Fusion of multimodal visual cues for model-based object tracking
基于驾驶场景的高效多模态融合检测方法
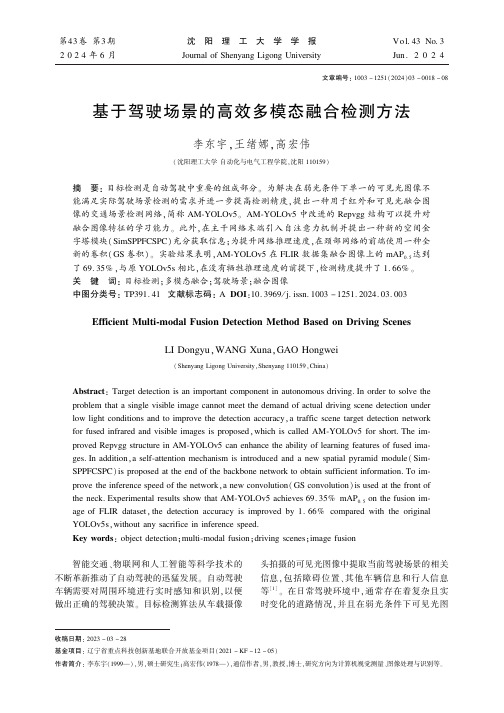
第43卷第3期2024年6月沈㊀阳㊀理㊀工㊀大㊀学㊀学㊀报JournalofShenyangLigongUniversityVol 43No 3Jun 2024收稿日期:2023-03-28基金项目:辽宁省重点科技创新基地联合开放基金项目(2021-KF-12-05)作者简介:李东宇(1999 )ꎬ男ꎬ硕士研究生ꎻ高宏伟(1978 )ꎬ通信作者ꎬ男ꎬ教授ꎬ博士ꎬ研究方向为计算机视觉测量㊁图像处理与识别等ꎮ文章编号:1003-1251(2024)03-0018-08基于驾驶场景的高效多模态融合检测方法李东宇ꎬ王绪娜ꎬ高宏伟(沈阳理工大学自动化与电气工程学院ꎬ沈阳110159)摘㊀要:目标检测是自动驾驶中重要的组成部分ꎮ为解决在弱光条件下单一的可见光图像不能满足实际驾驶场景检测的需求并进一步提高检测精度ꎬ提出一种用于红外和可见光融合图像的交通场景检测网络ꎬ简称AM ̄YOLOv5ꎮAM ̄YOLOv5中改进的Repvgg结构可以提升对融合图像特征的学习能力ꎮ此外ꎬ在主干网络末端引入自注意力机制并提出一种新的空间金字塔模块(SimSPPFCSPC)充分获取信息ꎻ为提升网络推理速度ꎬ在颈部网络的前端使用一种全新的卷积(GS卷积)ꎮ实验结果表明ꎬAM ̄YOLOv5在FLIR数据集融合图像上的mAP0.5达到了69.35%ꎬ与原YOLOv5s相比ꎬ在没有牺牲推理速度的前提下ꎬ检测精度提升了1.66%ꎮ关㊀键㊀词:目标检测ꎻ多模态融合ꎻ驾驶场景ꎻ融合图像中图分类号:TP391.41文献标志码:ADOI:10.3969/j.issn.1003-1251.2024.03.003EfficientMulti ̄modalFusionDetectionMethodBasedonDrivingScenesLIDongyuꎬWANGXunaꎬGAOHongwei(ShenyangLigongUniversityꎬShenyang110159ꎬChina)Abstract:Targetdetectionisanimportantcomponentinautonomousdriving.InordertosolvetheproblemthatasinglevisibleimagecannotmeetthedemandofactualdrivingscenedetectionunderlowlightconditionsandtoimprovethedetectionaccuracyꎬatrafficscenetargetdetectionnetworkforfusedinfraredandvisibleimagesisproposedꎬwhichiscalledAM ̄YOLOv5forshort.Theim ̄provedRepvggstructureinAM ̄YOLOv5canenhancetheabilityoflearningfeaturesoffusedima ̄ges.Inadditionꎬaself ̄attentionmechanismisintroducedandanewspatialpyramidmodule(Sim ̄SPPFCSPC)isproposedattheendofthebackbonenetworktoobtainsufficientinformation.Toim ̄provetheinferencespeedofthenetworkꎬanewconvolution(GSconvolution)isusedatthefrontoftheneck.ExperimentalresultsshowthatAM ̄YOLOv5achieves69.35%mAP0.5onthefusionim ̄ageofFLIRdatasetꎬthedetectionaccuracyisimprovedby1.66%comparedwiththeoriginalYOLOv5sꎬwithoutanysacrificeininferencespeed.Keywords:objectdetectionꎻmulti ̄modalfusionꎻdrivingscenesꎻimagefusion㊀㊀智能交通㊁物联网和人工智能等科学技术的不断革新推动了自动驾驶的迅猛发展ꎮ自动驾驶车辆需要对周围环境进行实时感知和识别ꎬ以便做出正确的驾驶决策ꎮ目标检测算法从车载摄像头拍摄的可见光图像中提取当前驾驶场景的相关信息ꎬ包括障碍位置㊁其他车辆信息和行人信息等[1]ꎮ在日常驾驶环境中ꎬ通常存在着复杂且实时变化的道路情况ꎬ并且在弱光条件下可见光图像包含的目标信息不足[2]ꎬ依靠单一视觉传感器的信息不能满足全天候检测的需求ꎬ于是针对多模态图像的目标检测任务逐渐受到关注ꎮ可见光图像包含丰富的细节信息但容易受到环境光线影响ꎻ红外图像可以突出目标信息ꎬ抗干扰能力强ꎬ但环境细节信息不足ꎮ而可见光图像和红外图像两种模态融合得到的融合图像同时具有两种模态的特点信息ꎬ对于目标检测任务有很大的增益ꎬ同时相比于可见光-激光雷达的多模态融合方式可以保留更多的视觉信息且方便部署ꎮ然而可见光红外融合图像具有的特征信息比单一可见光图像和红外图像复杂ꎬ对目标检测算法在准确度和推理速度方面提出了更高要求ꎮRedmon等[3]提出的YOLO系列算法ꎬ尤其是YOLOv5ꎬ在目标检测方面具有优异的性能ꎮYOLOv5的主干网络采用CSPDarknet53结构ꎬ颈部网络部分采用的是PANet结构ꎬ检测头为Yoloheadꎮ由于其兼顾了准确性和较快的检测速度ꎬ已成为单阶段目标检测算法的代表ꎬ尽管YOLOv5性能已经很优秀ꎬ但在处理复杂的交通环境中可见光红外融合图像信息时ꎬ原始网络的特征学习能力不足ꎮ本文选取YOLOv5作为基础网络并针对日常自动驾驶任务需求进行改进ꎬ简称为AM ̄YOLOv5ꎬ解决复杂驾驶场景融合图像的目标检测任务ꎮ算法在特征提取和学习方面加入了更高效的结构和模块ꎬ将多分支学习结构与一种改进的空间金字塔模块(SimSPPFCSPC)引入到主干网络中ꎬ实现了多分支的学习能力ꎬ在提高特征学习能力的同时不影响推理速度ꎬ提高计算效率ꎮ同时ꎬ对于图片中目标可能被遮挡的问题ꎬ本文将多注意力C3模块引入到主干网络中ꎬ使网络充分获得全局信息和丰富的上下文信息ꎮ此外ꎬ本文在网络的颈部使用了GS卷积ꎬ优化网络参数以提高效率ꎬ并使网络能够更好地学习多尺度特征ꎮ1㊀AM ̄YOLOv51.1㊀整体网络结构AM ̄YOLOv5在网络的主干部分将改进的Repvgg模块(Replite)与YOLOv5整合在一起ꎬ末端引入自注意力机制ꎬ加入了更高效的多注意C3模块(C3TR)和SimSPPFCSPCꎮ在此基础上ꎬ网络颈部的前端普通卷积被GS卷积替代ꎮAM ̄YOLOv5算法的结构如图1所示ꎮ1.2㊀高效特征提取主干网络1.2.1㊀改进的Repvgg模块主干网络设计对于自动驾驶场景多模态融合图像的目标检测至关重要ꎮ多分支结构的训练网络[4]虽然能获得较高的性能提升ꎬ但因为内存占用增加导致推理速度下降ꎮ普通单路径结构的网络由于没有分支ꎬ特征提取能力偏弱ꎬ但在操作完成后可以立即释放输入占用的内存ꎬ通过牺牲性能可实现较高的推理效率ꎮDing等[5]提出的结构重参数化可有效解决该问题ꎬ如图2(a)所示ꎬ训练结构由多个Repvgg模块组成ꎬ每个Repvgg模块一般由一个3ˑ3卷积ꎬ一个1ˑ1卷积和一个恒等分支组成ꎮ推理结构如图2(b)所示ꎬ原来的多分支结构经过重参数化操作等价变成了由多个3ˑ3卷积组成的单路经结构ꎮ通常在无重参数化的卷积神经网络算法中ꎬ卷积层参数在训练和推理时不会发生变化ꎬ卷积层参数相关公式如下ꎮPc=(K2ˑC+b)ˑN(1)Wout=Win+2p-ws+1(2)Hout=Hin+2p-hs+1(3)Dout=k(4)式中:Pc是卷积层所有参数量ꎻK代表卷积核尺寸ꎻC代表输入通道数ꎻN代表过滤器数量ꎻK2ˑC代表权重数量ꎻb代表偏置数量ꎻWin和Hin是输入层参数ꎻWout㊁Hout和Dout是经过卷积后的输出层参数ꎻk是卷积核个数ꎻp是填充大小ꎻs是步长ꎻw和h是卷积核参数ꎮ重参数化意味着卷积层参数发生相应改变ꎬ将卷积层与批量归一化(BN)层融合并对恒等分支转换如下ꎮWᶄi=γiσiWi(5)bᶄi=-μiγiσi+βi(6)式中:Wi是转换前的卷积层参数ꎻσi代表BN层标卷积准差ꎻμi代表BN层累计平均值ꎻγi和βi分别代表BN层学习的比例因子和偏差ꎻWᶄi和bᶄi分别代表融合后卷积层的权重与偏置ꎬi代表操作范围为所有通道ꎮ㊀㊀具有残差和串联连接的层不应存在恒等分支ꎬ会导致不同特征图存在更多的梯度多样性[6]ꎬ所以本研究提出了改进的Repvgg模块ꎬ去除91第3期㊀㊀㊀李东宇等:基于驾驶场景的高效多模态融合检测方法图1㊀AM ̄YOLOv5结构Fig.1㊀ThestructureofAM ̄YOLOv5图2㊀Repvgg在不同任务时的结构Fig.2㊀ThestructureofRepvggunderdifferenttasksRepvgg模块的恒等分支ꎬ只保留两个卷积分支ꎬ并命名为Replite模块ꎬ用其替换YOLOv5主干网络中末端的两个3ˑ3卷积块ꎬ以实现多分支训练结构与单路径推理结构的转换ꎬ不明显增加推理速度的同时提升网络对于细节特征的学习能力ꎮ训练阶段如图3(a)所示ꎬ由3ˑ3卷积和1ˑ1卷积组成的多分支结构可以被看作是多浅层模型的集成ꎬ有利于解决梯度消失问题ꎬ提高模型的准确度和训练时的算法性能ꎮ推理阶段采用与Repvgg同样的重参数化方法将原来的1ˑ1卷积融入到3ˑ3的卷积中ꎬ变回单路径结构ꎬ如图3(b)所示ꎮ图3㊀带有Replite模块的主干网络在不同任务时的结构Fig.3㊀ThestructureofthebackbonenetworkwithReplitemodulesunderdifferenttasks02沈㊀阳㊀理㊀工㊀大㊀学㊀学㊀报㊀㊀第43卷1.2.2㊀多注意力C3模块为使网络能够充分获得全局信息和丰富的上下文信息ꎬ提高对融合图像的检测能力ꎬ本研究受到VisionTransformer的启发ꎬ将自注意力机制引入YOLOv5ꎬ以提高在实际场景中检测被遮挡物体的能力[7]ꎮTransformer模块结构如图4所示ꎬ由输入嵌入㊁位置编码㊁Transformer编码器组成ꎮ每个Transformer编码器[8]包含一个多头注意力模块㊁一个前馈层以及残差连接ꎬ多头注意力模块可以更多地关注像素点并获得上下文信息ꎬ多头注意力处理过程表示如下ꎮMultiHead(QꎬKꎬV)=Concat(head1ꎬ ꎬheadh)WO(7)headi=Attention(QWQiꎬKWKiꎬVWVi)(8)式中:MultiHead代表多头注意力操作ꎻAttention代表注意力操作ꎻConcat代表拼接操作ꎻWQiɪRdmodelˑdkꎻWKiɪRdmodelˑdkꎻWViɪRdmodelˑdv(1ɤiɤh)ꎻWO代表权重矩阵且WOɪRhˑdvˑdmodelꎻdmodel代表模型的维度ꎻdk代表内容信息的维度ꎻdv代表信息的维度ꎻK㊁V㊁Q分别代表内容信息㊁信息本身和输入信息ꎻh代表头部或平行的注意力层数ꎮ图4㊀Transformer模块结构Fig.4㊀ThestructureofTransformermodule㊀㊀位置编码由一个全连接层构成ꎬ以保留位置信息ꎮ输入嵌入通过重塑㊁展开等操作将二维图像处理成一个序列ꎬ输入Transformer编码器ꎮ最终的嵌入因子通过位置编码与输入嵌入相加得到ꎬ再送入Transformer编码器ꎮ本文将C3模块与Transformer结合提出了多注意力C3模块(C3TR)且只有网络中主干末端的C3模块被替换成C3TRꎬ即将C3模块中Bot ̄tleneck模块用Transformer模块取代ꎬC3TR结构如图5所示ꎮ通过在分辨率较低的主干网络末端部署Transformer模块ꎬ网络可以获得更丰富的上下文信息和更好的全局信息ꎬ以提高大型物体的检测精度并影响所有的检测头ꎮ此外ꎬ部署在主干网络末端不会占用过多内存ꎬ并在提高检测效率的同时降低模型复杂度ꎮ图5㊀C3TR结构Fig.5㊀ThestruetureofC3TR1.2.3㊀改进的空间金字塔模块本研究提出了一个改进的空间金字塔模块SimSPPFCSPCꎬ其基于SPPF和SPPCSPC[6]的结构ꎬ将两者相结合并针对自动驾驶场景融合图像检测进一步优化以达到更好的特征学习能力ꎬSimSPPFCSPC的结构如图6所示ꎮ特征在输入SimSPPFCSPC模块后被分到两个分支进行处理ꎬ一个分支利用标准卷积对特征进行常规处理ꎬ另一个分支利用快速空间金字塔(SPPF)结构对特征进行处理ꎬ最后合并两个分支ꎬ此结构相比于按序处理减少了计算量并提高了精度ꎮ此外ꎬ在SP ̄PF分支中使用3ˑ3的最大池化层ꎬ可以在保证学习对象特征的同时节约计算成本ꎬ在一定程度上减轻了过拟合的风险ꎮ图6㊀SimSPPFCSPC结构Fig.6㊀ThestructureofSimSPPFCSPC㊀㊀假设SimSPPFCSPC模块的输入为XɪRCˑWˑHꎬ模块的输出为YɪRCˑWˑHꎬC是特征图的通道数ꎬW和H分别代表特征图的宽和高ꎬ卷积层为Convi(i=1ꎬ ꎬ7)ꎬ拼接操作层为Concatꎬ12第3期㊀㊀㊀李东宇等:基于驾驶场景的高效多模态融合检测方法则SimSPPFCSPC的输出Y可以表示为Y=Conv7(Concat(Conv2(X)ꎬYS))(9)YM=(MXP1(X1)ꎬMXP2(Y1)ꎬMXP3(Y2))(10)YS=Conv6(Conv5(Concat(YMꎬX1)))(11)X1=Conv4(Conv3(Conv1(X)))(12)式中:YS是SPPF分支的输出ꎻX1是SPPF结构的输入ꎻ最大池化层MXPi(i=1ꎬ2ꎬ3)的内核尺寸为3ˑ3ꎻY1是第一个最大池化层MXP1处理的输出ꎻY2是第二个最大池化层MXP2处理的输出ꎻYM是所有最大池化层分支输出ꎮ1.3㊀性能平衡的颈部网络在自动驾驶场景融合图像目标检测算法的实际应用中ꎬ平衡模型的精度与速度是十分必要的ꎮ为进一步提升网络的速度ꎬ本文考虑了在颈部网络部署当前常用的深度可分离卷积(DSC)[9-10]替换标准卷积以降低模型的参数量ꎮ深度可分离卷积的结构如图7所示ꎮ图7㊀DSC结构Fig.7㊀ThestructureofDSC㊀㊀图像的通道在进行卷积的过程中被分离ꎬ每个通道进行单独的运算ꎬ降低了卷积层的参数量ꎮ然而ꎬ各通道之间的信息缺失导致其特征融合能力变弱ꎬ不利于针对融合图像的模型训练和检测ꎮ本文在网络中引入了GS卷积[11]ꎬGS卷积的结构如图8所示ꎬGS卷积同时结合了标准卷积与深度可分离卷积中的逐通道卷积(DW卷积)ꎬ最后采用打乱操作重分配信息ꎬ从而促进了模型对更多正确特征的学习ꎬ使特征信息得到充分利用ꎮ通过使用GS卷积ꎬ可以在速度和精度之间取得良好的平衡ꎬ减少网络的计算量(FLOPs)和参数量ꎬ而不牺牲性能ꎮ图8㊀GS卷积结构Fig.8㊀GSconvolutionalstructure㊀㊀假设GS卷积的输入为XGSɪRCˑWˑHꎬ输出为YGSɪRCˑWˑHꎬC是特征图的通道数ꎬW和H分别代表特征图的宽和高ꎬ普通卷积层为Convꎬ逐通道卷积层为DWCꎬ拼接操作层为Concatꎬ打乱操作表示为SHFꎬ则GS卷积的输出YGS可以表示为YGS=SHF(Concat(Conv(XGS)ꎬDWC(YC)))(13)式中:YC是普通卷积的输出ꎬConv和DWC的卷积核参数分别为CˑCᶄˑ1ˑ1和CˑCᶄˑ5ˑ1ꎬ此处Cᶄ=C/2ꎮ本文将颈部网络部分中FPN结构的1ˑ1卷积替换成了GS卷积ꎬ以努力平衡模型在实际应用中部署时的准确性和速度ꎬ而且GS卷积的部署仅限于颈部网络部分ꎬ因为在卷积神经网络中ꎬ空间信息是逐渐传输到通道上的ꎬ由于特征图的空间压缩和通道扩展ꎬ传输过程中可能会导致语义信息的丢失ꎬ而GS卷积可以保留存在通道之间的隐藏连接从而保留部分语义信息ꎬ但是全阶段部署可能会造成数据流阻碍以及推理时间的增加ꎬ为了缓解此问题本文采用了部分部署的策略ꎮ2㊀实验结果和分析2.1㊀数据集FLIR数据集是近年发布ꎬ用于自动驾驶领域神经网络训练ꎬ包含可见光图像和标注过的红外图像的目标检测数据集(拍摄于白天和黑夜情况下)ꎬ共计14000张ꎮ本研究为实现多模态自动驾驶场景ꎬ充分验证所提出算法的提升效果ꎬ使用对齐的FLIR数据集[12](https://paperswithcode.com/dataset/flir ̄aligned)ꎬ选取4489可见光-红外图像对ꎬ保证了白天和黑夜道路情况的均匀分布ꎮ所有图像对采用基于非下采样剪切波变换(NSST)图像融合算法[13]进行融合ꎬ得到FLIR数据集融合图像作为实验的数据集ꎬ3476张作为训练集ꎬ1013张作为验证集和测试集ꎮ其中只使用三种常用的标注类别:汽车ꎬ自行车和人ꎬ融合图像标注信息与可见光图像和红外图像一致ꎬ数据集中每张图像的大小均为640ˑ512像素ꎮ2.2㊀评价指标本文对提出的AM ̄YOLOv5算法与其他目标检测代表算法进行性能对比实验和消融研究ꎬ采用的评价指标包括:平均精度均值(mAP)㊁计算量(FLOPs)㊁参数量和推理时间ꎮmAP是所包含种类的AP平均值ꎬ数值越大代表模型准确度越高ꎬFLOPs代表模型的复杂程度ꎬ推理时间代表模型在推理单张图片时所用的时间ꎬ数值越小代表22沈㊀阳㊀理㊀工㊀大㊀学㊀学㊀报㊀㊀第43卷推理速度越快ꎮ2.3㊀实验细节在Matlab软件环境下调用NSST算法得到FLIR数据集融合图像ꎮ在自动驾驶场景融合图像目标检测实验中ꎬ网络的输入为640ˑ640ˑ3ꎬ本文将FLIR数据集融合图像输入到训练网络进行端对端的权重模型训练ꎬ训练完成后对相应图像进行检测ꎮ所有训练建立在预训练过的YOLOv5s权重基础之上ꎬ初始学习率设置为0.01ꎬ设置动量为0.937ꎬ训练批次大小为8ꎬ设置权重衰减为0.0005ꎬ训练的迭代次数为100次ꎬ其余参数设置遵循YOLOv5默认的设置ꎮ本研究在Inteli7 ̄11800CPU㊁32GB运行内存㊁NVIDIARTX3070GPU的硬件环境以及Win ̄dows11㊁matlabR2020b㊁Opencv3.4.10图像处理视觉库和Pytorch1.10.1软件环境下完成ꎮ2.4㊀与其他检测器性能对比在FLIR数据集融合图像上进行不同检测算法训练ꎬ结果对比如表1所示ꎮ本文算法mAP0.5达到了69.35%ꎬ比原YOLOv5s提升了约1.66%ꎬ同时超越了一些代表性算法ꎬ如SSD[14]㊁CenterNet[15]和Faster ̄RCNN[16]算法的mAP0.5ꎬ涨幅都在14%以上ꎮ同时高于YOLOXs的mAP0.5ꎬ表明YOLOXs对于融合图像效果欠佳ꎮ表1㊀本文算法与其他目标检测算法在FLIR数据集融合图像上的对比实验结果Table1㊀ComparisonsofexperimentalresultsofthealgorithminthispaperandothertargetdetectionalgorithmsonfusedimagesofFLIRdataset检测网络mAP0.5/%FLOPs/109s-1参数量/MBSSD51.8662.826.29Faster ̄RCNN52.84939.628.48CenterNet55.4769.932.67YOLOXs66.9921.78.05YOLOv5s67.6915.87.02AM ̄YOLOv569.3521.213.41㊀㊀图9展示了YOLOv5s和AM ̄YOLOv5在FLIR数据集融合图像上的可视化检测结果对比ꎬ其中(a)列图像中的黄色框代表YOLOv5s未检测出来的对象ꎬ绿色框代表YOLOv5s错检出的对象ꎮAM ̄YOLOv5可以检测出YOLOv5s在远距离以及光线不强的情况下没有检测出的行人㊁自行车和汽车等目标ꎬ同时算法可以检测到被遮挡的物体ꎬ提高了检测精度ꎬ有效减少错检ꎮ图9㊀YOLOv5s和AM ̄YOLOv5在FLIR数据集融合图像上的检测结果对比Fig.9㊀ComparisonsofdetectionresultsofYOLOv5sandAM ̄YOLOv5onfusedimagesofFLIRdataset2.5㊀消融研究㊀㊀为进一步体现本文算法对于多模态自动驾驶场景图像检测性能的提升ꎬ依然采用FLIR数据集融合图像ꎬ以YOLOv5s为基础ꎬ添加㊁替换不同组件ꎬ对比结果如表2所示ꎮ1)添加改进的Repvgg模块的影响ꎮ网络在FLIR数据集上mAP0.5由67.69%提升到了68.50%ꎮ多分支结构会使整体参数量稍微增大ꎬ是由于在推理过程中融合了分支ꎬ检测时的推理时间没有增加反而有所下降ꎬ为增加精确度而采用Replite模块是值得的ꎮ2)添加多注意力C3模块的影响ꎮ单独添加C3TR模块ꎬmAP0.5比原YOLOv5s提高0.88%ꎬ参数量和FLOPs增加很少ꎬ在B组配置的基础上叠加此模块后精度提升了0.41%ꎮ因配置此模块的位置恰当ꎬ不会占用过多内存ꎬ参数量没有发生明显变化ꎬFLOPs数减少了0.2ˑ109s-1ꎬ推理时间与未添加此模块时极度接近ꎬ检测效率有效提高ꎬ证明添加C3TR模块有效ꎮ3)添加改进的空间金字塔模块的影响ꎮ单独添加SimSPPFCSPC模块ꎬmAP0.5比原始模型提高0.61%ꎬ网络在F组配置上叠加此模块之后模型的mAP0.5提高至了69.27%ꎮ因为结合了两个分支ꎬ参数量和FLOPs数量有了比较明显的上涨ꎮ32第3期㊀㊀㊀李东宇等:基于驾驶场景的高效多模态融合检测方法表2㊀在FLIR数据集融合图像上消融研究实验结果对比Table2㊀ComparisonsofexperimentalresultsofablationstudiesonfusedimagesofFLIRdataset方法mAP0.5/%mAP0.5:0.95/%FLOPs/109s-1参数量/MB推理时间/msAYOLOv5s67.6932.3115.87.029.3BA+Replite68.5033.0216.37.067.9CA+C3TR68.5733.1816.17.068.8DA+SimSPPFCSPC68.3032.4921.513.489.3EA+GSConv67.9733.1516.26.988.3FB+C3TR68.9133.1516.17.068.7GF+SimSPPFCSPC69.2732.7421.313.499.5HG+GSConv69.3532.7721.213.419.1此种结构对于按序处理结构已经降低了计算量且提升了模型精度ꎬ推理时间没有大幅提升ꎬ此模块的增益程度更大ꎮ4)添加GS卷积的影响ꎮ单独添加GS卷积ꎬ网络在没有牺牲精度的情况下参数量有所下降ꎬ得益于GS卷积轻量化的结构以及接近标准卷积效果的特点ꎮ在G组配置基础上叠加此模块后ꎬ模型的FLOPs数量降低了0.1ˑ109s-1ꎬ参数量也降低了0.08MBꎬ在FLIR数据集上推理时间有少许降低ꎮ因为局部使用GS卷积ꎬ特征被充分利用ꎬ语义信息没有过多丢失ꎬ模型准确度有了小幅提升ꎮ上述实验数据表明ꎬ本文网络所引入的模块对于检测性能的提升达到了预期效果ꎮ2.6㊀AM ̄YOLOv5检测结果分析本文将FLIR数据集可见光灰度图像和融合图像的训练集分别放入AM ̄YOLOv5网络内进行训练ꎬ保存训练好的权重参数ꎬ并对测试集图像进行检测ꎬ部分结果如图10所示ꎮ㊀㊀本文列出了具有代表性的四组实验结果ꎬ由第一组结果可以看出ꎬ融合图像因为具有红外图像的优点ꎬ所有行人在图像中被显著高亮且均被检测ꎬ行人被成功识别ꎮ此外ꎬ融合图像可以克服灯光反射造成的过度曝光现象并且削弱闪光影响ꎬ如第二组结果中对向车辆灯光通过地面反射到行人所在区域ꎬ融合图像中行人被成功识别ꎬ可见光图像则相反ꎮ第三组图像是由暗光隧道穿越到日光环境中ꎬ在可见光图像上隧道内的车辆可以被识别ꎬ但是远处道路几乎没有车辆的迹象ꎬ而融合图像中远处强光的目标和环境细节也可见ꎮ最后一组在正常日光条件下ꎬ所有图像均可以表现出良好的检测性能ꎬ但是融合图像上被检测的目标置信度更高ꎮ图10㊀在FLIR数据集可见光图像和融合图像上目标检测对比结果Fig.10㊀ComparisonresultsoftargetdetectionperformedonvisibleimagesandfusedimagesofFLIRdataset㊀㊀通过结合可见光和红外图像的优势ꎬ融合图像对目标检测的优势得到了明确的体现ꎬ同时本文提出的算法表现出了良好的检测性能ꎮ3㊀结论本文提出了一种应用于多模态自动驾驶场景42沈㊀阳㊀理㊀工㊀大㊀学㊀学㊀报㊀㊀第43卷的高性能目标检测算法AM ̄YOLOv5ꎮAM ̄YOLOv5的主干网络采用了Replite模块ꎬ实现了多分支训练结构和单路推理结构的转换ꎬ在提高精度的同时没有影响速度ꎻC3TR模块和SimSP ̄PFCSPC模块的加入提升了网络的计算效率ꎬ进一步提高了精度ꎻ颈部网络引用的全新卷积较好地平衡了网络的精度和速度ꎮAM ̄YOLOv5在FLIR数据集融合图像上的检测性能相比于原始的YOLOv5sꎬmAP0.5提升了1.66%ꎬ整体参数量有些许增加ꎬ但是没有牺牲推理速度ꎬ基本符合预期结果ꎮ参考文献(References):[1]㊀王麒.基于深度学习的自动驾驶感知算法[D].杭州:浙江大学ꎬ2022.[2]㊀祝文斌ꎬ苑晶ꎬ朱书豪ꎬ等.低光照场景下基于序列增强的移动机器人人体检测与姿态识别[J].机器人ꎬ2022ꎬ44(3):299-309.㊀㊀ZHUWBꎬYUANJꎬZHUSHꎬetal.Sequence ̄enhancement ̄basedhumandetectionandposturerecognitionofmobilero ̄botsinlowilluminationscenes[J].Robotꎬ2022ꎬ44(3):299-309.(inChinese)[3]㊀REDMONJꎬDIVVALASꎬGIRSHICKRꎬetal.Youonlylookonce:unifiedꎬreal ̄timeobjectdetection[C]//2016IEEEConferenceonComputerVisionandPatternRecognition(CVPR).LasVegasꎬNVꎬUSA:IEEEꎬ2016:779-788. [4]㊀NORKOBILSAYDIRASULOVICHSꎬABDUSALOMOVAꎬJAMILMKꎬetal.AYOLOv6 ̄basedimpro ̄vedfiredetectionapproachforsmartcityenvironme ̄nts[J].Sensorsꎬ2023ꎬ23(6):3161.[5]㊀DINGXHꎬZHANGXYꎬMANNꎬetal.RepVGG:makingVGG ̄styleConvNetsgreatagain[C]//2021IEEE/CVFConferenceonComputerVisionandPatternRecognition(CVPR).NashvilleꎬTNꎬUSA:IEEEꎬ2021:13733-13742. [6]㊀JIANGKLꎬXIETYꎬYANRꎬetal.Anattentionmechanism ̄improvedYOLOv7objectdetectionalgor ̄ithmforhempduckcountestimation[J].Agricultureꎬ2022ꎬ12(10):1659.[7]㊀于楠晶ꎬ范晓飚ꎬ邓天民ꎬ等.基于多头自注意力的复杂背景船舶检测算法[J].浙江大学学报(工学版)ꎬ2022ꎬ56(12):2392-2402.㊀㊀YUNJꎬFANXBꎬDENGTMꎬetal.Shipdetectionalgo ̄rithmincomplexbackgroundsviamulti ̄headself ̄attention[J].JournalofZhejiangUniversity(EngineeringScience)ꎬ2022ꎬ56(12):2392-2402.(inChinese)[8]㊀VASWANIAꎬSHAZEERNꎬPARMARNꎬetal.Attentionisallyouneed[C]//Proceedingsofthe31stInternationalCon ̄ferenceonNeuralInformationProcessingSystems.LongBeachꎬCaliforniaꎬUSA:ACMꎬ2017:6000-6010. [9]㊀CHOLLETF.Xception:deeplearningwithdepthwisesepara ̄bleconvolutions[C]//2017IEEEConferenceonComputerVisionandPatternRecognition(CVPR).HonoluluꎬHIꎬUSA:IEEEꎬ2017:1800-1807.[10]杨小冈ꎬ高凡ꎬ卢瑞涛ꎬ等.基于改进YOLOv5的轻量化航空目标检测方法[J].信息与控制ꎬ2022ꎬ51(3):361-368.㊀㊀YANGXGꎬGAOFꎬLURTꎬetal.LightweightaerialobjectdetectionmethodbasedonimprovedYOLOv5[J].Informa ̄tionandControlꎬ2022ꎬ51(3):361-368.(inChinese) [11]HUJEꎬWANGZBꎬCHANGMJꎬetal.PSG ̄Yolov5:apar ̄adigmfortrafficsigndetectionandrecognitionalgorithmbasedondeeplearning[J].Symmetryꎬ2022ꎬ14(11):2262. [12]ZHANGHꎬFROMONTEꎬLEFEVRESꎬetal.Mul ̄tispectralfusionforobjectdetectionwithcyclicfuse ̄and ̄refineblocks[C]//2020IEEEInternationalConfer ̄enceonImagePro ̄cessing(ICIP).AbuDhabiꎬUnitedArabEmirates:IEEEꎬ2020:276-280.[13]张全.基于NSST的红外与可见光图像融合算法研究[D].西安:西安电子科技大学ꎬ2020.[14]LIUWꎬANGUELOVDꎬERHANDꎬetal.SSD:singleshotMultiBoxdetector[C]//EuropeanConferenceonComputerVision.Cham:Springerꎬ2016:21-37.[15]DUANKWꎬBAISꎬXIELXꎬetal.CenterNet:keypointtrip ̄letsforobjectdetection[C]//ProceedingsoftheIEEE/CVFInternationalConferenceonComputerVision.SeoulꎬKorea(South):IEEEꎬ2020:6568-6577.[16]RENSQꎬHEKMꎬGIRSHICKRꎬetal.FasterR ̄CNN:to ̄wardsreal ̄timeobjectdetectionwithregionproposalnetworks[J].IEEETransactionsonPatternAnalysisandMachineIn ̄telligenceꎬ2017ꎬ39(6):1137-1149.(责任编辑:和晓军)52第3期㊀㊀㊀李东宇等:基于驾驶场景的高效多模态融合检测方法。
