Biographical Data Yuh-Jye Lee
未来舌尖上的幸福

图文 / 王治钧 审核 / 毕坤(中国农学会)细胞农业科学家伊莎·达塔(Isha Datar)提出“细胞农业”这一概念,并在TED 大会上展示了她在实验室“种植”的肉类。
吃肉,却不吃动物细胞农业指的是在实验室中用细胞培养物培育农产品。
2013年,一个成本为33万美元的牛肉汉堡,让细胞农业一词进入大众的视野。
这个汉堡中夹着的是世界上第一块在实验室中用细胞培养出来的牛肉饼。
细胞农业科学家伊莎·达塔(Isha Datar)未来舌尖上的幸福202252SEP.Copyright ©博看网. All Rights Reserved.53(责任编辑 / 高琳 张佳鑫 美术编辑 / 周游)俗话说“民以食为天”,到本世纪末,地球上的人口有可能达到110亿。
要保证这么多人吃饱饭并有足够的营养,种植业和畜牧业都需要更加高效的科技革新技术。
人造肉成了未来食物创新发展的大趋势。
人造肉按照原材料分为两种:植物肉和动物细胞培育肉。
植物肉是利用植物蛋白,通过技术生产加工成类似动物肉的口感、味道和外观的食品;动物细胞培育肉是在特定的培养条件下,利用动物肌肉细胞中的多能干细胞(一种具有自我更新和自我复制能力的干细胞)培养出来的具有传统肉类结构、风味口感的人造肉。
2020年,新加坡批准了动物细胞培育肉上市。
一些让食客大快朵颐的鸡肉,并不是通过宰杀鸡得来的,而是在实验室中用细胞培养物培育出来的。
未来饭碗里的高科技动物细胞培育肉的生产过程是怎样的呢?以牛肉为例,先从牛身上提取所需的细胞,然后放入实验室的生物反应器内培养,最终细胞发育成含有肌肉纤维、血管与胞外基质的肌肉组织。
动物细胞培育肉在满足人们口腹之欲的同时,减少了畜牧业给环境和气候带来的影响。
除了对环境友好外,动物细胞培育肉还可以减少动物性病毒的传播、降低对抗生素的使用,从而体现对健康食物的追求。
如今,动物细胞培育肉成为很多国家的发展重点。
中国在《“十四五”生物经济发展规划》中提到,有序发展全基因组选择、系统生物学、合成生物学、人工智能等生物育种技术,发展合成生物学技术,探索研发“人造蛋白”等新型食品。
西红花提取物调控免疫细胞,提高程序性死亡受体-1抑制剂治疗肺腺癌效果的实验研究
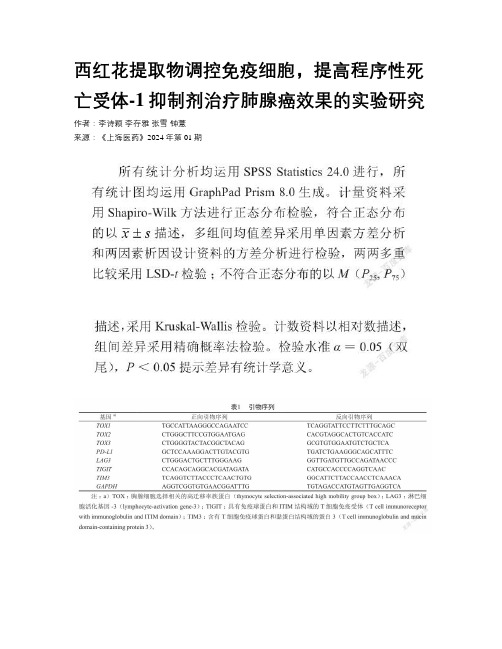
西红花提取物调控免疫细胞,提高程序性死亡受体-1抑制剂治疗肺腺癌效果的实验研究作者:李诗颖李存雅张雪钟薏来源:《上海医药》2024年第01期摘要目的:多项研究提示,西红花提取物能影响肿瘤的发展进程。
本实验探究西红花提取物在肺腺癌小鼠模型中对肿瘤免疫微环境和免疫治疗的影响,为西红花提取物抗肿瘤研究提供更多基础性数据。
方法:构建Lewis肺癌细胞和萤光素酶稳定结合的小鼠皮下瘤模型,观察西红花提取物对小鼠皮下瘤和肿瘤免疫微环境的影响:运用活体成像技术跟踪肿瘤生长情况;运用流式细胞技术检测小鼠CD4+、CD8+ T细胞的数量及占比;运用反转录-聚合酶链式反应技术检测程序性死亡受体配体1、含有T细胞免疫球蛋白和黏蛋白结构域的蛋白3(T cell immunoglobulin and mucin domaincontaining protein 3, TIM3)、淋巴细胞活化基因-3(lymphocyte-activation gene-3, LAG3)、具有免疫球蛋白和ITIM结构域的T细胞免疫受体(T cell immunoreceptor with immunoglobulin and ITIM domain, TIGIT)、胸腺细胞选择相关的高迁移率族蛋白(thymocyte selection-associated high mobility group box, TOX)1、TOX2、TOX3基因的mRNA表达情况。
结果:与对照组相比,给予西红花提取物能一定程度地抑制小鼠皮下瘤的生长(P关键词西红花免疫微环境肺腺癌免疫治疗中图分类号:R965; R282.71 文献标志码:A 文章编号:1006-1533(2024)01-0003-09引用本文李诗颖,李存雅,张雪,等. 西红花提取物调控免疫细胞,提高程序性死亡受体-1抑制剂治疗肺腺癌效果的实验研究[J]. 上海医药, 2024, 45(1): 3-11; 28.基金项目:上海市2022年度“科技创新行动计划”医学创新研究专项项目(22Y31920104);上海市虹口区第二轮“国医强优”三年行动计划(2022—2024年)中西医结合重点专科、薄弱专科建设项目(HKGYQYXM-2022-10);上海市2021年度“科技创新行动计划”扬帆计划项目(21YF444400);上海市2022年度“科技创新行动计划”启明星培育(扬帆专项)项目(22YF1444900);山东省乡村振兴基金会张秀兰慈善基金项目Experimental study of saffron extracts to modulate immune cells to improve the efficacy of a programmed death-1 inhibitor in the treatment of lung adenocarcinomaLI Shiying1, LI Cunya1, ZHANG Xue2, ZHONG Yi1(1. Department of Oncology, Shanghai TCM-Integrated Hospital, Shanghai University of Traditional Chinese Medicine, Shanghai 200082, China; 2. Shanghai Traditional Chinese Medicine Co., Ltd., Shanghai 200082, China)ABSTRACT Objective: A number of studies have shown that saffron extracts can affect the development of tumor. This study explored the effect of saffron extract on tumor immune microenvironment and immunotherapy in a mouse model of lung adenocarcinoma so as to provide more basic data for the anti-tumor research of saffron extracts. Methods: The transplanted tumor model of Lewis lung carcinoma-luciferase in mice was established to detect the effect of saffron extracts on the transplanted tumor in vivo. At the same time, the tumor growth was tracked by in vivo imaging