UQ_SOE Presentation March 2013 Shandong University
2013级研究生外语期末考试考场安排
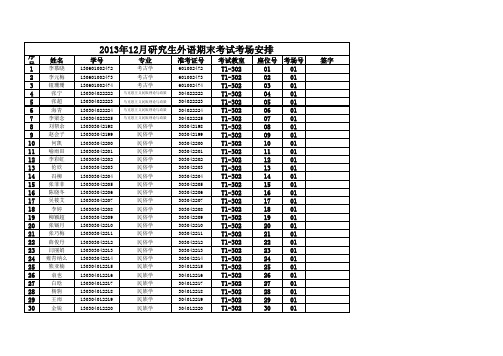
学号
120303042889 120304012250 120304012252 120304012253 120501042443 120501042444 120304032271 120304032270 120501072472 120501072464 120501072482 120501072474 120501072479 130351012267 130351012269 130351012272 130351012273 130351012274 130351012275 130351012276 130351012277 130351012278 130351012279 130351012280 130351012281 130351012282 130351012283 130351012285 130351012286 130351012287
专业名称
民俗学 民族学 民族学 民族学 中国古典文献学 中国古典文献学 中国少数民族经济 中国少数民族经济
中国少数民族语言文学 中国少数民族语言文学 中国少数民族语言文学 中国少数民族语言文学 中国少数民族语言文学
准考证号
303042889 304012250 304012252 304012253 501042443 501042444 304032271 304032270 501072472 501072464 501072482 501072474 501072479 351012267 351012269 351012272 351012273 351012274 351012275 351012276 351012277 351012278 351012279 351012280 351012281 351012282 351012283 351012285 351012286 351012287
Presentation of 1st Youth Olympic Games Singapore 2010
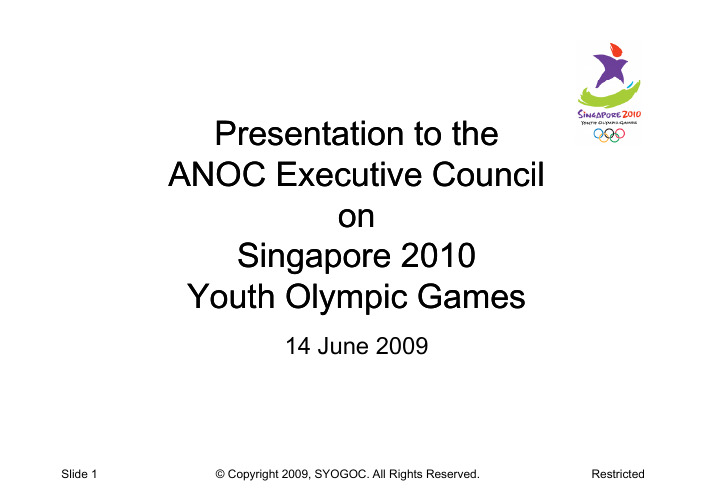
Presentation to the Presentation to theANOC Executive CouncilonSingapore 2010Singapore 2010 Youth Olympic Games y p14 June 2009Status of Singapore 2010 St t f Si2010 Youth Olympic Games Youth Olympic GamesEmblem &Sport Pictogram Emblem & Sport PictogramSpirit of Youth pTh di ti t l tThree distinct elements •The Flame of passion•The Star of Champions Sport PictogramThe Star of Champions •The Crescent of TomorrowPlanning Status Planning Status•Sport Competitionp–Competition formats finalised.–Equipment requirements andp p gprovision in progressPlanning StatusPlanning Status•CEP–5 themes and 7 format endorsed–Motivation system finalisedM ti ti t fi li d–Programmes preparation on trackDi l ith Ol iDialogue with OlympiansArts & Culture ActivitiesDiscovery ActivitiesCommunity ProjectsIsland Activity Learning JourneyWorld Culture VillagePlanning Status Planning Status•Venuesp p pp–Masterplan and concept approved–Upgrading works on track–Operations planning in progressp p g p gPlanning Status Planning Status•Youth Olympic Villageg p p–Planning and preparationon Track–Upgrading works inprogressPlanning StatusPlanning Status •NOC Interactions–Meetings & Assemblies –NOC bulletins–Chefs de Mission Seminar (22 –25 March 2010)PASOXLVI PASO G l A bl (GA)O t 08EOC37th EOC General Assembly (GA), Nov 0830th EOC Seminar, May 0938th EOC GA, Nov 0939th EOC GAOCAOCA General Assembly (GA)Oct 08XLVI PASO General Assembly (GA), Oct 08 XLVII PASO GA, Nov 09Olympic Solidarity Regional Forum, Aug 09XLVIII PASO GA27th OCA General Assembly (GA), Oct 08 28th OCA GA, Jul 09Olympic Solidarity Regional Forums, Feb & Mar 0929th OCA GAONOCANOCAANOCA Sec-Gen Meeting, Dec 08Olympic Solidarity Regional Forums, Mar & May 09ANOCA Continental General Assembly Jul 092009 ONOC General Assembly (GA) &Olympic Solidarity Regional Forum, Mar 092010 ONOC GAANOCA Continental General Assembly, Jul 09Friends@YOGTwinned 178Singapore schoolsTwinned 178 Singapore schoolswith schools from 85 NOCs [asat 29May]at 29 May]New MediaNew MediaMain website --www.singapore2010.sgMain websiteYouth Microsite ––www.whyohgee.sgYouth MicrositeFlickrFlickr ––/photos/singapore2010YoutubeYoutube ––/singapore2010Million Deeds ChallengeMillion Deeds ChallengeVisitors from 83countries(Correct as of 4 Jun 09)(C f)Challenges•Need to finalise service standards before communication with NOCscommunication with NOCsDetails of plans are still being worked out due to •Details of plans are still being worked out due to “Crunch” planning timeline –likely to be ready for sharing by End 2009for sharing by End2009Thank youDistribution of this material or derivative of this material in any form is strictly prohibited without the express written permission from the Singapore Youth Olympic Games Organising Committee (SYOGOC)。
EVALUATION OF MUSICAL FEATURES FOR EMOTION CLASSIFICATION
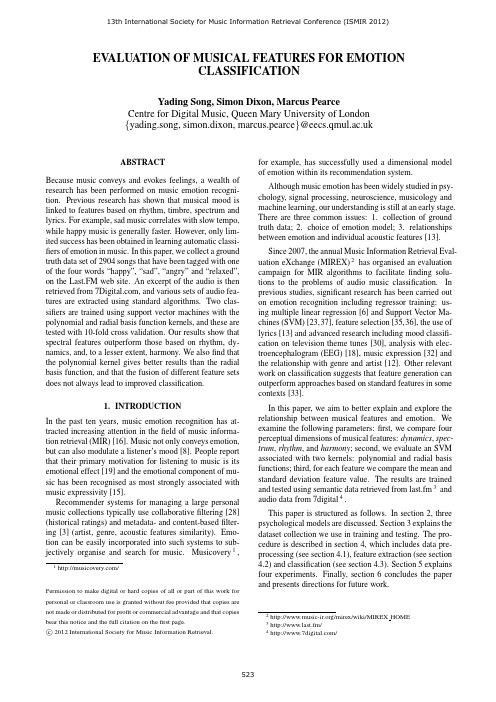
EV ALUATION OF MUSICAL FEATURES FOR EMOTIONCLASSIFICATIONYading Song,Simon Dixon,Marcus PearceCentre for Digital Music,Queen Mary University of London{yading.song,simon.dixon,marcus.pearce}@ABSTRACTBecause music conveys and evokes feelings,a wealth of research has been performed on music emotion recogni-tion.Previous research has shown that musical mood is linked to features based on rhythm,timbre,spectrum and lyrics.For example,sad music correlates with slow tempo, while happy music is generally faster.However,only lim-ited success has been obtained in learning automatic classi-fiers of emotion in music.In this paper,we collect a ground truth data set of2904songs that have been tagged with one of the four words“happy”,“sad”,“angry”and“relaxed”, on the Last.FM web site.An excerpt of the audio is then retrieved ,and various sets of audio fea-tures are extracted using standard algorithms.Two clas-sifiers are trained using support vector machines with the polynomial and radial basis function kernels,and these are tested with10-fold cross validation.Our results show that spectral features outperform those based on rhythm,dy-namics,and,to a lesser extent,harmony.We alsofind that the polynomial kernel gives better results than the radial basis function,and that the fusion of different feature sets does not always lead to improved classification.1.INTRODUCTIONIn the past ten years,music emotion recognition has at-tracted increasing attention in thefield of music informa-tion retrieval(MIR)[16].Music not only conveys emotion, but can also modulate a listener’s mood[8].People report that their primary motivation for listening to music is its emotional effect[19]and the emotional component of mu-sic has been recognised as most strongly associated with music expressivity[15].Recommender systems for managing a large personal music collections typically use collaborativefiltering[28] (historical ratings)and metadata-and content-basedfilter-ing[3](artist,genre,acoustic features similarity).Emo-tion can be easily incorporated into such systems to sub-jectively organise and search for music.Musicovery1, 1/Permission to make digital or hard copies of all or part of this work for personal or classroom use is granted without fee provided that copies are not made or distributed for profit or commercial advantage and that copies bear this notice and the full citation on thefirst page.c 2012International Society for Music Information Retrieval.for example,has successfully used a dimensional model of emotion within its recommendation system.Although music emotion has been widely studied in psy-chology,signal processing,neuroscience,musicology and machine learning,our understanding is still at an early stage. There are three common issues:1.collection of ground truth data;2.choice of emotion model;3.relationships between emotion and individual acoustic features[13].Since2007,the annual Music Information Retrieval Eval-uation eXchange(MIREX)2has organised an evaluation campaign for MIR algorithms to facilitatefinding solu-tions to the problems of audio music classification.In previous studies,significant research has been carried out on emotion recognition including regressor training:us-ing multiple linear regression[6]and Support Vector Ma-chines(SVM)[23,37],feature selection[35,36],the use of lyrics[13]and advanced research including mood classifi-cation on television theme tunes[30],analysis with elec-troencephalogram(EEG)[18],music expression[32]and the relationship with genre and artist[12].Other relevant work on classification suggests that feature generation can outperform approaches based on standard features in some contexts[33].In this paper,we aim to better explain and explore the relationship between musical features and emotion.We examine the following parameters:first,we compare four perceptual dimensions of musical features:dynamics,spec-trum,rhythm,and harmony;second,we evaluate an SVM associated with two kernels:polynomial and radial basis functions;third,for each feature we compare the mean and standard deviation feature value.The results are trained and tested using semantic data retrieved from last.fm3and audio data from7digital4.This paper is structured as follows.In section2,three psychological models are discussed.Section3explains the dataset collection we use in training and testing.The pro-cedure is described in section4,which includes data pre-processing(see section4.1),feature extraction(see section 4.2)and classification(see section4.3).Section5explains four experiments.Finally,section6concludes the paper and presents directions for future work.2/mirex/wiki/MIREX HOME3st.fm/4/2.PSYCHOLOGICAL EMOTION MODELS One of the difficulties in representing emotion is to distin-guish music-induced emotion from perceived emotion be-cause the two are not always aligned[5].Different psycho-logical models of emotion have been compared in a study of perceived emotion[7].Most music related studies are based on two popular approaches:categorical[10]and dimensional[34]mod-els of emotion.The categorical approach describes emo-tions with a limited number of innate and universal cate-gories such as happiness,sadness,anger and fear.The di-mensional model considers all affective terms arising from independent neurophysiological systems:valence(nega-tive to positive)and arousal(calm to exciting).Recently a more sophisticated model of music-induced emotion-the Geneva Emotion Music Scale(GEMS)model-consisting of9dimensions,has been proposed[42].Our results and analysis are based on the categorical model since we make our data collection through human-annotated social tags which are categorical in nature.3.GROUND-TRUTH DATA COLLECTIONAs discussed above,due to the lack of ground truth data, most researchers compile their own databases[41].Man-ual annotation is one of the most common ways to do this. However,it is expensive in terms offinancial cost and hu-man