Gamal. Synthesis of high dynamic range motion blur free image from multiple captures
长排工作区三维空间伽玛等值场构建及分析
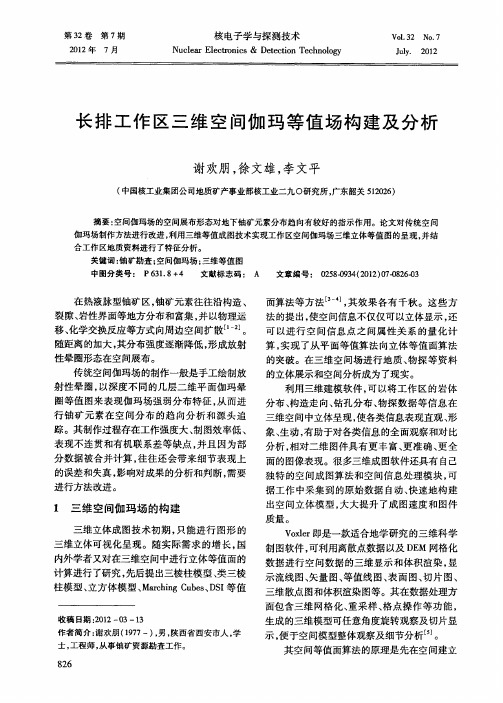
作者简 介 : 谢欢朋 (9 7一) 男 , 17 , 陕西省 西安 市人 , 学 士, 工程师 , 事铀矿资 源勘查工作 。 从
86 2
晶格 状 三 维 空 间模 型 , 每 个 晶格 体顶 点单 元 在 填充 包 含 坐 标 系统 及 属 性 的 四维 数 据 , 后 从 然 某 一 个 顶 点 开始 向其 他 几个 方 向追 踪 , 到 属 遇 性 等 值 点 处做 出等 值 标 记并 继 续 追 踪 , 完成 所 有 顶 点 追踪 ቤተ መጻሕፍቲ ባይዱ , 将 这 些 等 值标 记按 级别 连 接 再 成线 , 最后 用级 别相 同的线 构造 成面 , 渲染 后 进
合工作区地质资料进行了特征分析。
关键词 : 铀矿勘查 ; 空间伽玛场 ; 三维 等值 图
中图分类号 : P6 18+ 3 . 4 文献标志码 : A 文 章编 号 : 0 5 -9 4 2 1 )70 2 -3 2 803 ( 02 0 -8 60
在热 液脉 型铀矿 区 , 铀矿元 素 往往沿 构 造 、
第3 2卷
21 0 2年
第 7期
7月
核 电子学 与探 测技术
Nu l a e to i s& De e t n Te h oo y ce r El cr n c tc i c n l g o
Vo . 2 No 7 13 .
J l. 2 1 uy 02
长 排 工作 区三维 空 间伽 玛 等 值 场 构建 及 分 析
裂隙、 岩性界面等地方分布和富集 , 并以物理运 移 、 学交 换反应 等方 式 向周 边空 间 扩散 [ 】 化 1 。 随距离的加大 , 其分布强度逐渐降低 , 形成放射 性晕 圈形 态在空 间展 布 。 传统空间伽玛场 的制作一般是手工绘制放 射性晕圈, 以深度不同的几层二维平 面伽玛晕
高能γγ→γγ散射截面数值计算

高能γγ→γγ散射截面数值计算自20世纪30年代由许多研究者发现,γγ→γγ散射(γγ→γγ bremsstrahlung)是一种重要的现象,可以解释多种自然现象,如同步辐射(synchrotron radiation)、X射线、α射线等。
γγ→γγ散射发生时,两个γ射线在某一点发生相互作用,以产生一对新的γ射线。
由于这种作用是由强耦合的粒子间极度短程的弱相互作用而引起的,因此,它的散射截面大小一般是高能γγ能谱(γγenergy spectrum)上最小的量。
γγ→γγ散射是一种量子散射现象。
由于介子(Pion)级数收敛,以及短程弱作用性质,它的截面(cross section)较小。
由于这种交互作用紧紧耦合,粒子受到共同的影响,从而使散射截面大小远小于相同能量状态下的单个粒子散射截面。
这样,γγ→γγ散射截面就会受到起始γ能量、终止γ能量、以及粒子间的不同作用机制的影响。
γγ→γγ散射截面的数值计算是一项重要的研究领域,尤其是用于研究γ射线的传播。
这项研究可以帮助我们揭示与γ射线有关的各种现象,如用于高能天文学、高能物理学、X射线等应用中。
第一步,有必要确定γγ→γγ散射的物理机制,以及其在不同能量状态下的强度。
普通的方法是采用基本的多体理论方法来构建数值模型,以计算散射截面。
其中,最重要的是使用一组有限元方程来描述γγ→γγ散射的过程,通常使用相对论(relativity)中的量子电动力学(quantum electrodynamics,QED)技术作为原理模型,也可以使用其他技术,如强耦合理论(strong coupling theory)等。
第二步,基于预先定义的机制,运用数值方法进行建模,以计算γγ→γγ散射截面。
具体而言,通常使用Monte Carlo方法,通过随机抽样事件以模拟光子散射过程,从而计算ΦΦ→ΦΦ散射截面。
此外,可以使用Vlasov方法计算量子散射截面,该方法基于多体理论,可以考虑γγ→γγ散射截面中量子效应的影响。
CMAC最优设计及其算法——GA技术优化CMAC偏移矢量分布

CMAC最优设计及其算法——GA技术优化CMAC偏移矢量
分布
周旭东;王国栋
【期刊名称】《自动化学报》
【年(卷),期】1998(24)5
【摘要】采用GA(GeneticAlgorithm)技术实现CMAC(CerebelarModelArticulationControler)最优设计及算法.该方法解决了CMAC与其学习对象的整体优化问题,具有理论意义和实用价值.仿真结果证明该方法是成功的和有效的.对不同的客观对象(如空间曲面),可以采用GA技术找到CMAC的最优内部表示(偏移矢量分布),实现一般CMAC难以达到的精度.该方法比Albus的CMAC和Parks等的CMAC学习效果都有不同程度的提高,适合于要求高精度学习的情况.同时给出了任意偏移矢量分布的CMAC算法.
