A fuzzy semantics for semantic web languages
语用模糊

1.Peter is bald. 2.Winston is keeping a book. 3.This is a chair.
2017/9/26
Disinction between fuzziness,ambiguity, generality, vagueness
Ambiguity refers to a linguistic phenomenon whereby an expression can be potentially understood in more than one way.
2017/9/26
论引入模糊语言的研究。利用模糊集合论所建构的语义分析 模型对它们进行分析,以确定它们的语言边界的性状与样态。
伍铁平教授在他的《模糊语言学》中并没有专门为模糊性下定 义,但是根据他的论述,特别是《模糊的正名》(伍铁平 1999:114—126)一节,我们可以把伍铁平教授所言模糊性 的内核看作是边缘界限不分明。伍先生多次强调,即札德模糊 集论衍生出来的“边缘界限不明的模糊”是唯一正统的模糊 (fuzziness),他还批驳了诸多学者混淆模糊与概括、双关、 歧义、随机等现象之间区别的观点。
eg: I have a dog. She sat on the chair.
2017/9/26
Fuzzy continuum is a vague notion between truth and falsity while taxonomic category is observed as vague associations derived form hyponymy.
eg: The forehead is part of the head. The forehead is part of the face.
文本情感分析
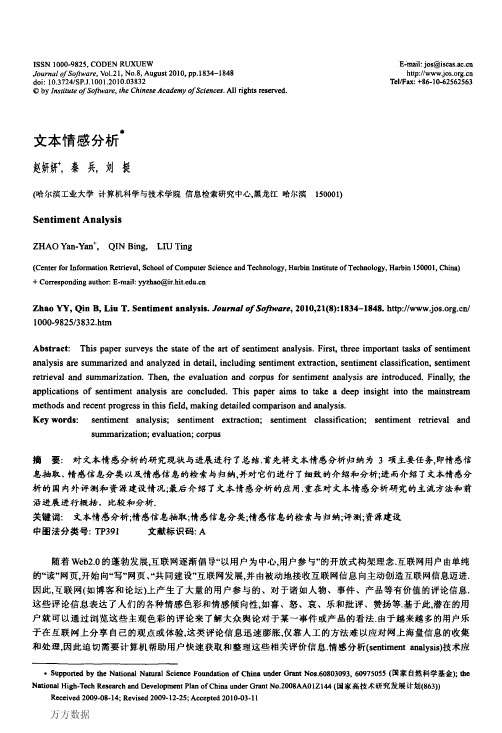
赵妍妍等:文本情感分析1835运而生(本文中提及的情感分析,都是指文本情感分析).文本情感分析又称意见挖掘,简单而言,是对带有情感色彩的丰观性文本进行分析、处理、归纳和推理的过程.最初的情感分析源自前人对带有情感色彩的词语的分析【l】,如,“美好”是带有褒义色彩的词语,而“丑陋”是带有贬义色彩的词语.随着互联网上大量的带有情感色彩的主观性文本的出现,研究者们逐渐从简单的情感词语的分析研究过渡到更为复杂的情感句研究以及情感篇章的研究.基于此,按照处理文本的粒度不同,情感分析可分为词语级、短语级、句子级、篇章级以及多篇章级等几个研究层次【2】.按照处理文本的类别不同。
可分为基于新闻评论的情感分析和基于产品评论的情感分析.其中,前者处理的文本主要是新闻评论,如情感句“他坚定地认为台湾是中国不可分割的一部分”,表明了观点持有者“他”对于事件“台湾归属问题”的立场:后者处理的主要是网络在线的产品评论文本,如“Polo的外观很时尚”。
表明了对评价对象“Polo的外观”的评价“时尚”是褒义的.由于基于产品评论的情感分析可以帮助用户了解某一产品在大众心目中的口碑,因此受到很多消费者和商业网站的青睐.而基于新闻评论的情感分析多用于舆情监控和信息预测中,是国内外评测中重要的评测任务.情感分析涉及多项非常有挑战性的研究任务.本文综合已有的研究成果,将情感分析归纳为3项层层递进的研究任务,即情感信息的抽取、情感信息的分类以及情感信息的检索与归纳,如图1所示.Fig.1Researchframeworkofsentimentanalysis图l情感分析的研究框架情感信息抽取是情感分析的最底层的任务,它旨在抽取情感评论文本中有意义的信息单元.其目的在于将无结构化的情感文本转化为计算机容易识别和处理的结构化文本,继而供情感分析上层的研究和应用服务.如将情感句“我觉得Canon的相片质量不错”转化为如图l所示的结构化文本形式.情感信息分类则利用底层情感信息抽取的结果将情感文本单元分为若干类别,供用户查看,如分为褒、贬两类或者其他更细致的情感类别(如喜、怒、哀、乐等).按照不同的分类目的,可分为主客观分析和褒贬分析;按照不同的分类粒度,可分为词语级、短语级、篇章级等多种情感分类任务.这些分类任务在情感分析初期吸引了大量的研究者.最高层的情感信息的枪索与归纳可以看作与用户直接交互的接口,着重强调检索和!f1纳两项应用.该层次的研究主要在前两项任务即情感信息抽取和分类的结果的基础上进行进一步的加工处理.情感分析是一个新兴的研究课题,具有很大的研究价值和应用价值【3-5】.鉴于此,该研究课题受到国内外越来越多的研究机构的重视.本文在接下来的部分首先分别详细阐述情感分析的3个主要研究任务,重点针对各任务的主流方法和前沿进展进行对比分析;接着介绍国内外主流的评测会议以及现有的资源建设情况:然后介绍情感分析的几个重要应用点;最后,展望情感分析技术的发展趋势.1情感信息抽取情感信息抽取旨在抽取情感文本中有价值的情感信息,它可以看作情感分析的基础任务.一直以来,学术界对它兴趣小减.纵观目前的研究现状,有价值的情感信息单元主要有评价词语(如优秀、好用)、评价对象(如GPS、1848【68】【69】【70】【7l】【72]【73】【74】【75]【76】【78]JournalofSoftware软件学报vol_2l,No.8,August2010TitovI,McDonaldR.Ajointmodeloftextandaspectratingsforsentimentsummarization.In:McKeownKed.Proc.oftheACL2008.MordstOWll:ACL.2008.308—316.BranavanS,ChenH,EisensteinJ.Learningdocument—level8em锄ticpropertiesfromfree-textannotations.In:McKeownKed.proc.oftheACL08:HLT.Morristown:ACL.2008.263-271.KuLW,LiangYT,ChenHH.Opinionextraction,summarizationandtrackinginnewsandBlogcorpora.In:GilY,MooneylU,eds.Proc.oftheAAAI2006SpringSymp.onComputationalApproachestoAnalyzingWeblogs.MenloPark:AAAIPress.2006.OunisI。
The Formal Syntax and Semantics of Web-PDDL

The Formal Syntax and Semantics of Web-PDDLDejing DouComputer and Information ScienceUniversity of OregonEugene,OR97403,USAdou@Abstract.This white paper formally defines the syntax and semanticsof Web-PDDL,a strongly typedfirst order logic ontology language usedby OntoEngine[2,1].The ability to express XML namespaces makesWeb-PDDL well compatible with other web(ontology)languages,such asRDF and OWL.The presence of types makes it possible to do meaningfuland efficient type checking during logic reasoning.The type equalityand multi inheritance make Web-PDDL more expressive for describingsemantic mapping rules between different ontologies.1IntroductionWeb-PDDL is a strongly typedfirst order language with Lisp like syntax.The ability to express namespaces with URIs and prefixes makes Web-PDDL well compatible with other web languages.The presence of types makes it possible to do meaningful type checking of Web-PDDL documents.The type equality and multi inheritance make Web-PDDL more expressive for describing the relation-ship of concepts from different ontologies in the Semantic Web model.Web-PDDL can be used to represent ontologies,datasets and queries.Here is an example,part of the yale bib ontology written in Web-PDDL:(define(domain yale_bib-ont)(:extends(uri"/2000/01/rdf-schema#":prefix rdfs)) (:types Publication-ObjArticle Book Incollection Inproceedings-PublicationLiteral-@rdfs:Literal)(:predicates(author p-Publication a-Literal).....))The:extends declaration expresses that this domain(i.e.,ontology)is extended from one or more other ontologies identified by the URIs.To avoid symbol clashes,symbols imported from other ontologies are given prefixes,such as @rdfs:Literal.These correspond to XML namespaces,and when Web-PDDL is translated to RDF,that’s exactly what they become.Types start with capital letters.A constant or variable is declared to be of a type T by writing“x-T”.There was a formal definition for the syntax and less formally,the semantics of PDDL in its manual[3].PDDL is intended to describe planning domains andproblems.It can describe sets of actions an agent can take,and what their pre-conditions and effects are.There is intense interest in development of notations for describing web services,and Web-PDDL,which is extended from PDDL,is ideal for that purpose[4].Besides web services,Web-PDDL also can describe other kinds of ontologies,datasets and queries on the Semantic Web model.This dissertation defines the formal syntax and semantics of Web-PDDL in a general way and describes its differences from PDDL.2The Syntax of Web-PDDLIn order to define the syntax of Web-PDDL,wefirst list the Symbols:–Parentheses:(,).–Negation:not.–Binary Connectives:if,iff.–n-ary Connectives:and,or.–Types(Classes):t1,t2,t3-T0...–Type inheritance(subClassOf):-.–Type Equal(sameClassAs):T->–Quantifiers:forall,exists.–Variables:x-t1,y-t2,z-t3...–n-ary predicates(properties):(P a1a2...a n)–n-ary functions:(f a1a2...a n)-t–Constants:c1-t1,c2-t2,c3-t3...–Query variables:?x-t1,?y-t2,?z-t3...–Prefix expression for namespace:@ns1:,@ns2:,@ns3:...–Comments:begin with a semicolon(“;”)and end with the next new line.We use Web-PDDL mainly to describe ontologies,dataset and queries on the Semantic Web.Before formally defining the syntax of ontology,dataset and queries in Web-PDDL,we define the notation for the syntax definition.Our notation is an BNF with following conventions.–Each rule is of the form<syntactic element>::=expansion.–Angle brackets delimit names of syntactic elements.–Square brackets surround optional material.–A plus(+)means”one or more of”.–<typed list(symbol)>means that a list of symbols with type definition.The symbol can be type,variable,query variable,constant,or function.Then we can define the syntax of an Ontology in Web-PDDL:<ontology>::=(define(domain<local name>)[<namespaces-def>][<extension-def>][<types-def>][<constants-def>][<predicates-def>][<functions-def>][<axioms-def>][<facts-def>])<namespaces-def>::=(:namespaces(:uri<URI>:prefix<prefix>)+)<extension-def>::=(:extends(:uri<URI>:prefix<prefix>)+)<types-def>::=(:types<typed list(type name)>)<constants-def>::=(:constants<typed list(constant name)>)<predicates-def>::=(:predicates<atomic formula skeleton>+)<atomic formula skeleton>::=(<predicate name><typed list(variable)>)<functions-def>::=(:functions<typed list<function skeleton>)<function skeleton>::=(<function name><typed list(variable)>)<axioms-def>::=(:axioms<axiom>+)<facts-def>::=(:facts<atomic formula>+)<axiom>::=(T-><type><type>)<axiom>::=(<quantifier>(<typed list(variable)>)<formula>)<formula>::=<atomic formula><formula>::=(not<formula>)<formula>::=(<n-ary connectives><formula>+)<formula>::=(<binary connectives><formula><formula>) <atomic formula>::=(is<type><term>)<atomic formula>::=(<predicate name><term>+)<term>::=<string><term>::=<number><term>::=<variable><term>::=<constant><term>::=<function term><function term>::=(<function name><term>+)The syntax of a Dataset in Web-PDDL is defined as:<dataset>::=(define(dataset<local name>)<ontologies-def>[<objects-def>]<facts-def>)<ontologies-def>::=(:domain(:uri<URI>:prefix<prefix>)+)<objects-def>::=(:objects<typed list(constant name)>)<facts-def>::=(:facts<atomic formula>+)<atomic formula>::=(<predicate name><term>+)<term>::=<string><term>::=<number><term>::=<constant><term>::=<function term><function term>::=(<function name><term>+)The syntax of a Query in Web-PDDL is defined as:<query>::=(define(query<local name>)<ontologies-def>[<objects-def>][<predicates-def>]<query-def>[<answer-def>])<ontologies-def>::=(:domain(:uri<URI>:prefix<prefix>)+) <objects-def>::=(:objects<typed list(constant name)>)<predicates-def>::=(:predicates<atomic formula skeleton>+) <atomic formula skeleton>::=(<predicate name><typed list(variable)>) <query-def>::=(:query(queryvars<typed list(query variable)>)<query formula>) <answer-def>::=(:output(queryvars<typed list(query variable)>)<query formula>) <query formula>::=<atomic query formula><query formula>::=(not<query formula>)<query formula>::=(<n-ary connectives><query formula>+)<atomic query formula>::=(<predicate name><term>+)<term>::=<string><term>::=<number><term>::=<query variable><term>::=<constant>Note,the syntax of Bridging Axiom(Semantic Mapping rules)in Web-PDDL is same as the syntax of general Web-PDDL axioms.However,the types and predicates in same bridging axiom may have different namespaces between they may come from different ontologies.Readers can check[2]for more detail ex-amples for bridging axioms.More bridging axioms with merged ontologies can be found in OntoMerge web site1and OntoGrate web site2.The Changes of Syntax from PDDLWeb-PDDL is mainly designed for web applications.There are some changes of syntax from PDDL:1.We add namespaces in Web-PDDL to distinguish the symbols with samename but from different ontologies or different data resources on the Web.For example,both the yale bib and cmu bib ontologies have a class(type) as Article.In Web-PDDL,we use@yale bib:Article and@cmu bib:Article to represent these two types.To represent namespaces,the keywords about URIs and prefixes are added into Web-PDDL.2.With the help of namespaces,we can represent the types from differentontologies in one Web-PDDL document.We may need to say two types are same or equal.There is no any symbol in PDDL to express this“type-equal”(sameClassAs)relationship.In Web-PDDL,we use“T->”to express it.For example,(T->@yale bib:Techreport@cmu bib:TechReport)means that the Techreport type in the yale bib ontology is same as the TechReport type in the cmu bib ontology.We can not use“=”for type-equal because a type in Web-PDDL or PDDL is not an object.3.Besides the built-in functions,such as+,-,∗,and/,we can use Web-PDDLto declare any other functions.4.In Web-PDDL,we use a simpler and moreflexible way to express axiomsthan PDDL.For example,an axiom in blocks world is expressed in PDDL: (:axiom:vars(x y-physob):context(on x y):implies(above x y))In Web-PDDL,it can be expressed like:(forall(x y-physob)(if(on x y)(above x y)))5.In order to process queries,we use symbols with question mark,such as?x,to represent query variables in Web-PDDL.There is no query processing in PDDL,both?x and x are used for variables in PDDL.3The Semantics of Web-PDDLSince Web-PDDL is afirst order logic language,in order to define its semantics, we must say what domain is involved for the quantifiers to quantify for.We must 1/ontomerge/ontoMerge.html2/ontograte/ontoGrate.jspsay how we are interpreting the constant,function and predicate with respect to that domain,an interpretation .These two items specify a model .Since the formulas may contain variables,we also need to give an assignment of values to them when we try to define the truth and satisfaction of formulas.Therefore,we formally define the semantics of Web-PDDL as following:Definition 3.1A model for the Web-PDDL is a pair M = D ,I where:D is a set of sets related to types,called the domain of M .D 0∈D s.t.D 0= D .I is a mapping,called an interpretation that associates expressions with:I assigns to primitive type τa set D τ:τI =D τ.Object I =D Object =D 0because Object is the built-in super type of all other types in a domain.To constant c ,c of type τ1,c I ∈D τ1.To n-ary function f with result type τ0and the arguments of types τ1,τ2...τn ,f I ⊆<D τ1,D τ2...D τn ,D τ0>.Here,<D τ1,D τ2...D τn ,D τ0>is a set of n+1-tuples from the domain.To n-ary predicate P ,the arguments of types τ1,τ2...τn ,P I ⊆<D τ1,D τ2...D τn >.Definition 3.2An assignment in a model M = D ,I is a mapping A from the set of variables to the sets of D .For a variable v of type τ1,v A ∈D τ1.Definition 3.3Let M = D ,I be a model for Web-PDDL,and let A be an assignment in this model.To each term t (a constant,variable or function)of Web-PDDL,we associate a value t I ,A in the sets of D as follows:1.For a constant symbol c ,c I ,A =c I .2.For a variable symbol v ,v I ,A =v A .3.For a function symbol f ,(f t 1t 2...t n )I ,A =v,where v is the unique object s.t.<t 1I ,A t 2I ,A ...t n I ,A ,v >∈f IDefinition 3.4Let x be a variable of type τ.The assignment B in the model M is an x-variant of the assignment A ,provided A and B assign the same values to every variable except possibly x .Definition 3.5Let M = D ,I be a model for Web-PDDL,and let A be an assignment in this model.To each formula Φof Web-PDDL,we associate a truth value ΦI ,A (t or f )as follows:1.For an atomic formula P :(P a 1a 2...a 3)I ,A =t ⇔<a 1I ,A a 2I ,A ...a n I ,A >∈P I .2.For the negation of a formula Φ,(not Φ)I ,A =(not ΦI ,A ).3.For the formulas connected by the connective conn :(conn Φ1Φ2...Φn )I ,A =(conn Φ1I ,A Φ2I ,A ...Φn I ,A ).4.(forall (x -t )Φ)I ,A =t ⇔ΦI ,B =t for every assignment B in M that is an x-variant of A .5.(exists (x -t )Φ)I ,A =t ⇔ΦI ,B =t for some assignment B in M that is an x-variant of A .Definition 3.6A formula Φof Web-PDDL is true in the model M = D ,I if ΦI ,A =t for all assignments A .A formula Φis valid if Φis true in all models.A set S of formulas is satisfiable in the model M = D ,I ,provided there is some assignment A such that ΦI ,A =t for all Φ∈S .S is satisfiable if it is satisfiable in some model.The Changes of Semantics from PDDLThe main changes of semantics from PDDL to Web-PDDL are related to its type system:1.In a model of PDDL,if we still define it as a pair M = D ,I where domain D is a set of sets related to types.D 0∈D s.t.D 0= D .The interpretation I assigns to primitive type τa set D τ:τI =D τ.In PDDL,any two types either are disjoint or have subsumption relationship:if τ1=τ2,then D τ1 D τ2=øor D τ1⊆D τ2or D τ2⊆D τ1.In Web-PDDL,there is no such constraint because we have to describe rela-tionships of types from different ontologies on Web.Two types can be equal,can be disjoint or can have subsumption relationship when we define them in ontologies:“(T->τ1τ2)”means D τ1=D τ2.“τ1-τ2”means D τ1⊆D τ2.“τ1τ2-τ0”means D τ1 D τ2=øand D τ1⊆D τ0and D τ2⊆D τ0.Otherwise,the relationship of two types is implicit.2.In PDDL,one type only directly inherit from one super type.It is called single inheritance .In Web-PDDL,one type can directly inherit from multiple super types.It is called multi inheritance :“τ1-τ2”and “τ1-τ3”mean that D τ1⊆D τ2 D τ3.References1.OntoEngine Source Code./projects/ontoengine/.2. D.Dou,D.V.McDermott,and P.Qi.Ontology Translation on the Semantic Web.Journal of Data Semantics ,2:35–57,2005.3. D.V.McDermott.The Planning Domain Definition Language Manual.Technical Report 98-003,Department of Computer Science,Yale University,1998.4. D.V.McDermott and D.Dou.Representing Disjunction and Quantifiers in RDF.In International Semantic Web Conference ,pages 250–263,2002.。