labour.Moreover,terms used may differ between in-dividuals.Different emotions may be described using the same term by different people which would result in poor prediction[38].However,with the emergence of music discovery and recommendation websites such as last.fm which support social tags for music,we can access rich human-annotated pared with the tradi-tional approach of web mining which gives noisy results, social tagging provides highly relevant information for mu-sic information retrieval(MIR)and has become an im-portant source of human-generated contextual knowledge [11].Levy[24]has also shown that social tags give a high quality source of ground truth data and can be effective in capturing music similarity[40].Thefive mood clusters proposed by MIREX[14](such as rollicking,literate,and poignant)are not popular in so-cial tags.Therefore,we use four basic emotion classes: happy,angry,sad and relaxed,considering these four emo-tions are widely accepted across different cultures and cover the four quadrants of the2-dimensional model of emo-tion[22].These four basic emotions are used as seeds to retrieve the top30tags from last.fm.We then obtain a list of songs labelled with the retrieved tags.Table1and table 2show an example of the retrieved results.Given the retrieved titles and the names of the singers, we use a public API to get previewfiles.The results cover different types of pop music,meaning that we avoid partic-ular artist and genre effects[17].Since the purpose of this step is tofind ground truth data,issues such as cold start, noise,hacking,and bias are not relevant[4,20].Most datasets on music emotion recognition are quiteHappy Angry Sad Relaxhappy angry sad relax happy hardcore angry music sad songs relax trance makes me happy angry metal happysad relax music happy music angry pop music sad song jazz relax happysad angry rock sad&beautiful only relax Table1.Top5tags returned by last.fmSinger TitleNoah And The Whale5Years TimeJason Mraz I’m YoursRusted Root Send Me On My WayRoyksopp Happy Up HereKaren O and the Kids All Is LoveTable2.Top songs returned with tags from the“happy”category.small(less than1000items),which indicates that2904 songs(see table3)for four emotions retrieved by social tags is a good size for the current experiments.The dataset will be made available5,to encourage other researchers to reproduce the results for research and evaluation.Emotion Number of SongsHappy753Angry639Sad763Relaxed749Overall2904Table3.Summary of ground truth data collection4.PROCEDURESThe experimental procedure consists of four stages:data collection,data preprocessing,feature extraction,and clas-sification,as shown infigure1.4.1Data PreprocessingAs shown in Table1,there is some noise in the data such as confusing tags and repeated songs.We manually remove data with the tag happysad which existed in both the happy and sad classes and delete the repeated songs,to make sure every song will only exist once in a single class.Moreover, we convert our dataset to standard wav format(22,050Hz sampling rate,16bit precision and mono channel).The song excerpts are either30seconds or60seconds,rep-resenting the most salient part of the song[27],therefore there is no need to truncate.At the end,we normalise the excerpts by dividing by the highest amplitude to mitigate the production effect of different recording levels.4.2Feature ExtractionAs suggested in the work of Saari and Eerola[35],two dif-ferent types of feature(mean and standard deviation)with 5The dataset can be found at https:///projects-/emotion-recognitionFigure1.Procedurea total of55features were extracted using the MIR tool-box6[21](shown in table4).The features are categorized into the following four perceptual dimensions of music lis-tening:dynamics,rhythm,spectral,and harmony.4.3ClassificationThe majority of music classification tasks[9](genre clas-sification[25,39],artist identification[29],and instrument recognition[31])have used k-nearest neighbour(K-NN) [26]and support vector machines(SVM)[2].In the case of audio input features,the SVM has been shown to per-form best[1].In this paper,therefore,we choose support vector ma-chines as our classifier,using the implementation of the se-quential minimal optimisation algorithm in the Weka data mining toolkit7.SVMs are trained using polynomial and radial basis function(RBF)kernels.We set the cost factor C=1.0,and leave other parameters unchanged.An in-ternal10-fold cross validation is applied.To better under-stand and compare features in four perceptual dimensions, our experiments are divided into four tasks.Experiment1:we compare the performance of the two kernels(polynomial and RBF)using various features.Experiment2:four classes(perceptual dimensions)of features are tested separately,and we compare the results tofind a dominant class.Experiment3:two types of feature descriptor,mean and standard deviation,are calculated.The purpose is to com-pare values for further feature selection and dimensionality reduction.6Version1.3.3:https://www.jyu.fi/music/coe/materials/mirtoolbox 7/ml/weka/Dimen.No.Features Acronyms Dynamics1-2RMS energy RMSm,RMSstd 3-4Slope Ss,Sstd5-6Attack As,Astd7Low energy LEm Rhythm1-2Tempo Ts,Tstd3-4Fluctuation peak(pos,mag)FPm,FMm5Fluctuation centroid FCm Spec.1-2Spectrum centroid SCm,SCstd3-4Brightness BRm,BRstd5-6Spread SPm,SPstd7-8Skewness SKm,SKstd9-10Kurtosis Km,Kstd11-12Rolloff95R95s,R95std13-14Rolloff85R85s,R85std15-16Spectral Entrophy SEm,SEstd17-18Flatness Fm,Fstd19-20Roughness Rm,Rstd21-22Irregularity IRm.IRstd23-24Zero crossing rate ZCRm,ZCRstd25-26Spectralflux SPm,SPstd27-28MFCC MFm,MFstd29-30DMFCC DMFm,DMFstd31-32DDMFCC DDm,DDstd Harmony1-2Chromagram peak CPm,CPstd3-4Chromagram centroid CCm,CCstd5-6Key clarity KCm,KCstd7-8Key mode KMm,KMstd9-10HCDF Hm,Hstd Table4.The feature set used in this work;m=mean,std =standard deviation.Experiment4:different combinations of feature classes (e.g.,spectral with dynamics)are evaluated in order to de-termine the best-performing model.5.RESULTS5.1Experiment1In experiment1,SVMs trained with two different kernels are compared.Previous studies[23]have found in the case of audio input that the SVM performs better than other classifiers(Logistic Regression,Random Forest,GMM, K-NN and Decision Trees).To our knowledge,no work has been reported explicitly comparing different kernels for SVMs.In emotion recognition,the radial basis func-tion kernel is a common choice because of its robustness and accuracy in other similar recognition tasks[1].Polynomial RBFFeature Class Accuracy Time Accuracy Time No.Dynamics37.20.4426.332.57Rhythm37.50.4434.523.25Harmony47.50.4136.627.410Spectral51.90.4048.114.332 Table5.Experiment1results:time=model building time, No.=number of features in each classThe results in table5show however that regardless of the features used,the polynomial kernel always achieved the higher accuracy.Moreover,the model construction times for each kernel are dramatically different.The av-erage construction time for the polynomial kernel is0.4 seconds,while the average time for the RBF kernel is24.2seconds,around60times more than the polynomial ker-nel.The following experiments also show similar results. This shows that polynomial kernel outperforms RBF in the task of emotion recognition at least for the parameter val-ues used here.5.2Experiment2In experiment2,we compare the emotion prediction re-sults for the following perceptual dimensions:dynamics, rhythm,harmony,and spectral.Results are shown infig-ure2).Dynamics and rhythm features yield similar re-sults,with harmony features providing better results,but the spectral class with32features achieves the highest ac-curacy of51.9%.This experiment provides a baseline model, and further exploration of multiple dimensions is performed in experiment4.parison of classification results for the four classes of features.5.3Experiment3In this experiment,we evaluate different types of feature descriptors,mean value and standard deviation for each feature across all feature classes,for predicting the emotion in music.The results in table6show that the use of both mean and standard deviation values gives the best results in each case.However,the processing time increased,so choosing the optimal descriptor for each feature is highly desirable.For example,choosing only the mean value in the harmony class,we lose2%of accuracy but increase the speed while the choice of standard deviation results in around10%accuracy loss.As the number of features in-creases,the difference between using mean and standard deviation will be reduced.However,more experiments are needed to explain why the mean in harmony and spectral features,and standard deviation values of dynamics and rhythm features have higher accuracy scores.5.4Experiment4In order to choose the best model,thefinal experiment fuses different perceptual features.As presented in table7, optimal accuracy is not produced by the combination of all features.Instead,the use of spectral,rhythm and harmony (but not dynamic)features produces the highest accuracy.Features Class Polynomial No.featuresDynamics all37.27Dynamics mean29.73Dynamics std33.83Rhythm all37.55Rhythm mean28.71Rhythm std34.21Harmony all47.510Harmony mean45.35Harmony std38.35Spectral all51.932Spectral mean49.616Spectral std47.516Spec+Dyn all52.339Spec+Dyn mean50.519Spec+Dyn std48.719Spec+Rhy all52.337Spec+Rhy mean49.817Spec+Rhy std47.817Spec+Har all53.342Spec+Har mean51.321Spec+Har std50.321Har+Rhy all49.115Har+Rhy mean45.66Har+Rhy std41.26Har+Dyn all48.817Har+Dyn mean46.98Har+Dyn std42.48Rhy+Dyn all41.712Rhy+Dyn mean32.04Rhy+Dyn std38.84parison of mean and standard deviation(std) features.Features Accuracy No.featuresSpec+Dyn52.339Spec+Rhy52.337Spec+Har53.342Har+Rhy49.115Har+Dyn48.817Rhy+Dyn41.712Spec+Dyn+Rhy52.444Spec+Dyn+Har53.849Spec+Rhy+Har54.047Dyn+Rhy+Har49.722All