A Rhetorical Analysis of Second Inaugural Address by Abraham Lincoln林肯第二次就职演讲的修辞分析
A practical scale evaluation of catalysts for the selective reduction of NOx with

temperature range, selectivity to N20 and emission of non-reacted or incompletely oxidized reductants, were similar to that shown when model gas mixture systems were used. 0 1998 Elsevier Science B.V.
Keywords:
Diesel exhaust; Nitrogen oxide
1. Introduction Since 1990, there have been a large number of reports about the selective catalytic reduction of nitrogen oxides (NO,) with organic substances under netoxidizing conditions. Many of these were aiming at finding the best catalysts, reductants and catalystreductant combinations. For example, at the annual meetings of the Chemical Society of Japan and that of the Catalytic Society of Japan from 1990 to 1995, more than 300 kinds of catalytic systems have been
Akira Obuchi*, Isamu Kaneko, Junk0 Oi, Akihiko Ohi, Atsushi Ogata, Gratian R. Bamwenda, Satoshi Kushiyama
rhetorical analysis essay范文
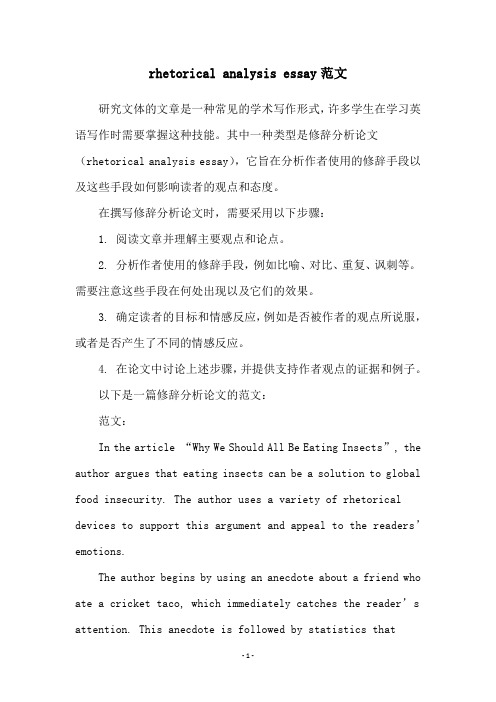
rhetorical analysis essay范文研究文体的文章是一种常见的学术写作形式,许多学生在学习英语写作时需要掌握这种技能。
其中一种类型是修辞分析论文(rhetorical analysis essay),它旨在分析作者使用的修辞手段以及这些手段如何影响读者的观点和态度。
在撰写修辞分析论文时,需要采用以下步骤:1. 阅读文章并理解主要观点和论点。
2. 分析作者使用的修辞手段,例如比喻、对比、重复、讽刺等。
需要注意这些手段在何处出现以及它们的效果。
3. 确定读者的目标和情感反应,例如是否被作者的观点所说服,或者是否产生了不同的情感反应。
4. 在论文中讨论上述步骤,并提供支持作者观点的证据和例子。
以下是一篇修辞分析论文的范文:范文:In the article “Why We Should All Be Eating Insects”, the author argues that eating insects can be a solution to global food insecurity. The author uses a variety of rhetorical devices to support this argument and appeal to the readers’emotions.The author begins by using an anecdote about a friend who ate a cricket taco, which immediately catches the reader’s attention. This anecdote is followed by statistics thatdemonstrate the unsustainability of current food production methods. By combining a personal story with facts, the author establishes credibility and engages the reader emotionally. The author emphasizes the benefits of eating insects, such as their high protein content and low environmental impact. These benefits are reinforced with imagery, such as the comparison of a cricket farm to a chicken farm. By using vivid imagery, the author creates a mental picture that supports the argument.Furthermore, the author uses repetition to emphasize the urgency of the issue, stating that “we need to act now.” This repetition is effective in creating a sense of urgency in the reader, encouraging them to take action.Although the author’s argument may seem unconventional, the use of rhetorical devices helps to persuade the reader. The combination of personal anecdotes, statistics, imagery, and repetition creates a persuasive argument that appeals to the reader’s emotions and encourages them to consider the benefits of eating insects.总结:以上范文简要说明了如何撰写修辞分析论文。
How to Write a Rhetorical Analysis

How to Write a Rhetorical AnalysisBy Robert RussellThe point of departure for rhetorical analysis is the realization that people are moved and persuaded in a variety of ways. Convincing an audience or reader by appealing to evidence and rational argument is only one way of making an argument. In some situations, a more convincing case may be achieved by appealing to the reader or audience at an emotional level. The goal of rhetorical analysis is to analyze and understand a text by breaking it down into its constitutive elements.1Carefully read the article and determine subject matter and goals of the author. The goal may be to explain or inform the reader about a particular issue or topic. The goal may be to amuse and entertain the reader about a more light-hearted topic or its aim may be motivating or persuading the reader.2Analyze the rhetorical elements of the author's writing style. Writers and speakers use words in language in different ways to achieve different effects. Rhetoric recognizes that a speaker or writer persuades his audience with different tools and techniques.Aristotle categorized rhetoric into three categories: logos, ethos and pathos. Logos uses reason and rationality to persuade the reader. Ethos seeks to persuade the reader by appealing to credibility of the writer or another expert. The expert's authority,rather than the evidence itself, convinces the reader. Pathos attempts to make the case by appealing to the reader at an emotional level. Pathos may appeal to the reader's sympathy or compassion, sadness or anger, love or hatred and so forth. Read the text and take notes about the different rhetorical styles used.o3Write an outline for the rhetorical analysis. The analysis should have three parts: an introduction, a main body and a conclusion. Divide the main body of theanalysis into subsections. The subsections may be devoted to the different rhetorical elements used in the text.o4Write a strong thesis sentence that clearly states the main points of the analysis.Describe the central argument made by the author and the general method used to make the argument. Outline the steps of your argument in the last one or two sentences of the introduction. Present the different elements of your analysis in the main body of the paper. Cite examples from the text as evidence for your analysis. Write a one paragraph conclusion that summarizes the central points of the analysis.。
Universities in Evolutionary Systems(系统变革中的大学)

Universities in Evolutionary Systems of InnovationMarianne van der Steen and Jurgen EndersThis paper criticizes the current narrow view on the role of universities in knowledge-based economies.We propose to extend the current policy framework of universities in national innovation systems(NIS)to a more dynamic one,based on evolutionary economic principles. The main reason is that this dynamic viewfits better with the practice of innovation processes. We contribute on ontological and methodological levels to the literature and policy discussions on the effectiveness of university-industry knowledge transfer and the third mission of uni-versities.We conclude with a discussion of the policy implications for the main stakeholders.1.IntroductionU niversities have always played a major role in the economic and cultural devel-opment of countries.However,their role and expected contribution has changed sub-stantially over the years.Whereas,since1945, universities in Europe were expected to con-tribute to‘basic’research,which could be freely used by society,in recent decades they are expected to contribute more substantially and directly to the competitiveness offirms and societies(Jaffe,2008).Examples are the Bayh–Dole Act(1982)in the United States and in Europe the Lisbon Agenda(2000–2010) which marked an era of a changing and more substantial role for universities.However,it seems that this‘new’role of universities is a sort of universal given one(ex post),instead of an ex ante changing one in a dynamic institutional environment.Many uni-versities are expected nowadays to stimulate a limited number of knowledge transfer activi-ties such as university spin-offs and university patenting and licensing to demonstrate that they are actively engaged in knowledge trans-fer.It is questioned in the literature if this one-size-fits-all approach improves the usefulness and the applicability of university knowledge in industry and society as a whole(e.g.,Litan et al.,2007).Moreover,the various national or regional economic systems have idiosyncratic charac-teristics that in principle pose different(chang-ing)demands towards universities.Instead of assuming that there is only one‘optimal’gov-ernance mode for universities,there may bemultiple ways of organizing the role of univer-sities in innovation processes.In addition,we assume that this can change over time.Recently,more attention in the literature hasfocused on diversity across technologies(e.g.,King,2004;Malerba,2005;Dosi et al.,2006;V an der Steen et al.,2008)and diversity offormal and informal knowledge interactionsbetween universities and industry(e.g.,Cohenet al.,1998).So far,there has been less atten-tion paid to the dynamics of the changing roleof universities in economic systems:how dothe roles of universities vary over time andwhy?Therefore,this article focuses on the onto-logical premises of the functioning of univer-sities in innovation systems from a dynamic,evolutionary perspective.In order to do so,we analyse the role of universities from theperspective of an evolutionary system ofinnovation to understand the embeddednessof universities in a dynamic(national)systemof science and innovation.The article is structured as follows.InSection2we describe the changing role ofuniversities from the static perspective of anational innovation system(NIS),whereasSection3analyses the dynamic perspective ofuniversities based on evolutionary principles.Based on this evolutionary perspective,Section4introduces the characteristics of a LearningUniversity in a dynamic innovation system,summarizing an alternative perception to thestatic view of universities in dynamic economicsystems in Section5.Finally,the concludingVolume17Number42008doi:10.1111/j.1467-8691.2008.00496.x©2008The AuthorsJournal compilation©2008Blackwell Publishingsection discusses policy recommendations for more effective policy instruments from our dynamic perspective.2.Static View of Universities in NIS 2.1The Emergence of the Role of Universities in NISFirst we start with a discussion of the literature and policy reports on national innovation system(NIS).The literature on national inno-vation systems(NIS)is a relatively new and rapidly growingfield of research and widely used by policy-makers worldwide(Fagerberg, 2003;Balzat&Hanusch,2004;Sharif,2006). The NIS approach was initiated in the late 1980s by Freeman(1987),Dosi et al.