【总页数】6页(P593-598)
【关键词】CMAC;最优设计;遗传算法;偏移矢量分布;神经网络
【作者】周旭东;王国栋
【作者单位】东北大学轧制技术及连轧自动化国家重点实验室
【正文语种】中文
【中图分类】TP18
【相关文献】
1.遗传算法对CMAC与PID并行励磁控制的优化 [J], 薛一涛
2.基于遗传算法的FUZZY+CMAC优化设计 [J], 张平;苑明哲;王宏
3.基于遗传禁忌搜索算法优化的CMAC-PID液压弯辊复合控制 [J], 郎宪明;屈宝存;张奎
4.基于GA优化的CMAC-PID板形复合控制 [J], 焉颖;王焱
5.任意偏移矢量分布的N维CMAC映射算法及其应用 [J], 周旭东;王国栋;李淑华因版权原因,仅展示原文概要,查看原文内容请购买。
HIGH DYNAMIC RANGE IMAGE GENERATION AND RENDERING
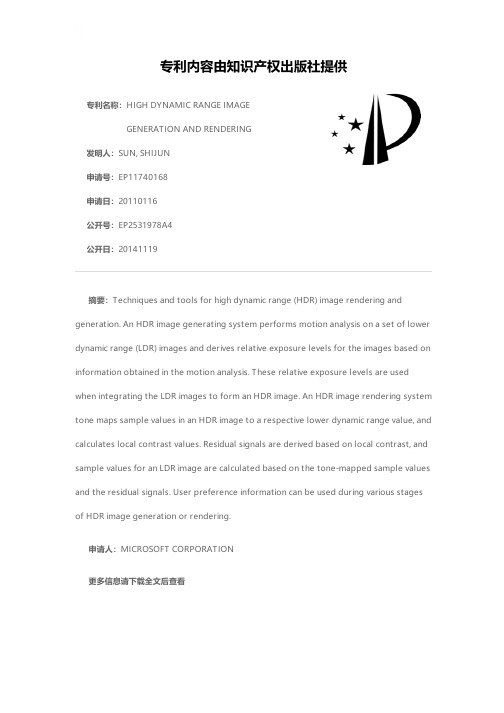
专利名称:HIGH DYNAMIC RANGE IMAGEGENERATION AND RENDERING 发明人:SUN, SHIJUN申请号:EP11740168申请日:20110116公开号:EP2531978A4公开日:20141119专利内容由知识产权出版社提供摘要:Techniques and tools for high dynamic range (HDR) image rendering and generation. An HDR image generating system performs motion analysis on a set of lower dynamic range (LDR) images and derives relative exposure levels for the images based on information obtained in the motion analysis. These relative exposure levels are used when integrating the LDR images to form an HDR image. An HDR image rendering system tone maps sample values in an HDR image to a respective lower dynamic range value, and calculates local contrast values. Residual signals are derived based on local contrast, and sample values for an LDR image are calculated based on the tone-mapped sample values and the residual signals. User preference information can be used during various stages of HDR image generation or rendering.申请人:MICROSOFT CORPORATION更多信息请下载全文后查看。
高动态范围图像的合成及可视化研究的开题报告
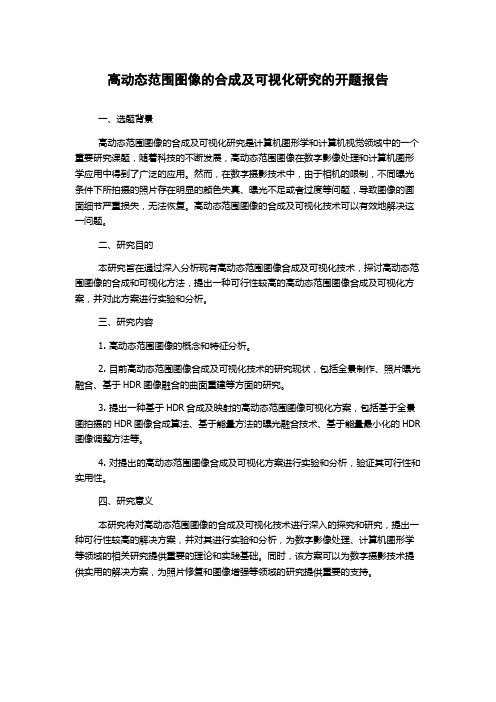
高动态范围图像的合成及可视化研究的开题报告
一、选题背景
高动态范围图像的合成及可视化研究是计算机图形学和计算机视觉领域中的一个重要研究课题,随着科技的不断发展,高动态范围图像在数字影像处理和计算机图形学应用中得到了广泛的应用。
然而,在数字摄影技术中,由于相机的限制,不同曝光条件下所拍摄的照片存在明显的颜色失真、曝光不足或者过度等问题,导致图像的画面细节严重损失,无法恢复。
高动态范围图像的合成及可视化技术可以有效地解决这一问题。
二、研究目的
本研究旨在通过深入分析现有高动态范围图像合成及可视化技术,探讨高动态范围图像的合成和可视化方法,提出一种可行性较高的高动态范围图像合成及可视化方案,并对此方案进行实验和分析。
三、研究内容
1. 高动态范围图像的概念和特征分析。
2. 目前高动态范围图像合成及可视化技术的研究现状,包括全景制作、照片曝光融合、基于HDR图像融合的曲面重建等方面的研究。
3. 提出一种基于HDR合成及映射的高动态范围图像可视化方案,包括基于全景图拍摄的HDR图像合成算法、基于能量方法的曝光融合技术、基于能量最小化的HDR 图像调整方法等。
4. 对提出的高动态范围图像合成及可视化方案进行实验和分析,验证其可行性和实用性。
四、研究意义
本研究将对高动态范围图像的合成及可视化技术进行深入的探究和研究,提出一种可行性较高的解决方案,并对其进行实验和分析,为数字影像处理、计算机图形学等领域的相关研究提供重要的理论和实践基础。
同时,该方案可以为数字摄影技术提供实用的解决方案,为照片修复和图像增强等领域的研究提供重要的支持。
高光谱数据可视化python实现 -回复

高光谱数据可视化python实现-回复标题:基于Python的高光谱数据可视化实现导言:高光谱数据是一种含有大量连续波段信息的数据,它具有广泛的应用价值,如农业、环境监测、地质勘探等领域。
可视化这些数据能够帮助我们更好地理解和分析数据,从而发现隐藏在其中的规律和信息。
本文将介绍如何使用Python实现高光谱数据的可视化,通过一步一步的详细实践,让读者熟悉高光谱数据可视化的实现过程。
第一步:获取高光谱数据首先,我们需要获取一组高光谱数据。
可以通过公开的数据集或者自行采集所需数据。
数据集通常包含多个波段,并以像素为单位存储。
这里我们以一个包含8个波段的高光谱遥感图像数据集为例。
第二步:导入所需库和数据集在Python中,我们可以使用多个库来处理和可视化高光谱数据。
常用的库包括NumPy、Pandas、Matplotlib和Seaborn。
首先,我们要确保这些库已经安装在本地环境中。
然后,我们可以使用以下代码导入数据集并加载所需库:pythonimport numpy as npimport pandas as pdimport matplotlib.pyplot as pltimport seaborn as sns# 导入高光谱数据集dataset = pd.read_csv('spectral_data.csv')第三步:数据探索与预处理在进行数据可视化前,我们需要对数据进行探索和预处理。
这包括查看数据的基本信息,检查数据是否存在缺失值或异常值,并对数据进行必要的处理。
python# 查看前几行数据print(dataset.head())# 查看数据形状print(dataset.shape)# 检查是否存在缺失值print(dataset.isnull().sum())# 检查数据的统计摘要print(dataset.describe())根据探索结果,我们可以根据需要对数据进行清洗、处理缺失值或异常值等操作。
联合最大熵的改进Niblack红外图像分割算法
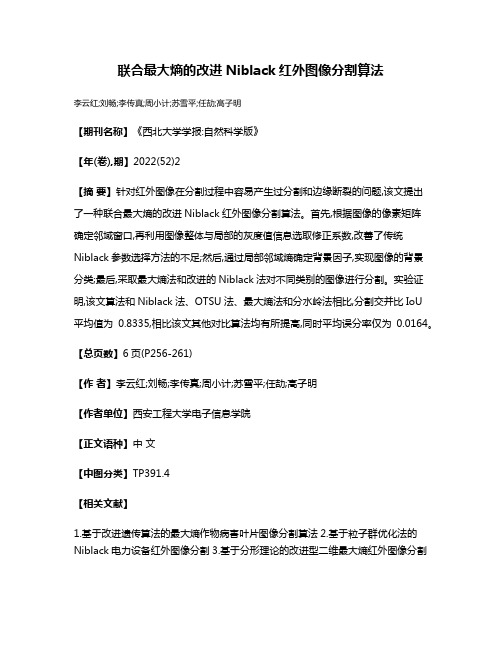
联合最大熵的改进Niblack红外图像分割算法
李云红;刘畅;李传真;周小计;苏雪平;任劼;高子明
【期刊名称】《西北大学学报:自然科学版》
【年(卷),期】2022(52)2
【摘要】针对红外图像在分割过程中容易产生过分割和边缘断裂的问题,该文提出了一种联合最大熵的改进Niblack红外图像分割算法。
首先,根据图像的像素矩阵
确定邻域窗口,再利用图像整体与局部的灰度值信息选取修正系数,改善了传统Niblack参数选择方法的不足;然后,通过局部邻域熵确定背景因子,实现图像的背景分类;最后,采取最大熵法和改进的Niblack法对不同类别的图像进行分割。
实验证明,该文算法和Niblack法、OTSU法、最大熵法和分水岭法相比,分割交并比IoU
平均值为0.8335,相比该文其他对比算法均有所提高,同时平均误分率仅为0.0164。
【总页数】6页(P256-261)
【作者】李云红;刘畅;李传真;周小计;苏雪平;任劼;高子明
【作者单位】西安工程大学电子信息学院
【正文语种】中文
【中图分类】TP391.4
【相关文献】
1.基于改进遗传算法的最大熵作物病害叶片图像分割算法
2.基于粒子群优化法的Niblack电力设备红外图像分割
3.基于分形理论的改进型二维最大熵红外图像分割
算法4.改进的OTSU和最大熵结合的迭代图像分割算法5.改进鲸鱼算法的二维最大熵图像分割研究
因版权原因,仅展示原文概要,查看原文内容请购买。
改进的低纬度化极稳定算法
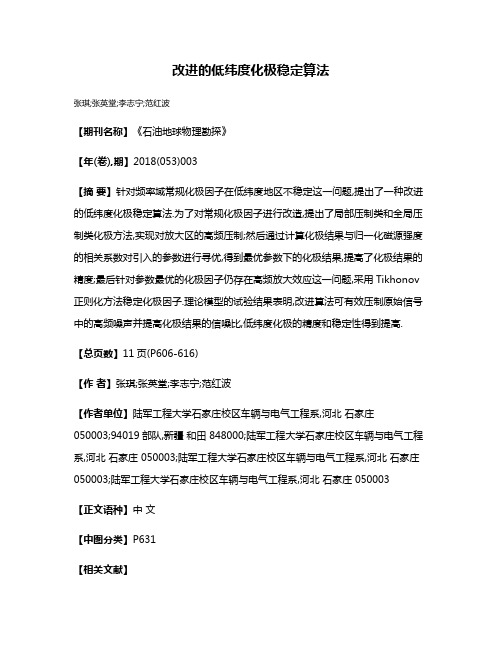
改进的低纬度化极稳定算法