Features53.654Table7.Classification results for combinations of feature sets.6.CONCLUSION AND FUTURE WORKIn this paper,we collected ground truth data on the emo-tion associated with2904pop songs from last.fm tags.Au-dio features were extracted and grouped into four percep-tual dimensions for training and validation.Four experi-ments were conducted to predict emotion labels.The re-sults suggest that,instead of the conventional approach us-ing SVMs trained with a RBF kernel,a polynomial ker-nel yields higher accuracy.Since no single dominant fea-tures have been found in emotion recognition,we explored the performance of different perceptual classes of feature for predicting emotion in music.Experiment3found that dimensionality reduction can be achieved through remov-ing either mean or standard deviation values,halving the number of features used,with,in some cases,only2%ac-curacy loss.The last experiment found that inclusion of dynamics features with the other classes actually impairedthe performance of the classifier while the combination of spectral,rhythmic and harmonic features yielded optimal performance.In future work,we will expand this research both in depth and breadth,tofind features and classes of features which best represent emotion in music.We will examine higher-level dimensions such as temporal evolution fea-tures,as well as investigating the use of auditory ing the datasets retrieved from Last.fm,we will compare the practicability of social tags with other human-annotated datasets in emotion recognition.Through these studies of subjective emotion,we will develop methods for incorporating other empirical psychological data in a sub-jective music recommender system.7.ACKNOWLEDGEMENTSWe acknowledge the support of the Queen Mary University of London Postgraduate Research Fund(QMPGRF)and the China Scholarship Council.We would like to thank the reviewers and Emmanouil Benetos for their advice and comments.8.REFERENCES[1]K.Bischoff,C.S.Firan,R.Paiu,W.Nejdl,urier,and M.Sordo.Music Mood and Theme Classification -A Hybrid Approach.In10th International Society for Music Information Retrieval Conference,number Is-mir,pages657–662,2009.[2]E.Boser,N.Vapnik,and I.M.Guyon.Training Algo-rithm Margin for Optimal Classifiers.In ACM Confer-ence on Computational Learning Theory,pages144–152,1992.[3]P.Cano,M.Koppenberger,and N.Wack.Content-based Music Audio Recommendation.In Proceedings of the13th annual ACM international conference on Multimedia,number ACM,pages211–212,2005. [4]O.Celma.Foafing the Music:Bridging the SemanticGap in Music Recommendation.In The Semantic Web-ISWC,2006.[5]T.Eerola.Are the Emotions Expressed in Mu-sic Genre-specific?An Audio-based Evaluation of Datasets Spanning Classical,Film,Pop and Mixed Genres.Journal of New Music Research,40(March 2012):349–366,2011.[6]T.Eerola,rtillot,and P.Toiviainen.Predictionof Multdimensional Emotional Ratings in Music from Audio Using Multivariate Regression Models.In10th International Society for Music Information Retrieval Conference,number Ismir,pages621–626,2009. [7]T.Eerola and J.K.Vuoskoski.A Comparison of theDiscrete and Dimensional Models of Emotion in Mu-sic.Psychology of Music,39(1):18–49,August2010.[8]Y.Feng and Y.Zhuang.Popular Music Retrieval byDetecting Mood.In International Society for Music In-formation Retrieval Conference,volume2,pages375–376,2003.[9]Z.Fu,G.Lu,K.M.Ting,and D.Zhang.A Sur-vey of Audio-based Music Classification and Anno-tation.IEEE Transactions on Multimedia,13(2):303–319,2011.[10]K.Hevner.Experimental studies of the elements of ex-pression in music.The American Journal of Psychol-ogy,48:246–268,1936.[11]X.Hu,M.Bay,and J.S.Downie.Creating a SimplifiedMusic Mood Classification Grouth-truth Set.In Inter-national Conference on Music Information Retrieval, pages3–4,2007.[12]X.Hu and J.S.Downie.Exploring Mood Metadata:Relationships with Genre,Artist and Usage Metadata.In8th International Conference on Music Information Retrieval,2007.[13]X.Hu,J.S.Downie,and A.F.Ehmann.Lyric Text Min-ing in Music Mood Classification.In10th Interna-tional Society for Music Information Retrieval Confer-ence,number Ismir,pages411–416,2009.[14]X.Hu,J.S.Downie, urier,and M.Bay.The2007MIREX Audio Mood Classification Task:Les-son Learned.In International Society for Music Infor-mation Retrieval Conference,pages462–467,2008.[15]P.N.Juslin,J.Karlsson,E.Lindstr¨o m,A.Friberg,andE.Schoonderwaldt.Play it Again with Feeling:Com-puter Feedback in Musical Communication of Emo-tions.Journal of experimental psychology.Applied, 12(2):79–95,June2006.[16]Y.E.Kim,E.M.Schmidt,R.Migneco,B.G.Morton,P.Richardson,J.Scott,J.A.Speck,and D.Turnbull.Music Emotion Recognition:A State of the Art Re-view.In11th International Society for Music Informa-tion Retrieval Conference,number Ismir,pages255–266,2010.[17]Y.E.Kim, D.S.Williamson,and S.Pilli.TowardsQuantifying the Album Effect in Artist Identification.In International Society for Music Information Re-trieval Conference,2006.[18]S.Koelstra,C.Muhl,and M.Soleymani.Deap:ADatabase for Emotion Analysis Using Physiological Signals.IEEE Trans.on Affective Computing,pages1–15,2011.[19]C.L.Krumhansl.Music:A Link Between Cogni-tion and Emotion.American Psychological Society, 11(2):45–50,2002.[20]mere.Social Tagging and Music Information Re-trieval.Journal of New Music Research,37(2):101–114,June2008.[21]rtillot and P.Toiviainen.MIR in Matlab(II):AToolbox for Musical Feature Extraction from Audio.In International Conference on Music Information Re-trieval,number Ii,pages237–244,2007.[22]urier and J.Grivolla.Multimodal Music MoodClassification Using Audio and Lyrics.In Int.Conf.Machine Learning and Applications,pages1–6,2008.[23]urier,P.Herrera,M.Mandel,and D.Ellis.AudioMusic Mood Classification Using Support Vector Ma-chine.In MIREX task on Audio Mood Classification, pages2–4,2007.[24]M.Levy.A Semantic Space for Music Derived fromSocial Tags.In Austrian Compuer Society,volume1, page12.Citeseer,2007.[25]B.Lines,E.Tsunoo,G.Tzanetakis,and N.Ono.Be-yond Timbral Statistics:Improving Music Classifica-tion Using Percussive.IEEE Transactions on Audio, Speech and Language Processing,19(4):1003–1014, 2011.[26]T.M.Cover and P.E.Hart.Nearest Neighbor PatternClassification.IEEE Transactions on Information The-ory,13(1):21–27,1967.[27]K.F.MacDorman,S.Ough,and C.Ho.AutomaticEmotion Prediction of Song Excerpts:Index Construc-tion,Algorithm Design,and Empirical Comparison.Journal of New Music Research,36(4):281–299,De-cember2007.[28]T.Magno and C.Sable.A Comparison of Signal ofSignal-based Music Recommendation to Genre Labels, Collaborative Filtering,Musicological Analysis,Hu-man Recommendation and Random Baseline.In Pro-ceedings of the9th International Conference of Music Information Retrieval,pages161–166,2008.[29]M.Mandel.Song-level Features and Support Vec-tor Machines for Music Classification.In Proc.Inter-national Conference on Music Information Retrieval, 2005.[30]M.Mann,T.J.Cox,and F.F.Li.Music Mood Classi-fication of Television Theme Tunes.In12th Interna-tional Society for Music Information Retrieval Confer-ence,number Ismir,pages735–740,2011.[31]J.Marques and P.J.Moreno.A Study of Musical In-strument Classification Using Gaussian Mixture Mod-els and Support Vector Machines,1999.[32]L.Mion and G.D.Poli.Score-Independent AudioFeatures for Description of Music Expression.IEEE Transactions on Audio,Speech,and Language Pro-cessing,16(2):458–466,2008.[33]F.Pachet and P.Roy.Analytical Features:AKnowledge-Based Approach to Audio Feature Gener-ation.EURASIP Journal on Audio,Speech,and Music Processing,2009(2):1–23,2009.[34]J.A.Russell,A.Weiss,and G.A.Mendelsohn.Af-fect Grid:A Single-item Scale of Pleasure and Arousal.Journal of Personality and Social Psychology, 57(3):493–502,1989.[35]P.Saari,T.Eerola,and rtillot.Generalizabilityand Simplicity as Criteria in Feature Selection:Appli-cation to Mood Classification in Music.IEEE Trans-actions on Audio,Speech,and Language Processing, 19(6):1802–1812,2011.[36]E.M.Schmidt,D.Turnbull,and Y.E.Kim.FeatureSelection for Content-Based,Time-Varying Musical Emotion Regression Categories and Subject Descrip-tors.In Multimedia Information Retrieval,pages267–273,2010.[37]B.Schuller,J.Dorfner,and G.Rigoll.Determinationof Nonprototypical Valence and Arousal in Popular Music:Features and Performances.EURASIP Journal on Audio,Speech,and Music Processing,2010:1–19, 2010.[38]D.Turnbull,L.Barrington,and nckriet.Five Ap-proaches to Collecting Tags for Music.In Proceedings of the9th International Conference of Music Informa-tion Retrieval,pages225–230,2008.[39]G.Tzanetakis and P.Cook.Musical Genre Classifica-tion of Audio Signals.IEEE Transactions on Speech and Audio Processing,10(5):293–302,2002.[40]D.Wang,T.Li,and M.Ogihara.Tags Better ThanAudio Features?The Effect of Joint use of Tags and Audio Content Features for Artistic Style Clutering.In11th International Society on Music Information Retrieval Conference,number ISMIR,pages57–62, 2010.[41]D.Yang and W.S.Lee.Disambiguating Music Emo-tion Using Software Agents.In Proceedings of the5th International Conference on Music Information Re-trieval,pages52–58,2004.[42]M.Zentner,D.Grandjean,and K.R.Scherer.Emo-tions evoked by the sound of music:characterization, classification,and measurement.Emotion(Washing-ton,D.C.),8(4):494–521,August2008.。
山东大学信号与系统 2013年试卷B
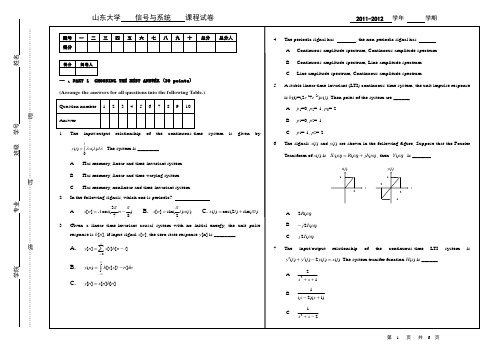
学号
3. 4. 8. For the following difference equations y[n 1] 0.8y[n] x[n] , compute y[n] for n=1,2,3, when x[ntime-invariant ]=u[n] and y[-1]=2. A linear continuous-time system has transfer function H (s)
t )2cos (t ) for x (t ) 5u (for t 1) system. 10cos(2(t 2)) , tthen (t ) t ) , find yx (t() 3t e e2tycos 21.414 t , t cos( 0 . Determine theytransfer function the 4
姓名
得分
阅卷人
B. C. CHOOSING THE BEST ANSWER (30 points)
一 、PART 1
5.
