A Case-Based Reasoning Framework for Enterprise Model Building, Sharing and Reusing
KBS

Knowledge-Based Systems E-Business Study Topicsa. Bayesian Network P45Bayesian networks:These are probabilistic models based on directed graphs capturingcausal relationships between a number of variables being modelled. These can provide veryaccurate tools for predication and diagnosis. Microsoft is a big supporter basing variousdiagnostic tools on the technology.b. Formal Representation P89Formal representation is the process of coding knowledge for input into a computer using a restricted syntax as defined by a formal grammar developed for the purpose. Formalrepresentation results in statements with which you can reason automatically using computersoftware.c. Expert System Shell P4Expert system shell: the middle ring shows the expert system shell that provides computational facilities for applying the knowledge base to user decision support: ♦the application system at the bottom tests the user's hypotheses or seeks to satisfy his goals by deriving the consequences of facts about a particular situation reported bythe user, using the general facts and inference rules supplied by the expert and editedby the knowledge engineer;♦the explanation system to the lower left answers queries about the way in which facts have been derived in terms of what information and inference rules have been used;♦the acquisition system to the upper left provides tools for interviewing the expert to obtain his vocabulary, and general facts and inference procedures in terms of it;♦the display system at the top provides tools for presenting the knowledge base in an understandable form--the relations between the facts and inference procedures;♦the edit system to the upper right provides tools for editing the knowledge base while maintaining its integrity in terms of the vocabulary, variables and operations used;♦the validation system to the lower right provides tools for checking the knowledge base against specific case histories with known consequences.d. Reasoning2.1 What Is Knowledge?p7In A.I. research the data and knowledge two have different meanings. Traditionally the termdata is used to describe simple information such as numbers, strings and Boolean values. Todeal with the real world we need more complex information such as processes, procedures, actions, causality, time, motivations, goals, and common sense reasoning. The term knowledge is used to describe this sort of information of which data is merely a subset. It could more formally be described as a symbolic description (or model) of a domain (or universe of discourse)).It is in artificial intelligence research that knowledge representation lends itself to utilization because knowledge is the second requirement of intelligent behavior (the first one is reasoning).1.Reasoning engine: Inference mechanisms for manipulating the symbolicinformation and knowledge in the knowledge base to form a line ofreasoning in solving a problem. The inference mechanism can range fromsimple modus ponens backward chaining of IF-THEN rules to case-basedreasoning.Reason using representations of human knowledge∙that is a previously encountered problem may even have additional information, such as how the problem was solved or symptoms that are associated with the problem.e. Knowledge Management P9Knowledge Management is the collection of processes that govern the creation, dissemination, and utilization of knowledge. In one form or another, knowledge management has been around for a very long time. Practitioners have included philosophers, priests, teachers, politicians, scribes, Liberians, etc.So if Knowledge Management is such an ageless and broad topic what role does it serve in today's Information Age? These processes exist whether we acknowledge them or not and they have a profound effect on the decisions we make and the actions we take, both of which are enabled by knowledge of some type. If this is the case, and we agree that many of our decisions and actions have profound and long lasting effects, it makes sense to recognize and understand the processes that effect or actions and decision and, where possible, take steps to improve the quality these processes and in turn improve the quality of those actions and decisions for which we are responsible?Knowledge management is not a, "a technology thing" or a, "computer thing" If we accept the premise that knowledge management is concerned with the entire process of discovery and creation of knowledge, dissemination of knowledge, and the utilization of knowledge then we are strongly driven to accept that knowledge management is much more than a "technology thing" and that elements of it exist in each of our jobs.f. Knowledge Elicitation P234.2 Knowledge ElicitationThe most important branch of knowledge acquisition is knowledge elicitation- obtaining knowledge from a human expert (or human experts) for use in an expert system.Knowledge elicitation is difficult. This is the principle reason why expert systems have not become more widespread - the knowledge elicitation bottleneck.It is necessary to find out what the expert(s) know, and how they use their knowledge.Expert knowledge includes:∙domain-related facts & principles;∙modes of reasoning;∙reasoning strategies;∙explanations and justifications.The knowledge elicitation (and analysis) task involves:∙Finding at least one expert in the domain who:o is willing to provide his/her knowledge;o has the time to provide his/her knowledge;o is able to provide his/her knowledge.∙Repeated interviews with the expert(s), plus task analysis, concept sorting, etc, etc..∙Knowledge structuring: converting the raw data (taken from the expert) into intermediate representations, prior to building a working system.∙This will improve the knowledge engineer's understanding of the subject;∙This provides easily-accessible knowledge for future KEs to work from (knowledge archiving).∙Building a model of the knowledge derived from the expert, for the expert to criticise.From then on, the development proceeds by stepwise refinement.One major obstacle to knowledge elicitation: experts cannot easily describe all they know about their subject.∙They do not necessarily have much insight into the methods they use to solve problems.Their knowledge is "compiled" (c.f. a compiled computer program - fast & efficient, but unreadable).Some of the techniques used in Knowledge Elicitation∙Various different forms of interview:o Unstructured. A general discussion of the domain, designed to provide a list of topics and concepts.Structured. Concerned with a particular concept within the domain.o Problem-solving. The expert is provided with a real-life problem, of a kind that they deal with during their working life, and asked to solve it. As they doso, they are required to describe each step, and their reasons for doing whatthey do. The transcript of their verbal account is called a protocol.o Think-aloud. As above, but the expert merely imagines that they are solving the problem presented to them, rather than actually doing it. Once again, theydescribe the steps involved in solving the problem.o Dialogue. The expert interacts with a client, in the way that they would normally do during their normal work routine.o Review. The KE and DE examine the record of one of the sessions described above, together.∙Sample lecture preparation. The expert prepares a lecture, and the KE analyses its content.∙Concept sorting ("card sort").∙Questionnaires. Especially useful when the knowledge is to be elicited from several different experts.∙Repertory grid (particularly the "laddered grid" technique).It is standard practice to tape record KE sessions. However, KEs should be aware of the costs this involves, in time and money.The above techniques will be discussed in detail later.g. Knowledge Acquisition P9Knowledge acquisition: how to translate human knowledge in current, written, conceptual and abstract representations into computer representations.Knowledge Acquisition is the process of obtaining knowledge for use in the knowledge base of an expert system.h. Semantics P11Semantic networks. A semantic network is a method of representing knowledge often used for critical analysis of literary texts. Similar to hypertext technologies in some ways, but with emphasis on typed links among concepts.i. Knowledge Networks P14Knowledge Network is a search tool for a knowledgebase.j. Knowledge Engineers P4♦knowledge engineers act as intermediaries between the expert and the system, helping him to encode his knowledge and validate the operation of the expert system.k. Inference Rule P4、P65♦the application system at the bottom tests the user's hypotheses or seeks to satisfy his goals by deriving the consequences of facts about a particular situation reported by the user, using the general facts and inference rules supplied by the expert and edited by the knowledge engineer;ImplementationDuring the next stage, implementation, the formalized knowledge is mapped or coded into the framework of the development tool to build a working prototype. The contents of knowledge structures, inference rules and control strategies established in the previous stages are organized into suitable format. Often, knowledge engineers will have been using the program development tool to build a working prototype to document and organize information collected during the formalization stage, so that implementation is completed at this point. If not, the notes from the earlier phases are coded at this time.The inference engine:1. Combines the facts of a specific case with the knowledge contained in the knowledge base to come up with a recommendation. In a rule-based expert system, the inference engine controls the order in which production rules are applied2. Directs the user interface to query the user for any information it needs for further inferencing.Inference Engine∙General problem-solving knowledge or methods∙Interpreter analyzes and processes the rules∙Scheduler determines which rule to look at next∙The search portion of a rule-based system∙It takes advantage of heuristic information∙Otherwise, the time to solve a problem could become prohibitively long∙This problem is called the combinatorial explosion∙Expert-system shell provides customizable inference engineKnowledge base: the central ring shows the knowledge base of facts and inference rules. This extends the facilities of a conventional database by enabling the storage not only of facts but also of rules that enable further facts to be derived.P1♦Interpretation - take observations and infer descriptions e.g. natural language understanding♦Prediction - recognise situations and infer likely consequences e.g. weather forecastingP12 and Groupware P12IntranetsAn intranet is an network, internal to the organization, based on Internet and World Wide Web technology. By using common Internet protocols, or core technologies, in conjunction with their own business applications, corporations can easily communicate, distribute information, and facilitate project collaboration across the entire enterprise while keeping unauthorized users out.GroupwareGroupware is software that was created in recognition of the significance of groups in offices by providing functions and services that support the collaborative activities of work groups.L OTUS N OTES D OMINO allows users to coordinate work with built-in calendars, scheduling, e-mail, web navigational tools, integrated support of internet standards. To Learn more about Lotus Notes and the Domino web version 5.0, please click on the URL above to go to that site.MS E XCHANGE S ERVER is Microsoft's software that is competing directly with Lotus Notes for customers. It gives businesses the ability to rely on their messaging and collaboration servers, provides for a comprehensive messaging platform that includes the tools necessary to create rich collaboration applications.Intellectual Asset Management is the management the intellectual asset of a corporation, including patents, copyrights, etc.A company called A URIGIN offers a solution called IPAM (Intellectual Property Asset Management System) which allows organizations to organize, analyze and manage intellectual property using an intranet.P27 and Observation P27InterviewsKnowledge engineers elicit knowledge from experts in conversation. The process is best started with free-formed questions, narrowing in specificity. The expert is in control, which has some advantages, but makes interviews very time-consuming.Observation of task performanceThe expert's performance, while working at a real problem, may be recorded by simply watching or by videotaping the process.and Sharing P101Knowledge creating - knowledge modelling, knowledge representation (in fact, all activities useful in a development process) are phases arising from methods elaborated in "knowledge engineering environments”.sharing –modern technologies (including intelligent browsers) offer almostunlimited access to knowledge resources from any place. For example: a well conceived e-commerce application contains knowledge on products and services, is able to explain how to use products in given contexts, how to connect several devices together, etc.