technique. The number and proportion of CD4+ and CD8+ T cells were determined by flow cytometry. The mRNA levels of programmed death-ligand 1, T cell immunoglobulin and mucin domain-containing protein 3 (TIM3), lymphocyte-activation gene-3 (LAG3), T cell immunoreceptor with immunoglobulin and ITIM domain (TIGIT), thymocyte selection-associated high mobility group box (TOX) 1, TOX2 and TOX3 were detected by reverse transcription-polymerase chain reaction (RT-PCR) and immunohistochemical techniques to verify the effect of saffron extracts on the regulation of tumor immune microenvironment. Results:Compared with the control group, the administration of saffron extracts could inhibit the growth of subcutaneous tumor in mice to a certain extent, and the number and proportion of CD4+ and CD8+ T cells were increased (PKEY WORDS saffron; immune microenvironment; lung adenocarcinoma; immunotherapy肿瘤是一类恶性疾病,2018年全球肿瘤死亡病例数达约960万人,较2008年增加26.3%,其中男性肿瘤死亡病例数增加最多的是肺癌,增加了23.4万人[1-2]。
马来西亚留学生物科技专业的最新介绍
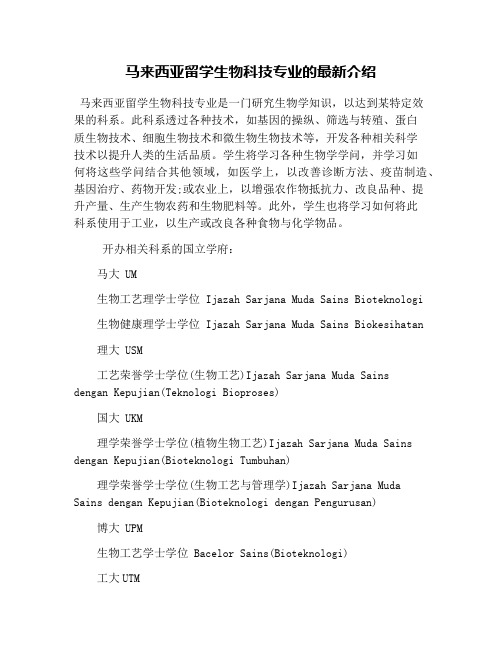
马来西亚留学生物科技专业的最新介绍马来西亚留学生物科技专业是一门研究生物学知识,以达到某特定效果的科系。
此科系透过各种技术,如基因的操纵、筛选与转殖、蛋白质生物技术、细胞生物技术和微生物生物技术等,开发各种相关科学技术以提升人类的生活品质。
学生将学习各种生物学学问,并学习如何将这些学问结合其他领域,如医学上,以改善诊断方法、疫苗制造、基因治疗、药物开发;或农业上,以增强农作物抵抗力、改良品种、提升产量、生产生物农药和生物肥料等。
此外,学生也将学习如何将此科系使用于工业,以生产或改良各种食物与化学物品。
开办相关科系的国立学府:马大 UM生物工艺理学士学位 Ijazah Sarjana Muda Sains Bioteknologi生物健康理学士学位 Ijazah Sarjana Muda Sains Biokesihatan理大 USM工艺荣誉学士学位(生物工艺)Ijazah Sarjana Muda Sainsdengan Kepujian(Teknologi Bioproses)国大 UKM理学荣誉学士学位(植物生物工艺)Ijazah Sarjana Muda Sains dengan Kepujian(Bioteknologi Tumbuhan)理学荣誉学士学位(生物工艺与管理学)Ijazah Sarjana Muda Sains dengan Kepujian(Bioteknologi dengan Pengurusan)博大 UPM生物工艺学士学位 Bacelor Sains(Bioteknologi)工大UTM工业生物学士学位 Ijazah Sarjana Muda Sains(Biologi Industri)砂大 UNIMAS科学荣誉学士学位(生物工艺)Sarjana Muda Sains Dengan Kepujian(Bioteknologi Sumber)沙大UMS科学荣誉学士学位(生物工艺)Sarjana Muda Sains dengan Kepujian(Bioteknologi)达鲁依曼大学UDM理学荣誉学士学位(农业生物工艺)Ijazah Sarjana Muda Sains Bioteknologi Pertanian dengan Kepujian丹大UMK应用科学学士学位(生物工业工艺)Sarjana Muda Sains Gunaan(Teknologi Bioindustri)开办相关科系的私立学府:The University of Nottingham Malaysia Campus 诺丁汉大学马来西亚分校Universiti Tunku Abdul Rahman 拉曼大学AIMST UniversityINTI International Education Group 英迪国际教育集团。
迪肯大学生物技术专业好不好
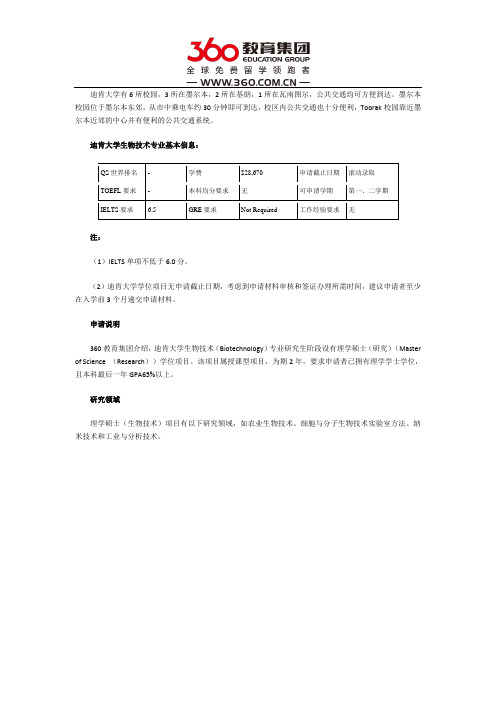
迪肯大学有6所校园,3所在墨尔本,2所在基朗,1所在瓦南图尔,公共交通均可方便到达。
墨尔本校园位于墨尔本东郊,从市中乘电车约30分钟即可到达,校区内公共交通也十分便利,Toorak校园靠近墨尔本近郊的中心并有便利的公共交通系统。
迪肯大学生物技术专业基本信息:
注:
(1)IELTS单项不低于6.0分。
(2)迪肯大学学位项目无申请截止日期,考虑到申请材料审核和签证办理所需时间,建议申请者至少在入学前3个月递交申请材料。
申请说明
360教育集团介绍,迪肯大学生物技术(Biotechnology)专业研究生阶段设有理学硕士(研究)(Master of Science (Research))学位项目。
该项目属授课型项目,为期2年,要求申请者已拥有理学学士学位,且本科最后一年GPA65%以上。
研究领域
理学硕士(生物技术)项目有以下研究领域,如农业生物技术、细胞与分子生物技术实验室方法、纳米技术和工业与分析技术。
统计学习工具箱KStattoolbox使用说明
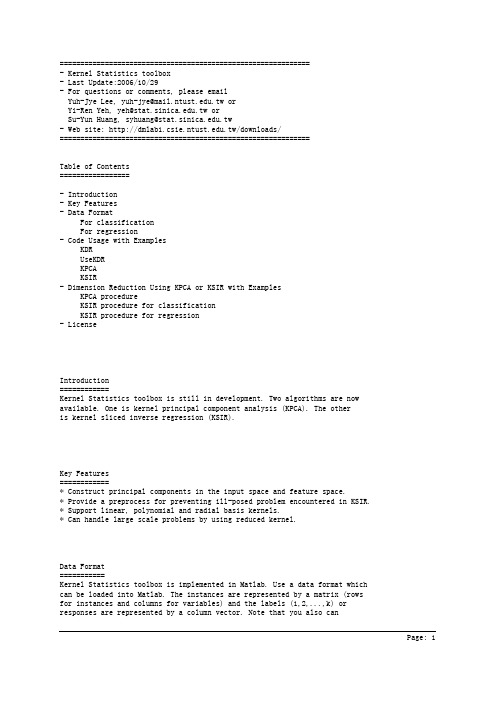