A stable linear time-invariant (LTI) continuous-time system, the unit impulse response is h(t)=(2e-t+e-2t)u(t). Then poles of the system are ______ . A. B. p1=0, p2=-1, p3=-2 p1=0, p2=-1 p1=-1, p2=-2
6e j 2 H ( ) 0 | | 8 | | 8
级
.
The input x(t ) C cos(0t ) is applied to the system for t 0 with zero initial conditions. The resulting steady-state response ySS (t ) is ySS (t ) 6cos(t 45 ), t 0 , compute C, 0 ,and
2013 CSAMSE Conference Program Chinesez国际市场营销年会
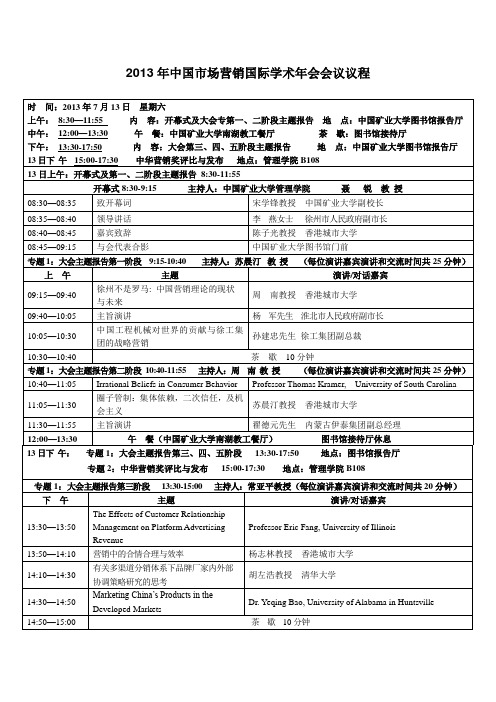
The Differential Effects of Customer Involvement on New Product Performance(英文演讲)
Fang Wu, University of Texas at Dallas
09:45—10:10
基于差距模型的服务质量的实证研究-多主体下的视角(中文演讲)
09:45—10:10
Organizational Ambidexterity and Innovation in Chinese International Services Firms(英文演讲)
Yuanyuan Zhang, City University of Hong Kong
10:10—10:30
李青
澳门城市大学工商管理系
Table 2:
圆桌讨论主持人:Sijun Wang地点:管理学院B401-1
Attendees
熊小明
中国武汉大学经济与管理学院
徐申
香港城市大学市场营销系
周雪敏
中国华中科技大学管理学院工商管理系
宁波
新西兰惠灵顿维多利亚大学
王聪
西南财经大学工商管理学院
王辉
武汉大学经济与管理学院市场营销专业
叶齐先生特步国际控股有限公司/执行董事兼副总裁
16:00—16:30
希悦尔营销案例分析
欧波先生希悦尔中国/北亚区食品与饮料业务总监
16:30—17:00
演讲
王兴元教授山东大学
17:00—17:30
颁奖环节
特邀颁奖嘉宾
欢迎晚宴13日下午18:20—20:00地点:云泉山庄大酒店
18:20—20:00
Solar Presentation for Shanghai Mar2013
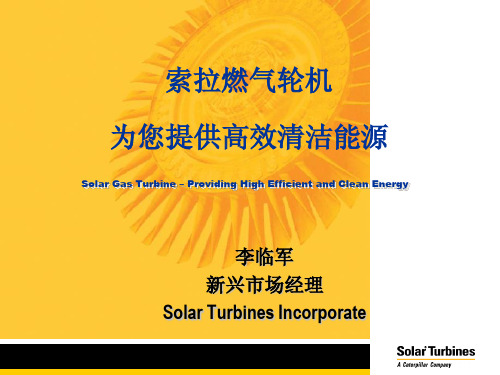
Xinjiang
Beijing
Yellow Sea
Henan
Jiangsu
East China Sea
Hubei
Anhui
Sha
Zhejiang ngh Hunan Jiangxi Fujian Taiwan Guangxi Guangdong
ai
Yunnan
Guizhou
Hainan
South China Sea
A DRIVING FORCE FOR POWER Caterpillar: Green
VPSAMPLE 10
工业及流程工艺设施
化工 Chemicals
制药 Pharmaceuticals 食品及原料 Foods and Ingredients 奶制品 Dairies and Dairy Products
VPSAMPLE 13
热电联供的特点
同时产出电能和热能 可用于蒸汽轮机或工业蒸汽
或两者并用 系统效率极高 系统可用率高 连续运行
A DRIVING FORCE FOR POWER Caterpillar: Green
VPSAMPLE 14
热电联供的热电比
1538 º C
独立加热器或蒸汽发生器+电网供电
热电联供 Combined Heat and Power
- 热电联产 Cogeneration - 联合循环 Combined Cycle - 区域供热 District Heating
基荷供电 Base Load Power 分布式发电 Distributed Power 峰值发电 Peaking Power 备用/紧急发电 Standby/Emergency
A type-A-choking-oriented unified model for fast fluidization dynamics
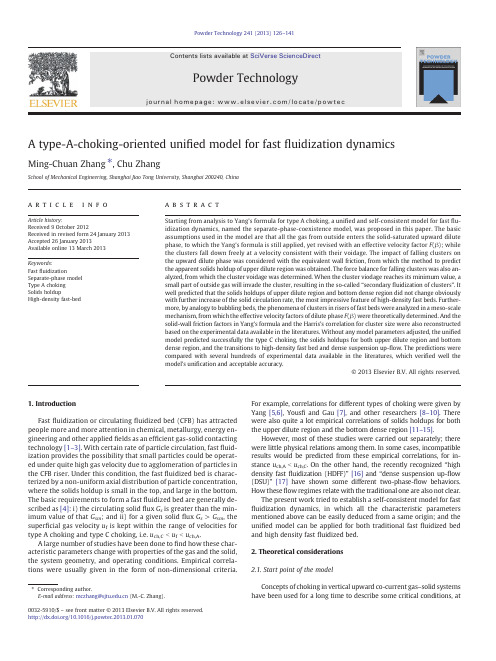
A type-A-choking-oriented uni fied model for fast fluidization dynamicsMing-Chuan Zhang ⁎,Chu ZhangSchool of Mechanical Engineering,Shanghai Jiao Tong University,Shanghai 200240,Chinaa b s t r a c ta r t i c l e i n f o Article history:Received 9October 2012Received in revised form 24January 2013Accepted 26January 2013Available online 13March 2013Keywords:Fast fluidizationSeparate-phase model Type A choking Solids holdupHigh-density fast-bedStarting from analysis to Yang's formula for type A choking,a uni fied and self-consistent model for fast flu-idization dynamics,named the separate-phase-coexistence model,was proposed in this paper.The basic assumptions used in the model are that all the gas from outside enters the solid-saturated upward dilute phase,to which the Yang's formula is still applied,yet revised with an effective velocity factor F (β);while the clusters fall down freely at a velocity consistent with their voidage.The impact of falling clusters on the upward dilute phase was considered with the equivalent wall friction,from which the method to predict the apparent solids holdup of upper dilute region was obtained.The force balance for falling clusters was also an-alyzed,from which the cluster voidage was determined.When the cluster viodage reaches its minimum value,a small part of outside gas will invade the cluster,resulting in the so-called “secondary fluidization of clusters ”.It well predicted that the solids holdups of upper dilute region and bottom dense region did not change obviously with further increase of the solid circulation rate,the most impressive feature of high-density fast beds.Further-more,by analogy to bubbling beds,the phenomena of clusters in risers of fast beds were analyzed in a meso-scale mechanism,from which the effective velocity factors of dilute phase F (β)were theoretically determined.And the solid-wall friction factors in Yang's formula and the Harris's correlation for cluster size were also reconstructed based on the experimental data available in the literatures.Without any model parameters adjusted,the uni fied model predicted successfully the type C choking,the solids holdups for both upper dilute region and bottom dense region,and the transitions to high-density fast bed and dense suspension up-flow.The predictions were compared with several hundreds of experimental data available in the literatures,which veri fied well the model's uni fication and acceptable accuracy.©2013Elsevier B.V.All rights reserved.1.IntroductionFast fluidization or circulating fluidized bed (CFB)has attracted people more and more attention in chemical,metallurgy,energy en-gineering and other applied fields as an ef ficient gas-solid contacting technology [1–3].With certain rate of particle circulation,fast fluid-ization provides the possibility that small particles could be operat-ed under quite high gas velocity due to agglomeration of particles in the CFB riser.Under this condition,the fast fluidized bed is charac-terized by a non-uniform axial distribution of particle concentration,where the solids holdup is small in the top,and large in the bottom.The basic requirements to form a fast fluidized bed are generally de-scribed as [4]:i)the circulating solid flux G s is greater than the min-imum value of that G sm ;and ii)for a given solid flux G s >G sm ,the super ficial gas velocity u f is kept within the range of velocities for type A choking and type C choking,i.e.u ch,C b u f b u ch,A .A large number of studies have been done to find how these char-acteristic parameters change with properties of the gas and the solid,the system geometry,and operating conditions.Empirical correla-tions were usually given in the form of non-dimensional criteria.For example,correlations for different types of choking were given by Yang [5,6],Yous fiand Gau [7],and other researchers [8–10].There were also quite a lot empirical correlations of solids holdups for both the upper dilute region and the bottom dense region [11–15].However,most of these studies were carried out separately;there were little physical relations among them.In some cases,incompatible results would be predicted from these empirical correlations,for in-stance u ch,A b u ch,C .On the other hand,the recently recognized “high density fast fluidization (HDFF)”[16]and “dense suspension up-flow (DSU)”[17]have shown some different two-phase-flow behaviors.How these flow regimes relate with the traditional one are also not clear.The present work tried to establish a self-consistent model for fast fluidization dynamics,in which all the characteristic parameters mentioned above can be easily deduced from a same origin;and the uni fied model can be applied for both traditional fast fluidized bed and high density fast fluidized bed.2.Theoretical considerations 2.1.Start point of the modelConcepts of choking in vertical upward co-current gas –solid systems have been used for a long time to describe some critical conditions,atPowder Technology 241(2013)126–141⁎Corresponding author.E-mail address:mczhang@ (M.-C.Zhang).0032-5910/$–see front matter ©2013Elsevier B.V.All rights reserved./10.1016/j.powtec.2013.01.070Contents lists available at SciVerse ScienceDirectPowder Technologyj o u r na l h o me p a g e :ww w.e l s e v i e r.c o m /l o c a t e /p o w t e cwhich the two-phase flow system cannot run properly or ef ficiently.For systems with different application purposes,the de finitions may also be different.In a pneumatic conveying system,the choking is usually de-fined as the onset of particle precipitation downward,which makes the transportation less ef ficient.However,it doesn't breach the system operation as a whole.On the opposite,in a CFB riser the choking is usu-ally de fined as a critical condition,at which a small decrease of operat-ing gas velocity or increase of circulating solid flux will cause signi ficant increase of bed pressure drop,leading to collapse,to some extents,of the whole system.Differences of the two types of choking did not get enough attention until Bi and Grace [18],where the former was de fined as the accumulative choking or type A choking,and the latter as the classical choking or type C choking.Predictions to type A choking and type C choking are obviously im-portant for fast fluidization,since they will determine the allowable ranges for gas velocity or solid circulation flux.There were quite a lot of formulas developed in the past to correlate the gas velocities and the solid fluxes at the two types of choking.However,only one of them can be chosen as the start point of the uni fied model,while the other should be deducted from the chosen one.Considering the re-search history for pneumatic conveying system is much longer than that for fast fluidized bed,and the two-phase flow structure in the for-mer is much simpler than that in the latter,it is believed that the theo-retical and experimental bases for type A choking are more reliable and universal.Among the numerous formulas existed in the literatures for type A choking,the form of Yang's formula [5,6]looks the best,since deriva-tion of the formula involves only two theoretical deductions.The first one is that the terminal velocity of particles for a uniform suspension of voidage εin a riser of diameter D t can be calculated from Eq.