Rapid and sensitive detection of CpG-methylation using methyl-binding (MB)-PCR
利用相关比相似性测度多分辨率配准MR和PET医学图像的方法
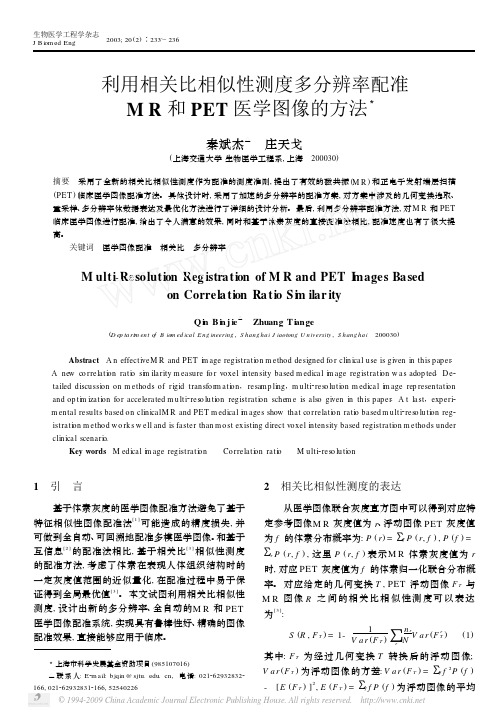
利用相关比相似性测度多分辨率配准M R和PET医学图像的方法3秦斌杰△ 庄天戈(上海交通大学生物医学工程系,上海 200030)摘要 采用了全新的相关比相似性测度作为配准的测度准则,提出了有效的磁共振(M R)和正电子发射端层扫描(PET)临床医学图像配准方法。
具体设计时,采用了加速的多分辨率的配准方案,对方案中涉及的几何变换选取、重采样、多分辨率体数据表达及最优化方法进行了详细的设计分析。
最后,利用多分辨率配准方法,对M R和PET 临床医学图像进行配准,给出了令人满意的效果,同时和基于体素灰度的直接配准法相比,配准速度也有了很大提高。
关键词 医学图像配准 相关比 多分辨率M ulti-Resolution Reg istra tion of M R and PET I mages Ba sedon Correla tion Ra tio Si m ilar ityQi n B i n j ie△ Zhuang T i ange(D ep ta rt m en t of B io m ed ica l E ng ineering,S hang ha i J iaotong U n iversity,S hang ha i 200030) Abstract A n effectiveM R and PET i m age registrati on m ethod designed fo r clinical use is given in th is paper.A new co rrelati on rati o si m ilarity m easure fo r voxel intensity based m edical i m age registrati on w as adop ted.D e2 tailed discussi on on m ethods of rigid transfo r m ati on,resamp ling,m ulti2reso luti on m edical i m age rep resentati on and op ti m izati on fo r accelerated m ulti2reso luti on registrati on schem e is also given in th is paper.A t last,experi2 m ental results based on clinicalM R and PET m edical i m ages show that co rrelati on rati o based m ulti2reso luti on reg2 istrati on m ethod w o rk s w ell and is faster than mo st existing direct voxel intensity based registrati on m ethods under clinical scenari o.Key words M edical i m age registrati on Co rrelati on rati o M ulti2reso luti on1 引 言基于体素灰度的医学图像配准方法避免了基于特征相似性图像配准法[1]可能造成的精度损失,并可做到全自动、可回溯地配准多模医学图像。
072 食品中甲醛的检测方法

methylated2CpGislandsincancer[J].LabInvest,2005,85(9):117221180.[14] GEBHARD C,SCHWARZFISCHERL,PHAMTH,etal.RapidandsensitivedetectionofCpG2methylationusingmethyl2binding(MB)2PCR[J].NucleicAcidsRes,2006,34(11):e82.[15] HAN W,CAUCHIS,HERMANJG,etal.DNAmethylationmappingbytag2modifiedbisulfitegenomicsequencing[J].AnalBiochem,2006,355(1):50261. [16] RAMSAHOYE BH.MeasurementofgenomewideDNAmethylationbyreversed2phasehigh2performanceliquidchromatography[J].Methods,2002,27(2):1562161. [17] FRAGAMF,URIOLE,BORJADIEGOL,etal.High2performancecapillaryelectrophoreticmethodforthequantificationof52methyl2’2deoxycytidineingenomicDNA:applicationtoplant,animalandhumancancertissues[J].Electrophoresis,2002,23(11):167721681. [18] YANG I,PARKIY,JANGSM,etal.RapidquantificationofDNAmethylationthroughdNMPanalysisfollowingbisulfite2PCR[J].NucleicAcidsRes,2006,34(8):e61.[19] WITTWERCT,REEDGH,GUNDRYCN,etal.High2resolutiongenotypingbyampliconmeltinganalysisusingLCGreen[J].ClinChem,2003,49(6Pt1):8532860. [20] WOJDACZT,KDOBROVICA.Methylation2sensitivehighresolutionmelting(MS2HRM):anewapproachforsensitiveandhigh2throughputassessmentofmethylation[J].NucleicAcidsRes,2007,35(6):e41.[21] HAMADA T,MURASAWAS,ASAHARAT.Simplescreeningmethodfordifferentiallymethylatedregionsofthegenomeusingasmallnumberofcells[J].BiochemBiophysResCommun,2007,353(2):2752279.072 食品中甲醛的检测方法韩宏伟综述(中国疾病预防控制中心营养与食品安全所,北京 100021)摘要: 测定甲醛没有固定的标准方法,应根据其可能的含量或浓度范围、潜在的干扰因素、检测方法的灵敏度、样品处理方式和现有的仪器设备条件,选择合适的分析方法。
基于深度学习和PnP模型的激光跟踪仪自动姿态测量
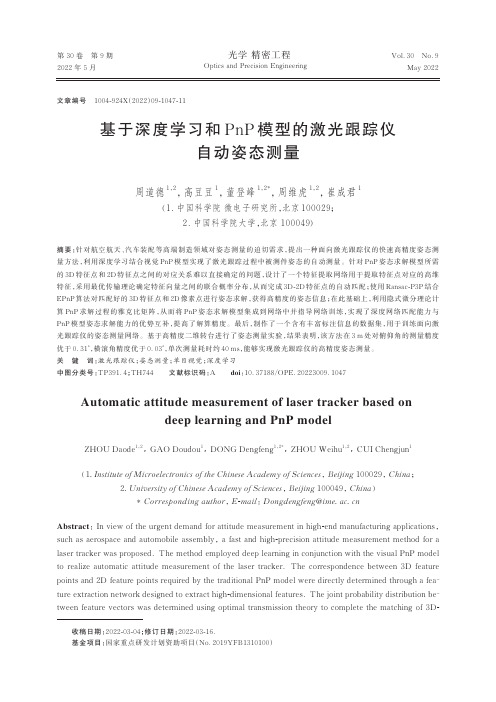
第30卷第9期2022年5月Vol.30No.9May2022光学精密工程Optics and Precision Engineering基于深度学习和PnP模型的激光跟踪仪自动姿态测量周道德1,2,高豆豆1,董登峰1,2*,周维虎1,2,崔成君1(1.中国科学院微电子研究所,北京100029;2.中国科学院大学,北京100049)摘要:针对航空航天、汽车装配等高端制造领域对姿态测量的迫切需求,提出一种面向激光跟踪仪的快速高精度姿态测量方法,利用深度学习结合视觉PnP模型实现了激光跟踪过程中被测件姿态的自动测量。
针对PnP姿态求解模型所需的3D特征点和2D特征点之间的对应关系难以直接确定的问题,设计了一个特征提取网络用于提取特征点对应的高维特征,采用最优传输理论确定特征向量之间的联合概率分布,从而完成3D-2D特征点的自动匹配;使用Ransac-P3P结合EPnP算法对匹配好的3D特征点和2D像素点进行姿态求解,获得高精度的姿态信息;在此基础上,利用隐式微分理论计算PnP求解过程的雅克比矩阵,从而将PnP姿态求解模型集成到网络中并指导网络训练,实现了深度网络匹配能力与PnP模型姿态求解能力的优势互补,提高了解算精度。
最后,制作了一个含有丰富标注信息的数据集,用于训练面向激光跟踪仪的姿态测量网络。
基于高精度二维转台进行了姿态测量实验,结果表明,该方法在3m处对俯仰角的测量精度优于0.31°,横滚角精度优于0.03°,单次测量耗时约40ms,能够实现激光跟踪仪的高精度姿态测量。
关键词:激光跟踪仪;姿态测量;单目视觉;深度学习中图分类号:TP391.4;TH744文献标识码:A doi:10.37188/OPE.20223009.1047Automatic attitude measurement of laser tracker based ondeep learning and PnP modelZHOU Daode1,2,GAO Doudou1,DONG Dengfeng1,2*,ZHOU Weihu1,2,CUI Chengjun1(1.Institute of Microelectronics of the Chinese Academy of Sciences,Beijing100029,China;2.University of Chinese Academy of Sciences,Beijing100049,China)*Corresponding author,E-mail:Dongdengfeng@Abstract:In view of the urgent demand for attitude measurement in high-end manufacturing applications,such as aerospace and automobile assembly,a fast and high-precision attitude measurement method for a laser tracker was proposed.The method employed deep learning in conjunction with the visual PnP model to realize automatic attitude measurement of the laser tracker.The correspondence between3D feature points and2D feature points required by the traditional PnP model were directly determined through a fea⁃ture extraction network designed to extract high-dimensional features.The joint probability distribution be⁃tween feature vectors was determined using optimal transmission theory to complete the matching of3D-文章编号1004-924X(2022)09-1047-11收稿日期:2022-03-04;修订日期:2022-03-16.基金项目:国家重点研发计划资助项目(No.2019YFB1310100)第30卷光学精密工程2D feature points.Subsequently,Ransac-P3P combined with EPnP algorithm was used to obtain high-pre⁃cision attitude information;Based on this,the Jacobian matrix of PnP solution process was calculated us⁃ing implicit differential theory,and the PnP attitude solution model was integrated into the network to guide the training of the network.The complementary advantages of strong depth network matching abili⁃ty and high attitude solution accuracy of the PnP model improved the solution accuracy of the network.In addition,a dataset with rich annotation information was used to train the attitude measurement network for the laser tracker.Finally,an attitude measurement test was conducted using a high-precision two-dimen⁃sional turntable.The experimental results show that the calculation error of pitch angle is less than0.31°,the rolling angle error is less than0.03°,and the single measurement takes approximately40ms.The pro⁃posed method can potentially be applied to attitude measurement scene of the laser tracker.Key words:laser tracker;attitude measurement;monocular vision;deep learning1引言随着制造业的快速发展,在航空航天、汽车装配等领域,大尺寸高精度姿态测量技术越来越重要。
!How Far are We from Solving Pedestrian Detection
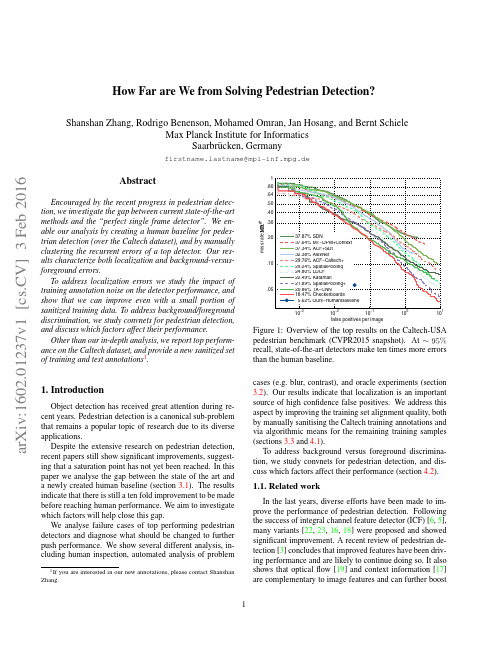
from Solving Pedestrian Detection?Mohamed Omran,Jan Hosang,and Bernt SchielePlanck Institute for Informatics Saarbrücken,Germanystname@mpi-inf.mpg.deAbstractEncouraged by the recent progress in pedestrian detec-tion,we investigate the gap between current state-of-the-art methods and the “perfect single frame detector”.We en-able our analysis by creating a human baseline for pedes-trian detection (over the Caltech dataset),and by manually clustering the recurrent errors of a top detector.Our res-ults characterize both localization and background-versus-foreground errors.To address localization errors we study the impact of training annotation noise on the detector performance,and show that we can improve even with a small portion of sanitized training data.To address background/foreground discrimination,we study convnets for pedestrian detection,and discuss which factors affect their performance.Other than our in-depth analysis,we report top perform-ance on the Caltech dataset,and provide a new sanitized set of training and test annotations 1.1.IntroductionObject detection has received great attention during re-cent years.Pedestrian detection is a canonical sub-problem that remains a popular topic of research due to its diverse applications.Despite the extensive research on pedestrian detection,recent papers still show significant improvements,suggest-ing that a saturation point has not yet been reached.In this paper we analyse the gap between the state of the art and a newly created human baseline (section 3.1).The results indicate that there is still a ten fold improvement to be made before reaching human performance.We aim to investigate which factors will help close this gap.We analyse failure cases of top performing pedestrian detectors and diagnose what should be changed to further push performance.We show several different analysis,in-cluding human inspection,automated analysis of problem1Ifyou are interested in our new annotations,please contact Shanshan Zhang.1010101010Figure 1:Overview of the top results on the Caltech-USA pedestrian benchmark (CVPR2015snapshot).At ∼95%recall,state-of-the-art detectors make ten times more errors than the human baseline.cases (e.g.blur,contrast),and oracle experiments (section 3.2).Our results indicate that localization is an important source of high confidence false positives.We address this aspect by improving the training set alignment quality,both by manually sanitising the Caltech training annotations and via algorithmic means for the remaining training samples (sections 3.3and 4.1).To address background versus foreground discrimina-tion,we study convnets for pedestrian detection,and dis-cuss which factors affect their performance (section 4.2).1.1.Related workIn the last years,diverse efforts have been made to im-prove the performance of pedestrian detection.Following the success of integral channel feature detector (ICF)[6,5],many variants [22,23,16,18]were proposed and showed significant improvement.A recent review of pedestrian de-tection [3]concludes that improved features have been driv-ing performance and are likely to continue doing so.It also shows that optical flow [19]and context information [17]are complementary to image features and can further boost 1a r X i v :1602.01237v 1 [c s .C V ] 3 F eb 2016detection accuracy.Byfine-tuning a model pre-trained on external data convolution neural networks(convnets)have also reached state-of-the-art performance[15,20].Most of the recent papers focus on introducing novelty and better results,but neglect the analysis of the resulting system.Some analysis work can be found for general ob-ject detection[1,14];in contrast,in thefield of pedestrian detection,this kind of analysis is rarely done.In2008,[21] provided a failure analysis on the INRIA dataset,which is relatively small.The best method considered in the2012 Caltech dataset survey[7]had10×more false positives at20%recall than the methods considered here,and no method had reached the95%mark.Since pedestrian detection has improved significantly in recent years,a deeper and more comprehensive analysis based on state-of-the-art detectors is valuable to provide better understanding as to where future efforts would best be invested.1.2.ContributionsOur key contributions are as follows:(a)We provide a detailed analysis of a state-of-the-art ped-estrian detection system,providing insights into failure cases.(b)We provide a human baseline for the Caltech Pedestrian Benchmark;as well as a sanitised version of the annotations to serve as new,high quality ground truth for the training and test sets of the benchmark.The data will be public. (c)We analyse how much the quality of training data affects the detector.More specifically we quantify how much bet-ter alignment and fewer annotation mistakes can improve performance.