=============================================================- Kernel Statistics toolbox- Last Update:2006/10/29- For questions or comments, please emailYuh-Jye Lee, yuh-jye@.tw orYi-Ren Yeh, yeh@.tw orSu-Yun Huang, syhuang@.tw- Web site: .tw/downloads/=============================================================Table of Contents=================- Introduction- Key Features- Data FormatFor classificationFor regression- Code Usage with ExamplesKDRUseKDRKPCAKSIR- Dimension Reduction Using KPCA or KSIR with ExamplesKPCA procedureKSIR procedure for classificationKSIR procedure for regression- LicenseIntroduction============Kernel Statistics toolbox is still in development. Two algorithms are now available. One is kernel principal component analysis (KPCA). The otheris kernel sliced inverse regression (KSIR).Key Features============* Construct principal components in the input space and feature space.* Provide a preprocess for preventing ill-posed problem encountered in KSIR. * Support linear, polynomial and radial basis kernels.* Can handle large scale problems by using reduced kernel.Data Format===========Kernel Statistics toolbox is implemented in Matlab. Use a data format which can be loaded into Matlab. The instances are represented by a matrix (rows for instances and columns for variables) and the labels (1,2,...,k) or responses are represented by a column vector. Note that you also canrepresent the labels of binary classification by 1 or -1.For classification-------------------instances| 10 -5 0.8 | => inst 1| 15 -6 0.2 | => inst 2| . | .| . | .| . | .| 21 1 -0.1 | => inst nlabels| 1 | => label of inst 1 => class 1| 5 | => label of inst 2 => class 5| . | .| . | .| . | .| 10 | => label of inst n => class 10For regression---------------instances| 11 -5 0.2 | => inst 1| 14 -7 0.8 | => inst 2| . | .| . | .| . | .| 20 2 -0.9 | => inst nlabels| 3.2 | => response of inst 1| 1.7 | => response of inst 2| . | .| . | .| . | .| -1.1 | => response of inst nCode Usage with Examples========================Kernel Statistics toolbox contains two main functions: KDR for constructingPCs and UseKDR for using the results of KDR. Besides, users also can directlyuse the two core programs, KPCA and KSIR, for a specific kernel matrix.Description for codesKDR : the main program for constructing PCs via different methods.UseKDR : using the results of KDR to build up the projected data matrix.KPCA : finds dimension reduction directions by PCA of a kernel matrix.KSIR : finds dimension reduction directions by sliced inverse regression of a kernel matrix.Usage of KDR:>>[Info] = KDR(label, inst, 'options')---------------------------------------------------------------------*Inputs of KDR:label : training data class label or responseinst : training data inputsoptions:-s statistic method. 0-PCA, 1-SIR (default:0)-t kernel type. 0-linear, 1-polynomial, 2-radial basis (default:2)-r ratio of random subset size to the full data size (default:1)-z number of slices (default:20)If NumOfSlice >= 1, it represents NumOfSlice slices.If NumOfSlice = 0, it extracts slices according toclass labels.-p number of principal components (default:1)If NumOfPC= r >= 1, it extracts the first r leadingeigenvectors.If NumOfPC= r < 1, it extracts leading eigenvectorswhose sum of eigenvalues is greater than 100*r% ofthe total sum of eigenvalues.