A combination method for modeling wind power plants in power systems reliability evaluation
混凝土HPC

Modelling of hygro-thermal behaviour of concreteat high temperature with thermo-chemical and mechanical material degradationD.Gawin a ,F.Pesavento b ,B.A.Schreflerb,*aDepartment of Building Physics and Building Materials,Technical University of L odz,Al.Politechniki 6,93-590L o dz,Poland bDepartment of Structural and Transportation Engineering,University of Padua,via Marzolo 9,35131Padua,ItalyReceived 19June 2002;received in revised form 22October 2002AbstractA mathematical model for analysis of hygro-thermal behaviour of concrete as a multi-phase porous material at high temperatures,accounting for material deterioration,is presented.Full development of the model equations,starting from the macroscopic balances of mass,energy and linear momentum of single constituents is presented.Constitutive relationships for concrete at high temperature,including those concerning material damage,are discussed.The classical isotropic non-local damage theory is modified to take into account the mechanical-and the thermo-chemical concrete damage at high temperature.The final form of the governing equations,their discretised FE form,and their numerical solution are presented.The results of two numerical examples,concerning fire performance of 1-D and 2-D HPC structures,are discussed.Ó2003Elsevier Science B.V.All rights reserved.Keywords:Concrete ;High temperature;Thermo-chemical damage;Mechanical damage;Numerical model;Hygro-thermo-mechanical behaviour1.IntroductionSince the second half of the 1990s there is a renewed interest in the modelling of concrete at high and very high temperature.Introduction of new high and ultra high performance concretes required their as-sessment as far as spalling is concerned.The BRITE EURAM Project ‘‘HITECO’’(HIgh TEmperature COncrete)[8],was aimed at this purpose and has yielded very interesting results [29].Further,increased recurrence of tunnel fires in Europe,often connected with losses of life,always with destruction of the concrete vaults and walls and causing heavy economical losses,have evidenced the dramatic need for an*Corresponding author.E-mail addresses:gawindar@ck-sg.p.lodz.pl (D.Gawin),pesa@caronte.dic.unipd.it (F.Pesavento),bas@caronte.dic.unipd.it (B.A.Schrefler).0045-7825/03/$-see front matter Ó2003Elsevier Science B.V.All rights reserved.doi:10.1016/S0045-7825(03)00200-7Comput.Methods Appl.Mech.Engrg.192(2003)1731–1771/locate/cma1732 D.Gawin et al./Comput.Methods Appl.Mech.Engrg.192(2003)1731–1771D.Gawin et al./Comput.Methods Appl.Mech.Engrg.192(2003)1731–177117331734 D.Gawin et al./Comput.Methods Appl.Mech.Engrg.192(2003)1731–1771D.Gawin et al./Comput.Methods Appl.Mech.Engrg.192(2003)1731–17711735 upgrading of existing tunnels and introduction of new standards.Again the need for a new modelling capacity for concretes under such extreme conditions(temperatures exceeding1200°C for considerable time spans)has been evidenced.The EU Project UPTUN(cost-effective sustainable and innovative upgrading methods forfire safety in existing tunnels),involving about40partners,is aimed at that purpose.Finally,the modelling of aging in concrete Nuclear Power Plant Structures requires also to take into account concrete under high(and cyclic) temperatures.This aspect is currently investigated within the EURATOM project MÆCENAS.It is commonly accepted[4–7,17–19]that all the above situations cannot satisfactorily be modelled by considering thermo-mechanical processes alone.Heat and mass transfer within the concrete body(and on its boundary)has to be taken into account,together with phase changes and dehydration.The model has hence to be a chemo-hygro-thermo-mechanical one.We have been(and are)involved in all three of the above mentioned research projects and have developed such a model.This model stems from early work of Gawin et al.[16],and Gawin and Schrefler[20]and has been further developed over the years[17].Initially its applicability was limited by critical temperature of water.This limit has now been overcome[19]. Mechanical damage alone was initially considered.A coupling of permeability with mechanical damage has been introduced in[18].When simulating a real experimentalfire situation in a motorway tunnel,it turned out that mechanical damage alone was not sufficient.Thermally induced deterioration due to strains at material meso-scale and due to concrete dehydration,called thermo-chemical damage(and a modification of stress–strain curve)is also needed.This is here introduced in a framework of the isotropic non-local damage theory.Since the theory underlying the overall model is scattered in several papers,a full devel-opment of the model equations and their FE discretization is also presented.A discussion of the choice of the state variables and ensuing constitutive relations follows.Finally,two examples showing the importance of material deterioration on hygro-thermal and mechanical performance of concrete structures at high temperature are shown and discussed.Recently several works devoted in particular to the thermal spalling phenomenon in concrete at elevated temperature has been published,e.g.[37,38,43,44].As pointed out in[43],there are two main reasons of the phenomenon:build-up of pore pressure and restrained thermal dilatation.The latter hypothesis was theo-retically justified in[43]to be the most important reason of thermal spalling and used for analysis of the ‘‘Chunnel’’fire[44].In the AuthorsÕopinion both of the above mentioned reasons of the phenomenon, acting together,are of importance for the thermal spalling occurrence,in particular for the explosive one,as shown by the experimental tests carried out by Phan et al.[38].At this point it is worth to discuss some limitations of the recently proposed,macroscopic phenome-nological theory by Ulm et al.[43].Effect of temperature on concrete strength is modelled there as chemo-plastic softening within the framework of chemo-plasticity,accounting explicitly for the dehydration of concrete and its cross-effects with deformation and temperature.In the model most material properties are practically unique functions of temperature only,even if formally they depend on hydrate mass and plastic softening parameter.Moisture transfer and evaporation within the heated concrete are not taken into account,hence effect of pore pressure cannot be considered.Effect of cracking on concrete behaviour is neglected.Most of these shortcomings are overcome by the theory presented in this paper.2.Macroscopic balance equationsThe balance equations are written by considering concrete as a multi-phase material.The solid skeleton voids arefilled partly by liquid water and partly by a gas phase.Below the critical temperature of water,T cr,the liquid phase consists of bound water,which is present in the whole range of moisture content,and capillary water,which appears when degree of water saturation exceeds the upper limit of the hygroscopic region,S ssp.Above the temperature T cr the liquid phase consistsof bound water only.In the whole temperature range the gas phase is a mixture of dry air and water vapour (condensable gas constituent for T <T cr ).The model equations are obtained by means of the hybrid mixture theory (HMT)originally proposed by Hassanizadeh and Gray [25–27]and then applied for geomaterials in general by Lewis and Schrefler [30]and Schrefler [42],and for building materials by Gawin [14].For the sake of brevity,only the final form of the macroscopic conservation equations is given here.The full development of the model equations,starting from the local,microscopic balance equations with successive volume averaging,are presented in [14,30,35,42].The general form of the macroscopic,volume averaged mass conservation equation of the p -phase is [30,42]:D pq pD tþq p div v p ¼q p e p ðq Þ;ð1Þwhere q p is apparent density (related to the whole volume of the medium),v p the velocity and q p e p ðq Þthe volumetric mass source,super or subscript p refers to the p -phase.This mass balance equation has the following form for the solid skeleton [14]:D sq sD tþq s div v s ¼À_mdehydr ;ð2Þwhere _mdehydr is mass source of liquid water (and corresponding skeleton mass sink)related to the cement dehydration process.After application of the relation between the phase averaged density,q p ,and the intrinsic phase averaged density,q p ,[25–27,30]:q p ¼g p q pð3Þwith g p being the volume fraction of the p -phase,and after some simple transformations,Eq.