(1).u 0t ¼u t ffiffiffiffiffiffiffiffiffiffiffiffiffiffiffiffiffiffiffiffiffiffiffiffiffiffiffiffiffiffiffiffiffiffiffiffiffiffiffiffi1þf p u 2pt!Âε4:7v u u t ð1ÞWhere,u p =G s /[ρs (1−ε)]is the particle velocity,while f p stands for the solid-wall friction factor.This is a theoretically logical formula,and detailed derivation and discussion on that can be found in Yang's series work from 1973to1975[5,19,20].The second deduction is when type A choking happens,the slip velocity between gas and solid,i.e.the terminal velocity of a particlesuspension in a finite diameter riser u t',is just equal to the terminal velocity of a single particle in the in finity u t [5,6].u 0t ¼u tð2ÞThis deduction was actually used in the derivation of Yang's pre-dictive equation [5,6](Eq.(3)),but was not clearly declared and explained in his work.2D t g ε−4:7ch −1 u ch −u t ðÞ2¼f p ð3ÞThe followings are the present authors'try to explain “what does the deduction really mean?”It can be seen from Eq.(1),the first item withsquare root sign ffiffiffiffiffiffiffiffiffiffiffiffiffiffiffiffiffiffiffiffiffiffi1þf pu 2p2gD tr represents the in fluence of wall friction onu t',since it will always be unity in an in finitely wide riser.When D t gets smaller,the wall friction gets greater,then u t'gets greater.The second item with square root sign ffiffiffiffiffiffiffiffiε4:7p represents the in fluence of bed voidage,i.e.the in fluence of surrounding particles.When the particleconcentration (1−ε)gets greater,εgets smaller,then u t 'gets smaller.The reason is that the surrounding particles will cause both increase ofreal gas velocity ffiffiffiffiffiε2pand more flexuous flow-pass of gas around theparticle,then increase of the drag force coef ficient ffiffiffiffiffiffiffiffiε2:7p[21].There-fore,the amendment of u t 'is on the basis of super ficial gas velocity.If we resolve the increased fluid drag on one particle in a uniform suspension into the basal fluid drag on a single particle F f and the sur-plus F s due to the surrounding particles,the overall force balance for the suspension,or a single particle in a long time duration,can be expressed asF f þF s ¼G þW ð4Þwhere,G stands for the gravity of particle or particle suspension,whileW for the wall friction.Comparing the values of F s and W ,two different situations can be distinguished.For a relatively dilute suspension,the drag force caused by the surrounding particles is relatively small,resulting in F s b W ,and then F f >G .The fluid drag given by the gas solely F f is greater than the gravity of the particle,which means that besides supporting the particle there will be something rest to balance the wall friction,simply shown as F f −G =W −F s .This will be a relatively simple dy-namic system centered on each single particles;the relatively indepen-dent movement of these particles will lead to a uniformly dispersed gas-solid two phase flow,i.e.the custom dilute suspension transporta-tion.On the opposite,if we have F s >W and F f b G ,the fluid drag given by the gas F f will no longer be able to support the particle gravity solely,but needs something else from the surrounding particles,simply shown as F f +(F s −W )=G .Then the force balance for any particle will depend more on the others,making the dynamic system more complex and easier to lose its uniformity.For instance,an occasionally local condensation of particles will result in less gas flowing through them and decrease of F f ,which will make these particles to move close further to increase F s as the overall force balance needs.This may probably be the physical reason of cluster formation for the case of F s >W .Therefore,what can be used to separate the two different types of flows mentioned above is just the criteria of F s =W or F f =G ,i.e.u t'=u t from their de finitions.It means that the in fluence of finite riser diameter D t on u t'is just compensated by the in fluence of bed voidage εat the type A choking.At this unique condition,the moving particle looks as if there is neither wall nor surrounding particles.The authors suggest that this is the real meaning of the deduction,and then can be seen as the physical essence of type A choking.Just because of its sound physical meaning,the relationship given by Eq.(3)for the super ficial gas velocity and the bed voidage under solid-saturated conditions (type A choking)can be considered as “inherent ”,then the equation as “constitutive equation ”.However,it should also be pointed out here,the functional relation of u ch and εch is the most important in Eq.(3),but not the value of f p at this moment.Actually,the solid friction factor f p =0.01was taken originally by Yang in 1975[5],and changed later to the present form in 1983[6]f p ¼6:81Â105ρgρs2:2:ð5ÞThe rationality or accuracy of above mentioned values for f p will bediscussed in detail and re-correlation of f p with more experimental data will be made later in Section 3.3.For the time being,the solid fric-tion factor used in the calculations before Section 3.3was f p =0.01,the value given in 1975[5],since it was better than the other according to the accuracy evaluation of Xu et al.[10].2.2.Physical description of the modelAs we discussed above,Eq.(3)shows the relationship of super ficial gas velocity u ch and bed voidage εch under solid-saturated conditions,127M.-C.Zhang,C.Zhang /Powder Technology 241(2013)126–141i.e.type A choking.For a given fluidizing system,when u ch increases,εch will decrease;then the particle concentration (or solids hold-up)(1−εch )increases,and the saturation carrying capacity of gas G s ⁎will increase even faster.G Ãs ¼ρs u ch −u t ðÞ1−εchεchð6ÞThe equation derived here is a little bit different from what was used in Yang's articles [5,6]by 1/εch ,since the modi fication of ffiffiffiffiffiffiffiffiε4:7p includes also the in fluence of the gas velocity ffiffiffiffiffiε2p ,then the real par-ticle velocity is (u ch −u t )/εch .As an example,Fig.1shows the cal-culated results of (1−εch )and G s ⁎varied with u ch for a FCC-air fluidizing system.It can be seen from the figure that the saturation carrying capacity G s ⁎varies with the super ficial gas velocity u ch in an exponential form with power >1.Just because of this special relationship between G s ⁎and u ch ,one can imagine when the circulating solid flux is greater than G s ⁎at a given gas velocity,the system will not completely collapse but run in a more complicated separate-phase-coexistence mode.Some par-ticles will segregate from the gas stream and get agglomerated to form a free-falling dense phase (clusters),which occupies a part of the riser cross-sectional area,but without outer gas getting in;while in the rest of the riser,more concentrated gas with higher ve-locity could carry even more particles upward in the form of dilute phase.Without outer gas getting in clusters is a logical deduction from two-way stability analysis,and is coincident with Mueller and Reh's investigation [22],i.e.