Combining relevance feedback and genetic algorithms in an Internet information personalizat
关联理论视角下双语词典词条信息的传递与加工
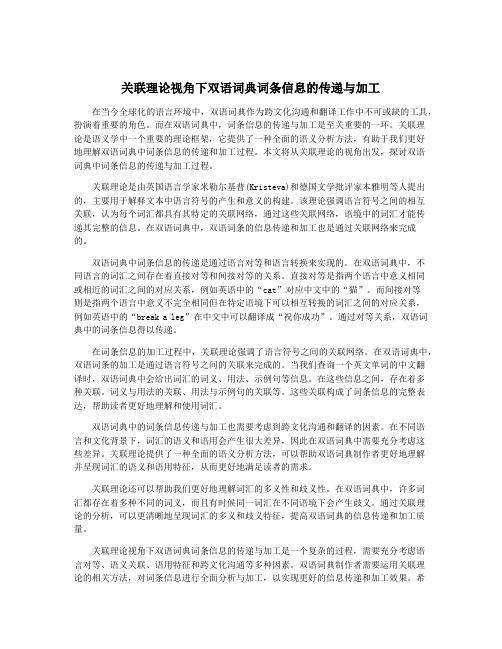
关联理论视角下双语词典词条信息的传递与加工在当今全球化的语言环境中,双语词典作为跨文化沟通和翻译工作中不可或缺的工具,扮演着重要的角色。
而在双语词典中,词条信息的传递与加工是至关重要的一环。
关联理论是语义学中一个重要的理论框架,它提供了一种全面的语义分析方法,有助于我们更好地理解双语词典中词条信息的传递和加工过程。
本文将从关联理论的视角出发,探讨双语词典中词条信息的传递与加工过程。
关联理论是由英国语言学家米勒尔基普(Kristeva)和德国文学批评家本雅明等人提出的,主要用于解释文本中语言符号的产生和意义的构建。
该理论强调语言符号之间的相互关联,认为每个词汇都具有其特定的关联网络,通过这些关联网络,语境中的词汇才能传递其完整的信息。
在双语词典中,双语词条的信息传递和加工也是通过关联网络来完成的。
双语词典中词条信息的传递是通过语言对等和语言转换来实现的。
在双语词典中,不同语言的词汇之间存在着直接对等和间接对等的关系。
直接对等是指两个语言中意义相同或相近的词汇之间的对应关系,例如英语中的“cat”对应中文中的“猫”。
而间接对等则是指两个语言中意义不完全相同但在特定语境下可以相互转换的词汇之间的对应关系,例如英语中的“break a leg”在中文中可以翻译成“祝你成功”。
通过对等关系,双语词典中的词条信息得以传递。
在词条信息的加工过程中,关联理论强调了语言符号之间的关联网络。
在双语词典中,双语词条的加工是通过语言符号之间的关联来完成的。
当我们查询一个英文单词的中文翻译时,双语词典中会给出词汇的词义、用法、示例句等信息。
在这些信息之间,存在着多种关联。
词义与用法的关联、用法与示例句的关联等。
这些关联构成了词条信息的完整表达,帮助读者更好地理解和使用词汇。
双语词典中的词条信息传递与加工也需要考虑到跨文化沟通和翻译的因素。
在不同语言和文化背景下,词汇的语义和语用会产生很大差异,因此在双语词典中需要充分考虑这些差异。
开始疯狂写作业英语
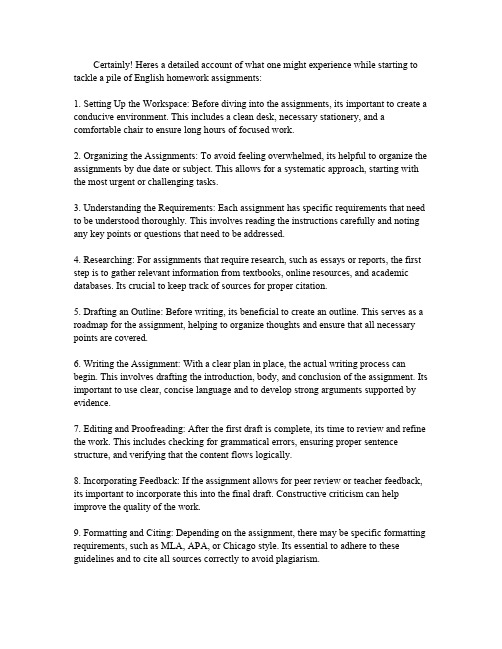
Certainly!Heres a detailed account of what one might experience while starting to tackle a pile of English homework assignments:1.Setting Up the Workspace:Before diving into the assignments,its important to create a conducive environment.This includes a clean desk,necessary stationery,and a comfortable chair to ensure long hours of focused work.anizing the Assignments:To avoid feeling overwhelmed,its helpful to organize the assignments by due date or subject.This allows for a systematic approach,starting with the most urgent or challenging tasks.3.Understanding the Requirements:Each assignment has specific requirements that need to be understood thoroughly.This involves reading the instructions carefully and noting any key points or questions that need to be addressed.4.Researching:For assignments that require research,such as essays or reports,the first step is to gather relevant information from textbooks,online resources,and academic databases.Its crucial to keep track of sources for proper citation.5.Drafting an Outline:Before writing,its beneficial to create an outline.This serves as a roadmap for the assignment,helping to organize thoughts and ensure that all necessary points are covered.6.Writing the Assignment:With a clear plan in place,the actual writing process can begin.This involves drafting the introduction,body,and conclusion of the assignment.Its important to use clear,concise language and to develop strong arguments supported by evidence.7.Editing and Proofreading:After the first draft is complete,its time to review and refine the work.This includes checking for grammatical errors,ensuring proper sentence structure,and verifying that the content flows logically.8.Incorporating Feedback:If the assignment allows for peer review or teacher feedback, its important to incorporate this into the final draft.Constructive criticism can help improve the quality of the work.9.Formatting and Citing:Depending on the assignment,there may be specific formatting requirements,such as MLA,APA,or Chicago style.Its essential to adhere to these guidelines and to cite all sources correctly to avoid plagiarism.10.Submitting the Assignment:Once the assignment is polished and meets all requirements,its ready for submission.This may involve uploading it to an online portal or handing it in physically.11.Reflecting on the Process:After submission,its helpful to reflect on the process. What worked well?What could be improved for next time?This reflection can lead to better strategies for future assignments.Remember,the key to successfully completing English homework is to break down the task into manageable parts,stay organized,and give yourself enough time to research, write,and revise.。
2007年全国硕士研究生考试英语真题及答案2
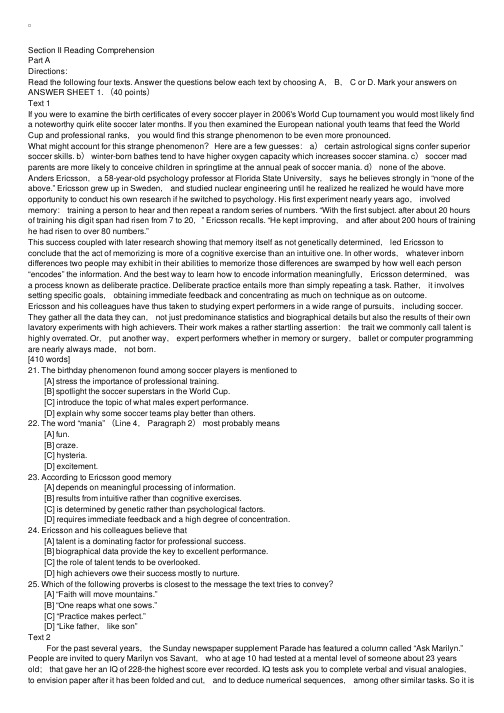
Section II Reading ComprehensionPart ADirections:Read the following four texts. Answer the questions below each text by choosing A, B, C or D. Mark your answers on ANSWER SHEET 1. (40 points)Text 1If you were to examine the birth certificates of every soccer player in 2006's World Cup tournament you would most likely find a noteworthy quirk elite soccer later months. If you then examined the European national youth teams that feed the World Cup and professional ranks, you would find this strange phenomenon to be even more pronounced.What might account for this strange phenomenon? Here are a few guesses: a) certain astrological signs confer superior soccer skills. b) winter-born bathes tend to have higher oxygen capacity which increases soccer stamina. c) soccer mad parents are more likely to conceive children in springtime at the annual peak of soccer mania. d) none of the above. Anders Ericsson, a 58-year-old psychology professor at Florida State University, says he believes strongly in “none of the above.” Ericsson grew up in Sweden, and studied nuclear engineering until he realized he realized he would have more opportunity to conduct his