A framework for automatic clustering of
国际自动化与计算杂志.英文版.
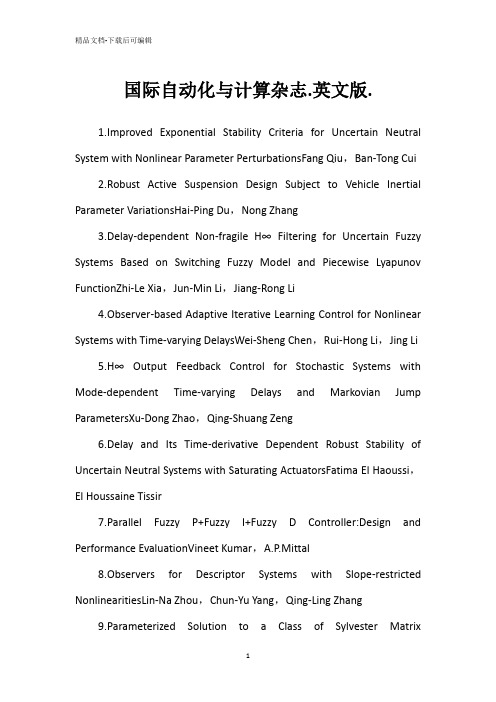
国际自动化与计算杂志.英文版.1.Improved Exponential Stability Criteria for Uncertain Neutral System with Nonlinear Parameter PerturbationsFang Qiu,Ban-Tong Cui2.Robust Active Suspension Design Subject to Vehicle Inertial Parameter VariationsHai-Ping Du,Nong Zhang3.Delay-dependent Non-fragile H∞ Filtering for Uncertain Fuzzy Systems Based on Switching Fuzzy Model and Piecewise Lyapunov FunctionZhi-Le Xia,Jun-Min Li,Jiang-Rong Li4.Observer-based Adaptive Iterative Learning Control for Nonlinear Systems with Time-varying DelaysWei-Sheng Chen,Rui-Hong Li,Jing Li5.H∞ Output Feedback Control for Stochastic Systems with Mode-dependent Time-varying Delays and Markovian Jump ParametersXu-Dong Zhao,Qing-Shuang Zeng6.Delay and Its Time-derivative Dependent Robust Stability of Uncertain Neutral Systems with Saturating ActuatorsFatima El Haoussi,El Houssaine Tissir7.Parallel Fuzzy P+Fuzzy I+Fuzzy D Controller:Design and Performance EvaluationVineet Kumar,A.P.Mittal8.Observers for Descriptor Systems with Slope-restricted NonlinearitiesLin-Na Zhou,Chun-Yu Yang,Qing-Ling Zhang9.Parameterized Solution to a Class of Sylvester MatrixEquationsYu-Peng Qiao,Hong-Sheng Qi,Dai-Zhan Cheng10.Indirect Adaptive Fuzzy and Impulsive Control of Nonlinear SystemsHai-Bo Jiang11.Robust Fuzzy Tracking Control for Nonlinear Networked Control Systems with Integral Quadratic ConstraintsZhi-Sheng Chen,Yong He,Min Wu12.A Power-and Coverage-aware Clustering Scheme for Wireless Sensor NetworksLiang Xue,Xin-Ping Guan,Zhi-Xin Liu,Qing-Chao Zheng13.Guaranteed Cost Active Fault-tolerant Control of Networked Control System with Packet Dropout and Transmission DelayXiao-Yuan Luo,Mei-Jie Shang,Cai-Lian Chen,Xin-Ping Guanparison of Two Novel MRAS Based Strategies for Identifying Parameters in Permanent Magnet Synchronous MotorsKan Liu,Qiao Zhang,Zi-Qiang Zhu,Jing Zhang,An-Wen Shen,Paul Stewart15.Modeling and Analysis of Scheduling for Distributed Real-time Embedded SystemsHai-Tao Zhang,Gui-Fang Wu16.Passive Steganalysis Based on Higher Order Image Statistics of Curvelet TransformS.Geetha,Siva S.Sivatha Sindhu,N.Kamaraj17.Movement Invariants-based Algorithm for Medical Image Tilt CorrectionMei-Sen Pan,Jing-Tian Tang,Xiao-Li Yang18.Target Tracking and Obstacle Avoidance for Multi-agent SystemsJing Yan,Xin-Ping Guan,Fu-Xiao Tan19.Automatic Generation of Optimally Rigid Formations Using Decentralized MethodsRui Ren,Yu-Yan Zhang,Xiao-Yuan Luo,Shao-Bao Li20.Semi-blind Adaptive Beamforming for High-throughput Quadrature Amplitude Modulation SystemsSheng Chen,Wang Yao,Lajos Hanzo21.Throughput Analysis of IEEE 802.11 Multirate WLANs with Collision Aware Rate Adaptation AlgorithmDhanasekaran Senthilkumar,A. Krishnan22.Innovative Product Design Based on Customer Requirement Weight Calculation ModelChen-Guang Guo,Yong-Xian Liu,Shou-Ming Hou,Wei Wang23.A Service Composition Approach Based on Sequence Mining for Migrating E-learning Legacy System to SOAZhuo Zhang,Dong-Dai Zhou,Hong-Ji Yang,Shao-Chun Zhong24.Modeling of Agile Intelligent Manufacturing-oriented Production Scheduling SystemZhong-Qi Sheng,Chang-Ping Tang,Ci-Xing Lv25.Estimation of Reliability and Cost Relationship for Architecture-based SoftwareHui Guan,Wei-Ru Chen,Ning Huang,Hong-Ji Yang1.A Computer-aided Design System for Framed-mould in Autoclave ProcessingTian-Guo Jin,Feng-Yang Bi2.Wear State Recognition of Drills Based on K-means Cluster and Radial Basis Function Neural NetworkXu Yang3.The Knee Joint Design and Control of Above-knee Intelligent Bionic Leg Based on Magneto-rheological DamperHua-Long Xie,Ze-Zhong Liang,Fei Li,Li-Xin Guo4.Modeling of Pneumatic Muscle with Shape Memory Alloy and Braided SleeveBin-Rui Wang,Ying-Lian Jin,Dong Wei5.Extended Object Model for Product Configuration DesignZhi-Wei Xu,Ze-Zhong Liang,Zhong-Qi Sheng6.Analysis of Sheet Metal Extrusion Process Using Finite Element MethodXin-Cun Zhuang,Hua Xiang,Zhen Zhao7.Implementation of Enterprises' Interoperation Based on OntologyXiao-Feng Di,Yu-Shun Fan8.Path Planning Approach in Unknown EnvironmentTing-Kai Wang,Quan Dang,Pei-Yuan Pan9.Sliding Mode Variable Structure Control for Visual Servoing SystemFei Li,Hua-Long Xie10.Correlation of Direct Piezoelectric Effect on EAPap under Ambient FactorsLi-Jie Zhao,Chang-Ping Tang,Peng Gong11.XML-based Data Processing in Network Supported Collaborative DesignQi Wang,Zhong-Wei Ren,Zhong-Feng Guo12.Production Management Modelling Based on MASLi He,Zheng-Hao Wang,Ke-Long Zhang13.Experimental Tests of Autonomous Ground Vehicles with PreviewCunjia Liu,Wen-Hua Chen,John Andrews14.Modelling and Remote Control of an ExcavatorYang Liu,Mohammad Shahidul Hasan,Hong-Nian Yu15.TOPSIS with Belief Structure for Group Belief Multiple Criteria Decision MakingJiang Jiang,Ying-Wu Chen,Da-Wei Tang,Yu-Wang Chen16.Video Analysis Based on Volumetric Event DetectionJing Wang,Zhi-Jie Xu17.Improving Decision Tree Performance by Exception HandlingAppavu Alias Balamurugan Subramanian,S.Pramala,B.Rajalakshmi,Ramasamy Rajaram18.Robustness Analysis of Discrete-time Indirect Model Reference Adaptive Control with Normalized Adaptive LawsQing-Zheng Gao,Xue-Jun Xie19.A Novel Lifecycle Model for Web-based Application Development in Small and Medium EnterprisesWei Huang,Ru Li,Carsten Maple,Hong-Ji Yang,David Foskett,Vince Cleaver20.Design of a Two-dimensional Recursive Filter Using the Bees AlgorithmD. T. Pham,Ebubekir Ko(c)21.Designing Genetic Regulatory Networks Using Fuzzy Petri Nets ApproachRaed I. Hamed,Syed I. Ahson,Rafat Parveen1.State of the Art and Emerging Trends in Operations and Maintenance of Offshore Oil and Gas Production Facilities: Some Experiences and ObservationsJayantha P.Liyanage2.Statistical Safety Analysis of Maintenance Management Process of Excavator UnitsLjubisa Papic,Milorad Pantelic,Joseph Aronov,Ajit Kumar Verma3.Improving Energy and Power Efficiency Using NComputing and Approaches for Predicting Reliability of Complex Computing SystemsHoang Pham,Hoang Pham Jr.4.Running Temperature and Mechanical Stability of Grease as Maintenance Parameters of Railway BearingsJan Lundberg,Aditya Parida,Peter S(o)derholm5.Subsea Maintenance Service Delivery: Mapping Factors Influencing Scheduled Service DurationEfosa Emmanuel Uyiomendo,Tore Markeset6.A Systemic Approach to Integrated E-maintenance of Large Engineering PlantsAjit Kumar Verma,A.Srividya,P.G.Ramesh7.Authentication and Access Control in RFID Based Logistics-customs Clearance Service PlatformHui-Fang Deng,Wen Deng,Han Li,Hong-Ji Yang8.Evolutionary Trajectory Planning for an Industrial RobotR.Saravanan,S.Ramabalan,C.Balamurugan,A.Subash9.Improved Exponential Stability Criteria for Recurrent Neural Networks with Time-varying Discrete and Distributed DelaysYuan-Yuan Wu,Tao Li,Yu-Qiang Wu10.An Improved Approach to Delay-dependent Robust Stabilization for Uncertain Singular Time-delay SystemsXin Sun,Qing-Ling Zhang,Chun-Yu Yang,Zhan Su,Yong-Yun Shao11.Robust Stability of Nonlinear Plants with a Non-symmetric Prandtl-Ishlinskii Hysteresis ModelChang-An Jiang,Ming-Cong Deng,Akira Inoue12.Stability Analysis of Discrete-time Systems with Additive Time-varying DelaysXian-Ming Tang,Jin-Shou Yu13.Delay-dependent Stability Analysis for Markovian Jump Systems with Interval Time-varying-delaysXu-Dong Zhao,Qing-Shuang Zeng14.H∞ Synchronization of Chaotic Systems via Delayed Feedback ControlLi Sheng,Hui-Zhong Yang15.Adaptive Fuzzy Observer Backstepping Control for a Class of Uncertain Nonlinear Systems with Unknown Time-delayShao-Cheng Tong,Ning Sheng16.Simulation-based Optimal Design of α-β-γ-δ FilterChun-Mu Wu,Paul P.Lin,Zhen-Yu Han,Shu-Rong Li17.Independent Cycle Time Assignment for Min-max SystemsWen-De Chen,Yue-Gang Tao,Hong-Nian Yu1.An Assessment Tool for Land Reuse with Artificial Intelligence MethodDieter D. Genske,Dongbin Huang,Ariane Ruff2.Interpolation of Images Using Discrete Wavelet Transform to Simulate Image Resizing as in Human VisionRohini S. Asamwar,Kishor M. Bhurchandi,Abhay S. Gandhi3.Watermarking of Digital Images in Frequency DomainSami E. I. Baba,Lala Z. Krikor,Thawar Arif,Zyad Shaaban4.An Effective Image Retrieval Mechanism Using Family-based Spatial Consistency Filtration with Object RegionJing Sun,Ying-Jie Xing5.Robust Object Tracking under Appearance Change ConditionsQi-Cong Wang,Yuan-Hao Gong,Chen-Hui Yang,Cui-Hua Li6.A Visual Attention Model for Robot Object TrackingJin-Kui Chu,Rong-Hua Li,Qing-Ying Li,Hong-Qing Wang7.SVM-based Identification and Un-calibrated Visual Servoing for Micro-manipulationXin-Han Huang,Xiang-Jin Zeng,Min