-g gamma in kernel function (default:0.1)-d degree of polynomial kernel (default:2)-b constant term of polynomial kernel (default:0)-m scalar factor of polynomial kernel (default:1)*Outputs of KDR:Info results of Kernel Statistics method (a structure in Matlab).PC principal components of data.EV eigenvalues respect to the principal components.Ratio.RS reduced set.Space the space of Kernel Statistics method.Params parameters specified by the user in the inputsExample: Construct ten PCs via KPCA by using Gaussian kernel>>[Info_one] = KDR([], inst, '-s 0 -t 2 -g 0.1 -p 10');Example: Construct five PCs via reduced KPCA (10%) by using Gaussian kernel>>[Info_two] = KDR([], inst, '-s 0 -t 2 -p 5 -g 0.2 -r 0.1');Example: Construct PCs of 90% eigenvalue via KPCA by using reduced polynomial kernel >>[Info_three] = KDR([], inst, '-s 0 -t 1 -p 0.9 -r 0.1 -d 2 -m 3 -b 2'); Example: Construct one PCs via KSIR by using Gaussian kernel for 2-class problems>>[Info_four] = KDR(label, inst, '-s 1 -t 2 -p 1 -z 0 -g 0.3');Example: Construct 5 PCs via KSIR (30 slices) by using Gaussian kernel for regression problems>>[Info_five] = KDR(label, inst, '-s 1 -t 2 -p 1 -z 30 -g 0.2');Usage of UseKDR:>>[ProjInst] = UseKDR(inst, Info)---------------------------------------------------------------------*Inputs of UseKDR:inst :testing data inputsInfo :results of Kernel Statistics method*Output of UseKDR:ProjInst: the projected instancesExample: Get the projected inst form Info_one>>[ProjInst_one] = UseKDR(inst, Info_one);Example: Get the projected inst form Info_four>>[ProjInst_four] = UseKDR(inst, Info_four);Usage of KPCA:>>[EigenVectors, EigenValues, ratio] = KPCA(K, NumOfPC);---------------------------------------------------------------------*Inputs of KPCAK : kernel matrix (reduced or full)NumOfPC: If NumOfPC= r >= 1, it extracts the first r leading eigenvectors.If NumOfPC= r < 1, it extracts leading eigenvectors whose sumof eigenvalues is greater than 100*r% of the total sum of eigenvalues.*Outputs of KPCAEigenValues : leading eigenvaluesEigenVectors: leading eigenvectorsratio : sum of leading eigenvalues over total sum of all eigenvalues. Example: Construct ten PCs via KPCA by using a specific kernel matrix.>>[EigenVectors, EigenValues, ratio] = KPCA(K, 10);Example: Construct PCs whose ratio to the total sum is 95%.>>[EigenVectors, EigenValues, ratio] = KPCA(K, 0.95);Usage of KSIR:>>[EigenVectors, EigenValues, ratio] = KSIR(K, y, NumOfSlice, NumOfPC)---------------------------------------------------------------------*Inputs of KSIRK : kernel matrix (reduced or full)y : class labels or responsesNumOfSlice: If numerical, it represents the number of slices.If a string 'Class', the number of slices is equal to number ofdistinct classes in y.NumOfPC : If NumOfPC >= 1, it extracts the leading NumOfPC eigenvectors.If NumOfPC < 1, it extracts leading eigenvectors whose sumof eigenvalues is greater than 100*r% of the total sum of eigenvalues. *Outputs of KISREigenValues : leading eigenvaluesEigenVectors: leading eigenvectorsratio : sum of leading eigenvalues over total sum of all eigenvalues. Example: Construct PCs via KSIR for classification by using a specific kernel matrix. >>[EigenVectors, EigenValues, ratio] = KSIR(K, y, 'CLASS');Example: Construct two PCs via KSIR for 3-class problem.