own research if he switched to psychology. His first experiment nearly years ago, involved memory: training a person to hear and then repeat a random series of numbers. “With the first subject. after about 20 hours of training his digit span had risen from 7 to 20,” Ericsson recalls. “He kept improving, and after about 200 hours of training he had risen to over 80 numbers.”This success coupled with later research showing that memory itself as not genetically determined, led Ericsson to conclude that the act of memorizing is more of a cognitive exercise than an intuitive one. In other words, whatever inborn differences two people may exhibit in their abilities to memorize those differences are swamped by how well each person “encodes” the information. And the best way to learn how to encode information meaningfully, Ericsson determined, was a process known as deliberate practice. Deliberate practice entails more than simply repeating a task. Rather, it involves setting specific goals, obtaining immediate feedback and concentrating as much on technique as on outcome.Ericsson and his colleagues have thus taken to studying expert performers in a wide range of pursuits, including soccer. They gather all the data they can, not just predominance statistics and biographical details but also the results of their own lavatory experiments with high achievers. Their work makes a rather startling assertion: the trait we commonly call talent is highly overrated. Or, put another way, expert performers whether in memory or surgery, ballet or computer programming are nearly always made, not born.[410 words]21. The birthday phenomenon found among soccer players is mentioned to [A] stress the importance of professional training. [B] spotlight the soccer superstars in the World Cup. [C] introduce the topic of what males expert performance. [D] explain why some soccer teams play better than others.22. The word “mania” (Line 4, Paragraph 2) most probably means [A] fun. [B] craze. [C] hysteria. [D] excitement.23. According to Ericsson good memory [A] depends on meaningful processing of information. [B] results from intuitive rather than cognitive exercises. [C] is determined by genetic rather than psychological factors. [D] requires immediate feedback and a high degree of concentration.24. Ericsson and his colleagues believe that [A] talent is a dominating factor for professional success. [B] biographical data provide the key to excellent performance. [C] the role of talent tends to be overlooked. [D] high achievers owe their success mostly to nurture.25. Which of the following proverbs is closest to the message the text tries to convey? [A] “Faith will move mountains.” [B] “One reaps what one sows.” [C] “Practice makes perfect.” [D] “Like father, like son”Text 2 For the past several years, the Sunday newspaper supplement Parade has featured a column called “Ask Marilyn.”People are invited to query Marilyn vos Savant, who at age 10 had tested at a mental level of someone about 23 years old; that gave her an IQ of 228-the highest score ever recorded. IQ tests ask you to complete verbal and visual analogies,to envision paper after it has been folded and cut, and to deduce numerical sequences, among other similar tasks. So it isa bit confusing when vos Savant fields such queries from the average Joe (whose IQ is 100) as, What's the difference between love and fondness? Or what is the nature of luck and coincidence? It's not obvious how the capacity to visualize objects and to figure out numerical patterns suits one to answer questions that have eluded some of the best poets and philosophers. Clearly, intelligence encompasses more than a score on a test. Just what does it means to be smart? How much of intelligence can be specified, and how much can we learn about it from neurology, genetics, computer science and other fields? The defining term of intelligence in humans still seems to be the IQ score, even though IQ tests are not given as often as they used to be. The test comes primarily in two forms: the Stanford-Binet Intelligence Scale and the Wechsler Intelligence Scales (both come in adult and children's version)。
Relevance feedback and inference networks

Abstract
Relevance feedback methods in information retrieval attempt to improve performance for a particular query by modifying the query, based on the user's reaction to the initial retrieved documents. Speci cally, the user's judgements of the relevance or non-relevance of some of the documents retrieved are used to add new terms to the query and to reweight query terms. For example, if all the documents, that the user judges as relevant, contain a particular term, then that term may be a good one to add to the original query 16]. Perhaps the relative importance of that term should also be increased. Given the apparent e ectiveness of relevance feedback techniques 15, 5], it is important that any proposed model of information retrieval includes these techniques.