Wang8.Action Control of Soccer Robots Based on Simulated Human IntelligenceTie-Jun Li,Gui-Qiang Chen,Gui-Fang Shao9.Emotional Gait Generation for a Humanoid RobotLun Xie,Zhi-Liang Wang,Wei Wang,Guo-Chen Yu10.Cultural Algorithm for Minimization of Binary Decision Diagram and Its Application in Crosstalk Fault DetectionZhong-Liang Pan,Ling Chen,Guang-Zhao Zhang11.A Novel Fuzzy Direct Torque Control System for Three-level Inverter-fed Induction MachineShu-Xi Liu,Ming-Yu Wang,Yu-Guang Chen,Shan Li12.Statistic Learning-based Defect Detection for Twill FabricsLi-Wei Han,De Xu13.Nonsaturation Throughput Enhancement of IEEE 802.11b Distributed Coordination Function for Heterogeneous Traffic under Noisy EnvironmentDhanasekaran Senthilkumar,A. Krishnan14.Structure and Dynamics of Artificial Regulatory Networks Evolved by Segmental Duplication and Divergence ModelXiang-Hong Lin,Tian-Wen Zhang15.Random Fuzzy Chance-constrained Programming Based on Adaptive Chaos Quantum Honey Bee Algorithm and Robustness AnalysisHan Xue,Xun Li,Hong-Xu Ma16.A Bit-level Text Compression Scheme Based on the ACW AlgorithmHussein A1-Bahadili,Shakir M. Hussain17.A Note on an Economic Lot-sizing Problem with Perishable Inventory and Economies of Scale Costs:Approximation Solutions and Worst Case AnalysisQing-Guo Bai,Yu-Zhong Zhang,Guang-Long Dong1.Virtual Reality: A State-of-the-Art SurveyNing-Ning Zhou,Yu-Long Deng2.Real-time Virtual Environment Signal Extraction and DenoisingUsing Programmable Graphics HardwareYang Su,Zhi-Jie Xu,Xiang-Qian Jiang3.Effective Virtual Reality Based Building Navigation Using Dynamic Loading and Path OptimizationQing-Jin Peng,Xiu-Mei Kang,Ting-Ting Zhao4.The Skin Deformation of a 3D Virtual HumanXiao-Jing Zhou,Zheng-Xu Zhao5.Technology for Simulating Crowd Evacuation BehaviorsWen-Hu Qin,Guo-Hui Su,Xiao-Na Li6.Research on Modelling Digital Paper-cut PreservationXiao-Fen Wang,Ying-Rui Liu,Wen-Sheng Zhang7.On Problems of Multicomponent System Maintenance ModellingTomasz Nowakowski,Sylwia Werbinka8.Soft Sensing Modelling Based on Optimal Selection of Secondary Variables and Its ApplicationQi Li,Cheng Shao9.Adaptive Fuzzy Dynamic Surface Control for Uncertain Nonlinear SystemsXiao-Yuan Luo,Zhi-Hao Zhu,Xin-Ping Guan10.Output Feedback for Stochastic Nonlinear Systems with Unmeasurable Inverse DynamicsXin Yu,Na Duan11.Kalman Filtering with Partial Markovian Packet LossesBao-Feng Wang,Ge Guo12.A Modified Projection Method for Linear FeasibilityProblemsYi-Ju Wang,Hong-Yu Zhang13.A Neuro-genetic Based Short-term Forecasting Framework for Network Intrusion Prediction SystemSiva S. Sivatha Sindhu,S. Geetha,M. Marikannan,A. Kannan14.New Delay-dependent Global Asymptotic Stability Condition for Hopfield Neural Networks with Time-varying DelaysGuang-Deng Zong,Jia Liu hHTTp://15.Crosscumulants Based Approaches for the Structure Identification of Volterra ModelsHouda Mathlouthi,Kamel Abederrahim,Faouzi Msahli,Gerard Favier1.Coalition Formation in Weighted Simple-majority Games under Proportional Payoff Allocation RulesZhi-Gang Cao,Xiao-Guang Yang2.Stability Analysis for Recurrent Neural Networks with Time-varying DelayYuan-Yuan Wu,Yu-Qiang Wu3.A New Type of Solution Method for the Generalized Linear Complementarity Problem over a Polyhedral ConeHong-Chun Sun,Yan-Liang Dong4.An Improved Control Algorithm for High-order Nonlinear Systems with Unmodelled DynamicsNa Duan,Fu-Nian Hu,Xin Yu5.Controller Design of High Order Nonholonomic System with Nonlinear DriftsXiu-Yun Zheng,Yu-Qiang Wu6.Directional Filter for SAR Images Based on NonsubsampledContourlet Transform and Immune Clonal SelectionXiao-Hui Yang,Li-Cheng Jiao,Deng-Feng Li7.Text Extraction and Enhancement of Binary Images Using Cellular AutomataG. Sahoo,Tapas Kumar,B.L. Rains,C.M. Bhatia8.GH2 Control for Uncertain Discrete-time-delay Fuzzy Systems Based on a Switching Fuzzy Model and Piecewise Lyapunov FunctionZhi-Le Xia,Jun-Min Li9.A New Energy Optimal Control Scheme for a Separately Excited DC Motor Based Incremental Motion DriveMilan A.Sheta,Vivek Agarwal,Paluri S.V.Nataraj10.Nonlinear Backstepping Ship Course ControllerAnna Witkowska,Roman Smierzchalski11.A New Method of Embedded Fourth Order with Four Stages to Study Raster CNN SimulationR. Ponalagusamy,S. Senthilkumar12.A Minimum-energy Path-preserving Topology Control Algorithm for Wireless Sensor NetworksJin-Zhao Lin,Xian Zhou,Yun Li13.Synchronization and Exponential Estimates of Complex Networks with Mixed Time-varying Coupling DelaysYang Dai,YunZe Cai,Xiao-Ming Xu14.Step-coordination Algorithm of Traffic Control Based on Multi-agent SystemHai-Tao Zhang,Fang Yu,Wen Li15.A Research of the Employment Problem on Common Job-seekersand GraduatesBai-Da Qu。
2000 【ng0500_25】 Gene Ontology-tool for the unification of biology
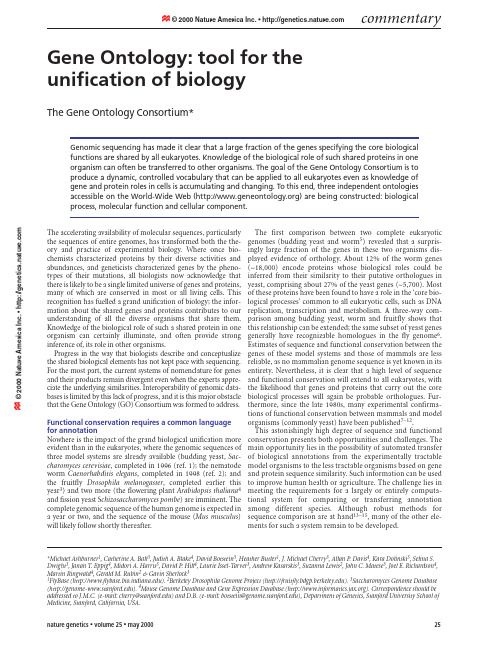
Gene Ontology: tool for the unification of biologyThe Gene Ontology Consortium**Michael Ashburner 1, Catherine A. Ball 3, Judith A. Blake 4, David Botstein 3, Heather Butler 1, J. Michael Cherry 3, Allan P. Davis 4, Kara Dolinski 3, Selina S.Dwight 3, Janan T. Eppig 4, Midori A. Harris 3, David P. Hill 4, Laurie Issel-Tarver 3, Andrew Kasarskis 3, Suzanna Lewis 2, John C. Matese 3, Joel E. Richardson 4,Martin Ringwald 4, Gerald M. Rubin 2& Gavin Sherlock 31FlyBase (http://www.fl). 2Berkeley Drosophila Genome Project (http://fruitfl). 3Saccharomyces Genome Database (). 4Mouse Genome Database and Gene Expression Database (). Correspondence should be addressed to J.M.C. (e-mail: cherry@) and D.B. (e-mail: botstein@), Department of Genetics, Stanford University School of Medicine, Stanford, California, USA.The accelerating availability of molecular sequences, particularly the sequences of entire genomes, has transformed both the the-ory and practice of experimental biology. Where once bio-chemists characterized proteins by their diverse activities and abundances, and geneticists characterized genes by the pheno-types of their mutations, all biologists now acknowledge that there is likely to be a single limited universe of genes and proteins,many of which are conserved in most or all living cells. This recognition has fuelled a grand unification of biology; the infor-mation about the shared genes and proteins contributes to our understanding of all the diverse organisms that share them.Knowledge of the biological role of such a shared protein in one organism can certainly illuminate, and often provide strong inference of, its role in other organisms.Progress in the way that biologists describe and conceptualize the shared biological elements has not kept pace with sequencing.For the most part, the current systems of nomenclature for genes and their products remain divergent even when the experts appre-ciate the underlying similarities. Interoperability of genomic data-bases is limited by this lack of progress, and it is this major obstacle that the Gene Ontology (GO) Consortium was formed to address.Functional conservation requires a common language for annotationNowhere is the impact of the grand biological unification more evident than in the eukaryotes, where the genomic sequences of three model systems are already available (budding yeast, Sac-charomyces cerevisiae , completed in 1996 (ref. 1); the nematode worm Caenorhabditis elegans , completed in 1998 (ref. 2); and the fruitfly Drosophila melanogaster , completed earlier this year 3) and two more (the flowering plant Arabidopsis thaliana 4and fission yeast S chizosaccharomyces pombe ) are imminent. The complete genomic sequence of the human genome is expected in a year or two, and the sequence of the mouse (Mus musculus )will likely follow shortly thereafter.The first comparison between two complete eukaryotic genomes (budding yeast and worm 5) revealed that a surpris-ingly large fraction of the genes in these two organisms dis-played evidence of orthology. About 12% of the worm genes (∼18,000) encode proteins whose biological roles could be inferred from their similarity to their putative orthologues in yeast, comprising about 27% of the yeast genes (∼5,700). Most of these proteins have been found to have a role in the ‘core bio-logical processes’ common to all eukaryotic cells, such as DNA replication, transcription and metabolism. A three-way com-parison among budding yeast, worm and fruitfly shows that this relationship can be extended; the same subset of yeast genes generally have recognizable homologues in the fly genome 6.Estimates of sequence and functional conservation between the genes of these model systems and those of mammals are less reliable, as no mammalian genome sequence is