Spatial-Spectral Joint Detection for Wideband Spectrum Sensing in Cognitive Radio Networks
两种类型线阵在声场环境下的探测和测向性能仿真研究
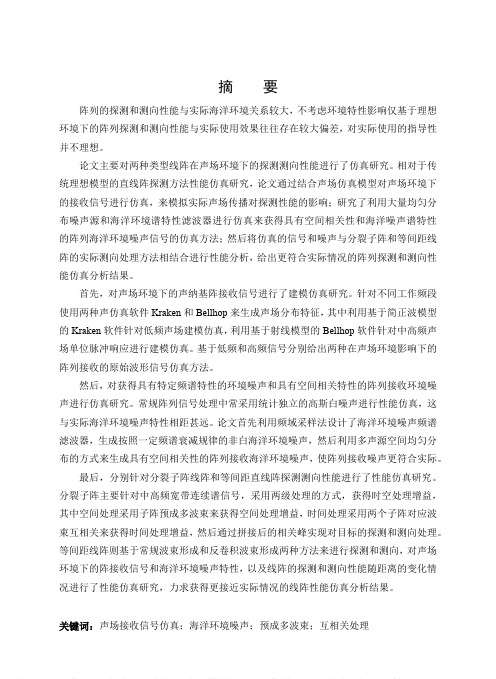
摘要阵列的探测和测向性能与实际海洋环境关系较大,不考虑环境特性影响仅基于理想环境下的阵列探测和测向性能与实际使用效果往往存在较大偏差,对实际使用的指导性并不理想。
论文主要对两种类型线阵在声场环境下的探测测向性能进行了仿真研究。
相对于传统理想模型的直线阵探测方法性能仿真研究,论文通过结合声场仿真模型对声场环境下的接收信号进行仿真,来模拟实际声场传播对探测性能的影响;研究了利用大量均匀分布噪声源和海洋环境谱特性滤波器进行仿真来获得具有空间相关性和海洋噪声谱特性的阵列海洋环境噪声信号的仿真方法;然后将仿真的信号和噪声与分裂子阵和等间距线阵的实际测向处理方法相结合进行性能分析,给出更符合实际情况的阵列探测和测向性能仿真分析结果。
首先,对声场环境下的声纳基阵接收信号进行了建模仿真研究。
针对不同工作频段使用两种声仿真软件Kraken和Bellhop来生成声场分布特征,其中利用基于简正波模型的Kraken软件针对低频声场建模仿真,利用基于射线模型的Bellhop软件针对中高频声场单位脉冲响应进行建模仿真。
基于低频和高频信号分别给出两种在声场环境影响下的阵列接收的原始波形信号仿真方法。
然后,对获得具有特定频谱特性的环境噪声和具有空间相关特性的阵列接收环境噪声进行仿真研究。
常规阵列信号处理中常采用统计独立的高斯白噪声进行性能仿真,这与实际海洋环境噪声特性相距甚远。
论文首先利用频域采样法设计了海洋环境噪声频谱滤波器,生成按照一定频谱衰减规律的非白海洋环境噪声,然后利用多声源空间均匀分布的方式来生成具有空间相关性的阵列接收海洋环境噪声,使阵列接收噪声更符合实际。
最后,分别针对分裂子阵线阵和等间距直线阵探测测向性能进行了性能仿真研究。
分裂子阵主要针对中高频宽带连续谱信号,采用两级处理的方式,获得时空处理增益,其中空间处理采用子阵预成多波束来获得空间处理增益,时间处理采用两个子阵对应波束互相关来获得时间处理增益,然后通过拼接后的相关峰实现对目标的探测和测向处理。
多波段红外目标的空谱关联检测算法
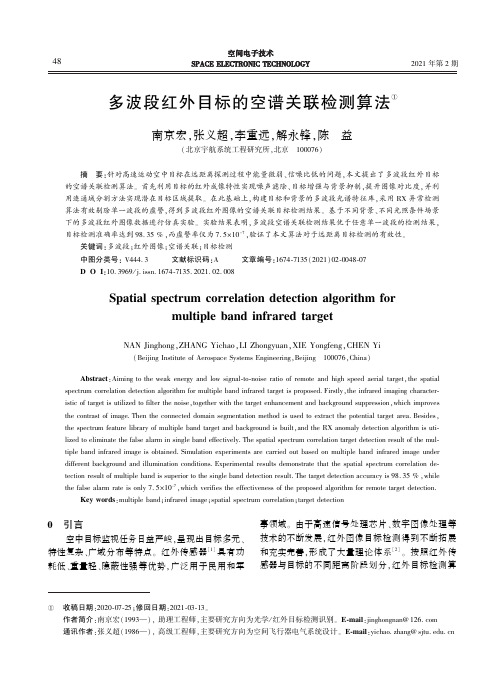
㊀㊀㊀㊀㊀㊀㊀㊀㊀㊀㊀㊀㊀㊀㊀㊀㊀㊀空间电子技术SPACE ELECTRONIC TECHNOLOGY㊀㊀㊀㊀㊀㊀㊀㊀㊀㊀㊀㊀㊀2021年第2期多波段红外目标的空谱关联检测算法①南京宏,张义超,李重远,解永锋,陈㊀益(北京宇航系统工程研究所,北京㊀100076)㊀㊀摘㊀要:针对高速运动空中目标在远距离探测过程中能量微弱㊁信噪比低的问题,本文提出了多波段红外目标的空谱关联检测算法㊂首先利用目标的红外成像特性实现噪声滤除㊁目标增强与背景抑制,提升图像对比度,并利用连通域分割方法实现潜在目标区域提取㊂在此基础上,构建目标和背景的多波段光谱特征库,采用RX异常检测算法有效剔除单一波段的虚警,得到多波段红外图像的空谱关联目标检测结果㊂基于不同背景㊁不同光照条件场景下的多波段红外图像数据进行仿真实验㊂实验结果表明,多波段空谱关联检测结果优于任意单一波段的检测结果,目标检测准确率达到98.35%,而虚警率仅为7.5ˑ10-7,验证了本文算法对于远距离目标检测的有效性㊂关键词:多波段;红外图像;空谱关联;目标检测中图分类号:V444.3㊀㊀㊀文献标识码:A㊀㊀㊀文章编号:1674-7135(2021)02-0048-07D O I:10.3969/j.issn.1674-7135.2021.02.008Spatial spectrum correlation detection algorithm formultiple band infrared targetNAN Jinghong,ZHANG Yichao,LI Zhongyuan,XIE Yongfeng,CHEN Yi(Beijing Institute of Aerospace Systems Engineering,Beijing㊀100076,China)Abstract:Aiming to the weak energy and low signal-to-noise ratio of remote and high speed aerial target,the spatial spectrum correlation detection algorithm for multiple band infrared target is proposed.Firstly,the infrared imaging character-istic of target is utilized to filter the noise,together with the target enhancement and background suppression,which improves the contrast of image.Then the connected domain segmentation method is used to extract the potential target area.Besides, the spectrum feature library of multiple band target and background is built,and the RX anomaly detection algorithm is uti-lized to eliminate the false alarm in single band effectively.The spatial spectrum correlation target detection result of the mul-tiple band infrared image is obtained.Simulation experiments are carried out based on multiple band infrared image under different background and illumination conditions.Experimental results demonstrate that the spatial spectrum correlation de-tection result of multiple band is superior to the single band detection result.The target detection accuracy is98.35%,while the false alarm rate is only7.5ˑ10-7,which verifies the effectiveness of the proposed algorithm for remote target detection.Key words:multiple band;infrared image;spatial spectrum correlation;target detection0㊀引言空中目标监视任务日益严峻,呈现出目标多元㊁特性复杂㊁广域分布等特点㊂红外传感器[1]具有功耗低㊁重量轻㊁隐蔽性强等优势,广泛用于民用和军事领域㊂由于高速信号处理芯片㊁数字图像处理等技术的不断发展,红外图像目标检测得到不断拓展和充实完善,形成了大量理论体系[2]㊂按照红外传感器与目标的不同距离阶段划分,红外目标检测算84①收稿日期:2020-07-25;修回日期:2021-03-13㊂作者简介:南京宏(1993 ),助理工程师,主要研究方向为光学/红外目标检测识别㊂E-mail:jinghongnan@ 通讯作者:张义超(1986 ),高级工程师,主要研究方向为空间飞行器电气系统设计㊂E-mail:yichao.zhang@法包括弱小目标检测算法和面目标检测算法㊂在弱小目标检测阶段,目标与红外传感器距离远,由于大气衰减㊁光学散射等因素,目标成像面积小,通常只占几个像素而不具有形状㊁纹理和结构信息,且易受背景噪声干扰,具有一定检测难度㊂国内外学者设计了众多的红外目标检测方法,传统的单帧图像检测方法包括最大均值滤波[3]㊁To-phat滤波[4,5]和小波变换[6]等,但是上述方法在目标信噪比较低时的虚警率高㊂为了同时实现低虚警率和高检测率,利用基于多显著图融合的方法[7,8]和基于稀疏表示的方法[9]实现低信噪比红外目标检测,但是上述方法由于迭代计算导致实时性差㊂近年来出现了基于人类视觉系统(HVS)的红外弱小目标检测方法[10-13],该系列方法通过定义和计算目标与周围背景的局部对比度实现背景抑制和目标增强,从而有效提取目标㊂然而,上述方法仅采用单一波段的红外图像完成目标检测,而超远距离的空中目标成像能量微弱㊁信噪比低,仍存在检测率低㊁虚警率高的问题㊂针对上述问题,本文研究多波段红外目标的空谱关联检测算法㊂1㊀基于空谱关联的多波段红外目标检测算法本文以多波段红外视频为输入信息,针对目标远距离成像能量微弱㊁信噪比低的问题,提出了空谱关联的目标检测算法,总体算法流程如图1所示,包括局部对比度提升的多尺度目标空域检测㊁多波段红外图像的潜在目标光谱鉴别两部分㊂图1㊀基于空谱关联的目标检测总体算法流程Fig.1㊀The overall flow of spatial spectrum correlation target detection algorithm 1.1㊀局部对比度提升的多尺度目标空域检测1.1.1㊀多波段红外图像预处理传统的图像预处理方法包括中值滤波和均值滤波㊂然而,针对远距离目标探测的目标信噪比低㊁点源分布特点,上述预处理方法存在噪声和目标同时滤除的问题㊂由于目标中心点对其八邻域存在一定的红外辐射影响,而噪声中心点孤立存在,所以噪声八邻域的灰度值都很小㊂利用目标邻域与噪声邻域的差异性,本文设计目标保护的高效去噪方法:d cen=18ð8i=1|I cen-I i|(1)㊀㊀其中,d cen表示中心像素灰度值与其八邻域像素灰度值差值的绝对值之和,I cen表示中心像素灰度值,I i表示邻域像素i的灰度值㊂I(x,y)=I(x,y)㊀㊀㊀㊀㊀㊀d cenɤTh dmediumfilter(I(x,y))d cen>Th d{(2)㊀㊀式(2)中,设定阈值Th d,I(x,y)为图像位置(x, y)处的灰度值,mediumfilter(㊃)表示3ˑ3中值滤波㊂在图像预处理过程中,计算每一个像素点的d cen,并与Th