-Based P33Rule-based programming is one of the most commonly used techniques for developing expert systems. In this programming paradigm, rules are used to represent heuristics, or "rules of thumb," which specify a set of actions to be performed for a given situation. A rule is composed of an if portion and a then portion. The if portion of a rule is a series of patterns which specify the facts (or data) which cause the rule to be applicable. The process of matching facts to patterns is called pattern matching. The expert system tool provides a mechanism, called the inference engine, which automatically matches facts against patterns and determines which rules are applicable. The if portion of a rule can actually be thought of as the whenever portion of a rule since pattern matching always occurs whenever changes are made to facts. The then portion of a rule is the set of actions to be executed when the rule is applicable. The actions of applicable rules are executed when the inference engine is instructed to begin execution. The inference engine selects a rule and then the actions of the selected rule are executed (which may affect the list of applicable rules by adding or removing facts). The inference engine then selects another rule and executes its actions. This process continues until no applicable rules remain-Symbolic Knowledge P18SubSymbolic.The knowledge is stored without the use of symbols. This typically means the architecture uses direct mapping from the inputs to outputs.P87and Graphs P86The decision table formalism supports presentation of conditions and conditioned actions. A decision table usually consists of four parts. The top left part lists the possible conditions while the bottom left lists the possible actions. The right part indicates the particular action to be taken (the bottom part) for each set of circumstances (the top right part). Tables are represented graphically; if each column contains simple states we call it expanded DTa, otherwise, if contractions or irrelevant conditions are allowed, we say the table is in consolidated form. Using a set of tables in order to modularise the tabular knowledge base, we are able to represent different knowledge bases after transforming them into rules or directly into tables.Decision tables in validation & verificationAcquiring the correct and complete knowledge is one of the main problems in building knowledge-based systems. Also, maintaining the knowledge base is not a trivial task and often introduces unnoticed inconsistencies or contradictions. Verification and validation (V&V) of knowledge based systems are receiving increased attention.It has been reported earlier (e.g. Vanthienen [19], Cragun and Steudel [4], Puuronen [16]) that, in a vast majority of cases, the decision table technique easily allows to check for common V&V problems, such as contradictions, inconsistencies, incompleteness, redundancy, etc. in the problem specification.10.5 Benefits Of Decision TablesDecision tables offer some important benefits as described below:CompletenessKnowledge bases often suffer from missing attribute values or combinations of attribute values, unreachable conclusions, etc. The nature of the (single hit) decision table easily allows to check for completeness, because the number of simple columns (before contraction) should equal the product of the number of states for every condition. Completeness of combinations of attribute values can, therefore, be enforced automatically.ConsistencyInconsistency occurs when rules with the same premises but different conclusions exist. When these conclusions are contradictory, the rules are in conflict. If the contradictory conclusions deal with opposite values of the same action, this will be called contradiction. When the conclusions do not necessarily contradict each other, the rules are ambiguous. Because in a (correct) decision table all columns are non-overlapping and each column refers to exactly one configuration of conclusions, inconsistency between columns will not occur. Non-redundancyBy definition, a single hit decision table eliminates redundant rules and premises, as a combination of condition states will be included in only one column.CorrectnessAfter the decision tables have been designed, the knowledge engineer may want to check the (semantic) correctness of the decision specification, verifying that for each possible case the right action(s) will be executed. The decision table format easily allows this kind of validation.More recently, also other researchers have realized the importance of identifying inconsistencies and redundancies early in the knowledge base development process. In Ngwenyama and Bryson [13], a formal method, based on decision table matrices, is presented to solve the problems of redundancy and inconsistency when integrating the rule sets of multiple experts.P18 and Frames P203.3 RulesCurrently, the most popular method of knowledge representation is in the form of rules (also known as production rules or rule-based systems).Below are illustrated the use of rules through a simple rule base of five rules created for the domain of credit approval. There are many questions a loan officer may ask in the process of deciding whether to approve or deny an application for credit. Some of the questions the officer may ask concern:♦the current salary of the person,♦the credit history of the person, and♦their current employmentA simple (fictitious) rule base that might be applicable to this domain is given below.3.4 FramesThe use of object-oriented methods in software development has impacted the development of E/KBS as well. Knowledge in an E/KBS can also be represented using the concept of objects to capture both the declarative and procedural knowledge in a particular domain. In E/KBS, the terminology that is used to denote the use of objects is frames, and frames are fast becoming a popular and economical method of representing knowledge. Frames are extremely similar to object-oriented technology and provide many of the benefits that have been attributed to object-oriented systems.A frame is a self-contained unit of knowledge that contains all of the data (knowledge) and the procedures associated with the particular object in the domain. In Figure 1.1, we show a hierarchy of objects using the classification of humans as the particular domain. Each of the frames in Figure 1.1 represents an object in the domain. The top-level object is known as the class. As you proceed down the tree each of the objects become a more specific example of the upper node. For instance, Jack is a particular example of a Male and Human; we call Jackan instance of the class Human, while Male is a subclass of Human.from research laboratories to business applications P16918.2 Stages In The Process Of Data MiningStage 1: Exploration.This stage usually starts with data preparation which may involve cleaning data, data transformations, selecting subsets of records and - in case of data sets with large numbers of variables ("fields") - performing some preliminary feature selection operations to bring the number of variables to a manageable range (depending on the statistical methods which are being considered). Then, depending on the nature of the analytic problem, this first stage of the process of data mining may involve anywhere between a simple choice of straightforward predictors for a regression model, to elaborate exploratory analyses using a wide variety of graphical and statistical methods in order to identify the most relevant variables and determine the complexity and/or the general nature of models that can be taken into account in the next stage.Stage 2: Model building and validation. This stage involves considering various models and choosing the best one based on their predictive performance (i.e., explaining the variability in question and producing stable results across samples). This may sound like a simple operation, but in fact, it sometimes involves a very elaborate process. There are a variety of techniques developed to achieve that goal - many of which are based on so-called "competitive evaluation of models," that is, applying different models to the same data set and then comparing their performance to choose the best. These techniques - which are often considered the core of predictive data mining - include: Bagging (Voting, Averaging), Boosting, Stacking (Stacked Generalizations), and Meta-Learning.Stage 3: Deployment.That final stage involves using the model selected as best in the previous stage and applying it to new data in order to generate predictions or estimates of the expected outcome.The concept of Data Mining is becoming increasingly popular as a business information management tool where it is expected to reveal knowledge structures that can guide decisions in conditions of limited certainty. Recently, there has been increased interest in developing new analytic techniques specifically designed to address the issues relevant to business Data Mining (e.g., Classification Trees), but Data Mining is still based on the conceptual principles of statistics including the traditional Exploratory Data Analysis (EDA) and modeling and it shares with them both some components of its general approaches and specific techniques. However, an important general difference in the focus and purpose between Data Mining and the traditional Exploratory Data Analysis (EDA) is that Data Mining is more oriented towards applications than the basic nature of the underlying phenomena. In other words, Data Mining is relatively less concerned with identifying the specific relations between the involved variables. For example, uncovering the nature of the underlying functions or the specific types of interactive, multivariate dependencies between variables are not the main goal of Data Mining. Instead, the focus is on producing a solution that can generate useful predictions. Therefore, Data Mining accepts among others a "black box" approach to data exploration or knowledge discovery and uses not only the traditional Exploratory Data Analysis (EDA) techniques, but also such techniques as Neural Networks which can generate valid predictions but are not capable of identifying the specific nature of the interrelations between the variables on which the predictions are based.P17618.8 Reasons for the growing popularity of Data MiningGrowing Data VolumeThe main reason for necessity of automated computer systems for intelligent data analysis is the enormous volume of existing and newly appearing data that require processing. The amount of data accumulated each day by various business, scientific, and governmental organizations around the world is daunting. According to information from GTE research center, only scientific organizations store each day about 1 TB (terabyte!) of new information. And it is well known that academic world is by far not the leading supplier of new data. It becomes impossible for human analysts to cope with such overwhelming amounts of data. Limitations of Human AnalysisTwo other problems that surface when human analysts process data are the inadequacy of the human brain when searching for complex multifactor dependencies in data, and the lack of objectiveness in such an analysis. A human expert is always a hostage of the previous experience of investigating other systems. Sometimes this helps, sometimes this hurts, but it is almost impossible to get rid of this fact.Low Cost of Machine LearningOne additional benefit of using automated data mining systems is that this process has a much lower cost than hiring an army of highly trained (and payed) professional statisticians. While data mining does not eliminate human participation in solving the task completely, it significantly simplifies the job and allows an analyst who is not a professional in statistics and programming to manage the process of extracting knowledge from data.Chapter 18 – Data Mining (2) Page 10a. Procedural P7 and Declarative Knowledge P29b. General and Domain-based Problem Solving Methodc. Tacit P99 and Explicit Knowledge P51、54d. Data Driven and Goal Driven Reasoning Methoda.Classification Of KnowledgeKnowledge can be classified as either static or dynamic. If it describes properties of, or relations between, objects and processes, we call it static or descriptive. If it provides tools that help to decide how to use (manipulate, reason with) this static knowledge when solving particular problems, or is goal-oriented, we call it dynamic or procedural knowledge.The two main forms of knowledge are experimental and theoretical. Experimental knowledge encapsulates experience, contains examples, precedents and situations, while, theoretical knowledge includes conceptual components (notions), declarative components (sentences that define relationships between concepts independent of any procedure to manipulate them), and operative components (actions).Knowledge may also be domain-specific, or related to a specific topic; or common-sense, or part of the everyday knowledge about the world and its contents that underlies most other reasoning. It may also represent the organization, structure and the usage of knowledge itself, and is called meta-level knowledge.Declarative knowledge representations are, for KA, preferable to procedural ones, as their meaning is explicit (can be "read directly").Furthermore, the notion of declarative knowledge representations is open-ended, in the sense that it will accommodate changes to the knowledge base. Current research at Carnegie-Mellon is aimed at reducing maintenance costs still further through the creation of higher level “shells” that actively support the process of knowledge acquisition and testing. New prototype versions of the configuration system are now being reengineered using these shells.b. the primary problem solving method is pattern recognition. Some additional features arethe following: a knowledge base viewable through objects, production rules, or decision tables; uncertainty handling through non-numerical means; support for report writing; and a self-documenting knowledge base. ACQUIRE is available for Microsoft Windows (3.1 or higher) only.The future for expert/knowledge-based systems development is bright; however, there remain。
英语正反论证作文模板
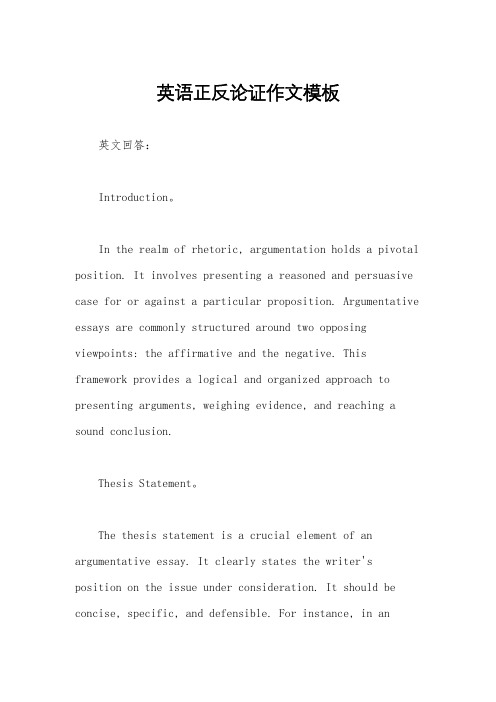
英语正反论证作文模板英文回答:Introduction。
In the realm of rhetoric, argumentation holds a pivotal position. It involves presenting a reasoned and persuasive case for or against a particular proposition. Argumentative essays are commonly structured around two opposing viewpoints: the affirmative and the negative. This framework provides a logical and organized approach to presenting arguments, weighing evidence, and reaching a sound conclusion.Thesis Statement。
The thesis statement is a crucial element of an argumentative essay. It clearly states the writer'sposition on the issue under consideration. It should be concise, specific, and defensible. For instance, in anessay about the benefits of social media, the thesis statement could be: "Social media has a profound impact on society, fostering both positive and negative outcomes."Body Paragraphs。
财务会计概念框架PPT
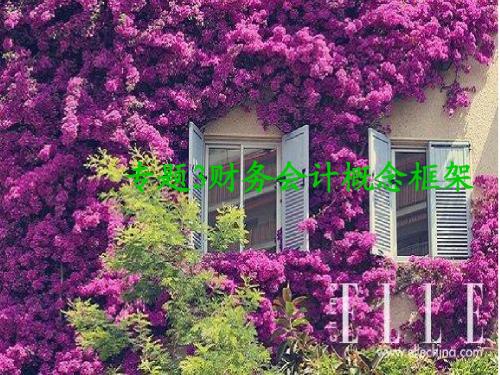
1980 年 5 月 FASB 发表的第二号财务会计概 念公告( SFAC NO.2 )以“财务会计概念 公告”为题作为前言,其中第二段对概念 框架也作了定义:全称财务会计与报告的 框 架 ( conceptual framework for
financial accounting and reporting).