yet known in its entirety. Nevertheless, it is clear that a high level of sequence and functional conservation will extend to all eukaryotes, with the likelihood that genes and proteins that carry out the core biological processes will again be probable orthologues. Fur-thermore, since the late 1980s, many experimental confirma-tions of functional conservation between mammals and model organisms (commonly yeast) have been published 7–12.This astonishingly high degree of sequence and functional conservation presents both opportunities and challenges. The main opportunity lies in the possibility of automated transfer of biological annotations from the experimentally tractable model organisms to the less tractable organisms based on gene and protein sequence similarity. Such information can be used to improve human health or agriculture. The challenge lies in meeting the requirements for a largely or entirely computa-tional system for comparing or transferring annotation among different species. Although robust methods for sequence comparison are at hand 13–15, many of the other ele-ments for such a system remain to be developed.Genomic sequencing has made it clear that a large fraction of the genes specifying the core biological functions are shared by all eukaryotes. Knowledge of the biological role of such shared proteins in one organism can often be transferred to other organisms. The goal of the Gene Ontology Consortium is to produce a dynamic, controlled vocabulary that can be applied to all eukaryotes even as knowledge of gene and protein roles in cells is accumulating and changing. To this end, three independent ontologies accessible on the World-Wide Web () are being constructed: biologicalprocess, molecular function and cellular component.© 2000 N a t u r e A m e r i c a I n c . • h t t p ://g e n e t i c s .n a t u r e .c o mA dynamic gene ontologyThe GO Consortium is a joint project of three model organism databases: FlyBase 16,Mouse Genome Informatics 17,18(MGI) and the Saccharomyces Genome Database 19(SGD). It is expected that other organism databases will join in the near future. The goal of the Consortium is to produce a structured, precisely defined, common, con-trolled vocabulary for describing the roles of genes and gene products in any organism.Early considerations of the problems posed by the diversity of activities that characterize the cells of yeast, flies and mice made it clear that extensions of standard indexing meth-ods (for example, keywords) are likely to be both unwieldy and, in the end, unworkable.Although these resources remain essential,and our proposed system will continue to link to and depend on them, they are not sufficient in themselves to allow automatic transfers of annotation.Each node in the GO ontologies will be linked to other kinds of information, includ-ing the many gene and protein keyword databases such as SwissPROT (ref. 20), Gen-Bank (ref. 21), EMBL (ref. 22), DDBJ (ref.23), PIR (ref. 24), MIPS (ref. 25), YPD &WormPD (ref. 26), Pfam (ref. 27), SCOP (ref. 28) and ENZYME (ref. 29). One reason for this is that the state of biological knowl-edge of what genes and proteins do is very incomplete and changing rapidly. Discover-ies that change our understanding of the roles of gene products in cells are published on a daily basis. To illustrate this, consider annotating two different proteins. One is known to be a transmembrane receptor ser-ine/threonine kinase involved in p53-induced apoptosis; the other is known only to be a membrane-bound protein. In one case, the knowledge about the protein is sub-stantial, whereas in the other it is minimal.© 2000 N a t u r e A m e r i c a I n c . • h t t p ://g e n e t i c s .n a t u r e .c o mWe need to be able to organize, describe, query and visualize bio-logical knowledge at vastly different stages of completeness. Any system must be flexible and tolerant of this constantly changing level of knowledge and allow updates on a continuing basis.Similar considerations suggested that a static hierarchical sys-tem, such as the Enzyme Commission 30(EC) hierarchy, although computationally tractable, was also likely to be inadequate to describe the role of a gene or a protein in biology in a manner that would be either intuitive or helpful for biologists. The hier-archical EC numbering system for enzymes is the standard resource for classifying enzymatic chemical reactions. The EC system does not address the classification of non-enzymatic pro-teins or the ability to describe the role of a gene product within a cell; also, the system has little facility for describing diverse pro-tein interactions. The vagueness of the term ‘function’ when applied to genes or proteins emerged as a particular problem, as this term is colloquially used to describe biochemical activities,biological goals and cellular structure. It is commonplace today to refer to the function of a protein such as tubulin as ‘GTPase’ or ‘constituent of the mitotic spindle’. For all these reasons, we are constructing three independent ontologies.Three categories of GOBiological process refers to a biological objective to which the gene or gene product contributes. A process is accomplished via one or more ordered assemblies of molecular functions.Processes often involve a chemical or physical transformation,in the sense that something goes into a process and something different comes out of it. Examples of broad (high level) bio-logical process terms are ‘cell growth and maintenance’ or ‘sig-nal transduction’. Examples of more specific (lower level)process terms are ‘translation’, ‘pyrimidine metabolism’ or ‘cAMP biosynthesis’.Molecular function is defined as the biochemical activity (including specific binding to ligands or structures) of a gene product. This definition also applies to the capability that a gene product (or gene product complex) carries as a potential. It describes only what is done without specifying where or when the event actually occurs. Examples of broad functional terms are ‘enzyme’, ‘transporter’ or ‘ligand’. Examples of narrower func-tional terms are ‘adenylate cyclase’ or ‘Toll receptor ligand’.Cellular component refers to the place in the cell where a gene product is active. These terms reflect our understanding of eukaryotic cell structure. As is true for the other ontologies, not all terms are applicable to all organisms; the set of terms is meant to be inclusive. Cellular component includes such terms as ‘ribo-some’ or ‘proteasome’, specifying where multiple gene products would be found. It also includes terms such as ‘nuclear mem-brane’ or ‘Golgi apparatus’.Ontologies have long been used in an attempt to describe all entities within an area of reality and all relationships between those entities. An ontology comprises a set of well-defined terms with well-defined relationships. The structure itself reflects the current representation of biological knowledge as well as serving as a guide for organizing new data. Data can be annotated to varying levels depending on the amount and completeness of available information. This flexibility also allows users to narrow or widen the focus of queries. Ultimately, an ontology can be a vital tool enabling researchers to turn data into knowledge. Com-puter scientists have made significant contributions to linguistic formalisms and computational tools for developing complex vocabulary systems using reason-based structures, and we hope that our ontologies will be useful in providing a well-developed data set for this community to test their systems. The Molecular Biology Ontology Working Group (/projects/bio-ontology/) is actively attempting to develop standards in this general field.Biological process, molecular function and cellular component are all attributes of genes, gene products or gene-product groups.Each of these may be assigned independently and, indeed, we believe that simply recognizing that biological process, molecular function and cellular location represent independent attributes is by itself clarifying in many situations, as in the annotation of gene-expression data. The relationships between a gene product (or gene-product group) to biological process, molecular func-tion and cellular component are one-to-many, reflecting the bio-logical reality that a particular protein may function in several processes, contain domains that carry out diverse molecular© 2000 N a t u r e A m e r i c a I n c . • h t t p ://g e n e t i c s .n a t u r e .c o mfunctions, and participate in multiple alternative interactions with other proteins, organelles or locations in the cell.The