“the acceleration of particle inside the strand (cluster)is equal to the acceleration of free fall,which implies that no drag force acts on the particle within the strand ”[21].Let βbe the cross-sectional area fraction occupied by the falling dense phase or clusters,which is also the volume fraction of randomlydistributed clusters;m s−as the corresponding solid flux downward,and m s +as the solid flux upward in solid-saturated dilute phase.Both m s+and m s −are de fined on the basis of the total cross-sectional area,but not their own occupied.Then,the solid flux circulating into the riser G s can be expressed as G s ¼m þs −m −s :ð7ÞThe sketch map for m s +and m s −varied with βat a constant gas velocity is shown in Fig.2.It can be seen from the figure,if we haved m þs d βj β¼0>d m −sd βj β¼0;ð8Þthe separate-phase-coexistence mode can really transport more par-ticles upward in the riser than the saturation carrying capacity ofgas at type A choking,i.e.G s =m s +−m s −>G s ⁎.This will be trueuntil a critical point β=βch is reached,where d m þs d βj β¼βch ¼d m −sd βj β¼βch;ð9Þand the transportable solid flux at the given gas velocity takes its max-imum value G s,max .Beyond this point the separate-phase-coexistence mode has no more ability to balance the excess solid flux,leading to the system being totally “collapsed ”,i.e.the type C choking.The analysis above indicates also that the critical requirement for separate-phase-coexistence mode is d m þs d βj β¼0¼d m −sd βj β¼0:ð10ÞThis criterion can be used for determination of G sm ,which will bediscussed later elsewhere.As a sum,the key points af firmed or the basic assumptions to be used in the uni fied model are as follows:i)the relationship between bed voidage εch and gas velocity u ch given by Yang's formula for type A chok-ing can be seen as “inherent ”or “constitutive ”for solid-saturated dilute phase,which provides the theoretical basis of the model;ii)with neces-sary amendment,this relationship can also be applied for the solid-saturated upward dilute phase when coexisted separate-phases appear;and iii)in the separate-phase-coexistence mode,all the gas from outside enters the solid-saturated upward dilute phase,while the clusters fall down freely at a velocity consistent with their voidage (ex-cept for HDFF or DSU).3.Mathematical model kernels3.1.Model kernels3.1.1.Dilute phase modelAs discussed above,at type A choking,the very beginning of the separate-phase-coexistence mode (β=0),the gas –solid slip velocity can take the value of terminal velocity of a single particle u t .When separate phases visibly appear,due to the impact of falling clusters,it is expected that the saturation carrying capacity per unit gas in the dilute phase will be less than that for β=0.It means that the gas –solid slip velocity in this case has increased.However,this im-pact can also be expressed by decrease of the effective gas velocity in dilute phase,while keeping the slip velocity unchanged.This can123456G *s (k g /m 2s )u ch (m/s)1-c hεFig.1.Saturated carryings G s ⁎and solid concentration (1−εch )at different gas velocity u ch (FCC –air system,ρs =1620kg/m 3,d p =100μm,D t =0.1m).G s ,m sG *schββFig.2.Sketch map for m s +and m s −varied with βat constant gas velocity.128M.-C.Zhang,C.Zhang /Powder Technology 241(2013)126–141be easily done by using an effective velocity factor of dilute phase F (β)b 1in the calculation.Thus,the upward solid flux based on unit dilute phase area can be expressed as G þs ¼ρsu f F βðÞ−u t !1−εch εch ;ð11Þand the relationship between the calculation velocityu Ãch¼u f F βðÞ1−βand the solid-saturated dilute phase viodage εch still fits the revised Yang's formula,as we discussed above.Therefore,the super ficial up-ward solid flux de fined on the basis of the total cross-sectional area of the riser will be m þs ¼ρs u f F βðÞ−u t 1−βðÞ½1−εchεch:ð12ÞFrom the analysis above,the function F (β)chosen should meet the requirements of F βðÞj β¼0¼1;andd F βðÞd βjβ¼0¼0:ð13ÞThe simplest form of that is F βðÞ¼1−c βn:ð14ÞAs βincreases,the impact of falling clusters gets greater andgreater.The upward solids flux of dilute phase m s+will reach its max-imum,then decrease gradually until slugging occurs at β=1.Sincethe solid flux m s+at slugging is still finite but not zero,it can be roughly estimated that m s +|β=1=G s ⁎,i.e.the saturation carryingcapacity of gas at type A choking G s ⁎(see Fig.2).From that,we haveρs u f F 1ðÞ½ 1−εslεsl ¼ρs u f −u t ½ 1−εch ;A εch ;Að15Þwhere F (1)is the value of F (β)at β=1;εsl and εch,A are the bedvoidages for slugging (β=1)and type A choking (β=0),respec-tively.Therefore,F 1ðÞ¼1−u t f1−εch ;A εch ;A εslsl:ð16ÞSuppose the solid span equals to the gas span when slugging occurs,and the voidage equals εmf in the solid span,then we have the average asεsl ¼1−1−εmf ðÞ=2:ð17ÞFinally,the effective velocity factor of dilute phase F (β)can be calcu-lated asF βðÞ¼1−1−F 1ðÞ½ βn:ð18Þ3.1.2.Dense phase modelThe sub-model for dense-phase or falling clusters is relatively simple in form,i.e.m −s ¼ρs βu cl 1−εcl ðÞ:ð19ÞAs we mentioned above,the falling velocity of clusters u cl should be in consistence with their voidage εcl to keep the outside gas flow within the cluster being zero.Therefore,the modi fied Richardson –Zaki'sequation [23]must be satis fied for the force balance of gas-particles in-side of clusters,i.e.u cl ¼u tεcl1m¼u t εm cl :ð20ÞHere,m ¼lg u mfu t=lg εmf ;ð21Þand εmf =0.45was used in the following calculations.However,to determine both εcl and u cl ,a supplementary condi-tion is needed.This will be discussed later in Section 5.1,i.e.the weight of clusters should be balanced by the inter-phase drag,which can be solved with other parameters together through itera-tions.Nevertheless,for the initial value of εcl with iteration or a sim-pli fied calculation (no iteration)a rough estimation is still needed.According to the experimental data collected by Harris and Davidson [24],the solid concentration in clusters can be seen approximately twice of that in the dilute phase.Then,we have εcl ;0¼1−21−εch ðÞ¼2εch −1:ð22Þ3.1.3.Empirical estimation of model parameter nAccording to the derivations above,the model equations can be used in following procedures.i)The type A choking velocity u ch,A is calculated for a given solids flux G s (>G sm ).ii)Decrease super ficialgas velocity to make u f b u ch,A ;then,m s+and m s −are calculated by using different βuntil G s =m s +−m s −is satis fied;the voidage of upward dilute phase εch at the operating velocity u f is then deter-mined.iii)Repeat the steps above until type C choking occur;the type C choking velocity u ch,C is finally ing a different model parameter n ,the variations of dilute phase voidage εch with operating gas velocity u f for a FCC –air system (D t =0.1m,the typ-ical diameter of laboratory scale risers)are shown in Fig.3.It can be seen from the figure,when the operating gas velocity u f is close to u ch,C ,a small reduction of gas velocity will cause a great increase of bed concentration (1−ε).That is the characteristic feature of type C choking.It can also be seen from the figure,as n gets greater,the type C chok-ing velocity calculated gets smaller.Then,the empirical correlation0.920.930.940.950.960.970.980.991.00u f (m/s)u ch,CεFig.3.Variations of dilute phase voidage εch with gas velocity u f calculated from different n for a FCC –air system (D t =0.1m,ρs =1620kg/m 3,d p =100μm,G s =100kg/(m 2s)).129M.-C.Zhang,C.Zhang /Powder Technology 241(2013)126–141given by Yous fiand Gau [7](Eq.(23)),which was veri fied to be the best for type C choking [10],can be used to estimate the proper value of n .u ch ;C ffiffiffiffiffiffiffiffigd pq ¼32Re −0:06tG s g u ch ;C!0:28ð23ÞFrom this kind of “calibration ”,the model parameter n =4.5can be chosen for a simpli fied version of the model without iteration.More fundamental determination of the model parameter n will be given in detail in Section 3.2later.Fig.4shows the comparison be-tween the model predictions with n =4.5and those given by Eq.(23),for both FCC –air and sand –air systems with different parti-cle sizes (50,100,150and 200μm)and solid fluxes (50,100and 200kg/(m 2s)).The result looks quite satisfactory.3.2.Mechanistic determination of F(β)and nThe wake effect was used quite often in the literature to explain how the cluster was formed in a CFB riser.For example,Basu et al.stated in their Book [25],“For a given velocity,the feed rate may be increased to a level where the solid concentration will be so high that one particle will enter the wake of the other.When that hap-pens,the fluid drag on the first particle will decrease,and it will fall under gravity to drop on the trailing particle.The effective surface area of the pair just formed is low,and so the fluid drag will be lower than their combined weight,making the pair fall further to collide with other particles.Thus an increasing number of particles combine together to form particle agglomerates known as clusters.These clusters are,however,not permanent.They are continuously torn apart by the up-flowing gas.Thus,the formation of clusters and their disintegration continue.”Though most of these words were actually from analytical consequence only,the description was quite reasonable.Recently,He et al.carried out an excellent PIV measurement for particle movement in a CFB riser [26]showing clearly the details of this phenomenon as in Fig.5(a)and (b).They described in their arti-cle,“it can be easily seen that a cluster is followed by a wake,in which particles move downward quickly ”,and “when a cluster is passing by,the particles are dragged down at a higher velocity ”.Be-sides a very clear veri fication of the wake effect,the measurement showed that those particles moved towards the cluster at velocitiesof the same order for up-flowing ones,which will result in a notable deposition on the back side of the cluster.And due to the size limitation for a stable cluster,there must be the same quantities of particles pouring out the cluster from its nose.The continuous deposition and pour out of those particles will cause the downward displacement of particles inside the cluster,too.The particles moving towards the clus-ter in the wake,the inside displacement,and the front pour out can then be viewed as an integrated penetration of these particles through the cluster.From this point of view,the phenomenon can be analogous to what happens around a rising bubble in a bubbling bed as shown in Fig.1(c)[27].It can be seen that except the directions are opposite-down,the flow patterns of the two are quite similar.Furthermore,through phase reversal the following correspondences could be easily af-firmed.