基于VGG-M网络模型的前方车辆跟踪
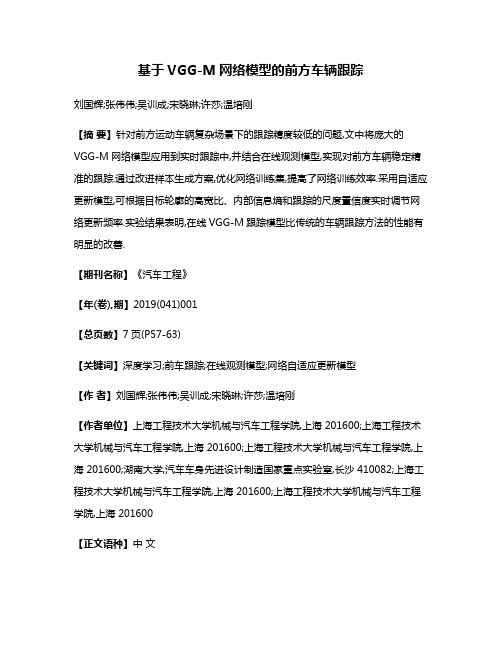
基于VGG-M网络模型的前方车辆跟踪刘国辉;张伟伟;吴训成;宋晓琳;许莎;温培刚【摘要】针对前方运动车辆复杂场景下的跟踪精度较低的问题,文中将庞大的VGG-M网络模型应用到实时跟踪中,并结合在线观测模型,实现对前方车辆稳定精准的跟踪.通过改进样本生成方案,优化网络训练集,提高了网络训练效率.采用自适应更新模型,可根据目标轮廓的高宽比、内部信息熵和跟踪的尺度置信度实时调节网络更新频率.实验结果表明,在线VGG-M跟踪模型比传统的车辆跟踪方法的性能有明显的改善.【期刊名称】《汽车工程》【年(卷),期】2019(041)001【总页数】7页(P57-63)【关键词】深度学习;前车跟踪;在线观测模型;网络自适应更新模型【作者】刘国辉;张伟伟;吴训成;宋晓琳;许莎;温培刚【作者单位】上海工程技术大学机械与汽车工程学院,上海 201600;上海工程技术大学机械与汽车工程学院,上海 201600;上海工程技术大学机械与汽车工程学院,上海 201600;湖南大学,汽车车身先进设计制造国家重点实验室,长沙 410082;上海工程技术大学机械与汽车工程学院,上海 201600;上海工程技术大学机械与汽车工程学院,上海 201600【正文语种】中文前言近年来,基于车载相机的视觉目标跟踪技术已被成功应用在ADAS(advanced driver assistance systems)上,其对于ADAS系统前方车辆的距离判断、碰撞预警等具有重要意义。
传统的视觉跟踪方法主要分为生成式和判别式[1]。
生成式的代表性算法有稀疏编码、在线密度估计和主成分分析等。
LU H等[2]使用了一种新颖的pooling校正方法探索目标的部分信息和空间信息,在局部补丁上进行pooling得到的相似性,不仅定位目标更准确,且能适应一定程度的遮挡,该方法有效地利用稀疏系数在目标与背景之间的差异性,降低了跟踪漂移概率。
与之相对地,判别式方法通过训练分类器来区分目标和背景,将目标跟踪转化为一个二分类问题。
基于改进Deep Sort算法的多目标跟踪算法
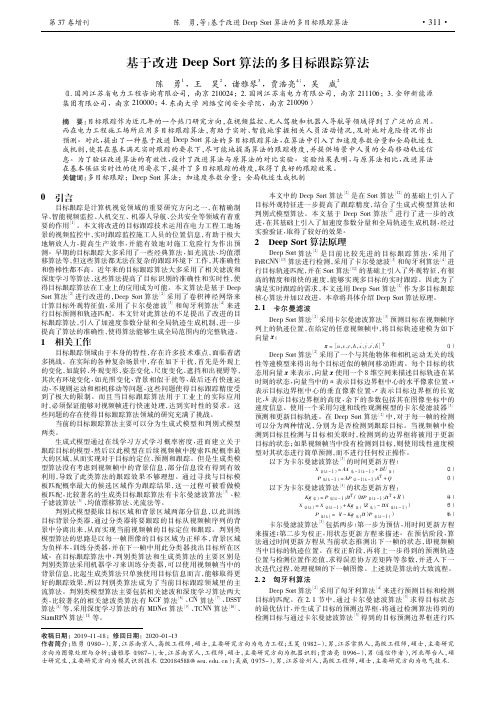
收稿日期:2019-11-18;修回日期:2020-01-13作者简介:陈勇(1980-),男,江苏南京人,高级工程师,硕士,主要研究方向为电力工程;王昊(1982-),男,江苏常熟人,高级工程师,硕士,主要研究方向为图像处理与分析;诸雅琴(1987-),女,江苏南京人,工程师,硕士,主要研究方向为机器识别;贾浩亮(1996-),男(通信作者),河北邢台人,硕士研究生,主要研究方向为模式识别技术(220184588@seu.edu.cn );吴威(1975-),男,江苏徐州人,高级工程师,硕士,主要研究方向为电气技术.基于改进Deep Sort 算法的多目标跟踪算法陈勇1,王昊2,诸雅琴3,贾浩亮4,吴威2(1.国网江苏省电力工程咨询有限公司,南京210024;2.国网江苏省电力有限公司,南京211106;3.金卯新能源集团有限公司,南京210000;4.东南大学网络空间安全学院,南京210096)摘要:目标跟踪作为近几年的一个热门研究方向,在视频监控、无人驾驶和机器人导航等领域得到了广泛的应用。
而在电力工程施工场所应用多目标跟踪算法,有助于实时、智能地掌握相关人员活动情况,及时地对危险情况作出预测。
对此,提出了一种基于改进Deep Sort 算法的多目标跟踪算法,在算法中引入了加速度参数分量和全局轨迹生成机制,使其在基本满足实时跟踪的要求下,尽可能地提高算法的跟踪精度,并提供场景中人员的全局移动轨迹信息。
为了验证改进算法的有效性,设计了改进算法与原算法的对比实验。
实验结果表明,与原算法相比,改进算法在基本保证实时性的使用要求下,提升了多目标跟踪的精度,取得了良好的跟踪效果。
关键词:多目标跟踪;Deep Sort 算法;加速度参数分量;全局轨迹生成机制0引言目标跟踪是计算机视觉领域的重要研究方向之一,在精确制导、智能视频监控、人机交互、机器人导航、公共安全等领域有着重要的作用[1]。
本文将改进的目标跟踪技术运用在电力工程工地场景的视频监控中,实时跟踪监控施工人员的位置信息,有助于极大地解放人力,提高生产效率,并能有效地对施工危险行为作出预测。
多模态目标跟踪综述
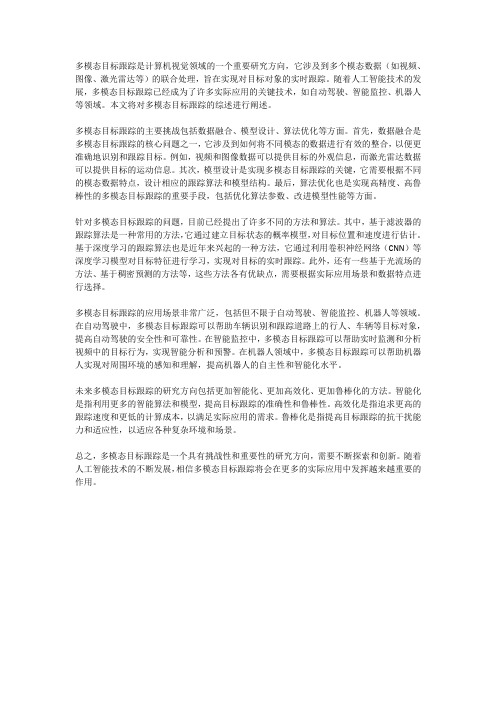
多模态目标跟踪是计算机视觉领域的一个重要研究方向,它涉及到多个模态数据(如视频、图像、激光雷达等)的联合处理,旨在实现对目标对象的实时跟踪。
随着人工智能技术的发展,多模态目标跟踪已经成为了许多实际应用的关键技术,如自动驾驶、智能监控、机器人等领域。
本文将对多模态目标跟踪的综述进行阐述。
多模态目标跟踪的主要挑战包括数据融合、模型设计、算法优化等方面。
首先,数据融合是多模态目标跟踪的核心问题之一,它涉及到如何将不同模态的数据进行有效的整合,以便更准确地识别和跟踪目标。
例如,视频和图像数据可以提供目标的外观信息,而激光雷达数据可以提供目标的运动信息。
其次,模型设计是实现多模态目标跟踪的关键,它需要根据不同的模态数据特点,设计相应的跟踪算法和模型结构。
最后,算法优化也是实现高精度、高鲁棒性的多模态目标跟踪的重要手段,包括优化算法参数、改进模型性能等方面。
针对多模态目标跟踪的问题,目前已经提出了许多不同的方法和算法。
其中,基于滤波器的跟踪算法是一种常用的方法,它通过建立目标状态的概率模型,对目标位置和速度进行估计。
基于深度学习的跟踪算法也是近年来兴起的一种方法,它通过利用卷积神经网络(CNN)等深度学习模型对目标特征进行学习,实现对目标的实时跟踪。
此外,还有一些基于光流场的方法、基于稠密预测的方法等,这些方法各有优缺点,需要根据实际应用场景和数据特点进行选择。
多模态目标跟踪的应用场景非常广泛,包括但不限于自动驾驶、智能监控、机器人等领域。
在自动驾驶中,多模态目标跟踪可以帮助车辆识别和跟踪道路上的行人、车辆等目标对象,提高自动驾驶的安全性和可靠性。
在智能监控中,多模态目标跟踪可以帮助实时监测和分析视频中的目标行为,实现智能分析和预警。
在机器人领域中,多模态目标跟踪可以帮助机器人实现对周围环境的感知和理解,提高机器人的自主性和智能化水平。
未来多模态目标跟踪的研究方向包括更加智能化、更加高效化、更加鲁棒化的方法。
基于自编码器与多模态数据融合的视频推荐方法
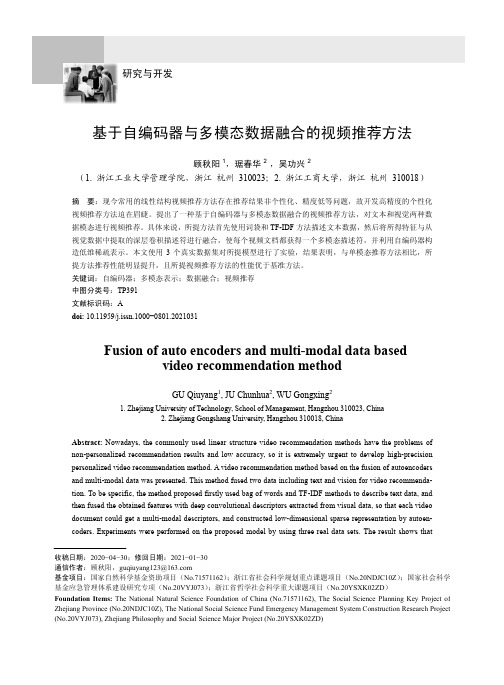
基于自编码器与多模态数据融合的视频推荐方法顾秋阳1,琚春华2 ,吴功兴2(1. 浙江工业大学管理学院,浙江杭州 310023;2. 浙江工商大学,浙江杭州 310018)摘 要:现今常用的线性结构视频推荐方法存在推荐结果非个性化、精度低等问题,故开发高精度的个性化视频推荐方法迫在眉睫。
提出了一种基于自编码器与多模态数据融合的视频推荐方法,对文本和视觉两种数据模态进行视频推荐。
具体来说,所提方法首先使用词袋和TF-IDF方法描述文本数据,然后将所得特征与从视觉数据中提取的深层卷积描述符进行融合,使每个视频文档都获得一个多模态描述符,并利用自编码器构造低维稀疏表示。
本文使用3个真实数据集对所提模型进行了实验,结果表明,与单模态推荐方法相比,所提方法推荐性能明显提升,且所提视频推荐方法的性能优于基准方法。
关键词:自编码器;多模态表示;数据融合;视频推荐中图分类号:TP391文献标识码:Adoi: 10.11959/j.issn.1000−0801.2021031Fusion of auto encoders and multi-modal data basedvideo recommendation methodGU Qiuyang1, JU Chunhua2, WU Gongxing21. Zhejiang University of Technology, School of Management, Hangzhou 310023, China2.Zhejiang Gongshang University, Hangzhou 310018, ChinaAbstract: Nowadays, the commonly used linear structure video recommendation methods have the problems of non-personalized recommendation results and low accuracy, so it is extremely urgent to develop high-precision personalized video recommendation method. A video recommendation method based on the fusion of autoencoders and multi-modal data was presented. This method fused two data including text and vision for video recommenda-tion. To be specific, the method proposed firstly used bag of words and TF-IDF methods to describe text data, and then fused the obtained features with deep convolutional descriptors extracted from visual data, so that each video document could get a multi-modal descriptors, and constructed low-dimensional sparse representation by autoen-coders. Experiments were performed on the proposed model by using three real data sets. The result shows that收稿日期:2020−04−30;修回日期:2021−01−30通信作者:顾秋阳,********************基金项目:国家自然科学基金资助项目(No.71571162);浙江省社会科学规划重点课题项目(No.20NDJC10Z);国家社会科学基金应急管理体系建设研究专项(No.20VYJ073);浙江省哲学社会科学重大课题项目(No.20YSXK02ZD)Foundation Items: The National Natural Science Foundation of China (No.71571162), The Social Science Planning Key Project of Zhejiang Province (No.20NDJC10Z), The National Social Science Fund Emergency Management System Construction Research Project(No.20VYJ073), Zhejiang Philosophy and Social Science Major Project (No.20YSXK02ZD)·83·电信科学 2021年第2期compared with the single-modal recommendation method, the recommendation results of the proposed method are significantly improved, and the performance is better than the reference method.Key words: autoencoder, multi-modal representation, data fusion, video recommendation1 引言随着信息技术的不断发展,用户和企业对推荐方法的需求不断提升[1]。
多模态在英语教学的应用研究
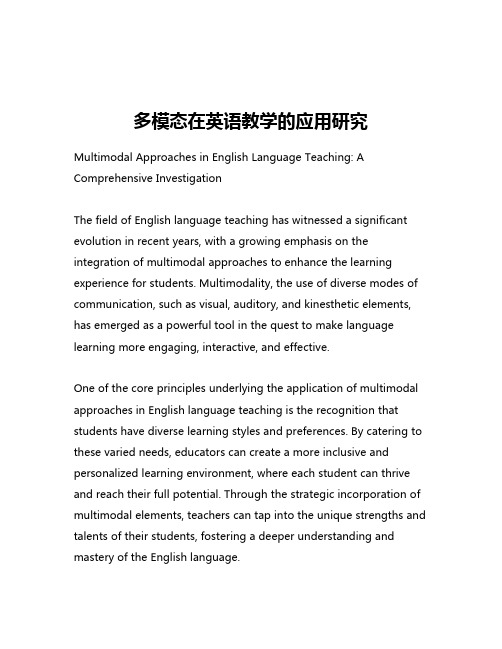