d进行比较,如果大于该阈值,则认为是潜在的噪声点,并采用3ˑ3中值滤波进行去噪,否则保留原始灰度值㊂1.1.2㊀多尺度块局部对比度计算在完成图像预处理后,图像存在目标及少量背景噪声㊂本文采用一种多尺度块局部对比度测量方法,进行目标增强和背景抑制,提升目标信噪比㊂针对红外目标局部对比度高于背景局部对比度的特点进行算法设计㊂由于目标面积不超过3ˑ3像素,所以给定尺度sˑs(s=2,3),则中心块区域设为T,其八邻域为B㊂图2㊀图像块八连通域结构图Fig.2㊀Connected domain structure of image block942021年第2期南京宏,等:多波段红外目标的空谱关联检测算法㊀㊀定义目标T 与背景B i 的不相似度为:D (T )=d (T ,B 1)d (T ,B 2)㊀︙d (T ,B 8)æèççççöø÷÷÷÷(3)㊀㊀其中:d (T ,B i )=m T -m B i (i =1,2, ,8)(4)㊀㊀m T 和m B i 分别为中心块区域及其背景区域的灰度均值,计算块局部对比度:d n =d (T ,B i )ˑd (T ,B i +4)(5)㊀㊀由于d n 包含四个值,选择最小值d min 作为当前中心块的局部对比度值㊂采用图像滑窗的方式计算多尺度s ˑs (s =2,3)的局部对比度㊂以多尺度最大响应值代替原始区域像素值并构建对比度响应图,即可实现红外目标增强与背景抑制㊂依据公式(6)对对比度图进行阈值分割和目标提取㊂图像局部对比度图的均值为μ,方差为σ,则定义二值化全局阈值T :T =μ+kσ(6)㊀㊀其中,k 是可调节参数㊂在完成图像阈值分割后,统计二值化图像中的连通体作为目标候选区域,并提取质心位置,得到单帧空间域潜在目标位置结果,如图3所示㊂(a)短波图像㊀㊀㊀㊀㊀㊀㊀㊀㊀㊀㊀㊀(b)中波图像㊀㊀㊀㊀㊀㊀㊀㊀㊀㊀㊀(c)长波图像㊀㊀图3㊀图像增强及潜在目标检测结果Fig.3㊀Image enhancement and potential target detection result㊀㊀由图3可知,在同一探测场景不同波段图像中,潜在目标检测结果存在差异㊂图中圆圈标记了三个5空间电子技术2021年第2期波段均检测到的潜在目标㊂所以需要实现多波段红外图像的目标光谱鉴别㊂1.2㊀多波段红外图像的潜在目标光谱鉴别1.2.1㊀潜在目标及背景光谱提取根据多波段红外图像的潜在目标检测结果,得到数据Y =[Y 1,Y 2,Y 3]T =[y 1,y 2, y N ],其中,Y k 为第k (k [1,3]个波段图像灰度值,y i 为第i (i [1,N ])个潜在目标像元的质心位置,N 为潜在目标像元总数㊂为了实现多波段红外图像的潜在目标光谱鉴别,首先需要采集潜在目标光谱和背景光谱,为分析光谱特征的差异性奠定基础,如图4所示㊂图4㊀多波段红外图像的潜在目标及背景光谱提取结果Fig.4㊀Spectrum extraction result of potential target and background in multiple band infrared image㊀㊀分析图4可知,背景光谱整体平滑且灰度值较低,而潜在目标光谱中部分波段的灰度值较高㊂然而,部分潜在目标受到噪声的影响需要剔除㊂所以利用光谱信息保留有效目标㊂1.2.2㊀多波段图像RX 异常检测为了描述目标光谱与背景光谱的差异性,采用RX 异常检测方法,设F 0表示异常不存在,F 1表示异常存在,则二元假设可表示为:F 0ʒy =O nF 1ʒy =s t +O n(7)㊀㊀式中,O n 表示背景噪声向量,s i 表示异常像元(目标)的光谱向量㊂假设待检验的光谱向量y i 来源于两个具有相同协方差矩阵C b 与不同均值的高斯分布㊂在假设F 0下,y i (背景)服从高斯分布N (0,C b );在另一假设F 1下,y i (目标)服从高斯分布N (s t ,C b ),则针对潜在目标光谱集合中的像元y i ,RX 方法的光谱检测结果可表示为:RX (y i )=(y i -yb )T C -1b (y i -yb )(8)㊀㊀式中,yb ㊁C b 分别为背景光谱的均值向量与协方差矩阵㊂设y bset =[y b 1,y b 2, ,y bN ]为背景像元集合,N 为背景像元总数,则yb ㊁C b 的计算公式为:yb =1N ðNi =1y bi(9)C b =1N ðNi =1(y bi -y b )(y bi -y b )T (10)㊀㊀设阈值为η=mean(RX ),如果RX (y i )ȡη,则像元y i 为空谱检测的目标结果㊂2㊀结果分析首先分析目标检测性能评价指标,并对算法进行定量分析和定性分析㊂2.1㊀性能评价指标本文采用平均检测准确率(TPR )和平均虚警率(FAR )两个指标进行定量分析:TPR =D nR nˑ100%(11)FAR =F pT pˑ100%(12)式中,D n 表示实际检测到的目标数量,R n 表示真实存在的目标数量,F p 表示误检测的像素总数,T p 表示图像全部像素数㊂2.2㊀算法定量分析本文提出的多波段红外目标的空谱关联检测算152021年第2期南京宏,等:多波段红外目标的空谱关联检测算法法包括空间域候选目标检测和光谱域目标精确鉴别两部分内容,实现 空间域快速提取+光谱域精确鉴别 的空谱关联检测㊂本文采用3类背景㊁2类光照条件共计6种典型场景,每个场景中包含180帧多波段图像(短波红外㊁中波红外㊁长波红外),每帧图像中包含3个目标和一定的背景噪声,分阶段验证本文算法的性能㊂2.2.1㊀单波段图像的空间域目标检测结果分析本文提出的空间域目标快速提取算法模块包含多波段红外图像预处理和多尺度块局部对比度计算两部分内容㊂基于6种典型场景的中波红外图像,将本文算法与三种通用的单帧图像目标检测算法LCM[11]㊁MPCM[12]和HB-MLCM[13]进行性能对比,结果如表1所示㊂表1㊀本文空间域检测方法与其它算法的性能对比结果Tab.1㊀Result comparison of our spatial domain detection method and other detection methods 评价指标LCM MPCM HB-MLCM本文方法平均TPR61.32%79.43%85.70%90.73%平均FAR7.12ˑ10-6 3.17ˑ10-6 2.79ˑ10-6 1.11ˑ10-6㊀㊀由表1可知,基于6种典型场景的中波红外图像,本文空间域检测方法的平均TPR为90.73%,平均FAR为1.11ˑ10-6,均优于其它三种对比的方法㊂结果表明本文采用的 图像空间域预处理+多尺度块局部对比度计算 可以有效剔除噪声,提高检测率并降低虚警率㊂2.2.2㊀多波段图像的光谱域目标检测结果分析在单波段图像空间域目标检测结果分析的基础上,对比单波段目标检测与多波段空谱关联检测的结果,如表2所示㊂表2㊀单波段目标检测与多波段空谱关联检测结果对比Tab.2㊀Result comparison of single band target detection and multiple band correlation detection 评价指标短波红外中波红外长波红外多波段红外平均TPR92.04%90.73%88.58%98.35%平均FAR 1.11ˑ10-6 1.11ˑ10-6 1.09ˑ10-67.50ˑ10-7㊀㊀由表2可知,多波段空谱关联检测的准确率和虚警率均优于单一波段㊂其中,多波段检测的平均TPR为98.35%,性能优于短波约6%㊁优于中波约8%㊁优于长波约10%;而多波段检测的虚警率比任意单一波段降低了约30%㊂所以,多波段空谱关联检测结果优于任意单一波段的空域检测结果,验证了 空谱关联检测 的有效性㊂2.3㊀算法定性分析本文定性分析算法结果,场景中包含180帧多波段图像,每帧图像包含3个目标和一定的背景噪声㊂为了方便展示,将目标检测结果用红色矩形框标记在中波输入图像㊂多波段红外目标检测结果如图5所示㊂在第135帧至第160帧图像(以5帧为间隔)中,均准确检测到目标1㊁目标2和目标3,在第155帧存在两个虚警,在第135㊁140㊁145㊁150㊁160帧不存在虚警㊂本文提出的多波段红外目标空谱关联检测算法适用于不同背景和不同光照条件,在保持较低虚警率的前提下,可以有效检测目标㊂25空间电子技术2021年第2期图5㊀多波段红外目标检测结果Fig.5㊀Multiple band infrared target detection result3㊀结论针对远距离多波段空中目标探测,本文提出了基于空谱关联的多波段红外目标检测算法,基于局部对比度提升的多尺度目标检测实现潜在目标提取,并利用多波段红外图像的潜在目标光谱鉴别实现虚警剔除㊂利用多波段(短波1μm~3μm㊁中波352021年第2期南京宏,等:多波段红外目标的空谱关联检测算法3μm~5μm㊁长波8μm~10μm)红外图像数据,在不同背景㊁不同光照条件的场景下验证了空谱关联目标检测算法性能㊂实验结果表明:多波段空谱关联检测结果优于任意单一波段的检测结果,目标检测准确率达到98.35%,而虚警率仅为7.5ˑ10-7㊂参考文献:[1]㊀孙刚.大视场红外搜索系统目标检测关键技术研究[D].长沙:国防科学技术大学,2015.[2]㊀温和,段崇棣,王伟伟,等.一种稳健的多帧联合虚假航迹剔除算法[J].空间电子技术,2019,16(2):40-47.[3]㊀DESHPANDE S D,MENG H E,VENKATESWARLU R,et al.Max-mean and Max-median Filters for Detection ofSmall Targets[C]//SPIE's International Symposium onOptical Science,Engineering,and Instrumentation,1999,3809:74-83.[4]㊀BAI X Z,ZHOU F G.Analysis of New Top-hat Transfor-mation and The Application for Infrared Dim Small TargetDetection[J].Pattern Recognition,2010,43(6):2145-2156.[5]㊀刘晓磊,董小萌,潘忠石,等.空中隐身目标天基探测技术浅析[J].空间电子技术,2020,17(3):8-12. [6]㊀LI L Q,TANG Y Y.Wavelet-hough Transform with Appli-cations in Edge and Target Detections[J].InternationalJournal of Wavelets Multiresolution and Information Pro-cessing,2006,4(3):567-587.