张琪;张英堂;李志宁;范红波
【期刊名称】《石油地球物理勘探》
【年(卷),期】2018(053)003
【摘要】针对频率域常规化极因子在低纬度地区不稳定这一问题,提出了一种改进的低纬度化极稳定算法.为了对常规化极因子进行改造,提出了局部压制类和全局压制类化极方法,实现对放大区的高频压制;然后通过计算化极结果与归一化磁源强度的相关系数对引入的参数进行寻优,得到最优参数下的化极结果,提高了化极结果的精度;最后针对参数最优的化极因子仍存在高频放大效应这一问题,采用Tikhonov 正则化方法稳定化极因子.理论模型的试验结果表明,改进算法可有效压制原始信号中的高频噪声并提高化极结果的信噪比,低纬度化极的精度和稳定性得到提高.【总页数】11页(P606-616)
【作者】张琪;张英堂;李志宁;范红波
【作者单位】陆军工程大学石家庄校区车辆与电气工程系,河北石家庄050003;94019部队,新疆和田 848000;陆军工程大学石家庄校区车辆与电气工程系,河北石家庄 050003;陆军工程大学石家庄校区车辆与电气工程系,河北石家庄050003;陆军工程大学石家庄校区车辆与电气工程系,河北石家庄 050003
【正文语种】中文
【中图分类】P631
【相关文献】
1.从化极算法误差方程看各种波数域低纬度化极方法 [J], 柴玉璞
2.改进的阻尼法低纬度磁异常化极方法 [J], 荆磊;杨亚斌;陈亮;郜晓亮
3.低纬度磁异常化极的伪倾角方法改进 [J], 石磊;郭良辉;孟小红;王延峰
4.一种改进的低纬度磁场化极方法——变频双向阻尼因子法 [J], 林晓星;王平
5.利用稳定滤波器在低纬度地区化极 [J], Mend.,CA;郑方中
因版权原因,仅展示原文概要,查看原文内容请购买。
- 1、下载文档前请自行甄别文档内容的完整性,平台不提供额外的编辑、内容补充、找答案等附加服务。
- 2、"仅部分预览"的文档,不可在线预览部分如存在完整性等问题,可反馈申请退款(可完整预览的文档不适用该条件!)。
- 3、如文档侵犯您的权益,请联系客服反馈,我们会尽快为您处理(人工客服工作时间:9:00-18:30)。
Synthesis of High Dynamic Range Motion Blur Free Image From Multiple Captures Xinqiao(Chiao)Liu,Member,IEEE,and Abbas El Gamal,Fellow,IEEEAbstract—Advances in CMOS image sensors enable high-speed image readout,which makes it possible to capture multiple images within a normal exposure time.Earlier work has demonstrated the use of this capability to enhance sensor dynamic range.This paper presents an algorithm for synthesizing a high dynamic range,mo-tion blur free,still image from multiple captures.The algorithm consists of two main procedures,photocurrent estimation and sat-uration and motion detection.Estimation is used to reduce read noise,and,thus,to enhance dynamic range at the low illumina-tion end.Saturation detection is used to enhance dynamic range at the high illumination end as previously proposed,while mo-tion blur detection ensures that the estimation is not corrupted by motion.Motion blur detection also makes it possible to extend exposure time and to capture more images,which can be used to further enhance dynamic range at the low illumination end.Our algorithm operates completely locally;each pixel’s final value is computed using only its captured values,and recursively,requiring the storage of only a constant number of values per pixel inde-pendent of the number of images captured.Simulation and ex-perimental results demonstrate the enhanced signal-to-noise ratio (SNR),dynamic range,and the motion blur prevention achieved using the algorithm.Index Terms—CMOS image sensor,dynamic range extension, motion blur restoration,motion detection,photocurrent estima-tion,saturation detection.I.I NTRODUCTIONM OST of today’s video and digital cameras use charge-coupled-device(CCD)image sensors[1],where the charge collected by the photodetectors during exposure time is serially read out resulting in slow readout speed and high power consumption.Also,CCDs are fabricated in a non-standard technology,and as a result,other analog and digital camera functions such as A/D conversion,image processing, and compression,control,and storage cannot be integrated with the sensor on the same chip.Recently developed CMOS image sensors[2],[3],by comparison,are read out nondestructivelyManuscript received March5,2002;revised November14,2002.This work was supported in part by Agilent,in part by Canon,in part by Hewlett-Packard, in part by Interval Research,and in part by Kodak,all under the Programmable Digital Camera Program.This paper was presented in part at the2001SPIE Electronic Imaging conference,San Jose,CA,January2001[23]and at the IEEE International Conference on Acoustics,Speech,and Signal Processing, Salt Lake City,UT,May,2001[24].This paper was recommended by Associate Editor B.E.Shi.X.Liu was with the Information Systems Laboratory,Department of Elec-trical Engineering,Stanford University,Stanford,CA94304.He