ontologies are developed for a generic eukaryotic cell;accordingly, specialized organs or body parts are not represented.Full integration of the ontologies with anatomical structures will occur as the ontologies are incorporated into each species’ data-base and are related to anatomical data within each database. GO terms are connected into nodes of a network, thus the connec-tions between its parents and children are known and form what are technically described as directed acyclic graphs. The ontolo-gies are dynamic, in the sense that they exist as a network that is changed as more information accumulates, but have sufficient uniqueness and precision so that databases based on the ontolo-gies can automatically be updated as the ontologies mature. The ontologies are flexible in another way, so that they can reflect the many differences in the biology of the diverse organisms, such as the breakdown of the nucleus during mitosis. In this way the GO Consortium has built up a system that supports a common lan-guage with specific, agreed-on terms with definitions and sup-porting documentation (the GO ontologies) that can be understood and used by a wide biological community.Examples of GO annotationAs one example, consider DNA metabolism, a biological process carried out by largely (but not entirely) shared elements in eukaryotes. The part of the process ontology (with selected gene names from S. cerevisiae , Drosophila and M. musculus ) shown is largely one parent to many children (Fig. 1a ). One notable excep-tion is the process of DNA ligation, which is a child of three processes, DNA replication, DNA repair and DNA recombina-tion. The yeast gene product Cdc9p is able to carry out the ligation step for all three processes, whereas it is uncertain whether the same enzyme is used in the other species. From the point of view of the ontology, it matters not, and a computer (or a human searcher) will find the appropriate nodes in either case using as the query either the enzyme, the gene name(s) or the GO term (or, if available, the unique GO identifier, in this case, GO:0003910).Also shown are the molecular function ontology for the MCM protein complex members that are known to regulate initiation of DNA replication in the three organisms (Fig. 1b ), and a por-tion of the cellular component ontology for these proteins (Fig.1c ). These ontologies reflect the finding that Mcm2–7 proteins are components of the pre-replicative complex in several model organisms, as well as sometimes localizing to the cytoplasm 30.The ontology supports both biological realities, and yet the mole-cular functions and the biological processes of the MCM homo-logues are conserved nevertheless.The usefulness of the GO ontologies for annotation received its first major test in the annotation of the recently completed sequence of the Drosophila genome. Little human intervention was required to annotate 50% of the genes to the molecular function and biological process ontologies using the GO method. Another use for GO ontologies that is gaining rapid adherence is the anno-tation of gene-expression data, especially after these have been clustered by similarities in pattern of gene expression 32,33. The results of clustering about 100 yeast experiments (of which about half are shown; Fig. 2) grouped together a subset of genes which, by name alone, convey little to most biologists. When the full short GO annotations for process, molecular function and location are added, however, the biological reason and import of the co-expres-sion of these genes becomes evident.The GO project is currently using a flat file format to store the ontologies, definitions of terms and gene associations. The ontologies, gene associations, definitions and documentation are available from the GO web site (),which also describes the principles and objectives used by the pro-ject. The ontologies are by no means complete. They are being expanded during the association of gene products from the col-laborating databases and we expect them to continue to evolve for many years. GO requires that all gene associations to the ontolo-gies must be attributed to the literature; for each citation the type of evidence will be encoded. As of early April 2000 there were 1,923, 2,094 and 490 nodes in the process, function and compo-nent ontologies, respectively. The three organism databases have made substantial progress to link gene products. Thus far the process, function and component ontologies have associations with 1,624, 1,602 and 1,577 yeast genes; 741, 2,334 and 1,061 fly genes; and 1,933, 2,896 and 1,696 mouse genes, respectively. A running table of these statistics can be found at the web site.The GO concept is intended to make possible, in a flexible and dynamic way, the annotation of homologous gene and protein sequences in multiple organisms using a common vocabulary that results in the ability to query and retrieve genes and proteins based on their shared biology. The GO ontologies produce a con-trolled vocabulary that can be used for dynamic maintenance and interoperability between genome databases. The ontologies are a work in progress. They can be consulted at any time on the World-Wide Web; indeed, their availability to human and machine alike is essential to maintain their flexibility and allow their evolution along with increased understanding of the under-lying biology. It is hoped that the GO concepts, especially the dis-tinctions between biological process, molecular function and cellular component, will find favour among biologists so that we can all facilitate, in our writing as well as our thinking, the grand unification of biology that the genome sequences portend.AcknowledgementsWe thank K. Fasman and M. Rebhan for useful discussions, and Astra Zeneca for financial support. SGD is supported by a P41, National Resources, grant from National Human Genome Research Institute (NHGRI) grantHG01315; MGD by a P41 from NHGRI grant HG00330; GXD by National Institute of Child Health and Human Development grant HD33745; and FlyBase by a P41 from NHGRI grant HG00739 and the Medical Research Council, London.Received 20 March; accepted 5 April 2000.© 2000 N a t u r e A m e r i c a I n c . • h t t p ://g e n e t i c s .n a t u r e .c o m1.Goffeau, A. et al.Life with 6000 genes. Science 274, 546 (1996).2.Worm Sequencing Consortium. Genome sequence of the nematode C. elegans : a platform for investigating biology. The C. elegans Sequencing Consortium.Science 282, 2012–2018 (1998).3.Adams, M.D. et al.The genome sequence of Drosophila melanogaster . Science 287, 2185–2195 (2000).4.Meinke, D.W. et al . Ar abidopsis thaliana : a model plant for genome analysis.Science 282, 662–682 (1998).5.Chervitz, S.A. et al. Using the Saccharomyces Genome Database (SGD) for analysis of protein similarities and structure. Nucleic Acids Res . 27, 74–78 (1999).6.Rubin, G.M. et parative genomics of the eukaryotes. Science 287,2204–2215 (2000).7.Tang, Z., Kuo, T., Shen, J. & Lin, R.J. Biochemical and genetic conservation of fission yeast Dsk1 and human SR protein-specific kinase 1. Mol. Cell. Biol . 20,816–824 (2000).8.Vajo, Z. et al . Conservation of the Caenorhabditis elegans timing gene clk-1 from yeast to human: a gene required for ubiquinone biosynthesis with potential implications for aging . Mamm. Genome 10, 1000–1004 (1999).9.Ohi, R. et al . Myb-related Schizosaccharomyces pombe cdc5p is structurally and functionally conserved in eukaryotes. Mol. Cell. Biol.18, 4097–4108 (1998).10.Bassett, D.E. Jr et al . Genome cross-referencing and XREFdb: implications for the identification and analysis of genes mutated in human disease. Nature Genet.15,339–344 (1997).11.Kataoka T. et al . Functional homology of mammalian and yeast RAS genes. Cell 40,19–26 (1985).12.Botstein, D. & Fink, G.R. Yeast: an experimental organism for modern biology.Science 240, 1439–1443 (1988).13.Tatusov, R.L., Galperin, M.Y ., Natale, D.A. & Koonin, E.V. The COG database: a tool for genome-scale analysis of protein functions and evolution. Nucleic Acids Res .28, 33–36 (2000).14.Andrade, M.A. et al . Automated genome sequence analysis and annotation.Bioinfor matics 15, 391–412 (1999).15.Fleischmann, W., Moller, S., Gateau, A. & Apweiler, R. A novel method for automatic functional annotation of proteins. Bioinformatics 15, 228–233 (1999).16.The FlyBase Consortium. The FlyBase database of the Drosophila Genome Projects and community literature. Nucleic Acids Res . 27, 85–88 (1999).17.Blake, J.A. et al . The Mouse Genome Database (MGD): expanding genetic and genomic resources for the laboratory mouse. Nucleic Acids Res . 