[7]㊀NASIRI M,MOSAVI M R,MIRZAKUCHAKI S.IR SmallTarget Detection Based on Human Visual Attention UsingPulsed Discrete Cosine Transform[J].IET Image Pro-cessing,2017,11(6):397-405.[8]㊀DONG L L,WANG B,ZHAO M,et al.Robust InfraredMaritime Target Detection Based on Visual Attention andSpatiotemporal Filtering[J].IEEE Transactions on Geo-science and Remote Sensing,2017,55(5):3037-3050.[9]㊀GAO C Q,MENG D Y,YANG Y,et al.Infrared Patch-im-age Model for Small Target Detection in A Single Image[J].IEEE Transactions on Image Processing,2013,22(12):4996-5009.[10]㊀HAN J H,MA Y,ZHOU B,et al.A Robust InfraredSmall Target Detection Algorithm Based on Human Vis-ual System[J].IEEE Geoscience and Remote SensingLetters,2014,11(12):2168-2172.[11]㊀CHEN C L P,LI H,WEI Y T,et al.A Local ContrastMethod for Small Infrared Target Detection[J].IEEETransactions on Geoscience and Remote Sensing,2014,52(1):574-581.[12]㊀WEI Y T,YOU X G,LI H.Multiscale Patch-based Con-trast Measure for Small Infrared Target Detection[J].Pattern Recognition,2016,58(4):216-226. [13]㊀SHI Y F,WEI Y T,YAO H,et al.High-boost-basedMultiscale Local Contrast Measure for Infrared SmallTarget Detection[J].IEEE Geoscience and RemoteSensing Letters,2018,15(1):33-37.45空间电子技术2021年第2期。
面向高光谱图像的目标检测研究
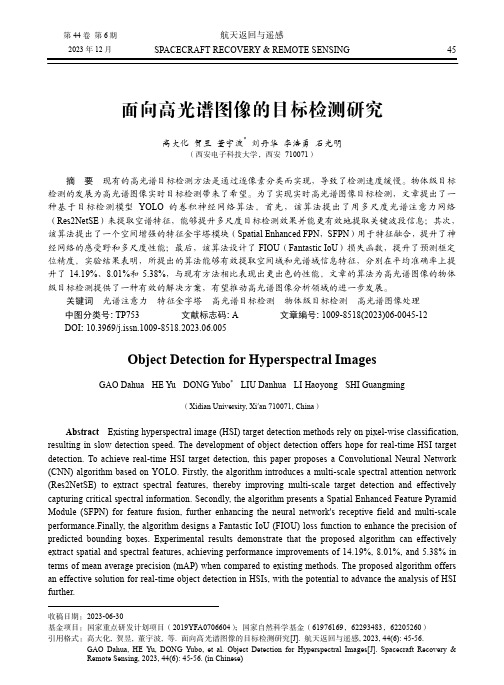
第44卷第6期航天返回与遥感2023年12月SPACECRAFT RECOVERY & REMOTE SENSING45面向高光谱图像的目标检测研究高大化贺昱董宇波*刘丹华李浩勇石光明(西安电子科技大学,西安710071)摘要现有的高光谱目标检测方法是通过逐像素分类而实现,导致了检测速度缓慢。
物体级目标检测的发展为高光谱图像实时目标检测带来了希望。
为了实现实时高光谱图像目标检测,文章提出了一种基于目标检测模型YOLO的卷积神经网络算法。
首先,该算法提出了用多尺度光谱注意力网络(Res2NetSE)来提取空谱特征,能够提升多尺度目标检测效果并能更有效地提取关键波段信息;其次,该算法提出了一个空间增强的特征金字塔模块(Spatial Enhanced FPN,SFPN)用于特征融合,提升了神经网络的感受野和多尺度性能;最后,该算法设计了FIOU(Fantastic IoU)损失函数,提升了预测框定位精度。
实验结果表明,所提出的算法能够有效提取空间域和光谱域信息特征,分别在平均准确率上提升了14.19%、8.01%和5.38%,与现有方法相比表现出更出色的性能。
文章的算法为高光谱图像的物体级目标检测提供了一种有效的解决方案,有望推动高光谱图像分析领域的进一步发展。
关键词光谱注意力特征金字塔高光谱目标检测物体级目标检测高光谱图像处理中图分类号: TP753文献标志码: A 文章编号: 1009-8518(2023)06-0045-12DOI: 10.3969/j.issn.1009-8518.2023.06.005Object Detection for Hyperspectral ImagesGAO Dahua HE Yu DONG Yubo*LIU Danhua LI Haoyong SHI Guangming(Xidian University, Xiʹan 710071, China)Abstract Existing hyperspectral image (HSI) target detection methods rely on pixel-wise classification, resulting in slow detection speed. The development of object detection offers hope for real-time HSI target detection. To achieve real-time HSI target detection, this paper proposes a Convolutional Neural Network (CNN) algorithm based on YOLO. Firstly, the algorithm introduces a multi-scale spectral attention network (Res2NetSE) to extract spectral features, thereby improving multi-scale target detection and effectively capturing critical spectral information. Secondly, the algorithm presents a Spatial Enhanced Feature Pyramid Module (SFPN) for feature fusion, further enhancing the neural network's receptive field and multi-scale performance.Finally, the algorithm designs a Fantastic IoU (FIOU) loss function to enhance the precision of predicted bounding boxes. Experimental results demonstrate that the proposed algorithm can effectively extract spatial and spectral features, achieving performance improvements of 14.19%, 8.01%, and 5.38% in terms of mean average precision (mAP) when compared to existing methods. The proposed algorithm offers an effective solution for real-time object detection in HSIs, with the potential to advance the analysis of HSI further.收稿日期:2023-06-30基金项目:国家重点研发计划项目(2019YFA0706604);国家自然科学基金(61976169,62293483,62205260)引用格式:高大化, 贺昱, 董宇波, 等. 面向高光谱图像的目标检测研究[J]. 航天返回与遥感, 2023, 44(6): 45-56.GAO Dahua, HE Yu, DONG Yubo, et al. Object Detection for Hyperspectral Images[J]. Spacecraft Recovery &Remote Sensing, 2023, 44(6): 45-56. (in Chinese)46航天返回与遥感2023年第44卷Keywords spectral attention; feature pyramid networks; HSI target detection; object detection; HSI processing0 引言高光谱成像技术是利用成像光谱仪,在光谱覆盖范围内的数十或数百条光谱波段对目标物体连续成像。
华为SecoManager安全控制器产品介绍说明书
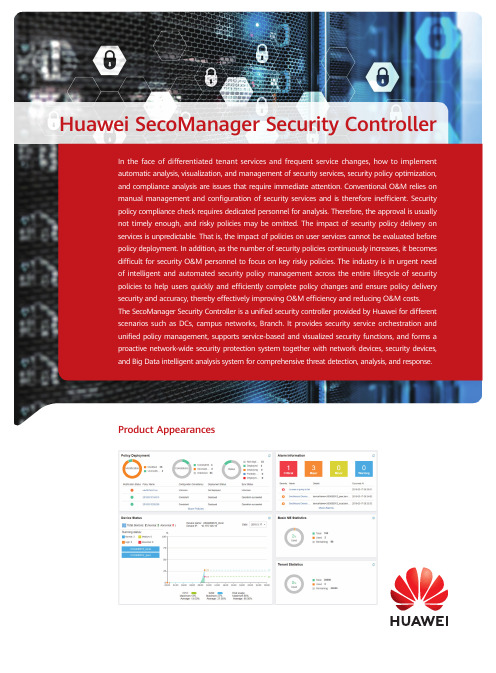