(2)can be rewritten asð1Àn Þq s D sq s D t ÀD sn D t þð1Àn Þdiv v s¼À_m dehydr q s:ð4ÞThe volume averaged mass conservation equation for liquid water (capillary and physically adsorbed)hasthe following form:D wq wD tþq w div v w ¼_mdehydr À_m vap ;ð5Þwhere _mvap is the vapour mass source caused by the liquid water evaporation or desorption (for low values of the relative humidity inside the material pores).It is worth to underline that for the liquid water we have two source terms.By introducing the water relative velocity and material derivative of water density,with respect to the skeleton,the latter equation can be written as follows:D sq wD tþv ws Ágrad q w þq w div ðv s þv ws Þ¼_mdehydr À_m vap ð6Þwhere v p s means the p -phase relative velocity with respect to the skeleton.1736 D.Gawin et al./Comput.Methods Appl.Mech.Engrg.192(2003)1731–1771After some algebraic transformations and application of (3)with g w ¼nS w ,we have D sn D t þn q w D sq w D t þn S w D sS w D t þ1S w q w div ðnS w q w v ws Þþn div v s¼_m dehydr À_m vap S w q w:ð7ÞIn order to eliminate the time derivative of porosity,D sn =D t ,from the latter equation,we sum it up with (4)and obtain the mass conservation equation of liquid water and solid skeleton as follows:ð1Àn Þq s D sq s D t þdiv v s þn q w D sq w D t þn S w D sS w D t þ1S w q w div ðnS wq w v wsÞ¼_m dehydr À_m vap S w q w À_m dehydr q s :ð8ÞThe macroscopic volume averaged mass conservation equation of dry air,[30,42]D gaq gaþq ga div v ga ¼0ð9Þafter changing the material time derivative D gaq ga =D t into D gq ga =D t and decomposition of the dry air velocity into the diffusional,u ga ¼v ga Àv g ,and advectional (i.e.related to the centre of gravity of the whole gas phase),v g ,components,[30,42],can be rewritten asD gq gaD t þdiv J ga d þq ga div v g¼0;ð10ÞwhereJ ga d ¼q ga u ga¼nS g q ga u ga ð11Þis the diffusive mass flux of dry air molecules in the gas phase.It is reminded that there is no source term related to the dry air.Eq.(10)is now transformed in a similar way as done for the liquid water balance,i.e.material time derivative and relative velocity with respect to the solid are introduced,relation (3)with g ga ¼n ð1ÀS w Þis applied and the resulting equation,divided by q ga ð1ÀS w Þ,is summed up with the solid skeleton mass balance (4).Finally,we obtain the following equation:n S g D sS g D t þn q ga D sq ga D t þ1S g q ga div J ga dþ1S g q ga div ðnS g q ga v gs Þþ1Àn q s D sq s D t þdiv v s¼À_m dehydr q s :ð12ÞThe macroscopic mass balance of the water vapour [14,30,42]D gwq gwD tþq gw div v gw ¼_mvap ;ð13Þcan be presented,similarly to the equation for the dry air,in the following form,D g q gwD tþdiv J gw d þq gw div v g¼_m vap ;ð14Þwhere the diffusive mass flux of vapour molecules in the gas is defined as [14,30,42]J gw d ¼q gw u gw¼nS g q gw u gw :ð15ÞOne should remember,that the gas phase is an ideal binary gas mixture of dry air and water vapour,hence [14,30,42]J gw d ¼ÀJ gad :ð16ÞD.Gawin et al./Comput.Methods Appl.Mech.Engrg.192(2003)1731–17711737By applying the same transformations as for the dry air mass balance and summing(14)with(4),we obtain the following equation:n S g DsS gD tþnq gwDsq gwD tþ1S g q gwdiv J gwdþ1S g q gwdivðnS g q gw v gsÞþ1Ànq sDsq sD tþdiv v s¼À_m dehydrq sþ_m vapS g q gw:ð17ÞWe do not have any constitutive relationship for the mass source term,_m vap,appearing in the latter equation and in(8),but we can use one of the two mass balance equations to eliminate this source term from the another one.The macroscopic,volume averaged enthalpy balance equation for the p-phase,after neglecting some terms related to viscous dissipation and mechanical work,caused by density variations due to temperature changes and caused by volume fraction changes,has the following general form[30,42]:q p C pp DpT pD t¼q p h pÀdiv~q pþq p R pHÀq p e pðqÞH p;ð18Þwhere C pp is the specific isobaric heat,~q p the heatflux,q p h p the volumetric heat sources,q p R pHthe termexpressing energy exchange with the other phases(including mechanical interaction and excluding enthalpy exchange due to mass sources),H p the specific enthalpy,of the p-phase.In concrete at high temperature all heat sources,except those related to phase changes and dehydration process,can be neglected.We assume here that all phases of the material are locally in thermodynamic equilibrium,hence their temperatures are the same,T p¼Tðp¼s;w;gÞ.These temperatures may however vary throughout the domain.Summing up the enthalpy balances for all the phases of the medium,taking into account the mass sources,specific for the particular constituents,and transforming all material time derivatives into those with respect to the solid skeleton,as well as having in mind that[30,42]:X p qpR pH¼0;ð19Þone obtains the following enthalpy balance equation for the whole medium:ðq C pÞeff DsTD tþðq w C wpv wsþq g C gpv gsÞÁgrad TÀdiv~q¼À_m vap D H vapÀ_m dehydr D H dehydr;ð20Þwhereðq C pÞeff ¼q s C spþq w C wpþq g C gp;~q¼~q sþ~q wþ~q g;D H vap¼H gwÀH w;D H dehydr¼H wÀH ws:ð21ÞAbove,H ws is the specific enthalpy of the chemically bound water,D H vap the specific enthalpy of evapo-ration and D H dehydr the specific enthalpy of dehydration.Hygro-thermal phenomena in concrete,even at high temperature,are relatively slow,hence inertial forces can be neglected.For such a case the macroscopic,volume averaged linear momentum balance equation for the p-phase has the following,general form[30,42]:div t pþq p gþq p½e pðq_rÞþ^t p ¼0;ð22Þ1738 D.Gawin et al./Comput.Methods Appl.Mech.Engrg.192(2003)1731–1771D.Gawin et al./Comput.Methods Appl.Mech.Engrg.192(2003)1731–17711739 where t p is the macroscopic stress tensor in the p-phase,g the acceleration of gravity,q p^t p the volumetric exchange term of linear momentum with other phases due to mechanical interaction,and q p e pðq_rÞthat due to phase changes or chemical reactions.These exchange terms are subject to the constraint Xq p½e pðq_rÞþ^t p ¼0:ð23ÞpAfter summing up the macroscopic linear momentum balances for all the phases and introducing the total stress tensorr¼t sþt wþt g;ð24Þtaking into account condition(23)and assuming continuity of stresses at thefluid–solid interfaces,one obtainsdiv rþq g¼0;ð25Þwhereq¼ð1ÀnÞq sþnS w q wþnð1ÀS wÞq gð26Þis the averaged apparent density of the medium.The volume averaged angular momentum balance equation shows[30,42],that for non-polar media,as moist concrete is assumed in this work,all macroscopic partial stress tensors are symmetric, t p¼ðt pÞT;ð27ÞFrom the macroscopic entropy inequalities for the medium constituents,limitations for the form of con-stitutive relationships can be deduced,ing the Colemann–Noll procedure,as done in[23].This as-sures that the constitutive relations do not violate the second law of thermodynamics.3.State variablesA proper choice of state variables for description of concrete at high temperature is of particular im-portance.From a practical point of view,the physical quantities used,should be possibly easy to measure during experiments,and from a theoretical point of view,they should uniquely describe the thermodynamic state of the medium[22].They should also assure a good numerical performance of the computer code based on the resulting mathematical model.The necessary number of the state variables may be signifi-cantly reduced if existence of local thermodynamic equilibrium at each point of the medium is assumed.In such a case physical state of different phases of water can be described by use of the same variable.When fast hygro-thermal phenomena in concrete at high temperature are analysed,the assumption is debatable, but it is almost always used in modelling.We also apply it in development of the present model.Having in mind all the aforementioned remarks,we will briefly discuss now the state variables chosen for the present e of temperature(the same for all constituents of the medium because of the as-sumption about the local thermodynamic equilibrium state)and solid skeleton displacement vector is rather obvious,thus it needs no further explanation.As a hygrometric state variable various physical quantities, which are thermodynamically equivalent,may be used,e.g.volumetric––or mass moisture content,vapour pressure,relative humidity,or capillary pressure.Analysing concrete at high temperature,one must re-member that at temperatures higher than the critical point of water(i.e.647.3K)there is no capillary(or free)water present in the pores of concrete,and there exists only the gas phase of water,i.e.vapour.Then, very different moisture contents may be encountered at the same moment in a heated concrete,ranging from full saturation with liquid water(e.g.in some nuclear vessels or in so called‘‘moisture clog’’zone in aheated concrete[12])up to almost completely dry material.For these reasons it is not possible to use,in a direct way,one single variable for the whole range of moisture contents.Instead,an appropriate StefanÕs problem could be formulated,with different state variables in zones separated by moving interfaces. However,such an approach is numerically very costly,e.g.[1,34,39],and usually avoided in practical applications,as already mentioned in[19].For description of concrete moisture state,Bazant et ed in their model[5,6]the relative humidity, but in zones fully saturated with liquid water,where pressures higher than the atmospheric one can occur,a different meaning must be given to this variable,permitting its value to be higher than one,what is physically inadmissible.Then,application of a shrinkage coefficient,relating strain changes with changes of the relative humidity,is consistent with the phenomenological approach,used in[5,6],but not with the mechanistic one,which is used in this paper.Apparently,the most natural choice for the state variable seems to be mass or volumetric moisture content,which are well defined for the whole range of temper-atures and pressures in concrete.However,this quantities are not continuous at interfaces between different materials,and are not well adapted for numerical simulations,both in fully saturated conditions and in a range of very low moisture contents.Moreover,there is not any direct,physically sound(from the mechanistic point of view)relation between moisture content and stresses.Another possible choice for the moisture state variable is vapour pressure,which,however,has no physical meaning in a medium fully saturated with water and then,it creates serious numerical problems for moisture contents close to these conditions,as shown by our extensive tests.The moisture state variable proposed by the authors in[19]is capillary pressure,which was shown to be a thermodynamic potential of the physically adsorbed water and,with an appropriate interpretation,can be also used for description of water at pressures higher than the atmospheric one[20].The capillary pressure has been shown to assure good numerical performance of the computer code[16–19],and is very convenient for analysis of stress state in concrete,because there is a clear relation between pressures and stresses [23,41].Application of capillary pressure as a state variable was avoided in some previous models,e.g.