is now with Canesta Inc.,San Jose,CA95134USA(e-mail:chiao@).A.El Gamal is with the Information Systems Laboratory,Department of Electrical Engineering,Stanford University,Stanford,CA94305(e-mail: abbas@).Digital Object Identifier10.1109/TCSI.2003.809815and in a manner similar to a digital memory and can thus be operated continuously at very high frame rates[4]–[6].A CMOS image sensor can also be integrated with other camera functions on the same chip ultimately leading to a single-chip digital camera with very small size,low power consumption, and additional functionality[7]–[10].In[11],it is argued that the high frame-rate capability of CMOS image sensors coupled with the integration of processing with capture can enable the efficient implementations of many still and standard video imaging applications that can benefit from high frame rates, most notably,dynamic range extension.CMOS image sensors generally suffer from lower dynamic range than CCDs due to their high readout noise and nonunifor-mity.To address this problem,several methods have been pro-posed for extending CMOS image sensor dynamic range.These include well-capacity adjusting[12],multiple capture[13],[14],[15],time to saturation[17],[18],spatially-varying exposure[16],logarithmic sensor[19],[20],and local adaptation[21]. With the exception of multiple capture,all other methods can only extend dynamic range at the high illumination end.Mul-tiple capture also produces linear sensor response,which makes it possible to use correlated double sampling(CDS)for fixed pattern noise(FPN)and reset noise suppression,and to perform conventional color processing.Implementing multiple capture, however,requires very high frame-rate nondestructive readout, which has only recently become possible using digital pixel sen-sors(DPS)[6].The idea behind the multiple-capture scheme is to acquire several images at different times within exposure time—shorter-exposure-time images capture the brighter areas of the scene, while longer-exposure-time images capture the darker areas of the scene.A high dynamic-range image can then be synthesized from the multiple captures by appropriately scaling each pixel’s last sample before saturation(LSBS).In[22],it was shown that this scheme achieves higher signal-to-noise ratio(SNR)than other dynamic range-extension schemes.However,the LSBS algorithm does not take full advantage of the captured images. Since read noise is not reduced,dynamic range is only extended at the high illumination end.Dynamic range can be extended at the low illumination end by increasing exposure time.However, extending exposure time may result in unacceptable blur due to motion or change of illumination.In this paper,we describe an algorithm for synthesizing a high dynamic range image from multiple captures while avoiding motion blur.The algorithm consists of two main procedures, photocurrent estimation and motion/saturation detection.Esti-mation is used to reduce read noise,and,thus,enhance dynamic range at the low-illumination end.Saturation detection is used1057-7122/03$17.00©2003IEEEFig.1.CMOS image-sensor pixel diagram.to enhance dynamic range at the high-illumination end as pre-viously discussed,while motion blur detection ensures that the estimation is not corrupted by motion.Motion blur detection also makes it possible to extend exposure time and to capture more images,which can be used to further enhance dynamic range at the low illumination end.Our algorithm operates com-pletely locally,each pixel’s final value is computed using only its captured values,and recursively,requiring the storage of only a constant number of values per pixel independent of the number of images captured.We present three estimation algorithms.