>>[EigenVectors, EigenValues, ratio] = KSIR(K, y, 'CLASS', 2);Example: Construct PCs via KSIR for regression (20 slices).>>[EigenVectors, EigenValues, ratio] = KSIR(K, y, 20);Example: Construct five PCs via KSIR for regression .>>[EigenVectors, EigenValues, ratio] = KSIR(K, y, 20, 5);Example: Construct PCs whose ratio to the total sum is 95% via KSIR for regression. >>[EigenVectors, EigenValues, ratio] = KSIR(K, y, 20, 0.95);Dimension Reduction Using KPCA or KSIR with Examples===================================================KPCA procedure-------------------------*Change your current directory to Kernel Statistics toolbox folder*Load dataset Ionosphere_dataset.mat (can be found in Kernel Statistics toolbox)>>load Ionosphere_dataset.mat*Construct PCs via KPCA>>[Info] = KDR([], inst, '-s 0 -t 2 -g 0.1 -p 10');*Read the contents of Info (PCs, eigenvalues, parameters, ...etc)>>Info>>Info.PC*Get the projected inst form the PCs>>[ProjInst] = UseKDR(inst, Info);KSIR procedure for classification---------------------------------*Change your current directory to Kernel Statistics toolbox folder*Load dataset Ionosphere_dataset.mat (can be found in Kernel Statistics toolbox)>>load Ionosphere_dataset.mat*Construct PCs via KSIR (note that we extracts m-1 PCs for m-class problems)>>[Info] = KDR(label, inst, '-s 1 -t 2 -g 0.165 -z 0 -p 1');*Read the contents of Info (PCs, eigenvalues, parameters, ...etc)>>Info>>Info.PC*Get the projected inst form the PCs>>[ProjInst] = UseKDR(inst, Info);KSIR procedure for regression-----------------------------*Change your current directory to Kernel Statistics toolbox folder*Load dataset Housing_dataset.txt (can be found in Kernel Statistics toolbox)>>load Housing_dataset.txt*Split the Housing data into inst and label>>inst =Housing_dataset(:,1:13);>>label = Housing_dataset(:,14);*Construct PCs via KSIR(note that we usually use 10~30 slices and extracts 3~5 PCs for regression problems) >>[Info] = KDR(label, inst, '-s 1 -t 2 -g 0.0037 -z 20 -p 5');*Read the contents of Info (PCs, eigenvalues, parameters, ...etc)>>Info>>Info.PC*Get the projected inst form the PCs>>[ProjInst] = UseKDR(inst, Info);License=======This software is available for non-commercial use only. The authors are not responsible for implications from the use of this software.。
生态系统(概念与结构)
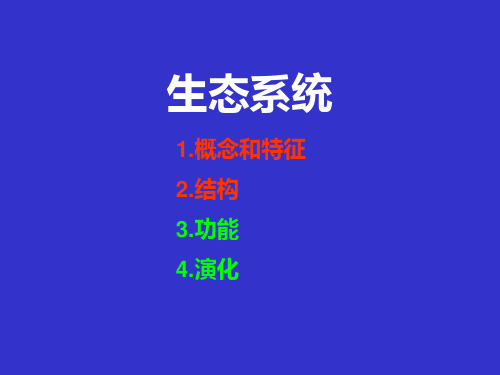
• 健康、可持续开展特性:进化的产物;与环境的 协调和对环境变化的方应;人类的作用;
研究方法: 1.系统生态学 2.模型模拟 3.实验生态学
一个以昆虫为 主的生态系统
生态系统研究的开展趋势
•根底理论研究 • 以结构和功能为根底。生态系统生产力; 自然生态系统的稳定性;生态系统管理;生态 系统的自维持模型;结合生态规划和生态设计。 •系统空间格局研究 • 生态系统、区域和全球等多层次;尺度转 换。
C.Tansley, A. G. 1935. The use and abuse of vegetational concepts and terms. Ecology, 16: 284-307
苏卡乔夫 Sukachev V. N. (1944):
地球外表的一个地段内,动物、植物、微 生物与其他地理环境组成的功能单元〔生 物地理群落,biogeocoenosis〕.