一种神经机器翻译中稀有词模糊语义表示方法
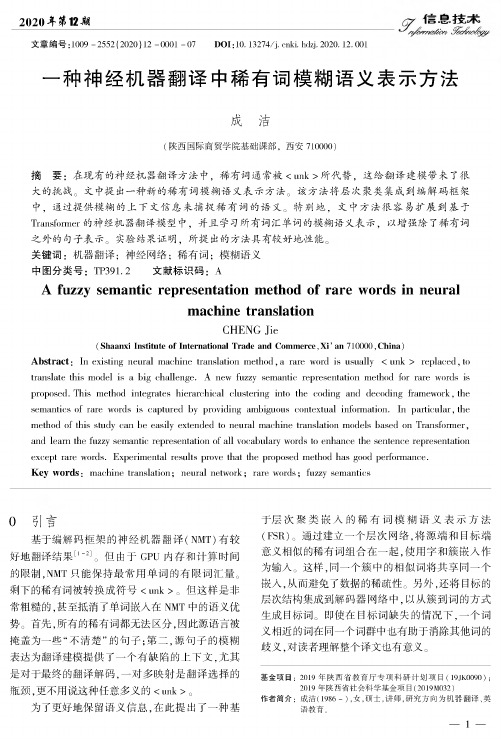
2020耳第12剧文章编号:1009-2552(2020)12-0001-07DOI:10.13274/ki.hdzj.2020.12.001一种神经机器翻译中稀有词模糊语义表示方法成洁(陕西国际商贸学院基础课部,西安710000)摘要:在现有的神经机器翻译方法中,稀有词通常被<unk>所代替,这给翻译建模带来了很大的挑战。
文中提出一种新的稀有词模糊语义表示方法。
该方法将层次聚类集成到编解码框架中,通过提供模糊的上下文信息来捕捉稀有词的语义。
特别地,文中方法很容易扩展到基于Transformer的神经机器翻译模型中,并且学习所有词汇单词的模糊语义表示,以增强除了稀有词之外的句子表示。
实验结果证明,所提出的方法具有较好地性能。
关键词:机器翻译;神经网络;稀有词;模糊语义中图分类号:TP391.2文献标识码:AA fuzzy semantic representation method of rare words in neuralmachine translationCHENG Jie(Shaanxi Institute of International Trade and Commerce,Xi'an710000,China) Abstract:In existing neural machine translation method,a rare word is usually<unk>replaced,to translate this model is a big challenge.A new fuzzy semantic representation method for rare words is proposed.This method integrates hierarchical clustering into the coding and decoding framework,the semantics of rare words is captured by providing ambiguous contextual information.In particular,the method of this study can be easily extended to neural machine translation models based on Transformer, and learn the fuzzy semantic representation of all vocabulary words to enhance the sentence representation except rare words.Experimental results prove that the proposed method has good performance.Key words:machine translation;neural network;rare words;fuzzy semantics0引言基于编解码框架的神经机器翻译(NMT)有较好地翻译结果[l-2]o但由于GPU内存和计算时间的限制,NMT只能保持最常用单词的有限词汇量o 剩下的稀有词被转换成符号<unk>。
Low-level interpretability and high-level interpretability a unified view of data-driven
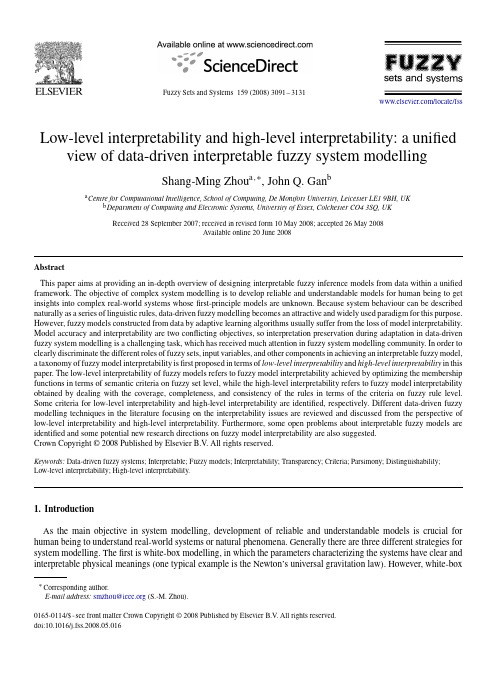
Fuzzy Sets and Systems159(2008)3091–3131/locate/fss Low-level interpretability and high-level interpretability:a unified view of data-driven interpretable fuzzy system modellingShang-Ming Zhou a,∗,John Q.Gan ba Centre for Computational Intelligence,School of Computing,De Montfort University,Leicester LE19BH,UKb Department of Computing and Electronic Systems,University of Essex,Colchester CO43SQ,UKReceived28September2007;received in revised form10May2008;accepted26May2008Available online20June2008AbstractThis paper aims at providing an in-depth overview of designing interpretable fuzzy inference models from data within a unified framework.The objective of complex system modelling is to develop reliable and understandable models for human being to get insights into complex real-world systems whosefirst-principle models are unknown.Because system behaviour can be described naturally as a series of linguistic rules,data-driven fuzzy modelling becomes an attractive and widely used paradigm for this purpose. However,fuzzy models constructed from data by adaptive learning algorithms usually suffer from the loss of model interpretability. Model accuracy and interpretability are two conflicting objectives,so interpretation preservation during adaptation in data-driven fuzzy system modelling is a challenging task,which has received much attention in fuzzy system modelling community.In order to clearly discriminate the different roles of fuzzy sets,input variables,and other components in achieving an interpretable fuzzy model, a taxonomy of fuzzy model interpretability isfirst proposed in terms of low-level interpretability and high-level interpretability in this paper.The low-level interpretability of fuzzy models refers to fuzzy model interpretability achieved by optimizing the membership functions in terms of semantic criteria on fuzzy set level,while the high-level interpretability refers to fuzzy model interpretability obtained by dealing with the coverage,completeness,and consistency of the rules in terms of the criteria on fuzzy rule level. Some criteria for low-level interpretability and high-level interpretability are identified,respectively.Different data-driven fuzzy modelling techniques in the literature focusing on the interpretability issues are reviewed and discussed from the perspective of low-level interpretability and high-level interpretability.Furthermore,some open problems about interpretable fuzzy models are identified and some potential new research directions on fuzzy model interpretability are also suggested.Crown Copyright©2008Published by Elsevier B.V.All rights reserved.Keywords:Data-driven fuzzy systems;Interpretable;Fuzzy models;Interpretability;Transparency;Criteria;Parsimony;Distinguishability;Low-level interpretability;High-level interpretability.1.IntroductionAs the main objective in system modelling,development of reliable and understandable models is crucial for human being to understand real-world systems or natural phenomena.Generally there are three different strategies for system modelling.Thefirst is white-box modelling,in which the parameters characterizing the systems have clear and interpretable physical meanings(one typical example is the Newton’s universal gravitation law).However,white-box∗Corresponding author.E-mail address:smzhou@(S.-M.Zhou).0165-0114/$-see front matter Crown Copyright©2008Published by Elsevier B.V.All rights reserved.doi:10.1016/j.fss.2008.05.0163092S.-M.Zhou,J.Q.Gan/Fuzzy Sets and Systems159(2008)3091–3131modelling usually becomes impractical when complex systems are considered.The second strategy is black-box mod-elling without using prior knowledge,in which relationships between inputs and outputs are established fully based on observational data.Black-box modelling can simulate a real-world system reliably and precisely,but the model structure and parameters usually give no explicit explanation about the system behaviours.For example,in medical domains, although black-box models,such as bagged decision trees and neural networks,can achieve goodfit performance,the resulting decisions received much suspicion[1,186].The third strategy,grey-box modelling[41,96],can be referred to as an eclecticism between precision and interpretability,in which the prior knowledge about the system is considered and unknown parts of the system are identified by black-box modelling approaches.As one of the most successful tools to develop grey-box models[112],fuzzy modelling describes systems by establishing relationships between input and output variables in terms of a fuzzy logic-based descriptive language[114–116,191,193].Compared to black-box modelling,fuzzy modelling formulates the system knowledge with rules in a transparent way to interpretation and analysis(to a certain degree)so as to gain insights into the system being modelled.This has opened up a brand-new approach to modelling complex systems in system identification and control,fault diagnosis, classification and intelligent data analysis,etc.Fuzzy rules can be generated based on human expert knowledge or heuristics,which offers a good high-level semantic generalization capability.However,for complex systems,the interactions between system behaviours are very difficult to be grasped so that the system model just based on expert knowledge may suffer from a loss of accuracy[68]and combinatorial rule explosion.On the other hand,due to the advanced development of modern information technology,the ever-increasing quantity of data is available from complex industrial and commercial processes(systems).In the past decade or so,data-driven fuzzy rule generation has been widely investigated and shown to be very pared to heuristic fuzzy rules,fuzzy rules generated from data are able to extract more specific knowledge for more complex systems.It is usually assumed that the interpretability of fuzzy models is automatically given just due to using linguistic rules. However,it is not true when adaptive learning techniques are used to optimize the fuzzy inference processes for complex systems.In accuracy-oriented adaptive learning processes,interpretation preservation during adaptation cannot always be guaranteed due to the conflicting objectives of accuracy and interpretation[8,24,28,67,68,86,121,132,160,180],so model interpretability is usually lost,which has been referred to as an incentive for Mamdani,the founder of fuzzy control,to recommend against the use of adaptation of fuzzy inference parameters[115].Particularly,because one of the important incentives of introducing fuzzy sets for modelling complex systems is that they can formulate the knowledge extracted from data or supplied by experts with a more transparent way to gain insights into the complex systems,it is often desirable and sometimes even essential to be able to interpret thefinal model structure.Rule base legibility is an important condition to take full advantage of fuzzy models.Hence,model interpretability improvement is regarded as one of the most important issues in data-driven fuzzy modelling[5,25,46,83,91,94,93,113,156,174,179,190]. However,global model accuracy and interpretability are two conflicting modelling objectives,as improving inter-pretability of fuzzy models generally degrades the global model performance of fuzzy models,and vice versa.Hence one challenging problem is how to construct a fuzzy model with not only good global performance but also good model interpretability,particularly in coping with high-dimensional input space,that is to say,how to achieve a fuzzy model with good performance trade-off between global model accuracy and model interpretability.An interpretable fuzzy model is expected to provide high numeric precision while incurring as little a loss of linguistic descriptive power as possible[5,45,83,139,190,198].It is noteworthy that as we address the interpretability issues in fuzzy modelling,transparency and interpretability issues in traditional statistical system modelling have already received much attention from the aspect of complexity reduction[33,55,73,75,97,164,177,178].The objective of complexity reduction-based statistical system modelling is to provide a trade-off between how well the modelfits the training data and model complexity.Model complexity depends on the number of independent and adjustable parameters,also called degrees of freedom,to be adapted during the learning process.Traditionally,the well-known complexity reduction techniques for achieving transparent and inter-pretable system models include input feature selection[196],orthogonal least square(OLS)[30],basis pursuit[29,31], sparse regression[170],support vector machines(SVM)[175,176],etc.More importantly,these complexity reduc-tion techniques possess strong potentials of improving fuzzy model interpretability when applied to fuzzy modelling.A natural question arises:what is the difference between the interpretability achieved by considering fuzzy systems’own idiosyncrasies and the one obtained by introducing the traditional complexity reduction techniques into fuzzy modelling?Guillaume has provided a good overview of interpretability-oriented fuzzy inference systems designed from data[67].However,the above question was not considered and addressed in[67].The purpose of this paper isS.