多模态在英语教学的应用研究Multimodal Approaches in English Language Teaching: A Comprehensive InvestigationThe field of English language teaching has witnessed a significant evolution in recent years, with a growing emphasis on the integration of multimodal approaches to enhance the learning experience for students. Multimodality, the use of diverse modes of communication, such as visual, auditory, and kinesthetic elements, has emerged as a powerful tool in the quest to make language learning more engaging, interactive, and effective.One of the core principles underlying the application of multimodal approaches in English language teaching is the recognition that students have diverse learning styles and preferences. By catering to these varied needs, educators can create a more inclusive and personalized learning environment, where each student can thrive and reach their full potential. Through the strategic incorporation of multimodal elements, teachers can tap into the unique strengths and talents of their students, fostering a deeper understanding and mastery of the English language.The visual mode, for instance, plays a crucial role in multimodal English language teaching. The use of images, videos, infographics, and other visual aids can help students better comprehend and retain grammatical concepts, vocabulary, and cultural nuances. Visual elements can also serve as powerful tools for storytelling, providing students with engaging narratives that captivate their attention and stimulate their imagination. Moreover, the integration of visual aids can facilitate the learning of complex topics, such as idiomatic expressions and figurative language, by offering concrete representations and contextual cues.Alongside the visual mode, the auditory component of multimodal approaches has gained significant traction in English language teaching. The incorporation of audio recordings, podcasts, and interactive audio-based activities can enhance students' listening comprehension, pronunciation, and fluency. By exposing learners to a diverse range of accents, intonations, and speech patterns, these auditory elements can help them develop a deeper understanding of the English language and improve their communicative competence.Furthermore, the kinesthetic mode, which involves physical movement and hands-on activities, has proven to be a valuable asset in English language teaching. Through the use of role-playing, simulations, and other interactive exercises, students can actively engage with the language, fostering a deeper connection betweenthe cognitive and physical aspects of learning. This approach not only enhances retention but also promotes the development of crucial skills, such as problem-solving, collaboration, and critical thinking.The integration of multimodal approaches in English language teaching has also been instrumental in addressing the challenges posed by the COVID-19 pandemic. As educational institutions worldwide shifted to remote and hybrid learning models, the need for innovative, technology-driven solutions became increasingly evident. Multimodal approaches have provided a versatile framework for educators to seamlessly transition to online and blended learning environments, leveraging a wide range of digital tools and platforms to deliver engaging and effective language instruction.One of the key benefits of multimodal approaches in the context of remote learning is the ability to cater to the diverse needs and preferences of students. By incorporating a variety of visual, auditory, and interactive elements, teachers can ensure that learners remain engaged and motivated, even in the absence of face-to-face interaction. Moreover, the use of collaborative online platforms and virtual breakout rooms can foster a sense of community and encourage students to actively participate in the learning process, further enhancing their language proficiency.The successful implementation of multimodal approaches in English language teaching requires a comprehensive and strategic approach. Educators must be equipped with the necessary skills and knowledge to effectively integrate these diverse modes of communication into their instructional practices. This may involve ongoing professional development, access to relevant resources and technology, and a willingness to experiment and adapt to the evolving needs of students.Furthermore, the assessment and evaluation of multimodal learning outcomes present unique challenges that educators must address. Traditional assessment methods may not fully capture the multifaceted nature of multimodal learning, necessitating the development of more holistic and authentic evaluation tools. This shift in assessment practices can help teachers gain a deeper understanding of their students' progress and identify areas for further growth and development.In conclusion, the application of multimodal approaches in English language teaching has the potential to revolutionize the way students engage with and master the language. By leveraging a diverse range of communication modes, educators can create dynamic, interactive, and personalized learning environments that cater to the unique needs and learning styles of their students. As the field of English language teaching continues to evolve, thestrategic integration of multimodal approaches will undoubtedly play a crucial role in empowering learners to become confident, competent, and adaptable communicators in the global landscape.。
基于多级全局信息传递模型的视觉显著性检测
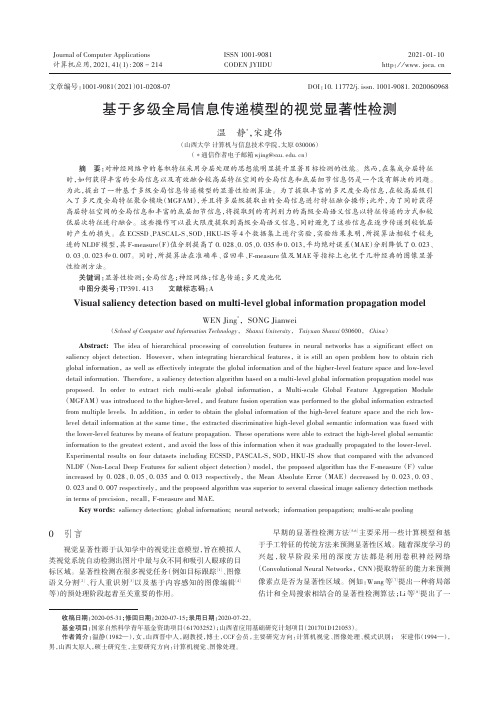
2021⁃01⁃10计算机应用,Journal of Computer Applications 2021,41(1):208-214ISSN 1001⁃9081CODEN JYIIDU http ://基于多级全局信息传递模型的视觉显著性检测温静*,宋建伟(山西大学计算机与信息技术学院,太原030006)(∗通信作者电子邮箱wjing@ )摘要:对神经网络中的卷积特征采用分层处理的思想能明显提升显著目标检测的性能。
然而,在集成分层特征时,如何获得丰富的全局信息以及有效融合较高层特征空间的全局信息和底层细节信息仍是一个没有解决的问题。
为此,提出了一种基于多级全局信息传递模型的显著性检测算法。
为了提取丰富的多尺度全局信息,在较高层级引入了多尺度全局特征聚合模块(MGFAM ),并且将多层级提取出的全局信息进行特征融合操作;此外,为了同时获得高层特征空间的全局信息和丰富的底层细节信息,将提取到的有判别力的高级全局语义信息以特征传递的方式和较低层次特征进行融合。
这些操作可以最大限度提取到高级全局语义信息,同时避免了这些信息在逐步传递到较低层时产生的损失。
在ECSSD 、PASCAL -S 、SOD 、HKU -IS 等4个数据集上进行实验,实验结果表明,所提算法相较于较先进的NLDF 模型,其F -measure (F )值分别提高了0.028、0.05、0.035和0.013,平均绝对误差(MAE )分别降低了0.023、0.03、0.023和0.007。
同时,所提算法在准确率、召回率、F -measure 值及MAE 等指标上也优于几种经典的图像显著性检测方法。