学科交叉和交叉学科: •生态经济、 •生态法学、 •生态哲学 •….等
生态系统的组成要素、结构及原理
组成要素:
生物群落 + 环境
组成要素的作用: 一、生产者〔producer〕:
自养生物:植物和光合细菌 固定能量 环境改造 能量流动和物质循环的重要环节
二、消费者〔consumer〕的主要作用: 异养生物〔heterotroph〕
•MAB (Man and the Biosphere Programme)(1971)
•IGBP (International Geosphere – Biosphere Program) (1986)
概念和特征
根本特征:
• 有时空概念的复杂的大系统: 多等级;多维; 网状;复杂性高
免疫组学的研究进展
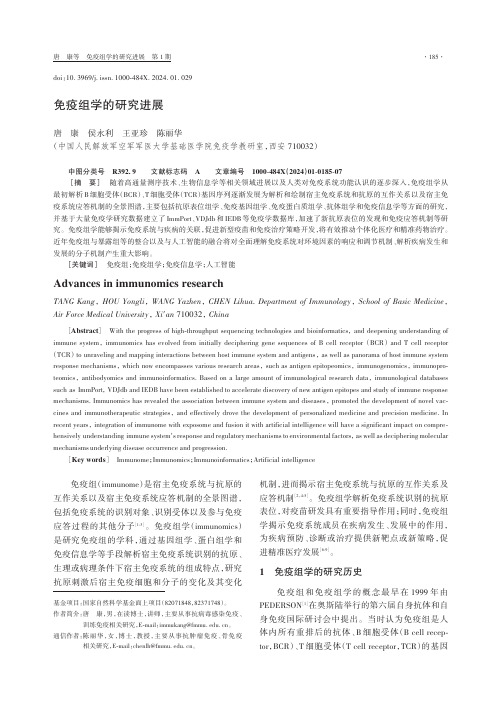
免疫组学的研究进展唐康侯永利王亚珍陈丽华(中国人民解放军空军军医大学基础医学院免疫学教研室,西安 710032)中图分类号R392.9 文献标志码 A 文章编号1000-484X(2024)01-0185-07[摘要]随着高通量测序技术、生物信息学等相关领域进展以及人类对免疫系统功能认识的逐步深入,免疫组学从最初解析B细胞受体(BCR)、T细胞受体(TCR)基因序列逐渐发展为解析和绘制宿主免疫系统和抗原的互作关系以及宿主免疫系统应答机制的全景图谱,主要包括抗原表位组学、免疫基因组学、免疫蛋白质组学、抗体组学和免疫信息学等方面的研究,并基于大量免疫学研究数据建立了ImmPort、VDJdb和IEDB等免疫学数据库,加速了新抗原表位的发现和免疫应答机制等研究。
免疫组学能够揭示免疫系统与疾病的关联,促进新型疫苗和免疫治疗策略开发,将有效推动个体化医疗和精准药物治疗。
近年免疫组与暴露组等的整合以及与人工智能的融合将对全面理解免疫系统对环境因素的响应和调节机制、解析疾病发生和发展的分子机制产生重大影响。
[关键词]免疫组;免疫组学;免疫信息学;人工智能Advances in immunomics researchTANG Kang, HOU Yongli, WANG Yazhen, CHEN Lihua. Department of Immunology, School of Basic Medicine,Air Force Medical University, Xi'an 710032, China[Abstract]With the progress of high-throughput sequencing technologies and bioinformatics, and deepening understanding of immune system,immunomics has evolved from initially deciphering gene sequences of B cell receptor (BCR)and T cell receptor (TCR) to unraveling and mapping interactions between host immune system and antigens, as well as panorama of host immune system response mechanisms, which now encompasses various research areas, such as antigen epitopeomics, immunogenomics, immunopro‐teomics, antibodyomics and immunoinformatics. Based on a large amount of immunological research data, immunological databases such as ImmPort, VDJdb and IEDB have been established to accelerate discovery of new antigen epitopes and study of immune response mechanisms. Immunomics has revealed the association between immune system and diseases, promoted the development of novel vac‐cines and immunotherapeutic strategies, and effectively drove the development of personalized medicine and precision medicine. In recent years, integration of immunome with exposome and fusion it with artificial intelligence will have a significant impact on compre‐hensively understanding immune system's response and regulatory mechanisms to environmental factors, as well as deciphering molecular mechanisms underlying disease occurrence and progression.[Key words]Immunome;Immunomics;Immunoinformatics;Artificial intelligence免疫组(immunome)是宿主免疫系统与抗原的互作关系以及宿主免疫系统应答机制的全景图谱,包括免疫系统的识别对象、识别受体以及参与免疫应答过程的其他分子[1-3]。
不同营养摄入方式在脑卒中后吞咽障碍中的临床应用
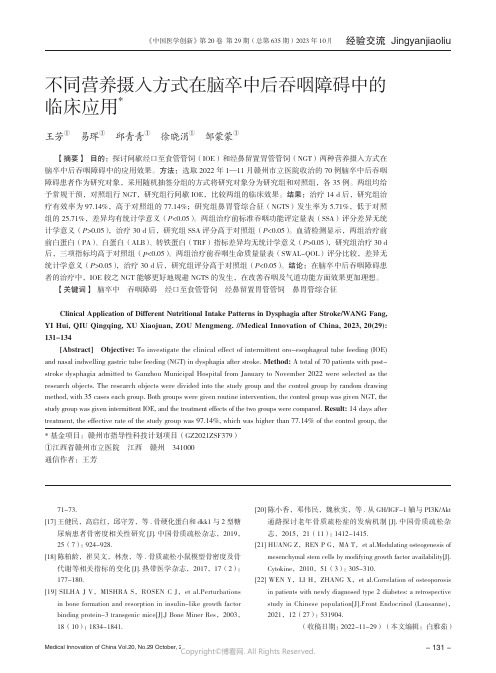
*基金项目:赣州市指导性科技计划项目(GZ2021ZSF379)①江西省赣州市立医院 江西 赣州 341000通信作者:王芳不同营养摄入方式在脑卒中后吞咽障碍中的临床应用*王芳① 易珲① 邱青青① 徐晓涓① 邹蒙蒙①【摘要】 目的:探讨间歇经口至食管管饲(IOE)和经鼻留置胃管管饲(NGT)两种营养摄入方式在脑卒中后吞咽障碍中的应用效果。
方法:选取2022年1—11月赣州市立医院收治的70例脑卒中后吞咽障碍患者作为研究对象,采用随机抽签分组的方式将研究对象分为研究组和对照组,各35例。
两组均给予常规干预,对照组行NGT,研究组行间歇IOE,比较两组的临床效果。
结果:治疗14 d 后,研究组治疗有效率为97.14%,高于对照组的77.14%;研究组鼻胃管综合征(NGTS)发生率为5.71%,低于对照组的25.71%,差异均有统计学意义(P <0.05)。
两组治疗前标准吞咽功能评定量表(SSA)评分差异无统计学意义(P >0.05),治疗30 d 后,研究组SSA 评分高于对照组(P <0.05)。
血清检测显示,两组治疗前前白蛋白(PA)、白蛋白(ALB)、转铁蛋白(TRF)指标差异均无统计学意义(P >0.05),研究组治疗30 d 后,三项指标均高于对照组(P <0.05)。
两组治疗前吞咽生命质量量表(SWAL-QOL)评分比较,差异无统计学意义(P >0.05),治疗30 d 后,研究组评分高于对照组(P <0.05)。
结论:在脑卒中后吞咽障碍患者的治疗中,IOE 较之NGT 能够更好地规避NGTS 的发生,在改善吞咽及气道功能方面效果更加理想。