•An optimal recursive algorithm when reset noise and offset FPN are ignored.In this case,only the latest estimate and the new sample are needed to update the pixel photocurrent estimate.•An optimal nonrecursive algorithm when reset noise and FPN are considered.•A suboptimal recursive estimator for the second case,which is shown to yield mean-square error close to the nonrecursive algorithm without the need to store all the samples.The later recursive algorithm is attractive since it requires the storage of only a constant number of values per pixel.The motion-detection algorithm we describe in this paper de-tects change in each pixel’s signal due to motion or change in illumination.The decision to stop estimating after motion is de-tected is made locally and is independent of other pixels signals. The rest of the paper is organized as follows.In Section II, we describe the image-sensor signal and noise model we as-sume throughout the paper.In Section III,we describe our high-dynamic-range image-synthesis algorithm.In Section IV,we present the three estimation algorithms.In Section V,we present our motion-detection algorithm.Experimental results are pre-sented in Section VI.II.I MAGE-S ENSOR M ODELIn this section,we describe the CMOS image-sensor opera-tion and signal-and-noise model we use in the development and analysis of our synthesis algorithm.We use the model to define sensor SNR and dynamic range.The image sensor used in an analog or digital camera con-sists of a2-D array of pixels.In a typical CMOS image sensor [3],each pixel consists of a photodiode,a reset transistor,and several other readout transistors(see Fig.1).The photodiode is reset before the beginning of capture.During exposure,the photodiode converts incident light into photocurrent,foris the exposure time.This process is quite linear,and,thus,is a good measure of incident light in-tensity.Since the photocurrent is too small to measure directly, it is integrated onto the photodiode parasiticcapacitoris the electron charge.•Reset noise(including offsetFPN),the saturation charge,also referred to as well capacity.If the photocurrent is constant over exposure time,SNR is given bySNR.Thus,it is always preferred to have the longest possible exposure time.Saturation and change in photocurrent due to motion,however,makes it impractical to make exposure time too long.Dynamic range is a critical figure of merit for image sensors. It is defined as the ratio of the largest nonsaturating photocurrent to the smallest detectable photocurrent,typically defined as the standard deviation of the noise under dark ing the sensor model,dynamic range can be expressed asDR,and/or decrease read noise.III.H IGH-D YNAMIC-R ANGE I MAGE S YNTHESISWe first illustrate the effect of saturation and motion on image capture using the examples in Figs.2and3.The first plot in Fig.2represents the case of a constant low light,where pho-tocurrent can be well estimatedfromFig.2.Q (t )versus t for three lighting conditions.(a)Constant low light.(b)Constant high light.(c)Light changing.represents the case of a constant high light,where,and,as follows:1)Capture first image,set.3)Capture next image.4)For each pixel:Use the motion-detection algorithm to check if motion/saturation has occurred.i)Motion/saturation detected :Set final photocurrentestimatefromIV.P HOTOCURRENT E STIMATIONDynamic range at the low-illumination end can be enhanced using multiple captures by appropriately averaging each pixel’s photocurrent samples to reduce readout noise.Since the sensor noise depends on the signal and the photocurrent samples are de-pendent,equal weight averaging may not reduce readout noise and can in fact be worse than simply using the LSBS to estimate photocurrent.In this section,we use linear