[4–7], because of theoretical problems related to its definition at the macro-scale.However,some recent works in Thermodynamics[22,23],resolved these theoretical problems.Hence,the chosen primary variables of the present model are the volume averaged values of:gas pressure,p g,capillary pressure,p c,temperature,T,and displacement vector of the solid matrix,u.For temperatures lower than the critical point of water,T<T cr,and for capillary saturation range, S>S sspðTÞ(S ssp means the upper limit of the hygroscopic moisture range,being at the same time the lower limit of the capillary one),the capillary pressure is defined asp c¼p gÀp w;ð28Þwhere p w denotes water pressure.This equation is,in reality,a constitutive relationship at thermodynamic equilibrium which can be obtained from an exploitation of the entropy inequality by means of the Cole-man–Noll method,see e.g.[22,23].For all other situations,and in particular T P T cr,when condition S<S ssp is always fulfilled(there is no capillary water in the pores),the capillary pressure only substitutes formally the water potential W c defined as:W c¼RTM wlnp gwf gws;ð29Þwhere M w is the molar mass of water,R the universal gas constant and f gws the fugacity of water vapour in thermodynamic equilibrium with saturatedfilm of physically adsorbed water[19].For physically adsorbed water at lower temperatures(S<S ssp and T<T cr)the fugacity f gws should be substituted in the definition of the potential W c(29),by the saturated vapour pressure p gws.Having in mind the Kelvin equation[24], valid for the equilibrium state of capillary water with water vapour above the curved interface(meniscus) 1740 D.Gawin et al./Comput.Methods Appl.Mech.Engrg.192(2003)1731–1771ln p gw p gws¼Àp cq wM wRT;ð30Þwe can note,that in the situations,where(29)is valid,the capillary pressure may be treated formally as the water potential multiplied by the density of the liquid water,q w,according to the relation[19] p c¼ÀW c q w;ð31ÞThanks to this similarity,it is possible to use during simulations‘‘formally’’the capillary pressure even in the low moisture content range,when the capillary water is not present in the pores.However,one should remember,that in such situations capillary pressure cannot be identified to a pressure in its normal physical meaning[19],see for example(49).4.Constitutive relationshipsAs constitutive relationships may be used:equations of state for constituents of the medium,material functions describing certain physical properties of the analysed material,as well as some physical relations betweenfluxes of extensive thermodynamic quantities and intensive thermodynamic quantities(called sometimes thermodynamic forces)which cause them.These physical relations can be obtained directly from the entropy inequality,e.g.[22,23],and they describe some well-known laws of physics,like FourierÕs law, DarcyÕs law or FickÕs law.In our model,dry air,water vapour and their mixture are assumed to behave as perfect gases,following DaltonÕs law and the Clapeyron equation of state:p g¼p gaþp gw;ð32Þq p¼p p M p=TR;ðp¼ga;gw;gÞ;ð33Þwhere1 M g ¼q gwq g1M wþq gaq g1M a:ð34ÞThe density of water vapour calculated by means of(33)differs significantly from the results of the lab-oratory tests for temperatures higher than approximately160°C,but as shown in[19],this difference has a small effect on the results of simulations concerning high temperature performance of concrete and(33) may be used for practical problems with a sufficient accuracy.The state equation of water should take into account the considerable,non-linear decrease of water density in the temperature range close to the critical point of water,what has an important influence on hygro-thermal phenomena in concrete at these temperatures[19].The following formula[13],gives a reasonable accordance with experimental results and assures a good numerical performance of the code [19]:q w¼ðb0þb1Tþb2T2þb3T3þb4T4þb5T5Þþðp w1Àp wrÞða0þa1Tþa2T2þa3T3þa4T4þa5T5Þ;ð35Þwhere p w1¼10MPa,p wr¼20MPa,a0¼4:89Â10À7,a1¼À1:65Â10À9,a2¼1:86Â10À12,a3¼2:43Â10À13,a4¼À1:60Â10À15,a5¼3:37Â10À18,b0¼1:02Â10À3,b1¼À7:74Â10À1,b2¼8:77Â10À3,b3¼À9:21Â10À5,b4¼3:35Â10À7and b5¼À4:40Â10À10.In the above formula water is assumed to be incompressible.At lower relative humidities,usually en-countered at higher temperatures,most of the liquid water consists of the physically adsorbed water,whichis exposed to strong interaction with the solid skeleton,resulting in an increase of the water density [11].At the same time one can expect a decrease of the density with an increase of the capillary pressure (decrease of the water pressure),what is the case in heated concrete.Having in mind these two opposite trends,as well as lack of sufficient experimental data,we have assumed here incompressibility of the liquid water inside the pores of concrete.For the solid skeleton,the following form of the state equation has been assumed:q s ¼q s ðT ;p s ;tr r 0;C dehydr Þ;ð36Þwhere p s means the solid skeleton pressure [30,42],given by (47),tr r 0the first invariant of effective stress tensor,defined by (48),and C dehydr is degree of cement dehydration at high temperature.The latter process starts at a temperature about 100°C and continues with variable intensity during increase of concrete temperature.The following relation for the rate of the first invariant of the effective stress tensor has been assumed [30,42]:D s ðtr r 0ÞD t ¼3e K T div v s 0@þ1e K s D s p sD t Àb s D sT D t 1A ;ð37Þwhere b s is thermal expansion coefficent of the solid,e KT and e K s the actual values of bulk moduli for the whole medium and the solid skeleton (grains),respectively,taking into account possible influence of both the dehydration and cracking process.The inner structure of concrete pores is very complex and it contains both very narrow gel pores and much greater macro-pores and cracks (especially at higher temperatures),resulting sometimes in not continuous capillary pores.Nevertheless,at the macro-scale level,the volume averaged advective flux (i.e.caused by pressure gradients)of liquid water may be still described by Darcy Õs law [11,40],in the following form [19,30,42]:nS p v p s ¼Àk r p klp ½grad p p Àq p g ð38Þwhere k is intrinsic permeability tensor,k r p and l p ðp ¼g ;w Þdenote relative permeability and dynamicviscosity of the gaseous phase and liquid water.The fluids Õviscosities change significantly with temperature increase,what should be taken into account during analysis of concrete at high temperature.The formulae describing these changes are given in [17].During heating of concrete,its intrinsic permeability k may increase by up to 4orders of magnitude when compared to its initial value at ambient temperature [7,12].The dehydration and cracking progress with increasing temperature (cracking may be caused also by the mechanical stress at macro-scale)and result in gradual increase of the permeability value.For description of these intrinsic permeability changes,different approaches may be followed [18].In this paper a mechanistic one has been adopted,assuming that intrinsic permeability does depend not only upon temperature and moisture content,as assumed in classical phenomenological approach,e.g.[5,6],but also upon gas pressure and mechanical damage parameter,d [17,19]:k ¼k 0Á10A T ðT ÀT 0ÞÁpg p g0 A pÁ10A d d ;ð39a Þwhere d is the mechanical damage parameter,A T ,A p and A d are material constants.The term related to mechanical damage describes the effect of concrete cracking [3],and the gas pressure term the effect of cracks Õopening,on increase of the permeability.The influence of particular terms in (39a)on performance of concrete at high temperature is discussed in [16,18].The latter relation can be alternatively expressed in terms of total damage parameter,D ,defined by Eq.(54),。
专业英语

Definition of polymers A simple understanding of polymers can be gained by imaging them to be like a chain or, perhaps, a string of pearls, where the individual pearl represent small molecules that are chemically bonded together. Therefore, a polymer is a molecule made up of smaller molecules that are joined together by chemical bonds. The word polymer means „many parts or units.‟ The parts or units are the small molecules that combine. The result of the combination is, of course, a chainlike molecule (polymer). Usually the polymer chains are long, often consisting of hundreds of units, but polymers consisting of only a few units linked together are also known and can be commercially valuable.
Figure 1.1 Diagram illustrating the definition of plastics.