(d)Using the insights of the analysis,we explore variants of top performing methods:filtered channel feature detector [23]and R-CNN detector[13,15],and show improvements over the baselines.2.PreliminariesBefore delving into our analysis,let us describe the data-sets in use,their metrics,and our baseline detector.2.1.Caltech-USA pedestrian detection benchmarkAmongst existing pedestrian datasets[4,9,8],KITTI [11]and Caltech-USA are currently the most popular ones. In this work we focus on the Caltech-USA benchmark[7] which consists of2.5hours of30Hz video recorded from a vehicle traversing the streets of Los Angeles,USA.The video annotations amount to a total of350000bound-ing boxes covering∼2300unique pedestrians.Detec-tion methods are evaluated on a test set consisting of4024 frames.The provided evaluation toolbox generates plotsFilter type MR O−2ACF[5]44.2SCF[3]34.8LDCF[16]24.8RotatedFilters19.2Checkerboards18.5Table1:Thefiltertype determines theICF methods quality.Base detector MR O−2+Context+FlowOrig.2Ped[17]48~5pp/Orig.SDt[19]45/8ppSCF[3]355pp4ppCheckerboards19~01ppTable2:Detection quality gain ofadding context[17]and opticalflow[19],as function of the base detector.for different subsets of the test set based on annotation size, occlusion level and aspect ratio.The established proced-ure for training is to use every30th video frame which res-ults in a total of4250frames with∼1600pedestrian cut-outs.More recently,methods which can leverage more data for training have resorted to afiner sampling of the videos [16,23],yielding up to10×as much data for training than the standard“1×”setting.MR O,MR N In the standard Caltech evaluation[7]the miss rate(MR)is averaged over the low precision range of [10−2,100]FPPI.This metric does not reflect well improve-ments in localization errors(lowest FPPI range).Aiming for a more complete evaluation,we extend the evaluation FPPI range from traditional[10−2,100]to[10−4,100],we denote these MR O−2and MR O−4.O stands for“original an-notations”.In section3.3we introduce new annotations, and mark evaluations done there as MR N−2and MR N−4.We expect the MR−4metric to become more important as de-tectors get stronger.2.2.Filtered channel features detectorFor the analysis in this paper we consider all methods published on the Caltech Pedestrian benchmark,up to the last major conference(CVPR2015).As shown infigure1, the best method at the time is Checkerboards,and most of the top performing methods are of its same family.The Checkerboards detector[23]is a generalization of the Integral Channels Feature detector(ICF)[6],which filters the HOG+LUV feature channels before feeding them into a boosted decision forest.We compare the performance of several detectors from the ICF family in table1,where we can see a big improve-ment from44.2%to18.5%MR O−2by introducingfilters over the feature channels and optimizing thefilter bank.Current top performing convnets methods[15,20]are sensitive to the underlying detection proposals,thus wefirst focus on the proposals by optimizing thefiltered channel feature detectors(more on convnets in section4.2). Rotatedfilters For the experiments involving train-ing new models(in section 4.1)we use our own re-implementation of Checkerboards[23],based on the LDCF[16]codebase.To improve the training time we decrease the number offilters from61in the originalCheckerboards down to9filters.Our so-called Rota-tedFilters are a simplified version of LDCF,applied at three different scales(in the same spirit as Squares-ChnFtrs(SCF)[3]).More details on thefilters are given in the supplementary material.As shown in table1,Ro-tatedFilters are significantly better than the original LDCF,and only1pp(percent point)worse than Checker-boards,yet run6×faster at train and test time. Additional cues The review[3]showed that context and opticalflow information can help improve detections. However,as the detector quality improves(table1)the re-turns obtained from these additional cues erodes(table2). Without re-engineering such cues,gains in detection must come from the core detector.3.Analysing the state of the artIn this section we estimate a lower bound on the re-maining progress available,analyse the mistakes of current pedestrian detectors,and propose new annotations to better measure future progress.3.1.Are we reaching saturation?Progress on pedestrian detection has been showing no sign of slowing in recent years[23,20,3],despite recent im-pressive gains in performance.How much progress can still be expected on current benchmarks?To answer this ques-tion,we propose to use a human baseline as lower bound. We asked domain experts to manually“detect”pedestrians in the Caltech-USA test set;machine detection algorithms should be able to at least reach human performance and, eventually,superhuman performance.Human baseline protocol To ensure a fair comparison with existing detectors,we focus on the single frame mon-ocular detection setting.Frames are presented to annotators in random order,and without access to surrounding frames from the source videos.Annotators have to rely on pedes-trian appearance and single-frame context rather than(long-term)motion cues.The Caltech benchmark normalizes the aspect ratio of all detection boxes[7].Thus our human annotations are done by drawing a line from the top of the head to the point between both feet.A bounding box is then automatically generated such that its centre coincides with the centre point of the manually-drawn axis,see illustration infigure2.This procedure ensures the box is well centred on the subject (which is hard to achieve when marking a bounding box).To check for consistency among the two annotators,we produced duplicate annotations for a subset of the test im-ages(∼10%),and evaluated these separately.With a Intersection over Union(IoU)≥0.5matching criterion, the results were identical up to a single boundingbox.Figure2:Illustration of bounding box generation for human baseline.The annotator only needs to draw a line from the top of the head to the central point between both feet,a tight bounding box is then automatically generated. Conclusion Infigure3,we compare our human baseline with other top performing methods on different subsets of the test data(varying height ranges and occlu-sion levels).Wefind that the human baseline widely out-performs state-of-the-art detectors in all settings2,indicat-ing that there is still room for improvement for automatic methods.3.2.Failure analysisSince there is room to grow for existing detectors,one might want to know:when do they fail?In this section we analyse detection mistakes of Checkerboards,which obtains top performance on most subsets of the test set(see figure3).Since most top methods offigure1are of the ICF family,we expect a similar behaviour for them too.Meth-ods using convnets with proposals based on ICF detectors will also be affected.3.2.1Error sourcesThere are two types of errors a detector can do:false pos-itives(detections on background or poorly localized detec-tions)and false negatives(low-scoring or missing pedes-trian detections).In this analysis,we look into false positive and false negative detections at0.1false positives per im-age(FPPI,1false positive every10images),and manually cluster them(one to one mapping)into visually distinctive groups.A total of402false positive and148false negative detections(missing recall)are categorized by error type. False positives After inspection,we end up having all false positives clustered in eleven categories,shown infig-ure4a.These categories fall into three groups:localization, background,and annotation errors.Background errors are the most common ones,mainly ver-tical structures(e.g.figure5b),tree leaves,and traffic lights. This indicates that the detectors need to be extended with a better vertical context,providing visibility over larger struc-tures and a rough height estimate.Localization errors are dominated by double detections2Except for IoU≥0.8.This is due to issues with the ground truth, discussed in section3.3.Reasonable (IoU >= 0.5)Height > 80Height in [50,80]Height in [30,50]020406080100HumanBaselineCheckerboards RotatedFiltersm i s s r a t eFigure 3:Detection quality (log-average miss rate)for different test set subsets.Each group shows the human baseline,the Checkerboards [23]and RotatedFilters detectors,as well as the next top three (unspecified)methods (different for each setting).The corresponding curves are provided in the supplementary material.(high scoring detections covering the same pedestrian,e.g.figure 5a ).This indicates that improved detectors need to have more localized responses (peakier score maps)and/or a different non-maxima suppression strategy.In sections 3.3and 4.1we explore how to improve the detector localiz-ation.The annotation errors are mainly missing ignore regions,and a few missing person annotations.In section 3.3we revisit the Caltech annotations.False negatives Our clustering results in figure 4b show the well known difficulty of detecting small and oc-cluded objects.We hypothesise that low scoring side-view persons and cyclists may be due to a dataset bias,i.e.these cases are under-represented in the training set (most per-sons are non-cyclist walking on the side-walk,parallel to the car).Augmenting the training set with external images for these cases might be an effective strategy.To understand better the issue with small pedestrians,we measure size,blur,and contrast for each (true or false)de-tection.We observed that small persons are commonly sat-urated (over or under exposed)and blurry,and thus hypo-thesised that this might be an underlying factor for weak detection (other than simply having fewer pixels to make the decision).Our results indicate however that this is not the case.As figure 4c illustrates,there seems to be no cor-relation between low detection score and low contrast.This also holds for the blur case,detailed plots are in the sup-plementary material.We conclude that the small number of pixels is the true source of difficulty.Improving small objects detection thus need to rely on making proper use of all pixels available,both inside the window and in the surrounding context,as well as across time.Conclusion Our analysis shows that false positive er-rors have well defined sources that can be specifically tar-geted with the strategies suggested above.A fraction of the false negatives are also addressable,albeit the small and oc-cluded pedestrians remain a (hard and)significant problem.20406080100120# e r r o r s 0100200300loc a liz a tion ba c k g round a nnota e rrors#e r r o r s (a)False positive sources15304560# e r r o r s (b)False negative sources(c)Contrast versus detection scoreFigure 4:Errors analysis of Checkerboards [23]on the test set.