(1988)and Lundvall(1992)and followed by Nelson (1993),Edquist(1997),and many others.Balzat and Hanusch(2004,p.196)describe a NIS as‘a historically grown subsystem of the national economy in which various organizations and institutions interact with and influence one another in the carrying out of innovative activity’.It is about a systemic approach to innovation,in which the interaction between technology,institutions and organizations is central.With the introduction of the notion of a national innovation system,universities were formally on the agenda of many innovation policymakers worldwide.Clearly,the NIS demonstrated that universities and their interactions with industry matter for innova-tion processes in economic systems.Indeed, since a decade most governments acknowl-edge that interactions between university and industry add to better utilization of scienti-fic knowledge and herewith increase the innovation performance of nations.One of the central notions of the innovation system approach is that universities play an impor-tant role in the development of commercial useful knowledge(Edquist,1997;Sharif, 2006).This contrasts with the linear model innovation that dominated the thinking of science and industry policy makers during the last century.The linear innovation model perceives innovation as an industry activity that‘only’utilizes fundamental scientific knowledge of universities as an input factor for their innovative activities.The emergence of the non-linear approach led to a renewed vision on the role–and expectations–of universities in society. Some authors have referred to a new social contract between science and society(e.g., Neave,2000).The Triple Helix(e.g.,Etzkowitz &Leydesdorff,1997)and the innovation system approach(e.g.,Lundvall,1988)and more recently,the model of Open Innovation (Chesbrough,2003)demonstrated that innova-tion in a knowledge-based economy is an inter-active process involving many different innovation actors that interact in a system of overlapping organizationalfields(science, technology,government)with many interfaces.2.2Static Policy View of Universities in NIS Since the late1990s,the new role of universi-ties in NIS thinking emerged in a growing number of policy studies(e.g.,OECD,1999, 2002;European Commission,2000).The con-tributions of the NIS literature had a large impact on policy makers’perception of the role of universities in the national innovation performance(e.g.,European Commission, 2006).The NIS approach gradually replaced linear thinking about innovation by a more holistic system perspective on innovations, focusing on the interdependencies among the various agents,organizations and institutions. NIS thinking led to a structurally different view of how governments can stimulate the innovation performance of a country.The OECD report of the national innovation system (OECD,1999)clearly incorporated these new economic principles of innovation system theory.This report emphasized this new role and interfaces of universities in knowledge-based economies.This created a new policy rationale and new awareness for technology transfer policy in many countries.The NIS report(1999)was followed by more attention for the diversity of technology transfer mecha-nisms employed in university-industry rela-tions(OECD,2002)and the(need for new) emerging governance structures for the‘third mission’of universities in society,i.e.,patent-ing,licensing and spin-offs,of public research organizations(OECD,2003).The various policy studies have in common that they try to describe and compare the most important institutions,organizations, activities and interactions of public and private actors that take part in or influence the innovation performance of a country.Figure1 provides an illustration.Thefigure demon-strates the major building blocks of a NIS in a practical policy setting.It includesfirms,uni-versities and other public research organiza-tions(PROs)involved in(higher)education and training,science and technology.These organizations embody the science and tech-nology capabilities and knowledge fund of a country.The interaction is represented by the arrows which refer to interactive learn-ing and diffusion of knowledge(Lundvall,Volume17Number42008©2008The AuthorsJournal compilation©2008Blackwell Publishing1992).1The building block ‘Demand’refers to the level and quality of demand that can be a pull factor for firms to innovate.Finally,insti-tutions are represented in the building blocks ‘Framework conditions’and ‘Infrastructure’,including various laws,policies and regula-tions related to science,technology and entre-preneurship.It includes a very broad array of policy issues from intellectual property rights laws to fiscal instruments that stimulate labour mobility between universities and firms.The figure demonstrates that,in order to improve the innovation performance of a country,the NIS as a whole should be conducive for innovative activities in acountry.Since the late 1990s,the conceptual framework as represented in Figure 1serves as a dominant design for many comparative studies of national innovation systems (Polt et al.,2001;OECD,2002).The typical policy benchmark exercise is to compare a number of innovation indicators related to the role of university-industry interactions.Effective performance of universities in the NIS is judged on a number of standardized indica-tors such as the number of spin-offs,patents and licensing.Policy has especially focused on ‘getting the incentives right’to create a generic,good innovative enhancing context for firms.Moreover,policy has also influ-enced the use of specific ‘formal’transfer mechanisms,such as university patents and university spin-offs,to facilitate this collabo-ration.In this way best practice policies are identified and policy recommendations are derived:the so-called one-size-fits-all-approach.The focus is on determining the ingredients of an efficient benchmark NIS,downplaying institutional diversity and1These organizations that interact with each other sometimes co-operate and sometimes compete with each other.For instance,firms sometimes co-operate in certain pre-competitive research projects but can be competitors as well.This is often the case as well withuniversities.Figure 1.The Benchmark NIS Model Source :Bemer et al.(2001).Volume 17Number 42008©2008The AuthorsJournal compilation ©2008Blackwell Publishingvariety in the roles of universities in enhanc-ing innovation performance.The theoretical contributions to the NIS lit-erature have outlined the importance of insti-tutions and institutional change.However,a further theoretical development of the ele-ments of NIS is necessary in order to be useful for policy makers;they need better systemic NIS benchmarks,taking systematically into account the variety of‘national idiosyncrasies’. Edquist(1997)argues that most NIS contribu-tions are more focused onfirms and technol-ogy,sometimes reducing the analysis of the (national)institutions to a left-over category (Geels,2005).Following Hodgson(2000), Nelson(2002),Malerba(2005)and Groenewe-gen and V an der Steen(2006),more attention should be paid to the institutional idiosyncra-sies of the various systems and their evolution over time.This creates variety and evolving demands towards universities over time where the functioning of universities and their interactions with the other part of the NIS do evolve as well.We suggest to conceptualize the dynamics of innovation systems from an evolutionary perspective in order to develop a more subtle and dynamic vision on the role of universities in innovation systems.We emphasize our focus on‘evolutionary systems’instead of national innovation systems because for many universities,in particular some science-based disciplinaryfields such as biotechnology and nanotechnology,the national institutional environment is less relevant than the institu-tional and technical characteristics of the technological regimes,which is in fact a‘sub-system’of the national innovation system.3.Evolutionary Systems of Innovation as an Alternative Concept3.1Evolutionary Theory on Economic Change and InnovationCharles Darwin’s The Origin of Species(1859)is the foundation of modern thinking about change and evolution(Luria et al.,1981,pp. 584–7;Gould,1987).Darwin’s theory of natural selection has had the most important consequences for our perception of change. His view of evolution refers to a continuous and gradual adaptation of species to changes in the environment.The idea of‘survival of thefittest’means that the most adaptive organisms in a population will survive.This occurs through a process of‘natural selection’in which the most adaptive‘species’(organ-isms)will survive.This is a gradual process taking place in a relatively stable environment, working slowly over long periods of time necessary for the distinctive characteristics of species to show their superiority in the‘sur-vival contest’.Based on Darwin,evolutionary biology identifies three levels of aggregation.These three levels are the unit of variation,unit of selection and unit of evolution.The unit of varia-tion concerns the entity which contains the genetic information and which mutates fol-lowing specific rules,namely the genes.Genes contain the hereditary information which is preserved in the DNA.This does not alter sig-nificantly throughout the reproductive life-time of an organism.Genes are passed on from an organism to its successors.The gene pool,i.e.,the total stock of genetic structures of a species,only changes in the reproduction process as individuals die and are born.Par-ticular genes contribute to distinctive charac-teristics and behaviour of species which are more or less conducive to survival.The gene pool constitutes the mechanism to transmit the characteristics of surviving organisms from one generation to the next.The unit of selection is the expression of those genes in the entities which live and die as individual specimens,namely(individual) organisms.These organisms,in their turn,are subjected to a process of natural selection in the environment.‘Fit’organisms endowed with a relatively‘successful’gene pool,are more likely to pass them on to their progeny.As genes contain information to form and program the organisms,it can be expected that in a stable environment genes aiding survival will tend to become more prominent in succeeding genera-tions.