(1)A falling cluster with concentrated particles vs.a rising bubble with null or few particles;(2)the upward dilute flow around the cluster vs.the downward dense particle flow around the bubble;(3)the downward penetrating particle flow through the cluster vs.the upward penetrating gas flow through the bubble.The scenarios in CFB risers described above are quite in consistent with the results from detailed numerical simulations by combining the two-fluid model with the EMMS approach [21],i.e.“the particles tend to enter into clusters instead of suspending in dilute broth (phase),whereas the gas tends to pass around,instead of penetrating through,the dense cluster phase.”[21]Then,some results obtained for bubbling beds may also be used to estimate the in fluence of fall-ing clusters on the upward dilute phase,as explained below.Suppose that a spherical cluster of voidage εcl falls down in a di-lute suspension with a constant pressure gradient d p /dz =−J ,i.e.the pressure drop per unit riser height which can be calculated in the way described in Section 4.1below.If we put the cluster in a sur-rounding bed with the same voidage εcl ,the through flow penetrat-ing the cluster u fu under the pressure gradient −J would be the same as that in the surroundings.On the other hand,the cluster is also penetrated by a particle flow from the opposite direction,which results in a downward displacement of particles at u sd inside the cluster,as we discussed above.To keep the gas flow inside the cluster being null,which is a primer assumption for the model estab-lishment,there must be u sd =u fu .Just because the null flows inside of cluster,the pressures everywhere inside are constant.Therefore,the isobars above and below the cluster will get condensed,which will then suck more gas flowing through the cluster.Though the cluster is full of particles,it functions as an empty bubble.According to the well-known Davidson's model for a single bubble immerged in an incipiently fluidized bed [27],the total volume flow-rate through the cluster is q ¼3u pen πR 2clð24Þwhere R cl stands for the radius of the cluster,and u pen stands for the super ficial percolation velocity in a packed bed of voidage εcl under the pressure gradient −J .It can be easily calculated by the Ergun's equation or the extended Ergun's equations [21,28],i.e.Ergun's for εb 0.8and Wen's for ε≥0.8.Then the interstitial gas velocity through the cluster,which will be counteracted finally by the down-ward displacement of particles inside,can be written asu sd ¼u fu ¼3u pen =εcl :ð25ÞIn bubbling fluidized beds [27],rising bubbles can be classi fied as the fast bubble or the slow bubble,according to the ratio of the bub-ble rising velocity u br to the interstitial gas velocity far away from the bubble u f,∞.For a fast bubble [27],i.e.u br >u f,∞,the gas leaves at the top of the bubble will be sucked in again from the bottom;the1.01.52.02.53.03.54.0u c h ,C (m /s ,t h i s m o d e l )u ch,C (m/s,Yousfi & Gau)parison of model predictions for type C choking (n =4.5,D t =0.1m)and those from Yous fiand Gau [7].130M.-C.Zhang,C.Zhang /Powder Technology 241(2013)126–141。
法语综合教程1课后练习答案L15

Leçon 15Corrigé d’exercicesDialogueCompréhension du texteI. Vrai ou faux ?1. Faux2. Vrai3. Vrai4. Faux5. VraiII. Relisez le dialogue et répondez :1. Parce qu’elle vient d’acheter un ordinateur portable.2. P arce que l’ordinateur portable est pratique. O n peu t l’emporter où l’on veut et o n peutl’utiliser ici ou ailleurs. 3. Elle veut découvrir Internet. 4. Oui, il peut l’a ider.5. Oui, le vendeur lui a appris à allumer son portable.III. Activité orale :Réponse libre.TexteC ompréhension du texteI. Vrai ou faux ?1. Faux2. Vrai3. Faux4. Vrai5. VraiII .Liez selon le texte et faites des phrases :avoir un ordinateurbavarder avec des gens inconnus oublier de manger et de dormir cliquer sur des icônespasser des heures et des heures devant l’ordinateur communiquer avec le monde entierIII. Répondez aux questions suivantes :Réponse libre.L e passé composé de l’indicatif avec l’auxiliaire « avoir »(以avoir 为助动词的直陈式复合过去时)E xercices de grammaireI. Liez l’infinitif et son participe passé :1.être - été 2. avoir - eu3. attendre - attendu4. faire - fait5. voir - vu6. entendre - entendu7. vouloir - voulu8. pouvoir - pu9. servir - servi 10. envoyer - envoyéII. Donnez les infinitifs correspondants aux participes passés suivants :1.appris → apprendre2.écrit → écrire3.su → sa voir4.ouvert → ouvrir5.vendu → vendrepris → comprendre7.mis → mettre8.pris → prendreIII. Faites des phrases selon les modèles :Modèle A :1.J’ai mal entendu.2.Il a bien parlé.3.Jean a trop bu.4.Je l’ai déjà su.5. Il a toujours été là.Modèle B :1.Anne n’a rien répondu.2.Tu n’as rien vu ?3.Vous n’avez rien fait.4.Ils n’ont rien su.5.Je n’ai rien entendu.Modèle C :1.Nous avons eu une voiture.2.Le vendeur m’a appris à allumer l’ordinateur.3.J’ai acheté un téléphone portable.4.Joëlle et Philippe ont bavardé pendant une heure sur Internet.5.On a pu envoyer des photos à son ami par e-mail.Modèle D :1.Oui, parce que je n’ai pas encore lu ce magazine./ Oui, parce que je ne l’ai pas encore lu.2.Oui, parce qu’il ne lui a pas encore téléphoné. / Oui, parce qu’il n’a pas encore eu letemps de lui téléphoner.3.Oui, parce que je n’ai pas encore vu ce film./ Oui, parce que je ne l’ai pas encore vu.4.Oui, parce que je n’ai pas encore pris de thé./ Oui, parce que je n’en ai pas encore pris./Oui, parce que je n’y ai pas encore pensé.5.Oui, parce qu’elle ne lui a pas encore dit bonjour./ Oui, parce qu’elle ne l’a pas encorerencontré aujourd’hui.IV. Mettez les verbes entre parenthèses au passé composé :1. a été2.(j’) ai lu3.avons (déjà) appris4. a eu5.(n’en) ai (pas) p ris6.(N’)as-tu (pas) trouvé7.as acheté8. a envoyé9.m’a (très vite) répondu (très vite)10.a ouvertV. Posez des questions avec le passé composé :1.Avez-vous dit bonjour à M. Dupont ? (As-tu dit bonjour à M. Dupont ?)2.Avez-vous aidé votre mère à préparer le dîner ? (As-tu aidé ta mère à préparer le dîner ?)3.A-t-il bavardé avec son ami pendant une demi-heure au téléphone ?4.Hier, avez-vous passé une heure à regarder la télé ? (As-tu passé une heure à regarder latélé ?)5.Avez-vous oublié d’envoyer vos photos à Martine ? (As-tu oublié d’envoyer tes photos àMartine ?)L’accord dans le passé composé avec l’auxiliaire « avoir »(以avoir为助动词的复合过去时中的配合问题)E xercices de grammaireI. Refaites les phrases suivantes d’après le modèle :1.Leur ami, ils l’ont présenté à leur professeur.2.Ces chaussures, je les ai achetées au supermarché.3.Ces romans, j’en ai lu seulement trois.4.Ces films, les avez-vous vus ?bien en avez-vous, des cassettes ?II. Répondez aux questions suivantes :1.Oui, je l’ai déjà mise sur votre pupitre. / Non, je ne l’ai pas encore mise sur votre pupitre.2.Oui, elle l’a déjà reconnue. / Non, elle ne l’a pas encore reconnue.3.Oui, ils les ont déjà vus. / Non, ils ne les ont pas encore vus.4.Oui, elle l’a déjà préparé. / Non, elle ne l’a pas encore prépa ré.5.Oui, je les ai déjà faits. / Non, je ne les ai pas encore faits.6.Oui, il lui en a déjà raconté. / Non, il ne lui en a pas encore raconté.7.Oui, elles l’ont déjà lue. / Non, elles ne l’ont pas encore lue.8.Oui, nous l’avons apprise pendant trois mois. / N on, nous ne l’avons pas apprise pendanttrois mois.9.Oui, je l’ai attendue hier. / Non, je ne l’ai pas attendue hier.10.Oui, je l’ai déjà visitée. / Non, je ne l’ai pas encore visitée.L e pronom neutre « le »(中性代词le)E xercices de grammaireI. Remplissez les blancs par un pronom convenable :1.le3.le4.l’5.l’6.le7.le8.l’9.l’10.lesII. Distinguez les fonctions grammaticales des pronoms soulignés :1.pronom neutre2.pronom COD3.pronom neutre4.pronom neutre5.pronom COD6.pronom COD7.pronom neutreE xercices de vocabulaireI. Complétez les phrases avec les adjectifs suivants :1.ravi(e)2.pratique3.extraordinaire4.GénialpliquéeII. Mettez les verbes suivants au présent de l’indicatif :A.1.servir2.sert3.sert4.se servir5.se sertB.1.apprenons2.(J’)app rends3.apprend4.apprend5.apprendreC.1.jouer2.jouer3.jouer4.jouer5.jouentD.1.passer2.passer3.passe4.passe5.passeE.1.