基因敲除与转基因互补

基因敲除与转基因互补英文回答:Gene knockout and genetic complementation are two important techniques used in molecular biology and genetics research.Gene knockout refers to the process of intentionally disabling or "knocking out" a specific gene in an organism. This is typically done by introducing a mutation into the gene, rendering it non-functional. Gene knockout is used to study the function of a particular gene by observing the effects of its absence. For example, if a gene is suspected to be involved in a specific disease, researchers can create a knockout mouse model by disabling that gene and then observe the resulting phenotype to understand the gene's role in the disease.On the other hand, genetic complementation is a technique used to determine whether a specific phenotype iscaused by a mutation in a particular gene. It involves introducing a functional copy of the gene into an organism that carries a mutation in the same gene. If the introduced gene is able to restore the normal phenotype, it indicates that the mutation in the original organism was responsible for the observed phenotype. This technique is often used to confirm the causative role of a gene mutation in a particular disease or trait.Both gene knockout and genetic complementation have their own advantages and limitations. Gene knockout allows researchers to study the function of a specific gene by observing the effects of its absence. It helps in understanding the role of genes in development, disease, and other biological processes. On the other hand, genetic complementation helps in confirming the causative role of a gene mutation by restoring the normal phenotype. It provides evidence for the involvement of a specific gene in a particular phenotype or disease.中文回答:基因敲除和转基因互补是分子生物学和遗传学研究中两种重要的技术。
理解促进交流的英语作文
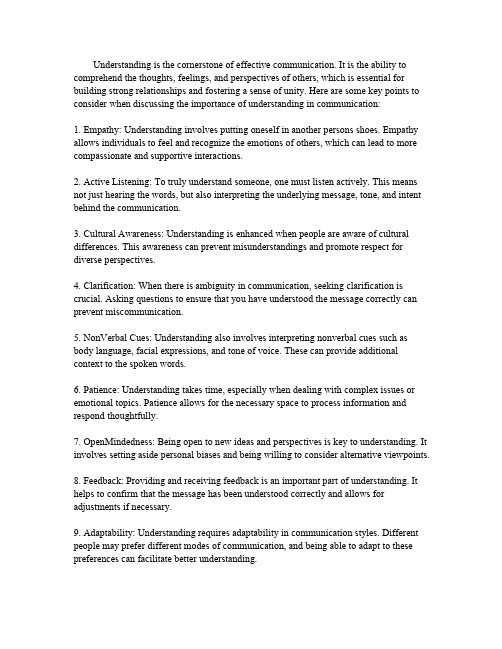
Understanding is the cornerstone of effective communication.It is the ability to comprehend the thoughts,feelings,and perspectives of others,which is essential for building strong relationships and fostering a sense of unity.Here are some key points to consider when discussing the importance of understanding in communication:1.Empathy:Understanding involves putting oneself in another persons shoes.Empathy allows individuals to feel and recognize the emotions of others,which can lead to more compassionate and supportive interactions.2.Active Listening:To truly understand someone,one must listen actively.This means not just hearing the words,but also interpreting the underlying message,tone,and intent behind the communication.3.Cultural Awareness:Understanding is enhanced when people are aware of cultural differences.This awareness can prevent misunderstandings and promote respect for diverse perspectives.4.Clarification:When there is ambiguity in communication,seeking clarification is crucial.Asking questions to ensure that you have understood the message correctly can prevent miscommunication.5.NonVerbal Cues:Understanding also involves interpreting nonverbal cues such as body language,facial expressions,and tone of voice.These can provide additional context to the spoken words.6.Patience:Understanding takes time,especially when dealing with complex issues or emotional topics.Patience allows for the necessary space to process information and respond thoughtfully.7.OpenMindedness:Being open to new ideas and perspectives is key to understanding.It involves setting aside personal biases and being willing to consider alternative viewpoints.8.Feedback:Providing and receiving feedback is an important part of understanding.It helps to confirm that the message has been understood correctly and allows for adjustments if necessary.9.Adaptability:Understanding requires adaptability in communication styles.Different people may prefer different modes of communication,and being able to adapt to these preferences can facilitate better understanding.10.Conflict Resolution:When conflicts arise,understanding is crucial for resolution.It involves recognizing the needs and concerns of all parties and working towards a mutually acceptable solution.In conclusion,understanding is a multifaceted aspect of communication that encompasses empathy,active listening,cultural awareness,and more.It is the foundation upon which effective communication is built,allowing for the exchange of ideas and feelings in a way that is respectful and productive.。
简述遗传和环境相互作用的反馈形式

简述遗传和环境相互作用的反馈形式反馈是指人们对于环境刺激所做出的各种相应行为。
1遗传与环境的相互作用之一(1)双向信息反馈双向信息反馈是指个体发生变异时所产生的结果可以通过个体自身的生化或分子反应进行检测,这就使遗传和环境之间的关系能够被理解,即:反馈可以在不同的水平进行。
例如,性别(一般女性在XX染色体上有XX基因)、身高、寿命等的遗传信息能够反馈给后代,而这些结果又可以在婴儿的成长中得到验证,即:儿童出生时的遗传优势或劣势会在成长的过程中逐渐显露。
作为信息的遗传物质的化学变化在生理上并不立即表现出来,而是要等到一段时间以后,从婴儿的血液里、细胞里、甚至大脑里才会出现,但是婴儿的智力却早已存在。
在心理方面,有经验的父母都知道,当宝宝对着镜子笑的时候,其实是在模仿他的父母。
(2)多级信息反馈一般认为,遗传和环境相互作用的最高级形式是多级信息反馈。
这里的多级信息反馈不仅包含了反馈信息传递的“量”,也包含了传递速度(反馈的速率)这样的“质”。
例如,在中国,高考状元们几乎没有一个人能够逃脱“状元定律”的影响,即使曾经遭遇过种种挫折的状元,也难免以重蹈覆辙。
家庭教育专家指出,父母是孩子最重要的榜样,父母希望孩子成为什么样的人,首先要努力去做那样的人;想让孩子有什么样的能力,父母自己也要有这样的能力。
从遗传学的角度看,孩子将成为什么样的人并不由他本身所决定,他必须接受父母的遗传基因,加上后天的培养和引导,才能达到我们期望的目标。
遗传和环境两种因素共同影响人类个体,在这两种因素中,遗传对人的影响比较大。
有研究显示,人的身高、容貌和体质特征主要由先天因素决定,遗传因素占60%;而后天因素则包括营养、锻炼和疾病等。
营养是基础,锻炼是保证,疾病是后盾。
遗传和环境对人的影响虽然相互交织在一起,但在遗传和环境对人的影响中,遗传对人的影响更具有决定性。
正是由于这个原因,我们的父母才会竭尽全力地把自己的最好的东西留给孩子。
Strategies for positive and negative relevance feedback in image retrieval

Strategies for positive and negative relevance feedback in image retrieval Henning M¨u ller,Wolfgang M¨u ller,St´e phane Marchand-Maillet and Thierry PunComputer Vision Group,University of Geneva,CH-1211Geneva4,SwitzerlandHenning.Mueller@cui.unige.ch tel.+41227057633,fax.+41227057780David McG.SquireSchool of Computer Science and Software Engineering,Monash University,Melbourne,AustraliaAbstractRelevance feedback has been shown to be a very effec-tive tool for enhancing retrieval results in text retrieval.In content-based image retrieval it is more and more fre-quently used and very good results have been obtained.However,too much negative feedback may destroy a queryas good features get negative weightings.This paper compares a variety of strategies for positiveand negative feedback.The performance evaluation of feed-back algorithms is a hard problem.To solve this,we ob-tain judgments from several users and employ an automatedfeedback scheme.We can then evaluate different techniquesusing the same ing automated feedback,theability of a system to adapt to the user’s needs can be mea-sured very effectively.Our study highlights the utility ofnegative feedback,especially over several feedback steps.1.IntroductionRelevance feedback(RF)has shown to be extremely use-ful in text retrieval(TR)applications[7],and is now be-ing applied in some content-based image retrieval systems(CBIRSs)[5,9].Since human perception of image similar-ity is both subjective and task-dependent[10,1],we believeRF to be an essential component of a CBIRS.By augment-ing the query with features from relevant and non-relevantretrieved images,a query can be produced which better rep-resents the user’s information need.Performance evaluation is a difficult problem in content-based image retrieval,largely due to the subjectivity andtask-dependence issues mentioned above.For these rea-sons evaluation must involve experiments with several realusers.Examples of such studies exist but much publishedwork contains little or no quantitative performance evalua-tion.The CBIR community still lacks a commonly accepted1Visual Information Processing for Enhanced Retrieval.Web page:http://cuiwww.unige.ch/˜viper/ploys both local and global image color and spatial fre-quency features,extracted at several scales,and their fre-quency statistics in both the image and the whole collection. The intention is to make available to the system low-level features which correspond(roughly)to those present in the human vision system.More than80000features are available to the system. Each image has such