‘Natural selection’,thus,is a gradual process selecting the‘fittest’organisms. Finally,there is the unit of evolution,or that which changes over time as the gene pool changes,namely populations.Natural selec-tion produces changes at the level of the population by‘trimming’the set of genetic structures in a population.We would like to point out two central principles of Darwinian evolution.First,its profound indeterminacy since the process of development,for instance the development of DNA,is dominated by time at which highly improbable events happen (Boulding,1991,p.12).Secondly,the process of natural selection eliminates poorly adapted variants in a compulsory manner,since indi-viduals who are‘unfit’are supposed to have no way of escaping the consequences of selection.22We acknowledge that within evolutionary think-ing,the theory of Jean Baptiste Lamarck,which acknowledges in essence that acquired characteris-tics can be transmitted(instead of hereditaryVolume17Number42008©2008The AuthorsJournal compilation©2008Blackwell PublishingThese three levels of aggregation express the differences between ‘what is changing’(genes),‘what is being selected’(organisms),and ‘what changes over time’(populations)in an evolutionary process (Luria et al.,1981,p.625).According to Nelson (see for instance Nelson,1995):‘Technical change is clearly an evolutionary process;the innovation generator keeps on producing entities superior to those earlier in existence,and adjustment forces work slowly’.Technological change and innovation processes are thus ‘evolutionary’because of its characteristics of non-optimality and of an open-ended and path-dependent process.Nelson and Winter (1982)introduced the idea of technical change as an evolutionary process in capitalist economies.Routines in firms function as the relatively durable ‘genes’.Economic competition leads to the selection of certain ‘successful’routines and these can be transferred to other firms by imitation,through buy-outs,training,labour mobility,and so on.Innovation processes involving interactions between universities and industry are central in the NIS approach.Therefore,it seems logical that evolutionary theory would be useful to grasp the role of universities in innovation pro-cesses within the NIS framework.3.2Evolutionary Underpinnings of Innovation SystemsBased on the central evolutionary notions as discussed above,we discuss in this section how the existing NIS approaches have already incor-porated notions in their NIS frameworks.Moreover,we investigate to what extent these notions can be better incorporated in an evolu-tionary innovation system to improve our understanding of universities in dynamic inno-vation processes.We focus on non-optimality,novelty,the anti-reductionist methodology,gradualism and the evolutionary metaphor.Non-optimality (and Bounded Rationality)Based on institutional diversity,the notion of optimality is absent in most NIS approaches.We cannot define an optimal system of innovation because evolutionary learning pro-cesses are important in such systems and thus are subject to continuous change.The system never achieves an equilibrium since the evolu-tionary processes are open-ended and path dependent.In Nelson’s work (e.g.,1993,1995)he has emphasized the presence of contingent out-comes of innovation processes and thus of NIS:‘At any time,there are feasible entities not present in the prevailing system that have a chance of being introduced’.This continuing existence of feasible alternative developments means that the system never reaches a state of equilibrium or finality.The process always remains dynamic and never reaches an optimum.Nelson argues further that diversity exists because technical change is an open-ended multi-path process where no best solu-tion to a technical problem can be identified ex post .As a consequence technical change can be seen as a very wasteful process in capitalist economies with many duplications and dead-ends.Institutional variety is closely linked to non-optimality.In other words,we cannot define the optimal innovation system because the evolutionary learning processes that take place in a particular system make it subject to continuous change.Therefore,comparisons between an existing system and an ideal system are not possible.Hence,in the absence of any notion of optimality,a method of comparing existing systems is necessary.According to Edquist (1997),comparisons between systems were more explicit and systematic than they had been using the NIS approaches.Novelty:Innovations CentralNovelty is already a central notion in the current NIS approaches.Learning is inter-preted in a broad way.Technological innova-tions are defined as combining existing knowledge in new ways or producing new knowledge (generation),and transforming this into economically significant products and processes (absorption).Learning is the most important process behind technological inno-vations.Learning can be formal in the form of education and searching through research and development.However,in many cases,innovations are the consequence of several kinds of learning processes involving many different kinds of economic agents.According to Lundvall (1992,p.9):‘those activities involve learning-by-doing,increasing the efficiency of production operations,learning-characteristics as in the theory of Darwin),is acknowledged to fit better with socio-economic processes of technical change and innovation (e.g.,Nelson &Winter,1982;Hodgson,2000).Therefore,our theory is based on Lamarckian evolutionary theory.However,for the purpose of this article,we will not discuss the differences between these theo-ries at greater length and limit our analysis to the fundamental evolutionary building blocks that are present in both theories.Volume 17Number 42008©2008The AuthorsJournal compilation ©2008Blackwell Publishingby-using,increasing the efficiency of the use of complex systems,and learning-by-interacting, involving users and producers in an interac-tion resulting in product innovations’.In this sense,learning is part of daily routines and activities in an economy.In his Learning Economy concept,Lundvall makes learning more explicit,emphasizing further that ‘knowledge is assumed as the most funda-mental resource and learning the most impor-tant process’(1992,p.10).Anti-reductionist Approach:Systems and Subsystems of InnovationSo far,NIS approaches are not yet clear and systematic in their analysis of the dynamics and change in innovation systems.Lundvall’s (1992)distinction between subsystem and system level based on the work of Boulding implicitly incorporates both the actor(who can undertake innovative activities)as well as the structure(institutional selection environment) in innovation processes of a nation.Moreover, most NIS approaches acknowledge that within the national system,there are different institu-tional subsystems(e.g.,sectors,regions)that all influence each other again in processes of change.However,an explicit analysis of the structured environment is still missing (Edquist,1997).In accordance with the basic principles of evolutionary theory as discussed in Section 3.1,institutional evolutionary theory has developed a very explicit systemic methodol-ogy to investigate the continuous interaction of actors and institutional structures in the evolution of economic systems.The so-called ‘methodological interactionism’can be per-ceived as a methodology that combines a structural perspective and an actor approach to understand processes of economic evolu-tion.Whereas the structural perspective emphasizes the existence of independent institutional layers and processes which deter-mine individual actions,the actor approach emphasizes the free will of individuals.The latter has been referred to as methodological individualism,as we have seen in neo-classical approaches.Methodological indi-vidualism will explain phenomena in terms of the rational individual(showingfixed prefer-ences and having one rational response to any fully specified decision problem(Hodgson, 2000)).The interactionist approach recognizes a level of analysis above the individual orfirm level.NIS approaches recognize that national differences exist in terms of national institu-tions,socio-economic factors,industries and networks,and so on.So,an explicit methodological interactionist approach,explicitly recognizing various insti-tutional layers in the system and subsystem in interaction with the learning agents,can improve our understanding of the evolution of innovation.Gradualism:Learning Processes andPath-DependencyPath-dependency in biology can be translated in an economic context in the form of(some-times very large)time lags between a technical invention,its transformation into an economic innovation,and the widespread diffusion. Clearly,in many of the empirical case studies of NIS,the historical dimension has been stressed.For instance,in the study of Denmark and Sweden,it has been shown that the natural resource base(for Denmark fertile land,and for Sweden minerals)and economic history,from the period of the Industrial Revolution onwards,has strongly influenced present specialization patterns(Edquist& Lundvall,1993,pp.269–82).Hence,history matters in processes of inno-vation as the innovation processes are influ-enced by many institutions and economic agents.In addition,they are often path-dependent as small events are reinforced and become crucially important through processes of positive feedback,in line with evolutionary processes as discussed in Section3.1.Evolutionary MetaphorFinally,most NIS approaches do not explicitly use the biological metaphor.Nevertheless, many of the approaches are based on innova-tion theories in which they do use an explicit evolutionary metaphor(e.g.,the work of Nelson).To summarize,the current(policy)NIS approaches have already implicitly incorpo-rated some evolutionary notions such as non-optimality,novelty and gradualism.However, what is missing is a more explicit analysis of the different institutional levels of the economic system and innovation subsystems (their inertia and evolution)and how they change over time in interaction with the various learning activities of economic agents. These economic