FASB 的概念框架
SFAC No.1 Objective of Financial Reporting by Business Enterprises(Nov. 1978) SFAC No.2 Qualitative Characteristics of Accounting Information(May 1980) SFAC No.3 Elements of Financial Statements( 1980, suspended by No.6) SFAC No.4, Objective of Financial Reporting by NonBusiness Organizations(Nov. 1978) SFAC No. 5 Recognition and Measurement in Financial Statements of Business Enterprises( Dec. 1984) SFAC No.6 Elements of Financial Statements(Dec1985) SFAC No.7 Using Cash Flow Information and Present Value in Accounting Measurements(Feb 2000)
Recognition of the elements of financial statements
一些相似的法律案例英语(3篇)

第1篇Introduction:Legal cases often share similarities in their factual scenarios, legal principles, and outcomes. By analyzing similar legal cases, we can gain insights into how the law is applied and interpreted in different contexts. This article presents a comparative analysis of several legal cases that share common threads, highlighting the similarities and differences in their resolutions.Case 1: Johnson v. Smith (2005)Factual Scenario:Johnson, a tenant, was evicted from his rental property by Smith, the landlord. Johnson claimed that the eviction was illegal due to the lack of proper notice. Smith argued that he had given the required notice as per the lease agreement.Legal Principles:The case revolves around the interpretation of the lease agreement and the provisions regarding eviction notice.Outcome:The court ruled in favor of Johnson, holding that the eviction notice was indeed improper. The court cited the lease agreement, whichspecified a 30-day notice period, and found that Smith had only given a 15-day notice. The court emphasized the importance of adhering to the terms of the lease agreement and protecting the rights of tenants.Case 2: Brown v. Johnson (2008)Factual Scenario:Brown, a shareholder, sued Johnson, the company's president, for breach of fiduciary duty. Brown alleged that Johnson had used company funds for personal gain, thereby violating his fiduciary obligations.Legal Principles:The case focuses on the duty of loyalty and the fiduciary duty owed by company officers and directors to the shareholders.Outcome:The court found Johnson liable for breach of fiduciary duty. The court held that Johnson's use of company funds for personal gain was a clear violation of his fiduciary obligations. The court emphasized the importance of trust and integrity in corporate governance and the need to protect the interests of shareholders.Case 3: Thompson v. Davis (2010)Factual Scenario:Thompson, a driver, was involved in a car accident with Davis, the other driver. Thompson claimed that Davis was driving under the influence of alcohol, which caused the accident. Davis denied the allegations and argued that he was not intoxicated at the time of the accident.Legal Principles:The case deals with the issue of negligence and the duty of care owed by drivers to others on the road.Outcome:The court ruled in favor of Thompson, finding Davis liable for negligence. The court determined that Davis had been driving under the influence of alcohol, as evidenced by his blood alcohol concentration (BAC) level. The court emphasized the importance of exercising due care and adhering to the legal drinking limit while driving.Case 4: Adams v. Washington (2013)Factual Scenario:Adams, a patient, sued Washington, a doctor, for medical malpractice. Adams claimed that Washington had failed to diagnose and treat his condition promptly, resulting in significant harm.Legal Principles:The case revolves around the standard of care owed by healthcare professionals to their patients and the elements of medical malpractice.Outcome:The court found Washington liable for medical malpractice. The court determined that Washington had deviated from the standard of care by failing to diagnose and treat Adams's condition promptly. The court emphasized the importance of healthcare professionals' duty to provide competent and timely medical treatment.Comparison:While these cases share similarities in their factual scenarios andlegal principles, they also present distinct differences in their outcomes. Some of the key similarities and differences are as follows:1. Similarities:- All cases involve disputes between parties.- Each case requires the interpretation of legal principles and the application of relevant statutes.- The outcomes in each case are based on the facts and evidence presented during the trial.2. Differences:- The nature of the disputes varies, ranging from landlord-tenant relationships to corporate governance and medical malpractice.- The legal principles involved in each case differ, depending on the nature of the dispute.- The outcomes vary based on the specific facts and evidence presentedin each case.Conclusion:Similar legal cases provide valuable insights into how the law is applied and interpreted in different contexts. By analyzing these cases,we can identify common threads and patterns in legal reasoning and decision-making. Understanding these similarities and differences can help legal professionals and individuals navigate the complexities of the legal system and make informed decisions.第2篇Introduction:Legal cases often share similarities in their circumstances, legal issues, and outcomes. This comparative analysis aims to examine some similar legal cases that have been widely discussed and debated in the legal community. By comparing these cases, we can gain insights into the evolution of legal principles and the reasoning behind judicial decisions.1. Case 1: Roe v. Wade (1973) vs. Planned Parenthood v. Casey (1992)Both Roe v. Wade and Planned Parenthood v. Casey are landmark Supreme Court cases concerning the right to abortion. In Roe v. Wade, the Court held that a woman's right to an abortion is protected under the Fourteenth Amendment's right to privacy. The Court established a trimester framework for regulating abortion, which allowed states to impose certain restrictions during the first trimester but prohibited any restrictions that would unduly burden a woman's right to an abortion during the second and third trimesters.In Planned Parenthood v. Casey, the Court revisited the issue of abortion rights and upheld the central holding of Roe v. Wade. However, the Court narrowed the scope of the trimester framework and allowed states to impose certain restrictions on abortion, such as parental notification requirements and a 24-hour waiting period, as long as they do not impose an "undue burden" on a woman's right to an abortion.Comparison:Both cases dealt with the same constitutional issue of a woman's right to an abortion. While Roe v. Wade established the framework for regulating abortion, Casey narrowed the scope of that framework. Thereasoning behind the Court's decisions in both cases was centered on the right to privacy and the protection of individual autonomy. However, Casey demonstrated a shift towards a more flexible approach toregulating abortion, allowing states to impose certain restrictions that do not impose an undue burden on a woman's right to an abortion.2. Case 2: Brown v. Board of Education (1954) vs. Parents Involved in Community Schools v. Seattle School District No. 1 (2007)Brown v. Board of Education was a landmark Supreme Court case that declared state laws establishing racial segregation in public schools unconstitutional. The Court held that "separate but equal" wasinherently unequal and violated the Equal Protection Clause of the Fourteenth Amendment.Parents Involved in Community Schools v. Seattle School District No. 1 was a case that dealt with the issue of race-conscious school admissions policies. The Court held that race cannot be used as a factor in assigning students to schools, overturning the precedent set by Brown v. Board of Education in cases involving school integration plans.Comparison:Both cases dealt with the issue of racial segregation in schools. Brown v. Board of Education established the principle that racial segregation in public schools is unconstitutional, while Parents Involved in Community Schools overturned that precedent by holding that race cannot be used as a factor in assigning students to schools. The reasoning behind the Court's decisions in both cases was centered on the Equal Protection Clause of the Fourteenth Amendment. However, the Court's approach to race-conscious policies evolved from a focus on integrating schools to a ban on the use of race in assigning students.3. Case 3: United States v. Nixon (1974) vs. Bush v. Gore (2000)United States v. Nixon was a landmark Supreme Court case involving the issue of executive privilege. The Court held that President Richard Nixon could not claim executive privilege to withhold tape recordings requested by the Watergate Special Prosecutor. The Court emphasized thatexecutive privilege is not absolute and can be overridden by the needfor information in a criminal investigation.In Bush v. Gore, the Supreme Court resolved a controversial dispute over the recount of Florida's electoral votes in the 2000 presidential election. The Court held that the recount process in Florida violated the Equal Protection Clause of the Fourteenth Amendment and stopped the recount, effectively awarding the presidency to George W. Bush.Comparison:Both cases involved issues of executive authority and the interpretation of constitutional provisions. United States v. Nixon dealt with the issue of executive privilege, while Bush v. Gore dealt with the issue of equal protection in the electoral process. The reasoning behind the Court's decisions in both cases was centered on the interpretation of constitutional provisions. However, the Court's approach to executive authority evolved from recognizing the limits of executive privilege to addressing equal protection concerns in the electoral process.Conclusion:The analysis of similar legal cases demonstrates the evolution of legal principles and the reasoning behind judicial decisions. While these cases share similarities in their circumstances and legal issues, they also reflect the changing landscape of constitutional interpretation and the development of legal principles over time. By examining these cases, we can gain a deeper understanding of the complexities of the legal system and the importance of judicial reasoning in shaping our society.