28, 108–111 (2000).18.Ringwald, M. et al . GXD: a gene expression database for the laboratorymouse current status and recent enhancements. Nucleic Acids Res . 28,115–119 (2000).19.Ball, C.A. et al . Integrating functional genomic information into theSaccha r omyces Genome Database. Nucleic Acids Res . 28, 77–80 (2000).20.Bairoch, A. & Apweiler, R. The SWISS-PROT protein sequence database and itssupplement TrEMBL in 2000. Nucleic Acids Res.28, 45–48 (2000).21.Benson, D.A. et al . GenBank. Nucleic Acids Res.28, 15–18 (2000).22.Baker, W. et al . The EMBL Nucleotide Sequence Database. Nucleic Acids Res.28,19–23 (2000).23.Tateno, Y. et al . DNA Data Bank of J apan (DDBJ ) in collaboration with masssequencing teams. Nucleic Acids Res.28, 24–26 (2000).24.Barker, W.C. et al . The Protein Information Resource (PIR). Nucleic Acids Res.28,41–44 (2000).25.Mewes, H.W. et al . MIPS: a database for genomes and protein sequences.Nucleic Acids Res.28, 37–40 (2000).26.Costanzo, M.C. et al . The Yeast Proteome Database (YPD) and Caenorhabditiselegans Proteome Database (WormPD): comprehensive resources for the organization and comparison of model organism protein information. Nucleic Acids Res.28, 73–76 (2000).27.Bateman, A. et al . The Pfam protein families database. Nucleic Acids Res.28,263–266 (2000).28.Lo Conte, L. et al . SCOP: a structural classification of proteins database. NucleicAcids Res.28, 257–259 (2000).29.Bairoch, A. The ENZYME database in 2000. Nucleic Acids Res.28, 304–305(2000).30.Enzyme Nomenclature. Recommendations of the Nomenclature Committee ofthe Inte national Union of Biochemist y and Molecula Biology on the Nomenclature and Classification of Enyzmes. NC-IUBMB.(Academic, New York,1992).31.Tye, B.K. MCM proteins in DNA replication. Annu. Rev. Biochem.68, 649–686(1999).32.Eisen, M., Spellman, P.T., Brown, P.O. & Botstein, D. Cluster analysis and displayof genome-wide expression patterns . Proc. Natl Acad. Sci. USA 95, 14863–14868(1998).33.Spellman, P.T. et al . Comprehensive identification of cell cycle-regulated genesof the yeast Sacchar omyces cer evisiae by microarray hybridization. Mol. Biol.Cell 9, 3273–3297 (1998).© 2000 N a t u r e A m e r i c a I n c . • h t t p ://g e n e t i c s .n a t u r e .c o m。
大数据和人工智能在足球项目比赛分析中的应用
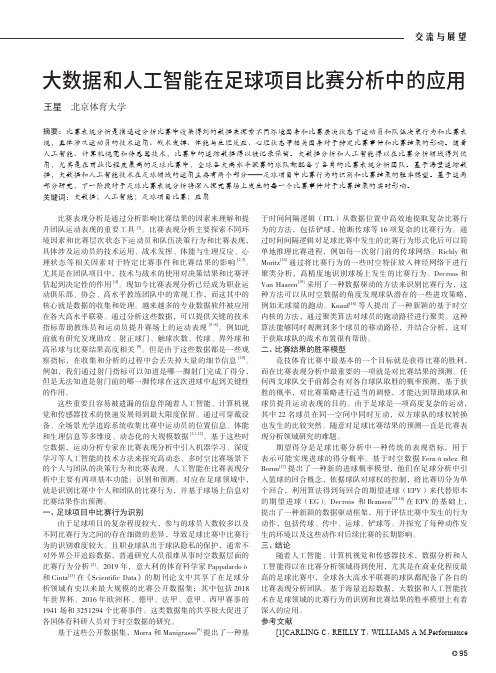
大数据和人工智能在足球项目比赛分析中的应用王星北京体育大学摘要:比赛表现分析是指通过分析比赛中收集得到的数据来探索不同环境因素和比赛层次状态下运动员和队伍决策行为和比赛表现,具体涉及运动员的技术运用、战术发挥、体能与生理反应、心理状态等相关因素对于特定比赛事件和比赛结果的影响。
随着人工智能、计算机视觉和传感器技术,比赛中的追踪数据得以被记录保留。
大数据分析和人工智能得以在比赛分析领域得到使用,尤其是在商业化程度最高的足球比赛中,全球各大高水平联赛的球队都配备了各自的比赛表现分析团队。
基于海量追踪数据,大数据和人工智能技术在足球领域的运用主要有两个部分——足球项目中比赛行为的识别和比赛结果的胜率模型。
基于这两部分研究,下一阶段对于足球比赛表现分析将深入探究赛场上发生的每一个比赛事件对于比赛结果的实时影响。
关键词:大数据;人工智能;足球项目比赛;应用比赛表现分析是通过分析影响比赛结果的因素来理解和提升团队运动表现的重要工具[1]。
比赛表现分析主要探索不同环境因素和比赛层次状态下运动员和队伍决策行为和比赛表现,具体涉及运动员的技术运用、战术发挥、体能与生理反应、心理状态等相关因素对于特定比赛事件和比赛结果的影响[2,3]。
尤其是在团队项目中,技术与战术的使用对决策结果和比赛评估起到决定性的作用[4]。
现如今比赛表现分析已经成为职业运动俱乐部、协会、高水平教练团队中的常规工作,而这其中的核心就是数据的收集和处理。
越来越多的专业数据软件被应用在各大高水平联赛。
通过分析这些数据,可以提供关键的技术指标帮助教练员和运动员提升赛场上的运动表现[5-8]。
例如此前就有研究发现助攻、射正球门、触球次数、传球、界外球和高吊球与比赛结果高度相关[9]。
但是由于这些数据都是一些观察指标,在收集和分析的过程中会丢失掉大量的细节信息[10]。
例如,我们通过射门指标可以知道是哪一脚射门完成了得分,但是无法知道是射门前的哪一脚传球在这次进球中起到关键性的作用。
车辆控制系统说明书
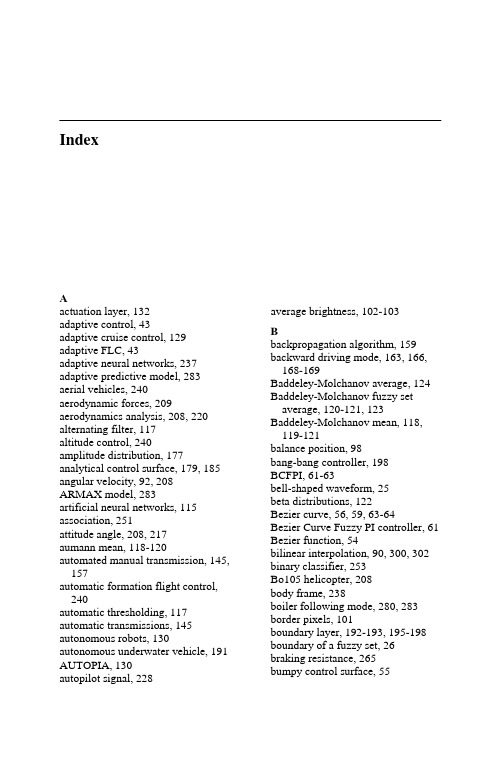
IndexAactuation layer, 132average brightness,102-103adaptive control, 43Badaptive cruise control, 129backpropagation algorithm, 159adaptive FLC, 43backward driving mode,163,166,168-169adaptive neural networks,237adaptive predictive model, 283Baddeley-Molchanov average, 124aerial vehicles, 240 Baddeley-Molchanov fuzzy set average, 120-121, 123aerodynamic forces,209aerodynamics analysis, 208, 220Baddeley-Molchanov mean,118,119-121alternating filter, 117altitude control, 240balance position, 98amplitude distribution, 177bang-bang controller,198analytical control surface, 179, 185BCFPI, 61-63angular velocity, 92,208bell-shaped waveform,25ARMAX model, 283beta distributions,122artificial neural networks,115Bezier curve, 56, 59, 63-64association, 251Bezier Curve Fuzzy PI controller,61attitude angle,208, 217Bezier function, 54aumann mean,118-120bilinear interpolation, 90, 300,302automated manual transmission,145,157binary classifier,253Bo105 helicopter, 208automatic formation flight control,240body frame,238boiler following mode,280,283automatic thresholding,117border pixels, 101automatic transmissions,145boundary layer, 192-193,195-198autonomous robots,130boundary of a fuzzy set,26autonomous underwater vehicle, 191braking resistance, 265AUTOPIA, 130bumpy control surface, 55autopilot signal, 228Index 326CCAE package software, 315, 318 calibration accuracy, 83, 299-300, 309, 310, 312CARIMA models, 290case-based reasoning, 253center of gravity method, 29-30, 32-33centroid defuzzification, 7 centroid defuzzification, 56 centroid Method, 106 characteristic polygon, 57 characterization, 43, 251, 293 chattering, 6, 84, 191-192, 195, 196, 198chromosomes, 59circuit breaker, 270classical control, 1classical set, 19-23, 25-26, 36, 254 classification, 106, 108, 111, 179, 185, 251-253classification model, 253close formation flight, 237close path tracking, 223-224 clustering, 104, 106, 108, 251-253, 255, 289clustering algorithm, 252 clustering function, 104clutch stroke, 147coarse fuzzy logic controller, 94 collective pitch angle, 209 collision avoidance, 166, 168 collision avoidance system, 160, 167, 169-170, 172collision avoidance system, 168 complement, 20, 23, 45 compressor contamination, 289 conditional independence graph, 259 confidence thresholds, 251 confidence-rated rules, 251coning angle, 210constant gain, 207constant pressure mode, 280 contrast intensification, 104 contrast intensificator operator, 104 control derivatives, 211control gain, 35, 72, 93, 96, 244 control gain factor, 93control gains, 53, 226control rules, 18, 27, 28, 35, 53, 64, 65, 90-91, 93, 207, 228, 230, 262, 302, 304-305, 315, 317control surfaces, 53-55, 64, 69, 73, 77, 193controller actuator faulty, 289 control-weighting matrix, 207 convex sets, 119-120Coordinate Measurement Machine, 301coordinate measuring machine, 96 core of a fuzzy set, 26corner cube retroreflector, 85 correlation-minimum, 243-244cost function, 74-75, 213, 282-283, 287coverage function, 118crisp input, 18, 51, 182crisp output, 7, 34, 41-42, 51, 184, 300, 305-306crisp sets, 19, 21, 23crisp variable, 18-19, 29critical clearing time, 270 crossover, 59crossover probability, 59-60cruise control, 129-130,132-135, 137-139cubic cell, 299, 301-302, 309cubic spline, 48cubic spline interpolation, 300 current time gap, 136custom membership function, 294 customer behav or, 249iDdamping factor, 211data cleaning, 250data integration, 250data mining, 249, 250, 251-255, 259-260data selection, 250data transformation, 250d-dimensional Euclidean space, 117, 124decision logic, 321 decomposition, 173, 259Index327defuzzification function, 102, 105, 107-108, 111 defuzzifications, 17-18, 29, 34 defuzzifier, 181, 242density function, 122 dependency analysis, 258 dependency structure, 259 dependent loop level, 279depth control, 202-203depth controller, 202detection point, 169deviation, 79, 85, 185-188, 224, 251, 253, 262, 265, 268, 276, 288 dilation, 117discriminated rules, 251 discrimination, 251, 252distance function, 119-121 distance sensor, 167, 171 distribution function, 259domain knowledge, 254-255 domain-specific attributes, 251 Doppler frequency shift, 87 downhill simplex algorithm, 77, 79 downwash, 209drag reduction, 244driver’s intention estimator, 148 dutch roll, 212dynamic braking, 261-262 dynamic fuzzy system, 286, 304 dynamic tracking trajectory, 98Eedge composition, 108edge detection, 108 eigenvalues, 6-7, 212electrical coupling effect, 85, 88 electrical coupling effects, 87 equilibrium point, 207, 216 equivalent control, 194erosion, 117error rates, 96estimation, 34, 53, 119, 251, 283, 295, 302Euler angles, 208evaluation function, 258 evolution, 45, 133, 208, 251 execution layer, 262-266, 277 expert knowledge, 160, 191, 262 expert segmentation, 121-122 extended sup-star composition, 182 Ffault accommodation, 284fault clearing states, 271, 274fault detection, 288-289, 295fault diagnosis, 284fault durations, 271, 274fault isolation, 284, 288fault point, 270-271, 273-274fault tolerant control, 288fault trajectories, 271feature extraction, 256fiber glass hull, 193fin forces, 210final segmentation, 117final threshold, 116fine fuzzy controller, 90finer lookup table, 34finite element method, 318finite impulse responses, 288firing weights, 229fitness function, 59-60, 257flap angles, 209flight aerodynamic model, 247 flight envelope, 207, 214, 217flight path angle, 210flight trajectory, 208, 223footprint of