features,the mapping from features to images being stored in an invertedfile.The use of such a data structure,in conjunction with the feature weighting scheme,means that textual features are treated inexactly the same way as visual ones.Further details about the architecture of the Viper system can be found in[9].We use2500diverse images supplied by T´e l´e vision Su-isse Romande.In the experiment,3users gave judgments for14query images.The users chose different and varying numbers of relevant images for each query.These experi-ments are described in detail in[3].4.Feedback strategiesThe two main strategies for RF are either(1)to make separate queries for each feedback image and merge the query results or(2)to create a“pseudo-image”from the feedback images and execute a query with this image.Viper uses the second method by combining the features from the feedback images and normalizing their frequencies.4.1.Automated feedbackAutomated RF can be applied once user judgments for an image collection exist.Thus a reproducible RF for ev-ery user can be simulated based upon the judgments and the initial query results of a system.Via this technique,the flexibility of a system with respect to users’needs can be measured,e.g.by feeding back the images the user judged as relevant and which were returned in the top of a query result.This technique can be used to compare differ-ent feedback strategies or to enhance user queries by auto-matically creating negative feedback.4.1.1.Only positive feedbackPositive feedback is limited to preselected images and weights the features of these images more strongly.As all high ranked returned images have many features in com-mon,the non-relevant images may also be ranked highly in the next step.For this feedback,we select as relevant all the images from the initial query result which the user judged to be relevant.We chose images for feedback from thefirst 20highest ranked response images,which is a reasonable number to display on screen simultaneously.50is regarded as the maximum number of images a user might normally browse,and100is used to show the improvements.0.10.20.30.40.50.60.70.80.9100.20.40.60.81PrecisionRecallWithout feedbackWith feedback from 20 imagesWith feedback from 50 imagesWith feedback from 100 imagesFigure1.Effect of positive feedback.The improvement in performance using RF is quite large as can be seen in Figure1.When using only feedback from thefirst20result images,the PR-graph is improved by20% in some ing50images for RF gives an additional improvement of about10%in most regions.The use of 100images improves only some parts of the graph by an additional5%.Some of the improvement comes only from relevant images being ranked higher in the top and not from returning new relevant images.4.1.2.Positive and negative feedbackNegative feedback can improve the query result greatly,but it is important to use the right images as negative feedback so as not to inhibit any important features.Many systems have problems with too much negative feedback.Based on these facts,we apply a variety of methods for automatic selection of negative RF.Positive images from the top20 returned were still all selected as positive feedback.As neg-ative feedback,we chose thefirst two and the last two non-relevant answer images.Since they influence different parts of the PR-graph we also combine the two strategies.We can see in Figure2that returning thefirst two im-ages as negative feedback improves the beginning of the PR-graph by4to5%;using the last two improves the mid-dle of the PR-graph by up to7%.The combination of both improves all parts of the graph by up to9%.This shows that different negative feedback images improve different parts of the graph significantly by removing different areas of feature space from the query.With this knowledge,a query from a user who only uses positive feedback can be improved by automatically supply-ing non-selected images as negative feedback.00.10.20.30.40.50.60.70.80.9100.20.40.60.81P r e c i s i o n RecallOnly positive feedback First two as negative feedback Last two as negative feedbackFirst two and last twoFigure 2.Different negative feedback images.4.1.3.Different feedback weightingsAs we know that different negative feedback images canimprove different parts of the PR-graph but also decrease performance when used in excess.We minimize the lattereffect by weighting the images with a factor other than,we can feed back all neutral images as negative RF.00.10.20.30.40.50.60.70.80.9100.20.40.60.81P r e c i s i o n RecallOnly positive feedback Weighting -1 for all negatives Weighting -0.1 for all negatives Weighting -0.2 for all negatives Weighting -0.3 for all negativesFigure 3.Various feedback weightings.In Figure 3,we can see that the value of yields the best curve in most areas,only in the end the curve withis better but these last parts of a PR-graph are not as impor-tant since they only give information about images which are not shown to the user.The curve is sometimes even worse than the curve with only positive feedback.Thevalue ofcreates improvements of up to 7or 8%.Using higher weightings does not bring any further improvements.A good idea might be to create negative feedback auto-matically with a low weighting when the user does not use any or enough negative feedback.4.1.4.Separately weighted feedbackProblems due to too much negative feedback in TR were ad-dressed by Rocchio in the 60s [4].Following this work,our system weights the features of positive and negative query images separately according to Equation 1,(1)where is the set of weighted features making up the query,and are the numbers of positive and negative images in the respectively,and are the (possibly weighted)features in the postive and negative images,and and de-termine the relative weightings of the positive and negativecomponents of the query.We useand .00.10.20.30.40.50.60.70.80.910.20.40.60.81P r e c i s i o n RecallOnly positive feedback Weighting -1 for all negativesAlgorithm of RocchioFigure 4.RF with modified Rocchio algorithm.This technique significantly improves the query results (up to 9%).This is better than the other methods for positive and negative feedback.Clearly,we still need to test whether the weightings of 0.65and 0.35are as good for CBIR as they proved to be for TR,but we already made the result more or less independent from the number of positive and negative feedback ing this method with a largernumber of result images (e.g.50as in 4.1.1.)improves the results even more.4.1.5.Several steps of feedbackTo measure the interactive performance of a system,we need to consider more than one step of RF since browsing is a crucial task for CBIR [2].We thus made experiments with several steps of RF.Figure 5shows the results using two steps of only pos-itive feedback.The major improvement occurs at the first feedback step (20%).For the second step,it is rather small (2to 3%).The improvement with positive and negative00.10.20.30.40.50.60.70.80.9100.20.40.60.81P r e c i s i o n RecallPositive feedback step 1Positive feedback step 2Negative Feedback step 1Negative Feedback step 2Negative Feedback step 3Negative Feedback step 4Figure 5.Several feedback steps.feedback is remarkable for the first four steps where the re-sults continuously get better.The first step already shows an improvement of about 25%and the second step an addi-tional 10%.In the third step the result improves by about 10%in the beginning and by 8%in the middle parts.The gain for the fourth is 5%in the middle and as well in the end.This improvement in the end means that images which were far away from the initial query have been moved closer.These results show the great importance of negative RF for the browsing process.The effect of positive feedback almost disappears after only one or two steps so the possi-bility to move in feature space is limited.Negative