第3篇Introduction:Legal cases often share similarities in terms of their legal issues, circumstances, and outcomes. By analyzing similar legal cases, we can gain insights into the evolution of law, the interpretation of legal principles, and the application of judicial reasoning. This essay aims to provide a comparative analysis of some legal cases that sharesimilarities in their core issues, highlighting the key similarities and differences between them.Case 1: Roe v. Wade (1973)This landmark case in the United States concerned the issue of a woman's right to an abortion. The Supreme Court held that a woman's constitutional right to privacy encompasses the right to terminate a pregnancy. The case has been frequently cited and analyzed in subsequent abortion-related cases.Case 2: Planned Parenthood v. Casey (1992)This case was a significant follow-up to Roe v. Wade. The Supreme Court upheld the central holding of Roe but relaxed the strict scrutiny standard for abortion regulations. The Court ruled that states could impose certain restrictions on abortion, as long as they did not impose an "undue burden" on a woman's right to choose.Case 3: Whole Woman's Health v. Hellerstedt (2016)This case dealt with a Texas law that imposed strict regulations on abortion clinics, requiring them to meet the standards of ambulatory surgical centers and imposing restrictions on abortion providers'ability to perform abortions. The Supreme Court struck down the law, holding that it placed an "undue burden" on a woman's right to an abortion.Similarities:1. Core Issue: All three cases deal with the issue of a woman's right to an abortion and the extent to which the state can regulate this right.2. Constitutional Right: Each case involves the interpretation and application of the constitutional right to privacy, particularly as it relates to reproductive rights.3. Undue Burden: The Supreme Court has emphasized the concept of an "undue burden" in evaluating the constitutionality of abortion regulations, which is a central issue in each case.4. Judicial Review: All three cases involve judicial review of state laws, with the Supreme Court ultimately deciding the constitutionalityof the challenged regulations.Differences:1. Legal Standards: While Roe v. Wade established a strict scrutiny standard for abortion regulations, Planned Parenthood v. Casey relaxed this standard, allowing for some restrictions as long as they do not impose an undue burden. Whole Woman's Health v. Hellerstedt further clarified the undue burden standard.2. Clinic Regulations: The regulations in each case differ in their scope and nature. Roe v. Wade did not address clinic regulations, while Planned Parenthood v. Casey and Whole Woman's Health v. Hellerstedtdealt with specific clinic regulations.3. Public Opinion: The level of public opinion regarding abortion has evolved over time, influencing the Court's decisions in each case. Roe v. Wade was decided during a period of increasing acceptance of abortion rights, while Planned Parenthood v. Casey and Whole Woman's Health v. Hellerstedt were decided during a more polarized era.4. Impact on Future Cases: The decisions in each case have had varying impacts on future abortion-related cases. Roe v. Wade laid the groundwork for subsequent cases, while Planned Parenthood v. Casey and Whole Woman's Health v. Hellerstedt have provided more specific guidance on the undue burden standard.Conclusion:The analysis of similar legal cases, such as Roe v. Wade, Planned Parenthood v. Casey, and Whole Woman's Health v. Hellerstedt, revealsthe evolution of legal principles and judicial reasoning regarding abortion rights. While the core issue remains the same, the Court's interpretation of constitutional rights, the nature of regulations, and the impact of public opinion have varied over time. These cases demonstrate the complexity of legal issues and the importance of a thorough understanding of judicial decisions in shaping the law.。
500_计算机网络英文单词

digital versatile disc 数字通用盘 specification 规范 compelling 激发兴趣的 a wealth of大量的 consumer electronics消费电子厂品 www 环球网
第三单元
scripting language脚本语言 browser 浏览器 wed page网页 validate确认,认证 form 表单 Cookie 历史记录 HTML 超文本置标语言 XHTML 可扩展超文本置标语言 Lightweight 轻量的 embed嵌入 compilation 编译 Sun公司 syntax 语法
9.2
Expertise专长 knowledge base知识库 test测试 refine 精炼 refinement精化 heuristic 启发式的 algorithmic 算法的 incremental增加的 shell 外壳 consultation咨询 symbolic 象征 neural network 神经网络 metaknowledge元知识 validate确认
video 视频的 spin-off 有用的副产品 audio cd激光唱片 record album唱片 disk drive 盘驱动器 rewritable 可重写的 overwrite 盖写 personalize个人化 alternative另一种选择 burn烧 eclipse超越 acronym只取首字母的缩写 successor继承者 vhs 家用录像系统 prerecorded预录的 videotape录像带 low-end Winchester disk抵挡温彻斯特盘 cost-effective 成本有效性 audio 音频 encode编码 single-layered,sing-sided单层 advocate提倡者
英语优秀作文解析模板

英语优秀作文解析模板英文回答:Introduction。
An outstanding English essay serves multiple purposes:it demonstrates a deep understanding of the topic, showcases writing proficiency, and effectively communicates ideas to the reader. Mastering the art of essay writing requires a comprehensive approach that encompasses organization, research, analysis, and compelling expression. This essay analysis template provides a structured framework to evaluate the effectiveness of an outstanding English essay.Structure。
Thesis Statement。
A strong thesis statement clearly articulates theessay's central argument or purpose.It should be specific, focused, and debatable.Paragraphs。
Body paragraphs present evidence, analysis, and examples to support the thesis statement.Each paragraph should have a clear topic sentence that develops a specific aspect of the argument.Transitions smoothly connect paragraphs and guide the reader through the essay.Evidence。
如何做一名合格的无神论者作文
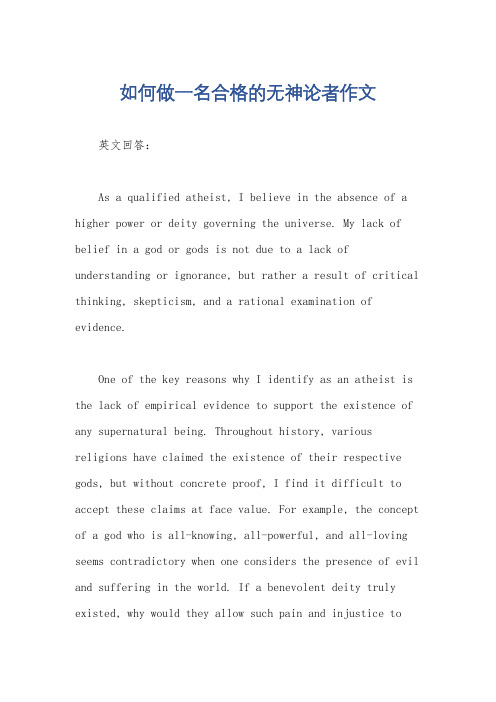
如何做一名合格的无神论者作文英文回答:As a qualified atheist, I believe in the absence of a higher power or deity governing the universe. My lack of belief in a god or gods is not due to a lack of understanding or ignorance, but rather a result of critical thinking, skepticism, and a rational examination of evidence.One of the key reasons why I identify as an atheist is the lack of empirical evidence to support the existence of any supernatural being. Throughout history, various religions have claimed the existence of their respective gods, but without concrete proof, I find it difficult to accept these claims at face value. For example, the concept of a god who is all-knowing, all-powerful, and all-loving seems contradictory when one considers the presence of evil and suffering in the world. If a benevolent deity truly existed, why would they allow such pain and injustice tooccur?Furthermore, the diversity of religious beliefs and the contradictions among them also contribute to my atheism. With so many different faiths claiming to have the ultimate truth, it is impossible for all of them to be correct. This leads me to question the validity of any particular religious doctrine and ultimately reject the idea of a supreme being.In addition, my atheism is also influenced by my understanding of science and the natural world. The scientific method, with its emphasis on observation, experimentation, and evidence-based reasoning, provides a more reliable and consistent framework for understanding the universe than religious dogma. For instance, the theory of evolution by natural selection offers a compelling explanation for the diversity of life on Earth, without the need for a divine creator.Overall, my atheism is not a rejection of spirituality or morality, but rather a rejection of supernaturalexplanations for the mysteries of the universe. I find meaning and purpose in human relationships, personal growth, and contributing to the well-being of others, rather than relying on the guidance of a deity.中文回答:作为一名合格的无神论者,我相信宇宙没有任何高于我们的存在。
功能点的A型-2模糊逻辑框架(IJISA-V5-N3-8)
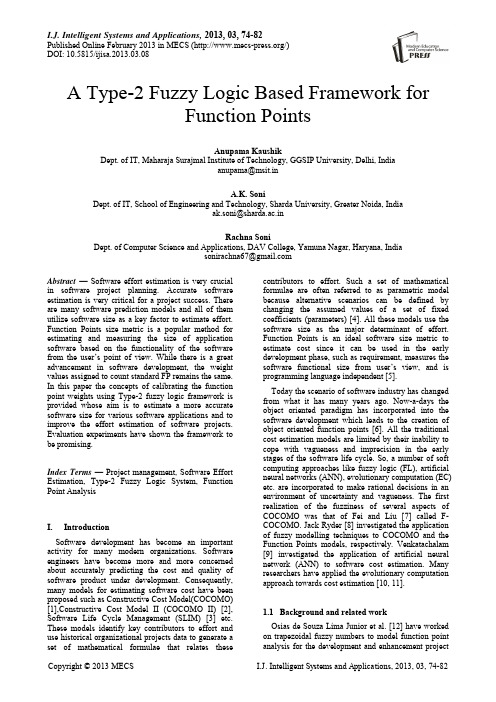
I.J. Intelligent Systems and Applications, 2013, 03, 74-82Published Online February 2013 in MECS (/)DOI: 10.5815/ijisa.2013.03.08A Type-2 Fuzzy Logic Based Framework forFunction PointsAnupama KaushikDept. of IT, Maharaja Surajmal Institute of Technology, GGSIP University, Delhi, Indiaanupama@msit.inA.K. SoniDept. of IT, School of Engineering and Technology, Sharda University, Greater Noida, Indiaak.soni@sharda.ac.inRachna SoniDept. of Computer Science and Applications, DAV College, Yamuna Nagar, Haryana, Indiasonirachna67@Abstract —Software effort estimation is very crucial in software project planning. Accurate software estimation is very critical for a project success. There are many software prediction models and all of them utilize software size as a key factor to estimate effort. Function Points size metric is a popular method for estimating and measuring the size of application software based on the functionality of the software from the user‘s point of view. While there is a great advancement in software development, the weight values assigned to count standard FP remains the same. In this paper the concepts of calibrating the function point weights using Type-2 fuzzy logic framework is provided whose aim is to estimate a more accurate software size for various software applications and to improve the effort estimation of software projects. Evaluation experiments have shown the framework to be promising.Index Terms —Project management, Software Effort Estimation, Type-2 Fuzzy Logic System, Function Point AnalysisI.IntroductionSoftware development has become an important activity for many modern organizations. Software engineers have become more and more concerned about accurately predicting the cost and quality of software product under development. Consequently, many models for estimating software cost have been proposed such as Constructive Cost Model(COCOMO) [1],Constructive Cost Model II (COCOMO II) [2], Software Life Cycle Management (SLIM) [3] etc. These models identify key contributors to effort and use historical organizational projects data to generate a set of mathematical formulae that relates these contributors to effort. Such a set of mathematical formulae are often referred to as parametric model because alternative scenarios can be defined by changing the assumed values of a set of fixed coefficients (parameters) [4]. All these models use the software size as the major determinant of effort. Function Points is an ideal software size metric to estimate cost since it can be used in the early development phase, such as requirement, measures the software functional size from user‘s view, and is programming language independent [5].Today the scenario of software industry has changed from what it has many years ago. Now-a-days the object oriented paradigm has incorporated into the software development which leads to the creation of object oriented function points [6]. All the traditional cost estimation models are limited by their inability to cope with vagueness and imprecision in the early stages of the software life cycle. So, a number of soft computing approaches like fuzzy logic (FL), artificial neural networks (ANN), evolutionary computation (EC) etc. are incorporated to make rational decisions in an environment of uncertainty and vagueness. The first realization of the fuzziness of several aspects of COCOMO was that of Fei and Liu [7] called F-COCOMO. Jack Ryder [8] investigated the application of fuzzy modelling techniques to COCOMO and the Function Points models, respectively. Venkatachalam [9] investigated the application of artificial neural network (ANN) to software cost estimation. Many researchers have applied the evolutionary computation approach towards cost estimation [10, 11].1.1 Background and related workOsias de Souza Lima Junior et al. [12] have worked on trapezoidal fuzzy numbers to model function point analysis for the development and enhancement projectassessment. Ho Leung [13] has presented a case study for evaluation of function points. Finnie et al. [14] provided the combination of machine learning approach with FP. They compared the three approaches i.e. regression analysis, artificial neural networks and case based reasoning using FP as an estimate of software size. The authors