uncertainty, 176, 179 formation geometry, 238, 247 formation trajectory, 246forward driving mode, 163, 167, 169 forward flight control, 217 forward flight speed, 217forward neural network, 288 forward velocity, 208, 214, 217, 219-220forward velocity tracking, 208 fossil power plants, 284-285, 296 four-dimensional synoptic data, 191 four-generator test system, 269 Fourier filter, 133four-quadrant detector, 79, 87, 92, 96foveal avascular zone, 123fundus images, 115, 121, 124 fuselage, 208-210Index 328fuselage axes, 208-209fuselage incidence, 210fuzz-C, 45fuzzifications, 18, 25fuzzifier, 181-182fuzzy ACC controller, 138fuzzy aggregation operator, 293 fuzzy ASICs, 37-38, 50fuzzy binarization algorithm, 110 fuzzy CC controller, 138fuzzy clustering algorithm, 106, 108 fuzzy constraints, 286, 291-292 fuzzy control surface, 54fuzzy damage-mitigating control, 284fuzzy decomposition, 108fuzzy domain, 102, 106fuzzy edge detection, 111fuzzy error interpolation, 300, 302, 305-306, 309, 313fuzzy filter, 104fuzzy gain scheduler, 217-218 fuzzy gain-scheduler, 207-208, 220 fuzzy geometry, 110-111fuzzy I controller, 76fuzzy image processing, 102, 106, 111, 124fuzzy implication rules, 27-28 fuzzy inference system, 17, 25, 27, 35-36, 207-208, 302, 304-306 fuzzy interpolation, 300, 302, 305- 307, 309, 313fuzzy interpolation method, 309 fuzzy interpolation technique, 300, 309, 313fuzzy interval control, 177fuzzy mapping rules, 27fuzzy model following control system, 84fuzzy modeling methods, 255 fuzzy navigation algorithm, 244 fuzzy operators, 104-105, 111 fuzzy P controller, 71, 73fuzzy PD controller, 69fuzzy perimeter, 110-111fuzzy PI controllers, 61fuzzy PID controllers, 53, 64-65, 80 fuzzy production rules, 315fuzzy reference governor, 285 Fuzzy Robust Controller, 7fuzzy set averages, 116, 124-125 fuzzy sets, 7, 19, 22, 24, 27, 36, 45, 115, 120-121, 124-125, 151, 176-182, 184-188, 192, 228, 262, 265-266fuzzy sliding mode controller, 192, 196-197fuzzy sliding surface, 192fuzzy subsets, 152, 200fuzzy variable boundary layer, 192 fuzzyTECH, 45Ggain margins, 207gain scheduling, 193, 207, 208, 211, 217, 220gas turbines, 279Gaussian membership function, 7 Gaussian waveform, 25 Gaussian-Bell waveforms, 304 gear position decision, 145, 147 gear-operating lever, 147general window function, 105 general-purpose microprocessors, 37-38, 44genetic algorithm, 54, 59, 192, 208, 257-258genetic operators, 59-60genetic-inclined search, 257 geometric modeling, 56gimbal motor, 90, 96global gain-scheduling, 220global linear ARX model, 284 global navigation satellite systems, 141global position system, 224goal seeking behaviour, 186-187 governor valves80, 2HHamiltonian function, 261, 277 hard constraints, 283, 293 heading angle, 226, 228, 230, 239, 240-244, 246heading angle control, 240Index329heading controller, 194, 201-202 heading error rate, 194, 201 heading speed, 226heading velocity control, 240 heat recovery steam generator, 279 hedges, 103-104height method, 29helicopter, 207-212, 214, 217, 220 helicopter control matrix, 211 helicopter flight control, 207 Heneghan method, 116-117, 121-124heuristic search, 258 hierarchical approaches, 261 hierarchical architecture, 185 hierarchical fuzzy processors, 261 high dimensional systems, 191 high stepping rates, 84hit-miss topology, 119home position, 96horizontal tail plane, 209 horizontal tracker, 90hostile, 223human domain experts, 255 human visual system, 101hybrid system framework, 295 hyperbolic tangent function, 195 hyperplane, 192-193, 196 hysteresis thres olding, 116-117hIIF-THEN rule, 27-28image binarization, 106image complexity, 104image fuzzification function, 111 image segmentation, 124image-expert, 122-123indicator function, 121inert, 223inertia frame, 238inference decision methods, 317 inferential conclusion, 317 inferential decision, 317 injection molding process, 315 inner loop controller, 87integral time absolute error, 54 inter-class similarity, 252 internal dependencies, 169 interpolation property, 203 interpolative nature, 262 intersection, 20, 23-24, 31, 180 interval sets, 178interval type-2 FLC, 181interval type-2 fuzzy sets, 177, 180-181, 184inter-vehicle gap, 135intra-class similarity, 252inverse dynamics control, 228, 230 inverse dynamics method, 227 inverse kinema c, 299tiJ - Kjoin, 180Kalman gain, 213kinematic model, 299kinematic modeling, 299-300 knowledge based gear position decision, 148, 153knowledge reasoning layer, 132 knowledge representation, 250 knowledge-bas d GPD model, 146eLlabyrinths, 169laser interferometer transducer, 83 laser tracker, 301laser tracking system, 53, 63, 65, 75, 78-79, 83-85, 87, 98, 301lateral control, 131, 138lateral cyclic pitch angle, 209 lateral flapping angle, 210 leader, 238-239linear control surface, 55linear fuzzy PI, 61linear hover model, 213linear interpolation, 300-301, 306-307, 309, 313linear interpolation method, 309 linear optimal controller, 207, 217 linear P controller, 73linear state feedback controller, 7 linear structures, 117linear switching line, 198linear time-series models, 283 linguistic variables, 18, 25, 27, 90, 102, 175, 208, 258Index 330load shedding, 261load-following capabilities, 288, 297 loading dock, 159-161, 170, 172 longitudinal control, 130-132 longitudinal cyclic pitch angle, 209 longitudinal flapping angle, 210 lookup table, 18, 31-35, 40, 44, 46, 47-48, 51, 65, 70, 74, 93, 300, 302, 304-305lower membership functions, 179-180LQ feedback gains, 208LQ linear controller, 208LQ optimal controller, 208LQ regulator, 208L-R fuzzy numbers, 121 Luenburger observer, 6Lyapunov func on, 5, 192, 284tiMMamdani model, 40, 46 Mamdani’s method, 242 Mamdani-type controller, 208 maneuverability, 164, 207, 209, 288 manual transmissions, 145 mapping function, 102, 104 marginal distribution functions, 259 market-basket analysis, 251-252 massive databases, 249matched filtering, 115 mathematical morphology, 117, 127 mating pool, 59-60max member principle, 106max-dot method, 40-41, 46mean distance function, 119mean max membership, 106mean of maximum method, 29 mean set, 118-121measuring beam, 86mechanical coupling effects, 87 mechanical layer, 132median filter, 105meet, 7, 50, 139, 180, 183, 302 membership degree, 39, 257 membership functions, 18, 25, 81 membership mapping processes, 56 miniature acrobatic helicopter, 208 minor steady state errors, 217 mixed-fuzzy controller, 92mobile robot control, 130, 175, 181 mobile robots, 171, 175-176, 183, 187-189model predictive control, 280, 287 model-based control, 224 modeless compensation, 300 modeless robot calibration, 299-301, 312-313modern combined-cycle power plant, 279modular structure, 172mold-design optimization, 323 mold-design process, 323molded part, 318-321, 323 morphological methods, 115motor angular acceleration, 3 motor plant, 3motor speed control, 2moving average filter, 105 multilayer fuzzy logic control, 276 multimachine power system, 262 multivariable control, 280 multivariable fuzzy PID control, 285 multivariable self-tuning controller, 283, 295mutation, 59mutation probability, 59-60mutual interference, 88Nnavigation control, 160neural fuzzy control, 19, 36neural networks, 173, 237, 255, 280, 284, 323neuro-fuzzy control, 237nominal plant, 2-4nonlinear adaptive control, 237non-linear control, 2, 159 nonlinear mapping, 55nonlinear switching curve, 198-199 nonlinear switching function, 200 nonvolatile memory, 44 normalized universe, 266Oobjective function, 59, 74-75, 77, 107, 281-282, 284, 287, 289-291,Index331295obstacle avoidance, 166, 169, 187-188, 223-225, 227, 231 obstacle avoidance behaviour, 187-188obstacle sensor, 224, 228off-line defuzzification, 34off-line fuzzy inference system, 302, 304off-line fuzzy technology, 300off-line lookup tables, 302 offsprings, 59-60on-line dynamic fuzzy inference system, 302online tuning, 203open water trial, 202operating point, 210optical platform, 92optimal control table, 300optimal feedback gain, 208, 215-216 optimal gains, 207original domain, 102outer loop controller, 85, 87outlier analysis, 251, 253output control gains, 92 overshoot, 3-4, 6-7, 60-61, 75-76, 94, 96, 193, 229, 266Ppath tracking, 223, 232-234 pattern evaluation, 250pattern vector, 150-151PD controller, 4, 54-55, 68-69, 71, 74, 76-77, 79, 134, 163, 165, 202 perception domain, 102 performance index, 60, 207 perturbed plants, 3, 7phase margins, 207phase-plan mapping fuzzy control, 19photovoltaic power systems, 261 phugoid mode, 212PID, 1-4, 8, 13, 19, 53, 61, 64-65, 74, 80, 84-85, 87-90, 92-98, 192 PID-fuzzy control, 19piecewise nonlinear surface, 193 pitch angle, 202, 209, 217pitch controller, 193, 201-202 pitch error, 193, 201pitch error rate, 