mean-square error (MSE)estimation to derive the optimal weights to be used in the averaging.We first formulate the problem.We then present estimation algorithms for three cases:1)when reset noise and offset FPN are ignored;2)when reset noise and FPN are consid-ered;and3)a recursive estimator for case2)without the need to store all the samples.Simulation results are presented and the performance of the three algorithms is compared in the last subsection.A.Problem FormulationWeassumeand define the pixelcurrent th charge sample is thus givenby(3)whereis the resetnoise.,and,,the linear MMSE problem is formulated as follows.Attime,we use superscript(k)to represent the number ofcaptures used and use subscript as the index of the coefficients for each capture.minimizes.Even though this assumption is not realistic for CMOS sensors,it is reasonable for high-end CCDs using very high-resolutionA/D converters.As we shall see,the optimal estimate in thiscase can be cast in a recursive form,which is not the case whenreset noise is considered.To derive the best estimate,define the pixel photocurrentsamplesas,i.e.,weights(6)This is a convex optimization problem with a linear constraintas in(5).To solve it,we define theLagrangian(7)whereat t=0,therefore,weights startwith aand we get[26](11)whereis known.C.Estimation Considering Reset Noise and FPNFrom(3)and(4),to minimize the MSE of the bestestimatorwhichgives(13)where(16)suchthat,asgiven in(14).The pixel current estimate given thefirst...requires the storage ofthevectorD.Recursive AlgorithmNow,we restrict ourselves to recursive estimates,i.e.,esti-mates of theformwhereagainand(20)The MSEof(21)To minimize the MSE,we requirethatwhichgivesFig.4.Distribution of estimation weights among total32samples used in thenonrecursive and recursivealgorithms.e-Fig.4plots the weights for the nonrecursive and recursive al-gorithms in Sections IV-C and D,respectively.Note that with atypical readout noise rms(60e)if sensor read noise is zero.On theother extreme,if shot noise can be ignored,then,the best es-timate is averaging(i.e.,).Also notethat weights for the nonrecursive algorithm can be negative.ItFig. 5.Simulated equivalent readout noise rms value versus number of samples k.Fig.6.Estimation enhances the SNR and dynamic range.is preferred to weight the later samples higher since they have higher SNR,and this can be achieved by using negative weights for some of the earlier samples under the unbiased estimate con-strain (sum of the weights equals one).Fig.5compares the equivalent readout noise rms at low il-lumination level correspondingtofA as a function of the number ofsamplesfor conventional sensor operation,where the lastsampleis constant and that satu-ration does not occurbeforedue to mo-tion or saturation before the new image is used to update the photocurrent estimate.Since the statistics of the noise are not completely known and no motion model is specified,it is not possible to derive an optimal detection algorithm.Our algorithm is,therefore,based on heuristics.By performing the detection step prior to each estimation step we form a blur free high dy-namic range image fromthethcapture,the best MSE linear estimateof(23)and the best predictorofchanged betweentimeto update the estimateof .Theconstant is chosen to achieve the desired tradeoff between SNR and motion blur.The higherthe(a)(b)(c)(d)(e)(f)Fig.7.Six of the65images of the high dynamic scene captured nondestructively at1000frames/s.(a)t=0ms.(a)t=0ms.(b)t=10ms.(c)t=20ms.(d)t=30ms.(e)t=40ms.