(a)double detectionFigure 5:Example of analysed false positive cases (red box).Additional ones in supplementary material.3.2.2Oracle test casesThe analysis of section 3.2.1focused on errors counts.For area-under-the-curve metrics,such astheones used in Caltech,high-scoring errors matter more than low-scoring ones.In this section we directly measure the impact of loc-alization and background-vs-foreground errors on the de-tection quality metric (log-average miss-rate)by using or-acle test cases.In the oracle case for localization,all false positives that overlap with ground truth are ignored for evaluation.In the oracle tests for background-vs-foreground,all false posit-ives that do not overlap with ground truth are ignored.Figure 6a shows that fixing localization mistakes im-proves performance in the low FPPI region;while fixing background mistakes improves results in the high FPPI re-gion.Fixing both types of mistakes results zero errors,even though this is not immediately visible due to the double log plot.In figure 6b we show the gains to be obtained in MR O −4terms by fixing localization or background issues.When comparing the eight top performing methods we find that most methods would boost performance significantly by fix-ing either problem.Note that due to the log-log nature of the numbers,the sum of localization and background deltas do not add up to the total miss-rate.Conclusion For most top performing methods localiz-ation and background-vs-foreground errors have equal im-pact on the detection quality.They are equally important.3.3.Improved Caltech-USA annotationsWhen evaluating our human baseline (and other meth-ods)with a strict IoU ≥0.8we notice in figure 3that the performance drops.The original annotation protocol is based on interpolating sparse annotations across multiple frames [7],and these sparse annotations are not necessar-ily located on the evaluated frames.After close inspection we notice that this interpolation generates a systematic off-set in the annotations.Humans walk with a natural up and down oscillation that is not modelled by the linear interpol-ation used,thus in most frames have shifted bounding box annotations.This effect is not noticeable when using the forgiving IoU ≥0.5,however such noise in the annotations is a hurdle when aiming to improve object localization.1010−210−110010false positives per image18.47(33.20)% Checkerboards15.94(25.49)% Checkerboards (localization oracle)11.92(26.17)% Checkerboards (background oracle)(a)Original and two oracle curves for Checkerboards de-tector.Legend indicates MR O −2 MR O −4 .(b)Comparison of miss-rate gain (∆MR O −4)for top performing methods.Figure 6:Oracle cases evaluation over Caltech test set.Both localization and background-versus-foreground show important room for improvement.(a)False annotations (b)Poor alignmentFigure 7:Examples of errors in original annotations.New annotations in green,original ones in red.This localization issues together with the annotation er-rors detected in section 3.2.1motivated us to create a new set of improved annotations for the Caltech pedestrians dataset.Our aim is two fold;on one side we want to provide a more accurate evaluation of the state of the art,in particu-lar an evaluation suitable to close the “last 20%”of the prob-lem.On the other side,we want to have training annotations and evaluate how much improved annotations lead to better detections.We evaluate this second aspect in section 4.1.New annotation protocol Our human baseline focused on a fair comparison with single frame methods.Our new annotations are done both on the test and training 1×set,and focus on high quality.The annotators are allowed to look at the full video to decide if a person is present or not,they are request to mark ignore regions in areas cov-ering crowds,human shapes that are not persons (posters,statues,etc.),and in areas that could not be decided as cer-tainly not containing a person.Each person annotation is done by drawing a line from the top of the head to the point between both feet,the same as human baseline.The annot-ators must hallucinate head and feet if these are not visible. When the person is not fully visible,they must also annotate a rectangle around the largest visible region.This allows to estimate the occlusion level in a similar fashion as the ori-ginal Caltech annotations.The new annotations do share some bounding boxes with the human baseline(when no correction was needed),thus the human baseline cannot be used to do analysis across different IoU thresholds over the new test set.In summary,our new annotations differ from the human baseline in the following aspects:both training and test sets are annotated,ignore regions and occlusions are also an-notated,full video data is used for decision,and multiple revisions of the same image are allowed.After creating a full independent set of annotations,we con-solidated the new annotations by cross-validating with the old annotations.Any correct old annotation not accounted for in the new set,was added too.Our new annotations correct several types of errors in the existing annotations,such as misalignments(figure 7b),missing annotations(false negatives),false annotations (false positives,figure7a),and the inconsistent use of“ig-nore”regions.Our new annotations will be publicly avail-able.Additional examples of“original versus new annota-tions”provided in the supplementary material,as well as visualization software to inspect them frame by frame. Better alignment In table3we show quantitative evid-ence that our new annotations are at least more precisely localized than the original ones.We summarize the align-ment quality of a detector via the median IoU between true positive detections and a give set of annotations.When evaluating with the original annotations(“median IoU O”column in table3),only the model trained with original annotations has good localization.However,when evalu-ating with the new annotations(“median IoU N”column) both the model trained on INRIA data,and on the new an-notations reach high localization accuracy.This indicates that our new annotations are indeed better aligned,just as INRIA annotations are better aligned than Caltech.Detailed IoU curves for multiple detectors are provided in the supplementary material.Section4.1describes the RotatedFilters-New10×entry.4.Improving the state of the artIn this section we leverage the insights of the analysis, to improve localization and background-versus-foreground discrimination of our baseline detector.DetectorTrainingdataMedianIoU OMedianIoU N Roerei[2]INRIA0.760.84RotatedFilters Orig.10×0.800.77RotatedFilters New10×0.760.85 Table3:Median IoU of true positives for detectors trained on different data,evaluated on original and new Caltech test.Models trained on INRIA align well with our new an-notations,confirming that they are more precise than previ-ous ones.Curves for other detectors in the supplement.Detector Anno.variant MR O−2MR N−2ACFOriginal36.9040.97Pruned36.4135.62New41.2934.33 RotatedFiltersOriginal28.6333.03Pruned23.8725.91New31.6525.74 Table4:Effects of different training annotations on detec-tion quality on validation set(1×training set).Italic num-bers have matching training and test sets.Both detectors im-prove on the original annotations,when using the“pruned”variant(see§4.1).4.1.Impact of training annotationsWith new annotations at hand we want to understand what is the impact of annotation quality on detection qual-ity.We will train ACF[5]and RotatedFilters mod-els(introduced in section2.2)using different training sets and evaluate on both original and new annotations(i.e. MR O−2,MR O−4and MR N−2,MR N−4).Note that both detect-ors are trained via boosting and thus inherently sensitive to annotation noise.Pruning benefits Table4shows results when training with original,new and pruned annotations(using a5/6+1/6 training and validation split of the full training set).As ex-pected,models trained on original/new and tested on ori-ginal/new perform better than training and testing on differ-ent annotations.To understand better what the new annota-tions bring to the table,we build a hybrid set of annotations. Pruned annotations is a mid-point that allows to decouple the effects of removing errors and improving alignment. Pruned annotations are generated by matching new and ori-ginal annotations(IoU≥0.5),marking as ignore region any original annotation absent in the new ones,and adding any new annotation absent in the original ones.From original to pruned annotations the main change is re-moving annotation errors,from pruned to new,the main change is better alignment.From table4both ACF and RotatedFilters benefit from removing annotation er-rors,even in MR O−2.This indicates that our new training setFigure 8:Examples of automatically aligned ground truth annotations.Left/right →before/after alignment.1×data 10×data aligned withMR O −2(MR O −4)MR N −2(MR N−4)Orig.Ø19.20(34.28)17.22(31.65)Orig.Orig.10×19.16(32.28)15.94(29.33)Orig.New 1/2×16.97(28.01)14.54(25.06)NewNew 1×16.77(29.76)12.96(22.20)Table 5:Detection quality of RotatedFilters on test set when using different aligned training sets.All mod-els trained with Caltech 10×,composed with different 1×+9×combinations.is better sanitized than the original one.We see in MR N −2that the stronger detector benefits more from better data,and that the largest gain in detection qual-ity comes from removing annotation errors.Alignment benefits The detectors from the ICF family benefit from training with increased training data [16,23],using 10×data is better than 1×(see section 2.1).To lever-age the 9×remaining data using the new 1×annotations we train a model over the new annotations and use this model to re-align the original annotations over the 9×portion.Be-cause the new annotations are better aligned,we expect this model to be able to recover slight position and scale errors in the original annotations.Figure 8shows example results of this process.See supplementary material for details.Table 5reports results using the automatic alignment pro-cess,and a few degraded cases:using the original 10×,self-aligning the original 10×using a model trained over original 10×,and aligning the original 10×using only a fraction of the new annotations (without replacing the 1×portion).The results indicate that using a detector model to improve overall data alignment is indeed effective,and that better aligned training data leads to better detection quality (both in MR O and MR N ).This is in line with the analysis of section 3.2.Already using a model trained on 1/2of the new annotations for alignment,leads to a stronger model than obtained when using original annotations.We name the RotatedFilters model trained using the new annotations and the aligned 9×data,Rotated-Filters-New10×.This model also reaches high me-dian true positives IoU in table 3,indicating that indeed it obtains more precise detections at test time.Conclusion Using high quality annotations for training improves the overall detection quality,thanks both to im-proved alignment and to reduced annotation errors.4.2.Convnets for pedestrian detectionThe results of section 3.2indicate that there is room for improvement by focusing on the core background versus foreground discrimination task (the “classification part of object detection”).Recent work [15,20]showed compet-itive performance with convolutional neural networks (con-vnets)for pedestrian detection.We include convnets into our analysis,and explore to what extent performance is driven by the quality of the detection proposals.AlexNet and VGG We consider two convnets.1)The AlexNet from [15],and 2)The VGG16model from [12].Both are pre-trained on ImageNet and fine-tuned over Cal-tech 10×(original annotations)using