193, 201pitch subsidence, 212planetary gearbox, 145point-in-time transaction, 252 polarizing beam-splitter, 86 poles, 4, 94, 96position sensor detectors, 84 positive definite matrix, 213post fault, 268, 270post-fault trajectory, 273pre-defined membership functions, 302prediction, 251, 258, 281-283, 287, 290predictive control, 280, 282-287, 290-291, 293-297predictive supervisory controller, 284preview distance control, 129 principal regulation level, 279 probabilistic reasoning approach, 259probability space, 118Problem understanding phases, 254 production rules, 316pursuer car, 136, 138-140 pursuer vehicle, 136, 138, 140Qquadrant detector, 79, 92 quadrant photo detector, 85 quadratic optimal technology, 208 quadrilateral ob tacle, 231sRradial basis function, 284 random closed set, 118random compact set, 118-120 rapid environment assessment, 191 reference beam, 86relative frame, 240relay control, 195release distance, 169residual forces, 217retinal vessel detection, 115, 117 RGB band, 115Riccati equation, 207, 213-214Index 332rise time, 3, 54, 60-61, 75-76road-environment estimator, 148 robot kinematics, 299robot workspace, 299-302, 309 robust control, 2, 84, 280robust controller, 2, 8, 90robust fuzzy controller, 2, 7 robustness property, 5, 203roll subsidence, 212rotor blade flap angle, 209rotor blades, 210rudder, 193, 201rule base size, 191, 199-200rule output function, 191, 193, 198-199, 203Runge-Kutta m thod, 61eSsampling period, 96saturation function, 195, 199 saturation functions, 162scaling factor, 54, 72-73scaling gains, 67, 69S-curve waveform, 25secondary membership function, 178 secondary memberships, 179, 181 selection, 59self-learning neural network, 159 self-organizing fuzzy control, 261 self-tuning adaptive control, 280 self-tuning control, 191semi-positive definite matrix, 213 sensitivity indices, 177sequence-based analysis, 251-252 sequential quadratic programming, 283, 292sets type-reduction, 184setting time, 54, 60-61settling time, 75-76, 94, 96SGA, 59shift points, 152shift schedule algorithms, 148shift schedules, 152, 156shifting control, 145, 147shifting schedules, 146, 152shift-schedule tables, 152sideslip angle, 210sigmoidal waveform, 25 sign function, 195, 199simplex optimal algorithm, 80 single gimbal system, 96single point mass obstacle, 223 singleton fuzzification, 181-182 sinusoidal waveform, 94, 300, 309 sliding function, 192sliding mode control, 1-2, 4, 8, 191, 193, 195-196, 203sliding mode fuzzy controller, 193, 198-200sliding mode fuzzy heading controller, 201sliding pressure control, 280 sliding region, 192, 201sliding surface, 5-6, 192-193, 195-198, 200sliding-mode fuzzy control, 19 soft constraints, 281, 287space-gap, 135special-purpose processors, 48 spectral mapping theorem, 216 speed adaptation, 138speed control, 2, 84, 130-131, 133, 160spiral subsidence, 212sporadic alternations, 257state feedback controller, 213 state transition, 167-169state transition matrix, 216state-weighting matrix, 207static fuzzy logic controller, 43 static MIMO system, 243steady state error, 4, 54, 79, 90, 94, 96, 98, 192steam turbine, 279steam valving, 261step response, 4, 7, 53, 76, 91, 193, 219stern plane, 193, 201sup operation, 183supervisory control, 191, 280, 289 supervisory layer, 262-264, 277 support function, 118support of a fuzzy set, 26sup-star composition, 182-183 surviving solutions, 257Index333swing curves, 271, 274-275 switching band, 198switching curve, 198, 200 switching function, 191, 194, 196-198, 200switching variable, 228system trajector192, 195y,Ttail plane, 210tail rotor, 209-210tail rotor derivation, 210Takagi-Sugeno fuzzy methodology, 287target displacement, 87target time gap, 136t-conorm maximum, 132 thermocouple sensor fault, 289 thickness variable, 319-320three-beam laser tracker, 85three-gimbal system, 96throttle pressure, 134throttle-opening degree, 149 thyristor control, 261time delay, 63, 75, 91, 93-94, 281 time optimal robust control, 203 time-gap, 135-137, 139-140time-gap derivative, 136time-gap error, 136time-invariant fuzzy system, 215t-norm minimum, 132torque converter, 145tracking error, 79, 84-85, 92, 244 tracking gimbals, 87tracking mirror, 85, 87tracking performance, 84-85, 88, 90, 192tracking speed, 75, 79, 83-84, 88, 90, 92, 97, 287trajectory mapping unit, 161, 172 transfer function, 2-5, 61-63 transient response, 92, 193 transient stability, 261, 268, 270, 275-276transient stability control, 268 trapezoidal waveform, 25 triangular fuzzy set, 319triangular waveform, 25 trim, 208, 210-211, 213, 217, 220, 237trimmed points, 210TS fuzzy gain scheduler, 217TS fuzzy model, 207, 290TS fuzzy system, 208, 215, 217, 220 TS gain scheduler, 217TS model, 207, 287TSK model, 40-41, 46TS-type controller, 208tuning function, 70, 72turbine following mode, 280, 283 turn rate, 210turning rate regulation, 208, 214, 217two-DOF mirror gimbals, 87two-layered FLC, 231two-level hierarchy controllers, 275-276two-module fuzzy logic control, 238 type-0 systems, 192type-1 FLC, 176-177, 181-182, 185- 188type-1 fuzzy sets, 177-179, 181, 185, 187type-1 membership functions, 176, 179, 183type-2 FLC, 176-177, 180-183, 185-189type-2 fuzzy set, 176-180type-2 interval consequent sets, 184 type-2 membership function, 176-178type-reduced set, 181, 183-185type-reduction,83-1841UUH-1H helicopter, 208uncertain poles, 94, 96uncertain system, 93-94, 96 uncertain zeros, 94, 96underlying domain, 259union, 20, 23-24, 30, 177, 180unit control level, 279universe of discourse, 19-24, 42, 57, 151, 153, 305unmanned aerial vehicles, 223 unmanned helicopter, 208Index 334unstructured dynamic environments, 177unstructured environments, 175-177, 179, 185, 187, 189upper membership function, 179Vvalve outlet pressure, 280vapor pressure, 280variable structure controller, 194, 204velocity feedback, 87vertical fin, 209vertical tracker, 90vertical tracking gimbal, 91vessel detection, 115, 121-122, 124-125vessel networks, 117vessel segmentation, 115, 120 vessel tracking algorithms, 115 vision-driven robotics, 87Vorob’ev fuzzy set average, 121-123 Vorob'ev mean, 118-120vortex, 237 WWang and Mendel’s algorithm, 257 WARP, 49weak link, 270, 273weighing factor, 305weighting coefficients, 75 weighting function, 213weld line, 315, 318-323western states coordinating council, 269Westinghouse turbine-generator, 283 wind–diesel power systems, 261 Wingman, 237-240, 246wingman aircraft, 238-239 wingman veloc y, 239itY-ZYager operator, 292Zana-Klein membership function, 124Zana-Klein method, 116-117, 121, 123-124zeros, 94, 96µ-law function, 54µ-law tuning method, 54。
人工智能基础(习题卷9)
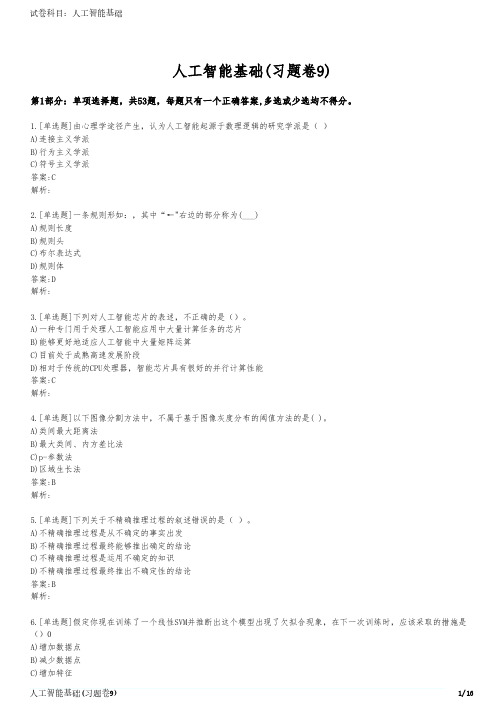
人工智能基础(习题卷9)第1部分:单项选择题,共53题,每题只有一个正确答案,多选或少选均不得分。
1.[单选题]由心理学途径产生,认为人工智能起源于数理逻辑的研究学派是( )A)连接主义学派B)行为主义学派C)符号主义学派答案:C解析:2.[单选题]一条规则形如:,其中“←"右边的部分称为(___)A)规则长度B)规则头C)布尔表达式D)规则体答案:D解析:3.[单选题]下列对人工智能芯片的表述,不正确的是()。
A)一种专门用于处理人工智能应用中大量计算任务的芯片B)能够更好地适应人工智能中大量矩阵运算C)目前处于成熟高速发展阶段D)相对于传统的CPU处理器,智能芯片具有很好的并行计算性能答案:C解析:4.[单选题]以下图像分割方法中,不属于基于图像灰度分布的阈值方法的是( )。
A)类间最大距离法B)最大类间、内方差比法C)p-参数法D)区域生长法答案:B解析:5.[单选题]下列关于不精确推理过程的叙述错误的是( )。
A)不精确推理过程是从不确定的事实出发B)不精确推理过程最终能够推出确定的结论C)不精确推理过程是运用不确定的知识D)不精确推理过程最终推出不确定性的结论答案:B解析:6.[单选题]假定你现在训练了一个线性SVM并推断出这个模型出现了欠拟合现象,在下一次训练时,应该采取的措施是()0A)增加数据点D)减少特征答案:C解析:欠拟合是指模型拟合程度不高,数据距离拟合曲线较远,或指模型没有很好地捕 捉到数据特征,不能够很好地拟合数据。
可通过增加特征解决。
7.[单选题]以下哪一个概念是用来计算复合函数的导数?A)微积分中的链式结构B)硬双曲正切函数C)softplus函数D)劲向基函数答案:A解析:8.[单选题]相互关联的数据资产标准,应确保()。
数据资产标准存在冲突或衔接中断时,后序环节应遵循和适应前序环节的要求,变更相应数据资产标准。
A)连接B)配合C)衔接和匹配D)连接和配合答案:C解析:9.[单选题]固体半导体摄像机所使用的固体摄像元件为( )。
一种大规模流式数据聚类方法在交通热点分析中的应用

一种大规模流式数据聚类方法在交通热点分析中的应用牟向伟;陈燕;曹妍【摘要】In order to improve the efficiency of traffic hotspots analysis in large-scale streaming data environment,a two-phase flow data clustering framework is proposed,in the first stage,this framework produces macro cluster based on improved Canopy algorithm,in the second K-means clustering algorithm uses the cluster center from macro cluster to generate more accurate clustering results.In the experiment,this framework was programed to analyze a dataset of Beijing taxi GPS positioning data which had been simulated as streaming data.Clustering results was used to analyze traffic hotspot and shown in the heat map and WebGIS,which can directly find relatively taxi activity hot spots and road,the results of hot spots are consistent with daily experience.These experiment results show that the two-phase flow data clustering framework can real-time cluster the streaming data and are available for the user query in any time window.the two-phase flow data clustering framework application in real-time traffic hotspot analysis provides theory value and application significance for real time traffic analysis,traffic planning and congestion management.%为了提高在大规模流式数据环境下交通热点区域分析的算法效率,提出了一种流式数据两阶段方法;该方法在第一阶段使用基于改进Canopy算法进行粗聚类并产生宏簇,在第二阶段使用K-means算法进行细聚类;并以粗聚类产生的宏簇个数和类簇中心位置为指导产生更加准确的微簇聚类结果.在试验中,使用流式数据两阶段方法对北京市出租车的定位数据进行了聚类分析;并结合热力图和电子地图对聚类结果进行可视化表达,在最终的热力分析结果中可以直观地发现出租车活动较为频繁的热点区域和线路,且与日常出行经验相符合.试验结果表明该算法能够实时地对流式数据进行聚类分析,产生的数据结果可供用户在任意时间窗口范围进行查询分析,有助于为交通活动情况实时分析、交通规划和拥堵治理等方面提供有价值的理论参考依据.【期刊名称】《科学技术与工程》【年(卷),期】2017(017)015【总页数】8页(P260-267)【关键词】流式数据聚类;实时计算;交通热点分析;Canopy;K-means【作者】牟向伟;陈燕;曹妍【作者单位】大连海事大学交通运输管理学院,大连116026;大连海事大学交通运输管理学院,大连116026;大连海事大学交通运输管理学院,大连116026【正文语种】中文【中图分类】TP391.4交通热点区域是指在一定时间范围内能够持续带来大规模、高频次交通活动的区域,交通热点区域往往对周边路造成区域性交通拥堵影响,因此交通热点区域的发现有助于及时对交通拥堵情况进行分析,是改善交通状况的关键技术与前提条件之一,可以为交通规划、资源调度、拥堵治理、政府决策等方面提供有价值的理论参考依据。