feedback offers many more options to move in feature space and find target images.Even hard queries are continuously improved at each feedback step.This flexibility to navigate in feature space is perhaps the most important aspect of a CBIRS.5.ConclusionsIn this article we show the influence of various RF strate-gies on the query result.RF always improves the re-sults.However,too much negative feedback can destroy the query.This can be avoided by using Rocchio’s technique of separately weighting positive and negative features.We showed that several steps of positive and negative feedback increasingly enhance the query results,thus allowing navi-gation within the ing a larger number of images as a source for feedback improves results,but this potential is limited by the number of images a user really ing a variety of automated RF strategies,we can eval-uate the flexibility of a CBIRS.It is important that using several steps of feedback continues to improve the results so,that feature space can be explored thoroughly.Several steps of positive and negative RF can form a basis for eval-uating the interactive performance of a CBIRS.The good performance of negative RF leads to the idea of automatically feeding back neutral images as negative ifnone are provided by the user.This can help novice users to get better results.References[1]Y .H.Kim,K.E.Lee,K.S.Choi,J.H.Yoo,P.K.Rhee,andY .C.Park.Personalized image retrieval with user’s pref-erence model.In C.-C.J.Kuo,S.-F.Chang,and S.Pan-chanathan,editors,Multimedia Storage and Archiving Sys-tems III (VV02),volume 3527of SPIE Proceedings ,pages 47–55,Boston,Massachusetts,USA,November 1998.[2]M.Markkula and E.Sormunen.Searching for photos -jour-nalists’practices in pictorial IR.In J.P.Eakins,D.J.Harper,and J.Jose,editors,The Challenge of Image Retrieval,A Workshop and Symposium on Image Retrieval ,Electronic Workshops in Computing,Newcastle upon Tyne,5–6Febru-ary 1998.The British Computer Society.[3]H.M¨u ller,D.M.Squire,W.M¨u ller,and T.Pun.Efficient ac-cess methods for content-based image retrieval with invertedfiles.In S.Panchanathan,S.-F.Chang,and C.-C.J.Kuo,ed-itors,Multimedia Storage and Archiving Systems IV (VV02),volume 3846of SPIE Proceedings ,Boston,Massachusetts,USA,September 20–221999.[4]J.J.Rocchio.Relevance feedback in information retrieval.In The SMART Retrieval System,Experiments in Automatic Document Processing ,pages 313–323.Prentice Hall,Engle-wood Cliffs,New Jersey,USA,1971.[5]Y .Rui,T.S.Huang,M.Ortega,and S.Mehrotra.Relevancefeedback:A power tool in interactive content-based image retrieval.IEEE Transactions on Circuits and Systems for Video Technology ,8(5):644–655,September 1998.(Special Issue on Segmentation,Description,and Retrieval of Video Content).[6]G.Salton.The state of retrieval system rma-tion Processing and Management ,28(4):441–450,1992.[7]G.Salton and C.Buckley.Improving retrieval performanceby relevance feedback.Journal of the American Society for Information Science ,41(4):288–287,1990.[8]J.R.Smith and S.-F.Chang.VisualSEEk:a fully automatedcontent-based image query system.In The Fourth ACM In-ternational Multimedia Conference and Exhibition ,Boston,MA,USA,November 1996.[9] D.M.Squire,W.M¨u ller,H.M¨u ller,and J.Raki.Content-based query of image databases,inspirations from text re-trieval:inverted files,frequency-based weights and rele-vance feedback.In The 11th Scandinavian Conference onImage Analysis (SCIA’99),pages 143–149,Kangerlussuaq,Greenland,June 7–111999.[10] D.M.Squire and T.Pun.A comparison of human and ma-chine assessments of image similarity for the organization of image databases.In M.Frydrych,J.Parkkinen,and A.Visa,editors,The 10th Scandinavian Conference on Image Anal-ysis (SCIA’97),pages 51–58,Lappeenranta,Finland,June 1997.[11]J.Tague-Sutcliffe.The pragmatics of information retrievalexperimentation,revisited.In K.Spark Jones and P.Willett,editors,Readings in Information Retrieval ,Multimedia In-formation and Systems,chapter 4,pages 205–216.Morgan Kaufmann,340Pine Street,San Francisco,USA,1997.。
- 1、下载文档前请自行甄别文档内容的完整性,平台不提供额外的编辑、内容补充、找答案等附加服务。
- 2、"仅部分预览"的文档,不可在线预览部分如存在完整性等问题,可反馈申请退款(可完整预览的文档不适用该条件!)。
- 3、如文档侵犯您的权益,请联系客服反馈,我们会尽快为您处理(人工客服工作时间:9:00-18:30)。
Combining Relevance Feedback and Genetic Algorithmsin an Internet Information Filtering EngineGuy Desjardins & Robert GodinUniversité du Québec à Montréal{ intellagent@ , godin.robert@uqam.ca }AbstractEver since the advent of the public network Internet, the quantity of available information is rapidly rising. One of the most important use of this public network is to find information. In such a huge and unstable information collection, today’s greatest problem is to find relevant information.This paper presents the development of IntellAgent, an Internet information filtering agent with a search engine using a hybrid evolutionary algorithm to optimize the user profile. The algorithm is a combination of the well known relevance feedback process and a genetic algorithm. This paper describes in detail the specifics of the combination and reports on its effectiveness measured using the TREC collection.The agent builds its own data collection statistics incrementally as it analyses the documents of the collection. Two construction methods are tested: one using all terms of all documents and a second one using only the the profile terms. The hybrid algorithm is tested against both methods.The results show that the hybrid algorithm performs better in recall than the relevance feedback process alone and better in precision than the genetic algorithm alone, revealing that the combination is building upon the strength of both processes. Using the incremental statistics collection built with the profile matching terms shows the same level of effectiveness but a slightly different ratio of recall/precision.KeywordsIntelligent agent; Information filtering; Genetic algorithm; Hybrid; Incremental statistics1. IntroductionThe number of computers linked by the Internet network grew from 5 million to 65 million over the past 6 years. Surveys estimated that there were over 200 million users in September 1999. The second most frequent use of the network is information retrieval (after e-mail use). Today the Internet is the biggest public collection of documents available worldwide. Because the information is changing continuously it is also the most unstable collection.Information filtering is concerned with finding information from unstable collections of documents such as the Internet. In the information filtering domain, the user query is called a profile. An agent usually builds the profile using examples provided by the user. Thus the query is not a list of words to search for but rather combinations of words extracted from various examples. Two of the major problems to solve are optimizing the significance of the profile and obtaining accurate collection statistics for calculating the term frequencies. For the later, Callan (1996) has proposed two methods: use the collection statistics of a similar domain sample or build your own incrementally. The first method can be used in a domain specific engine. The second method is more suitable for a general search engine. We have selected it for the development of a prototype information filtering agent, IntellAgent.Ribeiro proposed a classification of the various optimization techniques used for optimizing the user profile (1994). Enumerative and analytic techniques have shown limited effectiveness because the solution space is to vast. Yet the relevance feedback process has shown good results and can be classified as an iterative analytic method. Recently, guided-random techniques such as genetic algorithms and neural networks have been proposed as alternative solutions for information filtering.A number of researchers combined algorithms in search for a better solution (Yang & Korfhage, 1993; Sheth, 