agents reside at established firms,start-upfirms,universities,govern-ments,undertaking learning and innovation activities or strategic actions.The explicit use of the biological metaphor and an explicit use of the methodological interactionst approach may increase our understanding of the evolu-tion of innovation systems.Volume17Number42008©2008The AuthorsJournal compilation©2008Blackwell Publishing4.Towards a Dynamic View of Universities4.1The Logic of an Endogenous‘Learning’UniversityIf we translate the methodological interaction-ist approach to the changing role of universities in an evolutionary innovation system,it follows that universities not only respond to changes of the institutional environment(government policies,business demands or changes in scientific paradigms)but universities also influence the institutions of the selection envi-ronment by their strategic,scientific and entre-preneurial actions.Moreover,these actions influence–and are influenced by–the actions of other economic agents as well.So,instead of a one-way rational response by universities to changes(as in reductionist approach),they are intertwined in those processes of change.So, universities actually function as an endogenous source of change in the evolution of the inno-vation system.This is(on an ontological level) a fundamental different view on the role of universities in innovation systems from the existing policy NIS frameworks.In earlier empirical research,we observed that universities already effectively function endogenously in evolutionary innovation system frameworks;universities as actors (already)develop new knowledge,innovate and have their own internal capacity to change,adapt and influence the institutional development of the economic system(e.g., V an der Steen et al.,2009).Moreover,univer-sities consist of a network of various actors, i.e.,the scientists,administrators at technology transfer offices(TTO)as well as the university boards,interacting in various ways with indus-try and governments and embedded in various ways in the regional,national or inter-national environment.So,universities behave in an at least partly endogenous manner because they depend in complex and often unpredictable ways on the decision making of a substantial number of non-collusive agents.Agents at universities react in continuous interaction with the learn-ing activities offirms and governments and other universities.Furthermore,the endogenous processes of technical and institutional learning of univer-sities are entangled in the co-evolution of institutional and technical change of the evo-lutionary innovation system at large.We propose to treat the learning of universities as an inseparable endogenous variable in the inno-vation processes of the economic system.In order to structure the endogenization in the system of innovation analysis,the concept of the Learning University is introduced.In thenext subsection we discuss the main character-istics of the Learning University and Section5discusses the learning university in a dynamic,evolutionary innovation system.An evolution-ary metaphor may be helpful to make theuniversity factor more transparent in theco-evolution of technical and institutionalchange,as we try to understand how variouseconomic agents interact in learning processes.4.2Characteristics of the LearningUniversityThe evolution of the involvement of universi-ties in innovation processes is a learningprocess,because(we assume that)universitypublic agents have their‘own agenda’.V ariousincentives in the environment of universitiessuch as government regulations and technol-ogy transfer policies as well as the innovativebehaviour of economic agents,compel policymakers at universities to constantly respondby adapting and improving their strategiesand policies,whereas the university scientistsare partly steered by these strategies and partlyinfluenced by their own scientific peers andpartly by their historically grown interactionswith industry.During this process,universityboards try to be forward-looking and tobehave strategically in the knowledge thattheir actions‘influence the world’(alsoreferred to earlier as‘intentional variety’;see,for instance,Dosi et al.,1988).‘Intentional variety’presupposes that tech-nical and institutional development of univer-sities is a learning process.University agentsundertake purposeful action for change,theylearn from experience and anticipate futurestates of the selective environment.Further-more,university agents take initiatives to im-prove and develop learning paths.An exampleof these learning agents is provided in Box1.We consider technological and institutionaldevelopment of universities as a process thatinvolves many knowledge-seeking activitieswhere public and private agents’perceptionsand actions are translated into practice.3Theinstitutional changes are the result of inter-actions among economic agents defined byLundvall(1992)as interactive learning.Theseinteractions result in an evolutionary pattern3Using a theory developed in one scientific disci-pline as a metaphor in a different discipline mayresult,in a worst-case scenario,in misleading analo-gies.In the best case,however,it can be a source ofcreativity.As Hodgson(2000)pointed out,the evo-lutionary metaphor is useful for understandingprocesses of technical and institutional change,thatcan help to identify new events,characteristics andphenomena.Volume17Number42008©2008The AuthorsJournal compilation©2008Blackwell Publishing。
现代语言教学的十大原则
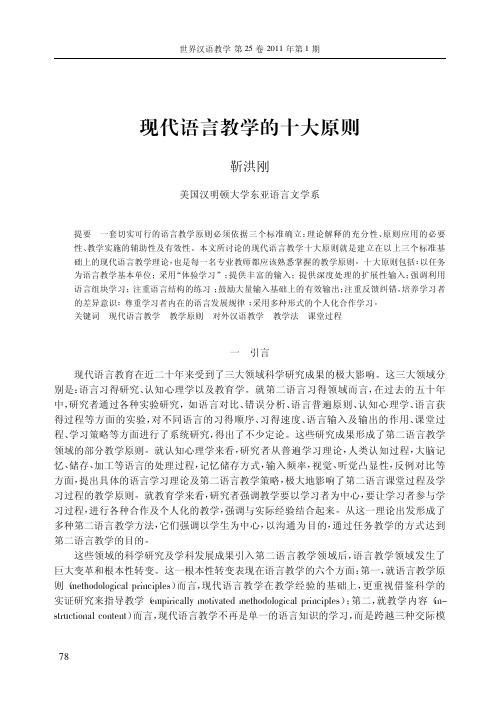
靳洪刚: 现代语言教学的十大原则
习者进一步明确语言学习的最终目的是完成生活中的各种任务,解决生活中的各种问题。 任务教学设计一般须包含三个任务阶段及五个基本组成部分。任务的三个阶段分别
是:前期任务、核心任务、后期任务。前期阶段是任务准备阶段,多在课下完成。前期任务的 目的是激活学习者已有知识,为新的语言学习奠定基础。从语言输入、信息来源、交际背景 等方面为学生提供语言及交际框架( scaffolding) ,帮助学生顺利进入核心任务阶段。因此, 这一阶段的教学主要以激活已有知识、处理语言输入为主,活动多以理解诠释性阅读、听力 为主。核心任务阶段包含两个方面的教学,一是以语言形式为中心的教学,目的是给学习者 建立完成核心任务的交际框架,帮助学习者整合信息;二是以语言使用为中心的任务模拟、 教学实施,多以口语输出、人际交流的方式在课上完成。核心任务的目的是提供具有一定认 知及语言复杂度的模拟任务,让学习者有目的地使用目标语言,完成任务,取得预期结果。 主要形式为,首先采用合班学习语言形式,然后分组互动,完成信息交换、信息组合、意见交 换等任务。后期阶段是任务总结、反思、实际生活应用阶段,多以实地操作、书面输出或口头 演说的方式在课上或课下完成,主要采用书面总结、实地调查、口头报告等形式。
一 引言 现代语言教育在近二十年来受到了三大领域科学研究成果的极大影响。这三大领域分 别是:语言习得研究、认知心理学以及教育学。就第二语言习得领域而言,在过去的五十年 中,研究者通过各种实验研究,如语言对比、错误分析、语言普遍原则、认知心理学、语言获 得过程等方面的实验,对不同语言的习得顺序、习得速度、语言输入及输出的作用、课堂过 程、学习策略等方面进行了系统研究,得出了不少定论。这些研究成果形成了第二语言教学 领域的部分教学原则。就认知心理学来看,研究者从普遍学习理论,人类认知过程,大脑记 忆、储存、加工等语言的处理过程,记忆储存方式,输入频率,视觉、听觉凸显性,反例对比等 方面,提出具体的语言学习理论及第二语言教学策略,极大地影响了第二语言课堂过程及学 习过程的教学原则。就教育学来看,研究者强调教学要以学习者为中心,要让学习者参与学 习过程,进行各种合作及个人化的教学,强调与实际经验结合起来。从这一理论出发形成了 多种第二语言教学方法,它们强调以学生为中心,以沟通为目的,通过任务教学的方式达到 第二语言教学的目的。 这些领域的科学研究及学科发展成果引入第二语言教学领域后,语言教学领域发生了 巨大变革和根本性转变。这一根本性转变表现在语言教学的六个方面:第一,就语言教学原 则( methodological principles) 而言,现代语言教学在教学经验的基础上,更重视借鉴科学的 实证研究来指导教学( empirically motivated methodological principles) ;第二,就教学内容( instructional content) 而言,现代语言教学不再是单一的语言知识的学习,而是跨越三种交际模
巴赫金.陀思妥耶夫斯基诗学问题 英文文献
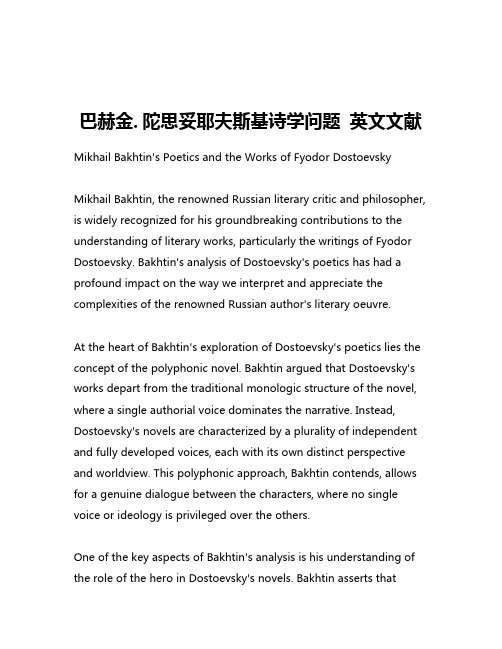
巴赫金.陀思妥耶夫斯基诗学问题英文文献Mikhail Bakhtin's Poetics and the Works of Fyodor DostoevskyMikhail Bakhtin, the renowned Russian literary critic and philosopher, is widely recognized for his groundbreaking contributions to the understanding of literary works, particularly the writings of Fyodor Dostoevsky. Bakhtin's analysis of Dostoevsky's poetics has had a profound impact on the way we interpret and appreciate the complexities of the renowned Russian author's literary oeuvre.At the heart of Bakhtin's exploration of Dostoevsky's poetics lies the concept of the polyphonic novel. Bakhtin argued that Dostoevsky's works depart from the traditional monologic structure of the novel, where a single authorial voice dominates the narrative. Instead, Dostoevsky's novels are characterized by a plurality of independent and fully developed voices, each with its own distinct perspective and worldview. This polyphonic approach, Bakhtin contends, allows for a genuine dialogue between the characters, where no single voice or ideology is privileged over the others.One of the key aspects of Bakhtin's analysis is his understanding of the role of the hero in Dostoevsky's novels. Bakhtin asserts thatDostoevsky's heroes are not passive objects of the author's design but rather autonomous individuals with the capacity to challenge and even contradict the author's own views. This notion of the hero as a fully autonomous and self-conscious being is central to Bakhtin's conception of the polyphonic novel.Furthermore, Bakhtin explores the concept of the "unfinalized" hero in Dostoevsky's works. He argues that Dostoevsky's characters are not static, predetermined entities but rather dynamic, ever-evolving individuals who resist being reduced to a single, fixed identity. This idea of the "unfinalized" hero is closely tied to Bakhtin's understanding of the polyphonic novel, where the characters are not merely vehicles for the author's ideas but active participants in the ongoing dialogue that constitutes the literary work.Bakhtin's analysis also delves into the role of language and discourse in Dostoevsky's novels. He suggests that Dostoevsky's works are characterized by a multiplicity of social and ideological languages, each with its own distinct perspective and mode of expression. This heteroglossia, as Bakhtin terms it, serves to undermine the notion of a single, authoritative voice and instead presents a cacophony of competing voices and worldviews.Moreover, Bakhtin's exploration of Dostoevsky's poetics extends to the notion of the carnivalesque, a concept that he sees as central tothe author's literary approach. The carnivalesque, in Bakhtin's view, represents a subversive and liberating force that challenges traditional hierarchies and social norms. He argues that Dostoevsky's novels often incorporate elements of the carnival, such as the inversion of power structures, the blurring of boundaries between the sacred and the profane, and the celebration of the body and its functions.Bakhtin's insights into Dostoevsky's poetics have had a lasting impact on the field of literary criticism and have influenced generations of scholars and readers. By illuminating the complex and multifaceted nature of Dostoevsky's literary works, Bakhtin has helped us to better understand the profound philosophical and existential questions that lie at the heart of the Russian author's oeuvre.In conclusion, Mikhail Bakhtin's exploration of Dostoevsky's poetics has been instrumental in shaping our understanding of the Russian author's literary genius. Through concepts such as the polyphonic novel, the "unfinalized" hero, and the carnivalesque, Bakhtin has provided a nuanced and insightful perspective on the rich tapestry of Dostoevsky's literary universe. His work continues to inspire and challenge scholars and readers alike, inviting us to engage with the complexities and contradictions that lie at the core of Dostoevsky's enduring literary legacy.。