observed that both artificial neural networks and case based reasoning performed well on the given dataset in contrast to regression analysis. They concluded that case based reasoning is appealing because of its similarity to the expert judgement approach and for its potential in supporting human judgement. Al-Hajri et al. [15] establish a new FP weight system using artificial neural network. Lima et al. [16] proposed the concepts and properties from fuzzy set theory to extend FP analysis into a fuzzy FP analysis and the calibration was done using a small database comprised of legacy systems developed mainly in Natural 2, Microsoft Access and Microsoft Visual Basic. Yau and Tsoi [17] introduced a fuzzified FP analysis model to help software size estimators to express their judgement and use fuzzy B-spline membership function to derive their assessment values. The weak point of their work is that they use limited in-house software to validate the model. Abran and Robillard‘s empirical study [18] demonstrates the clear relationship between FPA‘s primary component and work-effort. Kralj et al. [19] identified the function point analysis method deficiency of upper boundaries in the rating complexity process and proposed an improved FPA method. Wei Xia et al. [20] proposed a Neuro-Fuzzy calibration approach for function point complexity weights. Their model provided an equation between Unadjusted Function Points and work effort which is used to train the neural network and estimated the effort. Moataz A. Ahmed and Zeeshan Muzaffar [4] provided an effort prediction framework that is based on type-2 fuzzy logic to allow handling imprecision and uncertainty present in the effort prediction. Mohd. Sadiq et al. [21] developed two different linear regression models using fuzzy function point and non fuzzy function point in order to predict the software project effort.The above researches have concluded that the combination of soft computing approaches and the traditional cost estimation models yields a more accurate prediction of software costs and effort. All the earlier work on software cost estimation using fuzzy logic incorporated type-1 or type-2 fuzzy framework for effort prediction. This paper proposes an improved FPA method by calibrating the function point‘s weight using type-2 fuzzy logic framework.1.2 Function Point Analysis: A short description Function point analysis is a process used to calculate the software size from the user‘s point of view, i.e. on the basis of what the user requests and receives in return from the system. Allan J Albrecht [22] of IBM proposed Function Point Count (FPC) as a size measure in the late 1970s. Albrecht had taken up the task of arriving at size measures of software systems to compute a productivity measure that could be used across programming languages and development technologies. The current promoter of Albrecht‘s function point model is the International Function Point User‘s Group (IFPUG). IFPUG evolves the FPA method and periodically releases the Counting Practices Manual for consistent counting of function points across different organizations. In FPA, a system is decomposed into five functional units: Internal Logical Files (ILF), External Interface Files (EIF), External Inputs (EI), External Outputs (EO) and External Inquiry (EQ). These functional units are categorized into data functional units and transactional function units. All the functions do not provide the same functionality to the user. Hence, the function points contributed by each function varies depending upon the type of function (ILF, EIF, EI, EO or EQ) and complexity (Simple, Average or Complex) of the function. The data functions complexity is based on the number of Data Element Types (DET) and number of Record Element Types (RET). The transactional functions are classified according to the number of file types referenced (FTRs) and the number of DETs. The complexity matrix for all the five components is given in Table 1, Table 2 and Table 3. Table 4 illustrates how each function component is then assigned a weight according to its complexity.The actual calculation process of FPA is accomplished in three stages: (i) determine the unadjusted function points (UFP); (ii) calculate the value adjustment factor (VAF); (iii) calculate the final adjusted function points.The Unadjusted Function Points (UFP) is calculated using ―(1)‖, where W ij are the complexity weights and Z ij are the counts for each function component.∑∑ (1) The second stage, calculating the value adjustment factor (VAF), is derived from the sum of the degree of influence (DI) of the 14 general system characteristics (GSCs). The DI of each one of these characteristics ranges from 0 to 5 as follows: (i) 0 – no influence; (ii) 1 –incidental influence; (iii) 2 –moderate influence; (iv) 3 – average influence; (v) 4 – significant influence; and (vi) 5 – strong influence.The general characteristics of a system are: (i) data communications; (ii) distributed data processing; (iii) performance; (iv) heavily used configuration; (v) transaction rate; (vi) online data entry; (vii) end-user efficiency; (viii) on-line update; (ix) complex processing; (x) reusability; (xi) installation ease; (xii) operational ease; (xiii) multiple sites; and (xiv) facilitate change. VAF is then computed using ―(2)‖:∑ (2)x i is the Degree of Influence (DI) rating of each GSC. Finally, the adjusted function points are calculated as given in ―(3)‖.(3)Table 1: Complexity Matrix of ILF/EIFTable 2: Complexity Matrix of EITable 3: Complexity Matrix of EO/EQTable 4: Functional Units with weighting factorsII.Type 2 Fuzzy Logic SystemsFuzzy Logic is a methodology to solve problems which are too complex to be understood quantitatively. It is based on fuzzy set theory and introduced in 1965 by Prof. Zadeh in the paper fuzzy sets [23]. It is a theory of classes with unsharp boundaries, and considered as an extension of the classical set theory [24]. The membership µA(x) of an element x of a classical set A, as subset of the universe X, is defined by:µA(x) = {That is, x is a member of set A (µA (x) = 1) or not (µA (x) = 0). The classical sets where the membership value is either zero or one are referred to as crisp sets. Fuzzy sets allow partial membership. A fuzzy set A is defined by giving a reference set X, called the universe and a mapping;µA : X []called the membership function of the fuzzy set A µA(x), for x X is interpreted as the degree of membership of x in the fuzzy set A. A membership function is a curve that defines how each point in the input space is mapped to a membership value between 0 and 1. The higher the membership x has in the fuzzy set A, the more true that x is A. The membership functions (MFs) may be triangular, trapezoidal, Gaussian, parabolic etc.Fuzzy logic allows variables to take on qualitative values which are words. When qualitative values are used, these degrees may be managed by specific inferential procedures. Just as in fuzzy set theory the set membership values can range (inclusively) between 0 and 1, in fuzzy logic the degree of truth of a statement can range between 0 and 1 and is not constrained to the two truth values {true, false} as in classic predicate logic.Fuzzy Logic System (FLS) is the name given to any system that has a direct relationship with fuzzy concepts. The most popular fuzzy logic systems in the literature may be classified into three types [25]: pure fuzzy logic systems, Takagi and Sugeno‘s fuzzy system and fuzzy logic system with fuzzifier and defuzzifier also known as Mamdani system. As most of the engineering applications use crisp data as input and produce crisp data as output, the Mamdani system [26] is the most widely used one where the fuzzifier maps crisp inputs into fuzzy sets and the defuzzifier maps fuzzy sets into crisp outputs.Zadeh [27], proposed more sophisticated kinds of fuzzy sets, called type-2 fuzzy sets (T2FSs). A type-2 fuzzy set lets us incorporate uncertainty about the membership function into fuzzy set theory. In order to symbolically distinguish between a type-1 fuzzy set and a type-2 fuzzy set, a tilde symbol is put over the symbol for the fuzzy set; so, A denotes a type-1 fuzzy set, whereas à denotes the comparable type-2 fuzzy set. Mendel and Liang [28, 29] characterized T2FSs using the concept of footprint of uncertainty (FOU), and upper and lower MFs. To depict the concept, let us consider type-1 gauss MF shown in ―Fig. 1‖.As can be seen from the figure type-1 gaussian membership function is constrained to be in between 0 and 1 for all x X, and is a two dimensional function. These types of membership don‘t carry any uncertainty. There exists a clear membership value for every input data point.If the Gaussian function in ―Fig.1‖ is blurred ―Fig. 2‖can be obtained. The FOU represents the bounded region obtained by blurring the boundaries of type-1 MF. The upper and lower MFs represent the upper and lower boundaries of the FOU, respectively. In this case, for a specific input value, there is no longer a single certain value of membership; instead the MF takes on values wherever the vertical line intersects the blur. Those values do not have to be all weighted the same; hence, an amplitude distribution can be assigned to those points. Doing this for all input values x, a three dimensional MF is created, which is a type-2 MF. In this, the first two dimensions allow handlingimprecision via modelling the degree of membership of x; while the third dimension allows handling uncertainty via modelling the amplitude distribution of the degree of membership of x. Here also, like in type-1 MFs the degree of membership along the second dimension and the amplitude distribution values along the third dimension is always in the interval [0, 1]. Clearly, if the blur disappears; then a type-2 MF reduces to a type-1 MF.A general architecture of type-2 fuzzy logic system (T2FL) as proposed by Mendel is depicted in ―Fig. 3‖.Fig. 1: A Gaussian Type-1 membership functionFig. 2: A Gaussian Type-2 membership functionFig. 