1994; Chen & Kim, 1994).Figure 1 : Optimization techniques (source: Ribeiro, 1994)When developing IntellAgent, we aimed to find such a value added solution by combining a relevance feedback process with a genetic algorithm. The objective was to find a combination that would yield better results than each of its composing processes alone.Section 2 reviews the background of this work. Section 3 describes the functions of the search engine. Section 4 describes in detail the combination of the relevance feedback process and the genetic algorithm. Section 5 describes the two methods for building the collection statistics incrementally. Section 6 presents the experimental results. Section 7 concludes.2. BackgroundGenetic algorithms have been used for various problems since Holland first introduced them in 1975, particularly in the 80’s. They borrow their process from the Darwin natural process of survival. Genetic changes the individuals over generations. Nature selects the most fit individuals to survive. The overall result is a better adapted community to its environment. It is a continuous process since the environment itself changes over time. The changes are made by recombining the genetic codes between two individuals.The analogy in information filtering makes use of the vector space model to represent the documents. Using this model, a document can be represented by a vector of its unique words, the terms vector (t), along with their frequencies (f) (see figure 2). A weights vector (w) could be calculated based on the frequencies of the terms. Using this model, the genetic would represent a gene as a term, an individual as a document and the community as the profile. After recombining the terms of the two parent documents, an objective function is used as the survival process to decide whether or not to keep the two generated children documents into the profile.The relevance feedback process has also been used successfully by a number of researchers (Chen et al, 1995; Sheth, 1994; Yang & Korfhage, 1993; Yuwono & Lee, 1996b). Applied to the vector space model, this process changes the weights of the terms according to the user feedback whenever the agent proposes a document. The firing vectors in the profile get their weights increased or decreased when the user judges the proposed document relevant or irrelevant. Those modified vectors get stronger or weaker to influence the next retrievals.Figure 2 : Vector space modelWhile the relevance feedback is used to adapt the profile as the agent retrieves documents, the genetic algorithm is usually used for optimizing the profile once at the beginning of the search process. In Beagle (Ferguson, 1995), once the profile is optimized using a genetic algorithm, it stays static thereafter during the active search phase. In NEWT (Sheth, 1994), a genetic algorithm is used to optimize the initial profile and the relevance feedback is used thereafter to make the profile evolve with the user feedback. In GANNET (Chen & Kim, 1994), a genetic algorithm is used at the initial phase to train a neural network which is used during the active search phase.In IntellAgent, the genetic algorithm is also used to optimize the initial profile. But it is further used to re-optimize it as it evolves with the user relevance feedback. During the active search phase, both processes modify the profile.3. Search engineThis section gives an overview of IntellAgent processes and reviews the basic components of the search engine. It describes the use of the vector space model and introduces the various computations.3.1 Process overviewFirst, the documents provided by the user are translated into vectors which form the profile. Then the first collection statistics are calculated and the genetic algorithm optimizes this initial profile. IntellAgent needs at least two distinct document examples in order to perform the initial optimization. Indeed the genetic process needs at least two parents.In the active phase, the agent retrieves a new document, translates it into the vector model and performs the similarity calculations against the profile. Whenever it is found similar enough to at least one vector of the profile, the agent proposes it to the user.The user replies with his relevance judgment and the agent modifies the weights of the firing vectors accordingly. Then the genetic algorithm re-optimizes the modified profile and the agent proceeds with the next iteration. If the proposed document is judged relevant, the agent adds its vector to the profile, which further modifies it. In that case, the collection statistics are recalculated.Figure 3 : IntellAgent functional diagram3.2 Vector space modelIn IntellAgent, each document is represented by four vectors:• the terms vector contains the terms of the document after stopword removal and stemming; • the frequencies vector contains the frequencies of the terms in the document;• the weights vector contains the normalized weights of the terms calculated by a traditional (tf x idf) formula;• the feedback vector contains the cumulative feedback factors of the terms calculated when a document is proposed by the agent.The weights and the feedback factors are kept separately in order to better control the combination of the relevance feedback process and the genetic algorithm.3.3 Weight computationIntellAgent makes use of a stopword process to eliminate the useless words. Then it truncates the remaining words to their basic stem. The frequencies of those remaining terms are calculated and the weights are computed using a well known tf x idf (term frequency x inverse document frequency) formula (Salton & Buckeley, 1991). The formula is normalized to compensate for long documents using the maximum frequencies normalization variant.w tf tf N n tf tf N n ik ik ip k ik ip k k =+æèçöø÷æèççöø÷÷æèçöø÷+æèçöø÷æèççöø÷÷æèçöø÷å0505050522..log ..log max maxwhere tf ik = the frequency of term k in document i, andidf k = log(N /n k ), where N is the total number of documents in the corpus, andn k is the number of documents that include term k .3.4 Objective functionOne similarity function often selected as the objective function when using the vector space model is the scalar product of the two vectors:()S D P w w i j ik jk k ,,=×å where D and P represent the document and the profile respectively 1.This function is used to optimize the profile as well as to fire documents. It computes the similarity between vectors in the profile or between the profile’s vectors and the document vector under analysis. For the later case, the document is fired if at least one vector of the profile is found similar enough, i.e. the similarity is higher than a predetermined threshold.3.5 Fitness functionThe fitness function is used by the genetic algorithm to select the best fit parents for the next generation. It is also used to determine which parents to replace when the profile size reaches a predetermined maximum.The fitness function is defined as the average similarity measured through time: 1 The subscript k varies on the common terms only.