Inference with difference-in-differences and other panel data
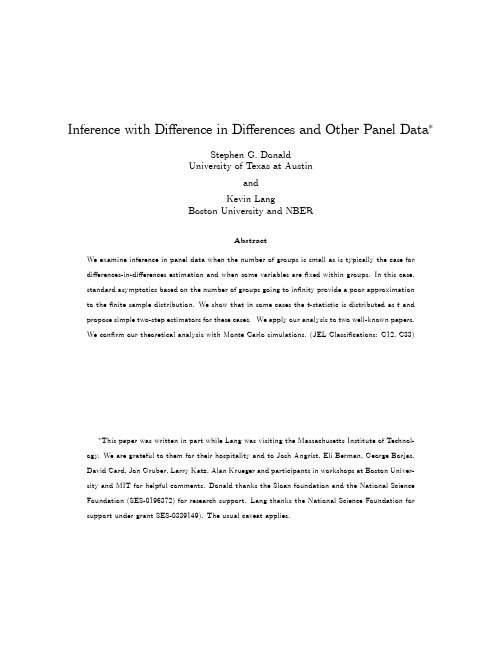
Inference with Difference in Differences and Other Panel Data∗Stephen G.DonaldUniversity of Texas at AustinandKevin LangBoston University and NBERAbstractWe examine inference in panel data when the number of groups is small as is typically the case fordifferences-in-differences estimation and when some variables arefixed within groups.In this case,standard asymptotics based on the number of groups going to infinity provide a poor approximationto thefinite sample distribution.We show that in some cases the t-statistic is distributed as t andpropose simple two-step estimators for these cases.We apply our analysis to two well-known papers.We confirm our theoretical analysis with Monte Carlo simulations.(JEL Classifications:C12,C33)∗This paper was written in part while Lang was visiting the Massachusetts Institute of Technol-ogy.We are grateful to them for their hospitality and to Josh Angrist,Eli Berman,George Borjas,David Card,Jon Gruber,Larry Katz,Alan Krueger and participants in workshops at Boston Univer-sity and MIT for helpful comments.Donald thanks the Sloan foundation and the National ScienceFoundation(SES-0196372)for research ng thanks the National Science Foundation forsupport under grant SES-0339149).The usual caveat applies.1IntroductionMany policy analyses rely on panel data in which the dependent variable differs across individuals but at least some explanatory variables,such as the policies being studied,are constant among all members of a group.For example,in the typical differences-in-differences model,we regress outcomes at the individual level(e.g.employment in afirm in state s in year t)on a policy that applies to all individuals in the group(e.g.the minimum wage in state s in year t).Moulton(1990)shows that in regression models with mixtures of individual and grouped data,the failure to account for the presence of common group errors can generate estimated standard errors that are biased downwards dramatically.1The differences-in-differences estimator is a special case of this model.Researchers use a number of standard techniques to adjust for common group effects:•random-effects feasible GLS estimation which under certain conditions is asymptotically efficient,•correcting the standard errors using the error covariance matrix based on common group errors as in Moulton,•correcting the standard errors using a robust covariance estimator according to a formula developed by Liang and Zeger(1986)and more commonly known as the Stata cluster command.This paper makes two simple,but,we believe,important points.First,when applied to variables that are constant within a group,the t-statistics generated using each of these techniques for correcting for common group errors are asymptotically normally distributed only as the number of groups goes to infinity.Second,under standard restrictions,the efficient estimator can be implemented by a simple two-step procedure,and the resulting t-statistic may have,under restrictions on the distribution of the group level error,an asymptotic t-distribution as the number of observations per group goes to infinity.In addition,under more restrictive assumptions,when the same procedure is used infinite samples,the t-statistics have a t-distribution.Consequently,standard asymptotics cannot be applied when the number of groups is small as in the case where we compare two states in two years,two cities over a small number of years,or self-employed workers and employees over a small number of years.In such cases,failing to take account of the group-error structure will not only generate underestimates of the standard errors as in Moulton, but applying the normal distribution to corrected t-statistics will dramatically overstate the significance of the statistics.Standard asymptotics should apply to comparisons across allfifty states although otherproblems may arise in common time-series/cross-section estimates based on states and using long panels (see Bertrand et al,2004).2In the next section,wefirst present an intuitive argument and then formalize the conditions under which we can derive the distribution of the t-statistic when the number of groups is small.Readers who are not interested in the details can skip the later part of this section and proceed to the third section,where we discuss the common two group/two period case and also apply our approach to two influential papers:the Gruber and Poterba(1994)paper on health insurance and self-employment and Card’s(1990)study of the Mariel boatlift.We show that analyzing the t-statistic,taking into account a possible group error component,dramatically reduces our estimate of the precision of their results.In the fourth section,we consider two other approaches to common group errors,the Moulton correc-tion and the commonly applied Stata cluster correction.In sectionfive,we present Monte Carlo evidence regarding the distribution of the t-statistic using a variety of estimators.Our results indicate that the t-statistics produced by standard estimators have distributions that differ quite substantially from both the normal and the t-distributions.However,when the theory predicts that they should,the two-step estimators we propose produce t-statistics with approximately a t-distribution with degrees of freedom equal to number of groups minus number of group-constant variables.Moreover,one of the two-step estimators we consider appears to be reasonably robust to the departures from the assumptions needed to guarantee that the t-statistic has a t-distribution.2The Error Components Model with a Small Number of Groups We begin with a standard time-series/cross-section model of the formY is=a+X sβ+Z isγ+αis+εis.(1)whereαis is an error term that is correlated within group s andεis is an individual-specific term that is independent of the other errors.With a single cross-section Y might be income,X state laws and Z characteristics of individuals.In this case it would be natural to follow Moulton and assume thatαis a state effect that does not vary among members in a group,that is thatαis=Σiαis/N s≡αs.We do not require that the error term take the Moulton structure,only that theσ2α(the variance ofαs) depend only on the number of observations from group s and that,as group size gets large,it converge in probability to somefinite value.3If the covariance matrix of the error term is known,GLS estimation of(1)is efficient.With some regularity conditions,feasible GLS is efficient if the covariance matrix can be estimated consistently.De-pending on the structure of the covariance matrix,GLS can be computationally burdensome.Moreover, if the exact structure of the dependence is unknown,GLS estimation may be infeasible.Estimatingβin two stages is often computationally simpler.In this case,we use OLS to estimatey=Zγ+WΓ+ε(2) where W is a set of dummy variables indicating group membership.Note thatΓ=Xβ+α.(3) We then can use the estimated bΓin GLS estimation ofbΓs=X sβ+αs+(bΓs−Γs)(4) where the error term has varianceσ2αI+var(bΓ).Amemiya(1978)shows that if the covariance matrices ofαandεare known,then the two-step procedure and the GLS procedure applied directly to(1)are numerically identical.If instead feasible GLS is used,then provided the covariance terms are estimated in the same fashion,numerical equivalence continues to hold.More commonly,the two approaches lend themselves to different methods for obtaining consistent estimates of the covariance terms.If so,the equivalence is asymptotic rather than numeric.Our contribution is twofold.First,we can see from(4)that if the number of groups is small,then it is not possible to rely on the consistency of estimates ofσ2αto justify feasible GLS estimation of(4). However,ifσ2αI+var(bΓ)is homoskedastic and diagonal,by the usual arguments,it is still possible to obtain an unbiased estimate of the variance of the error term in(4).Under normality assumptions onαs,the resulting t-statistic will have a t-distribution rather than a normal distribution.We explore circumstances under which the assumption of homoskedasticity is reasonable.In particular,the error term will be homoskedastic under at least two circumstances:1.if the number of observations per group is large,or2.if there are no within-group varying characteristics and the number of observations is the same forall groups.Second,when the error term in(4)is homoskedastic,by standard theorems,OLS estimation of(4) is efficient.Since OLS estimation of(4)is numerically equivalent to feasible GLS estimation of(1),we have full efficiency of estimation even when the number of groups is small.We begin our formal treatment with the case where all variables arefixed within groups.2.1Only Within-Group-Constant Explanatory VariablesWe begin by treating the case where X s is a scalar and there are no within-group varying explanatory variables(γ=0),so thatY is=a+X sβ+αis+εis.(5)This case provides much of the intuition for the more general case.4Throughout we will assume that theεis are independent of each other and ofαfor all i and s.We further assume thatαis andαjs0are independent for s=s0,but do not assume thatαis andαjs are uncorrelated.The two-step estimator in this case has a very simple interpretation.Thefirst-stage is equivalent to taking group means,b d s=P N s i=1Y is s(6) so that the second stage becomesb d s=Y s=a+X sβ+P N s i=1αis N s+P N s i=1εis N s(7)≡a+X sβ+αs+εs(8)≡a+X sβ+ηs.