3: A typical type-2 fuzzy logic system [29]Table 5: Example on FP complexity classificationT2FL systems contain five components –rules, fuzzifier, inference engine, type reducer, and defuzzifier. Rules are the heart of a T2FL system, and may be provided by experts or can be extracted from numerical data. These rules can be expressed as a collection of IF-THEN statements. The IF part of a rule is its antecedent, and the THEN part of the rule is its consequent. Fuzzy sets are associated with terms that appear in the antecedents or consequents of rules, and with inputs to and output of the T2FL system. The inference engine combines rules and gives mapping from input type-2 fuzzy sets to output type-2 fuzzy set. The fuzzifier converts inputs into their fuzzy representation. The defuzzifier converts the output of the inference engine into crisp output. The type reducer transforms the type-2 fuzzy output set into type-1 fuzzy set to be processed by the defuzzifier. A T2FL system is very similar to a T1FL system; the major difference being that the output processing block of T1FL system is just a defuzzifier while the output processing block of a T2FL system contains the type reducer as well. III.Problem Description and AnalysisIn cost estimation process, the primary input is the software size and the secondary inputs are the various cost drivers. There is a significant relationship between the software size and cost. There are mainly two types of software size metrics: lines of code (LOC) and Function Point (FP). Size estimation is best done when there is complete information about the system; but this is not available till the system is actually built. The challenge for the estimator is therefore to arrive at a reasonable estimate of the size of the system with partial information.LOC is usually not available until the coding phase, so FP has gained popularity because it can be used at an earlier stage of software development.In our work, we are using type-2 based fuzzy logic approach to calibrate the function point weight values which provides an improvement in the software size estimation process. There are 15 parameters in the FP complexity weight system to calibrate. These parameters are low, average and high values of External Inputs, External Outputs, Internal Logical Files, External Interface Files and External Inquiries respectively. A fuzzy based approach is chosen since it can capture human‘s judgement with ease and instead of giving an exact number to all 15 function points parameters we can define fuzzy linguistic terms and assign a fuzzy set within numeric range. This provides an ability to cope up with the vagueness and imprecision present in the earlier stages of software development.In Function Point Analysis (FPA) method each component is classified to a complexity level determined by the number of its associated files such as DET, RET or FTR as given in Table 4. If we determine the FPA complexity of a particular software application, in some cases it may not correctly reflect the complexity for its components.Table 5 shows a software project with three EIF‘s A, B and C. According to the complexity matrix, A and B are classified as having the same complexity and are assigned the same weight value of 10. However, A has 19 more DET than B and is certainly more complex. But both of them are assigned the same complexity. Also, EIF C is having only one DET less than EIF B and it is classified as average and assigned a weight value of 7. From the above example it is concluded that there is a huge scope of improvement in the FPA complexity classification. Processing the number of FP component associated files such as DET, RET and FTR using fuzzy logic can provide an exact complexity degree.IV.Fuzzy Logic calibration to improve FPAType-2 fuzzy inference system is developed for all the five FPA components (ILF, EIF, EI, EO, EQ) using the Mamdani approach. We define three new linguistic terms: small, medium and large, to express the inputs qualitatively. Also we use linguistic terms: simple, average and complex for the output. To fuzzify the inputs and outputs, we define fuzzy sets to represent the linguistic terms [30]. The fuzzy membership grade is captured through the membership functions of each fuzzy set. The inputs and outputs are represented using gaussian igaussstype2 membership which is represented in ―Fig. 4‖. It has certain mean m, and an uncertain standard deviation that takes on values in [σ1, σ2]. The shaded area represents the FOU. Using interval type-2 Gaussian MF‘s makes it easier to build T2FL systems since the mathematics behind the corresponding inferential procedures and training algorithms are less complicated [29]. ―Fig.5 (a)‖and ―Fig.5 (b)‖ shows how the inputs of EIF are assigned the membership functions and represented using linguistic variables of fuzzy sets. ―Fig. 6‖ depicts the output of EIF using membership functions. After representing the inputs and output of EIF using membership functions nine fuzzy rules are defined using rule editor based on the original complexity matrices and illustrated in Table 6. Each rule has two parts in its antecedent linked with an ‗AND‘ operatorand one part in its consequence. These fuzzy rules define the connection between the input and output fuzzy variables. A fuzzy rule has the form: IF <Antecedent> THEN <Consequent>, where antecedent is a compound fuzzy logic expression of one or more simple fuzzy expressions connected with fuzzy operators; and the consequent is an expression that assigns fuzzy values to output variables. The inference system evaluates all the rules of the rule base and combines the weights of the consequents of all relevantrules in one fuzzy set using the aggregate operation. Finally, the output fuzzy set is defuzzified to a crisp single number.Fig. 4: FOU for Gaussian MFFig. 5 (a): Input fuzzy set DET for EIFFig. 5 (b): Input fuzzy set RET for EIFFig. 6: Output fuzzy set Complexity for EIFTable 6: Truth table of fuzzy logic rule setFig. 7: Type-2 Fuzzy Inference process of Function Points Model Table 7: Calibration using type-2 fuzzy logicAn example of the complete fuzzy inference process is shown in ―Fig. 7‖. Input values are set to DET 51 and RET 5. These are represented using the antecedent part of the fuzzy rules. Finally, the consequent part isdefuzzified and the output is achieved as a single value of 7.63.A fuzzy logic system for each FPA element (ILF, EIF, EI, EO, EQ) is constructed. A fuzzy complexity measurement system that takes into account all five Unadjusted Function Points function components is built after the fuzzy logic system for each function component is established as shown in ―Fig. 8‖. The calibrated values for EIF A, EIF B and EIF C is listed in Table 7 and it is found that these calibrated weight values are more convincing than the original weight values.Fig. 8: Fuzzy complexity measurement system for Type-2 Fuzzyfunction points modelTable 8: Calculation of t2UFFP and UFP for ILFV.Experimental Methodology and ResultsWe have conducted some experiments to develop a type-2 fuzzy system for function points analysis using our framework as depicted in ―Fig. 8‖. Our model has been implemented in Matlab(R2008a). As it is the case with validating any prediction model, real industrial data necessary to use our framework to develop and tune the parameters of prediction models were not available. To get around this data scarcity problem for the sake of showing the validity of our framework for the industry where organizations have their own data available, we generated artificial datasets consisting of 20 projects. A complexity calculation for all the five components for each project is done using the type-2 fuzzy framework. The Tables (8, 9, 10, 11, 12) lists the complexity values for all the five components for the first project using type-2 fuzzy framework (t2UFFP) and conventional method i.e.UFP.Using ―(1)‖ total unadjusted function points from the type-2 technique and the conventional technique is calculated and listed in Table 13. It is found that the type-2 technique is at par than the conventional technique.Table 9: Calculation of t2UFFP and UFP for EIFTable 10: Calculation of t2UFFP and UFP for EITable 11: Calculation of t2UFFP and UFP for EOTable 12: Calculation of t2UFFP and UFP for EQTable 13: Comparison of t2UFFP and UFPTable 14: Comparison of type-2 fuzzy FP and conventional FPIn order to compute the value of the conventional function point and type-2 fuzzy function point, we have treated all the 14 general system characteristics as average. Using ―(2)‖and ―(3)‖VAF and FPA is calculated and listed in Table 14.From the above results it is concluded that the calibrated function points using type-2 fuzzy yields better results than conventional function points.VI.ConclusionsFP as a software size metric is an important topic in the software engineering domain. The use of type2 fuzzy logic to calibrate FP weight values further improves the estimation of FP. This in turn will improve the cost estimation process of software projects. Empirical evaluation has shown that T2FL is promising. But there are potentials for improvements when the framework is deployed in practice. As all the experiments were conducted using artificial datasets, a need to evaluate the prediction performance of the framework on real data still persists. Some future work can be directed towards developing inferential procedures using various other membership functions present in type-2 fuzzy systems. This work can also be extended using Neuro Fuzzy approach. AcknowledgementThe authors would like to thank the anonymous reviewers for their careful reading of this paper and for their helpful comments.References[1] B.W. Boehm. Software Engineering Economics.Prentice Hall, Englewood Cliffs, NJ, 1981.[2] B. Boehm, B. Clark, E. Horowitz, R. Madachy, R.Shelby, C. Westland. Cost models for future software life cycle processes: COCOMO 2.0.Annals of Software Engineering, 1995.[3]L.H. Putnam. A general empirical solution to themacro software sizing and estimation problem.IEEE Transactions on Software Engineering, vol.4, 1978, pp 345-361.[4]Moataz A. Ahmed, Zeeshan Muzaffar. Handlingimprecision and uncertainty in software development effort prediction: A type-2 fuzzylogic based framework. Information and Software Technology Journal. vol. 51, 2009, pp. 640-654. [5]Function Point Counting Practices Manual, fourthedition, International Function Point Users Group, 2004.[6]G. Antoniol, C. Lokan, G. Caldiera, R. Fiutem. Afunction point like measure for object oriented software. Empirical Software Engineering. vol. 4, 1999, pp. 263-287.[7]Fei. Z, X. Liu. f-COCOMO-Fuzzy ConstructiveCost Model in Software Engineering. Proceedings of IEEE International Conference on Fuzzy System. IEEE Press, New York, 1992, pp. 331-337.[8]J. Ryder. Fuzzy Modeling of Software EffortPrediction. Proceedings of IEEE Information Technology Conference. Syracuse, NY, 1998. [9] A.R. Venkatachalam. Software Cost Estimationusing artificial neural networks. Proceedings of the International Joint Conference on Neural Networks, 1993, pp. 987-990.[10]K.K. Shukla. Neuro-genetic Prediction ofSoftware Development Effort. Journal of Information and Software Technology, Elsevier.vol. 42, 2000, pp. 701-713.[11]Alaa.F.Sheta. An Estimation of the COCOMOmodel parameters using the genetic algorithms for the NASA project parameters. Journal of Computer Science, vol. 2, 2006, pp.118 -123. [12]Osias de Souza Lima Junior, Pedro PorfirioMuniaz Parias, Arnaldo Dias Belchior. A fuzzy model for function point analysis to development and enhancement project assessement. CLEI Electronic Journal, vol. 5, 1999, pp. 1-14.[13]Ho Leung, TSOI. To evaluate the function pointanalysis: A case study. International Journal of computer, Internet and management vol. 13, 2005, pp. 31-40.[14]G.R. Finnie, G.E. Wittig, J.M. Desharnais. Acomparison of software effort estimation techniques: using function points with neural networks, case-based reasoning and regression models. Journal of Systems Software, Elsevier.vol. 39, 1977, pp. 281-289.[15]M.A. Al-Hajri, A.A.A Ghani, M.S. Sulaiman,M.H. Selamat. Modification of standard function point complexity weights system. Journal of Systems and Software, Elsevier,vol. 74, 2005, pp.195-206.