SquaresChnFtrs proposals.Both networks are based on open source,and both are instances of the R-CNN framework [13].Albeit their training/test time architectures are slightly different (R-CNN versus Fast R-CNN),we expect the result differ-ences to be dominated by their respective discriminative power (VGG16improves 8pp in mAP over AlexNet in the Pascal detection task [13]).Table 6shows that as we improve the quality of the detection proposals,AlexNet fails to provide a consistent gain,eventually worsening the results of our ICF detect-ors (similar observation done in [15]).Similarly VGG provides large gains for weaker proposals,but as the pro-posals improve,the gain from the convnet re-scoring even-tually stalls.After closer inspection of the resulting curves (see sup-plementary material),we notice that both AlexNet and VGG push background instances to lower scores,and at the same time generate a large number of high scoring false positives.The ICF detectors are able to provide high recall proposals,where false positives around the objects have low scores (see [15,supp.material,fig.9]),however convnets have difficulties giving low scores to these windows sur-rounding the true positives.In other words,despite their fine-tuning,the convnet score maps are “blurrier”than the proposal ones.We hypothesise this is an intrinsic limita-tion of the AlexNet and VGG architectures,due to their in-ternal feature pooling.Obtaining “peakier”responses from a convnet most likely will require using rather different ar-chitectures,possibly more similar to the ones used for se-mantic labelling or boundaries estimation tasks,which re-quire pixel-accurate output.Fortunately,we can compensate for the lack of spatial resolution in the convnet scoring by using bounding box regression.Adding bounding regression over VGG,and ap-plying a second round of non-maximum suppression (first NMS on the proposals,second on the regressed boxes),has。
基于高分辨质谱的食品致敏蛋白检测研究进展
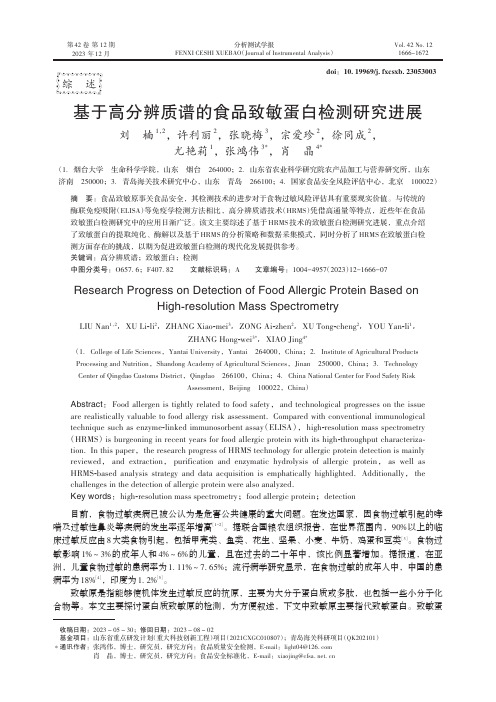
第42 卷 第 12 期2023 年12 月Vol.42 No.121666~1672分析测试学报FENXI CESHI XUEBAO (Journal of Instrumental Analysis )基于高分辨质谱的食品致敏蛋白检测研究进展刘楠1,2,许利丽2,张晓梅3,宗爱珍2,徐同成2,尤艳莉1,张鸿伟3*,肖晶4*(1.烟台大学 生命科学学院,山东 烟台 264000;2.山东省农业科学研究院农产品加工与营养研究所,山东 济南 250000;3.青岛海关技术研究中心,山东 青岛 266100;4.国家食品安全风险评估中心,北京 100022)摘要:食品致敏原事关食品安全,其检测技术的进步对于食物过敏风险评估具有重要现实价值。
与传统的酶联免疫吸附(ELISA )等免疫学检测方法相比,高分辨质谱技术(HRMS )凭借高通量等特点,近些年在食品致敏蛋白检测研究中的应用日渐广泛。
该文主要综述了基于HRMS 技术的致敏蛋白检测研究进展,重点介绍了致敏蛋白的提取纯化、酶解以及基于HRMS 的分析策略和数据采集模式,同时分析了HRMS 在致敏蛋白检测方面存在的挑战,以期为促进致敏蛋白检测的现代化发展提供参考。
关键词:高分辨质谱;致敏蛋白;检测中图分类号:O657.6;F407.82 文献标识码:A 文章编号:1004-4957(2023)12-1666-07Research Progress on Detection of Food Allergic Protein Based onHigh -resolution Mass SpectrometryLIU Nan 1,2,XU Li -li 2,ZHANG Xiao -mei 3,ZONG Ai -zhen 2,XU Tong -cheng 2,YOU Yan -li 1,ZHANG Hong -wei 3*,XIAO Jing 4*(1.College of Life Sciences ,Yantai University ,Yantai 264000,China ;2.Institute of Agricultural Products Processing and Nutrition ,Shandong Academy of Agricultural Sciences ,Jinan 250000,China ;3.Technology Center of Qingdao Customs District ,Qingdao 266100,China ;4.China National Center for Food Safety Risk Assessment ,Beijing 100022,China )Abstract :Food allergen is tightly related to food safety ,and technological progresses on the issue are realistically valuable to food allergy risk assessment. Compared with conventional immunological technique such as enzyme -linked immunosorbent assay (ELISA ),high -resolution mass spectrometry (HRMS ) is burgeoning in recent years for food allergic protein with its high -throughput characteriza⁃tion. In this paper ,the research progress of HRMS technology for allergic protein detection is mainly reviewed ,and extraction ,purification and enzymatic hydrolysis of allergic protein ,as well as HRMS -based analysis strategy and data acquisition is emphatically highlighted. Additionally ,the challenges in the detection of allergic protein were also analyzed.Key words :high -resolution mass spectrometry ;food allergic protein ;detection目前,食物过敏疾病已被公认为是危害公共健康的重大问题。
脑电信号情绪识别关键技术研究进展
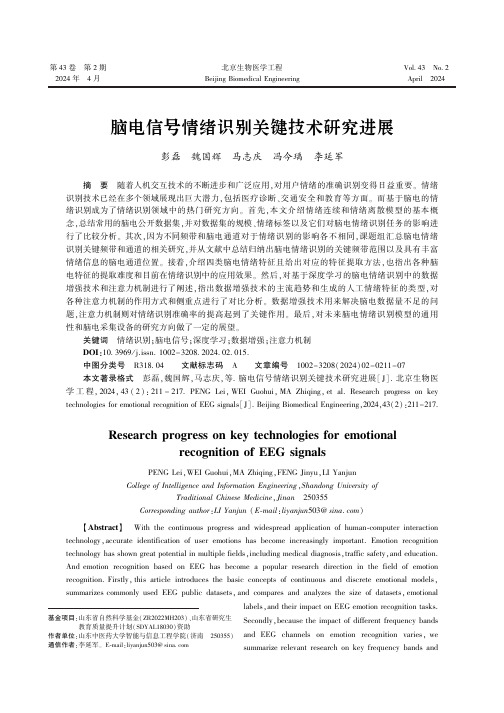
第43卷㊀第2期2024年㊀4月北京生物医学工程BeijingBiomedicalEngineeringVol 43㊀No 2April㊀2024基金项目:山东省自然科学基金(ZR2022MH203)㊁山东省研究生教育质量提升计划(SDYAL18030)资助作者单位:山东中医药大学智能与信息工程学院(济南㊀250355)通信作者:李延军㊂E⁃mail:liyanjun503@sina com脑电信号情绪识别关键技术研究进展彭磊㊀魏国辉㊀马志庆㊀冯今瑀㊀李延军摘㊀要㊀随着人机交互技术的不断进步和广泛应用,对用户情绪的准确识别变得日益重要㊂情绪识别技术已经在多个领域展现出巨大潜力,包括医疗诊断㊁交通安全和教育等方面㊂而基于脑电的情绪识别成为了情绪识别领域中的热门研究方向㊂首先,本文介绍情绪连续和情绪离散模型的基本概念,总结常用的脑电公开数据集,并对数据集的规模㊁情绪标签以及它们对脑电情绪识别任务的影响进行了比较分析㊂其次,因为不同频带和脑电通道对于情绪识别的影响各不相同,课题组汇总脑电情绪识别关键频带和通道的相关研究,并从文献中总结归纳出脑电情绪识别的关键频带范围以及具有丰富情绪信息的脑电通道位置㊂接着,介绍四类脑电情绪特征且给出对应的特征提取方法,也指出各种脑电特征的提取难度和目前在情绪识别中的应用效果㊂然后,对基于深度学习的脑电情绪识别中的数据增强技术和注意力机制进行了阐述,指出数据增强技术的主流趋势和生成的人工情绪特征的类型,对各种注意力机制的作用方式和侧重点进行了对比分析㊂数据增强技术用来解决脑电数据量不足的问题,注意力机制则对情绪识别准确率的提高起到了关键作用㊂最后,对未来脑电情绪识别模型的通用性和脑电采集设备的研究方向做了一定的展望㊂关键词㊀情绪识别;脑电信号;深度学习;数据增强;注意力机制DOI:10 3969/j.issn.1002-3208 2024 02 015.中图分类号㊀R318 04㊀㊀文献标志码㊀A㊀㊀文章编号㊀1002-3208(2024)02-0211-07本文著录格式㊀彭磊,魏国辉,马志庆,等.脑电信号情绪识别关键技术研究进展[J].北京生物医学工程,2024,43(2):211-217.PENGLei,WEIGuohui,MAZhiqing,etal.ResearchprogressonkeytechnologiesforemotionalrecognitionofEEGsignals[J].BeijingBiomedicalEngineering,2024,43(2):211-217.ResearchprogressonkeytechnologiesforemotionalrecognitionofEEGsignalsPENGLei,WEIGuohui,MAZhiqing,FENGJinyu,LIYanjunCollegeofIntelligenceandInformationEngineering,ShandongUniversityofTraditionalChineseMedicine,Jinan㊀250355Correspondingauthor:LIYanjun(E⁃mail:liyanjun503@sina com)ʌAbstractɔ㊀Withthecontinuousprogressandwidespreadapplicationofhuman⁃computerinteractiontechnology,accurateidentificationofuseremotionshasbecomeincreasinglyimportant.Emotionrecognitiontechnologyhasshowngreatpotentialinmultiplefields,includingmedicaldiagnosis,trafficsafety,andeducation.AndemotionrecognitionbasedonEEGhasbecomeapopularresearchdirectioninthefieldofemotionrecognition.Firstly,thisarticleintroducesthebasicconceptsofcontinuousanddiscreteemotionalmodels,summarizescommonlyusedEEGpublicdatasets,andcomparesandanalyzesthesizeofdatasets,emotionallabels,andtheirimpactonEEGemotionrecognitiontasks.Secondly,becausetheimpactofdifferentfrequencybandsandEEGchannelsonemotionrecognitionvaries,wesummarizerelevantresearchonkeyfrequencybandsandchannelsofEEGemotionrecognition,andsummarizethekeyfrequencybandrangeofEEGemotionrecognitionandthelocationofEEGchannelswithrichemotionalinformationfromtheliterature.Next,weintroducefourtypesofEEGemotionalfeaturesandprovidecorrespondingfeatureextractionmethods.ItalsopointsoutthedifficultyofextractingvariousEEGfeaturesandtheircurrentapplicationeffectsinemotionrecognition.Then,thedataaugmentationtechnologyandattentionmechanismindeeplearningbasedEEGemotionrecognitionareelaborated,pointingoutthemainstreamtrendsofdataaugmentationtechnologyandthetypesofartificialemotionfeaturesgenerated.Acomparativeanalysisisconductedonthewaysandfocusesofvariousattentionmechanisms.DataaugmentationtechnologyisusedtosolvetheproblemofinsufficientEEGdata,andattentionmechanismplaysakeyroleinimprovingtheaccuracyofemotionrecognition.Finally,certainprospectsaremadefortheuniversalityoffutureEEGemotionrecognitionmodelsandtheresearchdirectionofEEGacquisitiondevices.ʌKeywordsɔ㊀emotionalrecognition;EEGsignal;deeplearning;dataaugmentation;attentionmechanism图1㊀三种EEG情绪识别流程Figure1㊀ThreeEEGemotionrecognitionprocesses0㊀引言基于生理信号的情绪识别技术是实现人机交互智能化的关键技术㊂而脑电信号(electroencephalography,EEG)在众多生理信号中与情绪的相关性较高,且不易伪装,具有客观性和真实性[1],故采用EEG数据进行研究能够取得更为准确和真实的情绪识别效果㊂常见的情绪识别的流程图可分为三种,如图1所示㊂完整的EEG情绪识别流程如图中第1行所示㊂由于采集EEG数据的实验条件要求较高,许多研究者采用公开的EEG数据集进行情绪识别研究㊂而公开数据集在进行EEG数据采集时就已经去除了一些常见的干扰信号,故研究者在没有特殊要求时无需再对数据进行预处理操作,此类情绪识别流程如图中第2行所示㊂近年来,伴随着情绪识别算法的不断提出,有的研究者直接将数据输入到模型当中,利用模型自动提取EEG数据的深层情绪特征并进行情绪分类㊂这种方法被称为端到端EEG情绪识别,此类情绪识别流程如图中第3行所示㊂本文的主要贡献如下㊂(1)对EEG关键频带和通道文献进行总结,并提出用于EEG情绪识别的优势频带和通道㊂(2)对EEG数据增强技术进行归纳,简述EEG数据增强技术的发展㊂(3)对注意力机制应用于EEG情绪识别任务的相关文献进行整理,简述各类注意力机制的作用方式和侧重点,并提出通用型注意力机制的设想㊂1㊀情绪模型人的内在情绪状态可以通过观察个人的生理信号和外部表达来获取[2]㊂想要识别情绪,就必须将情绪进行数学量化,只有将情绪划分为细致的数学模型,才能实现情绪的识别㊂较为常用的模型分为情绪离散模型和情绪连续模型㊂情绪离散模型将人的情绪分为几种基本情绪,其他情绪都是基本情绪的混合㊂Ekman等[3]提出快乐㊁愤怒㊁悲伤㊁厌恶㊁恐惧㊁惊讶为6种基本情绪,在许多国家的文化中普遍被人们所认知㊂Plutchik[4]提出可以将情绪用色轮的方式概念化,将情绪比作一种颜色,其他情绪是由基本情绪混合而成的㊂情绪连续模型则是将情绪视为情绪基本维度的组合㊂在情绪识别领域中应用最为广泛的当属Russell[5]提出的效价-唤醒(valence⁃arousal)情绪模型,横轴效价也可称之为快乐维度,表示情绪愉悦程度,纵轴唤醒表示情绪强烈程度,两种维度的组合来描述人的各种情绪㊂二维情绪模型如图2二维情绪模型所示㊂㊃212㊃北京生物医学工程㊀㊀㊀㊀㊀㊀㊀㊀㊀㊀㊀㊀㊀㊀㊀㊀㊀㊀㊀第43卷图2㊀二维情绪模型Figure2㊀Two⁃dimensionalemotionalmodel2㊀EEG公开数据集2 1㊀常用数据集介绍在脑电情绪识别领域应用最为广泛的则是Koelstra等[6]提出名为DEAP的多模态数据集,其中的EEG数据是进行基于脑电的情绪识别研究的重要数据㊂每个样本数据长63s,其中包含了3s的基线时间㊂脑电数据分为两个数组,data为40(实验次数)ˑ40(通道数)ˑ8064(63ˑ128)的三维数组,label为40ˑ4的二维数组㊂由于脑电情绪诱发实验开展困难,目前脑电情绪识别领域的公开数据集较少,常用公开数据集总结归纳表如表1所示㊂表1㊀常用公开数据集总结归纳表Table1㊀Summarytableofcommonlyusedpublicdatasets参考文献数据库名称受试者数量通道数情绪标签Koelstra等[6]DEAP3240唤醒㊁效价㊁支配㊁喜好Soleymani等[7]MAHNOB⁃HCI2732唤醒㊁效价㊁支配Zheng等[8]SEED1562积极㊁中性㊁消极Katsigiannis等[9]DREAMER23少量通道效价㊁唤醒㊁支配Song等[10]MPED2362快乐㊁有趣㊁愤怒㊁恐惧等2 2㊀数据集对比在上述的几种数据集中,EEG数据量规模最大的是DEAP数据集,SEED数据量居中,MAHNOB⁃HCI数据集㊁DREAMER数据集和MPED数据集的数据量相对较小,这可能限制一些模型的情绪识别效果㊂其中DEAP㊁MAHNOB⁃HCI和DREAMER数据集的数据标签都具有效价和唤醒维度的数值记录,这有利于模型性能的验证工作,并容易确定受试者情绪在二维情绪模型中的位置㊂SEED和MPED数据集采用离散情绪标签,不利于确定受试者在二维情绪模型上的位置㊂3㊀基于EEG情绪识别的关键频带和通道EEG采集电极放置一般采用10-20电极放置系统,为了寻找最适合用于情感识别的脑电信号频带和通道,研究者们对EEG频带和通道进行了大量实验㊂Yang等[11]的实验分类结果表明,高γ(30100Hz)带特征在情感识别中更有效,所提出的融合特征在公共数据集的高γ波段中获得了最高的分类精度㊂Valenzi等[12]使用32通道脑电信号中的8个通道的脑电数据进行情绪识别,仍然实现了87 5%的平均情绪分类率㊂Zheng等[13]通过深度信念网络从多通道脑电信号中提取微分熵特征来进行情感识别,分析训练完成后的深度信念网络的权值分布,利用12个通道脑电信号就实现了利用64个通道脑电数据才能达到的识别准确率㊂Wang等[14]使用归一化互信息方法选择脑电32通道的最优子集,可以使EEG情绪识别在大幅减少EEG通道的同时获得更高的准确率㊂近年来EEG关键频带和通道文献总结如表2所示㊂从表2中可以看出脑电信号的情绪信息主要集中在β(13 30Hz)和γ频段,且在γ频段更加集中㊂其他频段所包含的情绪信息相对来说较为稀少㊂根据表中EEG情绪识别研究的通道统计和10-20系统图对照,发现大脑额叶区与颞叶区的EEG数据具有更多的情绪信息,隐藏着更多的情绪特征㊂建议多采用额叶区与颞叶区的EEG通道数据用于情绪识别研究,这有利于情绪识别技术的现实应用,实际设备应只需少量通道数据就能实现高准确率的情绪识别㊂4㊀EEG四类情绪特征介绍4 1㊀时域特征波幅㊁标准差㊁均方根等均是EEG的时域特征,可用统计方法提取此类特征㊂此方法操作简单,结㊃312㊃第2期㊀㊀㊀㊀㊀㊀彭磊,等:脑电信号情绪识别关键技术研究进展果清晰直观,但是由于脑电信号是一种非线性非稳态的信号,使用时域特征会加大后期特征识别的难度,现在脑电信号的时域特征多作为辅助手段应用在脑电情绪识别领域㊂Wagh等[22]从脑电信号中提取出了方差㊁标准偏差㊁峰度㊁偏度等时域特征进行情绪识别㊂Liu等[23]将时域的均值㊁标准差㊁原始信号与归一化信号的一阶差均值绝对值和二阶差均值绝对值6个统计特征融合进行情绪分类,发现相比于单一时域特征,使用融合的特征向量情绪识别准确率上升幅度较大㊂表2㊀EEG关键频带和通道文献总结Table2㊀SummaryofliteratureonkeyEEGfrequencybandsandchannels参考文献发表时间关键频带关键通道研究方法Zhang等[15]2016β和γ19通道取得最佳准确度基于ReliefF进行通道选择Özerdem等[16]2017未提及P3,FC2,AF3,O1,Fp1多层感知机神经网络(MLPNN)Zheng等[17]2019β和γ未提及相关系数特征选择方法分析Goshvarpour等[18]2019未提及FP1,C3,CP1,P3,Pz相干性分析和源定位方法Yildirim等[19]2021未提及F3,F4,FC5,AF4,T7,C3,CP2,PO3,O1,O2群体智能算法(swarm⁃intelligence,SI)Peng等[20]2021γ通道重要性从前到后递减:T7,TP7,Fp1,FT7,PO3,FPZ,FP2,TP8,AF3,P2GFIL框架识别Guo等[21]2022未提及FT7,T7,TP7,P3,FC6,FT8,T8,F8DCoT模型评估通道重要性4 2㊀频域特征频谱能量㊁功率谱密度㊁频带功率等是EEG的频域特征,提取此类特征的方法有傅里叶变换㊁小波变换等方法,相比于EEG时域特征,频域特征蕴含更为丰富的情绪信息,可以反映不同频带的能量分布和情绪相关性㊂Pusarla等[24]将EEG转换的二维频谱图提供给DCERNet模型,准确率提升幅度为8%㊂Mohammadi等[25]使用离散小波变换在多个通道比较了不同频带之间的准确度,结果发现Gamma频带的情绪准确率明显高于其他频带㊂4 3㊀时频特征时频功率分布㊁时频相干等为EEG的时频特征,特征提取方法有短时傅里叶变换㊁经验模态分解等㊂时频特征能够描述信号频率随时间的变化,可以同时反映时域和频域的信息㊂Cao等[26]利用小波变换对脑电信号分解,提取各个频段的时频信息,然后使用时间窗函数计算在时频域中的统计特征㊂Salankar等[27]利用经验模态分解将EEG分解为本征模态函数,保留了EEG中时频域中的情绪特征㊂4 4㊀非线性特征常见的非线性EEG特征有微分熵㊁模糊熵㊁分形维数等,这些特征需应用复杂度理论和分形理论进行计算提取,难度较大,应选择合适的非线性脑电特征进行提取㊂其中微分熵(differentialentropy,DE)在EEG情绪识别领域中应用广泛㊂Hwang等[28]提取脑电信号中的DE特征作为CNN模型的输入,实现了90%的情绪识别准确率㊂Zheng等[29]在系统评估流行情绪识别算法的效率时,发现应用DE的模型性能优于其他模型㊂5㊀深度学习在脑电情绪识别领域的应用5 