(9) which is just the“between-groups”estimator ofβ.A few points follow immediately from the equivalence of GLS estimation of(5)and(9).1.βcan always be estimated efficiently by appropriate weighted least squares estimation of(9)if theweights are known or by feasible weighted least squares if they can be estimated consistently.2.If eitherηis homoskedastic or var(ηs)is uncorrelated with X s,then the efficient estimator isthe unweighted between estimator.Note that homoskedasticity is a natural assumption whenever either all groups have the same number of observations(N s=N,∀s)or when the number of observations in each group is large.The latter point demonstrates that in many circumstances unweighted between-group estimation is the most efficient estimator5and that this efficient estimator can be achieved without knowledge of the exact covariance structure ofα,although as noted this does require that the variance of ofηs is constant across groups.2.1.1InferenceIt should be apparent that our ability to perform inference on bβdepends primarily on S and not on N s. If the number of groups is large,then the standard theorems establish that whenηis homoskedastic,bβols is normally distributed and the t-statistic follows the normal distribution.Whenηis heteroskedastic, the same is true either for feasible GLS or for appropriately calculated standard errors.In many cases it will be natural to treat S as large and the error term as homoskedastic.For example, studies that use difference in laws across states and have large samples for allfifty states are likely to meet this requirement approximately.However,in many applications the number of groups is small. The well-known Card and Krueger(1994)minimum-wage study is a case in point in which there is a large number of observations per group but only four groups(New Jersey before and after the law and eastern Pennsylvania before and after the law).Other studies(Gruber and Poterba,1994;Card,1990; Eissa and Liebman,1996)are based on a small number of group/year cells.When the number of groups is small,in order to have a standard solution for the distribution of the t-statistic,we require thatηbe i.i.d.normally distributed.Below,we present formal sufficient conditions for this requirement to be satisfied.If the distribution ofηis i.i.d.normal,then it follows from standard theorems that the t-statistic for bβhas a t-distribution with S−2degrees of freedom.In effect,failure to recognize that the variance of the error term is estimated using very few observations can dramatically overstate the significance of findings.Why does the distribution of b T remain t despite the large number of observations?The answer is quite intuitive.If we relied on published census data to estimate a relation based on the New England states,we would automatically assume that the resulting t-statistic had a t-distribution.Relying on the underlying individual data cannot help us if all of the information in the data is included in the mean.Somewhat more formally,rewrite(9)ase Y s=e X sβ+eηs(10)where˜denotes a deviation from the mean.The usual t-statistic for hypotheses concerningβis given byˆT=ˆβ−βˆση(P s X2s)1/2,ˆσ2η=1S−2S X s=1(e Y s−e X s bβ)2.(11) Given this fact,we can easily see that it will be reasonable to use a t(S−2)distribution for conducting inference wheneverηs is exactly or is approximately a homoskedastic normal random variable.Finite Sample Result:Here for theˆT statistic to have an exact t(S−2)distribution it is sufficient thatηs=P N s i=1αis N s+P N s i=1εis N s∼N(0,σ2η)where it is important thatσ2ηis constant across s.Although there may be a variety of conditions the most obvious case is whereαis=αs∼N(0,σ2α)for all i,εis∼N(0,σ2ε)and N s=N for all s so thatηs∼N(0,σ2η)where,σ2η=σ2α+σ2ε(12)This is the standard random effects time-series/cross-section model as well as the specification used by Moulton.This includes the possibility that there are no group specific effects.Large Sample Result:For theˆT statistic to have a distribution that is well approximated by t(S−2)it is sufficient that there be large N s and that,ηs A∼N(0,σ2η)(13) which requires some form of asymptotic theory regardingηs with N s→∞but with the number of groupsfixed.Here there are at least two interesting possibilities.(i)For each s,αis=αs∼N(0,σ2α)for all i andεis satisfy conditions for a Law of Large Numbers toimply thatp limN s→∞P N s i=1εis s=0(14) In this instancep limN s→∞ηs=αs∼N(0,σ2α)so that the condition(13)is met.This does not require that N s be the same in all groups but for the approximation to be valid we would need all groups to have large N s so that(14)is approximately true.(ii)For each s,αis=αs∼N(0,σ2α/N s)for all i,εis satisfy conditions for a Central Limit Theorem and N s/N t→1for any s=t then,ηs A∼N(0,(σ2α+σ2ε)/N s)where the variance asymptotically does not depend on s.This case is possible under a parameter sequence that keepsσ2αof the same(or smaller)order asσ2ε/N s as might be appropriate when the group effects are relatively small.Indeed this includes the possibility thatαs=0so that there are no group specific effects Also note that in this case we do not require normality for theεis but for the approximation to be a good one we require that there be similar numbers of observations per group.There may be other possibilities in the large sample case although we refrain from giving more explicit conditions.For instance provided that one can show that if(P iαis)/N s is approximately normal(with constant variance independent of s asymptotically)and thatεis satisfy conditions for a Law of Large Numbers then the condition in(13)will hold and using a t(S−2)will provide a good approximation. There are a variety of possibile assumptions one could use to obtain the approximate normality of (P iαis)/N s that relate to the dependence across observations within a group—the simplest case is given in(i)above,but it is apparent that the two-stage technique can accommodate inference even when the nature of the dependence within groups is unknown provided that there is no correlation of errors across groups.6Also,as in case(ii)for this result to hold one would require N s to be(asymptotically) constant across groups.It is worth noting that estimating the between groups estimator is a matter of convenience.When N s=N,the between-groups and OLS estimators are identical.If N s/N t→1∀s,t,then OLS converges to the between-groups estimator.Therefore,it is possible to calculate a corrected standard error for the OLS estimate and generate a t-statistic that has a t-distribution.The between-groups estimator is, however,much more convenient.2.2Variables that Vary Within-GroupWe consider now hybrid models in which some variables differ across observations within groups.For simplicity we ignore complications associated with nonconstant correlation of the group effect since they do not add to the analysis.As was seen in the discussion without within-group varying variables,all that is important is the variance of the mean group error.Thus we analyze the standard Moulton modelY is=a+X sβ+Z isγ+αs+εis.(15)We assume for simplicity that Y is and X s are scalars.The extension to the case of more than one group-varying or group variable is straightforward.We further assume thatαs∼N(0,σ2α)for all sεis∼N(0,σ2ε)for all i,sand that these residuals are mutually independent for all i and s.As before we know that GLS estimation of(15)is efficient and that if we can obtain consistent estimates ofσ2αandσ2εfeasible GLS estimation is asymptotically efficient.Finally,we know from Amemiya that if these variance terms are estimated in the same way that feasible GLS estimation is numerically identical to the following estimator—first use OLS to estimate the“within-group”estimate ofγY is=d s+Z isγ+εis.(16) Then estimateβby feasible GLS estimation of¯Ys−¯Z0sˆγ=b d s=a+βX s+u s(17) where,¯Y=P N s i=1Y is s,¯Z s=P N s i=1Z is s,sb d s is the estimate of d s in(16)and var(u)=σ2αI+Σe dwhereΣe d is the covariance matrix of thefixed-effect parameter estimates.Note that estimates ofΣe d can be obtained by selecting the covariance matrix corresponding to the fixed effects.σ2a can be estimated byfirst estimating(17)by OLS and then usingP S i=1b u2i−1S X i=1var(b d i)=bσ2awhere var(b d i)is the variance of the i thfixed effect and K is the number of explanatory variables in(17). Finally,it is worth noting that since the groups are distinct,covariance of the b d0s arises only because γis estimated rather than known.If each bγi is calculated from a separate sample,the covariances will be zero.Thus the covariance problem can be avoided by estimatingγfor the s th state asˆγ(s)=(Z0s M s Z s)−1Z0s M s Y s(18) whereM s=I Ns−ιN s(ι0N sιN s)−1ι0N sis a vector of10s of length N s.andιNsSinceˆγW involves the restriction thatγis constant across states,it is more efficient but less robust.7 Our focus,however,is not on estimation ofγbut ofβ.When the number of groups is large,it is possible to estimate(17)by feasible GLS.If the number of groups is small,then we can still get efficient estimates and determine the distribution of the t-statistic if the error term in(17)is i.i.d.normal except possibly for an error term common to all groups.The following propositions summarize conditions under which this condition holds and the resulting t-statistic has the t-distribution.Let b T1be the t-statistic using bγw and b T2be the t-statistic using bγ(s).Proposition1:If theεis are normally distributed,(i)ˆT1∼t(S−2)when N s are identical for all s and either(a)there are no Z is or(b)¯Z s is constantacross s(ii)ˆT2∼t(S−2)when N s are identical for all s and either(a)there are no Z is or(b)¯Z0s(Z0s M s Z s)−1¯Z s is constant across sWe can also show that the statistics will have asymptotic t(S−2)distributions under more general situations so that the t(S−2)distribution can be used quite generally.Proposition2:If theεis are not normally distributed and ifσ2αisfixed,thenˆT j A∼t(S−2)(for j=1,2)when N s→∞for all s.As in the case where there are no individual specific covariates one can show that the statisticsˆT j will be approximately t(S−2)when the group specific errors are small relative to the idiosyncratic errors.The conditions that give rise to this are essentially asymptotic analogs of the conditions in Proposition 1.Proposition3:Ifσ2αis small in the sense thatσ2α=O(N−1/2s)then regardless of the distribution of εis(i)ˆT1A∼t(S−2)when N s are asymptotically identical8for all s and either(a)there are no Z is or(b)p lim¯Z s is constant across s(ii)ˆT2A∼t(S−2)when N s are asymptotically identical for all s and either(a)there are no Z is or(b) p lim¯Z0s(Z0s M s Z s/N s)−1¯Z s is constant across sThese situations can be stated with reference to the residuals in the second-stage equation:¯Ys−¯Z0sˆγ=a+βX s+αs+εs+¯Z0s(γ−ˆγ).