()()F P S Dp P Dp i k i k =å,#, where S(Dp k ,P i ) is the similarity between profile’s vector i and the k threlevant document proposed by the agent, and #Dp represents the total number of relevant documents proposed by the agent.3.6 Relevance feedbackWhenever the agent proposes a document, the user judges its relevance and replies 1 if it is relevant or -1 if it is not. The agent uses this information to modify the weights of the firing vectors in the profile. The weights are modified according to the formula:w w f w ik p ik p k d =+××αwhere the feedback power α is a predetermined parameter between 0 and 1, W p are the weights of the firing vectors of the profile, W d are the weights of the proposed document and f is the user feedback 2. The relevance feedback is a competition process where the useful terms get their strength reinforced and the useless terms get their strength reduced. The more a term is proven useful, the higher its influence will be on future retrievals, and vice versa.3.7 Genetic algorithmUnlike traditional optimization processes, genetic algorithms work from many initial solutions simultaneously to reach a near optimal solution (Goldberg, 1989). They follow a structured process for exchanging information randomly.The two main operators are crossover which exchanges genes between the parents creating two new individuals and mutation which mutates a random gene. IntellAgent uses a four sections crossover where the terms of the two parents vector in section one and three are exchanged. The sections are selected randomly. The mutation operator will make a term disappear or introduce a new term in the offspring.Figure 4 : Crossover operatorThe genetic algorithm first selects the two most fit parents according to the fitness function. Then it proceeds with the crossover operation, adds the offspring to the profile and recalculates the average similarity of the whole profile. This process goes on until one of the following events occurs:• there are no more parents available to process;• the average similarity decreases with the last generation;• we have reached the maximum number of crossovers allowed, which is a parameter expressed in percentage of the size of the profile;• we have reached the maximum size allowed for the profile, which is a parameter expressed in number of vectors.2 The subscript k varies on the common terms only. The subscript i varies on the vectors of the profile.When the last event occurs, the genetic algorithm does not stop but rather starts replacing vectors into the profile. In doing so, it will select the two weakest individuals, according to the fitness function, to be replaced by the offspring.The mutation process occurs randomly on one of the genetically generated vectors. A parameter sets the mutation rate. It is expressed in percentage of the number of genetically generated vectors. Generally, the selection of individuals follows rules whereas the selection of genes are randomized. This is why it is called a structured process for exchanging information randomly, or a guided-random process.4. A hybrid algorithmThe novelty in IntellAgent is that the relevance feedback process and the genetic algorithm influence each other continuously. Thus both algorithms affect the future retrievals after each proposed document, unlike Beagle (Ferguson, 1995), NEWT (Sheth, 1994) and GANNET (Chen & Kim, 1994). Here is how the two algorithms are combined (refer to figure 5 below). First, any new document analysis generates new terms and updates the frequencies of existing terms into the corpus statistics. This changes the idf factors thus there is a need to recalculate the weights of the profile vectors. Second, after the similarity calculations, if the document is found similar enough to at least one of the profile’s vector, the document is proposed to the user. The feedback changes the weights of the firing vectors into the profile, thus changing the dynamic again for future retrievals. Third, if the proposed document is judged relevant by the user, it is added to the profile, changing both the tf and the idf factors. Fourth, the genetic algorithm optimizes that new profile by adding new generated vectors to it, changing the idf factors again.Figure 5 : Events diagramThe frequencies of the genetic vector terms are initialized to zero and the weights are taken from the parents. These weights will never change since they have no frequency. But their feedback factors will make them evolve. That is why we need to keep the feedback factors in a separate vector. The genetically generated vectors will influence the idf factors in the corpus statistics. The weights of the non-genetic vectors will be recalculated.In summary, the relevance feedback influences the future retrievals by directly modifying the weights of the terms. The genetic algorithm influences the future retrievals in two ways: by adding new combinations of terms into the profile and by modifying the inverse document frequencies into the corpus statistics which will have an effect on the weights of the non-genetic vectors. The relevance feedback process makes the profile evolve by changing the relative importance of the terms within each vector. The genetic algorithm mainly makes the profile evolve by adding new combinations ofterms which brings in different term relations. A document could thus be fired based on a genetic vector only rather than on an original vector provided by the user.The relevance feedback process introduces a competition process at the term level within each vector. A proven useful term as judged by the user will get its weight increased. The genetic algorithm introduces a competition process at the vector level. A proven useless vector in the pass will eventually disappear from the profile. A proven useful vector will survive and multiply by passing its genetic code to its offspring.5. Incremental collection statisticsTesting with different incremental collection statistics building methods was not part of the original objectives of this experiment. It soon appeared that this issue was important to improve the performance. The genetic algorithm increases the number of calculations dramatically. Reducing the corpus size was the best alternative to cope with the GA computational cost.All “tf x idf” algorithms work with the corpus statistics. These are needed for the idf factors calculations. In traditional information retrieval, the collection of documents is static. Thus one can calculate the statistics in advance and store them for further use by a search engine. In information filtering the collection is unknown in advance. The collection statistics have to be incrementally updated as the search engine goes through the collection.IntellAgent was first programmed with an incremental update of the collection statistics using all terms of each document of the collection. Based on the work of Callan (1996), we alternatively computed the incremental update of the statistics using only the terms of collection that matched at least one term of the profile. This reduced the total number of terms to one fourth of the original size and cut the processing time by two thirds. We wanted to further test the hybrid algorithm with that alternate method to ensure a similar level of effectiveness before adopting it. The results are detailed at the end of the next section.6. Experiment resultsThe experiment was conducted using an ad hoc type of test from the TREC (Text REtrieval Conference) categorized collection of documents. We have selected a sub-collection of 7532 documents from the TREC-6 collection along with five topics to be search for: #301, #306, #319, #337 and #347. The selection was made to ensure a sufficient number of relevant documents for each topic to allow the agent for adaptation in time. The number of relevant documents in that sub-collection ranged from 24 to 129.The parameters of the algorithms were set as following:• relevance feedback power α = 0.20;• maximum crossover rate = 60 %;• maximum number of vectors in the profile = 30;• mutation rate = 1 %;• similarity threshold = 0.058.Since the similarity threshold was fixed, the recall and the precision metrics were measured simultaneously.TRECTotal/Average#301#306#319#337 #347 topicTREC relevant # 271129562924 33 Agent fired # 75866166117186 223 Agent relevant # 825191318 27%81.82Recall 30.26 3.8833.9344.8375.00Precision 10.827.5811.4511.119.68 12.11 %Table 1 : Relevance feedback resultsTotal/Average#301#306#319#337 #347 topicTRECTREC relevant # 271129562924 33 Agent fired # 393111671024590509 641 Agent relevant # 22292552221 3296.97%Recall 81.9271.3298.2175.8687.50Precision 5.657.88 5.37 3.73 4.13 4.99 %Table 