As Figure 1.1 shows, all materials can be classified as gases, simple liquids, or solids, with the understanding that most materials can be converted from one state to another through heating or cooling. If only materials that are structural solids at normal temperatures are examined, three major types of materials are encountered: metals, polymers, and ceramics. The polymer materials can be further divided into synthetic polymers and natural polymers. Most synthetic polymers are those that do not occur naturally and are represented by materials such as nylon, polyethylene, and polyester. Some synthetic polymers could be manufactured copies of naturally occurring materials (such as
模型 生成方法
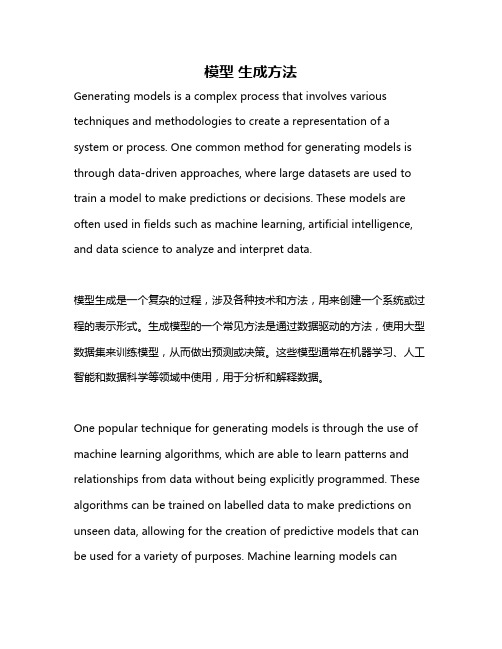
模型生成方法Generating models is a complex process that involves various techniques and methodologies to create a representation of a system or process. One common method for generating models is through data-driven approaches, where large datasets are used to train a model to make predictions or decisions. These models are often used in fields such as machine learning, artificial intelligence, and data science to analyze and interpret data.模型生成是一个复杂的过程,涉及各种技术和方法,用来创建一个系统或过程的表示形式。
生成模型的一个常见方法是通过数据驱动的方法,使用大型数据集来训练模型,从而做出预测或决策。
这些模型通常在机器学习、人工智能和数据科学等领域中使用,用于分析和解释数据。
One popular technique for generating models is through the use of machine learning algorithms, which are able to learn patterns and relationships from data without being explicitly programmed. These algorithms can be trained on labelled data to make predictions on unseen data, allowing for the creation of predictive models that can be used for a variety of purposes. Machine learning models canrange from simple linear regression models to complex deep learning models, depending on the complexity of the data and the problem being addressed.一种流行的生成模型的技术是通过使用机器学习算法,这些算法能够从数据中学习模式和关系,而无需进行显式编程。
2022届浙江省衢州、丽水、湖州三地市高三教学质量检测(二模)英语试题

2022届浙江省衢州、丽水、湖州三地市高三教学质量检测(二模)英语试题一、短对话1.What are the speakers mainly talking about?A.A camera. B.A picture. C.A festival.2.What will the speakers do?A.Go cycling. B.Play tennis. C.Play football.3.What did the woman look for?A.A path. B.A house. C.A forest.4.Where does the conversation take place?A.In a classroom. B.In a restaurant. C.In a library.5.What does the man think of his cat?A.Too old. B.Quite lovely. C.Very active.二、长对话听下面一段较长对话,回答以下小题。
6.What is said about the man?A.He likes taking pictures of birds.B.He has some pen friends in the United States.C.He gets stamps from other people with the same hobby.7.When did the stamp with the picture of a parrot come out?A.In 1935. B.In 1967. C.In 1998.听下面一段较长对话,回答以下小题。
8.What is Sarah doing?A.Doing her homework. B.Playing computer games. C.Cleaning up her room.9.What do we know about the woman’s room?A.It’s warm.B.Its heater is working. C.It has a bright lamp.听下面一段较长对话,回答以下小题。
英文模特培训课程安排计划
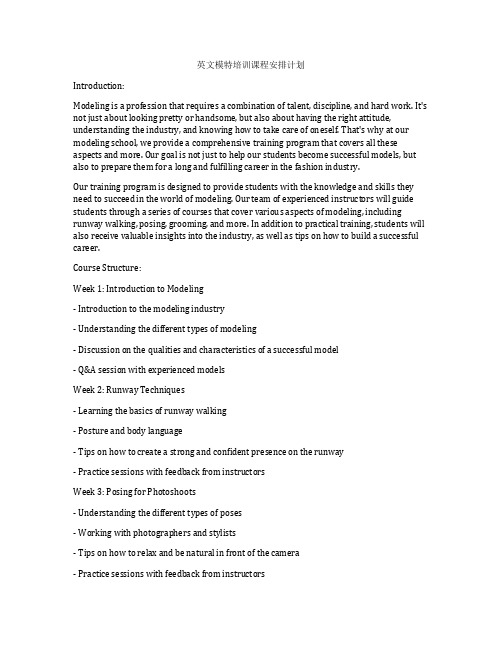
英文模特培训课程安排计划Introduction:Modeling is a profession that requires a combination of talent, discipline, and hard work. It's not just about looking pretty or handsome, but also about having the right attitude, understanding the industry, and knowing how to take care of oneself. That's why at our modeling school, we provide a comprehensive training program that covers all these aspects and more. Our goal is not just to help our students become successful models, but also to prepare them for a long and fulfilling career in the fashion industry.Our training program is designed to provide students with the knowledge and skills they need to succeed in the world of modeling. Our team of experienced instructors will guide students through a series of courses that cover various aspects of modeling, including runway walking, posing, grooming, and more. In addition to practical training, students will also receive valuable insights into the industry, as well as tips on how to build a successful career.Course Structure:Week 1: Introduction to Modeling- Introduction to the modeling industry- Understanding the different types of modeling- Discussion on the qualities and characteristics of a successful model- Q&A session with experienced modelsWeek 2: Runway Techniques- Learning the basics of runway walking- Posture and body language- Tips on how to create a strong and confident presence on the runway- Practice sessions with feedback from instructorsWeek 3: Posing for Photoshoots- Understanding the different types of poses- Working with photographers and stylists- Tips on how to relax and be natural in front of the camera- Practice sessions with feedback from instructorsWeek 4: Grooming and Makeup- Skincare and haircare tips- Understanding different makeup styles- Tips on how to do your own makeup for auditions and castings - Practical sessions with professional makeup artistsWeek 5: Nutrition and Fitness- Importance of a healthy diet for models- Tips on how to maintain a fit and toned physique- Creating a personalized fitness and nutrition plan- Q&A session with a nutritionist and fitness trainerWeek 6: Building Your Portfolio- Understanding the importance of a strong portfolio- Tips on how to select the right photographers and stylists- Practice photoshoot sessions with professional photographers - Review and feedback on portfolio developmentWeek 7: The Business of Modeling- Understanding contracts and agreements- Tips on how to negotiate with clients and agencies- Building a professional network- Q&A session with industry professionalsWeek 8: Industry Insights- Understanding the different roles in the fashion industry- Tips on how to navigate through the industry- Developing a personal brand- Q&A session with successful models and industry professionals Week 9: Building Your Personal Brand- Understanding the importance of personal branding- Tips on how to create and maintain a strong personal brand- Developing a social media strategy- Practical sessions on creating a personal brandWeek 10: Marketing Yourself- Understanding the different marketing channels for models- Creating a strong online presence- Tips on how to get noticed by agencies and clients- Q&A session with successful models and industry professionalsWeek 11: Casting and Audition Techniques- Understanding the casting process- Tips on how to prepare for auditions- Practice auditions with industry professionals- Feedback and tips on improving audition skillsWeek 12: Final Project - Fashion Show- Organizing a fashion show featuring the students- Runway walking, posing, and showcasing personal style- Feedback and evaluation from industry professionals- Graduation ceremony and award presentationConclusion:Our model training course is designed to provide students with a comprehensive understanding of the modeling industry and to equip them with the skills and knowledge they need to succeed. Our goal is to not only help students become successful models, but also to prepare them for a long and fulfilling career in the fashion industry. We believe that with the right training and guidance, anyone can achieve their dreams of becoming a successful model.。
叶片建模方法

叶片建模方法Creating a 3D model of a blade is a significant process that requires precision and attention to detail. 叶片的三维建模是一个复杂的过程,需要精确的操作和对细节的关注。
There are several methods that can be used to create a blade model, each with its advantages and disadvantages.有几种方法可以用来创建叶片模型,每种方法都有其优点和缺点。
One of the most common methods is using computer-aided design (CAD) software to create a digital model of the blade.其中最常见的方法之一是使用计算机辅助设计(CAD)软件来创建叶片的数字模型。
This method allows for precise measurements and the ability to easily make changes to the model.这种方法可以进行精确的测量,并且可以很容易地对模型进行修改。
Another method is using 3D scanning technology to create a digital model of an existing blade.另一种方法是使用3D扫描技术来创建现有叶片的数字模型。
This method is useful for reverse engineering or replicating existing blades.这种方法对于逆向工程或复制现有叶片非常有用。
Finally, some blades may be handcrafted using traditional modeling techniques.最后,一些叶片可能是使用传统的建模技术手工制作的。
卷积神经网络机器学习外文文献翻译中英文2020
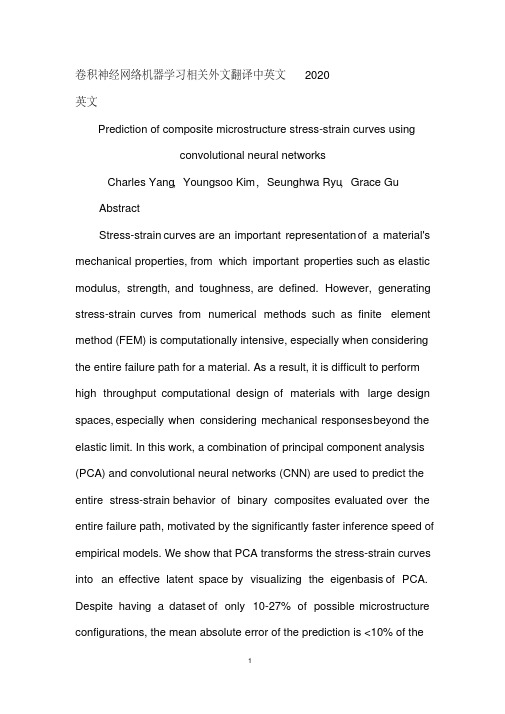
卷积神经网络机器学习相关外文翻译中英文2020英文Prediction of composite microstructure stress-strain curves usingconvolutional neural networksCharles Yang,Youngsoo Kim,Seunghwa Ryu,Grace GuAbstractStress-strain curves are an important representation of a material's mechanical properties, from which important properties such as elastic modulus, strength, and toughness, are defined. However, generating stress-strain curves from numerical methods such as finite element method (FEM) is computationally intensive, especially when considering the entire failure path for a material. As a result, it is difficult to perform high throughput computational design of materials with large design spaces, especially when considering mechanical responses beyond the elastic limit. In this work, a combination of principal component analysis (PCA) and convolutional neural networks (CNN) are used to predict the entire stress-strain behavior of binary composites evaluated over the entire failure path, motivated by the significantly faster inference speed of empirical models. We show that PCA transforms the stress-strain curves into an effective latent space by visualizing the eigenbasis of PCA. Despite having a dataset of only 10-27% of possible microstructure configurations, the mean absolute error of the prediction is <10% of therange of values in the dataset, when measuring model performance based on derived material descriptors, such as modulus, strength, and toughness. Our study demonstrates the potential to use machine learning to accelerate material design, characterization, and optimization.Keywords:Machine learning,Convolutional neural networks,Mechanical properties,Microstructure,Computational mechanics IntroductionUnderstanding the relationship between structure and property for materials is a seminal problem in material science, with significant applications for designing next-generation materials. A primary motivating example is designing composite microstructures for load-bearing applications, as composites offer advantageously high specific strength and specific toughness. Recent advancements in additive manufacturing have facilitated the fabrication of complex composite structures, and as a result, a variety of complex designs have been fabricated and tested via 3D-printing methods. While more advanced manufacturing techniques are opening up unprecedented opportunities for advanced materials and novel functionalities, identifying microstructures with desirable properties is a difficult optimization problem.One method of identifying optimal composite