(19) For the distribution of the t-statistic to be exactly t,we require that the error term be normally distributed and i.i.d.except possibly for a common component for all observations.When all groups have the same sample size,we can readily check whether:¯Zis identical across groups,in the case where we use the within estimator to obtain bγw,or svar(bγ(s))is identical for all groups,in the case whereγis estimated separately for each group.The t(S−2)can be justified as an approximation based on large N s asymptotics under more general conditions.This occurs because the error term in(19)converges to the homoskedastic normal errorαs as N s→∞because of the consistency ofˆγand the fact thatεs p→0by the usual Law of Large Numbers. Whenσ2αis small,as in Proposition3,the approximation can be justified because¯εs and¯Z0s(γ−ˆγ) are approximately normally distributed by the Central Limit Theorem so that the error term in(19)is approximately normal.While the theory above and the Monte Carlo evidence below suggest that using one of the two-stage estimators will generally be preferable to using OLS,there are two caveats which must be recognized. First,whenσ2α=0,OLS is the efficient estimator of equation(15)and the t-statistic has the conventional distribution.If one knows thatσ2α=0,OLS is the preferred estimator.What Proposition3tells us is that if we proceed under the mistaken belief thatσ2α>0,two-stage estimation will still produce a statistic with a t-distribution.Second,the distinction between two-stage estimation and one-stage estimation is really one of con-venience.Whenever b T1has a t-distribution,the same statistic can be produced by relying on the OLS coefficients.For example,in the case where there are no group-varying covariates and each group has the same number of observations,the OLS coefficient is identical to the between-groups(two-step)estima-tor.However,it is more convenient to estimate the standard error of the estimate using between-group estimation.Similarly,it is easy to show that when¯Z s is identical across groups and all groups have the same sample size,OLS produces the same bβas in the case where we use the within estimator to obtain bγw and estimateβin two steps.Again,however,estimation of the correct standard error is much easier using the two-step estimator.3ExamplesIn this section,wefirst review the two by two case,which features prominently in the literature.The main feature of this case is that we cannot calculate the standard error of the estimate and thus must exercise considerable caution in drawing conclusions.We then review two prominent papers that provide at least some differences-in-differences estimates in which there are no covariates that vary within group. Thefirst case,Gruber and Poterba(1994),shows that accounting properly for error components can dramatically reduce the implied precision of the estimates in some specifications but that the estimate remains precise in at least one specification.In the second case we reexamine Card’s(1990)Mariel boatlift study and suggest that the data cannot exclude large effects of the migration on blacks in Miami.This is consistent with the results of Angrist and Krueger’s(1999)finding of a large impact of the“Mariel boatlift that didn’t happen.”3.1The Two by Two CaseIn the canonical differences-in-differences model,mean outcomes are calculated for groups A(the treat-ment group)and B(the control group)in each of periods0(the pre-treatment period)and1(the post-treatment period).A standard table shows each of these means,plus the difference between groups A and B in each period and the difference between the pre-and post-treatment outcomes for each group.Finally the difference between either pair of differences is the classic differences-in-differences estimate.Classic and recent examples that include tables in this form are Card and Krueger’s study of the minimum wage(1994),Eissa and Leibman’s study of the effect of the earned-income tax credit (1996),Meyer et al’s study of workers’compensation(1995),Imbens et al’s study of lottery winners andlabor supply(2001),Eberts et al’s study of merit pay for teachers(2002)and Finkelstein’s(2002)study of tax subsidies and health insurance provision.Each of these studies provides additional analysis,but in each case,the two by two analysis is an important component of the study.In a well developed two by two case,the authors make a compelling case that other than the treat-ment,there is no reason to expect the outcome variable to evolve differently for the treatment and control groups.The statistical model isy igt=αgt+bT gt+εigt(20)where y is the outcome being measured for individual i in group g in year t,T is a dummy variable for the treatment group in the post-treatment period.αis a group/year error which may be correlated over time or across groups andεis an i.i.d.error term.Without loss of generality,we can subtract thefirst period from the second period and rewrite the equation as∆y ig=∆a g+b∆T g+εig1−εig0.(21) Now∆T equals1for the treatment group and0for the control group.Therefore we can replace∆T with A,a dummy variable for group A.And we can rewrite∆a g=c+eαA,where eα=∆αA−∆αB. Lettingεig2−εig1=εig,we have∆y ig=c+(eα+b)A g+εig.(22)The weighted least squares(where weights are chosen to make the sample sizes identical)coefficient on A is the differences-in-differences estimator and is numerically identical to taking the difference between the change in the outcome for the treatment and control groups.It is an unbiased estimate of eα+b. Since E(eα)=0,it is also an unbiased estimate of b.However,it is not consistent.No matter how many observations from either the control or treatment groups we add to the sample,the coefficient will not converge in probability to b.The variance reported by econometric packages includes the sampling variance but not that part of the variance due to the common error.Thus if there are any shocks that are correlated within year/group cells,the reported t-statistic will be too high.We will tend tofind an effect of the treatment even if none exists.Unfortunately,if there are common errors,the two by two model has zero degrees of freedom.Therefore it is not possible to determine the significance of any estimate solely from within-sample information.It may be possible to use information from outside the sample to get a plausible estimate of the magnitude of common within-group errors,but even in this case,we will not know the sampling distribution of the resulting statistic.Thus analysis of the two by two case requires extreme caution.3.2Card(1990)Card examines the impact of the mass migration of Cubans to Miami during the Mariel boatlift.He compares,among other outcomes,unemployment rates for whites,blacks and Hispanics in Miami with unemployment rates of these groups in four comparison cities(Atlanta,Houston,Los Angeles and Tampa-St.Petersburg).Surprisingly,hefinds little evidence that the mass migration significantly affected the Miami labor market.For example,from1979to1981black unemployment in Miami increased by1.3 percentage points compared with2.6percentage points in the comparison communities.Angrist and Krueger(1999)replicate Card’s study for a Cuban boatlift that was anticipated but did not occur.They find that“the Mariel boatlift that didn’t happen”had a large adverse effect on unemployment in Miami. Their analysis cast doubt on the power of Card’s originalfinding.Our analysis helps to explain why Card found no effect and why it is possible tofind a large effect of a nonexistent event.To understand this,we need to examine the true confidence interval around Card’s estimates.Because Card provides seven years of data for both Miami and the comparison cities,we can, with auxiliary assumptions,calculate the variance of his estimate.Wefirst assume that the difference between the annual unemployment rates in Miami and the com-parison cities is subject to an i.i.d.shock.This allows for a common year shock which may be persistent but assumes that any shocks that are idiosyncratic to a city are not persistent.Given this assumption, we use the data reported by Card to regress the difference between the unemployment rate for blacks in Miami and the control cities on a dummy for the period after1980on all years except1980.The resulting coefficient is1.4with a standard error of4.0.Under the assumption that the error terms are homoskedastic and normal,and given that we have four degrees of freedom,the confidence interval is from-9.7to12.1,effectively including very large positive and negative impacts on blacks.In sum,while the data certainly provide no support for the view that the Mariel immigration dra-matically increased unemployment among blacks in Miami,they do not provide much evidence against this view either.In this case,the differences-in-differences approach lacks power.。
Chapter 11 Second Language Acquisition

•
L1
FL/SL(TL)
•
•
Interlanguage (IL)
•
NL: TL comparisons ( Contrastive Analysis)
•
IL: TL comparisons (Error Analysis)
•
NL:IL comparisons (Transfer Analysis)
11.3. Contrastive analysis (CA) (1960s)
11.4 Error analysis (EA)
• Error analysis involves, first independently or objectively,
describing the learners’ interlanguage and the target language, then a comparison of the two languages is followed to locate mismatches. Different from contrastive analysis, error analysis gave less consideration to learners’ native language.