(f)t=50ms.One potential problem with this“hard”decision rule is that gradual drift into update and set,,,.The counters(a)(b)(c)Fig.10.Readout values(marked by“+”)and estimated values(solid lines)for(a)pixel in the dark area,(b)pixel in bright area,and(c)pixel with varying illumination due to motion.VII.C ONCLUSIONThe high frame-rate capability of CMOS image sensors makes it possible to nondestructively capture several images within a normal exposure time.The captured images provide additional information that can be used to enhance the perfor-mance of many still and standard video imaging applications [11].The paper describes an algorithm for synthesizing a high dynamic range,motion blur free image from multiple captures. The algorithm consists of two main procedures,photocurrent estimation and motion/saturation detection.Estimation is used to reduce read noise,and,thus,enhance dynamic range at the low illumination end.Saturation detection is used to enhance dynamic range at the high illumination end,while motion blur detection ensures that the estimation is not corrupted by motion.Motion blur detection also makes it possible to extend exposure time and to capture more images,which can be used to further enhance dynamic range at the low illumination end. Experimental results demonstrate that this algorithm achieves increased SNR,enhanced dynamic range,and motion blur prevention.A CKNOWLEDGMENTThe authors would like to thank T.Chen,H.Eltoukhy, A.Ercan,S.Lim,and K.Salama for their feedback.R EFERENCES[1] A.J.Theuwissen,Solid-State Imaging With Charge-Coupled De-vices.Norwell,MA:Kluwer,May1995.[2] E.R.Fossum,“Active pixel sensors:Are CCDs dinosaurs,”Proc.SPIE,vol.1900,pp.2–14,Feb.1993.[3],“CMOS image sensors:Electronic camera-on-chip,”IEEE Trans.Electron Devices,vol.44,pp.1689–1698,Oct.1997.[4] A.Krymski,D.Van Blerkom,A.Andersson,N.Block,B.Mansoorian,and E.R.Fossum,“A high speed,500frames/s,102421024CMOS active pixel sensor,”in Proc.1999Symp.VLSI Circuits,June1999,pp.137–138.[5]N.Stevanovic,M.Hillegrand,B.J.Hostica,and A.Teuner,“A CMOSimage sensor for high speed imaging,”in Dig.Tech.Papers2000IEEE Int.Solid-State Circuits Conf.,Feb.2000,pp.104–105.[6]S.Kleinfelder,S.H.Lim,X.Liu,and A.El Gamal,“A10000frames/sCMOS digital pixel sensor,”IEEE J.Solid-State Circuits,vol.36,pp.2049–2059,Dec.2001.[7]M.Loinaz,K.Singh,A.Blanksby,D.Inglis,K.Azadet,and B.Ackland,“A200mW3.3V CMOS color camera IC producing352228824b video at30frames/s,”in Dig.Tech.Papers1998IEEE Int.Solid-State Circuits Conf.,Feb.1998,pp.168–169.[8]S.Smith,J.Hurwitz,M.Torrie,D.Baxter,A.Holmes,M.Panaghiston,R.Henderson,A.Murray,S.Anderson,and P.Denyer,“A single-chip 3062244-pixel CMOS NTSC video camera,”in Dig.Tech.Papers 1998IEEE Int.Solid-State Circuits Conf.,Feb.1998,pp.170–171. [9]S.Yoshimura,T.Sugiyama,K.Yonemoto,and K.Ueda,“A48kframes/s CMOS image sensor for real-time3-D sensing and motion detection,”in Dig.Tech.Papers2001IEEE Int.Solid-State Circuits Conf.,Feb.2001,pp.94–95.[10]T.Sugiyama,S.Yoshimura,R.Suzuki,and H.Sumi,“A1/4-inchQVGA color imaging and3-D sensing CMOS sensor with analog frame memory,”in Dig.Tech.Papers2002IEEE Int.Solid-State Circuits Conf.,Feb.2002,pp.434–435.[11]S.H.Lim and A.El Gamal,“Integration of image capture and pro-cessing—Beyond single chip digital camera,”Proc.SPIE,vol.4306,pp.219–226,Mar.2001.[12]S.J.Decker,R.D.McGrath,K.Brehmer,and C.G.Sodini,“A2562256CMOS imaging array with wide dynamic range pixels and col-lumn-parallel digital output,”IEEE J.Solid-State Circuits,vol.33,pp.2081–2091,Dec.1998.[13]O.Yadid-Pecht and E.Fossum,“Wide intrascene dynamic range CMOSAPS using dual sampling,”IEEE Trans.Electron Devices,vol.44,pp.1721–1723,Oct.1997.[14] D.Yang,A.El Gamal,B.Fowler,and H.Tian,“A6402512CMOSimage sensor with ultra-wide dynamic range floating-point pixel level ADC,”IEEE J.Solid-State Circuits,vol.34,pp.1821–1834,Dec.1999.[15]O.Yadid-Pecht and A.Belenky,“Autoscaling CMOS APS with cus-tomized increase of dynamic range,”in Dig.Tech.Papers2001IEEE Int.Solid-State Circuits Conf.,Feb.2001,pp.100–101.