自动聚类算法确定cluster数目的方法
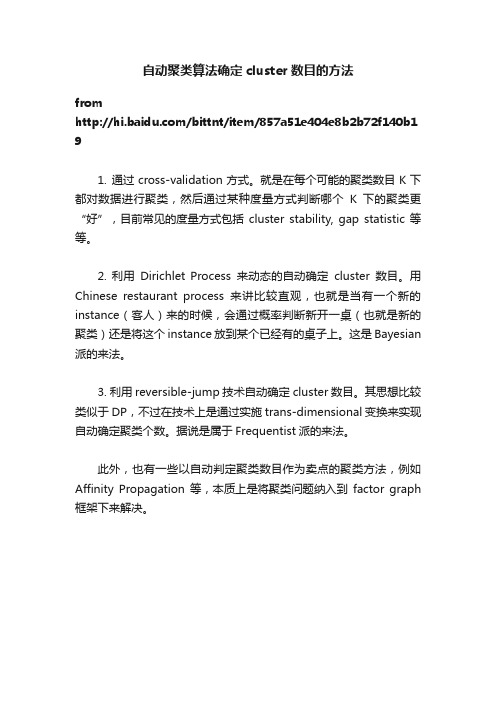
自动聚类算法确定cluster数目的方法
from
/bittnt/item/857a51e404e8b2b72f140b1 9
1. 通过cross-validation方式。
就是在每个可能的聚类数目K下都对数据进行聚类,然后通过某种度量方式判断哪个K下的聚类更“好”,目前常见的度量方式包括cluster stability, gap statistic等等。
2. 利用Dirichlet Process来动态的自动确定cluster数目。
用Chinese restaurant process来讲比较直观,也就是当有一个新的instance(客人)来的时候,会通过概率判断新开一桌(也就是新的聚类)还是将这个 instance放到某个已经有的桌子上。
这是Bayesian 派的来法。
3. 利用reversible-jump技术自动确定cluster数目。
其思想比较类似于DP,不过在技术上是通过实施trans-dimensional变换来实现自动确定聚类个数。
据说是属于Frequentist派的来法。
此外,也有一些以自动判定聚类数目作为卖点的聚类方法,例如Affinity Propagation等,本质上是将聚类问题纳入到factor graph 框架下来解决。
通俗讲解dirichlet 聚类

通俗讲解dirichlet 聚类英文回答:Dirichlet clustering, also known as Dirichlet process mixture modeling, is a probabilistic clustering algorithm that allows for the automatic determination of the number of clusters in a dataset. It is named after the Dirichlet distribution, which is used to model the distribution of cluster assignments.In Dirichlet clustering, each data point is assigned to one of the clusters, and the cluster assignments are determined based on the similarity between data points. However, unlike traditional clustering algorithms,Dirichlet clustering does not require the number ofclusters to be specified in advance. Instead, it uses anon-parametric Bayesian approach to automatically determine the number of clusters based on the data.The Dirichlet process is a stochastic process thatallows for an infinite number of clusters. It is characterized by two parameters: a concentration parameter, which controls the number of clusters, and a base distribution, which specifies the distribution of cluster assignments. The concentration parameter determines the probability of creating a new cluster when a new data point is encountered, while the base distribution determines the distribution of data points within each cluster.To perform Dirichlet clustering, we start with an empty set of clusters and iteratively assign data points to clusters. At each iteration, we calculate the probability of assigning a data point to each existing cluster, as well as the probability of creating a new cluster. The data point is then assigned to the cluster with the highest probability. If the probability of creating a new clusteris higher than the probability of assigning the data point to any existing cluster, a new cluster is created.The process continues until all data points have been assigned to clusters. The resulting clusters can then be used for further analysis or visualization.中文回答:Dirichlet聚类,也被称为Dirichlet过程混合建模,是一种概率聚类算法,可以自动确定数据集中的聚类数量。
- 1、下载文档前请自行甄别文档内容的完整性,平台不提供额外的编辑、内容补充、找答案等附加服务。
- 2、"仅部分预览"的文档,不可在线预览部分如存在完整性等问题,可反馈申请退款(可完整预览的文档不适用该条件!)。
- 3、如文档侵犯您的权益,请联系客服反馈,我们会尽快为您处理(人工客服工作时间:9:00-18:30)。
Nicolai Czink1,2 , Pierluigi Cera1 , Jari Salo3 , Ernst Bonek1 , Jukka-Pekka Nuutinen4 , Juha Ylitalo4,5
I. I NTRODUCTION Many advanced radio channel models base on the concept of multi-path clusters consisting of many multi-path components (MPCs) showing similar parameters such as azimuth and elevation of arrival and departure, and delay [1], [2]. The major problem of these models is the accurate parametrisation of clusters, where the parameters have to be extracted from measurement data. Currently there is no fully automatic clustering algorithm available to identify clusters from multi-dimensional parametric MIMO channel estimates. In many papers visual inspection of measurement data was used [3], [4], which becomes impractical for large amounts of measurement data. Recently, a semi-automatic algorithm was introduced in [5], which bases on clustering windowed parametric estimates and tracking the cluster centroids. The window-based clustering algorithm was subsequently improved by using the multi-path component distance (MCD) as the distance function in [6], [7]. In this paper we propose to use a new framework consisting of three algorithms to significantly improve the clustering performance: (i) a new clustering algorithm, (ii) a cluster validation metric and (iii) an improved cluster shape pruning. This framework leads to an intrinsic and intuitive definition
1
Institut f¨ ur Nachrichtentechnik und Hochfrequenztechnik, Technische Universit¨ at Wien, Austria 2 Forschungszentrum Telekommunikation Wien (ftw.), Wien, Austria 3 Radio Laboratory/SMARAD, Helsinki University of Technology, Finland 4 Elektrobit Testing Ltd., Oulu, Finland 5 Centre for Wireless Communications, University of Oulu, Finland of a “cluster” itself. In the following we will describe the framework, its single components and their interaction. Finally we assess the performance of the new framework by applying it to measurement data. Cluster parameters gained from this procedure are presented in [8]. II. P ROBLEM DESCRIPTION The starting point is a large number of multi-dimensional parametric channel estimation data, obtained from MIMO measurements. The measurements provide numerous snapshots of the impulse response of the – typically time-varying – radio channel. These measurements are fed to a high-resolution algorithm, e.g. SAGE [9], to estimate the channel parameters for each snapshot individually. It has been found in several MIMO studies that these parameters tend to appear in clusters, i.e. in groups of multi-path components (MPCs) with similar parameters, e.g. [3], [4]. The problem is to find an automatic procedure to identify and track these clusters. We consider one data window with a number of L MPCs, where every single MPC is represented by its power Pl , l = 1 . . . L, and a parameter vector xl containing the delay (τ ), azimuth and elevation AoA (ϕAoA , θAoA ) and azimuth and elevation AoD (ϕAoD , θAoD ). The data for all paths are collected in the vector P = [P1 . . . PL ]T and the matrix X = [x1 . . . xL ]T . III. F RAMEWORK For automatic clustering, a range for the expected number of clusters has to be specified first, then the algorithm assigns each MPC to a cluster and estimates the correct number of clusters (“cluster validation”) (see Algorithm 1). Initially, a range [Kmin , Kmax ] for the possible number of clusters has to be specified by the user. The number of clusters, K , and the data from all MPCs, P and X, are external parameters for the clustering algorithm. For each possible K , the clustering algorithm KPowerMeans is performed, the results are collected in the data sets RK . Subsequently, each result is validated by CombinedValidate which provides the validation index vK . The optimum number of clusters Kopt is finally determined by the largest validation index vK with corresponding cluster set Ropt . This optimum set is then pruned by ShapePrune for improved visualisation.
Abstract— We present a solution to the problem of identifying clusters from MIMO measurement data in a data window, with a minimum of user interaction. Conventionally, visual inspection has been used for the cluster identification. However this approach is impractical for a large amount of measurement data. Moreover, visual methods lack an accurate definition of a “cluster” itself. We introduce a framework that is able to cluster multi-path components (MPCs), decide on the number of clusters, and discard outliers. For clustering we use the K-means algorithm, which iteratively moves a number of cluster centroids through the data space to minimize the total difference between MPCs and their closest centroid. We significantly improve this algorithm by following changes: (i) as the distance metric we use the multipath component distance (MCD), (ii) the distances are weighted by the powers of the MPCs. The implications of these changes result in a definition of a “cluster” itself that appeals to intuition. We assess the performance of the new algorithm by clustering real-world measurement data from an indoor big hall environment. Keywords—MIMO channel; MPC clustering; geometry-based stochastic channel models