1㊀EEG数据增强技术目前虽有公开数据集能够为基于脑电的情绪识别研究提供数据,但是相比较其他领域的实验数据量而言,EEG数据量太少,且采集脑电数据又是一项成本较高的试验㊂于是研究者们利用数据增强技术产生更多的数据样本,能够在一定程度上解决样本不足的问题㊂目前EEG数据增强技术主要分为两种[30]:第一种是为信号添加噪声(如高斯噪声㊁泊松噪声等),第二种是使用深度学习生成模型㊂常用的数据增强模型有生成对抗网络(generativeadversarialnetworks,GAN)[31]和变分自编码器(auto⁃encodingvariationalBayes,VAE)[32],其中GAN是目前脑电数据增强领域中的重要理论,它能够学习数据的真实分布,从而产生更多类似分布的EEG数据㊂Liu等[33]利用GAN生成多通道脑电图数据的差分熵特征图,再通过模型提取差分熵特征当中隐㊃412㊃北京生物医学工程㊀㊀㊀㊀㊀㊀㊀㊀㊀㊀㊀㊀㊀㊀㊀㊀㊀㊀㊀第43卷藏的情绪信息,实验结果表明在基于脑电图的情感识别任务中有明显的改进㊂Wang等[34]在原始训练数据的每个特征样本中加入高斯噪声,以获得新的训练样本,从而一定程度上缓解深度学习模型的EEG数据中缺乏训练样本的问题,实现了模型性能的改进㊂Pan等[35]利用GAN生成功率谱密度形式的训练样本,在DEAP数据集和MAHNOB⁃HCI数据集的实验结果表明,数据增强后识别精度有显著提高㊂准确率的提高有两个主要原因:一方面,数据增强增加了样本数量㊂另一方面,数据增强将每个类别的样本量增加到相同水平,从而实现了类别之间的平衡㊂近年的EEG数据增强文献总结如表3所示㊂表3㊀EEG数据增强文献总结Table3㊀SummaryofliteratureonEEGdataenhancement参考文献发表时间产生数据样本类型数据增强方法Luo等[36]2020功率谱密度和微分熵条件WassersteinGAN㊁选择性VAE和选择性WGANBao等[37]2021DE特征转化的拓扑图像VAE-D2GAN模型扩充数据Zhang等[38]2021DE特征人工数据多生成器条件WGAN(multiplegeneratorCWGAN,MG⁃CWGAN)Ari等[39]2022CWT尺度图像极限学习机自编码器(extremelearningmachineautoencoder,ELM⁃AE)从表3中能够看出,EEG数据增强技术的主流趋势是使用深度神经网络学习EEG数据的分布特征,生成人工提取的情绪特征如DE特征㊁功率谱密度和连续小波变换(continuouswavelettransform,CWT)尺度图等特征数据样本,较少研究生成EEG原始数据㊂另外,利用噪声的数据增强技术没有进一步新的方法提出㊂5 2㊀基于注意力机制EEG情绪识别神经网络人的大脑在处理信息时会将大部分注意力放在对自己来说最重要的信息区域,注意力机制的提出就是为了模仿人的这种信息处理方式㊂在脑电情绪识别中,注意力机制可以帮助模型更好地关注与情绪相关的特征㊂注意力机制在EEG情绪识别研究常见的有通道注意力机制㊁自我注意力机制等㊂注意力机制可以改变情绪特征的权重,能够提高情绪识别的准确率㊂Tao等[40]提出了一种基于注意力的卷积递归神经网络(attention⁃basedconvolutionalrecurrentneuralnetwork,ACRNN),从脑电信号中提取更多的区别特征,提高情感识别的准确性㊂此模型能自适应地分配不同通道的权重,并采用CNN提取编码的脑电信号的空间信息㊂在DEAP和DREAMER数据库上分别取得了平均93 72%和93 38%的准确率㊂近年来关于基于注意力机制的EEG情绪识别文献具体的总结如表4所示㊂从表4中可以看出,注意力机制与神经网络的融合能够取得良好的实验结果㊂但各种注意力机制改进试验的方式各有侧重,通道㊁区域和空间注意力机制其实质都是对脑电通道进行情绪权重划分,并随着模型训练逐步改进各个通道的情绪权重,从而提高情绪识别准确率㊂多头注意力机制能够使模型能够同时关注不同时间点之间的不同信息,更全面地捕捉脑电信号中的特征和结构,从而进一步提高模型的表现能力和泛化能力㊂频谱注意力机制侧重于学习不同频段的脑电信号在情绪识别任务中的重要性,此方法可以根据任务需求,自适应地调整不同频段的情绪权重,从而改进情绪识别效果㊂未来应将各种注意力机制进一步融合,形成同时对频带㊁通道和时间进行情绪权重划分的通用型注意力机制模型,进一步挖掘EEG数据中的隐藏情绪信息,进一步提升模型的情绪识别准确率㊂6㊀总结与展望第一个问题是情绪识别神经网络模型的通用性较弱,目前大多数模型主要是针对主流EEG公开数据集中的受试者的EEG数据所设计的,如果更换EEG数据源进行情绪识别,情绪识别的准确率会有较大幅度的下降,而迁移学习能够提高算法对不同EEG数据的通用性,故应该在迁移学习方面投入时间精力,解决模型的通用性问题,并使模型具有跨域情绪识别能力㊂第二个问题是便携性的EEG采集设备需要进一步革新,应该根据对EEG情绪识别关键频带和通道的相关研究和与情绪相关的脑区研究,尽快研发一种便携性,低数量EEG通道,抗干扰能力强,具有频带选择功能的EEG采集设备㊂此种设备能够促进小型EEG数据集的产生,而众多研究者的小型㊃512㊃第2期㊀㊀㊀㊀㊀㊀彭磊,等:脑电信号情绪识别关键技术研究进展表4㊀基于注意力机制的EEG情绪识别文献总结Table4㊀SummaryofliteratureonEEGemotionrecognitionbasedonattentionmechanism参考文献发表时间神经网络及所提取的情绪特征注意力机制数据集情绪分类标签各个维度的准确率/%Zhang等[41]2021局部到全局BiLSTM(regiontoglobal⁃spatiotemporal⁃BiLSTM,R2G⁃ST⁃BiLSTM)情绪特征:局部到全局脑区更具区分性的时空脑电特征区域注意机制,确定不同脑区的权重,从而增强或削弱各脑区对情绪识别的贡献DEAP效价,唤醒,支配,喜欢平均94 69Hu等[42]2022CNN⁃BiLSTM⁃MHSA情绪特征:时间序列,空间信息多头自注意力机制DEAP效价,唤醒,支配,喜欢平均98 10Xiao等[43]20224D⁃ANN情绪特征:空间,频谱,时间特征频谱和空间注意机制,时间注意机制SEED积极,中性,消极平均96 10Jiang等[44]2022基于注意机制的混合网络(FFT_CNN⁃LSTM⁃Attention,FFT_CLA)情绪特征:空间特征,时间特征通道注意力机制,自注意力机制DEAP效价,唤醒,支配,喜欢平均92 38Li等[45]2022空间频率卷积自注意网络(spatial⁃frequencyconvolutionalself⁃attentionnetwork,SFCSAN)情绪特征:空间和频带特征频带内自注意力学习频率信息,频带间最终注意力学习互补频率信息DEAP效价,唤醒,支配,喜欢95 15,95 76,95 64,95 86EEG数据集能够融合为数据量足够的EEG公开数据集,故此种设备的研发将极大促进EEG情绪识别研究㊂参考文献[1]㊀BlackMH,ChenNTM,IyerKK,etal.Mechanismsoffacialemotionrecognitioninautismspectrumdisorders:insightsfromeyetrackingandelectroencephalography[J].Neuroscience&BiobehavioralReviews,2017,80:488-515.[2]㊀LinW,LiC.Reviewofstudiesonemotionrecognitionandjudgmentbasedonphysiologicalsignals[J].AppliedSciences,2023,13(4):2573.[3]㊀EkmanP,FriesenWV,O sullivanM,etal.Universalsandculturaldifferencesinthejudgmentsoffacialexpressionsofemotion[J].JournalofPersonalityandSocialPsychology,1987,53(4):712-717.[4]㊀PlutchikR.Thenatureofemotions:humanemotionshavedeepevolutionaryroots,afactthatmayexplaintheircomplexityandprovidetoolsforclinicalpractice[J].AmericanScientist,2001,89(4):344-350.[5]㊀RussellJA.Acircumplexmodelofaffect[J].JournalofPersonalityandSocialPsychology,1980,39(6):1161-1178.[6]㊀KoelstraS,MuhlC,SoleymaniM,etal.Deap:adatabaseforemotionanalysis;usingphysiologicalsignals[J].IEEETransactionsonAffectiveComputing,2011,3(1):18-31.[7]㊀SoleymaniM,LichtenauerJ,PunT,etal.Amultimodaldatabaseforaffectrecognitionandimplicittagging[J].IEEETransactionsonAffectiveComputing,2011,3(1):42-55.[8]㊀ZhengWL,LiuW,LuY,etal.Emotionmeter:amultimodalframeworkforrecognizinghumanemotions[J].IEEETransactionsonCybernetics,2019,49(3):1110-1122.[9]㊀KatsigiannisS,RamzanN.DREAMER:adatabaseforemotionrecognitionthroughEEGandECGsignalsfromwirelesslow⁃costoff⁃the⁃shelfdevices[J].IEEEJournalofBiomedicalandHealthInformatics,2018,22(1):98-107.[10]㊀SongT,ZhengW,LuC,etal.MPED:amulti⁃modalphysiologicalemotiondatabasefordiscreteemotionrecognition[J].IEEEAccess,2019,7:12177-12191.[11]㊀YangK,TongL,ShuJ,etal.HighgammabandEEGcloselyrelatedtoemotion:evidencefromfunctionalnetwork[J].FrontiersinHumanNeuroscience,2020,14:89.[12]㊀ValenziS,IslamT,JuricaP,etal.IndividualclassificationofemotionsusingEEG[J].JournalofBiomedicalScienceandEngineering,2014(8):604-620.[13]㊀ZhengWL,LuBL.InvestigatingcriticalfrequencybandsandchannelsforEEG⁃basedemotionrecognitionwithdeepneuralnetworks[J].IEEETransactionsonAutonomousMentalDevelopment,2015,7(3):162-175.[14]㊀WangZM,HuSY,SongH.ChannelselectionmethodforEEGemotionrecognitionusingnormalizedmutualinformation[J].IEEEAccess,2019,7:143303-143311.[15]㊀ZhangJ,ChenM,ZhaoS,etal.ReliefF⁃basedEEGsensorselectionmethodsforemotionrecognition[J].Sensors,2016,16:1558.[16]㊀ÖzerdemMS,PolatH.EmotionrecognitionbasedonEEGfeaturesinmovieclipswithchannelselection[J].BrainInformatics,2017,4:241-252.[17]㊀ZhengWL,ZhuJY,LuBL.IdentifyingstablepatternsovertimeforemotionrecognitionfromEEG[J].IEEETransactionson㊃612㊃北京生物医学工程㊀㊀㊀㊀㊀㊀㊀㊀㊀㊀㊀㊀㊀㊀㊀㊀㊀㊀㊀第43卷AffectiveComputing,2017,10(3):417-429.[18]㊀GoshvarpourA,GoshvarpourA.AnovelapproachforEEGelectrodeselectioninautomatedemotionrecognitionbasedonlaggedPoincare sindicesandsLORETA[J].CognitiveComputation,2020,12(3):602-618.[19]㊀YildirimE,KayaY,KiliçF.AchannelselectionmethodforemotionrecognitionfromEEGbasedonswarm⁃intelligencealgorithms[J].IEEEAccess,2021,9:109889-109902.[20]㊀PengY,QinF,KongW,etal.GFIL:aunifiedframeworkfortheimportanceanalysisoffeatures,frequencybands,andchannelsinEEG⁃basedemotionrecognition[J].IEEETransactionsonCognitiveandDevelopmentalSystems,2022,14(3):935-947.[21]㊀GuoJY,CaiQ,AnJP,etal.AtransformerbasedneuralnetworkforemotionrecognitionandvisualizationsofcrucialEEGchannels[J].PhysicaA:StatisticalMechanicsanditsApplications,2022,603:127700.[22]㊀WaghKP,VasanthK.Performanceevaluationofmulti⁃channelelectroencephalogramsignal(EEG)basedtimefrequencyanalysisforhumanemotionrecognition[J].BiomedicalSignalProcessingandControl,2022,78:103966.[23]㊀LiuY,FuG.Emotionrecognitionbydeeplylearnedmulti⁃channeltextualandEEGfeatures[J].FutureGenerationComputerSystems,2021,119:1-6.[24]㊀PusarlaN,SinghA,TripathiS.LearningDenseNetfeaturesfromEEGbasedspectrogramsforsubjectindependentemotionrecognition[J].BiomedicalSignalProcessingandControl,2022,74:103485.[25]㊀MohammadiZ,FrounchiJ,AmiriM.Wavelet⁃basedemotionrecognitionsystemusingEEGsignal[J].NeuralComputingandApplications,2017,28:1985-1990.[26]㊀CaoX,ZhaoK,XuD.Emotionrecognitionofsingle⁃electrodeEEGbasedonmulti⁃featurecombinationintime⁃frequencydomain[J].JournalofPhysics:ConferenceSeries,2021,1827(1):012031.[27]㊀SalankarN,MishraP,GargL.EmotionrecognitionfromEEGsignalsusingempiricalmodedecompositionandsecond⁃orderdifferenceplot[J].BiomedicalSignalProcessingandControl,2021,65:102389.[28]㊀HwangS,HongK,SonG,etal.LearningCNNfeaturesfromDEfeaturesforEEG⁃basedemotionrecognition[J].PatternAnalysisandApplications,2020,23:1323-1335.[29]㊀ZhengWL,ZhuJY,LuBL.IdentifyingstablepatternsovertimeforemotionrecognitionfromEEG[J].IEEETransactionsonAffectiveComputing,2017,10(3):417-429.[30]㊀LashgariE,LiangD,MaozU.Dataaugmentationfordeep⁃learning⁃basedelectroencephalography[J].JournalofNeuroscienceMethods,2020,346:108885.[31]㊀BhatS,HortalE.GAN⁃baseddataaugmentationforimprovingtheclassificationofEEGsignals[C]//The14thPervasiveTechnologiesRelatedtoAssistiveEnvironmentsConference.Corfu,Greece:ACM,2021:453-458.[32]㊀TianC,MaY,CammonJ,etal.Dual⁃encoderVAE⁃GANwithspatiotemporalfeaturesforemotionalEEGdataaugmentation[J].IEEETransactionsonNeuralSystemsandRehabilitationEngineering,2023,31:2018-2027.[33]㊀LiuQ,HaoJ,GuoY.EEGdataaugmentationforemotionrecognitionwithatask⁃drivenGAN[J].Algorithms,2023,16:118.[34]㊀WangF,ZhongS,PengJ,etal.DataaugmentationforEEG⁃basedemotionrecognitionwithdeepconvolutionalneuralnetworks[M]//MultiMediaModeling.Bangkok,Thailand:SpringerInternationalPublishing,2018:82-93.[35]㊀PanB,ZhengW.EmotionrecognitionbasedonEEGusinggenerativeadversarialnetsandconvolutionalneuralnetwork[J].ComputationalandMathematicalMethodsinMedicine,2021,2021:2520394.[36]㊀LuoY,ZhuLZ,WanZY,etal.DataaugmentationforenhancingEEG⁃basedemotionrecognitionwithdeepgenerativemodels[J].JournalofNeuralEngineering,2020,17(5):056021.[37]㊀BaoG,YanB,TongL,etal.DataaugmentationforEEG⁃basedemotionrecognitionusinggenerativeadversarialnetworks[J].FrontiersinComputationalNeuroscience,2021,15:723843.[38]㊀ZhangA,SuL,ZhangY,etal.EEGdataaugmentationforemotionrecognitionwithamultiplegeneratorconditionalWassersteinGAN[J].Complex&IntelligentSystems,2022,8:3059-3071.[39]㊀AriB,SiddiqueK,AlçinÖF,etal.WaveletELM⁃AEbaseddataaugmentationanddeeplearningforefficientemotionrecognitionusingEEGrecordings[J].IEEEAccess,2022,10:72171-72181.[40]㊀TaoW,LiC,SongR,etal.EEG⁃basedemotionrecognitionviachannel⁃wiseattentionandselfattention[J].IEEETransactionsonAffectiveComputing,2023,14(1):382-393.[41]㊀ZhangP,MinC,ZhangK,etal.Hierarchicalspatiotemporalelectroencephalogramfeaturelearningandemotionrecognitionwithattention⁃basedantagonismneuralnetwork[J].FrontiersinNeuroscience,2021,15:738167.[42]㊀HuZ,ChenL,LuoY,etal.EEG⁃basedemotionrecognitionusingconvolutionalrecurrentneuralnetworkwithmulti⁃headself⁃attention[J].AppliedSciences,2022,12(21):11255.[43]㊀XiaoG,ShiM,YeM,etal.4Dattention⁃basedneuralnetworkforEEGemotionrecognition[J].CognitiveNeurodynamics,2022,16(4):805-818.[44]㊀JiangH,WuD,TangX,etal.EEGemotionrecognitionusinganattentionmechanismbasedonanoptimizedhybridmodel[J].Computers,Materials&Continua,2022,73(2):2697-2712.[45]㊀LiD,XieL,ChaiB,etal.Spatial⁃frequencyconvolutionalself⁃attentionnetworkforEEGemotionrecognition[J].AppliedSoftComputing,2022,122:108740.(2023-07-26收稿,2023-10-24修回)㊃712㊃第2期㊀㊀㊀㊀㊀㊀彭磊,等:脑电信号情绪识别关键技术研究进展。
血浆游离DNA Septin 9基因甲基化检测方法_NormalPdf
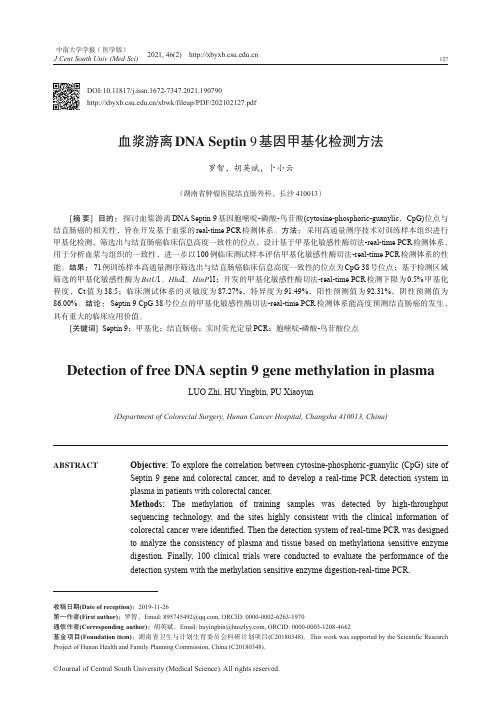
中南大学学报(医学版)J Cent South Univ (Med Sci)2021,46(2)血浆游离DNA Septin 9基因甲基化检测方法罗智,胡英斌,卜小云(湖南省肿瘤医院结直肠外科,长沙410013)[摘要]目的:探讨血浆游离DNA Septin 9基因胞嘧啶-磷酸-鸟苷酸(cytosine-phosphoric-guanylic ,CpG)位点与结直肠癌的相关性,旨在开发基于血浆的real-time PCR 检测体系。
方法:采用高通量测序技术对训练样本组织进行甲基化检测,筛选出与结直肠癌临床信息高度一致性的位点,设计基于甲基化敏感性酶切法-real-time PCR 检测体系,用于分析血浆与组织的一致性,进一步以100例临床测试样本评估甲基化敏感性酶切法-real-time PCR 检测体系的性能。
结果:71例训练样本高通量测序筛选出与结直肠癌临床信息高度一致性的位点为CpG 38号位点;基于检测区域筛选的甲基化敏感性酶为BstU 1、Hha I 、HinP 1I ;开发的甲基化敏感性酶切法-real-time PCR 检测下限为0.5%甲基化程度,Ct 值为38.5;临床测试体系的灵敏度为87.27%,特异度为91.49%,阳性预测值为92.31%,阴性预测值为86.00%。
结论:Septin 9CpG 38号位点的甲基化敏感性酶切法-real-time PCR 检测体系能高度预测结直肠癌的发生,具有重大的临床应用价值。