Huawei SecoManager Security ControllerIn the face of differentiated tenant services and frequent service changes, how to implementautomatic analysis, visualization, and management of security services, security policy optimization,and compliance analysis are issues that require immediate attention. Conventional O&M relies onmanual management and configuration of security services and is therefore inefficient. Securitypolicy compliance check requires dedicated personnel for analysis. Therefore, the approval is usuallynot timely enough, and risky policies may be omitted. The impact of security policy delivery onservices is unpredictable. That is, the impact of policies on user services cannot be evaluated beforepolicy deployment. In addition, as the number of security policies continuously increases, it becomesdifficult for security O&M personnel to focus on key risky policies. The industry is in urgent needof intelligent and automated security policy management across the entire lifecycle of securitypolicies to help users quickly and efficiently complete policy changes and ensure policy deliverysecurity and accuracy, thereby effectively improving O&M efficiency and reducing O&M costs.The SecoManager Security Controller is a unified security controller provided by Huawei for differentscenarios such as DCs, campus networks, Branch. It provides security service orchestration andunified policy management, supports service-based and visualized security functions, and forms aproactive network-wide security protection system together with network devices, security devices,and Big Data intelligent analysis system for comprehensive threat detection, analysis, and response.Product AppearancesProduct HighlightsMulti-dimensional and automatic policy orchestration, security service deployment within minutes• Application mutual access mapping and application-based policy management: Policymanagement transitions from the IP address-based perspective to the application mutual access relationship-based perspective. Mutual-access relationships of applications on the network are abstracted with applications at the core to visualize your application services so that you can gain full visibility into the services, effectively reducing the number of security policies. The model-based application policy model aims to reduce your configuration workload and simplify network-wide policy management.• Policy management based on service partitions: Policy management transitions from thesecurity zone-based perspective to the service partition-based perspective. Conventional network zones are divided into security zones, such as the Trust, Untrust, DMZ, and Local zones. In a scenario with a large number of security devices and a large network scale, factors of security zone, device, policy, service rollout, and service change are intertwined, making it difficult to visualize services and to effectively guide the design of security policies. However, if security policies are managed, controlled, and maintained from the perspective of service partitions, users need to pay attention only to service partitions and security services but not the mapping among security zones, devices, and services, which effectively reduces the complexity of security policy design.Service partition-based FW1untrusttrustDMZ XXX FW2untrust trustDMZ XXX FW3untrust trust DMZ XXX InternetGuest partition R&D partition Data storage partitionExternal service partition Internal service partition• Management scope of devices and policies defined by protected network segments to facilitate policy orchestration: A protected network segment is a basic model of security service orchestration and can be considered as a range of user network segments protected by a firewall.It can be configured manually or through network topology learning. The SecoManager Security Controller detects the mapping between a user service IP address and a firewall. During automatic policy orchestration, the SecoManager Security Controller automatically finds the firewall that carries a policy based on the source and destination addresses of the policy.• Automatic security service deployment: Diversified security services bring security assurance for data center operations. Technologies such as protected network segment, automatic policy orchestration, and automatic traffic diversion based on service function chains (SFCs) enable differentiated tenant security policies. Policies can be automatically tiered, split, and combined so that you can gain visibility into policies.Intelligent policy O&M to reduce O&M costs by 80%• Policy compliance check: Security policy compliance check needs to be confirmed by the security approval owner. The average number of policies to be approved per day ranges from several to hundreds. Because the tool does not support all rules, the policies need to be manually analyzed one by one, resulting in a heavy approval workload and requiring a dedicated owner to spend hours in doing so. The SecoManager Security Controller supports defining whitelists, risk rules, and hybrid rules for compliance check. After a policy is submitted to the SecoManager Security Controller, the SecoManager Security Controller checks the policy based on the defined check rules and reports the check result and security level to the security approval owner in a timely manner.In this way, low-risk policies can be automatically approved, and the security approval owner needs to pay attention only to non-compliant policy items, improving the approval efficiency and avoiding the issues that the approval is not timely and that a risky policy is omitted.