(J’)attends2.attend3.Attends / Attendez4.attendre5.attendIII. Associez les mots ou expressions suivants et puis faites des phrases : avoir l’air contentemporter un téléphone mobilecliquer sur cette icôneconnecter l’ordinateur à Internetenvoyer des messagescommuniquer avec des amisoublier de manger et de dormirIV. Remplacez les points par une préposition :1.de, en, sur2.à, avec3.à, par, de4.sur5.sur, sur, de, à, aux6.à, avec7.à8.deV. Dites les nombres ordinaux en français :1er : premier2ème : deuxième 5ème : cinquième 7ème : septième 9ème : neuvième 3ème : troisième 6ème : sixième 4ème : quatrième10ème : dixième8ème : huitième20ème : vingtième31ème : trente et unième 15ème : quinzième101ème : cent unièmeE xercices de structureI. Faites de petits dialogues selon le modèle :1. A : Je veux ouvrir la radio.B : Mais comment ouvrir la radio, tu sais ? / Mais tu s ais comment l’ouvrir ?A : Oui, Jacques me l’a déjà dit.2. A : Je veux photographier le coucher du soleil.B : Mais comment te servir de l’appareil photo, tu sais ? / Mais tu sais comment t’enservir ?A : Oui, Paul me l’a déjà appris.3. A : Je veux chercher des informations sur Internet.B : Mais comment surfer sur Internet, tu sais ? / Mais tu sais comment y surfer ?A : Oui, Martin me l’a déjà démontré.4. A : Je veux envoyer un e-mail à Fanny.B : Mais comment lui envoyer l’e-mail, tu sais ? / Mais tu sais comment le lui envoyer ?A : Oui, je l’ai déjà appris.5. A : Je veux scanner une photo.B : Mais comment scanner la photo, tu sais ? / Mais tu sais comment la scanner ?A : Oui, mon père me l’a déjà expliqué.II. Répondez aux questions avec « en » :1.Oui, j’en ai pris./ Non, je n’en ai pas pris.2.Oui, il lui en a posé. / Non, il ne lui en a pas posé.3.Oui, elle en a mangé. / Non, elle n’en a pas mangé.4.Oui, nous en avons acheté. / Non, nous n’en avons pas acheté.5.Oui, ils en ont voulu. / Non, ils n’en ont pa s voulu.III. Faites des phrases au passé composé avec les éléments suivants :1.Ce matin, il a fallu partir très tôt.2.Dimanche dernier, nous avons joué au ping-pong.3.Il y a quelques minutes, le facteur a sonné à la porte. / Le facteur a sonné à la porte il y aquelques minutes.4.Hier, vous avez pris un taxi pour aller à l’école.5.Il a plu pendant deux jours.6.Mon ami et moi, nous avons regardé la télé de six heures à dix heures.7.Ma sœur a dormi jusqu’à midi.8.Ils ont communiqué en ligne sur Internet avant-hier.IV. Posez des questions sur les mots soulignés :1.Qu’est-ce que vous avez eu comme cours hier ?ment ont-ils compris la grammaire de cette leçon ?3.Quand/En quelle année Marc a-t-il voyagé en Chine ?4.Qui a déjà fait de grands progrès dans ses études ?bien de romans a-t-elle lus le mois dernier ?6.Qu’a-t-il fait hier soir ?E xercices audio-orauxI. Écoutez et remplissez les blancs :Projet de vacancesHier, après le dîner, p apa m’a regardé, l’air content , et il m’a dit : « Écoute bien, cette année, nous allons dans le Midi pour passer les vacances. J’ai l’adresse d’une villa à louer près de Plages-les-Pins. Trois pièces, eau courante (自来水) , électricité.- Eh bien, mon chéri, a dit maman, ça nous paraît une très bonne idée .- Chic ! ai-je dit et applaudi (鼓掌), parce que quand on est content, c’est dur de rester assis .- Alors, peut-on voir la mer de la villa ? a demandé maman.- Non, mais la mer n’est pas très loin , à deux kilomètres, nous a expliqué papa.- Mais j’ aimerais voir la mer de la fenêtre de ma chambre, ai-je crié.- Ah ! bon, peut-être on peut aller à Bains-les-Mers, a dit maman, en Bretagne. »Papa a hésité un moment, enfin il a répondu : «D’accord , je vais voir s’il y a encore des chambres.- Pas la peine (不必了), mon chéri, a dit maman avec un sourire , c’est déjà fait. Nous avon s la chambre 29 à l’Hôtel Beau R ivage, face à la mer , avec salle de bains .- Oh ! quelle surprise pour moi ! »II. C’est vrai ou faux ?1.vrai2.vrai3.faux4.vrai5.faux6.fauxIII. Parlez de votre propre projet de voyage :Réponse libre.E xercices de traductionI. Traduisez les mots et locutions :看起来高兴avoir l’air content了解一些网络知识avoir des connaissances sur Internet用鼠标双击图标cliquer deux fois sur une icône avec la souris 等待做某事attendre de faire quelque chose网上冲浪surfer sur Internet硬盘un disque dur键盘un clavier优盘une clé USB扫描仪un scannerII. Rendez les phrases en français :1.Je viens d’acheter un ordinateur, c’est compliqué(i l a l’air compliqué). Mais quandl’ordinateur est connecté àInternet, c’est extraordinaire (Mais une fois connectéàInternet, il devient extraordinaire).2.Hier, j’ai envoyé deux e-mails (courriers électroniques/courriels) à mon frère.3.Paul aime jouer aux jeux vidéo. Hier soir, il a joué jusqu’à dix heures et il a oublié defaire ses devoirs.4.- Je ne sais pas comment me servir de l’ordinateur, peux-tu m’aider ?- D’accord, pas de problème.5.J’ai entendu dire qu’il a passé toute la nuit à bavarder avec son ami (ses amis/son amie)sur Internet dimanche dernier.课文参考译文有一台笔记本电脑太好了!Dialogue(马蒂娜和菲利普之间的对话)菲利普:哟!你看上去很高兴嘛,马蒂娜!马蒂娜:是啊,菲利普,我太开心了!看!菲利普:是什么呀?哎哟,你买了一台笔记本电脑啊!马蒂娜:是的,我昨天买的。
- 1、下载文档前请自行甄别文档内容的完整性,平台不提供额外的编辑、内容补充、找答案等附加服务。
- 2、"仅部分预览"的文档,不可在线预览部分如存在完整性等问题,可反馈申请退款(可完整预览的文档不适用该条件!)。
- 3、如文档侵犯您的权益,请联系客服反馈,我们会尽快为您处理(人工客服工作时间:9:00-18:30)。
WHY STUDY ECONOMICS AT UQ?
Economics has been part of the University of Queensland’s curriculum since its foundation in 1910 We have over 65 academic staff from over 25 different countries and 1,400 students from more than 40 different countries. Our honours students have been accepted into the Harvard PhD program with full scholarships two years in succession In 2011 academic staff from our school were recognised for excellence in teaching at the UQ excellence in Teaching and Learning Awards and also by the Australian Learning and Teaching Council. In January 2012 the UQ School of Economics was ranked the top department of economics in Australia and 47th in the world (RePec).
Founding member of the National Group of Eight (Go8) Universitas 21 Member
OUR STUDENTS
Our diverse and vibrant community is made up of over 45,400 students, with more than 11,300 international students enrolled from 134 countries. Who are they?
Top 10 in the Asia Pacific – Shanghai Jiao Tong Academic Ranking of World Universities 2011 Top 100 – Times Higher Education World University Rankings 2011
Undergraduate Postgraduate coursework Postgraduate research Non-award 71.4% 18.1% 8.7% 1.7%
Where do they come from?
Domestic
International
75%
25%
FOUR VIBRANT CAMPUSES
Student support
UQ provides a professional service in the areas of student advice, careers and employment, personal counselling, financial assistance & disability support
LEADING RESEARCH UNIVERSITY
UQ recognises the pursuit of excellence in both research and research training as being central to its mission. UQ has created more world-class research institutes than any other Australian university. Students have the opportunity to study with researchers of international renown. UQ leads industry collaboration and research funding in Australia. Undertaking a research higher degree (PhD or Master of Philosophy) at UQ allows you to pursue cutting-edge research at our state-of-theart facilities. UQ is one of the top three research universities in the country.
SEVEN FACULTIES
Arts (Humanities) Science Business, Economics and Law Engineering, Architecture and Information Technology Health Sciences Natural Resources, Agricultural and Veterinary Sciences Social and Behavioural Sciences
WORLD CLASS FACILITIES
UQ’s state-of-the-art Library, unsurpassed information technology support and superior teaching facilities are key to unlocking learning potential.
Library The largest research collection in Queensland with millions of books; tens of thousands of journals, eBooks and multimedia; networked databases; and primary material.
STUDENT SUPPORT
Getting Started & Orientation sessions
designed to assist students to settle into University life by creating awareness of the various services and activities available
UQ’s campuses are renowned as among the most beautiful and well-equipped in Australia. UQ ST LUCIA With its distinctive sandstone buildings, jacaranda trees and extensive riverside grounds, UQ St Lucia has a reputation as one of Australia’s most attractive university campuses. UQ IPSWICH Magnificently restored heritage-listed buildings have been blended with the latest technology to create this campus of the future. UQ GATTON Set on 1068 hectares and just under an hour’s drive west of Brisbane, UQ Gatton links the future leaders of Australia’s rural industries with the latest technology and learning. UQ HERSTON Herston is UQ’s major clinical health teaching and research location. The campus is close to Brisbane’s CBD and is located alongside the Royal Children’s Hospital and Royal Brisbane and Women’s Hospital.
Accommodation services
provides information and services to assist you to find a ome that's right for you, and make your stay as smooth as possible