2 : Genetic algorithm resultsTotal/Average#301#306#319#337 #347 topicTRECTREC relevant # 271129562924 33 Agent fired # 1814639545157217 256 Agent relevant # 21097521321 27Recall 77.4975.1992.8644.8387.5081.82%Precision 11.5815.189.548.289.68 10.55 %Table 3 : Hybrid algorithm resultsFor the relevance feedback alone, the average precision is good but the average recall is very low. The recall results are quite variable among the topics. It seems that the relevance feedback process is unstable and topic dependent.The genetic algorithm alone yielded a very good average recall but the average precision is low. The results seems stable across the topics.The hybrid algorithm yielded a better average precision than the two others and better average recall than the relevance feedback process. The average recall is still within the genetic algorithm range but a little below. The detailed results showed the same stability as with the genetic algorithm.A t-test with a confidence interval α = .1 and a degree of freedom df = 4 (5 TREC subjects - 1) showed that the hybrid algorithm has significantly better recall results than the relevance feedback process with no significant difference in their precision and the hybrid algorithm has significantly better precision results than the genetic algorithm with no significant difference in their recall.The test of the hybrid algorithm with the alternate method for building the collection statistics shows a better average recall with a lower average precision (see table 4). The overall results show the same stability among the topics.topicsWeighted TRECKind of corpus Metric average #301#306#319#337 #347Based on allcollection% Recall 77.4975.1992.8644.8387.50 81.82 terms(66010 terms) % Precision 11.5815.189.548.289.68 10.55onBasedprofile terms % Recall 90.0492.2583.9389.6679.17 100.00(14148 terms) % Precision 9.8913.119.147.077.76 7.66 Table 4 : Comparative results for incremental corpus statistics buildingIt seems a little surprising that the recall is better. This could be explained by noting that the profile’s matching terms method concentrates more the search on the useful combinations of terms, eliminating useless terms at the beginning of the process. Although the overall results showed more or less thesame level of effectiveness. One can argue that changing the level of the threshold parameter could bring back the same ratio of recall/precision.The gain in the performance obtained by using the statistics built from the profile matching terms onlywas based on a 7532 documents collection. Needless to say, the bigger the collection is, the bigger the performance gain will be and the results will tend to the same as the number of terms in the corpus tends to its upper bound.7. ConclusionIn this work, a combination of relevance feedback and genetic algorithms was studied for information filtering purposes. The hybrid algorithm developed was tested within the IntellAgent search engine using a subset of the TREC collection.The results show that the hybrid algorithm is significantly better in recall than the relevance feedback process alone and it is significantly better in precision than the genetic algorithm alone. Using an alternate method based on the profile’s terms matching only for building the incremental collection statistics cut the processing time by three. Surprisingly, it also improved the overall recall. Both the genetic algorithm and the hybrid algorithm showed more stable results than the relevance feedback process across the topics.The preliminary results of this experiment highlight potential for a hybrid algorithm combining traditional relevance feedback methods and genetic algorithms for information filtering. To further support these results, more tests with a larger collection of documents and more topics are needed. Also, conducting independent tests for each parameter, precision and recall, would give more insightinto the relative effect of these parameters on retrieval effectiveness.ReferencesAllan, J. (1996). Incremental Relevance Feedback for Information Filtering. Proceedings of the 19th annual international ACM SIGIR conference on research and development in information retrieval (pp. 270-278).Belkin, N.J. & Croft, W.B. (1992). Information Filtering and Information Retrieval: Two Sides of the Same Coin ?. Communications of the ACM (Vol.35, No.12, pp. 29-37).Callan, J. (1996). Document Filtering with Inference Network. Computer Science Department, University of Massachusetts, Proceedings of the 19th annual international ACM SIGIR conferenceon research and development in information retrieval (pp. 262-269).Chen, H. (1994). Machine Learning for Information Retrieval: Neural Networks, Symbolic Learning,and Genetic Algorithms. MIS Department, College of Business and Public Administration, University of Arizona, /papers/mlir93/mlir93.html.Chen, H. & Kim, J. (1994). GANNET: Information Retrieval Using Genetic Algorithms and Neural Nets. MIS Department, College of Business and Public Administration, Electrical and Engineering Department, College of Engineering, University of Arizona, /papers/gannet93.html.Chen, H. et al (1995). A machine Learning Approach to Inductive Query by Examples: An Experiment Using Relevance Feedback, ID3, Genetic Algorithms, and Simulated Annealing. MIS Department, College of Business and Public Administration, University of Arizona, /papers/expert94.html.Cheong, F-C. (1996). Internet Agents - Spiders, Wanderers, Brockers, and Bots. New Riders Publishing, Indianapolis, Indiana.Ferguson, S. (1995). BEAGLE: A Genetic Algorithm for Information Filter Profile Creation. University of Alabama, http://www/cis/uab/edu/info/grads/sf/papers/cs692.report.html.Genesereth, M.R. & Ketchpel, S.P. (1994). Software Agents. Communications of the ACM (Vol.37, No.7, pp. 48-53).Goldberg, D.E. (1989). Genetic Algorithms in Search, Optimization & Machine Learning. Universityof Alabama, Addison-Wesley Publishing Company, Inc., ISBN 0-201-15767-5.Jacobs, P.S., et al (1993). A Boolean Approximation Method for Query Construction and Topic Assignment in TREC. GE Research and Development Center, Second Anual Symposium on Document Analysis and Information Retrieval, IEEE (pp. 191-200).Ribeiro, J.L. et al (1994). Genetic-Algorithm Programming Environments. University College London, Politecnico di Milano, IEEE (pp. 28-43).Salton, G. & Buckley, C. (1991). Global Text Matching for Information Retrieval. Cornell University, Science (Vol. 253, 974).Salton, G. & McGill, M.J. (1983). Introduction to Modern Information Retrieval. Cornell University, Syracuse University, Computer Science Series, McGraw-Hill Company (pp. 120-122).Sheth, B. (1994). NEWT (News Tailor). MIT Media Lab, Autonomous Agent Group. /groups/agents/papers/newt-thesis/main.html.Singhal A. et al (1996). Pivoted Document Length Normalization. Department of Computer Science, Cornell University, Ithaca, NY 14853.Srinivas, M. & Patnaik, L.M. (1994). Genetic Algorithms: A Survey. Motorola Indian Electronics Ltd., Indian Institute of Science, IEEE (pp. 17-26).Yan, T.W. & Garcia-Molina, H. (1993). Index Structures for Information Filtering Under the Vector Space Model. Department of Computer Science, Stanford University, ICDE (pp. 337-347).Yang, J-J. & Korfhage, R.R. (1993). Effects of Query Term Weights Modification in Document Retrieval - A Study Based on a Genetic Algorithm. University of Pittsburgh, Second Anual Symposium on Document Analysis and Information Retrieval, IEEE (pp. 271-285).Yuwono, B. & Lee, D.L. (1996a). Search and Ranking Algorithms for Locating Resources on the World Wide Web. New Orleans, Proceedings 12 Int’l Conference Data Engineering (pp. 164-171). Yuwono, B. & Lee, D.L. (1996b). WISE: A World Wide Web Resource Database System. Ohio State University, Hong Kong University, IEEE Transactions on Knowledge and Data Engineering (Vol.8, No.4, pp. 548-554).。