[关键词]Septin 9;甲基化;结直肠癌;实时荧光定量PCR ;胞嘧啶-磷酸-鸟苷酸位点Detection of free DNA septin 9gene methylation in plasmaLUO Zhi,HU Yingbin,PU Xiaoyun(Department of Colorectal Surgery,Hunan Cancer Hospital,Changsha 410013,China)ABSTRACT Objective:To explore the correlation between cytosine-phosphoric-guanylic (CpG)site of Septin 9gene and colorectal cancer,and to develop a real-time PCR detection system in plasma in patients with colorectal cancer.Methods:The methylation of training samples was detected by high-throughput sequencing technology,and the sites highly consistent with the clinical information of colorectal cancer were identified.Then the detection system of real-time PCR was designed to analyze the consistency of plasma and tissue based on methylationa sensitive enzyme digestion.Finally,100clinical trials were conducted to evaluate the performance of the detection system with the methylation sensitive enzyme digestion-real-time PCR.DOI :10.11817/j.issn.1672-7347.2021.190790/xbwk/fileup/PDF/202102127.pdf收稿日期(Date of reception):2019-11-26第一作者(First author):罗智,Email:895745492@,ORCID:0000-0002-6263-1970通信作者(Corresponding author):胡英斌,Email:huyingbin@,ORCID:0000-0003-1208-4662基金项目(Foundation item):湖南省卫生与计划生育委员会科研计划项目(C20180348)。
基于SURF算法和OpenCV的掌纹识别技术研究

基于SURF算法和OpenCV的掌纹识别技术研究周新宇;姚茂国;竺乐庆【摘要】该文提出了一种基于快速鲁棒特征算法和OpenCV的掌纹识别方法.首先应用固定阈值法将手掌二值化,并提取出有效掌纹区域.然后用快速鲁棒特征算法提取并描述该区域的特征点,再通过随机采样一致性算法进行特征点之间的匹配.最后,通过一系列测试对该系统的性能进行了评估,实验结果证明该系统对掌纹图像的旋转、亮度和尺度变化具有较好的鲁棒性,且具有比较高的识别精度和时间性能.【期刊名称】《杭州电子科技大学学报》【年(卷),期】2012(032)003【总页数】4页(P45-48)【关键词】快速鲁棒特征;掌纹识别;鲁棒性;随机采样一致性算法【作者】周新宇;姚茂国;竺乐庆【作者单位】浙江工商大学计算机与信息工程学院,浙江杭州310018;浙江工商大学计算机与信息工程学院,浙江杭州310018;浙江工商大学计算机与信息工程学院,浙江杭州310018【正文语种】中文【中图分类】TP391.40 引言掌纹识别技术是一种生物识别技术。
人的掌纹具有唯一性和终身不变性,掌纹图像拥有丰富的纹理特征,而且图像易获取,因此,掌纹图像可用于人的身份识别。
目前已提出的掌纹识别方法有基于结构的,基于子空间,基于编码和基于统计等[1]。
主成分分析法[2],二维主成分分析法[3-4],独立主成分分析法[5-7],Fisher线性判别算法[8]的相继提出,以及多线性独立成分分析的掌纹识别方法使得掌纹识别研究日益成熟[9]。
本文提出了一种基于SURF算法的掌纹识别新方法,完善和丰富了掌纹图像鉴别分析理论,是对现有掌纹识别算法的一种补充和扩展。
1 基于SURF算法的掌纹识别1.1 图像预处理系统首先对原始掌纹图像进行预处理,预处理过程如图1所示。
首先对图1(a)进行平滑处理和二值化处理得到图1(b),然后取得图1(b)的最大内切圆,得到图1(c),最后以该内切圆半径的0.8倍为边长取得正方形区域(以内切圆的圆心为正方形的中心)得到图1(d),显而易见,图1(d)集中了手掌大部分掌纹信息。
- 1、下载文档前请自行甄别文档内容的完整性,平台不提供额外的编辑、内容补充、找答案等附加服务。
- 2、"仅部分预览"的文档,不可在线预览部分如存在完整性等问题,可反馈申请退款(可完整预览的文档不适用该条件!)。
- 3、如文档侵犯您的权益,请联系客服反馈,我们会尽快为您处理(人工客服工作时间:9:00-18:30)。
Rapid and sensitive detection of CpG-methylation using methyl-binding (MB)-PCRClaudia Gebhard,Lucia Schwarzfischer,Thu Hang Pham,Reinhard Andreesen,Andreas Mackensen and Michael Rehli*Department of Hematology and Oncology,University Hospital,93042,Regensburg,GermanyReceived April 12,2006;Revised June 2,2006;Accepted June 5,2006ABSTRACTMethylation of CpG islands is associated with transcriptional repression and,in cancer,leads to the abnormal silencing of tumor suppressor genes.We have developed a novel technique for detecting CpG-methylated DNA termed methyl-binding (MB)-PCR.This technique utilizes a recombinant protein with high affinity for CpG-methylated DNA that is coated onto the walls of a PCR vessel and selectively captures methylated DNA fragments from a mixture of genomic DNA.The retention and,hence,the degree of methylation of a specific DNA fragment (e.g.a CpG island promoter of a specific gene)is detected in the same tube by gene-specific PCR.MB-PCR does not require bisulfite treatment or methylation-sensitive restriction and provides a quick,simple and extremely sensitive technique allowing the detection of methylated DNA,in particular in tumor tissue or tumor cells from limited ing this novel approach,we determined the methylation status of several estab-lished and candidate tumor suppressor genes and identified the ICSBP gene,encoding the myeloid and B-cell-specific transcription factor interferon consensus sequence-binding protein,as a target for aberrant hypermethylation in acute myeloid leukemia.INTRODUCTIONDuring the past few years it has become increasingly clear that the formation of tumors is supported not only by genetic lesions (e.g.mutations or translocations)but also by epigen-etic changes,including alterations in the methylation status of DNA (1,2).In mammals,methylation of DNA occurs at spe-cific cytosine residues which precede a guanosine residue (CpG-dinucleotides)and generally correlates with stable tran-scriptional repression (3–5).The aberrant gain of DNAmethylation (hypermethylation)in neoplastic cells frequently affects DNA sequences with a relatively high content in CpG-dinucleotides,the so-called CpG islands.These regions often contain transcription initiation sites and promoters and with only few exceptions are generally not methylated in normal cells (3,4,6–8).It is particularly in tumors that CpG islands that are normally not methylated can be present in a hypermethylated form.In many cases,genes affected by the hypermethylation encode proteins that counteract the growth of a tumor such as tumor suppressor genes (1,2,9,10).Reasons for the tumor-specific hypermethylation are unknown.Interestingly,certain kinds of tumors seem to have their own hypermethylation profiles (11,12).Promoter-hypermethylation events may actually provide some of the most promising markers that can be used to improve cancer detection and the assessment of cancer risk.Hence,the detec-tion of CpG-methylated DNA and thus the identification of dysregulated tumor suppressor genes and/or oncogenes is of outmost clinical interest (2,13,14).Here,we present a novel,single tube,PCR-based techni-que that we termed methyl-binding (MB)-PCR,allowing the sensitive detection of CpG island-specific methylation.This novel method avoids the manipulation of DNA by methylation-sensitive restriction endonucleases or bisulfite treatment and relies on the ability of a recombinant,bivalent,methyl-CpG-binding polypeptide to specifically bind CpG-methylated DNA fragments.The single tube assay involves the fractionated binding of restricted genomic DNA by the recombinant methyl-CpG-binding polypeptide immobilized on the walls of a PCR vessel and the subsequent detection of bound DNA by PCR in the same tube.Given the enormous amplification capability and specificity of PCR,MB-PCR can reliably detect the methylation degree of a specific genomic DNA fragment from <30cells.Using the described technique,we determined the methyla-tion status of several established (ESR1;CDKN2B )and candidate tumor suppressor genes (ICSBP ,ETV3,DDX20)in leukemia cell lines,normal cells and blasts from 35patients with newly diagnosed,untreated acute myeloid leukemia (AML).We confirm earlier studies showing the frequent methylation of the estrogen receptor (ESR1)as well as the CDKN2B (also known as p15INK4b )CpG island promoter*To whom correspondence should be addressed.Tel:+499419445587;Fax:+499419445593;Email:michael.rehli@klinik.uni-regensburg.deÓ2006The Author(s).This is an Open Access article distributed under the terms of the Creative Commons Attribution Non-Commercial License (/licenses/by-nc/2.0/uk/)which permits unrestricted non-commerical use,distribution,and reproduction in any medium,provided the original work is properly cited.Nucleic Acids Research,2006,Vol.34,No.11e82doi:10.1093/nar/gkl437Published online July 5, 2006 at Wuhan University on January 19, 2011 Downloaded from(15–17).We also identify ICSBP as a target for aberrant promoter methylation in a subset of AML patients.MATERIALS AND METHODS CellsPeripheral blood mononuclear cells (MNC)were separated by leukapheresis of healthy donors,followed by density gra-dient centrifugation over Ficoll/Hypaque.Monocytes were isolated from MNC by countercurrent centrifugal elutriationin a J6M-E centrifuge (Beckman,Mu¨nchen,Germany)as described previously (18).Drosophila S2cells were obtained from ATCC and cultured in Insect-Xpress medium (Bio Whittaker)containing 10%fetal calf serum (FCS;PAA)in an incubator at 21 C.The human myeloid leukemia cell lines THP-1,NB-4,KG-1,K562,HL-60and U937were grown in RPMI 1640medium supplemented with 10%FCS.The human myeloid leukemia cell line Mono Mac 6was grown in RPMI 1640medium plus 10%FCS and 1%OPI media supplement (Sigma).The human myeloid leuk-emia cell line MUTZ-3was maintained in a MEM plus 20%FCS and 10ng/ml stem cell factor.For DNA-demethylation,U937cells were treated with the indicated amounts of Decitabine (2-deoxy-50-azacytidine;Sigma)for several days.Patient samplesFresh peripheral blood samples and bone marrow specimens from 35patients with newly diagnosed and untreated de novo or secondary AML were used for the study.All patients were treated according to the protocol AMLCG-2000of the German AML Cooperative Group.The study was approved by the Institutional Ethics Committee,and written informed consent was obtained from each patient before entering the study.Recombinant expression of the methyl-binding polypeptide MBD-F cA detailed description of the design and generation of the MBD-F c protein will be given elsewhere (19).In brief,a cDNA corresponding to the methyl-CpG-binding domain of human MBD2(amino acids 144–230)was PCR-amplified,fused to the F c -tail of human IgG1,and subcloned into the inducible expression vector pMTBiP/V5-His (Invitrogen).The resulting vector was stably transfected into Drosophila S2cells using Effectene transfection reagent (Qiagen)and hygromycin selection.For large-scale protein production,1–5·108cells were cultured in 100–200ml Insect-Xpress medium without FCS in 2000ml roller bottles for 2days before the addition of 0.5mM CuSO 4.Culture medium was harvested every 4–7days and cells were replated in medium plus CuSO 4for continued protein production.Cell culture supernatants were pooled and purified using a protein A–Sepharose (Amersham)column.The purified protein (200–700m g/ml)was dialyzed against TBS and 0.6%gelatine/0.02%NaN 3was added as preservative.DNA preparationGenomic DNA from various cellular sources was prepared using the Blood and Cell Culture DNA Midi Kit from Qiagen.Quality of the genomic DNA preparation was controlled by agarose gel electrophoresis and DNA concen-tration was determined by UV spectrophotometry.Genomic DNA was digested with MseI (NEB)and quantified using PicoGreen dsDNA Quantification Reagent (Molecular Probes).Where indicated,DNA was in vitro methylated using SssI methylase (NEB).Preparation of PCR tubes for MB-PCRMBD-F c -coated PCR tubes were prepared using heat stable TopYield ÔStrips (Nunc).Recombinant MBD-F c protein (50m l,diluted at 15m g/ml in 10mM Tris–HCl,pH 7.5)were added to each tube and incubated overnight at 4 C.Tubes were washed three times with 200m l TBS (20mM Tris,pH 7.5,containing 170mM NaCl)and blocked for 3h with 100m l blocking solution [10mM Tris,pH 7.5,containing 170mM NaCl,5%skim milk powder,5mM EDTA and 1m g/ml of each poly(dI–dC),poly(dA–dT)and poly(dC–dG)(Amersham)].Tubes were washed two times with 200m l TBST (TBS containing 0.05%Tween-20)and once with binding buffer (20mM Tris,pH 7.5,containing 400mM NaCl,2mM MgCl 2,0.5mM EDTA and 0.05%Tween-20).Binding of methylated DNA (M -reaction)Binding buffer (50m l)(20mM Tris,pH 7.5,containing 400mM NaCl,2mM MgCl 2,0.5mM EDTA and 0.1%Tween-20)was added to each coated,blocked and washed tube and 2m l digested DNA (5ng/m l)was added to every second tube (M -reaction).The salt concentration in the bind-ing buffer was initially determined in pilot experiments to allow the efficient binding of highly methylated but not unmethylated CpG island fragments.The salt concentration determines the ‘cut-off’and may be adjusted for individual DNA fragments.Tubes were incubated on a shaker at room temperature for 40min,washed two times with 200m l bind-ing buffer and once with 200m l of 10mM Tris–HCl,pH 8.0.Detection of methylated DNA fragmentsPCR was carried out directly in the