric for range-efficient F0is the processing time per item,for which no useful lower bounds are known.The work of Feigenbaum et al.[FKSV02]on estimating the L1-difference be-tween streams also has the idea of reductions between data stream algorithms and uses a range-efficient algorithm in their reduction.Bar-Yossef,Kumar,and Sivaku-mar[BYKS02]mention that their notion of reductions and list-efficiency were in-spired by the above-mentioned work of Feigenbaum et al.The algorithm for the L1-difference[FKSV02]is not based on random sampling but relies on quickly sum-ming many random variables.They develop limited independence random variables which are range summable,while our sampling-based algorithm makes use of a range sampling technique.Further work on range summable random variables includes Gilbert et al.[GKMS03],Reingold and Naor(for a description see[GGI+02]),and Calderbank et al.[CGL+05].There is much other interesting work on data stream algorithms.For an overview,364 A.PAVAN AND SRIKANTA TIRTHAPURAthe reader is referred to the excellent surveys in[Mut05,BBD+02].Organization of this paper.The rest of the paper is organized as follows: Section2gives a high level overview of the algorithm for range-efficient F0.Section3 gives the range sampling algorithm,its proof,and analysis of complexity.Section4 presents the algorithm for computing F0using the range sampling algorithm as the subroutine.Section5gives extensions of the basic algorithm to distributed streams and the computation of dominance norms.2.A high level overview.Our algorithm is based on random sampling.A random sample of the distinct elements of the data stream is maintained at an appro-priate probability,and this sample is used infinally estimating the number of distinct elements in the stream.A key technical ingredient is a novel range sampling algo-rithm,which quickly computes the number of integers in a range[x,y]which belong to the current random sample.The other technique needed to make random sampling work here is adaptive sampling(see Gibbons and Tirthapura[GT01]),where the sam-pling probability is decreased every time the sample overflows.The range sampling algorithm,when combined with adaptive random sampling,yields the algorithm for range-efficient estimation of F0.2.1.A random sampling algorithm for F0.At a high level,our random sampling algorithm for computing F0follows a similar structure to the algorithm by Gibbons and Tirthapura[GT01].Wefirst recall the main idea of their random sampling algorithm.The algorithm keeps a random sample of all the distinct elements seen so far.It samples each element of the stream with a probability P,while making sure that the sample has no duplicates.Finally,when an estimate is asked for F0,it returns the size of the sample,multiplied by1/P.However,the value of P cannot be decided in advance.If P is large,then the sample might become too big if F0is large.On the other hand,if P is too small,then the approximation error might be very high if the value of F0was small.Thus,the algorithm starts offwith a high value of P=1and decreases the value of P every time the sample size exceeds a predetermined maximum sample size,α.The algorithm maintains a current sampling level which determines a sampling probability P .The sampling probability P decreases as level increases.Initially, =0,and never decreases.The sample at level ,denoted S( ),is determined by a hash function S(·, )for =0,1,..., max,where max is some maximum level to be specified later.When item x is presented to the algorithm,if S(x, )=1,then x is stored in the sample S( ),and it is not stored otherwise.Each time the size of S( )exceedsα(i.e.,an overflow occurs),the current sampling level is incremented,and an element x∈S( )is placed in S( +1)only if S(x, +1)=1.We will always ensure that if S(x, +1)=1,then S(x, )=1.Thus S( +1)is a subsample of S( ).Finally, anytime an estimate of the number of distinct elements is asked for,the algorithmreturns|S( )|P ,where is the current sampling level.We call the algorithm describedso far as the single-item algorithm.2.2.Range efficiency.When each element of the stream is an interval of items rather than a single item,the main technical problem is to quicklyfigure out how many points in this interval belong in the sample.More precisely,if is the current sampling level and an interval r i=[x i,y i]arrives,it is necessary to know the size of the set{x∈r i|S(x, )=1}.We call this the range sampling problem.A naive solution to range sampling is to consider each element x∈r i individuallyDISTINCT ELEMENTS IN DATA STREAMS365 and check if S(x, )=1,but the processing time per item would beΘ(y i−x i),which could be as much asΘ(n).We show how to reduce the time per interval significantly, to O(log(y i−x i))operations.The range-efficient algorithm simulates the single-item algorithm as follows:When an interval r i=[x i,y i]arrives,the size of the set{x∈r i|S(x, )=1}is computed. If the size is greater than zero,then r i is added to the sample;otherwise,r i is dis-carded.If the number of intervals in the sample becomes too large,then the sampling probability is decreased by increasing the sampling level from to +1.When such an increase in sampling level occurs,every interval r in the sample such that {x∈r|S(x, +1)=1}is empty is discarded.Finally,when an estimate