-M.Zhou,J.Q.Gan/Fuzzy Sets and Systems159(2008)3091–31313093 not only to provide an overview of the state-of-the-art of data-driven interpretable fuzzy modelling techniques,but also to offer an overall view of this domain in a framework by syncretizing the traditional parsimonious statistical system modelling with interpretable fuzzy system modelling,so that one may form a systematic picture of thefield,instead of focusing on specific parts,dealing with particular methods and techniques.To the best of our knowledge,this type of effort has not been reported yet in literature.As a matter of fact,in the traditional statistical modelling techniques,only the influences of input variables on system outputs are taken into account to construct transparent and interpretable system models.While in fuzzy system modelling,due to its own idiosyncrasies,additional influences on system outputs should also be taken into account to achieve a transparent and interpretable fuzzy model,such as the number of membership functions(MFs),coverage and distinguishability of MFs,normality of MFs.In order to clearly discriminate the different roles of fuzzy sets,input variables and other components in achieving a transparent fuzzy model,a framework is suggested in this paper to categorize fuzzy model interpretability into low-level interpretability and high-level interpretability,and the criteria are defined for low-level interpretability and high-level interpretability,respectively.Low-level interpretability of fuzzy models is achieved on fuzzy set level by optimizing MFs in terms of the semantic criteria on MFs,whilst high-level interpretability is obtained on fuzzy rule level by conducting overall complexity reduction in terms of some criteria, such as a moderate number of variables and rules,completeness and consistency of rules.The complexity reduction techniques used in traditional system modelling,if exploited in fuzzy system modelling,can serve as fuzzy rule optimization in nature,which corresponds to aiming at the parsimony of the fuzzy rule base,one of the main high-level interpretability criteria of fuzzy systems.This clarification is helpful,as there are plentiful traditional system modelling methods on complexity reduction with great potentials of being used to induce compact rule base in fuzzy system modelling.This paper is organized as follows.Section2briefly presents the currently used fuzzy rule structures.Various definitions of fuzzy model interpretability are given in Section3,followed by criteria for fuzzy model interpretability in Section4.Constructive techniques for fuzzy model interpretability are analysed and reviewed in Section5.Section 6addresses some open problems and potential new research topics on fuzzy model interpretability,followed by conclusions in Section7.2.Rule structures of fuzzy rule-based systemsBasically,in fuzzy modelling the relationships between input and output variables are established in terms of a descriptive language based on if–then rules:If antecedent proposition then consequent proposition(1) Depending on the consequent part there exist different rule structures with different capabilities of description and approximation for rule-based fuzzy modelling.Linguistic fuzzy model,also known as Mamdani type fuzzy model[114–116,193],is represented by linguistic rules with the following structure:Rule i:If x1is A i,1and···x n is A i,n then y is B i(i=1,...,L)(2) where Rule i denotes the i th rule,L is the number of rules in the rule base,x=(x1,...,x n)T and y are the input and output linguistic variables,respectively,A i,j and B i are the linguistic labels expressed as fuzzy sets with specific semantic meanings regarding the behaviours of the system being modelled.These fuzzy sets are characterized by MFs generated by expert knowledge or fully from data.An outstanding advantage of the linguistic fuzzy model lies in that it may offer a high semantic level with good interpretability and a good generalization capability.In order to enhance the representation power of a fuzzy system,Takagi and Sugeno proposed a fuzzy model(TS fuzzy model)that differs from the linguistic one by using the following different consequent structure[168]: Rule i:If x1is A i,1and···x n is A i,n theny i=a0i+a1i x1+···+a ni x n(i=1,...,L)(3) where x i are input variables and y i are local output variables that determines local linear input–output relations by means of the real-valued coefficients a ji.The output of a fuzzy system with a knowledge base composed of L TS rules3094S.-M.Zhou,J.Q.Gan/Fuzzy Sets and Systems159(2008)3091–3131 is computed as the weighted average of the individual rule outputs y i,i=1,...,L:y=Li=1r i(x)y iLi=1r i(x)(4)with r i(x)=T j i,j(x j)being the matching degree between the antecedent part of the i th rule and the current system inputs x1,...,x n,where T is a t-norm and i,j(·)the MF of fuzzy set A i,j.Compared to a linguistic model,the rule consequent part in the TS model is an affine linear function of input variables. As such,each rule can be considered as a local linear model that is fused together by means of aggregation to produce an overall output y.A special case of the affine function with offset a0i=0(i=1,...,L)results in a homogeneous TS system.Another interesting special case is that the consequent is a constant,i.e.,y i=a0i,which is called0-order TS system.Actually the0-order TS model retains certain linguistic interpretability in the manner of a linguistic model while possessing the attractive properties of the TS system,particularly the automatic determination of model parameters from data[157].Some researchers have made efforts to modify the form of the consequent polynomials of TS models, which allows specific interpretation to the systems being modelled[7,18,54].However,either the Mamdani fuzzy rule in the form of(2)or the TS rule in the form of(3)shares the same fuzzy sets of each input variable with other rules,i.e.,the Mamdani rule(2)and the TS rule(3)are based on the global grid, which offers good linguistic readability[67].The disadvantage is that the fuzzy systems built based on(2)or(3)suffer from the curse of dimensionality in high-dimensional input space:the problem will increase exponentially in volume associated with adding extra dimensions to the input space.The curse of dimensionality is a significant obstacle to solving machine learning problems that involve learning a“state-of-nature’’from afinite number of data samples in a high-dimensional space.One efficient way of evading this problem for fuzzy systems is to generate the input space via scatter-partition(prototype)rather than grid-partition.The Mamdani and TS fuzzy rules generated by scatter-partition have the following forms respectively:Rule i:If x is A i then y is B i(5) andRule i:If x is A i then y i=a0i+a1i x1+···+a ni x n(6) where x=t(x1,x2,...,x n)∈ n is an n-dimensional input vector and A i∈F( n)are the fuzzy sets defined on n with multi-dimensional MFs.Very interestingly,the fuzzy systems consisting of multi-dimensional Mamdani rules(5) are totally equivalent to fuzzy graphs introduced by Zadeh[192,195].A fuzzy graph is build by a collection of fuzzy points corresponding to fuzzy rules,which represents a functional dependency f∗of Y on X:f∗=A1×B1+···+A n×B n(7) Some researchers have made efforts to construct fuzzy graphs from data[6,13].More details about how to generate the prototype-based fuzzy systems and their pros and cons will be reviewed in Section5.2.4)of this paper. Interestingly,different from the above rule structures Gegov proposed to use Boolean matrices and binary relations to express fuzzy models[62].For example,given the following fuzzy rule base:Rule1:If x1is Small and x2is Small then y is NegativeRule2:If x1is Small and x2is Big then y is PositiveRule3:If x1is Big and x2is Small then y is NegativeRule4:If x1is Big and x2is Big then y is ZeroIf Small=1,Big=2,Negative=1,Zero=2,Positive=3,then a Boolean relation and a binary relation that equivalently express the above fuzzy system individually are indicated in Table1and(8).{[and(11,1)]or[and(12,3)]or[and(21,1)]or[and(22,2)]}(8) Moreover,for the sake of inducing compact rule base a formal approach to manipulation of rule bases is suggested by performing operations on Boolean matrices and binary relations[62].Because Boolean transformations of rule baseS.-M.Zhou,J.Q.Gan/Fuzzy Sets and Systems159(2008)3091–31313095 Table1Boolean relation for a fuzzy systemInputs Outputs123 11100 12001 21100 22010can lead to more compact representations,Klose and Nurnberger presented an approach to building more expressive rules by performing Boolean transforming during and after learning from data[103].3.Definitions of fuzzy model interpretabilityAlthough interpretability issues in fuzzy system modelling and statistical system modelling have received much attention in recent years,there is no well-established definition about model interpretability.In this section,we review the existing efforts made by the researchers on addressing the connotations of model interpretability and propose a new framework for fuzzy model interpretability.3.1.Transparency and interpretabilityModel transparency is defined as a property that enables us to understand and analyse the influence of each system parameter on the system output[22,74,156].In connection with transparency in fuzzy modelling,another symbiotic term is interpretability mentioned by Jang et al.[86],in which some basic ideas were discussed about how to constrain the optimization of the adaptive-network-based fuzzy inference system(ANFIS)[87]to preserve the interpretability. In Riid’s opinion[142],transparency and interpretability do not match when used to characterize fuzzy systems. Interpretability is a property of fuzzy systems which exists by default,being established with linguistic rules and fuzzy sets associated with these rules.Transparency,on the other hand,is not a default property of fuzzy systems,but a measure of how valid or how reliable the linguistic interpretation of the system is.However,the meanings of these two concepts possess so much synonymity in describing system modelling.Particularly,in fuzzy modelling,it may not be reasonable to distinguish them,because the two terms had been used in parallel in traditional statistical system modelling[30,33,55,73,164,170],long before they were used in fuzzy system modelling[3,11,19,20,26].Transparency and interpretability share the same connotations according to the two definitions in practice.In this paper,the two terms are used in parallel with the same meaning in fuzzy modelling,unless otherwise stated.3.2.Formalized definitionsSome researchers have tried to give formalized definitions for interpretability or transparency of fuzzy models.Definition1(Bodenhofer and Bauer[19,20]).Consider a linguistic variable V=(N,T,X,G,S)and an index set I, where N is the name of the linguistic variable V,G is a grammar,T is the so-called term set,i.e.,the set of linguistic expressions resulting from G,X is the universe of discourse,and S is a T→F(X)mapping which defines the semantics, i.e.,a fuzzy set on X,of each linguistic expression in T.Let RE=(RE i)i∈I be a family of relations on the set of verbal values T,where each relation RE i has afinite entry a i.Assume that,for every relation RE i,there exists a relation Q i on the fuzzy power set F(X)with the same entry.1Let Q represent the family(Q i)i∈I.Then the linguistic variable V is called R-Q-interpretable if and only if the following holds for all i∈I and all x1,...,x a i∈T: RE i(x1,...,x a i)⇒Q i(s(x1),...,s(x a i))(9) where⇒represent an implication relation.1Qi is associated with the“semantic counterpart’’of RE i,i.e.,the relation that models RE i on the semantic level.3096S.-M.Zhou,J.Q.Gan/Fuzzy Sets and Systems159(2008)3091–3131Riid and Rustern gave a formalized definition for transparency of linguistic fuzzy model as follows.Definition2(Riid[142],Riid et al.[143]).The i th rule of the linguistic fuzzy system(2)is transparent if its activation degree satisfiesr i(x)=T j i,j(x j)=1(10) and results in system output as y=y i,where y i is the centre of the output MF B i(y)associated with the activated rule. In such a way,to define the transparency of1-order TS fuzzy system(3)and(4)is much more complicated[142–144]. Riid et al.suggested that thefirst order TS fuzzy system is transparent if the global output y can be derived directly on the basis of its local output y i,and they proposed a measure for transparency evaluation,but this measure results in further problems[143],i.e.,the minimum transparency error is obtained for systems that are non-fuzzy,and there is no trivial way for preserving this transparency for thefirst order TS fuzzy system.Unlike the above two formalized definitions,Nauck suggested an index to measure the interpretability of fuzzy rule bases for classification problems in terms of complexity,the degree of coverage of fuzzy partition over the domain,and a partition index that penalizes partitions with a high granularity[124].However,this definition only considers special circumstances of model construction for classification instead of prediction.More importantly,it ignores many other aspects of interpretable fuzzy models,such as distinguishability,rule base simplicity,etc.In interpretable fuzzy modelling,it might be not feasible to give a formalized definition for transparency of fuzzy models in practice[28],because the interpretability of fuzzy models heavily depends on human’s prior knowledge [58,59,198].To decide whether a specific fuzzy model is interpretable or not is a highly subjective task and it is contro-versial sometimes[38,121].Some researchers have become aware of this matter and proposed some constraint criteria that fuzzy models should meet to assure good interpretability during model adaptation or optimization[45,67,135–139].3.3.A framework for fuzzy model interpretabilityIn order to clearly discriminate the different roles of fuzzy sets,input variables and other components in achieving an interpretable fuzzy model,we propose in this paper a taxonomy of fuzzy model interpretability:low-level interpretability and high-level interpretability.First let us review fuzzy models from the perspective of statistical system modelling.3.3.1.Review of fuzzy models from the perspective of traditional statistical system modellingAtfirst sight,fuzzy modelling algorithms for the purpose of improving interpretability may seem rather strange and hardly related to the existing methods of traditional statistical parsimonious system modelling techniques.Once a fuzzy model is cast into a more standard mathematical notation,we will observe its connections to the traditional statistical system modelling methods.In the following,we consider the TS fuzzy models.However,depending on the defuzzification method,the Mamdani models can also be expressed in the similar manner,i.e.,as linear-in-parameters models for regression problems.Eq.(4)can be rewritten in a slightly different form,y=Li=1p i(x)(a0i+a1i x1+···+a ni x n)(11)wherep i(x)=r i(x)Li=1r i(x)(12)is the normalizedfiring strength of the i th rule.The great advantage of the fuzzy model is its representative power,that is,to describe a highly nonlinear system by using simple local models(rules).On the other hand,Eq.(11)can also be viewed as a linear-in-parameters model,y(x, )=Li=1w T i(x) i(13)S.