【关键词】 脑卒中 吞咽障碍 经口至食管管饲 经鼻留置胃管管饲 鼻胃管综合征 Clinical Application of Different Nutritional Intake Patterns in Dysphagia after Stroke/WANG Fang, YI Hui, QIU Qingqing, XU Xiaojuan, ZOU Mengmeng. //Medical Innovation of China, 2023, 20(29): 131-134 [Abstract] Objective: To investigate the clinical effect of intermittent oro-esophageal tube feeding (IOE) and nasal indwelling gastric tube feeding (NGT) in dysphagia after stroke. Method: A total of 70 patients with post-stroke dysphagia admitted to Ganzhou Municipal Hospital from January to November 2022 were selected as the research objects. The research objects were divided into the study group and the control group by random drawing method, with 35 cases each group. Both groups were given routine intervention, the control group was given NGT, the study group was given intermittent IOE, and the treatment effects of the two groups were compared. Result: 14 days after treatment, the effective rate of the study group was 97.14%, which was higher than 77.14% of the control group, the 71-73.[17]王健民,高启红,邱守芳,等.骨硬化蛋白和dkk1与2型糖尿病患者骨密度相关性研究[J].中国骨质疏松杂志,2019,25(7):924-928.[18]陈柏龄,崔昊文,林焘,等.骨质疏松小鼠模型骨密度及骨代谢等相关指标的变化[J].热带医学杂志,2017,17(2):177-180.[19] SILHA J V,MISHRA S,ROSEN C J,et al.Perturbationsin bone formation and resorption in insulin-like growth factor binding protein-3 transgenic mice[J].J Bone Miner Res,2003,18(10):1834-1841.[20]陈小香,邓伟民,魏秋实,等.从GH/IGF-1轴与PI3K/Akt通路探讨老年骨质疏松症的发病机制[J].中国骨质疏松杂志,2015,21(11):1412-1415.[21] HUANG Z,REN P G,MA T,et al.Modulating osteogenesis ofmesenchymal stem cells by modifying growth factor availability[J].Cytokine,2010,51(3):305-310.[22] WEN Y,LI H,ZHANG X,et al.Correlation of osteoporosisin patients with newly diagnosed type 2 diabetes: a retrospective study in Chinese population[J].Front Endocrinol (Lausanne),2021,12(27):531904.(收稿日期:2022-11-29) (本文编辑:白雅茹) 脑卒中是临床上常见的一种潜在致残致死风险高的脑血管疾病,吞咽障碍则是脑卒中高发的合并症及后遗症,会大幅度增加误吸和吸入性肺炎的发生率[1],加上经口进食量降低,会造成不同程度的营养问题,这也是脑卒中患者预后不良及致残率和死亡率高的主要原因之一[2-3]。
- 1、下载文档前请自行甄别文档内容的完整性,平台不提供额外的编辑、内容补充、找答案等附加服务。
- 2、"仅部分预览"的文档,不可在线预览部分如存在完整性等问题,可反馈申请退款(可完整预览的文档不适用该条件!)。
- 3、如文档侵犯您的权益,请联系客服反馈,我们会尽快为您处理(人工客服工作时间:9:00-18:30)。
Yuh-Jye LeeCurriculum VitaUniversity Address:Computer Sciences DepartmentUniversity of Wisconsin-Madison1210West Dayton StreetMadison,WI53706-1685Telephone:002-1-608-262-6619Email:yuh-jye@/∼yuh-jyeBiographical DataMale.Born January27,1968in Taipei,Taiwan.EducationPh.D.in Computer Sciences,University of Wisconsin-Madison,August2001(expected). M.S.in Applied Mathematics,National Tsing Hua University,Taiwan,June1992.B.S.in Applied Mathematics,Chinese Culture University,Taiwan,June1990. Research interestsMy research interests are Data Mining,Machine Learning,Operations Research and Mathematical Programming.I develop the new algorithms for large data mining prob-lems such as classification problem,clustering and regression(linear and nonlinear). Using the methodologies such as support vector machines,chunking and smoothing techniques allow us to get a very robust solution(prediction)for a large dataset.I also apply these methods to solve many real world problems.An important aspect of my research is the use of data mining techniques in the breast cancer prognosis.1Teaching interestsI am interested in teaching courses such as Data Mining,Artificial Intelligence,Ma-chine Learning and Optimization(linear,nonlinear,integer and dynamic program-ming).These courses can be ranged over a broad spectrum that encompass undergrad-uate