[16]O.S. Lima, P.F.M. Farias, A.D. Belchior. Fuzzymodeling for function point analysis. Software Quality Journal, vol. 11, 2003, pp. 149-166. [17]C. Yau, H. L. Tsoi. Modelling the probabilisticbehavior of function point analysis. Journal ofInformation and Software Technology, Elsevier.vol. 40, 1998, pp. 59-68.[18]A. Abran, P. Robillard. Function Points Analysis:An empirical study of its measurement processes.IEEE Transactions on Software Engineering, vol.22, 1996, pp.895-910.[19]T. Kralj, I. Rozman, M. Hericko, A. Zivkovic.Improved standard FPA method- resolving problems with upper boundaries in the rating complexity process. Journal of Systems and Software, Elsevier, vol. 77, 2005, pp. 81-90. [20]Wei Xia, Luiz Fernando Capretz, Danny Ho,Faheem Ahmed. A new calibration for function point complexity weights. Journal of Information and Software Technology, Elsevier. vol. 50, 2008 pp.670-683.[21]Mohd. Sadiq, Farhana Mariyam, Aleem Ali,Shadab Khan, Pradeep Tripathi. Prediction of Software Project Effort using Fuzzy Logic.Proceedings of IEEE International Conference on Fuzzy System, 2011, pp. 353-358.[22]A. Albrecht. Measuring application developmentproductivity. Proceedings of the Joint SHARE/GUIDE/IBM Application Development Symposium, 1979, pp. 83-92.[23] L. A. Zadeh. Fuzzy Sets. Information and Control,vol. 8, 1965, pp. 338-353.[24]M. Wasif Nisar, Yong-Ji Wang, Manzoor Elahi.Software Development Effort Estimation using Fuzzy Logic – A Survey. Fifth International Conference on Fuzzy Systems and Knowledge Discovery, 2008, pp 421-427.[25]L. Wang. Adaptive Fuzzy System and Control:Design and Stability Analysis. Prentice Hall, Inc., Englewood Cliffs, NJ 07632, 1994.[26]E.H. Mamdani. Applications of fuzzy algorithmsfor simple dynamic plant. Proceedings of IEEE, vol. 121, 1974, pp. 1585-1588.[27]L. A. Zadeh. The Concept of a Linguistic Variableand Its Application to Approximate Reasoning–1. Information Sciences, vol. 8, 1975, pp. 199-249.[28]J.M. Mendel, Q. Liang. Pictorial comparison ofType-1 and Type-2 fuzzy logic systems.Proceedings of IASTED International Conference on Intelligent Systems and Control, Santa Barbara, CA, October 1999.[29]J.M. Mendel. Uncertain Rule-Based Fuzzy LogicSystems, Prentice Hall, Upper Saddle River, NJ 07458, 2001.[30]E.H. Mamdani. Application of fuzzy logic toapproximate reasoning using linguistic synthesis.IEEE transactions on computers, vol. 26, 1977, pp.1182-1191. Anupama Kaushik is an Assistant Professor at Maharaja Surajmal Institute of Technology, New Delhi, India. Her research area includes Software Engineering, Object Oriented Software Engineering and Soft Computing.Dr. A.K Soni has done his Ph.D. and M.S.(Computer Science) both from Bowling Green State University in Ohio, USA . He is the Professor and Head, Department of Information Technology, Sharda University, Greater Noida, India. His research area includes Software Engineering, Datamining, Database Management Systems and Object Oriented Systems.Dr. Rachna Soni did her M. Phil from IIT Roorkee and Ph.D. from Kururukshetra University, Kurukshetra. She is the Associate Professor and Head, Dept. of Computer Science and Applications, D.A.V. College, Yamunanagar, India. Her area of interest includes Software Risk Management, Project Management, Requirement Engineering, Simulation and Component based Software Engineering.。
- 1、下载文档前请自行甄别文档内容的完整性,平台不提供额外的编辑、内容补充、找答案等附加服务。
- 2、"仅部分预览"的文档,不可在线预览部分如存在完整性等问题,可反馈申请退款(可完整预览的文档不适用该条件!)。
- 3、如文档侵犯您的权益,请联系客服反馈,我们会尽快为您处理(人工客服工作时间:9:00-18:30)。
A Case-Based Reasoning Framework for EnterpriseModel Building,Sharing and ReusingYun-Heh Chen-Burger and David Robertson and Jussi StaderAbstract.Enterprise model development is essentially a labour-intensive exercise.Human experts depend heavily on priorexperience when they are building new models making it a naturaldomain to apply Case Based Reasoning techniques.Through theprovision of model building knowledge,automatic testing anddesign guidance can be provided by rule-based facilities.Exploringthese opportunities requires us not only to determine which formsof knowledge are generic and therefore re-usable,but also how thisknowledge can be used to provide useful model building support.This paper presents our experiences in identifying and classifyingthe knowledge which exists in IBM’s BSDM Business Models andapplying AI techniques,CBR and Rule-Based reasoning togetherwith a symbolic simulator,to provide more complete supportthroughout the enterprise model development life cycle.Key-words Enterprise Modelling,Model Development Life Cycle,Case Based Reasoning,Business Modelling,Process Modelling,Knowledge Management,BSDM,Formal Method.1IntroductionThe main task of BSDM’s Business Modelling is to identify two con-ceptual components:entities and dependencies.Entities are thingsthat a business needs to manage and dependencies are the relation-ships between these things.Certain kinds of scenarios or relation-ships between entities are common to many businesses.Hence,onewould expect that the corresponding BSDM Business Model mapsreflect these commonalities.In practice,IBM provides a catalogue of such generic entity mod-els[8]:some of them are standard and example models from themethod and some of them were specifically developed for selectedindustries.Provided with these generic models,BSDM practition-ers help clients build their business model by using this informationimplicitly or explicitly.For BSDM consultancy,King[9]suggestedthree possible ways of re-using generic/known models when address-ing a new problem domain.Back-Pocket Approach:the clients are made aware of the existenceof these generic models,but they are only used to support con-sultancy.The client will see little or none of the generic model.Aconsultant keeps these generic models at the back of his/her mindand tailors them to the clients’special requirements.formalised to provide coherent and comprehensive support through-out the model development life cycle .It considers two issues:is such knowledge generic and reusable,and how can this knowledge be used to provide automatic support.The paper first describes how Case Based Reasoning techniques can be used to provide a common platform for knowledge sharing.It then presents to which extent this knowledge can be formalised and provide assistance for model build-ing activities.2The Modelling Support Frameworkof a business model development.Figure 1.Architecture of Generic Model AdvisorFigure 1shows the modelling framework which provides auto-matic facilities to support the iterative plan-build-test-refine model-ling development life cycle as shown in Figure 2.Figure 2.The Plan-Build-Test-Refine development cycleTwo integrated knowledge based support tools,Generic Model Advisor(GMA)and Knowledge Based Support Tool for Business Modelling(KBST-BM),have been built.Since a BSDM’s business model is organised and presented in views and diagrams,these are the ”units”that GMA stores and retrieves.GMA identifies and assigns indices (features which characterise a model)to the problem,i.e.the user-defined BSDM model.These indices,together with the embed-ded domain knowledge,in our case the Entity Conceptual Hierarchy and Match Rules ,are passed to the pattern matching algorithm which compares the indices of the problem and those of the generic modelsin the Generic Model Library to retrieve a set of reference models which exhibit similar characteristics to the input model.At this stage the retrieved similar generic models are not yet ex-amined to determine which is a better match for the current problem.For such a comparison,GMA provides a flexible Similarity Assess-ment Function which enables the deployment of a built-in heuristic method or the users can dynamically make up their own evaluation method to explore specific matches based on the identified indices of the model.The best matching case,according to the chosen similarity assess-ment method and an analysis report of similarities and differences between the user model and the retrieved reference model together with suggestions about how to eliminate the causes of the differences,are given to the user.The user can then read the report and/or ask the system to present a different matching result for another generic model.Matches are shown in the descending order of their scores in the chosen similarity assessment method.A summary of all of the matches shown to the user is produced separately which records the similarity measurements of each match to give the user an overview of all possible mappings and to allow revisiting of selected generic models.A user-defined model may be matched with more than one generic models.The user can choose to modify his/her model and repeat the above modelling cycle as a part of an iterative process.If the user has decided to use the reference model as a basis to generate a new model,the user can export the chosen reference model from the lib-rary.At any stage of the model development,the user can choose to use the verification and validation facilities provided by KBST-BM to check for the completeness,soundness and appropriateness of the built model.When the user is sufficiently satisfied with his/her model,he/she can retain this new model,i.e.write it back to GMA,by firstly gen-eralising the new model,verifying and validating the generalised model using the integrated tool KBST-BM ,and then storing the new generic model back to the Generic Model Library .The Case Based Reasoning Cycle is now completed,and GMA’s knowledge can be enriched and evolved through time via the inclusion of newly ac-quired knowledge during operations.GMA does not provide an auto-matic adaptation facility for two reasons.First,there is no absolute standard which fits all businesses in determining whether or not a par-ticular design is the most appropriate one for a business.Secondly,although common practices are shared by many businesses,business models are in general organisation-dependent and building a good model requires understanding of the organisation’s operation and a consensus within the organisation which may not be available or formalisable due to the size and nature of the required knowledge[4].Both issues have to be resolved before high quality automatic adapt-ation can be provided.The inner KBST-BM system box in the Figure 1illustrates how KBST-BM can assist in completing the CBR cycle .It provides an in-dependent verification and validation (V&V)facilities (from the user)and is included in the “Test”activity in the standard model develop-ment process shown in Figure 2.This V&V approach and implement-ation details of KBST-BM are given in [3].3Indexing,Matching and Similarity AssessmentIndices are features which can be used to distinguish models in the case memory and to find appropriate matches between a given prob-lem and previous models.In the context of a BSDM business model,these distinguishing characteristics are embedded in the semantics ofentities,the architecture of a business model,and the business area that a model describes.Simply comparing the graphical representation of business mod-els is not sufficient.For example,drawing an existing model upside-down does not make it a different model,the semantics of the inter-relationships (dependencies)between entities must be taken into account.Furthermore,business contextual similarities may be dis-guised.For instance,if a business model is a more elaborated