-M.Zhou,J.Q.Gan/Fuzzy Sets and Systems159(2008)3091–31313097 where w i(x)=[p i(x),p i(x)x T]T∈R n+1and i=[a0i,a1i,...,a ni]T∈R n+1.If basis functions w i(x)arefixed, parameterization of(13)becomes linear with respect to parameters i.Given N input–output data sequences{x(k),y(k)}, k=1,...,N,(13)can be expressed in the matrix form,Y=W + (14) where Y=[y(1),y(2),...,y(N)]T∈R N,W=[w(1),w(2),...,w(L)]∈R N×(n+1)L,with w(i)=[w i(x(1)), w i(x(2)),...,w i(x(N))]T∈R N×(n+1),which is calledfiring strength matrix with each column corresponding to one of the fuzzy rules, =[ 1, 2,..., L]T∈R(n+1)L,and represents model error.Thus,the linear-in-parameters model can be addressed further from the angle of statistical regression analysis.According to the taxonomy developed in[33]to categorize methods for estimating continuous-valued functions from noisy samples,(13)is a dictionary representation in nature,comparing to kernel representation in which a continuous-valued function estimator is expressed as a distance-weighted combinations of observed output values.The set of w i(x)is called a dictionary.The goal of a predictive learning system that employs(13)for function regression is to adjust the degrees of freedom in(13)based on training samples,in such a way,the approximation function provides minimum prediction risk.In case the transparency of the fuzzy model with(13)and(14)is considered,the problem is reduced to the automatic selection of the dictionary from observed data accounting for the interpretability of the rule base.Thereupon,many statistical regression techniques,such as regularization and sparse regression,can be used or extended to attack this problem.However,one fundamental problem is that as the number of predictors in the regression function increases the curse of dimensionality applies.On the other hand,when viewing regression model(13)as a function estimation problem,one attractive approach for ameliorating the curse of dimensionality is to model the regression function as an additive function of the predictors [164,177].This approach has been popularized by Hastie and Tibshirani[75],who emphasized the use of back-fitting together with a one-dimensional smoother tofit the additive models to data as follows:f(x1,...,x n)=f0+ni=1f i(x i)(15)where f i are“smooth’’functions obtained by some smoothing processes,including the use of cubic smoothing splines. More general models for f,which allow the explicit modelling and visualization of possible interactions between variables,are expressed via functional analysis of variance decompositions,that is,the output can be represented by the ANalysis Of V Ariance(ANOV A)decomposition[177,178].An often used special ANOV A decomposition is expressed as follows:f(x)=f0+ni=1f i(x i)+ni=1nj=i+1f i,j(x i,x j)+···+f1,2,...,n(x)(16)where f0is a constant,f i are the x i main effects,f i,j are the effects from interactions between x i and x j,and so on.The regression function is thus represented as additive components by the subset of the terms from this expansion,which shows the attraction of decomposing the model into simpler and more transparent pieces that can be easily interpreted. Another statistical system modelling technique that can produce a parsimonious model structure is the method of orthogonal-least squares(OLS)[30]when applied to a linear-in-parameters model(14).The OLS method transforms the columns of the coefficient matrix W into a set of orthogonal basis vectors.The Gram–Schmidt orthogonalization procedure is used to perform this decomposition,i.e.,W=UA,where U is an orthogonal matrix such that U T U=I(I is the unity matrix)and A is an upper-triangular matrix with unity diagonal elements.Substituting W=UA into(14), we haveY=U A + =U z+ (17) where z=A .Let u i be the i th column of U,so the OLS solution of the system isˆz i=u T i Yu i u i(i=1,...,L)(18)。
fuzzy tassel Is Required

The dicer-like1Homolog fuzzy tassel Is Required for theRegulation of Meristem Determinacy in the In florescence and Vegetative Growth in MaizeW OPENBeth E.Thompson,a,1Christine Basham,a Reza Hammond,b,c Queying Ding,a Atul Kakrana,b,c Tzuu-Fen Lee,c Stacey A.Simon,c Robert Meeley,d Blake C.Meyers,c and Sarah Hake ea Department of Biology,East Carolina University,Greenville,North Carolina 27858b Centerfor Bioinformatics and Computational Biology,University of Delaware,Newark,Delaware 19714c Department of Plant and Soil Sciences,University of Delaware,Newark,Delaware 19711d Pioneer,A Dupont Company,Johnston,Iowa 50131e Plant Gene Expression Center and University of California-Berkeley,Albany,California 94710ORCID ID:0000-0003-3436-6097(B.C.M.)Plant architecture is determined by meristems that initiate leaves during vegetative development and flowers during reproductive development.Maize (Zea mays )in florescences are patterned by a series of branching events,culminating in floral meristems that produce sexual organs.The maize fuzzy tassel (fzt )mutant has striking in florescence defects with indeterminate meristems,fasciation,and alterations in sex determination.fzt plants have dramatically reduced plant height and shorter,narrower leaves with leaf polarity and phase change defects.We positionally cloned fzt and discovered that it contains a mutation in a dicer-like1homolog,a key enzyme required for microRNA (miRNA)biogenesis.miRNAs are small noncoding RNAs that reduce target mRNA levels and are key regulators of plant development and physiology.Small RNA sequencing analysis showed that most miRNAs are moderately reduced in fzt plants and a few miRNAs are dramatically reduced.Some aspects of the fzt phenotype can be explained by reduced levels of known miRNAs,including miRNAs that in fluence meristem determinacy,phase change,and leaf polarity.miRNAs responsible for other aspects of the fzt phenotype are unknown and likely to be those miRNAs most severely reduced in fzt mutants.The fzt mutation provides a tool to link speci fic miRNAs and targets to discrete phenotypes and developmental roles.INTRODUCTIONPlant development is dependent on the activity of meristems,groups of indeterminate,self-renewing cells that initiate new organs.Maintenance of the balance between organ initiation at the periphery and self-renewal in the central stem cells is critical for plant growth (Steeves and Sussex,1989).The shoot apical meristem initiates leaf primordia during vegetative development.As the plant becomes reproductive,leaf primordia become smaller and axillary branch meristems,in the axils of leaves,become more prominent.Ultimately,in florescence meristems are formed that will produce flowers.Meristems are considered indeterminate if the central stem cells are maintained during the production of meristem or organ primordia,whereas meristems are considered determinate if the central stem cells are consumed,as in a floral meristem.Maize (Zea mays )produces two in florescences,the tassel and the ear,which produce male and female flowers,re-spectively.The tassel is the product of the apical in florescence meristem,while the ear is the product of an axillary meristem.Inboth the tassel and the ear,the in florescence meristem initi-ates secondary and higher order meristems,culminating in the formation of floral meristems.The imposition of determi-nacy on the higher order meristems determines in florescence architecture.MicroRNAs (miRNAs)are key regulators of meristem fate and function in maize and other plants.In maize,tasselseed4(ts4),which encodes miR172e,is required for meristem determinacy in multiple higher order meristems,in addition to playing a role in sex determination.ts4/miR172e represses two AP2-like genes,ids1and sid1(Chuck et al.,2007b,2008),a regulatory module that is conserved in Arabidopsis thaliana and rice (Oryza sativa )(Aukerman and Sakai,2003;Lee and An,2012).miR156is re-quired for leaf suppression in the in florescence and plays a key role in determining the meristem and leaf boundary (Chuck et al.,2010).The dominant mutant Corngrass1(Cg1),which is caused by the overexpression of miR156,has a fasciated in florescence meristem,indicating that miR156also plays a role in stem cell homeostasis (Chuck et al.,2007a).miRNAs have key roles in vegetative development.The bal-ance between miR156and miR172is critical to determine the switch from vegetative to reproductive development,also known as phase change,in maize,Arabidopsis ,and other plants (Wu and Poethig,2006;Chuck et al.,2007a,2011;Poethig,2013).miR165and miR166repress class III homeodomain-leucine zipper tran-scription factors to regulate abaxial/adaxial leaf polarity (McConnell et al.,2001;Juarez et al.,2004).1Addresscorrespondence to thompsonb@.The author responsible for distribution of materials integral to the findings presented in this article in accordance with the policy described in the Instructions for Authors ()is:Beth Thompson (thompsonb@).WOnline version contains Web-only data.OPENArticles can be viewed online without a /cgi/doi/10.1105/tpc.114.132670This article is a Plant Cell Advance Online Publication.The date of its first appearance online is the official date of publication.The article has been edited and the authors have corrected proofs,but minor changes could be made before the final version is published.Posting this version online reduces the time to publication by several weeks.The Plant Cell Preview, ã2014American Society of Plant Biologists.All rights reserved.1of 16miRNAs are small noncoding RNAs of20to22nucleotides in length that posttranscriptionally repress gene expression in plants and animals(Bushati and Cohen,2007;Bartel,2009;Chen,2009; Voinnet,2009;Krol et al.,2010).miRNA genes are transcribed by RNA polymerase II as long,primary microRNA(pri-miRNA)tran-scripts that contain one or more hairpin structures(Xie et al., 2005).The pri-miRNA undergoes two sequential processing events to generate the mature miRNA.The pri-miRNA is cleaved to liberate the hairpin(60to80nucleotides in animals,more variable in plants)and generate the precursor microRNA (pre-miRNA).The pre-miRNA is then cleaved to release a small RNA duplex,consisting of the miRNA and its complement,the miRNA*.In plants,processing of both the pri-miRNA and pre-miRNA occurs in the nucleus,primarily by the RNA endonu-clease DICER-LIKE1(DCL1)(Kurihara and Watanabe,2004).In Arabidopsis,DCL1also requires additional protein partners for efficient and accurate miRNA processing,including the con-served double-stranded RNA binding protein HYPONASTIC LEAVES1(HYL1),a zincfinger protein,SERRATE(SE) (Vazquez et al.,2004;Yang et al.,2006),and a second RNA binding protein,TOUGH(TGH)(Ren et al.,2012).The fork-head protein DAWDLE(DDL)and the cap binding proteins CPB80/ABH1and CBP-20are also required for pri-miRNA processing(Gregory et al.,2008;Laubinger et al.,2008;Yu et al.,2008).Finally,plant miRNAs are methylated by HUA ENHANCER1(HEN1),a modification that stabilizes miRNAs (Yu et al.,2005).In Arabidopsis a related enzyme,DCL4,can also process some miRNAs,in particular newly evolved miRNAs with hairpins of high degrees of complementarity (Rajagopalan et al.,2006;Fahlgren et al.,2007;Ben Amor et al.,2009).miRNAs repress target mRNAs by two major mechanisms: mRNA cleavage and translational inhibition(Bartel,2004).The mature miRNA is incorporated into the RNA-induced silencing complex and guides it to mRNAs containing miRNA comple-mentary sequences.Most plant miRNAs have nearly perfect complementarity with target mRNAs and cleave their targets, although increasing evidence suggests that translational inhibition is also widespread(Brodersen et al.,2008).Target cleavage requires the endonuclease ARGONAUTE1(Vaucheret et al., 2004),and translational inhibition takes place on the endoplasmic reticulum,although the exact mechanism is still unknown(Li et al., 2013).The key role of miRNAs during development is underscored by the broad range of developmental defects in miRNA bio-genesis mutants.For example,null alleles of Arabidopsis DCL1 are embryonic lethal,and hypomorphic alleles have defects in integument,ovule,andfloral development(Schauer et al.,2002). In rice,strong DCL1RNA interference knockdowns result in developmental arrest,while weak knockdowns exhibit defects in plant growth,shoot,root,and leaf development(Liu et al.,2005). In addition,Arabidopsis mutants in other miRNA biogenesis enzymes,including hyl1,se,tgh,ddl,abh1,and hen1,have pleiotropic developmental defects,presumably due to the mis-regulation of miRNA target mRNAs(Clarke et al.,1999;Lu and Fedoroff,2000;Hugouvieux et al.,2001;Prigge and Wagner, 2001;Chen et al.,2002;Calderon-Villalobos et al.,2005;Morris et al.,2006;Yang et al.,2006).We isolated a maize mutant,fuzzy tassel(fzt),with a broad range of vegetative and reproductive defects.fzt mutants have particularly striking inflorescence defects,including increased indeterminacy of multiple meristems and defects in stem cell homeostasis and sex determination.fzt mutants also have vegetative defects,including reduced plant stature,and short narrow leaves with mild polarity defects.Positional cloning showed that fzt contains a mutation in DCL1,a key enzyme in the miRNA biogenesis pathway.The levels of most miRNAs are moderately reduced in fzt mutants;however,a few miRNAs are more dramatically reduced,suggesting that developmental defects in the fzt mutant are caused by reduced levels of a subset of miRNAs and the upregulation of specific miRNA-targeted mRNAs. RESULTSfzt Is Required during Vegetative andReproductive Developmentfzt was isolated by screening an M2population of A619ethyl methanesulfonate(EMS)-mutagenized plants.The mutant was backcrossed to A619a minimum of three times prior to analysis; analysis was done in the A619inbred background unless noted otherwise.fzt is recessive,100%penetrant,and has striking reproductive defects and reduced plant stature(Figure1; Supplemental Figure1).We also backcrossed the fzt mutation to Mo17and B73a minimum of three times for analysis.fzt phe-notypes are qualitatively similar in all inbred backgrounds ex-amined;however,some defects appear more severe in the Mo17and B73inbred backgrounds(see below).Plant stature is dramatically reduced in fzt mutants.fzt plants are less than one-third the height