关键词:显著性检测;全局信息;神经网络;信息传递;多尺度池化中图分类号:TP391.413文献标志码:AVisual saliency detection based on multi -level global information propagation modelWEN Jing *,SONG Jianwei(School of Computer and Information Technology ,Shanxi University ,Taiyuan Shanxi 030600,China )Abstract:The idea of hierarchical processing of convolution features in neural networks has a significant effect onsaliency object detection.However ,when integrating hierarchical features ,it is still an open problem how to obtain rich global information ,as well as effectively integrate the global information and of the higher -level feature space and low -leveldetail information.Therefore ,a saliency detection algorithm based on a multi -level global information propagation model was proposed.In order to extract rich multi -scale global information ,a Multi -scale Global Feature Aggregation Module(MGFAM )was introduced to the higher -level ,and feature fusion operation was performed to the global information extracted from multiple levels.In addition ,in order to obtain the global information of the high -level feature space and the rich low -level detail information at the same time ,the extracted discriminative high -level global semantic information was fused with the lower -level features by means of feature propagation.These operations were able to extract the high -level global semantic information to the greatest extent ,and avoid the loss of this information when it was gradually propagated to the lower -level.Experimental results on four datasets including ECSSD ,PASCAL -S ,SOD ,HKU -IS show that compared with the advanced NLDF (Non -Local Deep Features for salient object detection )model ,the proposed algorithm has the F -measure (F )valueincreased by 0.028、0.05、0.035and 0.013respectively ,the Mean Absolute Error (MAE )decreased by 0.023、0.03、0.023and 0.007respectively ,and the proposed algorithm was superior to several classical image saliency detection methods in terms of precision ,recall ,F -measure and MAE.Key words:saliency detection;global information;neural network;information propagation;multi -scale pooling引言视觉显著性源于认知学中的视觉注意模型,旨在模拟人类视觉系统自动检测出图片中最与众不同和吸引人眼球的目标区域。
基于踪片Tracklet关联的视觉目标跟踪

第43卷第11期自动化学报Vol.43,No.11 2017年11月ACTA AUTOMATICA SINICA November,2017基于踪片Tracklet关联的视觉目标跟踪:现状与展望刘雅婷1,2,3王坤峰1,3王飞跃1,4摘要近年来,由于计算机视觉技术的发展和计算机硬件性能的提高,基于视觉的目标跟踪方法得到了飞速的发展.其中,基于踪片(Tracklet)关联的目标跟踪方法因为具有对目标遮挡的强鲁棒性、算法运行的快速性等优点得到了广泛关注,本文对这类方法的最新研究进展进行了综述.首先,简明地介绍了视觉目标跟踪的基本知识、研究意义和研究现状.然后,通过感兴趣目标检测、跟踪特征提取、踪片生成、踪片关联与补全四个步骤,系统详尽地介绍了基于踪片关联的目标跟踪方法,分析了近年来提出的一些踪片关联方法的优缺点.最后,本文指出了该研究问题的发展方向,一方面要提出更先进的目标跟踪模型,另一方面要采用平行视觉方法进行虚实互动的模型学习与评估.关键词视觉目标跟踪,踪片关联,网络流,马尔科夫随机场,平行视觉引用格式刘雅婷,王坤峰,王飞跃.基于踪片Tracklet关联的视觉目标跟踪:现状与展望.自动化学报,2017,43(11): 1869−1885DOI10.16383/j.aas.2017.c170117Tracklet Association-based Visual Object Tracking:The State ofthe Art and BeyondLIU Ya-Ting1,2,3WANG Kun-Feng1,3WANG Fei-Yue1,4Abstract In the past decade,benefitting from the progress in computer vision theories and computing resources,there has been a rapid development in visual object tracking.Among all the methods,the tracklet-based object tracking method has gained its popularity due to its robustness in occlusion scenarios and high computational efficiency.This paper present a comprehensive survey of research methods related to tracklet-based object tracking.First,the basic concepts,research significance and research status of visual object tracking are introduced briefly.Then,the tracklet-based tracking approach is described from four aspects,including object detection,feature extraction,tracklet generation,and tracklet association and completion.Afterwards,we propose a detailed review and analyze the characteristics of state-of-the-art tracklet-based tracking methods.Finally,potential challenges and researchfields are discussed.In our opinion,more advanced object tracking models should be proposed and the parallel vision approach should be adopted to learn and evaluate tracking models in a virtual-real interactive way.Key words Visual object tracking,tracklet association,networkflow,Markov randomfield,parallel visionCitation Liu Ya-Ting,Wang Kun-Feng,Wang Fei-Yue.Tracklet association-based visual object tracking:the state of the art and beyond.Acta Automatica Sinica,2017,43(11):1869−1885视觉目标跟踪是指利用目标的颜色、纹理等视觉信息以及运动信息,确定视频数据中感兴趣目标收稿日期2017-03-04录用日期2017-08-18Manuscript received March4,2017;accepted August18,2017国家自然科学基金(61533019,71232006,91520301)资助Supported by National Natural Science Foundation of China (61533019,71232006,91520301)本文责任编委张军平Recommended by Associate Editor ZHANG Jun-Ping1.中国科学院自动化研究所复杂系统管理与控制国家重点实验室北京1001902.中国科学院大学北京1000493.青岛智能产业技术研究院青岛2660004.国防科技大学军事计算实验与平行系统技术研究中心长沙4100731.The State Key Laboratory of Management and Control for Complex Systems,Institute of Automation,Chinese Academy of Sciences,Beijing1001902.University of Chinese Academy of Sciences,Beijing1000493.Qingdao Academy of Intelligent Industries,Qingdao2660004.Research Center for Computa-tional Experiments and Parallel Systems Technology,National University of Defense Technology,Changsha410073的位置、速度等信息,并将相邻图像帧的相同目标进行关联,实现对目标的位置预测和持续追踪,以便完成更高级的任务.视觉目标跟踪不仅可以获得目标的运动状态和运动轨迹,也为运动分析、场景理解、行为或事件监测提供先验知识.它融合了模式识别、人工智能、图像处理等多个学科,在智能监控、人机交互、视觉导航、军事指导以及医疗诊断等领域有着广泛的应用[1−3].由于视觉跟踪技术具有广阔的市场前景和理论价值,国内外很多大学和科研机构都开展了相关理论研究.国外研究启动相对较早,牛津大学动态视觉研究组针对视觉目标跟踪展开了大量研究,包括灵活目标跟踪、对抗伪装,并应用到交通监控、安保等领域;加利福尼亚大学视觉研究实验室(VRL)展开了摄像机网络中的行人跟踪研究[4];1870自动化学报43卷诺丁汉大学计算机视觉实验室(CVL)展开使用视频中语义信息进行人或人群跟踪研究;瑞士联邦理工学院(ETH,Zurich)计算机视觉实验室开展了动态场景自动驾驶中的目标跟踪研究[5],将视觉跟踪技术与机器人技术相结合;南加州大学计算机视觉实验室研究无约束环境中的视觉跟踪问题,并提出基于语境的跟踪方法[6];NEC实验室研究视觉监控场景中的多人跟踪问题,以期满足实时性要求[7];卡耐基梅隆大学机器人研究所计算机视觉小组则针对机器人可能遇到的环境约束中的视觉跟踪问题进行了大量研究[8].美国国防部高级研究计划局(Defense Advanced Research Projects Agency, DARPA)开展了重大视频监控项目VSAM(Visual Surveillance and Monitoring)并产生了先进的成果[9−10],在国内,视觉跟踪的研究也逐渐取得一系列成果,许多高校和科研单位在视觉跟踪理论方面进行了深入研究.早在2001年,清华大学运用相关目标识别和跟踪技术开发了一套适用于野外环境的视觉侦查系统;中科院自动化所在行人视觉分析,交通场景与行为事件理解、视觉监控等领域也取得了科研成果.近年来,以深度学习为代表的机器学习热潮再次掀起,激发越来越多的企业与科研机构投入到视觉目标跟踪领域.随着目标检测算法的成熟、检测准确度的提高,越来越多的研究者[1,11−13]采用Tracking-by-detection思路进行研究,通过提取感兴趣目标的SIFT、HOG、LBP等特征[14−17],找出单帧图像的目标区域,再运用生成模型或者训练分类器得到跟踪轨迹.进一步地利用该轨迹来解决目标之间、目标与背景遮挡的问题,并且融入先验知识提升跟踪精度.本文综述了一类Tracking-by-detection方法—基于踪片(Tracklet)关联的目标跟踪方法.需要指出,目前对英文专业术语Tracklet的中文翻译不统一,常见的译名有轨迹片段、短轨迹、踪迹片段等,都不够简洁达意;本文将Tracklet翻译为“踪片”,言简意赅.基于踪片关联的目标跟踪方法依据目标检测的结果,找到目标能被稳定检测的视频帧,将其置信度较高的位置进行关联形成踪片,再将不同的踪片进一步关联,形成最终的完整轨迹.当目标发生遮挡、重叠时,通过填补成功关联踪片之间的空缺能够得到完整的轨迹集合;当目标再次进入视野时,提取其特征与之前的轨迹进行匹配,实现目标的稳定关联,提高跟踪的鲁棒性.除上述优点外,基于踪片关联的目标跟踪还可用在不同的跟踪情形中.目标跟踪情形可按如下方式分类:按照跟踪目标的个数可划分为单目标跟踪和多目标跟踪;按照跟踪目标的类型分为刚体跟踪与非刚体跟踪;按照摄像头数量分为单摄像头与多摄像头跟踪;按照摄像头的运动状态分为静止摄像头与运动摄像头跟踪;按照应用场景分为单场景和多场景目标跟踪[18].在以上不同的情况中,基于踪片关联的跟踪都能根据相应的目标检测结果进行关联形成对应的踪片,并填补空缺形成完整轨迹,因此有较强的普适性.本文其他部分内容安排如下:第1节介绍基于踪片关联的视觉目标跟踪算法流程;第2节总结目标跟踪常用的公开数据集;第3节详细阐述基于踪片关联的视觉目标跟踪研究进展,包括算法介绍、算法的优缺点分析、在公共数据集上的测试结果比较;第4节分析了现有的目标跟踪方法的优点和局限性,并对该研究领域的发展趋势做出展望;第5节总结全文.1基于踪片关联的视觉目标跟踪算法流程基于踪片关联的目标跟踪算法主要包括感兴趣目标检测、跟踪特征提取、踪片生成、踪片关联四个步骤,基本流程如图1所示.输入视频序列,首先通过目标检测获得感兴趣目标的位置等特征,并将相关检测结果进行分析,提取出恰当的跟踪特征后关联形成踪片,通过图论等数学方法将踪片进一步关联形成长轨迹,通过轨迹补全、轨迹校正等后处理方法填补轨迹空缺,进行轨迹平滑,校正轨迹关联错误,从而得到最终的输出轨迹.1.1感兴趣目标检测基于踪片关联的目标跟踪方法以目标检测的结果为前提,继而进行关联直到形成最终的跟踪轨迹,因此目标检测是该方法的基础.目标检测是指依据一定的算法和先验信息把图像中的前景目标从背景中提取出来.