• Policy simulation: Based on the learning result of service mutual access relationships, the policies to be deployed are compared, and their deployment is simulated to assess the impact of the deployment, effectively reducing the risks brought by policy deployment to services.• Redundant policy deletion: After a policy is deployed, redundancy analysis and hit analysis are performed for policies on the entire network, and the policy tuning algorithm is used, deleting redundant policies and helping you focus on policies closely relevant to services.Network collaboration and security association for closed-loop threat handling within minutes • Collaboration with network for threat handling: In a conventional data center, application deployment often takes a long time. The application service team relies on the network team to deploy the network; the network team needs to understand the requirements of the application service team to deploy a network that is suitable for the application service team. The SecoManager Security Controller learns mappings between service policies and security policies based on the network topology, and collaborates with the data center SDN management and control system (IMaster NCE-Fabric) or campus SDN management and control system to divert tenant traffic to corresponding security devices based on SFCs on demand. The SecoManager Security Controller automatically synchronizes information about the tenants, VPCs, network topology (including logical routers, logical switches, logical firewalls, and subnets), EPGs, and SFCs from the SDN management and control system and combines the learned application service mutual access relationships to automatically orchestrate and deliver security policies, implementing security-network synergy.• Collaboration with security: Advanced persistent threats (APTs) threaten national infrastructure of the finance, energy, government, and other sectors. Attackers exploit 0-day vulnerabilities, use advanced evasion techniques, combine multiple attack means such as worm and ransomware, and may remain latent for a long period of time before they actually initiate attacks. The Big Data security product HiSec Insight can effectively identify unknown threats based on network behavior analysis and correlation analysis technologies. The threat handling method, namely isolation or blocking, is determined based on the threat severity. For north-south threats, the SecoManager Security Controller delivers quintuple blocking policies to security devices. For east-west threats, isolation requests are delivered to the network SDN management and control system to control switches or routers to isolate threatened hosts.Product Deployment• Independent deployment: The SecoManager Security Controller is deployed on a server or VM as independent software.• Integrated deployment: The SecoManager Security Controller and SDN management and control system are deployed on the same physical server and same VM.Database• Collaboration with the SDN management and control system to detect network topology changes and implement tenant-based automatic security service deployment.• North-south threat blocking, east-west threat isolation, and refined SDN network security control through SFC-based traffic diversion.• Interworking with the cloud platform to automatically convert service policies to security policies. Product SpecificationsOrdering InformationNote: This product ordering list is for reference only. For product subscription, please consult Huawei representatives. GENERAL DISCLAIMERThe information in this document may contain predictive statement including, without limitation, statements regarding the future financial and operating results, future product portfolios, new technologies, etc. There are a number of factors that could cause actual results and developments to differ materially from those expressed or implied in the predictive statements. Therefore, such information is provided for reference purpose only and constitutes neither an offer nor an acceptance. Huawei may change the information at any time without notice.Copyright © 2020 HUAWEI TECHNOLOGIES CO., LTD. All Rights Reserved.。