of normal sibling plants(Figure 1A;Supplemental Figure1A).We counted the number of leaves, including thefirst juvenile leaves,to determine if this loss of stature was due to short or missing internodes.Whereas normal siblings produced on average15leaves,fzt mutants produced only an average of12leaves,suggesting that the short stature was a combination of both missing and shorter internodes (Supplemental Figure1B).To confirm thisfinding,we quantified the number and length of internodes at maturity and found that normal plants had an average of10.5nodes per plant whereas fzt plants had an average of only8nodes per plant(Supplemental Figure1C).We also measured internode length in fzt and normal plants.fzt plants had significantly shorter internodes,except for the top-most internode of fzt plants(internode8)(Supplemental Figure1D).Leaf size was also reduced in fzt plants;fzt leaves were only about two-thirds the length and less than one-half the width of normal leaves(Supplemental Figures1E and1F).To determine if reduced leaf size was due to a decrease in cell size or cell number, we counted total epidermal cell number per unit area as a measure of cell size;increased cell number per unit area would be indicative of decreased cell size in fzt plants.Surprisingly,we found that fzt plants had a slight decrease in cell number per unit area compared with normal siblings,suggesting that cell size is slightly increased in fzt leaves compared with normal siblings (Supplemental Figure1G).Thus,the decrease in leaf size is likely2of16The Plant Celldue to a decrease in total cell number rather than decreased cell size.The reproductive defects in fzt plants are particularly striking (Figures 1B to 1I).In normal maize plants,tassels produce sta-minate flowers and ears produce pistillate flowers,due to the abortion of pistils in the tassel and stamen arrest in the ear.In addition to sex organs,maize florets contain grass-speci fic or-gans,including lodicules,palea,and lemma.Lodicules have ho-mology to petals (Ambrose et al.,2000),and palea and lemma are bract-like organs.Spikelets are produced in pairs;each spikelet consists of two florets enclosed by two bracts,called glumes (Figure 1C).In ears,one of the two florets aborts (Figure 1G).In fzt plants,both male and female in florescences exhibit multiple de-fects resulting in complete sterility.fzt tassels often produce extra spikelets,and the spikelets contain more than two florets (Figure 1E).fzt tassel florets make an excess of palea/lemma-like organs,and the stamens are small,undeveloped,and never shed pollen.fzt spikelets also lack recognizable glumes,resulting in exposed floral organs and their “fuzzy ”appearance (Figure 1D).On average,fzt tassels produce only one-half the number of tassel branches as normal siblings (5branches in fzt versus 9.5branches in normal siblings)(Supplemental Figure 1H).fzt tassels occasionally pro-duce silks,indicating that carpel abortion is defective.The fzt mutation also severely affects ear development.fzt ear spikelets are enclosed by bracts that morphologically resembletassel glumes and contain extra florets (Figure 1I).Ear florets also make extra palea-like organs and often contain immature stamens,indicating that fzt is required for multiple aspects of sex determination in the ear.We observed similar phenotypes when fzt was introgressed into the Mo17and B73inbred backgrounds (Supplemental Figure 2).The fzt mutation had similar effects on plant stature in all three inbred backgrounds (Supplemental Figures 2A,2B,2J,and 2K);however,the in florescence defects were more severe in Mo17and B73than in the A619inbred.fzt [Mo17]and fzt [B73]tassels were highly branched and formed no recognizable flor-ets,although tassel “spikelets ”produced lemma/palea-like or-gans and a few undeveloped and abnormal stamens (Supplemental Figures 2D,2G,2M,and 2N).fzt [B73]ears were highly branched and contained many immature meristems at maturity.Almost no floral organs were produced,except for rare immature and abnor-mal stamens (Supplemental Figures 2F,2H,and 2I).fzt [Mo17]plants generally lacked ears.fzt Contains a Mutation in DCL1To gain insight into the molecular underpinnings of the fzt phenotype,we positionally cloned the gene.fzt mapped to the short arm of chromosome 1between the simple sequence re-peat markers bnlg1124and umc1292.We developednewFigure 1.fzt Mutants Have Severe Vegetative and In florescence Defects.(A)Normal sibling (left)next to an fzt mutant (right).fzt mutants are much shorter than normal siblings.(B)Normal tassel.(C)A normal tassel spikelet containing two florets.Glumes have been removed to expose the florets.(D)fzt mutant tassel.Spikelets lack recognizable glumes.(E)fzt tassel spikelet containing extra florets.fzt florets contain abnormal stamens that do not shed pollen and other abnormal floral organs.(F)Normal ear.(G)Dissected ear spikelet from a normal ear contains a single floret.(H)fzt ear contains few silks and abnormal bracts and is sterile.(I)fzt ear spikelet contains extra florets,abnormal floral organs,and undeveloped stamens.White arrowheads indicate florets.Bars =2cm.fzt Regulates Meristem Determinacy 3of 16polymorphic markers to narrow the fzt-containing region to an ;3.2-centimorgan region,which included33predicted genes, 26of which had functional annotations(gene predictions were obtained from thefiltered gene set of the maize B73RefGen_v2) (Figure2A).One gene in this interval,dcl1,stood out as a par-ticularly strong candidate.DCL1is a key enzyme required for miRNA biogenesis in Arabidopsis and other plant species and is broadly expressed during development(Sekhon et al.,2011). Given the well-established role of miRNAs in many developmental processes,including the regulation of meristem determinacy in maize,a mutation in dcl1seemed likely to underlie the pleiotropic phenotypes of fzt.DCL proteins contain several conserved domains,including a bipartite helicase domain,a DUF283domain that was recently defined as a novel RNA binding motif(Qin et al.,2010),two RNase III domains(RNase IIIa and RNase IIIb),and two double-stranded RNA binding domains(Figure2B).The dcl1locus corresponds to gene model GRMZM2G040762.To assemble the full dcl1genomic sequence and predict a full-length coding sequence,we assembled maize BAC sequences and used similarity with the rice and Arabidopsis DCL1protein sequences to predict a full-length maize DCl1protein(1929amino acids) and corresponding coding sequence(5790nucleotides)(see Methods).We sequenced the predicted dcl1coding region from fzt and A619plants and found a G-to-A mutation in fzt mutants corresponding to exon17and predicted to cause an S-to-N substitution in the RNase IIIa domain(Figure2B;Supplemental Figure3).To generate additional alleles and confirm that we isolated the correct gene,we conducted a noncomplementation screen in which Mo17EMS-mutagenized pollen was crossed onto fzt heterozygotes and the resulting progeny were scored for fzt phenotypes.We found one plant with the fzt phenotype that contained a G-to-A mutation in exon19,which is predicted to introduce a premature stop codon and truncate DCL1by39 amino acids.The fzt/fzt-EMS plant was sterile,and we were unable to recover the new allele for further experiments.We obtained four additional dcl1alleles(dcl1-mum1to dcl1-mum4) through reverse genetics resources(Bensen et al.,1995).Three alleles(dcl1-mum2to dcl1-mum4)contain Mu insertions intheFigure2.fzt Contains a Mutation in dcl1.(A)Mapping data for fzt.(B)Genomic region of dcl1with mutant alleles indicated.Black triangles indicate insertion sites of Mutator transposon insertions(dcl1-mum1-4). Orange boxes indicate protein-coding exons,and gray boxes indicate39untranslated regions.(C)Schematic of the DCL1protein with conserved domains indicated.The predicted effects of the mutant alleles on the DCL1protein are indicated.a.a.,amino acids.4of16The Plant Cellprotein-coding region of exon1and are likely null alleles;all three alleles fail to complement fzt.Based on dcl1null pheno-types in Arabidopsis,we hypothesized that null alleles of dcl1 are embryonic lethal.We examined the self-progeny of dcl1-mum3heterozygotes,one-quarter of which are predicted to be dcl1-mum3homozygotes.We found that49of172(28.4%) seeds lacked recognizable embryos,and all seeds with a rec-ognizable embryo contained at least one normal dcl1allele (Supplemental Figure4B).Thus,dcl1-mum3homozygotes are indeed early embryonic lethal.Homozygous dcl1-mum2and dcl1-mum4plants were never recovered,suggesting that these alleles are also embryonic lethal.We also asked if dcl1-mum3is transmitted normally by analyzing progeny from reciprocal crosses. The dcl1-mum3allele is inherited in the expected Mendelian ratios (P>0.1in x2test),indicating that dcl1-mum3is transmitted nor-mally by both the male and female gametophytes(Supplemental Figure4C).Based on these results,we conclude that fzt is a dcl1 allele,and we refer to our allele as dcl1-fzt.We also obtained three dcl1tilling alleles from the Maize Tilling Project(Till et al.,2004)that contained nonsilent point mutations in or near the helicase domain (Figures2B and2C).Interestingly,plants homozygous for these alleles were phenotypically normal and complemented the fzt mu-tation,suggesting that the helicase domain may not be critical for DCL1function in an otherwise normal genetic background,al-though it is also possible that these mutations do not impair heli-case function.dcl1-fzt Mutants Are Defective in miRNA-Regulated ProcessesmiRNAs have well-known roles in plant development(Bartel, 2009;Chen,2009;Rubio-Somoza and Weigel,2011).Given that dcl1-fzt carries a mutation in a key enzyme required for miRNA biogenesis,we predicted that dcl1-fzt mutants would have re-duced miRNA levels.Therefore,we examined dcl1-fzt mutants for defects in several miRNA-regulated processes.miRNA regulation is required to regulate meristem determinacy in the inflorescence.The dcl1-fzt inflorescence defects resemble those of the ts4mutant,which is caused by a loss-of-function mutation in miR172e.Reduced miR172e expression leads to the loss of meristem determinacy and sex determination defects (Chuck et al.,2007b,2008).To more closely examine dcl1-fzt mutants for these defects,we examined early inflorescence de-velopment by scanning electron microscopy.In normal plants, the inflorescence meristem(IM)gives rise to ordered rows of spikelet pair meristems(SPMs).Each SPM gives rise to two spikelet meristems(SMs),which in turn give rise to twofloral meristems(FMs),which produce thefloral organs.Development in the tassel and ear is similar,except that in the tassel the IM also initiates branch meristems(BMs)and in the ear only onefloret per spikelet develops to maturity.In ts4mutants,SPMs initiate extra spikelets and SMs initiate extra FMs(Chuck et al., 2007b).We observed similar defects in dcl1-fzt plants(Figure 3).The SPMs of fzt mutants initiated more than two SMs(as-terisks in Figure3J)and the SMs of fzt mutants persisted(arrow in Figure3L),initiating more than the normal two FMs(asterisks in Figure3L).We also found that miR172e was substantially reduced in dcl1-fzt mutants compared with normal controls (see below),consistent with the observed meristem de-terminacy defects.Normal meristems grow as a single apex by balancing stem cell growth with the rate of primordium initiation.Meristems that lose this homeostasis broaden and become fasciated(Aichinger et al.,2012;Pautler et al.,2013).We consistently noted fascia-tion in dcl1-fzt IMs(Figure3H;Supplemental Figure5).The broadened tip produced many more meristems than normal.We also observed fasciation in the BMs(Figure3H,arrowhead). Finally,SPMs were not initiated in ordered rows and were ir-regular in shape and size(Figures3E and3G;Supplemental Figure5).These defects are not observed in the ts4mutant, suggesting that miRNAs in addition to miR172e are reduced in the dcl1-fzt mutant and have key roles in meristem homeostasis and determinacy.miRNAs also have well-established roles in vegetative de-velopment.Normal maize leaves have a ligule on the adaxial surface and distinct hairs on the adaxial and abaxial surfaces. Mutations in the miR166binding site of Rolled-leaf1cause the adaxialization of leaf surfaces,resulting in curled leaves and abaxial ligules(Juarez et al.,2004).Since dcl1-fzt leaves did not exhibit these macroscopic polarity defects,we examined the leaves for subtle polarity defects.In normal leaves,macrohairs are restricted to the adaxial surface of leaf blades and are often used as adaxial markers.We examined the distribution of macrohairs on dcl1-fzt and normal leaf blades and found that dcl1-fzt leaves contained fewer macrohairs on the adaxial blade compared with normal siblings(Figures4A and4B).In addition, dcl1-fzt leaves contained macrohairs on the abaxial blade,while normal leaves did not(Figures4C and4D).Interestingly, this defect was more severe in the Mo17inbred background (Figures4A to4D)than in the A619background(Supplemental Figure6),in which abaxial macrohairs were restricted to the leaf margins and were not present throughout the blade (Supplemental Figure6D).Vascular bundles are also polarized,with the xylem positioned at the adaxial pole and phloem positioned at the abaxial pole. We examined the polarity of vascular bundles in dcl1-fzt[Mo17] and normal sibling plants in cross sections.We found that dcl1-fzt[Mo17]mutants had subtle defects in vascular organization. The xylem was more disorganized in dcl1-fzt[Mo17]mutants than in normal siblings and extended farther toward the abaxial pole than normal(Figures4E and4F).Together,these results are consistent with mild leaf polarity defects in dcl1-fzt mutants,in which the abaxial surface acquired adaxial characteristics. Phase change from the juvenile to adult life phase is another well-known miRNA-regulated process in plants and is controlled by the antagonistic activities of miR156and miR172(Chuck et al.,2007a;Poethig,2013).Juvenile and adult leaves make distinct epicuticular waxes,which can be distinguished by to-luidine blue staining:juvenile cells stain violet in color,while adult cells stain blue.We found that in the A619inbred back-ground,dcl1-fzt mutants begin making adult waxes about one leaf later than normal siblings(Supplemental Figure7).Com-bined with the node number data(Supplemental Figure1C),this indicates that,on average,dcl1-fzt[A619]plants gain one juvenile internode and lose four adult internodes.By contrast,in the Mo17inbred background,dcl1-fzt mutants begin makingfzt Regulates Meristem Determinacy5of16adult leaf waxes approximately one leaf earlier than normal siblings (Supplemental Figure 7).While initially it seems para-doxical that dcl1-fzt has opposite effects on phase change de-pending on the inbred background,this is not necessarily unexpected,given that the timing of phase change is regulated by the opposing activities of miR156and miR172and their target genes.Slight changes in the relative levels of these genes could shift the balance of downstream target genes and the timing of phase change.An alternative explanation for the apparent background effects on phase change is incomplete introgression.A619and Mo17flower at different times,with Mo17up to 2weeks later than A619,and thus the early phase change in dcl1-fzt [Mo17]plants could be due to residual A619alleles that promote early phase change.We think that this hy-pothesis is unlikely for two reasons.First,we compared dcl1-fzt [Mo17]with normal siblings;both groups should have similar amounts of residual A619DNA.Second,normal siblings from both the A619and Mo17introgressions transition at thesameFigure 3.dcl1-fzt In florescences Make Abnormal Meristems.