在关联过程中,只对需要关注的目标而不是所有目标进行实时检测的特定目标检测方法被称为感兴趣目标检测.然而物体运动复杂多变,感兴趣目标在视频序列中可能会出现暂时离开场景或者被遮挡的情况;目标与背景外观等特征较为相似,前景和背景区分不准确;受天气、光照等外界条件以及背景自身内部因素影响,图像中的背景具有复杂性和动态变化性,这些都增加了提取感兴趣目标的难度.近年来,机器学习特别是深度学习技术在目标检测领域获得了广泛应用,越来越多的研究者使用人工神经网络[10,19](CNN、RCNN、Fast R-CNN、GAN等)、支持向量机[20]、Adaboost[21]等方法训练分类器实现前景与背景的分离.这些方法通常首先选定样本(包括正、负样本),将所有样本分11期刘雅婷等:基于踪片Tracklet 关联的视觉目标跟踪:现状与展望1871图1基于踪片关联的视觉目标跟踪方法流程图Fig.1Flowchart of visual object tracking based on tracklet association成训练集和测试集两部分.在训练集上运用机器学习算法训练分类器,生成分类模型;在测试集上运用该模型生成预测结果;最后用相应的评估指标评估分类器性能.机器学习方法能够克服背景扰动,处理目标运动复杂的场景,抗干扰性强.通过以上方法实现了感兴趣目标的检测和定位,为踪片关联打下基础.1.2跟踪特征提取对视频图像进行逐帧检测并得到感兴趣目标的检测结果后,该方法需要从结果中提取恰当的特征从而形成可靠稳定的踪片,以便实现踪片的准确关联,提高目标跟踪精度.目标跟踪中常用到的目标特性表达主要包括视觉特征、统计特征、代数特征等.视觉特征包括图像边缘、轮廓、区域、纹理;统计特征包括直方图、矩;代数特征如图像矩阵的奇异值分解.在视觉特征中,沿着边缘方向移动,像素变化较缓慢,而垂直于边缘方向移动,像素变化很剧烈为边缘特征,可以采用梯度、Sobel 、Roberts [22]等梯度算子以及卷积神经网络[23]提取.将检测到的像素不连续的部分连接成完整边界就形成了轮廓.轮廓跟踪[24]利用封闭的曲线轮廓表示运动目标,并且能够实时更新轮廓位置,去除了背景像素,对非刚体与其他轨迹复杂的运动有良好的跟踪效果.区域特征不仅包括运动目标,也包括部分背景区域,通常用矩形或者椭圆形框表示.区域特征跟踪[25]对无遮挡目标的跟踪精度高,但其计算复杂度高,对目标有遮挡时跟踪效果差.区域特征提取的方法包括区域生长法[26]、区域分裂与聚合[27]、阈值法[28].图像纹理[29]通过图像的颜色、光强信息描述,提取方法有结构建模法和统计数据法,研究者需根据场景不同来选择适合的纹理识别方式.在统计特征中,用直方图[30]描述图像的灰度、HOG 、HOF 等特征信息,帮助分析图片中曝光水平,粗略描绘出目标区域颜色分布,计算效率高.针对一幅图像,若用二维随机变量表示像素位置,则可以用二维灰度密度函数表示灰度图像,或用矩描述灰度图像的特征[31−34].代数特征[35]是将图像看作矩阵,运用代数方法得到空间表征能力强的特征向量作为图像特征.相关代数方法包括奇异值分解[36]、主成分分析[37]、独立成分分析[38]等.除了上述特征提取方法外,近年来SIFT 算子[39]、卷积神经网络[40−42]等特征提取方法得到了广泛的应用,获得了较好的特征提取效果.另外,还可以通过融合多种特征代替单一特征的方式来提高特征提取的鲁棒性和精确性.1.3踪片生成提取到感兴趣目标的特征后,进一步将检测结果进行关联生成踪片.该过程通常需要牺牲连接片段的长度来生成置信度较高的踪片确保已关联的片段准确,称为初级关联.目标在连续两帧图像中变化缓慢,相邻帧之间目标的尺寸、运动状态、外表形态等特征变化不大,逐帧进行关联具有高的可靠性.具体地,对相邻帧的检测结果可以提取位置、速度、外观属性计算相似性,并设置阈值来进行匹配,相似度高于阈值则认为属于同一目标.形成初级关联的踪片初步关联的相似性可以表示为:P associate (f 1,f 2)=A position (f 1,f 2)×A appearance (f 1,f 2)A velocity (f 1,f 2)(1)其中,f 1、f 2表示待比较的两帧,P associate (·)表示相邻帧中待关联目标的相似性,A appearance (·)表示外观相似性,A velocity (·)表示速度相似性.其中外观1872自动化学报43卷相似性可以通过面积和颜色的相似性衡量,由于相邻两帧之间目标移动缓慢,研究者通常将该过程看做线性运动,在保证关联准确的情况下尽可能简化计算.位置和面积相似性可以采用高斯核函数的方式来计算;颜色的相似性计算方式如下:首先计算每帧目标的颜色直方图,接着计算相邻帧之间的距离(Hellinger距离[43]、巴氏距离[44]等)作为相似性的衡量标准.通过以上所述的方法构建不同帧中待关联检测的相似性,将踪片生成的过程进行量化表示.决定踪片生成数量和长度的因素是阈值的选取,以实现漏检率与错误率的折中.阈值选取较大值时,生成的踪片数量较少,关联精确度高,但也增加了漏检的可能性;阈值取值较小时,生成的踪片数量较多,但也可能将不属于同一目标的片段错误关联起来.由于在初级关联时要保证生成的踪片足够精确,所以此时往往选较大阈值.将所有检测结果按照以上准则计算相似度并进行关联,最终得到待跟踪视频初级关联结果.1.4踪片关联形成踪片后,对其进行高层关联形成长轨迹.该过程也可视为踪片间的匹配问题,即如何进行踪片匹配使得关联后的轨迹具有更高的可靠性、稳定性以及鲁棒性.获得踪片之间相似性需要综合考虑时间关系、外观以及运动等特征,从而保证踪片关联的精度和完整度.该步骤是基于踪片跟踪的关键,有效的踪片关联算法能够大幅提高跟踪精度.这里总结了踪片关联的一般方法.轨迹关联在时间上满足以下两种约束:1)同一目标在同一时间不可能出现在多于一条运动轨迹;2)同一运动轨迹不可能同时属于多个目标.由上面的结论可知,时间上重叠的踪片一定不属于同一目标,可公式化表示为:P t(T i,T j)=1,if f js−f ie>00,otherwise(2)其中P t表示T i与T j关联的可能性,T i与T j表示两条待匹配片段,且T i出现时间早于T j.f js为T i 的初始帧号,f ie为T j的结尾帧号.与踪片形成阶段相似,踪片关联也要综合考虑片段的颜色、纹理、面积等因素,从而判断待关联的踪片是否属于同一目标.外观相似性模型按照式(3)建立,其中P app(·)表示相似度,A Ti 和A Tj表示轨迹T i和T j的外观(颜色、面积、纹理等)约束.P app(T i,T j)=corr(A Ti ,A Tj)(3)对运动特征,基于目标运动轨迹连续的原则,时间差与目标移动距离之间有着相关关系.通过对前一个踪片的尾部帧和当前踪片的起始帧进行运动相似性匹配确定关联情况.运动模型可以按照如下方程建立:P mo(T i,T j)=corr(P eT i+V eT i∆t,P sT j)corr(P sT j−V sT j∆t,P eT i)(4)其中,P eT i表示踪片T i的结束位置,P sT i表示踪片T j起始位置,V eT i表示T i结束时刻的速度,V sT j表示T j 起始时刻速度,∆t为T i结束时刻与T j起始时刻的时间差.由于候选关联踪片之间时间间隔较短,目标速度在该短时间内可看作是恒定的.因此该过程可以按照如下步骤进行:提取前一条踪片结束时刻的位置与速度,通过线性预测方法预测其经过∆t时间间隔后的位置,并与后一条轨迹的起始位置进行比较,计算相关性;另外,将后一轨迹中的起始时刻按照同样的方法进行倒推,得到∆t时刻之前的状态,并与前一条轨迹的结束位置进行比较,得到位置的相关性.如图2所示.图2位置相关性示意图Fig.2Sketch map of position relations 最后将时间、外观和运动相似性模型结合起来计算两个踪片的关联概率表示为:P ass(T i,T j)=P t(T i,T j)P app(T i,T j)P mo(T i,T j)(5)判断关联过程中轨迹是否生成或终止可以采用如下判定依据:1)当前帧与前一帧进行匹配计算相似度小于阈值,认为当前帧出现了新目标,生成新轨迹;2)当前帧与后一帧进行匹配计算相似度小于阈值,认为当前帧的目标轨迹已终止.根据以上方法可以得到踪片之间的关联概率.对比不同踪片的关联概率,并将概率值最大的踪片关联起来,可以获得目标相对较长的运动轨迹.最后,由于交叉重叠等因素影响,获得的长轨迹还需要通过插值法[45]等进一步连接,从而形成平滑完整的轨迹,最终实现目标轨迹跟踪.2目标跟踪的公共数据集为了方便研究者进行目标跟踪实验以及评估实11期刘雅婷等:基于踪片Tracklet关联的视觉目标跟踪:现状与展望1873验结果,促进目标跟踪领域的发展,学术界建立了部分开放的公共数据集.这些数据集由不同的场景、光照、天气、视角、采集而来,包含行人、车辆等各种要素以及不同要素相互遮挡、轨迹重叠、离开以及重回视野等复杂的运动模式.将算法在这些数据集上运行,对跟踪结果与已有的基准进行比较,能够全面地反映算法的性能,客观地评价算法的优缺点.本文将数据集划分为实际数据集和虚拟数据集两种类型分别介绍.常见的数据集名称及其特点如下表1所示.实际数据集由实际场景中采集到并通过人工方式被标记,传统数据集一般都属于实际数据集,如表1所示.但是这种获取数据的方法不仅成本昂贵,而且在复杂天气条件或是低照度情况下人工标注准确率也难以得到保证.此外,受到实际条件约束,实际数据集无法模拟如极端恶劣天气、目标复杂运动等不常见的情景,获取的数据集规模也受制约,这些因素都促使了研究者开展人工场景研究.近年来,游戏引擎、虚拟现实技术的发展也进一步推动了虚拟数据集的建立,表1中所示的Virtual KITTI和SYNTHIA已成为常见的虚拟数据集.这些数据集利用计算机图形学等综合性生成复杂多样的、动态的、可自动标注的虚拟场景,从而实现逼真地模拟各种复杂挑战的实际场景.相关实验[46]已经表明:经过真实环境训练的跟踪方法在虚拟数据集和真实数据集上有相同的表现程度,并且在虚拟数据上进行预训练能够提高目标跟踪性能.3基于踪片关联的视觉目标跟踪进展近年来,基于踪片关联的跟踪引起了研究者的广泛关注,取得了一定的研究进展.解决踪片跟踪问题的关键是对生成的踪片进行准确关联,从而形成可靠完整的轨迹.本文将基于踪片关联的跟踪方法分为图论方法和其他方法,并具体介绍部分代表性成果.3.1图论方法得到目标检测结果并进行初级关联形成踪片后,可以利用图论知识建立匹配模型.概率图可以具体的图论方法有:贝叶斯网络(Bayesian network)、条件随机场(Condition randomfield,CRF)、马尔科夫随机场(Markov randomfield,MRF)等概率图模型以及网络流(Networkflow,NF)、二分图匹配(Bipartite graph match)等模型.3.1.1概率图模型1)贝叶斯网络Huang等[55]首次提出了基于检测的三层次关联方法,以解决单摄像机、嘈杂环境下的多目标跟踪问题.在低层次的关联中,通过极大化连接相似性的约束产生可靠的轨迹,该阶段只连接相邻帧的检测结果,并且用双阈值的方式抑制错误的连接;在中层次的关联中,从低层次获得的踪片被迭代地输入,通表1多目标跟踪常见的公共数据集Table1Frequently used public datasets for multi-target tracking research数据集建立时间描述规模类型PETS[47]2009年拥挤的公共区域多传感器跟踪和事件识别3个不同环境视频序列8个视角实际数据集MOT challenge[48]2015年不仅标记了行人,车辆、静态的人、遮挡物体等都被标注22个视频序列,共11286帧图像实际数据集CAVIAR[49]2003年行人会面、购物,穿越拥挤人群及在公共场所遗失行李等复杂场景28段视频实际数据集i-LIDS[50]2006年多摄像机配置,可以选择多视角的数据进行实验10小时视频实际数据集UA-DETRAC[51]2015年多个数据采集地;涉及汽车、公共汽车、货车等多种车辆;包含多云、夜晚、晴天和下雨等天气条件10小时视频实际数据集文献[52]中的数据集2014年从拥挤繁忙的火车站采集42million的轨迹实际数据集KITTI[53]2012年每幅图像多达15辆车和30个行人;包含三维立体,光流,视觉光度法,3D物体检测和3D跟踪50个视频序列实际数据集Virtual KITTI[46]2016年数据从不同的成像和天气条件下的五个虚拟世界生成.有准确,完整的2D和3D多对象跟踪注释,并有像素级别和实例级别标签,以及深度标签50个高分辨率单目视频,共21260帧虚拟数据集SYNTHIA[54]2016年多样化的场景;多种动态物体种类;多季节;不同的照明条件和天气情况;多传感器多视角2分23秒雪景及1分48秒傍晚车载视频序列虚拟数据集1874自动化学报43卷过复杂的相似性测量方法将上述的踪片进行关联形成长轨迹,关联过程被看作最大后验概率[56−57]问题,其不仅考虑轨迹片的初始化,终止和ID转换,还考虑踪片的误报警等.在高层次的关联中,文章基于前一级得到的踪片估计出一个新的场景结构模型,它能有效地建模目标进入、退出和场景遮挡问题.借助于基于场景知识的推理执行长轨迹关联,以减少轨迹分割并防止ID转换.该方法通过有效地将踪片与不准确的检测响应和长时间遮挡相结合,显著地改善了跟踪性能.文章提出的这种分层框架是一种通用的方法,其他相似性度量或优化方法可以很容易地集成到这个框架中.2)条件随机场模型在现有的主流目标跟踪工作中,外观模型是预先定义的或通过在线学习的方式得到.虽然多数情况下这种方法能够有效区分目标,但当目标具有相似外观并且在空间上接近时该方法将会失效.运动模型,线性运动模型目前也被广泛使用.轨迹之间的关联概率通常基于满足线性运动假设的程度,即假定目标以恒速度沿着原方向运动.然而,如果目标不遵循线性运动模型或是相机运动造成视角变化,利用线性假设估计踪片之间的关联性会出现很大偏差.在线学习条件随机场(CRF)模型能够在相机运动下可靠地跟踪目标,并提高不同目标的区分度,特别是在空间上接近并且具有相似外观的难区分困难的目标.因此条件随机场模型也广泛用在踪片关联中.Yang等[58]提出了一种条件随机场模型在线学习方法.该方法主要分为CRF创建、一元项学习、二元项学习、最小化能量函数得到踪片关联四步:首先寻找首尾间隔满足一定阈值条件的踪片对,作为CRF节点.然后基于运动模型以及外观辨别模型定义了一元项和二元项能量函数,分别用于区分踪片之间的关联程度以及邻近的踪片对之间的关联程度.其中运动模型的一元项由踪片线性运动模型所得的估计位置之间的差别定义,二元项则由踪片对的尾部位置相近(Tail-close)或者头部位置相近(Head-close)的关系得到,如图3所示;外观模型的一元项与二元项则通过选取颜色、纹理、形状等特征,采用在线学习外观区分模型(OLDAMs)以得到正负学习样本,最后使用RealBoost[59]算法学习得到最终外观模型.