简析两种不同数据采集方式对卫星干扰源定位性能的影响
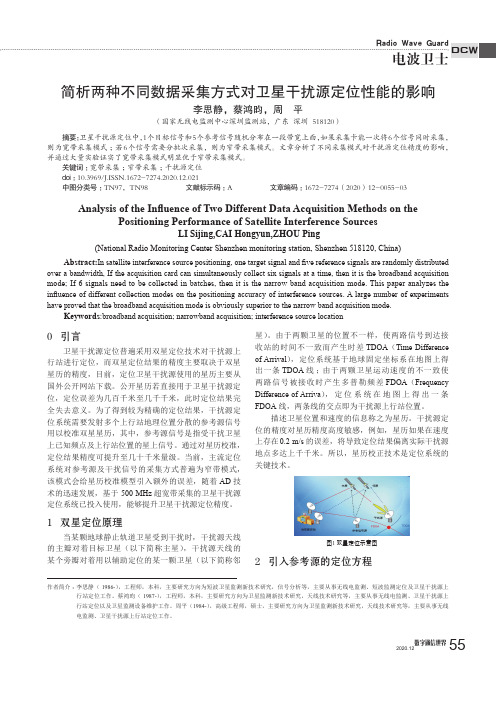
Radio Wave Guard电波卫士DCW55数字通信世界2020.120 引言卫星干扰源定位普遍采用双星定位技术对干扰源上行站进行定位,而双星定位结果的精度主要取决于双星星历的精度,目前,定位卫星干扰源使用的星历主要从国外公开网站下载。
公开星历若直接用于卫星干扰源定位,定位误差为几百千米至几千千米,此时定位结果完全失去意义。
为了得到较为精确的定位结果,干扰源定位系统需要发射多个上行站地理位置分散的参考源信号用以校准双星星历,其中,参考源信号是指受干扰卫星上已知频点及上行站位置的星上信号。
通过对星历校准,定位结果精度可提升至几十千米量级。
当前,主流定位系统对参考源及干扰信号的采集方式普遍为窄带模式,该模式会给星历校准模型引入额外的误差,随着 AD 技术的迅速发展,基于 500 MHz 超宽带采集的卫星干扰源定位系统已投入使用,能够提升卫星干扰源定位精度。
1 双星定位原理当某颗地球静止轨道卫星受到干扰时,干扰源天线的主瓣对着目标卫星(以下简称主星),干扰源天线的某个旁瓣对着用以辅助定位的某一颗卫星(以下简称邻星)。
由于两颗卫星的位置不一样,使两路信号到达接收站的时间不一致而产生时差TDOA (Time Difference of Arrival ),定位系统基于地球固定坐标系在地图上得出一条TDOA 线;由于两颗卫星运动速度的不一致使两路信号被接收时产生多普勒频差FDOA (Frequency Difference of Arriva ),定位系统在地图上得出一条FDOA 线,两条线的交点即为干扰源上行站位置。
描述卫星位置和速度的信息称之为星历。
干扰源定位的精度对星历精度高度敏感,例如,星历如果在速度上存在0.2 m/s 的误差,将导致定位结果偏离实际干扰源地点多达上千千米。
所以,星历校正技术是定位系统的关键技术。
图1 双星定位示意图2 引入参考源的定位方程简析两种不同数据采集方式对卫星干扰源定位性能的影响李思静,蔡鸿昀,周 平(国家无线电监测中心深圳监测站,广东 深圳 518120)摘要:卫星干扰源定位中,1个目标信号和5个参考信号随机分布在一段带宽上面,如果采集卡能一次将6个信号同时采集,则为宽带采集模式;若6个信号需要分批次采集,则为窄带采集模式。
GF2遥感影像预处理全流程
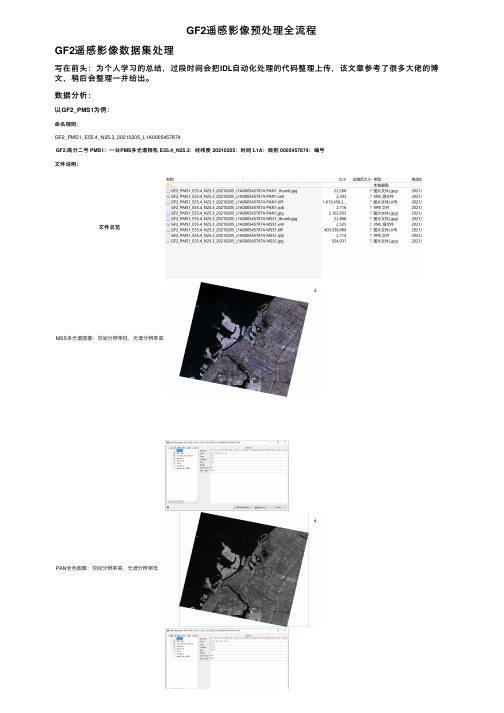
GF2遥感影像预处理全流程GF2遥感影像数据集处理写在前头:为个⼈学习的总结,过段时间会把IDL⾃动化处理的代码整理上传,该⽂章参考了很多⼤佬的博⽂,稍后会整理⼀并给出。
数据分析:以GF2_PMS1为例:命名规则:GF2_PMS1_E55.4_N25.3_20210205_L1A0005457874GF2:⾼分⼆号PMS1:⼀台PMS多光谱相机E55.4_N25.3:经纬度20210205:时间L1A:级别0005457874:编号⽂件说明:⽂件总览MSS多光谱图像:空间分辨率低,光谱分辨率⾼PAN全⾊图像:空间分辨率⾼,光谱分辨率低rpb ⽂件:⽤于图像正射校正xml ⽂件:图像对应参数⽂件总览⾼分影像处理流程:1.辐射定标(⼤⽓校正的准备⼯作):⼀般来讲,辐射定标就是将图像的数字量化值(DN )转化为辐射亮度值或者反射率或者表⾯温度等物理量的处理过程。
2.⼤⽓校正:当太阳辐射通过⼤⽓以某种⽅式⼊射到物体表⾯然后再反射回传感器,由于⼤⽓⽓溶胶、地形和邻近地物等影像,使得原始影像包含物体表⾯,⼤⽓,以及太阳的信息等信息的综合。
⽽如果我们想要了解某⼀物体表⾯的光谱属性,我们必须将它的反射信息从⼤⽓和太阳的信息中分离出来,这就需要进⾏⼤⽓校正过程。
3.正射校正(⼏何校正):先进⾏辐射定标和⼤⽓校正,然后进⾏⼏何校正。
因为⼏何校正的时候会重采样,重采样会改变像元值,从⽽影响辐射定标和⼤⽓校正的结果。
4.NOTE :辐射校正=辐射定标+⼤⽓校正全⾊图像不进⾏⼤⽓校正以GF2_PMS1为例:多光谱图像(MSS )与全⾊图像(PAN ):PAN总览图PAN部分细节图多光谱图像(MSS):辐射定标部分(Radiometric Calibration):辐射定标步骤:说明⽰例右击然后点击View Metadata,可以看到影像的元数据信息,点击Spectral,查看辐射定标系数,可与中国资源卫星中⼼下载得到的绝对辐射定标系数对⽐。
什么是回声定位-蝙蝠和人类世界(中英)
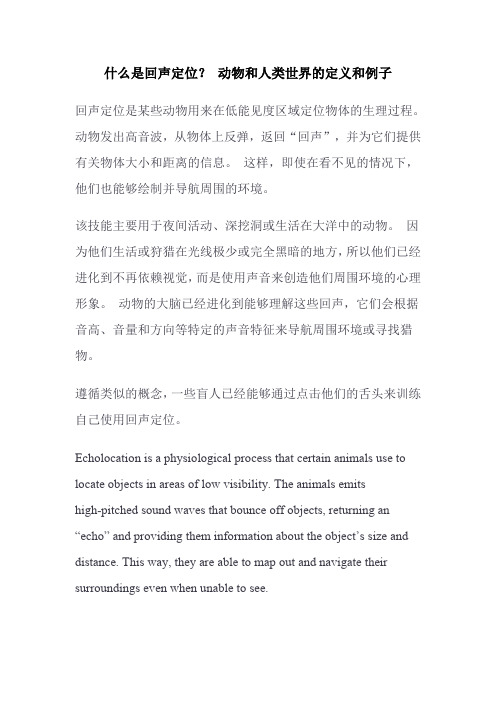
什么是回声定位?动物和人类世界的定义和例子回声定位是某些动物用来在低能见度区域定位物体的生理过程。
动物发出高音波,从物体上反弹,返回“回声”,并为它们提供有关物体大小和距离的信息。
这样,即使在看不见的情况下,他们也能够绘制并导航周围的环境。
该技能主要用于夜间活动、深挖洞或生活在大洋中的动物。
因为他们生活或狩猎在光线极少或完全黑暗的地方,所以他们已经进化到不再依赖视觉,而是使用声音来创造他们周围环境的心理形象。
动物的大脑已经进化到能够理解这些回声,它们会根据音高、音量和方向等特定的声音特征来导航周围环境或寻找猎物。
遵循类似的概念,一些盲人已经能够通过点击他们的舌头来训练自己使用回声定位。
Echolocation is a physiological process that certain animals use to locate objects in areas of low visibility. The animals emitshigh-pitched sound waves that bounce off objects, returning an “echo” and providing them information about the object’s size and distance. This way, they are able to map out and navigate their surroundings even when unable to see.The skill is mainly reserved for animals who are nocturnal, deep burrowing, or live in large oceans. Because they live or hunt in areas of minimal light or complete darkness, they have evolved to rely less on sight, using sound to create a mental image of their surroundings instead. The animals' brains, which have evolved to understand these echoes, pick up on specific sound features like pitch, volume, and direction to navigate their surroundings or find prey.Following a similar concept, some people who are blind have been able to train themselves to use echolocation by clicking their tongues.How Does Echolocation Work?To use echolocation, an animal must first create some kind of sound pulse. Typically, the sounds consist of high-pitched or ultrasonic squeaks or clicks. Then, they listen back for the echoes from the emitted sound waves bouncing off objects within their environment. Bats and other animals that use echolocation are specially tuned to the properties of these echos. If the sound comes back quickly, the animal knows the object is closer; if the sound is more intense, it knows the object is bigger. Even the echo’s pitch helps the animal map its surroundings. An object in motion towards them creates ahigher pitch, and objects moving in the opposite direction result in a lower-pitched returning echo.Studies on echolocation signals have found genetic similarities between species that use echolocation. Specifically, orcas and bats, who’ve shared specific changes in a set of 18 genes connected to cochlear ganglion development (the group of neuron cells responsible for transmitting information from the ear to the brain).1 Echolocation isn’t just reserved for nature anymore, either. Modern technologies have borrowed the concept for systems like sonar used for submarines to navigate, and ultrasound used in medicine to display images of the body.Animal EcholocationThe same way that humans can see through the reflection of light, echolocating a nimals