(A)to (H)Scanning electron micrographs of normal ([A]to [D])and dcl1-fzt mutant ([E]to [H])in florescences.A young normal ear (A)is compared with a young dcl1-fzt mutant ear (E).dcl1-fzt IMs are flattened and broader than normal,indicating mild fasciation.dcl1-fzt SPMs are enlarged and not initiated in ordered rows.An older normal ear (B)is compared with a dcl1-fzt older ear (F).Normal tassels ([C]and [D])are compared with dcl1-fzt mutant tassels ([G]and [H]).The white arrowhead in (H)indicates fasciated BMs.(I)Normal spikelet pair contains two SMs.Each SM is subtended by a glume.(J)fzt mutant spikelet “pair ”contains extra SMs,and not all SMs are subtended by glumes.(K)Normal spikelet pair during floral development.Each spikelet consists of an upper FM and a lower FM.Floral organs are initiated in a stereotypical,ordered manner.(L)Older fzt mutant spikelet pair.Spikelets initiate extra FMs.Floral development is abnormal,and floral organs are not initiated properly.An in-determinate branch-like meristem persists (black arrow).Black asterisks indicate SMs,and white asterisks indicate FMs/developing florets.Bars in (A)to (H)=0.5mm;bars in (I)to (L)=100m m.6of 16The Plant Cellpoint in development (leaf 5to 6),whereas dcl1-fzt [Mo17]plants begin to transition two leaves before dcl1-fzt [A619]plants (leaf 5in Mo17versus leaf 7in A619).Thus,Mo17alleles promote an earlier transition in the presence of the dcl1-fzt mutation.To-gether,these results indicate that dcl1-fzt mutants have defects in known miRNA-regulated processes in plants,including mer-istem determinacy,leaf polarity,and phase change,consistent with decreased miRNA levels in dcl1-fzt mutants.miRNA Levels Are Reduced in fzt MutantsTo determine the effect of the dcl1-fzt mutation on miRNA bio-genesis,we analyzed the small RNA populations in 14-d-old seedlings and tassel primordia of dcl1-fzt and normal plants by deep sequencing.In total,small RNA libraries representing threebiological replicates of seedlings and tassel primordia from dcl1-fzt and normal controls were generated and sequenced (Supplemental Table 1).The raw small RNA sequences were processed to remove adapter sequences and matched to the maize genome (AGPv2).To compare between libraries,the count/abundance of each small RNA was normalized based on the sequencing depth as reads per 10million.We found that the small RNA pro files from normal and dcl1-fzt seedlings and tassel primordia are similar (Supplemental Figure 8).To determine if the dcl1-fzt mutation affected the accumulation of a subset of miRNAs,we compared the levels of individual miRNAs in dcl1-fzt seedlings and tassel primordia with normal controls.Ap-proximately one-third of the detectable miRNAs (22of 63in seedlings and 14of 45in tassel primordia)were differentially expressed in dcl1-fzt mutants compared with normal controls (P <0.05and false discovery rate [FDR]<0.05)(Figure 5;Supplemental Table 2).miRNAs are denoted as -5p or -3p to indicate from which arm of the hairpin precursor the mature miRNA is processed (Grif fiths-Jones et al.,2006).Eight miRNAs were reduced in both seedlings and tassel primordia tissues,consistent with a defect in the processing of speci fic miRNAs (miRNAs in boldface and underlined in Figure 5).Not all miRNAs are affected to the same extent in dcl1-fzt mutants,suggesting a processing defect in a subset of miRNAs.For example,miR167a-d-5p was reduced 20-to 30-fold in both seedlings and tassel primordia,whereas miR160a-e,g-5p was decreased only 3-to 5-fold,and many miRNAs did not meet the statistical threshold for differential expression.We note,however,that al-though many miRNAs did not meet the statistical threshold for differential expression,nearly all of these “nonsigni ficant miRNAs ”(38of 40in seedlings and 24of 31is tassel primordia)appeared to decrease in dcl1-fzt mutants,suggesting a broad,moderate re-duction in the levels of miRNAs.Mature miRNAs are processed from primary miRNA tran-scripts,and miRNA processing mutants often have increased levels of miRNA precursors (Kurihara and Watanabe,2004;Kurihara et al.,2006;Yang et al.,2006;Laubinger et al.,2010).Therefore,we also examined the expression of pri-miRNA transcripts in dcl1-fzt and normal controls using RNA sequencing (RNA-seq;see below).In both seedlings and tassel primordia,;50%of detectable miRNAs were differentially expressed be-tween dcl1-fzt and normal controls (P <0.05;16of 38in seed-lings and 20of 39in tassel primordia).Nearly all differentially expressed precursors were increased in dcl1-fzt mutants com-pared with normal controls (16of 16in seedlings and 17of 20in tassel primordia)(Supplemental Figure 9and Supplemental Table 3).For approximately half of the upregulated precursors,the cor-responding mature miRNA was decreased in dcl1-fzt mutant plants (pri-miRNAs are in boldface and underlined in Supplemental Figure 9).The molecular analysis of miRNA and pri-miRNA levels,combined with genetic analysis of the dcl1-fzt allele,strongly support the conclusion that dcl1-fzt is a hypomorphic allele and indicate that the DCL1-FZT enzyme is defective in processing a subset of miRNA precursors.Plant miRNAs primarily regulate gene expression by promoting the cleavage and degradation of target mRNAs (Bartel,2004).Therefore,we expect mRNAs targeted by miRNAs reduced in dcl1-fzt to be increased in dcl1-fzt plants compared withnormalFigure 4.dcl1-fzt Is Required for Normal Leaf Cell Differentiation.Macrohairs (arrows)are present on the adaxial surface of a normal leaf blade (A)but absent from the abaxial surface (C).dcl1-fzt [Mo17]con-tains fewer macrohairs on the adaxial surface (B),but macrohairs are present on the abaxial surface (D).Vascular polarity is also perturbed in dcl1-fzt [Mo17]mutants ([E]and [F]).A normal vascular bundle is shown in (E).Xylem cells (red asterisks)are positioned adaxially relative to the phloem cells (P).In dcl1-fzt [Mo17]mutants (F),the xylem cells are dis-organized and extend farther toward the abaxial pole than normal.Bars in (A)and (B)=1mm;bars in (C)and (D)=400m m;bars in (E)and (F)=50m m.fzt Regulates Meristem Determinacy 7of 16。
semantics知识点总结

semantics知识点总结Semantics is the study of meaning in language. It is concerned with how words and sentences are interpreted, how meaning is assigned to linguistic expressions, and how meaning is inferred from language. In this summary, we will explore some key concepts and topics in semantics, including the following:1. Meaning and reference2. Sense and reference3. Truth-conditional semantics4. Lexical semantics5. Compositional semantics6. Pragmatics and semantics7. Ambiguity and vagueness8. Semantic changeMeaning and referenceMeaning is a fundamental concept in semantics. It refers to the content or interpretation that is associated with a linguistic expression. The study of meaning in linguistics is concerned with understanding how meaning is established and conveyed in language. Reference, on the other hand, is the relationship between a linguistic expression and the real world entities to which it refers. For example, the word "dog" refers to the concept of a four-legged animal that is commonly kept as a pet. The study of reference in semantics is concerned with understanding how words and sentences refer to objects and entities in the world.Sense and referenceThe distinction between sense and reference is an important concept in semantics. Sense refers to the meaning or concept associated with a linguistic expression, while reference refers to the real world entities to which a linguistic expression refers. For example, the words "morning star" and "evening star" have the same reference - the planet Venus - but different senses, as they are used to describe the planet at different times of the day. Frege, a prominent philosopher of language, introduced this important distinction in his work on semantics.Truth-conditional semanticsTruth-conditional semantics is an approach to semantics that seeks to understand meaning in terms of truth conditions. According to this view, the meaning of a sentence isdetermined by the conditions under which it would be true or false. For example, the meaning of the sentence "The cat is on the mat" is determined by the conditions under which this statement would be true - i.e. if there is a cat on the mat. Truth-conditional semantics has been influential in the development of formal semantics, and it provides a formal framework for analyzing meaning in natural language.Lexical semanticsLexical semantics is the study of meaning at the level of words and lexical items. It is concerned with understanding the meanings of individual words, as well as the relationships between words in a language. Lexical semantics examines how words are related to each other in terms of synonymy, antonymy, hyponymy, and other semantic relationships. It also explores the different senses and meanings that a word can have, and how these meanings are related to each other. Lexical semantics plays a crucial role in understanding the meaning of sentences and discourse.Compositional semanticsCompositional semantics is the study of how the meanings of words and sentences are combined to create complex meanings. It seeks to understand how the meanings of individual words are combined in sentences to produce the overall meaning of a sentence or utterance. Compositional semantics is concerned with understanding the rules and principles that govern the composition of meaning in natural language. It also explores the relationship between syntax and semantics, and how the structure of sentences contributes to the interpretation of meaning.Pragmatics and semanticsPragmatics is the study of how language is used in context, and how meaning is influenced by the context of language use. Pragmatics is closely related to semantics, but it focuses on the use of language in communication, and how meaning is affected by factors such as the speaker's intentions, the hearer's inferences, and the context in which the language is used. While semantics is concerned with the literal meaning of linguistic expressions, pragmatics is concerned with the implied meaning that arises from the use of language in context.Ambiguity and vaguenessAmbiguity and vagueness are common phenomena in natural language, and they pose challenges for semantic analysis. Ambiguity refers to situations where a linguistic expression has multiple possible meanings, and it is unclear which meaning is intended. For example, the word "bank" can refer to a financial institution or the edge of a river. Vagueness, on the other hand, refers to situations where the boundaries of a linguistic expression are unclear or indistinct. For example, the word "tall" is vague because it is not always clear what height qualifies as "tall". Semantics seeks to understand how ambiguity and vagueness arise in language, and how they can be resolved or managed in communication.Semantic changeSemantic change refers to the process by which the meanings of words and linguistic expressions evolve over time. Over the course of history, languages undergo semantic change, as words acquire new meanings, lose old meanings, or change in their semantic associations. Semantic change can occur through processes such as metaphor, metonymy, broadening, narrowing, and generalization. Understanding semantic change is important for the study of historical linguistics and the diachronic analysis of language.ConclusionSemantics is a rich and complex area of study that plays a fundamental role in understanding the meaning of language. It encompasses a wide range of topics and concepts, and it has important implications for fields such as philosophy of language, cognitive science, and natural language processing. By exploring the key concepts and topics in semantics, we can gain valuable insights into how meaning is established and conveyed in language, and how we can analyze and understand the rich complexity of linguistic expressions.。
Semantic Web Query Languages

More expression testing (date-time support, for example)
Using DESCRIBE clauses to return descriptions of the resources matching the query part. Enables sorting. Specify OPTIONAL triple or graph query patterns Testing the absence, or non-existence, of tuples.