designs is by constructing analytical theories. For conventional particulate/fiber-reinforced composites, a variety of homogenizationtheories have been developed to predict the mechanical properties of composites as a function of volume fraction, aspect ratio, and orientation distribution of reinforcements. Because many natural composites, synthesized via self-assembly processes, have relatively periodic and regular structures, their mechanical properties can be predicted if the load transfer mechanism of a representative unit cell and the role of the self-similar hierarchical structure are understood. However, the applicability of analytical theories is limited in quantitatively predicting composite properties beyond the elastic limit in the presence of defects, because such theories rely on the concept of representative volume element (RVE), a statistical representation of material properties, whereas the strength and failure is determined by the weakest defect in the entire sample domain. Numerical modeling based on finite element methods (FEM) can complement analytical methods for predicting inelastic properties such as strength and toughness modulus (referred to as toughness, hereafter) which can only be obtained from full stress-strain curves.However, numerical schemes capable of modeling the initiation and propagation of the curvilinear cracks, such as the crack phase field model, are computationally expensive and time-consuming because a very fine mesh is required to accommodate highly concentrated stress field near crack tip and the rapid variation of damage parameter near diffusive cracksurface. Meanwhile, analytical models require significant human effort and domain expertise and fail to generalize to similar domain problems.In order to identify high-performing composites in the midst of large design spaces within realistic time-frames, we need models that can rapidly describe the mechanical properties of complex systems and be generalized easily to analogous systems. Machine learning offers the benefit of extremely fast inference times and requires only training data to learn relationships between inputs and outputs e.g., composite microstructures and their mechanical properties. Machine learning has already been applied to speed up the optimization of several different physical systems, including graphene kirigami cuts, fine-tuning spin qubit parameters, and probe microscopy tuning. Such models do not require significant human intervention or knowledge, learn relationships efficiently relative to the input design space, and can be generalized to different systems.In this paper, we utilize a combination of principal component analysis (PCA) and convolutional neural networks (CNN) to predict the entire stress-strain c urve of composite failures beyond the elastic limit. Stress-strain curves are chosen as the model's target because t hey are difficult to predict given their high dimensionality. In addition, stress-strain curves are used to derive important material descriptors such as modulus, strength, and toughness. In this sense, predicting stress-straincurves is a more general description of composites properties than any combination of scaler material descriptors. A dataset of 100,000 different composite microstructures and their corresponding stress-strain curves are used to train and evaluate model performance. Due to the high dimensionality of the stress-strain dataset, several dimensionality reduction methods are used, including PCA, featuring a blend of domain understanding and traditional machine learning, to simplify the problem without loss of generality for the model.We will first describe our modeling methodology and the parameters of our finite-element method (FEM) used to generate data. Visualizations of the learned PCA latent space are then presented, a long with model performance results.CNN implementation and trainingA convolutional neural network was trained to predict this lower dimensional representation of the stress vector. The input to the CNN was a binary matrix representing the composite design, with 0's corresponding to soft blocks and 1's corresponding to stiff blocks. PCA was implemented with the open-source Python package scikit-learn, using the default hyperparameters. CNN was implemented using Keras with a TensorFlow backend. The batch size for all experiments was set to 16 and the number of epochs to 30; the Adam optimizer was used to update the CNN weights during backpropagation.A train/test split ratio of 95:5 is used –we justify using a smaller ratio than the standard 80:20 because of a relatively large dataset. With a ratio of 95:5 and a dataset with 100,000 instances, the test set size still has enough data points, roughly several thousands, for its results to generalize. Each column of the target PCA-representation was normalized to have a mean of 0 and a standard deviation of 1 to prevent instable training.Finite element method data generationFEM was used to generate training data for the CNN model. Although initially obtained training data is compute-intensive, it takes much less time to train the CNN model and even less time to make high-throughput inferences over thousands of new, randomly generated composites. The crack phase field solver was based on the hybrid formulation for the quasi-static fracture of elastic solids and implementedin the commercial FEM software ABAQUS with a user-element subroutine (UEL).Visualizing PCAIn order to better understand the role PCA plays in effectively capturing the information contained in stress-strain curves, the principal component representation of stress-strain curves is plotted in 3 dimensions. Specifically, we take the first three principal components, which have a cumulative explained variance ~85%, and plot stress-strain curves in that basis and provide several different angles from which toview the 3D plot. Each point represents a stress-strain curve in the PCA latent space and is colored based on the associated modulus value. it seems that the PCA is able to spread out the curves in the latent space based on modulus values, which suggests that this is a useful latent space for CNN to make predictions in.CNN model design and performanceOur CNN was a fully convolutional neural network i.e. the only dense layer was the output layer. All convolution layers used 16 filters with a stride of 1, with a LeakyReLU activation followed by BatchNormalization. The first 3 Conv blocks did not have 2D MaxPooling, followed by 9 conv blocks which did have a 2D MaxPooling layer, placed after the BatchNormalization layer. A GlobalAveragePooling was used to reduce the dimensionality of the output tensor from the sequential convolution blocks and the final output layer was a Dense layer with 15 nodes, where each node corresponded to a principal component. In total, our model had 26,319 trainable weights.Our architecture was motivated by the recent development and convergence onto fully-convolutional architectures for traditional computer vision applications, where convolutions are empirically observed to be more efficient and stable for learning as opposed to dense layers. In addition, in our previous work, we had shown that CNN's werea capable architecture for learning to predict mechanical properties of 2Dcomposites [30]. The convolution operation is an intuitively good fit forpredicting crack propagation because it is a local operation, allowing it toimplicitly featurize and learn the local spatial effects of crack propagation.After applying PCA transformation to reduce the dimensionality ofthe target variable, CNN is used to predict the PCA representation of thestress-strain curve of a given binary composite design. After training theCNN on a training set, its ability to generalize to composite designs it hasnot seen is evaluated by comparing its predictions on an unseen test set.However, a natural question that emerges i s how to evaluate a model's performance at predicting stress-strain curves in a real-world engineeringcontext. While simple scaler metrics such as mean squared error (MSE)and mean absolute error (MAE) generalize easily to vector targets, it isnot clear how to interpret these aggregate summaries of performance. It isdifficult to use such metrics to ask questions such as “Is this modeand “On average, how poorly will aenough to use in the real world” given prediction be incorrect relative to some given specification”. Although being able to predict stress-strain curves is an importantapplication of FEM and a highly desirable property for any machinelearning model to learn, it does not easily lend itself to interpretation. Specifically, there is no simple quantitative way to define whether two-world units.stress-s train curves are “close” or “similar” with real Given that stress-strain curves are oftentimes intermediary representations of a composite property that are used to derive more meaningful descriptors such as modulus, strength, and toughness, we decided to evaluate the model in an analogous fashion. The CNN prediction in the PCA latent space representation is transformed back to a stress-strain curve using PCA, and used to derive the predicted modulus, strength, and toughness of the composite. The predicted material descriptors are then compared with the actual material descriptors. In this way, MSE and MAE now have clearly interpretable units and meanings. The average performance of the model with respect to the error between the actual and predicted material descriptor values derived from stress-strain curves are presented in Table. The MAE for material descriptors provides an easily interpretable metric of model performance and can easily be used in any design specification to provide confidence estimates of a model prediction. When comparing the mean absolute error (MAE) to the range of values taken on by the distribution of material descriptors, we can see that the MAE is relatively small compared to the range. The MAE compared to the range is <10% for all material descriptors. Relatively tight confidence intervals on the error indicate that this model architecture is stable, the model performance is not heavily dependent on initialization, and that our results are robust to differenttrain-test splits of the data.Future workFuture work includes combining empirical models with optimization algorithms, such as gradient-based methods, to identify composite designs that yield complementary mechanical properties. The ability of a trained empirical model to make high-throughput predictions over designs it has never seen before allows for large parameter space optimization that would be computationally infeasible for FEM. In addition, we plan to explore different visualizations of empirical models-box” of such models. Applying machine in an effort to “open up the blacklearning to finite-element methods is a rapidly growing field with the potential to discover novel next-generation materials tailored for a variety of applications. We also note that the proposed method can be readily applied to predict other physical properties represented in a similar vectorized format, such as electron/phonon density of states, and sound/light absorption spectrum.ConclusionIn conclusion, we applied PCA and CNN to rapidly and accurately predict the stress-strain curves of composites beyond the elastic limit. In doing so, several novel methodological approaches were developed, including using the derived material descriptors from the stress-strain curves as interpretable metrics for model performance and dimensionalityreduction techniques to stress-strain curves. This method has the potential to enable composite design with respect to mechanical response beyond the elastic limit, which was previously computationally infeasible, and can generalize easily to related problems outside of microstructural design for enhancing mechanical properties.中文基于卷积神经网络的复合材料微结构应力-应变曲线预测查尔斯,吉姆,瑞恩,格瑞斯摘要应力-应变曲线是材料机械性能的重要代表,从中可以定义重要的性能,例如弹性模量,强度和韧性。