or spe-cialised version of another one (or vice versa),then these two models normally will not have the same architecture (e.g.one may expand parts of the model in some areas),and often they do not share the same entities (ing domain specific vocabularies instead).How-ever,because they are essentially describing the similar business op-erations,it will be useful to refer one to the other.To be able to make meaningful comparisons between BSDM mod-els,one must have an integral understanding of the business context which is described in both the architecture of a model as well as the business context that each entity represents.We capture part of this context through typing of entities via a concept hierarchy.3.1Entity Conceptual Hierarchy (ECH)BSDM provides Entity Families which provide entities in groups ac-cording to where and how they can be used in a business model.BSDM modellers use Entity Families as a starting point when trying to identify entities for a new model.They also use it as a guideline to check the architecture of the model.We organise information given in the Entity Families in a taxonomic hierarchy,called the Entity Con-ceptual Hierarchy.Figure 3.A Part of Entity Conceptual Hierarchy (ECH)Figure 3shows a screen shot from GMA which captures a part of the Entity Conceptual Hierarchy which contains the suggested en-tities for the top layer (layer 1)of a BSDM business model.Two types of classes have been used to describe entities:the shaded rect-angular boxes represent the Abstract Entity Types ,and the clear rect-angular boxes represent the Concrete Entity Types .Abstract EntityTypes provide a structure to allocate conceptual categories and nor-mally describe more “general”concepts.Concrete Entities present more specialised concepts and include entities which are used in real business models (as opposed to a generalised model).An arrow from entity B to entity A indicates an is-a relationship from B to A,i.e.B is-a A.The Entity Conceptual Hierarchy captures the semantics of all of the entities (in the user and reference models)as well as the relation-ships between them and it can be used to identify and match similar entities used in the user and reference models.3.2Case Retrieving and Similarity AssessmentThe Pattern Matching Algorithm compares the contextual and archi-tecture information of the given user model with that of all of the ref-erence models stored in the Generic Model Library .Several types of information is taken into account.Do these models describe a similar business area?Are they capturing similar concepts?Do they follow similar business rules?The contextual and architecture information is stored in the business area,view ,links,dependencies ,and in the entities .Provided with knowledge embedded in ECH ,one can now match views,dependencies and entities to determine if two different mod-els are sufficiently similar.To match entities,for instance,entities which have the same name in both user and reference models pro-duce a positive match.However,similar but variant entities (sibling relationships in the ECH ),or “stream-line”specialisations (e.g.par-ent and child,or grandparent and grandchild relationships)may also produce a positive match.When deciding which is a better match between entities,the closer the relationship is between the two entit-ies on the ECH ,the better quality of a match it is.A user model may include several generic models.On the other hand,a generic model may include or partially overlap with the user model.Figure 4shows the possibilities how a user model may be mapped to a generic model.UCASE I CASE I CASE I IIICASE IV GGEquivalent CASE VUser model is included in the Generic Model GUGUG GUGUGUser model is partially overlapping with the generic modelCASE VI User model is not included in the generic model, but the generic model is fully included in the User ModelU U UCASE VIICASE VIIIFigure 4.Possible Matching between User Models and Generic ModelsAs our aim is to seek for the best or better match,naturally a 100%match is always given the highest priority,therefore CASE I.The second preference goes to a match in which an user model is fully included by the selected generic model,hence CASE II and III.How-ever,CASE II is superior than CASE III because its generic model is more similar to the user model:it has a smaller difference compared between the two models.When a user model is not fully covered,we prefer a match where the user model has a better coverage from the selected generic model, hence CASE IV is superior to CASE V,VI and VII which are all more superior than CASE VIII.In the case of V,VI and VII where the coverage of commonality of the user model are similar,the qual-ity of the matched generic model should be taken into account,i.e.a generic model which is more similar to the user mode should be pre-ferred.Since the generic model in CASE V is entirely included in the user model,it is the most similar(or relevant)one to the user model, CASE VI is in second place,and CASE VII is the least similar one to the user model since it has a comparatively smaller common portion with the user model.Based on our preferences,five discriminating criteria are identi-fied:the matching result of the captured business areas,the match-ing ratio of links(dependencies)in the selected reference model,the matching ratio of entities in the selected reference model,the match-ing ratio of links(dependencies)in the user model and the matching ratio of entities in the user model.HEURISTIC SIMILARITY ASSESSMENT FUNCTIONGiven two matches, X and Yif match-view(X) > match-view(Y) then SELECT Xelse if match-view(X) = match-view(Y)) andmatch-data-link(X) > match-data-link(Y) then SELECT Xelse if match-view(X) = match-view(Y)) andmatch-data-link(X) = match-data-link(Y) andmatch-data-entity(X) > match-data-entity(Y) then SELECT Xelse if match-view(X) = match-view(Y)) andmatch-data-link(X) = match-data-link(Y) andmatch-data-entity(X) = match-data-entity(Y) andmatch-case-link(X) > match-case-link(Y) then SELECT Xelse if match-view(X) = match-view(Y)) andmatch-data-link(X) = match-data-link(Y) andmatch-data-entity(X) = match-data-entity(Y) andmatch-case-link(X) = match-case-link(Y) andmatch-case-entity(X) > match-case-entity(Y) then SELECT Xelse SELECT YFigure5.The Heuristic Similarity Evaluation FunctionFigure5shows the heuristic evaluation method provided by GMA. It provides a means to use the evaluation criteria in selecting a bet-ter model which complies with the preference order demonstrated earlier.This method produces good results using our test data(see Section4).Alternatively,the user can dynamically design their own evaluation methods using Weighted City-Block evaluation function based on the above criteria,if they wish to explore specific aspects of models in the library.4EvaluationFor evaluation purposes,we obtained a variety of BSDM models from different domains.Part of a real industrial model which was de-veloped by an international automobile company.3One generic busi-ness model for small and medium-sized restaurant was developed based on interviews of three independent family restaurant(ex-)owners to enlarge our testing base.We also captured example and standard models from BSDM and stored them in our Generic ModelKBST-BM integrates with GMA together provide an adequate framework for CBR,i.e.automatic indexing input data,retrieving relevant cases from library,comparing and analysing input with se-lected cases,revising cases for current problem,verifying and val-idating input,and retaining the new inputs for future reference.This allows us to use the larger KBST-BM BSDM modelling environment in the adaptation phase of the CBR cycle.We tested this route using the automobile and restaurant models.5ConclusionSuccessful business model development requires both methodolo-gical and application domain knowledge and experience.Unfortu-nately,few people possess all of these capabilities.Our studies of applying CBR and Rule-Based techniques which are based on a co-herent underlying formal method shows how model building know-ledge can be obtained,reused and used to provide automatic verific-ation and validation facilities.We believe that with this support we are able to enhance the level of knowledge sharing,and ability of problem solving.More importantly,it adds to our understanding of how this sort of seemingly informal method canfit into parts of the design lifecycle which require formal models. REFERENCES[1]Klaus-Dieter Althoff,Eric Auriol,Ralph Barletta,and Michel Manago,An AI Perspectives Report:A Review of Industrial Case-Based Reas-oning Tools,An AI Perspective Report,AI Intelligence,P.O.Box95, Oxford OX27XL,1995.[2]J.Barber,S.Bhatta,A.Goel,M.Jacobsen,M.Pearce,L.Penberthy,M.Shankar,and E.Stroulia,Integrating Case-Based Reasoning And Multimedia Technologies For Interface Design Support,In Artificial In-telligence In Design,Editor:J.G.Boston,Kluwer Academic Publisher, 1992.[3]Yun-Heh Chen-Burger,Dave Robertson,and Jussi Stader,‘Knowledge-Based Automatic Verification and Validation for Business Mod-els’,DARPA-JFACC Symposium on Advances in Enterprise Control, (November1999).[4]Yun-Heh Chen-Burger,David Robertson,and Jussi Stader,‘FormalSupport for an Informal Business Modelling Method’,The Special Issue for The International Journal of Software Engineering and Knowledge Engineering,(February2000).[5] E.Domeshek,J.Kolodner,and C.Zimring,‘The Design of a Tool Kitfor Case-Based Design Aids’,Proceedings of the Third International Conference on Artificial Intelligence in Design,(1994).[6] B.Faltings,Case Reuse By Model-Based Interpretation,in Issues andApplications of Case-Based Reasoning in Design,Editor:M.L.Maher, P.Pu,Lawrence Erlbaum Associates,Hillsdale,N.J.,1997.pp.30-60.[7]T.R.Hinrichs,‘Towards an Architecture for Open World Problem Solv-ing’,Proceedings of CBR workshop,pp.182–189,(1988).Morgan Kaufmann,San Francisco.[8]IBM United Kingdom Limited,389Chriswick High Road,London W44AL,England,Business System Development Method:Business Map-ping Part1:Entities,2nd edn.,May1992.[9]Martin King,‘Knowledge Reuse in Business Domains Experience withIBM BSDM’,Technical report,Artificial Intelligence Application Insti-tute,(1995).[10]Janet Kolodner,Case-Based Reasoning,Morgan Kaufmann Publishers,Inc.,2929Campus Drive,suite260,SanMateo,CA,USA,1993. [11]M.L.Maher,B.Balachandran,and D.M.Zhang,Case-Based Reason-ing In Design,Lawrence Erlbaum,1995.[12]M.L.Maher and A.Gomez de Silva Garza,‘Developing Case-BasedReasoning for Structural Design’,IEEE Expert,Intelligent Systems and Their Applications,11(3),(June1996).[13]S.Narashiman,K.Sycara,and D.Navin-Chandra,Representation andSynthesis of Non-Monotonic Mechanical Devices,In Issues and Applic-ations of Case-Based Reasoning in Design,Editor:M.L.Maher,P.Pu, Lawrence Erlbaum Associates,Hillsdale,N.J.,1997.[14] E.Stroulia and A.K.Goel,‘Generic Teleological Mechanisms andTheir Use in Case Adaptation’,Proceedings of the Fourteenth Annual Conference of the Cognitive Science,(1992).Northvale,N.J.,Erlbaum.[15]K.Sycara,R.Guttal,J.Koning,S.Narasimhan,and D.Navin-chandra,‘CADET:A Case-based Synthesis Tool for Engineering Design’,International Journal for Expert Systems,4(2),pp.157–188,(1992)./afs//project/cadet/ftp/ docs/CADET.html.。