Query File
Query File
Executing SPARQL Queries Using Jena and Java
Set class path, this may differ according to Jena version. Write your java program and execute it. Using Jena and Java gives you the ability to process query output in the way you like. Example program
SeRQL )Sesame RDF Query Language(
Based on several existing languages, most notably RQL, RDQL and N3. SeRQL is easier to parse than RQL. Missing functions: eg. aggregation (minimum, maximum, average, count) SeRQL is not safe as it provides various recursive built-infunctions.
- 1、下载文档前请自行甄别文档内容的完整性,平台不提供额外的编辑、内容补充、找答案等附加服务。
- 2、"仅部分预览"的文档,不可在线预览部分如存在完整性等问题,可反馈申请退款(可完整预览的文档不适用该条件!)。
- 3、如文档侵犯您的权益,请联系客服反馈,我们会尽快为您处理(人工客服工作时间:9:00-18:30)。
A Fuzzy Semantics for Semantic Web LanguagesMauro Mazzieri1and Aldo Franco Dragoni21mauro.mazzieri@2Universit`a Politecnica delle MarcheDipartimento di Elettronica,Intelligenza Artificiale e Telecomunicazioni(DEIT)a.f.dragoni@univpm.itAbstract.Although the model-theoretic semantics of the languagesused in the Semantic Web are crisps,the need arise to extend themto represent fuzzy data,in the same way fuzzy logic extendfirst-order-logic.We will define a fuzzy counterpart of the RDF Model Theory forRDF(section2)and RDF Schema(section3).Last,we show how toimplement the extended semantics in inference rules(section4).Keywords:Fuzzy Logic,Knowledge Representation,Semantic Web,RDF, RDF Schema.1Knowledge representation on the webThe Semantic Web is an extension of the current web in which information is given well-defined meaning[1]by the use of knowledge representation(KR) languages.The KR languages used(RDF,RDF Schema and OWL)have the character-istics that make them useful on the web[2]:–the elements of the domain are represented by URI;–there is no global coherence requirements,as local sources can make asser-tions independently without affecting each other’s expressiveness.The languages have the ability to describe,albeit not formally,much more than their semantics can express.Their model theory captures only a formal no-tion of meaning,captured by inference rules;the exact‘meaning’of a statement can depend on many factors,not all accessible to machine processing[3].This feature can be useful to represent information fromfields that require knowl-edge representation paradigms other than the FOL-like RDF Model Theory or the expressive Description Logic used by OWL.Amongst those paradigms there is fuzzy logic,to represent vague or ambiguous knowledge.2Fuzzy RDFRDF has its own model-theoretic semantics,similar to that offirst-order logic. To represent fuzzy data,we will define a syntactic and semantic extension of RDF,similar to the extension fromfirst-order logic to fuzzy logic.Even if fuzzy data can be simply seen as a juxtaposition of a triple and a number,the model-theoretic approach has well-known theoretical advantages.We will try to be as plain as possible.Starting from RDF Syntax and RDF Model Theory,we will make as few changes as possible.In the rest of the paper, for the sake of brevity only the changes from RDF Semantics[3]are shown.2.1SyntaxThe RDF syntax must be extended to add to the triple subject,predicate, object a value.Such a value can be taken as a real number in the interval[0, 1],but every bounded real interval will do.This is not an extension from a3-elements tuple to a4-elements tuple as it may seem at afirst glance.The added element has a syntactic nature different from the others:it is not an element of the domain of the discourse,but a property related to the formalism used by the language to represent uncertainty and vagueness.The simple concrete syntax we define is as an extension of the EBNF of N-Triples as given in[4].Our extension is given in table1.N-Triples is a line-based,plain text format for encoding an RDF Graph,used for expressing RDF test cases.A statement has the form s p o.,where s,p and o are respectively the subject,the predicate and the object of the statement.Our extended syntax add an optional prefix n:to a statement in N-triple notation, where n is a decimal number representing the fuzzy truth-value of the triple. The use of decimal numbers instead of real numbers is only a limitation of the syntax and does not undermine the discussion.The term triple,used in the EBNF for N-Triple,is replaced with the more generic term statement.Triple and statement are often used in semantic web lit-erature as a synonym,but we prefer to use the latter to avoid confusion between a plain RDF statement(made actually of three parts)and a fuzzy RDF statement (that,although is still a triple semantically,is made up of four elements).The fuzzy value is defined as optional.This way,the syntax is backward-compatible;the intended semantics is that a statement with the form s p o.is equivalent to the statement1:s p o..With such a(syntactic only)default,we could take an inference engine implementing fuzzy RDF,let it parse plain RDF statements,and get the same results of a conventional RDF inference engine. Furthermore,as it would be clear in the description of fuzzy RDF inference rules (section4),even the complexity of the computation would be of the same order.We will not give an abstract syntax,nor a RDF/XML based syntax,as they would not be useful.It can be shown that all“physical“data(i.e.,data transmitted between host or processes)can be encoded using plain RDF reified statements.The extended syntax will be used only in the paper to write down the examples.fuzzyNtripleDoc::=line*line::=ws*(comment|statement)?eolncomment::=‘#’(character−(cr|lf))*statement::=(value ws+)?subject ws+predicate ws+object ws*‘.’ws* value::=1|0.[0–9]+subject::=uriref|nodeIDpredicate::=urirefobject::=uriref|nodeID|literaluriref::=‘<’absoluteURI‘>’nodeID::=‘_:’nameliteral::=langString|datatypeStringlangString::=‘"’string‘"’(‘@’language)?datatypeString::=‘"’string‘"’‘^^’urireflanguage::=[a–z]+(‘-’[a–z0–9]+)*encoding a language tag.ws::=space|tabeoln::=cr|lf|cr lfspace::=#x20/*US-ASCII space-decimal32*/cr::=#xD/*US-ASCII carriage return-decimal13*/lf::=#xA/*US-ASCII line feed-decimal10*/tab::=#x9/*US-ASCII horizontal tab-decimal9*/string::=character*(with escapes as defined in section Strings of[4]) name::=[A-Za-z][A–Za–z0–9]*absoluteURI::=character+(with escapes as defined in section URI Referencesof[4])character::=[#x20–#x7E]/*US-ASCII space to decimal126*/Table1.EBNF for Fuzzy N-Triples2.2Simple interpretationThe RDF Model Theory[3]is based on the concept of extension.An interpre-tation satisfies a triple s p o.if the couple formed by the interpretation of the subject and the interpretation of the object belongs to the extension of the interpretation of the property.In this fuzzy counterpart,a couple subject,object has a membership de-gree to the extension of the predicate,given by the number prepended to the statement.The extension is not an ordinary set of couples anymore,but a fuzzy set of couples.In other words,a fuzzy RDF interpretation satisfies a statement n:s p o.if the membership degree of the couple,formed by the interpreta-tion of the subject and the interpretation of the object,to the extension of the interpretation of the predicate,is greater or equal than n.We have chosen not to make the mapping between vocabulary items and domain fuzzy.Instead,the membership of a resource to the domain is fuzzy. This is a step which poses some theoretical problems,in particular when we have to deal with properties in simple interpretations.In RDF interpretation, the property domain IP is a subset of the resource domain IR,so in fuzzy RDFinterpretations would be enough to make IP a fuzzy subset of IR;in simple interpretations,instead,there is no formal relation between IP and IR,so when the mapping IS from URI references to(IR∪IP)becomes fuzzy we need a further device.The chosen solution is to define a domain IDP for properties, so that IP is a fuzzy subset of IDP,and to modify the definition of IS to a mapping URI references∈V→(IR∪IDP).RDF interpretations does not need IDP,as IP can be shown to be a fuzzy subset of IR.Definition of a simple interpretation A simple fuzzy interpretation I of a vocab-ulary V is defined by:1.A non empty set IR of resources,called the domain or universe of I2.A non empty set IDP,called the property domain of I3.A fuzzy subset IP of IDP,called the set of properties of I4.A fuzzy mapping IEXT:IP→2IR×IR,i.e.the fuzzy set of pairs x,ywith x,y∈IR.5.A mapping IS from URI references∈V→(IR∪IDP)6.A mapping IL from typed literals∈V→IR7.A distinguished subset LV⊆IR,called the set of literal values,which con-tains all the plain literals of VThe belonging of an element to the properties domain is strictly related to the use of such element as a property in a statement.Therefore,we have defined a membership degree to the property domain,intuitively related to the truth value of the statements in which the resource is used as a property.2.3Denotations for ground graphsThe next step is to define the semantic conditions an interpretation must satisfy in order to be a model for a graph.We state the semantic conditions that relate the membership degree of a couple subject,object to an extension and the truth of a fuzzy statement.We will use the abbreviated Zadeh’s notation A(x)=n,instead ofµA(x)=n, to state that the membership degree of the element x to the set A is equal to n[5].Semantic conditions for ground graphs–if E is a plain literal aaa∈V,then I(E)=aaa–if E is a plain literal aaa@ttt∈V,then I(E)= aaa,ttt–if E is a typed literal∈V,then I(E)=IL(E)–if E is a URI reference∈V,then I(E)=IS(E)–if E is a ground triple n:s p o.,then I(E)=true if s,p and o∈V, IP(I(p))≥n and IEXT(I(p))( I(s),I(o) )≥n,otherwise I(E)=false.–if E is a ground RDF graph,than I(E)=false if I(E )=false for some triple E ∈E,otherwise I(E)=trueOnly the condition of truth and falsity of a ground statement in the interpre-tation is affected.The given formulation of the condition has as a consequence that a graph where the same statement appears more than once,with differ-ent membership degrees,is equivalent to a graph where the statement appears only once,with a membership degree equal to the maximum of the membership degrees.Note that whether a statement is a model for a graph or not is not a fuzzy concept;it is either true or false.However,it could be interesting to compute the minimum and maximum membership degree to an extensions a couple must have in an interpretation to be a model of a given graph.This minimum degree has a role similar to the degree of truth of a statement in a knowledge base. 2.4Simple entailmentThe definition of simple interpretation is not affected.A set S of RDF graphs (simply)entails a graph E if every interpretation which satisfies every member of S also satisfies E.Given a graph G and a triple s,p,o ,it could be interesting to compute the minimum and maximum value of n such that G entails n:s p o..Those bounds must be taken in account when we compute the deductive closure of the graph,as it is not unique.Section2of RDF Semantics[3]shows many lemmas that apply to simple interpretations.All of them retain their validity within fuzzy RDF Model Theory, making some adjustments in the proof of some of them.We will show these.The Empty Graph Lemma can be shown using the same proof.The definition of an empty graph is the same as in plain RDF:an empty graph is a graph with no statements at all.It is important to note that an empty graph can not be defined as a graph with no not-zero-valued statements.Statements such as0:s p o., although pretty useless,cannot be ignored,as the semantic requirement that s, p and o must belong to the graph’s vocabulary still applies.Subgraph Lemma,Instance Lemma and Merging Lemma retain both their validity and their proofs with the new semantics.Interpolation Lemma,Anonymity Lemma,Monotonicity Lemma and Com-pactness Lemma make use in their proof of a way of constructing an interpre-tation of a graph using lexical items in the graph itself,the so called Herbrand interpretation[6].To prove the lemmas,we need to construct a similar interpre-tation for a fuzzy graph.The(simple)Herbrand fuzzy interpretation of G,written Herb(G),can be defined as follows.