hybrid method

hybrid methodHybrid MethodHybrid Method is a powerful technique that has been used in many areas of research and development, including optimization, simulation, control, and machine learning. A hybrid method combines the strengths of both numerical and symbolic methods to develop an efficient and accurate algorithm. In this article, we will discuss the basic concept, applications, advantages, and limitations of the Hybrid Method.Basic Concept of Hybrid MethodA hybrid method combines the numerical power of mathematical modeling and the symbolic power of algorithmic approach. It is designed to tackle difficult problems that are beyond the reach of traditional numerical and symbolic methods. Hybrid method is a methodology that integrates numerical and symbolic algorithms in a single algorithmic framework. It combines the numerical accuracy of numerical methods with the symbolic reasoning ofsymbolic methods, thus providing a powerful toolfor solving complex problems.Applications of Hybrid MethodHybrid method has wide applications in many areas. For example, in optimization problems,hybrid methods are used to combine the strengths of different optimization techniques such as linear programming, genetic algorithms, and simulated annealing. This combination of methods results in a more efficient and effective optimization method. Hybrid methods are also used in control systems, especially for modeling and control of complex systems such as power plants, chemical plants, and robotics.In machine learning, hybrid methods are used in the development of intelligent algorithms, such as support vector machines, deep learning, anddecision trees. These algorithms combine the advantages of both numerical and symbolic approaches, resulting in better accuracy and performance. Hybrid methods are also used in simulation, particularly in the simulation ofphysical systems, where mathematical models and simulations are combined to create a more accurate and realistic simulation.Advantages of Hybrid MethodThe main advantage of hybrid method is its ability to tackle complex problems that are beyond the reach of traditional methods. Hybrid methods are capable of handling problems that are mathematically complex, computationally intensive, or have high dimensionalities. The hybrid method also provides a robust and flexible framework that can handle different types of problems and data structures.Hybrid methods provide accurate and efficient solutions by combining the strengths of different approaches. They allow for a more holistic understanding of the problem by utilizing multiple perspectives. Additionally, these methods are extensible, meaning that they allow for the integration of new techniques and algorithms as they become available.Limitations of Hybrid MethodDespite the many advantages of hybrid methods, they also have some limitations. First, hybrid methods can be computationally intensive, and they may require high-performance computing resources. Additionally, these methods may be difficult to implement and may require specialized expertise. As such, they may not be accessible to less experienced users or those without access to advanced computing resources.Another limitation is that hybrid methods may not always result in the best solution. Although hybrid methods can provide accurate and efficient solutions, they may not always be the optimal solution. This is because the hybrid method relies on combining multiple methods, and the final solution can depend on the specific combination of methods used. Additionally, the hybrid method may not always be the most transparent approach. This means that it may be difficult to understand why the algorithm produced a particular result.ConclusionHybrid Method is an important and valuable technique that has been widely used in various fields of research and development. The hybrid method combines the strengths of both numerical and symbolic methods to develop an efficient and accurate algorithm. The hybrid method has been used in optimization, simulation, control, and machine learning. It provides accurate and efficient solutions by combining the strengths of different approaches. Although hybrid methods have limitations, they are still an essential tool for solving complex problems. As such, hybrid methods remain an important area of research and development, and we can expect to see continued growth and application of this technique in the future.。
- 1、下载文档前请自行甄别文档内容的完整性,平台不提供额外的编辑、内容补充、找答案等附加服务。
- 2、"仅部分预览"的文档,不可在线预览部分如存在完整性等问题,可反馈申请退款(可完整预览的文档不适用该条件!)。
- 3、如文档侵犯您的权益,请联系客服反馈,我们会尽快为您处理(人工客服工作时间:9:00-18:30)。
A combination method for modeling wind power plants in power systems reliability evaluationqSoodabeh Soleymani a ,Mohammad Ehsan Mosayebian a ,Sirus Mohammadi b ,⇑a Department of Electrical Engineering,Science and Research Branch,Islamic Azad University,Tehran,Iran bDepartment of Electrical Engineering,Yasuj Branch,Islamic Azad University,Yasuj,Irana r t i c l e i n f o Article history:Received 24May 2014Received in revised form 4December 2014Accepted 5December 2014Keywords:Power system reliability Wind powerMonte Carlo simulation Markov chaina b s t r a c tThe production of wind energy often involves uncertainties due to the stochastic nature of wind speeds and the variation of the power curve.In this study,a method for modeling wind power plant in power systems reliability evaluation is proposed.This method is a combination of analytical and simulation methods.Initially,the mechanical behavior of each wind turbine generator (WTG)is modeled through sequential Monte Carlo method.Then,considering the wind speed of the area,the Markov model is used for modeling the power output of wind farm.The combination method is compared with the Auto Regressive Moving Average (ARMA)time series method and some other techniques such as Weibull and normal distribution methods in wind speed modeling.Also in this study,the effects of different parameters,such as failure,repair rate and number of output power levels of WTG on the system reliability are analyzed.Ó2014Elsevier Ltd.All rights reserved.1.IntroductionDue to enhanced public concern for adverse environmental impacts and reduction of fossil fuel resources,renewable energy,especially wind energy for electricity production has been increasing rapidly.However,the variable and intermittent nature of wind energy creates difficulties in power system planning and operation.Hence,it becomes increasingly more important to evaluate the impact of wind power generation on power system reliability security.Reliability assessment of power system integrated with wind energy is a complex process.An important step in reliability evaluation of power systems including wind power is wind speed modeling to determine the power output of a WTG.There are different methods for modeling the wind speed.However,these methods require historical wind speed data collected over a number years related to a specific site in order to determine the necessary parameters of the wind speed model.The wind speed time series can usually be represented by many distributions,including Weibull distribution [1]and normal distribution [2,3].Refs.[3–5]use an ARMA time series to simulate the hourly wind speed in order to create wind speed model.This model should be combined with power curve of a WTG to create power output of a wind farm model.Therefore,this model can be used in reliability evaluation of power system integrated with wind power./10.1016/peleceng.2014.12.0050045-7906/Ó2014Elsevier Ltd.All rights reserved.qReviews processed and recommended for publication to the Editor-in-Chief by Associate Editor Dr.Danielo G.Gomes.⇑Corresponding author.Tel.:+98(917)(7220625);fax:+98(711)(7353502).E-mail address:s.mohammadi@srbiau.ac.ir (S.Mohammadi).Another important method which has received remarkable attention in recent years is known as Markov approach.A Markov process with a discrete index set is called a Markov chain.Since each value of time of wind speed can be associated to a random value,the Markov chain can be used in wind speed modeling.Each WTG of a wind farm is modeled as a two state Markov process and thus the amount of output power state of a wind farm and computing time increase [6].Several publications provide guidelines to reduce the numerical difficulties associated with the stochastic transitional probability matrix of a large system obtained from its Markov chain model.Refs.