for F0is asked for,suppose the current sample is the set of intervals S={r1,...,r k}and is the current sampling level.The algorithm returns|T|/P ,where T={x∈r i|r i∈S,S(x, )=1}.2.2.1.Hash functions.Since the choice of the hash function is crucial for the range sampling algorithm,wefirst precisely define the hash functions used for sampling.We use a standard2-universal family of hash functions[CW79].First choose a prime number p between10n and20n,and then choose two numbers a,b at random from{0,...,p−1}.Define hash function h:{1,...,n}→{0,...,p−1}as h(x)=(a·x+b)mod p.The two properties of h that are important to the F0algorithm are as follows:1.For any x∈{1,...,n},h(x)is uniformly distributed in{0,...,p−1}.2.The mapping is pairwise independent.For x1=x2and y1,y2∈{0,...,p−1},Pr[(h(x1)=y1)∧(h(x2)=y2)]=Pr[h(x1)=y1]·Pr[h(x2)=y2].For each level =0,..., log n ,we define the following:•Region R ⊂{0,...,p−1}:R =0,...,p2−1.•For every x∈[1,n],the sampling function S(x, )is defined asS(x, )=1if h(x)∈R and S(x, )=0otherwise.•The sampling probability at level is denoted by P .For all x1,x2∈[1,n], Pr[S(x1, )=1]=Pr[S(x2, )=1],and we denote this probability by P .Since h(x)is uniformly distributed in{0,...,p−1},we have P =|R |/p.2.2.2.Range sampling.During range sampling,the computation of the num-ber{x∈r i|S(x, )=1}reduces to the following problem:Given numbers a,b,and p such that0≤a,b<p,function f:[1,n]→[0,p−1]is defined as f(x)=(a·x+b) mod p.Given intervals[x1,x2]⊂[1,n]and[0,q]⊂[0,p−1],quickly compute the size of the set{x∈[x1,x2]|f(x)∈[0,q]}.Note that n could be a large number,since it is the size of the universe of integers in the stream.We present an efficient solution to the above problem.Our solution is a recursive procedure which works by reducing the above problem to another range sampling problem but over a significantly smaller range,whose length is less than half the length of the original range[x1,x2].Proceeding thus,we get an algorithm whose time complexity is O(log(x2−x1)).Our range sampling algorithm might be of independent interest and may be useful in the design of other range-efficient algorithms for the data stream model.The algorithms for computing F0found in[AMS99,GT01,BYJK+02]use hash functions defined over GF(2m),which are different from the ones that we use.Bar-366 A.PAVAN AND SRIKANTA TIRTHAPURAYossef et al.[BYKS02],in their range-efficient F 0algorithm,use the Toeplitz family of hash functions [Gol97],and their algorithm uses specific properties of those hash functions.3.Range sampling algorithm.In this section,we present a solution to the range sampling problem,its correctness,and time and space complexities.The al-gorithm RangeSample (r i =[x i ,y i ], )computes the number of points x ∈[x i ,y i ]for which h (x )∈R ,where h (x )=(ax +b )mod p .This algorithm is later used as a subroutine by the range-efficient algorithm for F 0,which is presented in the next section:RangeSample ([x i ,y i ], )= x ∈[x i ,y i ]|h (x )∈ 0, p 2−1 .Note that the sequence h (x i ),h (x i +1),...,h (y i )is an arithmetic progression over Z p (Z k is the ring of integers modulo k )with a common difference a .Thus,we consider the following general problem.Problem 1.Let M >0,0≤d <M ,and 0<L ≤M .Let S = u =x 1,x 2,...,x n be an arithmetic progression over Z M with common difference d ,i.e,x i =(x i −1+d )mod M .Let R be a region of the form [0,L −1]or [−L +1,0].Compute |S ∩R |.We first informally describe the idea behind our algorithm for Problem 1and then go on to the formal description.For this informal description,we consider only the case R =[0,L −1].We first define a total order among the elements of Z m .Observe that an element of Z m has infinitely many representations.For example,if x ∈Z m ,then x,x +m,x +2m,...are possible representations of x .For x ∈Z m ,the standard representation of x is the smallest nonnegative integer std (x )such that x ≡std (x )in Z m .If x and y are two elements of Z m ,then we say x <y if std (x )is less than std (y )in the normal ordering of integers.We say x >y if std (x )=std (y )and x <y .In the rest of the paper,we use the standard representation for elements over Z m .Given an element x ∈Z m ,we define −x as m −x .3.1.Intuition.Divide S into subsequences S 0,S 1,...,S k ,S k +1as follows:S 0= x 1,x 2,...,x i ,where i is the smallest natural number such that x i >x i +1.The subsequences S j ,j >0are defined inductively.If S j −1= x t ,x t +1,...,x m ,then S j = x m +1,x m +2,...,x r ,where r is the smallest number such that r >m +1and x r >x r +1;if no such r exists,then x r =x n .Note that if S j = x t ,x t +1,...,x m then x t <d and x t ,x t +1,...,x m are in ascending order.Let f j denote the first element of S j ,and let e j denote the last element of S j .We treat the computation of |S 0∩R |and |S k +1∩R |as special cases and now focus on the the computation of |S i ∩R |for 1≤i ≤k .Let L =d ×q +r ,where r <d .Note that the values of q and r are unique.We observe that,for each i ∈[1,k ],it is easy to compute the number of points of S i that lie in R .More precisely we make the following observation.Observation 1.For every i ∈[1,k ],if f i <r ,then |S i ∩R |= L d +1,else |S i ∩R |= L d .Thus Problem 1is reduced to computing the size of the following set:{i |1≤i ≤k,f i ∈[0,r −1]}.The following observation is critical.。