–LV Herb(G)is the set of all plain literals in G;–IR Herb(G)is the set of all names and blank nodes which occur in subject or object position of statements in G;–IDP Herb(G)is the set of URI references which occur in the property position of statements in G;–IP Herb(G)(p)is the maximum of n for all statements in which p occur in property position;–IEXT Herb(G)( s,o )is the maximum n for all the statements n:s p o.in G–IS Herb(G)and IL Herb(G)are both identity mappings on the appropriate parts of the vocabulary of G.Using this definition of Herbrand interpretation instead of that in AppendixA of[3],the proofs for cited lemmas still apply.2.5RDF InterpretationRDF Semantic Conditions–IP(x)=IEXT(I(rdf:type))( x,I(rdf:Property))–If”xxx”∧∧rdf:XMLLiteral∈V and xxx is a well-typed XML literal string, then•IL(”xxx”∧∧rdf:XMLLiteral)is the XML value of xxx;•IL(”xxx”∧∧rdf:XMLLiteral)∈LV;•IEXT(I(rdf:type))( IL(”xxx”∧∧rdf:XMLLiteral),I(rdf:XMLLiteral) )=1–If”xxx”∧∧rdf:XMLLiteral∈V and xxx is an ill-typed XML literal string, then•IL(”xxx”∧∧rdf:XMLLiteral)/∈LV;•IEXT(I(rdf:type))( IL(”xxx”∧∧rdf:XMLLiteral),I(rdf:XMLLiteral) )=0Thefirst RDF semantic condition has the consequence that IP must be a subset of IR.Given such a fact,there is no more need of IDP,as it was for simple interpretation.IP can be directly defined as a fuzzy subset of IR.The second and third conditions equal to see the well-formedness of an XML Literal as crisp truth-valued.We could conceive an external machinery that can be considered completely trustworthy as it classify an XML literal as well-formed or not.RDF axiomatic triples By definition,we give axiomatic triples a unit truth value.Given the(syntactic)convention that a triple s p o.is equivalent to the fuzzy statement1:s p o.,we can take the table of axiomatic triples of RDF in section3.1of[3]and copy it as-is as the table of axiomatic statements of fuzzy RDF.3Fuzzy RDF SchemaThe path from RDF Schema to Fuzzy RDF Schema follows the same guidelines of the previous section.The RDFS semantics is conveniently stated in terms of a new semantic con-struct:the class[3].A class is a resource with a class extension,ICEXT,which represents a set of things in the universe which all have that class as the object of their rdf:type property.Thus,the definition of a class roots in the definition of extension.In fuzzy RDF,extensions are fuzzy set of couples;in fuzzy RDFS,class extensions are fuzzy sets of domain’s elements.3.1RDFS InterpretationA RDFS interpretation define the domains for resources(IR),literals(IL)and literal values(LV)in terms of classes.In fuzzy RDFS they are fuzzy subdomains of IR.We will give RDFS semantic conditions and axiomatic triples,then we will try to explain the more problematic definitions:domains/ranges(section3.2) and subproperties/subclasses(section3.3).RDFS semantic conditions–ICEXT(y)(x)=IEXT(I(rdf:type))( x,y )•IC=ICEXT(I(rdfs:Class))•IR=ICEXT(I(rdfs:Resource))•IL=ICEXT(I(rdfs:Literal))–ICEXT(y)(u)≥min(IEXT(I(rdfs:domain))( x,y ),IEXT(x)( u,v ))–ICEXT(y)(u)≥min(IEXT(I(rdfs:range))( x,y ),IEXT(x)( u,v ))–IEXT(I(rdfs:subPropertyOf))is transitive and reflexive on IP–If IEXT(rdfs:subPropertyOf)( x,y )=n,then IP(x)≥n,IP(y)≥n, min a,b {1−IEXT(x)( a,b )+IEXT(y)( a,b )}≥n–IEXT(I(rdfs:subClassOf))( x,I(rdfs:Resource) )=IC(x)–If IEXT(rdfs:subClassOf)( x,y )=n,then IC(x)≥n,IC(y)≥n, min a{1−IC(x)(a)+IC(y)(a)}≥n.–IEXT(I(rdfs:subClassOf))is transitive and reflexive on IC–IEXT(I(rdfs:subPropertyOf))( x,I(rdfs:member) )=ICEXT(I(rdfs:ContainerMembershipProperty))(x)–ICEXT(I(rdfs:Datatype))(x)=IEXT(I(rdfs:subClassOf))( x,I(rdfs:Literal) )RDFS axiomatic triples As for RDF axiomatic triples,fuzzy RDFS axioms are the same of plain RDFS,from section4.2of RDF Semantics[3].3.2Domains and rangesThe semantic condition on domains looks quite complicated.To explain it,we will proceed by grades.In plain RDF Schema,if x,y ∈IEXT(I(rdfs:domain))and u,v ∈IEXT(x)then u∈ICEXT(y).In fuzzy set theory,let R be a fuzzy relation on X×Y.Then the domain is defined as dom(R)(x)=sup y R(x,y)[7],i.e.the least upper bound of R(x,y) for all y.In fuzzy RDFS,we have to deal both with a fuzzy notion of domain,and with a fuzzy assignment of a domain to a property.Let consider a resource u and a class y.For each property x,we take the mini-mum between IEXT(I(rdfs:domain))( x,y )and IEXT(x)( u,v ).Then,fol-lowing the original RDFS condition,ICEXT(y)(u)must be greater or equal than this value.The previous condition must hold for every property x,so it’s equivalent to state that must be taken the maximum value.The conditions for ranges are analogous.3.3Subproperties and subclassesSubproperties and subclasses are fully analogous concepts.The set inclusion is between extensions for the former,between class extensions of the latter.To define the semantics of subClassOf and subPropertyOf,we need a rela-tion of set inclusion between fuzzy sets that takes into account also the degree of the relation of inclusion itself.This relation must be transitive and reflexive.Zadeh’s definition of fuzzy subset[8]3(A⊆B⇐⇒∀x∈X A(x)≤B(x)) is transitive and reflexive,but is not a fuzzy relation:either the set A is a subset of B,or not.What we need is instead a weaker fuzzy subset relation;a relation that reduces to the Zadeh’s one when the subclass/subproperty relation has a unit truth value.It must also maintain the reflexivity and transitivity properties.Dubois and Prade[7]define weak inclusion αasA αB⇐⇒x∈(A∪B)α∀x∈X,whereαis a parameter and(·)αis theα-cut4.This relation is transitive only forα>12.Other definitions of weak inclusion make use of inclusion grades.An inclusion grade I(A,B)is a scalar measure of the inclusion of the set A in the set B. In general,A⊆αB iffI(A,B)≥α,where⊆αdenote a weak inclusion with inclusion gradeα.We have chosen to use the inclusion grade defined as[7]:3Again,we use the abbreviation A(x)for the membership functionµA (x).4Theα-cut Aαof A is the set of all elements with a membership value to A greater thanα,withα∈(0,1]Aα={x|A(x)≥α}I(A,B)=inf x∈X x)where inf is the infimum and|−|is the bounded difference5.When A⊆B,I(A,B)=1[7].This inclusion grade could also be written as I(A,B)=inf x∈X(1−max(0,A(x)−B(x)))=inf x∈X min(1,(1−A+B)).Furthermore,let’s suppose that there is at least an x such that A(x)>B(x). Then I(A,B)could be written as inf x∈X(1−A+B).The semantic condition requires such measure to be greater than or equal to n,where n is the truth value of the statement.In this case the semantic condition reduces toinf x∈X(1−A+B)≥n.It could be interesting to ask how much this definition differs from the con-dition for classical fuzzy subsets,A(x)≤B(x).If A⊆B,then I(A,B)=1,so the semantic condition holds for any n∈[0,1].Let’s call d(x)the difference d(x)=A(x)−B(x),so that1−A+B=1−d.We suppose that there is at least an x such that A(x)>B(x),so d(x)has at least a positive value.The semantic condition could then be written inf x∈X(1−d(x))≥n.The maximum positive value of the difference d equal to1−n.As n is the truth value of the statement that asserts the relation of subprop-erty or subclass,and1−n represent the lack of truth of the same statement,we can conclude that the maximum allowable positive difference between A(x)andB(x)is equal to the lack of truth on the subproperty or subclass relation.4Fuzzy RDF entailment rulesRDF Model Theory’s entailment rules[3]are all of the same form:add a state-ment to a graph when it contains triples conforming to a pattern.Each rule has only one or two antecedent statements and derive only one new inferred statement;either P R or P,Q R.Given the way fuzzy RDF semantics is defined,the corresponding inference rules for fuzzy RDF are analogous;only the fuzzy truth values of inferred state-ments must be computed.The simplest possible choice that respect the semantics is:–With rules as P Q,having only one antecedent,the truth value of the consequent Q is taken to be the same of the antecedent P.–With rules as P,Q R,the truth value of R is the minimum between the truth values of P and Q.The inference rules for RDF/RDFS are shown in table2.They were derived from the rules used by the Sesame[10]forward-chaining inferencer.5∀x∈X,(A|−|B)(x)=max(0,A(x)−B(x))[9]Sesame is a generic architecture for storing and querying RDF and RDF Schema.It makes use of a forward-chaining inferencer to compute and store the closure of its knowledge base whenever a transaction adds data to the reposi-tory[11].Sesame applies RDF-MT inference rules in a optimized way,making use of the dependencies between them to eliminate most redundant inferencing steps.To obtain a fuzzy RDF storage and inference tool it is only a matter of modify Sesame RDF-MT inferencer,making it compute the correct truth values for inferred statements,and to extend the underlying storage to make room for a truth value(i.e.,a number)for each statement.This shows how a simple proof-of-concept fuzzy RDF inferencer is easy to implement.The starting point is the code base of an inference engine that im-plements the RDF model theory.It can be shown that an inference engine implementing such rules is correct: all its rules are valid,in the sense that a graph entails any larger graph that is obtained by applying the rules to the original graph.There is no formal proof that it is also complete,but there is not such a proof for plain RDF Model Theory inference rules either.References1.Hendler,J.,Lassila,O.,Berners-Lee,T.:The semantic web.Scientific American(2001)28–372.Berners-Lee,T.:What the semantic web can represent.W3C design issues,WorldWide Web Consortium(September1998)3.Hayes,P.:RDF Semantics.W3C recommendation,World Wide Web Consortium(10February2004)4.Grant,J.,Beckett,D.:RDF test cases.W3C recommendation,World Wide WebConsortium(2004)/TR/rdf-testcases/.5.Zadeh,L.A.:A fuzzy set theoretic interpretation of linguistic hedges.Journal ofCybernetics2(1972)4–346.Goldfarb,W.D.,ed.:Logical Writings of Jacques Herbrand.Harvard UniversityPress,Cambridge(1971)7.Dubuois,D.,Prade,H.:Fuzzy sets and Systems.Academic Press,New York,NJ(1980)8.Zadeh,L.A.:Fuzzy rmation and Control(1965)338–3539.Zadeh,L.A.:Calculus of Fuzzy Restrictions.In:Fuzzy Sets and Their Applicationsto Cognitive and Decision Processes.Academic Press,New York(1975)1–39 10.Broekstra,J.,Kampman,A.,van Harmelen,F.:Sesame:A generic architecturefor storing and quering RDF and RDF Schema.In Horrocks,I.,Handler,J.,eds.: Proceedings of thefirst International Semantic Web Conference(ISWC2002), Sardinia,Italy(2002)54–6811.Broekstra,J.,Kampman,A.:Inferencing and truth maintenance in RDF Schema:exploring a naive practical approach.In:Workshop on Practical and Scalable Semantic Systems(PSSS)2003.Second International Semantic Web Conference (ISWC),Sanibel Island,Florida,USA(2003)#antecedents consequent1iii:xxx aaa yyy iii:aaa rdf:type rdf:Property2.1iii:xxx aaa yyy kkk:xxx rdf:type zzzjjj:aaa rdfs:domain zzz where kkk=min(iii,jjj)2.2iii:aaa rdfs:domain zzz kkk:xxx rdf:type zzzjjj:xxx aaa yyy where kkk=min(iii,jjj)3.1iii:xxx aaa uuu kkk:uuu rdf:type zzzjjj:aaa rdfs:range zzz where kkk=min(iii,jjj)3.2iii:aaa rdfs:range zzz kkk:uuu rdf:type zzzjjj:xxx aaa uuu where kkk=min(iii,jjj)4a iii:xxx aaa yyy jjj:xxx rdf:type rdfs:Resource4b iii:xxx aaa uuu iii:uuu rdf:type rdfs:Resource5a.1iii:aaa rdfs:subPropertyOf bbb kkk:aaa rdfs:subPropertyOf cccjjj:bbb rdfs:subPropertyOf ccc where kkk=min(iii,jjj)5a.2iii:bbb rdfs:subPropertyOf ccc kkk:aaa rdfs:subPropertyOf cccjjj:aaa rdfs:subPropertyOf bbb where kkk=min(iii,jjj)5b iii:xxx rdf:type rdf:Property iii:xxx rdfs:subPropertyOf xxxreflexivity of rdfs:subPropertyOf6.1iii:xxx aaa yyy kkk:xxx bbb yyyjjj:aaa rdfs:subPropertyOf bbb where kkk=min(iii,jjj)6.2iii:aaa rdfs:subPropertyOf bbb kkk:xxx bbb yyyjjj:xxx aaa yyy where kkk=min(iii,jjj)7a iii:xxx rdf:type rdfs:Class iii:xxx rdfs:subClassOf rdfs:Resource7b iii:xxx rdf:type rdfs:Class iii:xxx rdfs:subClassOf xxxreflexivity of rdfs:subClassOf8.1iii:xxx rdfs:subClassOf yyy kkk:xxx rdfs:subClassOf zzzjjj:yyy rdfs:subClassOf zzz where kkk=min(iii,jjj)8.2iii:yyy rdfs:subClassOf zzz kkk:xxx rdfs:subClassOf zzzjjj:xxx rdfs:subClassOf yyy where kkk=min(iii,jjj)9.1iii:xxx rdfs:subClassOf yyy kkk:aaa rdf:type yyyjjj:aaa rdf:type xxx where kkk=min(iii,jjj)9.2iii:aaa rdf:type xxx kkk:aaa rdf:type yyyjjj:xxx rdfs:subClassOf yyy where kkk=min(iii,jjj)10iii:xxx rdf:type iii:xxx rdfs:subPropertyOf rdfs:member rdfs:ContainerMembershipProperty11iii:xxx rdf:type rdfs:Datatype jjj:xxx rdfs:subClassOf rdfs:LiteralX1iii:xxx rdf:_*yyy jjj:rdf:_*rdf:type rdfs:ContainerMembershipPropertyThis is an extra rule for list membershipproperties(_1,_2,_3,...).The RDF MTdoes not specify a production for this.Table2.Fuzzy RDF inference rules。