treated and washed TopYield ÔStrips.The PCR mixture (50m l/well)included 10pmol of each gene-specific primer (synthesized by Metabion).Primer sequences were P15S (50-GGC TCA GCT TCA TTA CCC TCC-30),P15AS (50-AAA GCC CGG AGC TAA CGA C-30),ESR1S (50-GAC TGC ACT TGC TCC CGT C-30),ESR1AS (50-AAG AGC ACA GCC CGA GGT TAG-30),ICSBP S (50-CGG AAT TCC TGG GAA AGC C-30),ICSBP AS (50-TTC CGA GAA ATC ACT TTC CCG-30),METS S (50-AAT TGC GTC TGA AGT CTG CGG-30),METS AS (50-TCC CAC ACA ACA GAG AGG CG-30),DP103S (50-GCT GTT AGT CCA GTT CCA GGT TCC-30),DP103AS (50-GTG CAA CCA CAT TTA TCT CCG G-30),Chr6-S (50-GAA ACC CTC ACC CAG GAG ATA CAC-30),Chr6-AS (50-TGC AGT GGG ACT TTA TTC CAT AGA AGA G-30),Chr9-S (50-GTG TCC ACA TCT CTT CTG GGT AAC TC-30)and Chr6-AS (50-AGT AAG CTC CTT TCC CTA AGC CA-30).Aftere82Nucleic Acids Research,2006,Vol.34,No.11P AGE 2OF9at Wuhan University on January 19, 2011 Downloaded fromadding the PCR mixture,1m l MseI-digested DNA (5ng/m l)was added to every other well,that was not incubated previ-ously with DNA fragments (P -reaction).PCR was performed on a MJResearch engine with the following cycling condi-tions:95 C for 3min (denaturation),94 C for 20s,60 C for 20s and 72 C for 70s (36cycles)and 72 C for 5min (final extension).Initial pilot experiments showed that the reaction was still in the linear phase of amplification using the above conditions (Figure 2B).The MB-PCR products (20m l)were analyzed using 3%agarose gel electrophoresis and the ethidium bromide stained gels were scanned using a Typhoon 9200Imager (Amersham/Pharmacia).Sodium bisulfite sequencingModification of DNA with sodium bisulfite (20)was per-formed as described previously (21).Bisulfite-treated DNA was amplified in a nested PCR using the primers icsbp-out S (50-GGG GTA GTT AGT TTT TGG TTG-30)and icsbp-out AS (50-ATA AAT AAT TCC ACC CCC AC-30)for the first and icsbp-in S (50-TTG TGG ATT TTG ATT AAT GGG-30)and icsbp-in AS (50-CCR CCC ACT ATA CCT ACC TAC C-30)for the second round of amplification.PCR products were cloned using TOPO-TA Cloning Kit (Invitrogen)and several independent clones were sequenced.RNA-preparation,real-time PCRTotal RNA was isolated from different cell lines by the guanidine thiocyanate/acid phenol method (22).RNA (2m g)was reverse transcribed using Superscript II MMLV-RT (Invitrogen).Real-time PCR was performed on a Light-Cycler (Roche)using the Quantitect kit (Qiagen)according to the manufacturer’s instructions.Primers used were ICSBP ,sense 50-CGT GGT GTG CAA AGG CAG-30,anti-sense 50-CTG TTA TAG AAC TGC TGC AGC TCT C-30;and ACTB ,sense 50-TGA CGG GGT TCA CCC ACA CTG TGC CCA TCT A-30,antisense 50-CTA GAA GCA TTT GTG GTG GAC GAT GGA GGG-30.Cycling parameters were denaturation 95 C,15min,amplification 95 C,15s,57 C,20s,72 C,25s,for 50cycles.The product size was initially controlled by agarose gel electrophoresis and melting curves were analyzed to control for specificity of the PCRs.ICSBP data were normalized for expression of the ACTB gene.The relative units were calculated from a standard curve plotting three different concentrations of log dilutions against the PCR cycle number (CP)at which the measured fluorescence intensity reached a fixed value.The amplifi-cation efficiency E was calculated from the slope of the standard curve by the formula E ¼10À1/slope .E ICSBP ranged from 1.87to 1.98and E ACTB ranged from 1.76to 1.84.For each,sample data of three independent analyses were averaged.RESULTSOutline of the MB-PCR techniqueWe reasoned that a methyl-CpG DNA-binding polypeptide covalently coupled to the vessel walls of a PCR tube might allow the binding and detection of CpG-methylated DNA in a manner comparable to the standard ELISA techniques.Initial pilot experiments led to the establishment of a proce-dure,termed MB-PCR,that could reliably detect CpG-methylated DNA fragments.The basic steps of this novel technique are outlined in Figure 1.In the first step,a protein with high affinity for CpG-methylated DNA is coated onto the walls of a PCR-cycler compatible reaction tube and used to selectively capture strongly methylated DNA frag-ments from a genomic DNA mixture.The retention of a specific DNA fragment (e.g.a CpG island promoter of a spe-cific gene)is detected in the same tube using PCR (the M -reaction).The degree of methylation may be estimated relative to a PCR of the genomic input DNA (the P-reaction).Figure 1.Outline of MB-PCR.The major steps of the MB-PCR procedure are illustrated.MB-PCR comprises two separate reactions,the control-PCR (P -reaction)which amplifies a candidate locus directly from a genomic template,and the MB-PCR which amplifies the candidate locus from the template DNA that was bound previously by the methyl-CpG-binding polypeptide in the reaction vessel (M -reaction).In the first step,the inner walls of both reaction vessels are coated with a methyl-binding polypeptide and subsequently saturated using blocking reagents (step 2).The template DNA (genomic DNA restricted with MseI or similar enzymes)is then added to one tube (M -reaction)and allowed to bind (step 3).In the last step,the PCR mixture is added directly into both tubes and 50%of template DNA used previously for the M -reaction is added to the P -reaction.After gene-specific PCR,products may be analyzed,e.g.by agarose gel electrophoresis.P AGE 3OF9Nucleic Acids Research,2006,Vol.34,No.11e82at Wuhan University on January 19, 2011 Downloaded fromTo enable the high-affinity binding of double-stranded, CpG-methylated DNA,we used a fusion protein comprising the methyl-CpG-binding domain(MBD)of human MBD2, aflexible linker polypeptide and the F c portion of human IgG1.MBD2was chosen,because previous binding studies suggested that its methyl-binding domain shows a high affinity to CpG-methylated DNA when compared to other mammalian MBD proteins.The bivalent structure of the antibody-like MBD-F c protein likely further increases its affinity and avidity towards CpG-methylated DNA.Design, production and properties of the MBD-F c protein will be described elsewhere(19).Briefly,its affinity to a given DNA fragment depends on the following variables:(i)the amount of salt in the reaction or washing buffer(low salt requires little CpG methylation for binding,high salt requires more CpG methylation for binding);(ii)the number of methylated CpG-dinucleotides;and(iii)the density of the MBD-F c protein on the interaction surface.The binding reaction and two washing steps are performed in a stringent high salt buffer(400mM NaCl)assuring that fragments with little or no methylation are washed off.In contrast to most previous methods,detection of CpG methylation by MB-PCR therefore largely depends on the degree/number of methylated cytosine residues in a given DNA fragment.We explored the MB-PCR method by analyzing the degree of CpG methylation of single CpG island promoters that were shown previously to be frequently methylated in leukemia cells,namely the human CDKN2B gene(also known as p15INK4b)and the human estrogen receptor1(ESR1)gene. In addition to the well-established tumor markers we selected three additional genes with CpG island promoters that could potentially act as tumor suppressor genes:the human interferon consensus-binding protein(ICSBP)gene,the human Ets variant3gene(ETV3)and the human DEAD box polypeptide20gene(DDX20).ICSBP,a transcription factor of the interferon(IFN)regulatory factor family (IRF),is frequently down-regulated in human myeloid leuk-emia(23)and ICSBP-deficient mice display hematological alterations similar to chronic myelogenous leukemia(CML) in humans(24),suggesting a tumor suppressor function for ICSBP in hemopoietic cells.In mice,the Ets repressor ETV3(also known as METS or PE1)and its co-repressor DDX20(also known as DP103)were shown to link terminal monocytic differentiation to cell-cycle arrest(25),which may also indicate a possible tumor suppressor role.As negative controls for the binding and washing procedure,we also designed two sets of primers that detect CpG-free genomic MseI fragments on chromosomes6and9.To allow for a semi-quantitative analysis of the genomic PCR,we initially tested a series of DNA dilutions at various cycle numbers.As shown in Figure2B using36cycles our PCR approach was able to detect a range of5ng down to at least250pg,suggesting that ethidium bromide stained PCR fragments could be semi-quantitatively analyzed under these conditions.As a validation of our MB-PCR approach, genomic DNA from normal cells was either left untreated or methylated in vitro using SssI,digested with MseI and subjected to MB-PCR.Genomic DNA was digested with MseI because this enzyme is methylation insensitive and cuts DNA into small fragments while leaving CpG islands relatively intact(26).Locations of the detected MseI fragments relative to thefirst exon of their respective genes as well as positions of gene-specific primers usedforFigure2.MB-PCR detects methylation of CpG island promoters.(A)Schematic presentation of the detected MseI-fragments(indicated as gray boxes)of ESR1,CDKN2B(p15INK4b),ICSBP,ETV3and DDX20.The position of CpG-dinucleotides,MseI-restriction sites,transcription start site,first exon and relative positionof primers are marked.(B)P-reaction for ICSBP using a serial dilution of MseI-digested genomic DNA.(C)Representative MB-PCR results of normal (unmethylated)and in vitro methylated genomic DNA for the indicated promoters and CpG-free regions.The P-reaction directly amplifies the genomic DNA,whereas the M-reaction only amplifies CpG-methylated DNA fragments.e82Nucleic Acids Research,2006,Vol.34,No.11P AGE4OF9at Wuhan University on January 19, 2011Downloaded fromMB-PCR are shown in Figure 2A.All fragments in CpG-containing regions include the putative proximal promoter regions.As shown in Figure 2C,the M -reactions of all five gene loci were negative when normal DNA was used,indic-ating that these genomic regions are,as expected,free of methylation in normal blood cells.However,each locus was amplified in the corresponding M -reaction when the same DNA was in vitro methylated using SssI-methylase before it was subjected to MB-PCR.The CpG-free genomic regions were not amplified in untreated and SssI-treated DNA,suggesting that the amplification of a genomic DNA fragment requires the presence of DNA methylation and that no unmethylated DNA is retained after washing.Hence,MB-PCR is able to discriminate the methylated and unmethylated state at these loci.Methylation status of specific CpG island promoters in tumor cell lines analyzed by MB-PCRTo test whether MB-PCR is able to detect the methylation status of the above loci in biological samples,we analyzed several leukemia cell lines.Routinely,a total of 10ng of restricted DNA was used for the M -reaction and 5ng of the same digested genomic DNA was used for the P -reaction.The result of a representative MB-PCR experiment from eight different leukemia cell lines is shown in Figure 3A.The ESR1promoter was amplified to varying degrees in the M -reaction of all eight samples,which is in line with previous reports demonstrating its aberrant methylation in >80%of human hemopoietic tumors.The P -reaction for the CDKN2B promoter failed completely in three cell lines (THP-1,NB-4and K562)suggesting mutations or deletions on both alleles,which has been demonstrated previously in the cases of NB-4(16)and K562(27).The two cell lines KG-1and MUTZ-3showed a positive M -reaction for the CDKN2B promoter,whereas three cell lines (U937,MonoMac6and HL-60)were negative.The observed results were in good concordance with previously published methylation analyses of ESR1(28)and CDKN2B promoters (15,16,27).In some cases,P -reactions were weaker in com-parison with other cell types,suggesting the loss or mutation of one allele (e.g.ESR1in U937cells).Interestingly,the ICSBP promoter was also amplified in M -reactions of six cell lines,whereas no significant methylation was detected at the promoters of ETV3and DDX20genes.The two negat-ive control fragments on chromosomes 6and 9were positive in the P -reaction but also negative in the M -reaction (data not shown).Since aberrant CpG methylation at the ICSBP locus has not been described previously,the degree and effect of ICSBP promoter methylation was analyzed to further validate the experimental potential of MB-PCR.To determine how MB-PCR results correlate with the exact pattern of CpG methylation at the ICSBP promoter in individual cell lines,we analyzed ICSBP promoter methylation by bisulfite sequencing.The results shown in Figure 3B indicate that the degree of promoter methylation corresponds with results obtained by MB-PCR.Strong amplification signals (com-parable with the corresponding P -reaction)as seen in KG-1,U937,MUTZ-3,HL-60and K562cell lines,appear to indic-ate a high degree,whereas weaker signals (as observed for NB-4cells)indicate a lesser degree of methylation.In the absence of DNA methylation (THP-1and MonoMac6cells)the MB-PCR is negative.As shown in Figure 4A,mRNA expression levels analyzed by LightCycler real-time PCR inversely correlated with methylation degree as determined by MB-PCR and bisulfite sequencing.Treat-ment of U937cells,which show a high degree of ICSBP promoter methylation with the demethylating agent Decitabine (5-Aza-20Deoxycytidine)led to a marked,dose-and time-dependent induction of ICSBP mRNA exp-ression (Figure 4B),indicating that the methylation-induced repression of ICSBP transcription is reversible in thesecells.Figure 3.Detecting CpG methylation in leukemia cell lines by MB-PCR.(A )Shown are representative MB-PCR results of eight different leukemia cell lines for the indicated promoters.(B )Genomic DNA from the same cell lines was analyzed by bisulfite sequencing.The indicated region of the ICSBP gene was amplified and cloned.Several independent inserts were sequenced and results are presented schematically.Squares mark the position of CpG-dinucleotides (open,unmethylated;closed,methylated).P AGE 5OF9Nucleic Acids Research,2006,Vol.34,No.11e82at Wuhan University on January 19, 2011 Downloaded fromLinearity and sensitivitySamples derived from tumors may contain significant num-bers of normal cells,that would be expected to be unmethyl-ated at most CpG islands.To test how linear the detection of CpG methylation is with respect to cell purity,MB-PCR was performed using mixtures of DNA from normal blood cells (freshly isolated blood monocytes)and a leukemia cell line KG-1showing high levels of CpG island methylation at thepromoters investigated in this study.As shown in Figure 5A,the ICSBP promoter fragment was only detected in samples containing KG-1DNA and the signal gradually increased with the proportion of methylated DNA in the sample.Similar results were obtained for ESR1and CDKN2B loci (data not shown).To test the sensitivity of our approach,we performed MB-PCR experiments using decreasing amounts of DNA.Dilutions of genomic DNA from three different cell lines (U937,NB-4and THP-1)were subjected to MB-PCR.To account for the reduced amount of template DNA in the PCRs,the cycle number was increased proportionally.As shown in Figure 5B,comparable results were obtained using either 10ng,2.5ng,625pg or even 160pg of digested genomic DNA,suggesting that MB-PCR is able to detect the methylation status of a single promoter in DNA derived from as few as 30cells.Detecting methylation of CpG island promoters in primary tumor cellsDNA was prepared from blood monocytes of several healthy persons (n ¼4)and leukemic blasts of patients with previ-ously untreated AML (n ¼35),digested with MseI,and sub-jected to MB-PCR.Figure 6shows representative ICSBP MB-PCR and corresponding bisulfite sequencing results for nine AML patients and one normal individual.In general,the intensity of the band observed in the M -reaction (as com-pared with the corresponding P -reaction)showed good cor-relation with the mean density of methylation in the sample.Out of 35AML patients tested,7patients (20%)showed positive MB-PCR results for ICSBP ,21patients (60%)for ESR1and 25patients (71%)for CDKN2B (data not shown).The frequencies for ESR1and CDKN2B methylation observed concur with those described in previous studies (16,28).ICSBP methylation apparently only affects a sub-group of patients.Twelve patients were tested for methyla-tion of ETV3and DDX20genes and,as observed for the leukemia cell lines,no significant methylation was detected in any of thesamples.Figure 4.Methylation of the ICSBP promoter inversely correlates with ICSBP expression in leukemia cell lines.(A )Transcription levels of ICSBP were determined by LightCycler real-time PCR relative to the housekeeping gene ACTB .(B )U937cells,treated with Decitabine (DAC)for the indicated time periods were analyzed for ICSBP expression.Results were normalized to ACTB expression.Data represent mean values ±SD of two independent LightCycleranalyses.Figure 5.Sensitivity of MB-PCR.(A )MB-PCRs for the ICSBP promoter from mixtures of DNA from a healthy donor (N,unmethylated)and DNA from the cell line KG-1(methylated).The relative contribution of each DNA is indicated in the table on the right.(B )DNA samples from three cell lines were subjected to MB-PCR using 10ng and down to 160pg of DNA for the M -reaction.The number of amplification cycles (given in parentheses)was adjusted to the amount of DNA.e82Nucleic Acids Research,2006,Vol.34,No.11P AGE 6OF9at Wuhan University on January 19, 2011 Downloaded fromDISCUSSIONWe have developed a novel one tube assay,termed MB-PCR,to detect genomic DNA fragments according to their level of CpG methylation.The novel technique requires little amounts of DNA and allows the rapid screening of multiple loci.MB-PCR is particularly useful to screen for methylation levels of candidate genes in tumor tissue or tumor cells as exemplified for acute myeloid leukemia in this report.It may,however,also be useful to detect changes in DNA methylation in other situations,including normal cellular differentiation and aging.Comparison with existing methodsAt present,mainly two technical approaches are used to detect the level of CpG methylation of known candidate gene loci:methylation-sensitive restriction or bisulfite treatment of DNA (29).Isoschizomers of bacterial restriction endonucleases with different sensitivities for 5-methylcytosine can be used to determine the methylation status of specific CpG-dinucleotides (29).The use of methylation-sensitive restric-tion enzymes,however,has several limitations.Apart from the fact that incomplete restriction digests may complicatethe analysis,the greatest disadvantage is that methylation-sensitive restriction merely informs on the methylation status of the cytosine residues which are recognized by the methylation-sensitive restriction enzymes used.A global picture of the methylation pattern in a candidate gene locus may be obtained by bisulfite sequencing as originally described by Frommer et al .(20).The treatment of double-stranded genomic DNA with sodium bisulfite leads to the deamination of unmethylated cytosine residues (but not 5-methyl cytosine)into uracil residues.DNA treated with bisulfite can be used directly in PCR in which uracil residues (previously unmethylated cytosine)and thymidine residues are amplified as thymidine and only 5-methylcytosine residues are amplified as cytosine residues (20).Depending on the application,the primers used for the PCR differentiate between methylated and unmethy-lated sequences or amplify fragments independently of the methylation status (29).PCR fragments which have been amplified using non-discriminating primers can,for instance,be sequenced directly to determine the position of methylated and unmethylated CpGs.Other methodical approaches that allow high-throughput analyses utilize the differences in sequence for the specific amplification of methylated and unmethylated sequences by discriminating primers or probes (e.g.methylation-specific PCR,MethyLight)(29).In contrast to the methylation-sensitive restriction enzymes,the DNA treated with bisulfite can potentially provide information on the methylation status of several CpG residues in an amplified genomic fragment.The detection of CpG methylation by using discriminating primers or probes,however,is limited to the methylation status of single (or few)cytosine residues.Hence,the information provided by all currently known assays that are suitable for high-throughput methylation analysis of single gene loci is limited to one or only a few CpG residues within the gene of interest.Rather than analyzing single CpG residues,MB-PCR ana-lyses target DNA fragments according to their methylation degree.The information provided by MB-PCR will be at least as relevant as that obtained with other existing PCR techniques—the methylation density of a proximal promoter may actually correlate better with the transcriptional status of a gene than the methylation status of a single CpG residue within the region.We believe that the high methyl-CpG affinity of MBD2combined with the bivalent,antibody-like structure of the recombinant MBD-F c protein greatly increases its binding capacity,enabling the efficient retention of a DNA fragment on the basis of its degree of methylation.A comparable approach discriminating DNA fragments on the basis of their methylation density was developed in the laboratory of A.Bird already 10years ago (26).A recombi-nant MeCP2protein bound to a matrix was used in this and a number of recent studies for binding and enriching highly methylated DNA through affinity chromatography (26).Although we have not tested recombinant MeCP2,it is possible that it may also work as methyl-binding polypeptide in MB-PCR.The company Panomics introduced recently a commer-cially available kit that differentiates promoters with methyl-ated groups from unmethylated promoters.In principle,the company’s method consists of a spin column affinity purifica-tion using MeCP2.This method also appears to berapid,Figure 6.Detection of aberrant CpG methylation in primary AML blasts.Two independent MB-PCRs (independent restriction digest and preparation of MBD-F c )for the ICSBP promoter of one representative healthy donor (N)and nine AML patients are shown together with corresponding sequencing results.(Results of bisulfite sequencing are presented as described in Figure 3.)P AGE 7OF9Nucleic Acids Research,2006,Vol.34,No.11e82at Wuhan University on January 19, 2011 Downloaded from。