通过最小化总的能量函数即可得到踪片关联.该方法利用CRF一阶和二阶能量项提高了算法的鲁棒性,时间复杂度为指数级别.该算法保证了良好的快速性,并在多个公共数据集实验结果中的多个性能指标中表现良好.3)马尔科夫随机场Wu等[60]将人脸聚类和跟踪结合起来,用以同时提升人脸识别与轨迹跟踪问题.该方法通过两个问题相互提供有用的信息以及约束条件,提高彼此的性能.文章通过隐马尔科夫随机场模型将人脸聚类标签和人脸轨迹跟踪结合起来,转化为贝叶斯推理问题,提出了有效的坐标下降解法.输入一个视频序列,利用Viola-Jones脸部检测方法[61]来产生可靠的检测结果,通过外观、边框位置、尺度等将检测结果关联起来形成踪片.为避免身份转换,作者对匹配分数设置了阈值.文章基于隐马尔科夫随机场模型表示聚类标签和轨迹连接关系的联合依赖,提出了同时聚类和关联长视频序列中不同人类的面孔.该方法不仅减少了由于关联不同聚类标签踪片而导致的错误,而且在同一目标的长追踪轨迹中进行聚类能够极大增强聚类准确性.图3踪片对运动相似性估计[58]Fig.3Estimation of motion similarity between a pair oftracklets[58]Leung等[62]尝试使用马尔科夫逻辑网络解决目标长时间遮挡问题.首先利用常见的跟踪方法得到跟踪轨迹,再检查其中错误关联的部分并断开形成踪片,最后通过马尔科夫逻辑网络将这些踪片重新关联形成正确的轨迹.对踪片之间关系建立如下的三个查询谓词来描述:相同目标(sameObject)、连接(join)以及聚类(isGroup).利用踪片的外观相似性和时空一致性构造马尔科夫逻辑网络,其中踪片的外观采用该轨迹特征颜色直方图的均值和标准差建模,相似性依据它们均方差归一化直方图交集的大小衡量,时空一致性则应用踪片之间的时间差和空间位置差计算.通过最优化该网络获得每个踪片或者踪片对之间的三个查询谓词的赋值情况,进而可以形成稳定的跟踪轨迹.例如,对于如图4形式的轨迹,isGroup(tracklet3)的赋值结果为1,sameObject(tracklet1,tracklet4)的赋值结果为1,join(tracklet1,tracklet3)的赋值结果为1,而sameObject(tracklet1,tracklet5)为0,isGroup (tracklet1)为0,join(tracklet1,tracklet5)为0.该算法适用于较拥挤的场景和有长期遮挡的情况,对于无遮挡或短期遮挡情况,该算法的复杂度较高并。
- 1、下载文档前请自行甄别文档内容的完整性,平台不提供额外的编辑、内容补充、找答案等附加服务。
- 2、"仅部分预览"的文档,不可在线预览部分如存在完整性等问题,可反馈申请退款(可完整预览的文档不适用该条件!)。
- 3、如文档侵犯您的权益,请联系客服反馈,我们会尽快为您处理(人工客服工作时间:9:00-18:30)。
Fusion of Multimodal Visual Cues for Model-Based Object TrackingGeoffrey Taylor and Lindsay KleemanIntelligent Robotics Research CentreDepartment of Electrical and Computer Systems EngineeringMonash University,Victoria3800{Geoffrey.Taylor;Lindsay.Kleeman}@.auAbstractWhile many robotic applications rely on visualtracking,conventional single-cue algorithms typi-cally fail outside limited tracking conditions.Fu-sion of multimodal visual cues with complemen-tary failure modes allows tracking to continue de-spite losing individual cues.While previous ap-plications have addressed multi-cue2D feature-based tracking,this paper develops a fusion schemefor3D model-based tracking using a Kalmanfilterframework.Our algorithm fuses colour,edge andtexture cues predicted from a textured CAD modelof the tracked object to recover the3D pose.Thefusion framework allows additional cues to be in-tegrated provided a suitable measurement functionexists.We also show how measurements from mul-tiple cameras can be integrated without requiringexplicit correspondences between views.Experi-mental results demonstrate the increased robustnessachieved by fusing multiple cues.1IntroductionVisual tracking plays an important role in an increasing va-riety of robotic applications,such as mobile robot navigation [Davison,1988],machine learning[Bentivegna et al.,2002] and visual servoing[Hutchinson et al.,1996].Our research aims to enable a humanoid robot(see Figure1)to manipulate a priori unknown objects in an unstructured office/domestic environment.While many simple manipulation tasks may be executed using a sense-then-move framework,object tracking improves performance by addressing issues such as handling dynamic scenes,detecting unstable grasps,and compensating for camera motion and internal calibration errors.Object tracking algorithms are typically based on the de-tection of a particular cue,most commonly colour[Ben-tivegna et al.,2002],edges[Marchand et al.,1999;Kragic and Christensen,2002;Tonko et al.,1997]or feature tem-plates[Nickels and Hutchinson,2001;Nelson and Khosla, 1995].However,robots cannot rely on the regularpresenceFigure1:An upper-torso humanoid robot platform.of distinctive colours,high contrast backgrounds or easily de-tected textures when tracking arbitrary objects in an unstruc-tured domestic environment.As a result,individual cues only provide robust tracking under limited conditions.This paper will demonstrate how the integration of multimodal cues in a model-based tracking framework provides long-term robust-ness,despite short-term failures of individual cues.Multi-cue integration exploits the observation that cues typically exhibit independent and complementary failure modes.Multi-cue integration has been exploited extensively in feature-based tracking applications,such as people tracking [Spengler and Schiele,2003;Darrell et al.,2000]and image-based visual servoing[Kragic and Christensen,2001].In these schemes,image plane features are tracked byfinding a consensus between cues such as colour,edges,shape,tex-ture and motion using voting,fuzzy logic or Bayesian frame-works.Multi-cue tracking has also been performed by dy-namically selecting one of a range of cues based on per-formance metrics and an order of preference[Toyama and Hager,1999;Darrell et al.,2000].The integration techniques described above only address the problem of tracking features on the image plane.In this paper,we demonstrate how multi-cue integration can be applied to3D model-based tracking,which is requiredfor robotic applications such as position-based visual servo-ing.In our model-based tracking algorithm,an explicit CAD model specifies the geometry and surface texture of an ob-ject.Models are produced autonomously by the robot using techniques developed in[Taylor and Kleeman,2003].During tracking,the algorithm uses the model to predict multimodal visual cues and associates these with actual image plane mea-surements.The3D pose of the object is then updated to mini-mize the difference between predicted and measured features. Measurement prediction and state updates are imple-mented in the familiar Kalmanfilter framework.The Kalman filter has been previously applied to multimodal sensory fu-sion in applications such as mobile robot navigation[Klee-man,1992],but in visual tracking algorithms is typically only used to fuse state estimates and predict object trajectories.In addition to integrating multiple cues,this paper will demon-strate how the Kalmanfilter provides a framework for fusing measurements from multiple cameras without requiring ex-plicit feature correspondences between views.The following section gives an overview of the proposed framework.Section3describes the Kalmanfilter used to in-tegrate multimodal cues from multiple views,and Section4 describes each cue in detail.Finally,Section5provides im-plementation details and experimental results to demonstrate the increased robustness gained through fusion.2System OverviewModel-based tracking aims to estimate the trajectory of an object in a sequence of images from n cameras,given a3D model and an initial estimate of the pose.The pose of the object in the k th frame