[7–9]discuss the use of Markov chain Monte Carlo (MCMC)methods as an appropriate representation of wind speed and power.Generally,there are two different techniques in reliability evaluation of power systems.One technique is based on ana-lytical method and the other on simulation method [10,11].In this paper,two mentioned methods are combined to evaluate the reliability of power system inclusion of a large amount of wind energy located in Swift Current wind farm.Sequential Monte Carlo simulation (state duration sampling)method is utilized to model mechanical behavior of power system com-ponents and load in chronological order.The Markov model is also used in the proposed method to model the power output of the wind farm.Unlike MCMC method which uses non-sequential Monte Carlo simulation,we proposed utilizing the sequential Monte Carlo simulation approach in Markov model of a wind farm in order to generate time series of wind power.The sequential Monte Carlo simulation approach according to its characteristics can provide frequency and duration of each state of the Markov model.Therefore,the sequential simulation can incorporate the chronological characteristics of wind speed and mechanical behavior of the system components simultaneously,while the non-sequential method involves non-chronolog-ical system state considerations when utilizing the non-sequential simulation approach.By considering power curve of a WTG,in the zero and nominal power regions,wind speed data become independent in the wind power domain.Therefore in this study,instead of constructing Markov model of a wind farm by means of wind speed data which leads to complicated Markov chain models,wind power domain of wind farm is used,a simpler model of which may be achieved by reduction of Markov model states.In order to illustrate and compare the results of the proposed,ARMA time series,Weibull and normal distribution meth-ods with actual data,the RTS system is used [12].In this study,it is shown that,using the proposed method the total number of output power states of the wind farm reduces.Also,the appropriate number of states in multistate WTG model is deter-mined when mechanical behavior of WTG changes.The remainder of the paper is organized as follows:Section 2provides a general description of the WTG model.Section 3presents a case study and some performance reliability indices which are used in reliability evaluation.Section 4describes the two conventional methods in wind speed modeling (i.e.ARMA time series and Markov model).Section 5gives the pro-cedure for the design of the proposed method.Section 6presents the numerical results obtained by the proposed model.Finally,some concluding remarks are made in Section 7.2.Wind turbine generator modelThe power output of a WTG can be obtained from its power curve which is a mapping of the wind speed into power out-put of wind resources.A typical power curve of the WTG is shown in Fig.1.It can be seen that the power output of a WTG is equal to zero when the wind speed is lower than cut-in speed.The WTG generates rated power when wind speed is between the rated speed and cut-out speed,and for safety reasons at cut-out speed the WTG is shutdown.The mathematical expression for a typical WTG is as follows:P WTG¼0x <V ci ðA þBx þCx 2ÞÁPrV ci x <V r P r V r x <V co 0x >V co8>>><>>>:ð1ÞS.Soleymani et al./Computers and Electrical Engineering 41(2015)28–3929where the constants (A ,B and C )are presented in [13].The cut-in,rated and cut-out wind speed of the WTG used in this paper is 4,19and 25m/s respectively.Also,rated power of the WTG is 2MW.3.Test system and reliability indicesIn this study the IEEE Reliability Test System (IEEE-RTS)has been used to evaluate power system reliability.The IEEE-RTS consists of 32conventional generating units with a total capacity of 3405MW and the system peak load is 2850MW.The generating units and reliability data are shown in Table 1.The generating unit parameter used in capacity assessment is the probability of the unit existing on forced outage at some distant time in the future.This probability was defined as the unit unavailability and is known as the unit forced outage rate (FOR)which can be calculated by Eq.(2)[14].Unavailability ðFOR Þ¼MTTR MTTF þMTTRð2ÞIn which MTTF and MTTR are mean time to failure and mean time to repair of each component respectively.Table 1gives a list of the generating unit ratings and reliability data.In addition to size,number and FOR of each generation unit,the param-eters needed in frequency and duration calculations are given as MTTF and MTTR.Historical wind speed data are related to Canadian site (i.e.Swift Current)used to estimate wind speed model.Evaluating the ability of the generation system to supply reliable electrical energy to major loads is the main issue the electric system reliability analysis encounters.Therefore,this study can also be classified as hierarchical level I (HL-I)reli-ability analysis [15].Adequacy indices are calculated by using some main parameters including frequency,duration and magnitude of power outage events.Four overall system indices including loss of load expectation (LOLE),expected energy not supplied (EENS),loss of load frequency (LOLF)and loss of load duration (LOLD)are introduced as follows [15]:LOLE ¼X 8760h ¼1t hð3Þt h ¼1if Load >generation in hour h 0otherwiseEENS ¼X 8760h ¼1e hð4Þe h ¼Load-generation if Load >generation in hour h 0otherwiseLOLF ¼X 8760h ¼1f hð5Þt h ¼1if Load >generation in hour h &Load <generation in hour h À10otherwise8><>:Table 1Comparison between different methods.Rated power (MW)No.of unit FOR MTTF (h)MTTR (h)1250.022*********.10450505060.011980207640.0219604010030.0412005015530.049604019740.0595********.0811********10.12110015030S.Soleymani et al./Computers and Electrical Engineering 41(2015)28–39LOLD¼LOLELOLFð6Þ4.Wind speed modelingThe power produced by a WTG depends primarily on the wind speed and varies randomly with time and location.There-fore,the important step in reliability evaluation of power system integrated with wind power is wind speed modeling to determine the power output of a WTG.In this section two methods will be evaluated.4.1.Wind speed modeling using ARMA time series methodThe wind speed at a specific hour is related to wind speeds of previous hours.The wind regime,therefore,can be modeled using ARMA time series models.The mathematical expression for the ARMA model is as follows[16]: yt¼u1y tÀ1þu2y tÀ2þ...þu n y tÀnþa tÀh1a tÀ1Àh2a tÀ2À...Àh n a tÀmð7Þwhere u i(i=1,2,3,...,n)and h j(j=1,2,3,...,m)are the Auto Regressive and Moving Average parameters of the model respectively,and{a t}is a normal white noise process with zero mean and variance r a2.Then the hourly simulated wind speed SW t at time t can be obtained from the mean speed l t,its standard deviation r t and the time series y t as shown in Eq.(8).SW t¼l tþr t y tð8ÞThe procedure for calculating the order(n,m)determines the adequacy of thefitted wind pattern.The ARMA model for the Swift Current site is given in Eq.(9)[5].yt¼1:1772y tÀ1þ0:1001y tÀ2À0:3572y tÀ3þ0:0379y tÀ4þa tÀ0:5030a tÀ1À0:2924a tÀ2þ0:1317a tÀ3a t2NIDð0;0:4094232Þð9ÞThe ARMA time series model generates both positive and negative values of wind speeds.Reliability study of wind power systems is usually done by setting all the negative values equal to zero[16].4.2.Wind speed modeling using Markov chainOne of the important techniques in reliability evaluation of power system that has received considerable attention in the past years is known as the Markov approach.Markov chain is one of the most effective methods to generate wind speed time series in reliability assessment of a wind farm[17,18].In order to reduce computation time in reliability evaluation of large electric power systems the concept of graph theory and Markov model can be useful[19,20].Past[21]and recent publica-tions[22]demonstrate results for the quality of wind speed simulation on different simulation time scales by Markov model.A Markov process with a discrete index set is called a Markov chain.As mentioned before,wind speed is a continuous phys-ical phenomenon that varies with time and location.Therefore wind speed can be considered as a stochastic process with a continuous state space.Nevertheless,it can be approximated as a discrete state space[23].Thus,historical data on hourly wind speed can be used to determine Markov chain with afinite number of states.The transition rates between these states can be defined by Eq.(10).k ij¼N ij=T ið10Þwhere N ij and T i are the number of transitions from state i to state j and remaining time in state i respectively.It should be noted that in the Markov model,the time remaining in each state follows the exponential distribution[23].The Markov model for wind speed(m-state)is shown in Fig.2.So,the stochastic transitional probability matrix for m-state system is given by Eq.(11).1Àðk V1ÞD t k V1V2D t k V1V3D tÁÁÁk V1V mD tk V2V1D t1Àðk V2ÞD t k V2V3D tÁÁÁk V2V mD tk V3V1D t k V3V2D t1Àðk V3ÞD tÁÁÁk V3V mD t ...............k Vm V1D t k Vm V2D t k Vm V2D tÁÁÁ1Àðk VmÞD t2 66 66 66 64377777775ð11Þwherek V1¼k V1V2þk V1V3þÁÁÁþk V1V m;k V2¼k V2V1þk V2V3þÁÁÁþk V2V m;etc:ð12ÞS.Soleymani et al./Computers and Electrical Engineering41(2015)28–3931Limiting state probability for a system with m -state which has Markovian process can be obtained using matrix multi-plication method.In order to compare the two discussed methods for modeling the wind speed with actual results,historical data on hourly wind speeds for 7years (from January 1,2003to December 31,2009)was obtained from Canadian site (Swift Current).According to Fig.3,the results using the Markov model are closer than ARMA time series,Weibull and normal model to the actual results.As mentioned earlier,ARMA model generates both positive and negative values of wind speeds and this study is done by setting all the negative values equal to zero.Hence,Fig.3demonstrates the tremendous difference between the two models in wind speed modeling,especially when wind speed is equal to zero.However,the wind speed model should be combined with power curve of the WTG to obtain the WTG power generation model as shown in Fig.4.Therefore,using this method each WTG can be modeled to obtain the wind farm power generation model.It should be noted that the failure and repair rate of the WTG cannot be modeled directly in this procedure.5.Markov model of a wind farmThe values of wind speed in the wind farm can be grouped in different levels which determine different state of wind farm power output.Each WTG in a wind farm has two possible states:the state of operation and the state of damage.Indeed,the power gen-erated by a wind farm is not only dependent on wind regime,but also the changes of machine state that resulted from failure and repair rate of WTG’s effect on it.Thus,in order to obtain the plenary model of a wind farm,the stochastic characteristics of the wind turbines behavior should be combined with the characteristics of the wind behavior.The total number of states for a wind farm with n wind turbines if m power levels are considered for each wind turbine can be calculated by Eq.