level as well as graduate school level.I can also teach many mathematical and statistical courses such as Calculus,Linear Algebra,Numerical Analysis,Probability, Stochastic Process and Statistical Inference.Work Experience1999-present:Research Assistant,O.L.Mangasarian,Computer Sciences Department,University of Wisconsin-Madison.1996-1999:Teaching Assistant,Computer Sciences Department,University of Wisconsin-Madison.1994-1995:Administration Assistant,International Mathematics Olympiad,Mathematics Department,National Taiwan Normal University.1992-1994:Platoon leader,R.O.C.Marine.PublicationsSurvival-Time Classification of Breast Cancer PatientsYuh-Jye Lee,O.L.Mangasarian and W.H.WolbergData Mining Institute Technical Report01-03,Mrach,2001,DIMACS Workshop:Data Mining and Scalable Learning Algorithms,Rutgers University, August22-24,2001,submitted.ftp:///pub/dmi/tech-reports/01-03.ps RSVM:Reduced Support Vector MachinesYuh-Jye Lee and O.L.MangasarianData Mining Institute Technical Report00-07,July,2000,First SIAM International Conference on Data Mining,Chicago,April5-7,2001.ftp:///pub/dmi/tech-reports/00-07.ps2Breast Cancer Survival and Chemotherapy:A Support Vector Machine Analysis Yuh-Jye Lee,O.L.Mangasarian and W.H.WolbergData Mining Institute Technical Report99-10,December,1999,DIMACS Series in Discrete Mathematics and Theoretical Computer Science, Vol.55(2000),1-10.ftp:///pub/dmi/tech-reports/99-10.ps SSVM:Smooth Support Vector Machine for ClassificationYuh-Jye Lee and O.L.MangasarianData Mining Institute Technical Report99-03,September,1999,Computational Optimization and Applications,to appear.ftp:///pub/dmi/tech-reports/99-03.psA Non-weakly Balanced Game with Nonempty Bargaining SetChih Chang and Yuh-Jye LeeJournal of Mathematical Economics22(1993),195-198.Conference PresentationsSurvival-Time Classification of Breast Cancer PatientsUW-Madison Data Mining Institute Annual Review,June1,2001.RSVM:Reduced Support Vector MachinesFirst SIAM International Conference on Data Mining,Chicago,April5-7,2001. Smooth Support Vector Machines&Breast Cancer Prognosis with Chemotherapy UW-Madison Data Mining Institute Annual Review,June2,2000.SSVM:Smooth Support Vector MachinesInstitute for Operations Research and the Management Sciences Fall1999Meeting, Philadelphia,Pennsylvania,November9,1999.Professional Activities and ServicesMember of INFORMSJournal Referee:Optimization Methods and SoftwareJournal of Optimization Theory and Applications3Honors and AwardsMember,Phi Tau Phi Honorary Society,1992.SIAM Student Travel Award,2001,First SIAM International Conference on Data Mining.Technical SkillsExperience and knowledge in:Programming Languages:C++,JavaMathematical tools:MATLAB,GAMS,CPLEXOperating systems:Windows95/98/NT,UNIXApplication software:Excel,PowerPointResearch and Academic ReferencesProfessor Olvi L.Mangasarian,Computer Sciences Department,Universityof Wisconsin-Madison,1210West Dayton Street,Madison,WI53706U.S.A. Telephone:002-1-608-262-6593.Email:olvi@Professor Jude W.Shavlik,Computer Sciences and Biostatistics&Medical Informatics, University of Wisconsin-Madison,1210West Dayton Street,Madison,WI53706U.S.A. Telephone:002-1-608-262-7784.Email:shavlik@Professor Chih Chang,Mathematics Department,National Tsing Hua University,101Section2Kuang Fu Road,Hsinchu,Taiwan300 Telephone:011-886-3-5715131ext.3117.Email:cchang@.tw4。