11. Some students do not care others. 12. They are busy searching jobs. 13. It has been existed for a long time. 14. The bus is arrived. 15. You should return the library the books. 16. He reported the police the accident. 17. I forgot the car accident happened at the corner of that
- 1、下载文档前请自行甄别文档内容的完整性,平台不提供额外的编辑、内容补充、找答案等附加服务。
- 2、"仅部分预览"的文档,不可在线预览部分如存在完整性等问题,可反馈申请退款(可完整预览的文档不适用该条件!)。
- 3、如文档侵犯您的权益,请联系客服反馈,我们会尽快为您处理(人工客服工作时间:9:00-18:30)。
A Rhetorical Analysis of Abraham Lincoln’s Second InauguralAddressAbstract: Abraham Lincoln delivered his second inaugural address on March 4, 1865, during his second inauguration as president of the United States. This address is planned; it is adapted to his audience; it is shaped by Lincoln’s motives; it seeks persuasion. Lincoln made full use of argument, appeals, arrangement, and aesthetics which are four resources of symbols assisting the goal of persuasion. Thus it is categorized as rhetoric. This thesis makes a rhetorical analysis of Abraham Lincoln’s Second Inaugural Address.Key words:Abraham Lincoln’s second inaugural address, rhetoric, analysis1. IntroductionThe custom of delivering an address on Inauguration Day started with the very first Inauguration—George Washington's—on April 30, 1789. After taking his oath of office on the balcony of Federal Hall in New York City, Washington proceeded to the Senate chamber where he read a speech before members of Congress and other dignitaries. Every President since Washington has delivered an Inaugural address. While many of the early Presidents read their addresses before taking the oath, current custom dictates that the Chief Justice of the Supreme Court administer the oath first, followed by the President's speech. Today, Presidents deliver their Inaugural address on the west front of the Capitol, but this has not always been the case. Until Andrew Jackson's first Inauguration in 1829, most Presidents spoke in either the House or Senate chambers. Jackson became the first President to take his oath of office and deliver his address on the east front portico of the U.S. Capitol in 1829. With few exceptions, the next 37 Inaugurations took place there, until 1981, when Ronald Reagan's swearing-in ceremony and Inaugural address occurred on the west front terrace of the Capitol. The west front has been used ever since.Abraham Lincoln delivered his second inaugural address on March 4, 1865, during his second inauguration as president of the United States. At a time when victory over the secessionists in the American Civil War was within days and slavery was near an end, Lincoln did not speak of happiness, but of sadness. Some see this speech as a defense of his pragmatic approach to Reconstruction, in which he sought to avoid harsh treatment of the defeated South by reminding his listeners of how wrong both sides had been in imagining what lay before them when the war began four years earlier. Lincoln balanced that rejection of triumphalism, however, withrecognition of the unmistakable evil of slavery, which he described in the most concrete terms possible. This address is planned, adapted to his audience, shaped by Lincoln’s m otives, responsive to a situation and persuasion-seeking. Especially he made full use of argument, appeals, arrangement, and aesthetics including metaphor, allusion, and consonance.2. The characteristics of Abraham Lincoln’s Second Inaugural Address2.1It is planned―Regardless of the goal at which it aims, r hetorical discourse involves forethought or planning‖ (Herrick, 2005, P.8). This address was planned before Lincoln addressed to his countrymen rather than improvisational. From the pictures on Wikipedia, we can see that Lincoln stood in the center, with papers in his hand during addressing.2.2 It is adapted to an audience―Rhetoric is planned with audience in mind. Audience should not be understood strictly in the traditional sense of a large group of people seated in rows of chairs in a large hall‖ (Herrick, 2005, P.8). At the beginning of the address, Lincoln pointed out hi s audience ―Fellow-countrymen‖ that were not only the audience around him, but also other Americans who were not present at the scene. ―Rhetorical discourse, then, forges links between the rhetor’s views and those of an audience. This means attending to an audience’s values, experiences, beliefs, social statues and aspiration‖(Herrick, 2005, P.8). His address was mainly about The Civil War, which the Americans was suffering and worrying about. Another apparent example is he mentioned many times of God and Bible.Most Americans believed in God, so his audience could understand the address easily. ―The progress of our arms, upon which all else chiefly depends, is as well known to the public as to myself, and it is, I trust, reasonably satisfactory and encouraging t o all.‖Through this sentence, we can see that all Americans including Lincoln held the same belief that the North would won, and he ensured success because their arms were strong. The same experience, belief, and confidence about their success were forged between Lincoln and his audience.2.3 It reveals Lincoln’s motives―In rhetoric we find people acting symbolically in response to their motives, a general term taking in commitments, goals, desires, or purposes that lead to action. Rhetors address audiences with goals in mind, and the planning and adaptationprocesses that mark rhetoric are governed by the desire to achieve these goals‖(Herrick, 2005, P.8). At the end of the address, Lincoln expressed his motives as a president of United States that experienced the Civil War. ―With high hope for the future, no prediction in regard to it is ventured.‖The North and the game in their hands. He called on all Americans ―let us strive on to finish the work we are in, to bind up the nation's wounds, to care for him who shall have borne the battle and for his widow and his orphan, to do all which may achieve and cherish a just and lasting peace among ourselves and with all nations.‖2.5 It seeks persuasion―Rhetorical discourse is usually inten ded to influence an audience to accept an idea, and then to act in a manner consistent with that idea.‖(Herrick, 2005, P.12).Arguments, appeals, arrangements, and aesthetics are four resources of symbols assisting the goal of persuasion.2.5.1Argument―An argument is made when a conclusion is supported by reasons. An argument is simply reasoning made public with the goal of influencing an audience‖ (Herrick, 2005, P.13). Most Americans believes in God; God is almighty. In his address, Lincoln quoted many times of God and Bible to make his argument more persuasive, such as ―Both read the same Bible and pray to the same God, and each invokes His aid against the other.‖ ―It may seem strange that any men should dare to ask a just God's assistance in wringing their bread from the sweat of other men's faces, but let us judge not, that we be not judged.‖ ―That of neither has been answered fully. The Almighty has His own purpos es‖ and so on. In the second paragraph Lincoln began the shift in substance and tenor that would give this address its remarkable meaning. He employed several rhetorical strategies that guided and aided the listener. First, Lincoln's approach was to emphasize common actions and emotions. In this paragraph he used "all" and "both" to include North and South. Second, Lincoln used the word "war" nine times. The centrality of war is because the word appears in every sentence. Previously war had been used as the direct object, both historically and grammatically, of the principal actors. In his speech, however, war became the subject rather than the object. The second paragraph concludes, "And the war came." In this brief, understated sentence, Lincoln acknowledged that the war came in spite of the best intentions of the political leaders of the land. When Lincoln introduced the Bible, early in the third paragraph, he entered new territory in presidential inauguraladdresses. Before Lincoln there were eighteen inaugural addresses delivered by fourteen presidents. Each referred to God or the deity. The Bible, however, had been quoted only once.2.5.2 Appeals―Appeals are those symbolic strategies to elicit an emotion or to engage the audience’s loyalties or commitments‖(Herrick, 2005, P.1). Slavery was the direct reason of the Civil War, and African-Americans were the firm supporters of the war, so Lincoln said ―One-eighth of the whole population were colored slaves, not distributed generally over the Union, but localized in the southern part of it. These slaves constituted a peculiar and powerful interest. All knew that this interest was somehow the cause of the war‖ to arouse African-Am ericans’ commitments to the United States. He was opposed to Slavery and God was on his side―If we shall suppose that American slavery is one of those offenses which, in the providence of God, must needs come, but which, having continued through His appointed time, He now wills to remove, and that He gives to both North and South this terrible war as the woe due to those by whom the offense came, shall we discern therein any departure from those divine attributes which the believers in a living God always ascribe to Him?‖ His determination won all African-Americans’ support.2.5.3 Arrangement―Arrangement refers to the planned ordering of a message to achieve the greatest effect, whether of persuasion, clarity or beauty‖(Herrick, 2005, P.14).At the beginning of the address, Lincoln stated that it was the second time for him to take the oath of the Presidential office; then he introduced the war; he used God and Bible to support his determination –getting rid of Slavery; at last he called all Americans to reconstruct their country. He put the most important part, his appeal, at the end, to make his audience more impressive and emotional. Of course, he made it."Mr. Lincoln, that was a sacred effort." So spoke Frederick Douglass ①soon after he heard Lincoln’s second inaugural address.2.5.4 Aesthetics―The aesthetics of rhetoric are elements adding form, beauty, and force to symbolic expression‖ (Herrick, 2005, P.14). Lincoln engaged in aesthetic resources of①Frederick Douglass(born Frederick Augustus Washington Bailey, c. February 1818 – February 20, 1895) was an American social reformer, orator, writer and statesman. After escapingfrom slavery, he became a leader of the abolitionist movement, gaining note for his dazzling oratory and incisive antislavery writing.language in a traditional way to make his speech more beautiful and thus more moving and memorable. ―Fondly do we hope, fervently do we pray , that this mighty scourge of war may speedily pass awa y‖,in this sentence, ―pray‖rhymes with―away‖; he compared the chaos resulted from the war to―mighty scourge‖, and this metaphor which clearly informed people the consequences of the war added vividness to his speech. Parallelism is also obvious in this address, such as ―All dreaded it, all sought to avert it‖and ―Fondly do we hope, fervently do we pray‖.Lincoln wondered what God’s will might have been in allowing the war to come, and why it had assumed the terrible dimensions it had taken. He endeavored to address some of these dilemmas, using allusions taken from the Bible. These words ―wringing their bread from the sweat of other men’s faces‖ is an allusion to the Fall of Man in the Book of Genesis. As a result of Adam's sin, God tells Adam that henceforth "In the sweat of thy face shalt thou eat bread, till thou return unto the ground; for out of it wast thou taken: for dust thou art, and unto dust shalt thou return" (Gen. 3:19, King James Version). Lincoln's phrase, "but let us judge not, that we be not judged," is an allusion to the words of Jesus in Matthew 7:1, which in the King James Version reads, "Judge not, that ye be not judged." Lincoln quotes another of Jesus' sayings: "Woe unto the world because of offenses; for it must needs be that offenses come, but woe to that man by whom the offense cometh." Lincoln's quoted language comes from Matthew 18:7 in the King James Bible; a similar discourse by Jesus appears in Luke 17:1 in the King James Bible. The quotation "the judgments of the Lord are true and righteous altogether" is from Psalm 19:9 in the King James Bible.The original is ―The fear of the LORD is clean, enduring for ever: the judgments of the LORD are true and righteous altogether.‖3. ConclusionAbove all, Lincoln’s second inaugural address is categorized as a typical rhetoric not only it is planned, adoptive to his audience, and shaped by his motives, persuasion seeking, but also he used a perfect persuasion mode which is revealed by arguments, appeals, arrangements, and aesthetics. As a rhetorical discourse, it was a surprisingly brief but profound reflection on the meaning of the Civil War that speculated on the purposes of God to help reunite the country.The Inaugural address plays a very important role in the inauguration and term of office of American presidents. It shows the new president’s intention and confidenceand i nspires people to move forward under new leadership. The inaugural addresses are regarded as rhetorical discourses and analyzed many times by linguistists, of course including Lincoln’s second inaugural address. This thesis analyze s it from the perspective of its characteristics as a rhetorical discourse. Finally I hope that the thesis would be helpful to those who are interested in rhetoric, inaugural addresses, and even politics.BibliographyJames A. Herrick (2005). The History and Theory of Rhetoric: An Introduction (2nd ed.). Allyn & Bacon 2005. 8—14Abraham Lincoln's second Inaugural Address. Retrieved May, 12, 2013 from /wiki/Abraham_Lincoln's_second_inaugural_addressLincoln’s “Almost Sacred Effort”: The Second Inaugural Address. Retrieved May, 12, 2013 from/groups/literacy/blog/2013/03/04/lincolns-almost-sacred-eff ort-the-second-inaugural-address。