can “see” through the reflection of sound. The throat of a bat has particular muscles that allow it to emit ultrasonic sounds, while its ears have unique folds that make them extremely sensitive to the direction of sounds. While hunting at night, bats let out a series of clicks and squeaks that are sometimes so high-pitched that they are undetectable to the human ear. When the sound reaches an object, it bounces back, creating an echo and informing the bat ofits surroundings. This helps the bat, for example, catch an insect in mid-flight.Studies on bat social communication show that bats use echolocation to respond to certain social situations and distinguish between sexes or individuals, as well. Wild male bats sometimes discriminate approaching bats based solely on their echolocation calls, producing aggressive vocalizations towards other males and courtship vocalizations after hearing female echolocation calls.2Toothed whales, like dolphins and sperm whales, use echolocation to navigate the dark, murky waters deep beneath the ocean’s surface. Echolocating dolphins and whales push ultrasonic clicks through their nasal passages, sending the sounds into the marine environment to locate and distinguish objects from near or far distances.The sperm whale’s head, one of the largest anatomical structures found in the animal kingdom, is filled with spermaceti (a waxy material) that helps sound waves bounce off the massive plate in its skull. The force focuses the sound waves into a narrow beam to allow for more accurate echolocation even over ranges of up to 60 kilometers. Beluga whales use the squishy round part of their foreheads(called a “melon”) to echolocate, focusing signals similarly to sperm whales.Human EcholocationEcholocation is most commonly associated with non-human animals like bats and dolphins, but some people have also mastered the skill. Even though they aren’t capable of hearing the high-pitched ultrasound that bats use for echolocation, some people who are blind have taught themselves to use noises and listen to the returning echoes to make better sense of their surroundings. Experiments in human echolocation have found that those who train in “human sonar” may present better performance and target detection if they make emissions with higher spectral frequencies.3 Others have discovered that human echolocation actually activates the visual brain.4Perhaps the most famous human echolocator is Daniel Kish, president of World Access for the Blind and an expert in human echolocation. Kish, who has been blind since he was 13 months old, uses mouth clicking sounds to navigate, listening to echoes as they reflect from surfaces and objects around him. He travels the world teaching other people to use sonar and has been instrumental in raising awareness for human echolocation and inspiring attention among the scientific community. In an interview with Smithsonian Magazine, Kish described his unique experience with echolocation:It’s flashes. You do get a continuous sort of vision, the way you might if you used flashes to light up a darkened scene. It comes into clarity and focus with every flash, a kind of three-dimensional fuzzy geometry. It is in 3D, it has a 3D perspective, and it is a sense of space and spatial relationships. You have a depth of structure, and you have position and dimension. You also have a pretty strong sense of density and texture, that are sort of like the color, if you will, of flash sonar.。
SPOT多光谱影像与全色波段融合
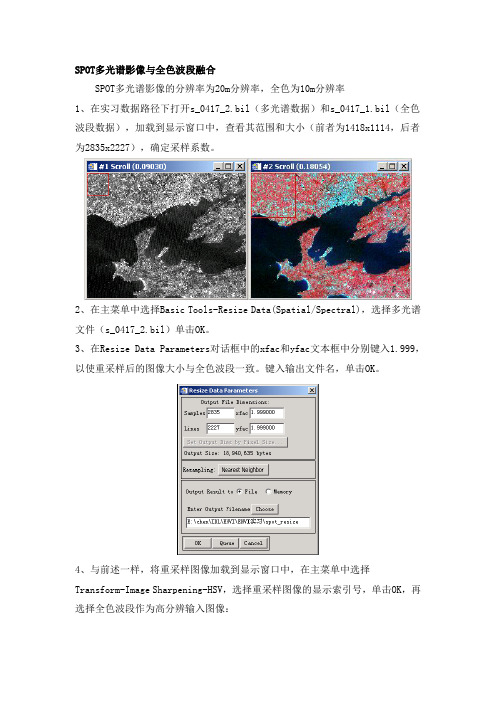
SPOT多光谱影像与全色波段融合
SPOT多光谱影像的分辨率为20m分辨率,全色为10m分辨率
1、在实习数据路径下打开s_0417_2.bil(多光谱数据)和s_0417_1.bil(全色波段数据),加载到显示窗口中,查看其范围和大小(前者为1418x1114,后者为2835x2227),确定采样系数。
2、在主菜单中选择Basic Tools-Resize Data(Spatial/Spectral),选择多光谱文件(s_0417_2.bil)单击OK。
3、在Resize Data Parameters对话框中的xfac和yfac文本框中分别键入1.999,以使重采样后的图像大小与全色波段一致。
键入输出文件名,单击OK。
4、与前述一样,将重采样图像加载到显示窗口中,在主菜单中选择
Transform-Image Sharpening-HSV,选择重采样图像的显示索引号,单击OK,再选择全色波段作为高分辨输入图像:
5、单击OK,键入输出文件名,单击OK,执行融合,查看结果,并比较其与多波段合成影像与全波段灰度影像的区别。
下图为融合图像:。
- 1、下载文档前请自行甄别文档内容的完整性,平台不提供额外的编辑、内容补充、找答案等附加服务。
- 2、"仅部分预览"的文档,不可在线预览部分如存在完整性等问题,可反馈申请退款(可完整预览的文档不适用该条件!)。
- 3、如文档侵犯您的权益,请联系客服反馈,我们会尽快为您处理(人工客服工作时间:9:00-18:30)。
‡
arXiv:0801.3049v1 [cs.IT] 19 Jan 2008