[16]M.Aggarwal and N.Ahuja,“High dynamic range panoramic imaging,”in Proc.8th IEEE puter Vision,vol.1,2001,pp.2–9. [17]W.Yang,“A wide-dynamic range,low power photosensor array,”in Dig.Tech.Papers1994IEEE Int.Solid-State Circuits Conf.,Feb.1994,pp.230–231.[18] E.Culurciello,R.Etienne-Cummings,and K.Boahen,“Arbitrated ad-dress event representation digital image sensor,”in Dig.Tech.Papers 2001IEEE Int.Solid-State Circuits Conf.,Feb.2001,pp.92–93. [19]M.Loose,K.Meier,and J.Schemmel,“A self-calibrating single-chipCMOS camera with logarithmic response,”IEEE J.Solid-State Circuits, vol.36,pp.586–596,Apr.2001.[20]S.Kavadias,B.Dierickx,D.Scheffer,A.Alaerts,D.Uwaerts,and J.Bogaerts,“A logarithmic response CMOS image sensor with on-chip calibration,”IEEE J.Solid-State Circuits,vol.35,pp.1146–1152,Aug.2000.[21]T.Delbruck and C.A.Mead,“Analog VLSI phototransduction,”Cali-fornia Institute of Technology,Pasadena,CNS Memo no.30,May11, 1994.[22] D.Yang and A.El Gamal,“Comparative analysis of SNR for image sen-sors with enhanced dynamic range,”Proc.SPIE,vol.3649,pp.197–211, 1999.[23]X.Liu and A.El Gamal,“Photocurrent estimation from multiple non-destructive samples in a CMOS image sensor,”Proc.SPIE,vol.4306, pp.450–458,2001.[24],“Simultaneous image formation and motion blur restoration viamultiple capture,”in Proc.ICASSP2001,vol.3,Salt Lake City,UT,May 2001,pp.1841–1844.[25]H.Sorenson,Parameter Estimation,Principles and Problems.NewYork:Marcell Dekker,1980.[26]X.Liu,“CMOS image sensors dynamic range and SNR enhancement viastatistical signal processing,”Ph.D.dissertation,Stanford Univ.,Stan-ford,CA,2002.[27] A.Ercan,F.Xiao,X.Liu,S.H.Lim,A.El Gamal,and B.Wandell,“Ex-perimental high speed CMOS image sensor system and applications,”in Proc.IEEE Sensors2002,Orlando,FL,June2002,pp.15–20.Xinqiao(Chiao)Liu(S’97–M’02)received the B.S.degree in physics from the University of Science andTechnology of China,Anhui,China,in1993,and theM.S.and Ph.D.degrees in electrical engineering fromStanford University,Stanford,CA,in1997and2002,respectively.In the summer of1998,he worked as a ResearchIntern at Interval Research Inc.,Palo Alto,CA,onimage sensor characterization and novel imagingsystem design.He is currently with Canesta Inc.,San Jose,CA,developing3-D image sensors.At Stanford,his research was focused on CMOS image sensor dynamic range and SNR enhancement via innovative circuit design,and statistical signal processingalgorithms.Abbas El Gamal(S’71–M’73–SM’83–F’00)received the B.S.degree in electrical engineeringfrom Cairo University,Cairo,Egypt,in1972,theM.S.degree in statistics,and the Ph.D.degree inelectrical engineering,both from Stanford Univer-sity,Stanford,CA,in1977and1978,respectively.From1978to1980,he was an Assistant Professorof Electrical Engineering at the University ofSouthern California,Los Angeles.He joined theStanford faculty in1981,where he is currently aProfessor of Electrical Engineering.From1984 to1988,while on leave from Stanford,he was Director of LSI Logic Re-search Lab,Sunnyvale,CA,later,Cofounder and Chief Scientist of Actel Corporation,Sunnyvale,CA.From1990to1995,he was a Cofounder and Chief Technical Officer of Silicon Architects,Mountainview,CA,which was acquired by Synopsis.He is currently a Principal Investigator on the Stanford Programmable Digital Camera project.His research interests include digital imaging and image processing,network information theory,and electrically configurable VLSI design and CAD.He has authored or coauthored over 125papers and25patents in these areas.Dr.El Gamal serves on the board of directors and advisory boards of sev-eral IC and CAD companies.He is a member of the ISSCC Technical Program Committee.。