(13).2n Âmð13ÞIf all wind turbines of a wind farm are similar,then the total number of states for a wind farm reduces and is equal to:m ðn þ1Þð14Þ0.020.040.060.080.10.120.140.160.180123456789101112131415161718Wind Speed (m/s)Actual Data Markov Model ARMA Model Wiebull Model Normal ModelComparison between ARMA,Markov,Weibull and normal Model with actual data in wind speed 32S.Soleymani et al./Computers and Electrical Engineering 41(2015)28–39It must be noted that due to the existence of the states with similar output power,the accurate number of states is less than the total number of states that resulted from Eq.(14).Fig.5shows the Markov diagram of a wind farm with n wind turbines,if m power levels are considered.The stochastic transitional probability state matrix for the model of Fig.5is given by Eq.(15).M ¼m 11m 12ÁÁÁm 1n þ1m 21m 22ÁÁÁm 2n þ1............m n þ11m n þ12ÁÁÁm n þ1n þ1266664377775ð15ÞFor i =j :j #i !m ij ¼1Àðk p 1þg ÞD tk p 1p 2D t ÁÁÁk p 1p m D t k p 2p 1D t 1Àðk p 2þg ÞD tÁÁÁk p 2p m D t ............k p m p 1D tk p m p 2D t1Àðk p m þg ÞD t266664377775ð16Þwhere g =(n +1Ài )k +(i À1)l .And,k p 1¼k p 1p 2þk p 1p 3þÁÁÁþk p 1p m ;k p 2¼k p 2p 1þk p 2p 3þÁÁÁþk p 2p m ;etc :ð17ÞS.Soleymani et al./Computers and Electrical Engineering 41(2015)28–3933For i=jÀ1:m ij¼ðnþ1ÀiÞk D tðnþ1ÀiÞk D t...ðnþ1ÀiÞk D t266664377775ð18ÞAnd for i=j+1:m ij¼ðiÀ1Þl D tðiÀ1Þl D t...ðiÀ1Þl D t266664377775ð19ÞThe probability of different states can be determined using matrix multiplication method.But this method does not play a significant role in reliability assessment of a large system due to the increment of processing time with the number of states [8].5.1.Proposed algorithmThe implemented algorithm is a combination of analytical(Markov process)and simulation(sequential Monte Carlo) methods.The Monte Carlo simulation can be useful in breaking a complicated system down into several subsystems.Monte Carlo simulation can be divided into two general types designated as non-sequential and sequential methods.Two tech-niques are designated as the state sampling and state duration sampling approaches.The state sampling approach is a non-sequential simulation procedure.In this approach the behavior of each component can be described by a uniform dis-tribution between0and1,and each sample is random and independent from previous samples.The state duration sampling approach is a sequential simulation procedure.This approach is based on sampling the probability distribution of the com-ponent state duration,and each system state sample is related to the previous set of system states[24].Generating units (conventional and WTG units)up and down residence times can be calculated using Eq.(20)[25].T¼ÀmÁlnðX iÞð20Þwhere T is alternatively up and down time,m is alternatively mean time to failure and repair,and X i is a random number between0and1drawn at an instant i.Fig.6shows the chronological component state transition processes of a wind farm (with85WTG)for one year.Considering the number of wind turbines that are in performance mode in each hour(as shown in Fig.6)and according to wind speed at the same time and power curve of wind turbine,the output power of a wind farm can be achieved.This output power and wind speed related to Swift Current wind farm is shown in Fig.7.This output power can be considered as a Markov chain.In general,the proposed algorithm to evaluate the reliability of power system including wind power is performed with the following steps:34S.Soleymani et al./Computers and Electrical Engineering41(2015)28–391.For the generic iteration i th,two uniformly distributed numbers (r 1i ,r 2i )between 0and 1are generated in order to cal-culate the up and down residence times for each WTG in the duration of simulation period.The failure processes (time to failure,TTF)and repair process (time to repair,TTR)are assumed to follow an exponential distribution and also,it is assumed that all the WTG in the wind farm are initially in the up state.TTF ¼Àln ðr i 1Þ=k t ð21ÞTTR ¼Àln ðr i 2Þ=l tð22Þwhere k t and l t are failure and repair rate of WTGs.2.As mentioned earlier,in iteration i th,the stochastic characteristics of wind speed should be combined with characteristics of wind turbines (due to the failure of machinery of wind turbines)to obtain wind power in the duration of simulation period.This wind power time series can be considered as a Markov chain.So,calculating the transition rate between the states obtained in the i th simulation,the stochastic transitional probability matrix can be obtained.And the dimension of the stochastic transitional probability matrix is determined by number of states obtained in the i th iteration.3.The duration time to exit (DTTE)from current state (m )to the next state (n )is calculated using Eq.(23).DTT E mn ¼Àln ðr n Þ=k mn ðn ¼1;2;...;k Þ;ðm –n Þð23Þwhere k is the number of states obtained in i th iteration.The smallest of the k values calculated from Eq.(23)defines the new state that the current power moves to and also how long it stays in the current state before moving to a different state (e.g.if DTTE mj is smaller than all,it is assumed that the current state (S m )goes to the next state (S j )after DTTE mj hours).And variable t is updated as follows:t ¼t þDTT E mjð24ÞThis step is repeated until the simulation time (e.g.8760h or one year)is over.4.Conventional units are modeled for one year and their output power is calculated using the sequential Monte Carlo simulation.5.The total generation system model is obtained through combining wind farm generation model and conventional unit generation model.6.Reliability indices in i th iteration,is calculated by placing the status of production and consumption system (in 8760h).7.Steps 1–6are repeated until a specified degree of confidence has been achieved.The accuracy level of simulation can be expressed by Eq.(25).r ðx Þ=E ðx ÞffiffiffiffiN p eð25Þwhere e is the maximum error allowed (tolerance),i.e.5%or 0.05,x is the reliability index,N is the number of sampling years,E (x )is the function mean value and r (x )is the function standard deviation.The implemented algorithm is shown in Fig.8.It is important to note that a large number of simulation years are required in order to obtain a reasonable result when utilizing the proposed algorithm.S.Soleymani et al./Computers and Electrical Engineering 41(2015)28–393536S.Soleymani et al./Computers and Electrical Engineering41(2015)28–39For reasonable failure rates,the probability of multiple failures during the simulation time is not very high.Therefore,the total number of states reduces through iterations.6.Numerical results and discussionsIn this section,the proposed method is compared with the ARMA time series,Weibull and normal distribution method in wind speed modeling and thus reliability evaluation of power system integrated with wind power.The reliability indices areS.Soleymani et al./Computers and Electrical Engineering41(2015)28–3937Table2Comparison between different methods.LOLD(h/occ)LOLF(occ/yr)EENS(MW h/yr)LOLE(h/yr)Proposed method 4.619 1.617918.17.47 Actual data––908.77.45 ARMA method 4.078 1.747884.97.12 Weibull model 4.069 1.912976.77.78 Normal model 4.59 1.514858.5 6.95recalculated using state sampling approach and the actual wind speed data (as shown in Fig.2)is used in this procedure.Therefore,170MW wind capacity (85WTG)is added to the RTS.It should be noted that in this study,all the WTG units in the Swift Current wind farm are simulated as a 14-state model for reliability evaluation (i.e.m =14).Figs.9and 10show sample of convergence for the LOLE and EENS after adding wind units to the RTS using different meth-ods for long process.Figs.9and 10clearly show that the results obtained using the proposed method are closer than ARMA time series,Wei-bull and normal distribution method to the actual results which were obtained using state sampling approach.However,this difference can affect on planners and operators decision making.The advantage of the algorithm presented in this paper is that it can be used to calculate frequency indices.This algorithm can determine the impact of failure state transition and transition associated with chronological load model,while the state sampling approach is not useful to calculate frequency indices.Different indices such as LOLF and LOLD can be calculated using the proposed method.Table 2show the final result for Different indices.Table 2clearly demonstrates that the results obtained using the proposed method are closer than other methods to the actual results data in calculating reliability indices.Also,according to this table,the proposed method based on its characteristics is able to calculate the frequency indices.As mentioned earlier,the number of output power states for a wind farm can be calculated using the proposed method.Fig.11shows the number of output power states in each simulation year for Swift Current wind farm with 85WTG,if 8power levels are considered.Also,it should be noted that the failure and repair rate of each WTG is considered equal to 10and 190times per year respectively.According to Fig.5the total number of output power states of the wind farm is equal to 688while using the proposed method the number of wind farm output power states is considerably reduced and is approximately 40.Indeed,for typical failure rates,the probability of multiple failures during the simulation time is not very high.However,the failure and repair rate of each WTG,can affect the number of wind farm output power states.Fig.12illus-trates the effect of increasing failure rate (and decreasing repair rate)of each WTG on the number of wind farms power out-put states.Fig.12shows a high increase in the total number of output power states of the Swift Current wind farm with increasing failure rate (and decreasing repair rate)of WTG’s.Also,it is obviously observed from Fig.12that the total number of output power states significantly increases with increasing the power levels of WTG.Fig.13shows the results of reliability evaluation of RTS system with 170MW wind power in Swift Current wind farm using the proposed method.As expected,the LOLE value increases with increasing failure rate (and decreasing repair rate)50100150200250300λ=2 , μ=198 λ=4 , μ=196λ=6 , μ=194λ=8, μ=192λ=10 , μ=190Failure and Repair Rate per year5 Power Levels 8 Power Levels 14 Power Levels 24 Power LevelsNumber of output power states versus failure and repair rate of WTG.77.17.27.37.47.57.6λ=2 , μ=198 λ=4 , μ=196λ=6 , μ=194λ=8, μ=192λ=10 , μ=190Failure and Repair Rate Per Year5 Power Levels8 Power Levels 14 Power Levels 24 Power LevelsFig.13.System LOLE versus failure and repair rate of WTG.38S.Soleymani et al./Computers and Electrical Engineering 41(2015)28–39。