ABSTRACT Spectrum sensing is an essential functionality that enables cognitive radios to detect spectral holes and opportunistically use under-utilized frequency bands without causing harmful interference to primary networks. Since individual cognitive radios might not be able to reliably detect weak primary signals due to channel fading/shadowing, this paper proposes a cooperative wideband spectrum sensing scheme, referred to as spatial-spectral joint detection, which is based on a linear combination of the local statistics from spatially distributed multiple cognitive radios. The cooperative sensing problem is formulated into an optimization problem, for which suboptimal but efficient solutions can be obtained through mathematical transformation under practical conditions. Index Terms— Spectrum sensing, distributed detection, nonlinear optimization, and cognitive radio. 1. INTRODUCTION As an essential functionality of cognitive radio (CR) networks [1], spectrum sensing needs to reliably detect weak primary radio signals of possibly-unknown formats. Generally, spectrum sensing techniques can be classified into three categories: energy detection, matched filter coherent detection [2], and cyclostationary feature detection. Since non-coherent energy detection is simple and able to generate the spectrum-occupancy information quickly, we adopt it as the building block for constructing the proposed wideband spectrum sensing schemes. The literature on wideband spectrum sensing for CR networks is limited. An earlier approach is to use a tunable narrowband bandpass filter at the RF front-end to sense one narrow frequency band at a time, over which the existing narrowband spectrum sensing techniques can be applied. In order to search over multiple frequency bands at a time, the RF frontend needs a wideband architecture, and spectrum sensing usually operates over an estimate of the power spectral density (PSD) of the wideband signal. In [3], wavelet transformation
SPATIAL-SPECTRAL JOINT DETECTION FOR WIDEBAND SPECTRUM SENSING IN COGNITIVE RADIO NETWORKS Zhi Quan† , Shuguang Cui‡ , Ali H. Sayed† , and H. Vincent Poor§
This research was supported in part by NSF under Grants ANI-0338807, CNS-06-25637, ECS-06-01266, ECS-07-25441, CNS-06-25637, and by DoD under Grant HOTRN-07-1-0037.
Spectrum holes
Subbands occupied by primary users
M −1
Yk =
m=0
|Rk (m)|
2
k = 0, 1, . . . , K − 1
(6)
and the decision rule is given by
Department of Electrical Engineering, University of California, Los Angeles, CA 90095 Department of Electrical and Computer Engineering, Texas A&M University, College Station, TX 77843 § Department of Electrical Engineering, Princeton University, Princeton, NJ 08544 Email: {quan, sayed}@, cui@, poor@
was used to estimate the PSD over a wide frequency range given its multi-resolution features. However, no prior work attempts to make decisions over multiple frequency bands jointly, which is essential for implementing efficient CR networks. In this paper, we consider the situation in which spectrum sensing is compromised by destructive channel conditions between the target-under-detection and the detecting cognitive radios, which makes it hard to distinguish between a white spectrum and a weak signal. We propose a cooperative wideband spectrum sensing scheme that exploits the spatial diversity among cognitive radios to improve the sensing reliability. The cooperation is based on a linear combination of local statistics from spatially distributed cognitive radios [4] [5], where the signals are assigned different weights according to their positive contributions to joint sensing. In such a scenario, we treat the design of distributed wideband spectrum sensing as a spatial-spectral joint detection problem, which is further formulated into an optimization problem with the objective of maximizing the overall opportunistic throughput under constraints on the interference to primary users. Through mathematical reformulation, we derive a suboptimal but efficient solution for the optimization problem, which can considerably improve sensing performance. 2. SYSTEM MODEL Consider a primary communication system (e.g., multicarrier based) over a wideband channel that is divided into K nonoverlapping subchannels. At a particular time, some of the K subchannels might not be used by the primary users and are available for opportunistic spectrum access. Multiuser orthogonal frequency division multiplexing (OFDM) schemes are suitable candidates for such a scenario since they make it convenient to nullify or activate some portion of multiple narrow bands. We model the detection problem over the subband k as one to choose between hypothesis H0,k (“0”), which represents the absence of primary signals, and hypothesis H1,k (“1”), which represents the presence of primary signals. An illustration where only some of the K bands are occupied by primary users is illustrated in Fig. 1. The crucial task of spec-