is represented by a6dimensional vec-tor p(k)=(X,Y,Z,φ,θ,ψ) ,where X,Y,and Z describe position,and Euler anglesφ,θ,andψgive orientation. The object is represented by a3D polygonal approximation consisting of triangular facets and associated textures.To successfully achieve long-term robust tracking using multi-cue fusion,the selected cues should be redundant and com-plementary.Consequently,our tracking algorithm is based on colour,edges and texture,which exhibit different failure modes with respect to external perturbations from lighting and background variations.Furthermore,partial pose track-ing is possible provided any one of the cues is observable. Tracking is divided into three sub-tasks:feature predic-tion,detection/association and state update.Feature predic-tion consists of estimating the expected visual cues from the object model and predicted pose.As usual,the pose is pre-dicted by evolving the state estimate from the previous frame using the system dynamics model in the Kalmanfiing the familiar central projection model,the predicted object is projected onto the image plane of each camera and rendered with standard computer graphics techniques.Figure2shows a typical frame from a tracking sequence with the predicted object overlaid as a wireframe model.The projection defines a bounding box(also shown in Figure2)for detectingasso-Figure2:Captured image,predicted pose and searchbox.(a)edges(b)textureFigure3:Predicted appearance of features.ciated measurements in the captured frame,which reduces computational load and eliminates distracting clutter.The computer renderings in Figure3show the expected appearance of the object in the current frame,from which a set of colour,edge and texture features are predicted.The tracking algorithm uses these predictions to guide the detec-tion of associated features in the captured image(Figure2). The prediction,detection and association algorithms for each cue are detailed in Section4.Finally,the associated mea-surements are used to estimate the current pose through the standard Kalmanfilter model described in the next section. While many tracking algorithms use afixed set of features, an important component of our approach is the dynamic se-lection of new tracking features in each frame.The possi-bility of association errors is reduced by only allowing pre-dicted and detected cues to participate in the state update.In this way,the tracking algorithm adapts naturally to the cur-rent viewing context.For example,when an object contains no discriminating texture,tracking automatically relies more heavily on colour and edge cues.Furthermore,when feature detection and association errors occur,the offending features do not persist for more than a single frame.In the Kalmanfilter framework,cues are integrated through measurement equations which predict the observed features as a function of thefilter state.The pose of the object is re-covered by minimizing the weighted error between the pre-dicted and measured features.Thus,any additional cue can be integrated provided a suitable measurement equation ex-ists.The same mechanism allows features to be fused from multiple cameras by simply applying the measurement equa-tions to each image plane.Unlike conventional n-view re-construction,this approach eliminates the need to search for correspondences as all features correspond implicitly through thefilter state.This allows the tracking algorithm to tolerate camera failures;in fact,tracking will continue even if the ob-ject is obscured from all but one of the cameras.3Kalman Filter FrameworkOur tracking algorithm uses an Iterated Extended Kalman Fil-ter(IEKF)to integrate visual cues and estimate the pose of the object.A detailed treatment of the IEKF can be found in[Bar-Shalom and Li,1993].Thefilter state is described by a12di-mensional vector x(k)=(p(k),p (k)) ,where p(k)and p (k) are the pose and velocity of the object in the k th frame.The filter also maintains an estimate of the state covariance matrix P(k),which is useful for performance evaluation.Assum-ing the object exhibits reasonably smooth motion,the state evolves according to a constant velocity dynamics model:ˆp(k+1|k)=p(k)+p (k)∆t(1)ˆp (k+1|k)=p (k)+v(k)(2)whereˆp(k+1|k)andˆp (k+1|k)are the predicted pose and velocity,∆t is the sample time between frames,and the state transition noise v(k)consumes unmodelled dynamics. Colour,edge and texture patch measurements extracted from the n cameras are stored in a measurement vector y(k+1),which has variable dimension depending on the number of detected features.For captured frame k+1,a measurement equation predicts the expected measurements ˆy(k+1)given the predicted state:ˆy(k+1)=h(ˆx(k+1|k))+w(k+1)(3)where w(k+1)represents the measurement noise.The mea-surement equations in h for each type of cue are described in Section4.The current stateˆx(k+1)is then estimated from a weighted sum of the estimated state and observation error:ˆx(k+1)=ˆx(k+1|k)+K(k+1)[y(k+1)−ˆy(k+1)](4)where thefilter gain K(k+1)is a function of the state transi-tion noise v(k),measurement noise w(k+1),predicted state covariance P(k+1|k)and the measurement function.The IEKF computes K(k+1)by linearizing the measurement function about an operating point,determined by iteration of (3)and(4)to give thefinal estimated pose.Noise vectors v(k)and w(k+1)are estimated from empirical observation in our current implementation,although we intend to estimate these terms on-line from the expected system dynamics and measurement process model in futurework.Figure4:Result of colourfilter applied to region of interest. The orientation component of the state vector must be han-dled carefully in the IEKF,as Euler angles are non-unique and even degenerate for some orientations.These issues could be addressed by representing the orientation as a normal-ized quaternion,which is always unique and non-degenerate. However,this adds a redundant degree of freedom to the state vector,and linear approximations in the IEKF necessitate fre-quent renormalization of the quaternion and corresponding covariance terms.We thus adopt the alternative approach de-scribed in[Welch and Bishop,1997];the orientation of the object is represented by an external quaternion,and the Euler anglesφ,θandψin the state vector describe only incre-mental changes.At each state update,the IEKF estimates the differential change in pose from the external quaternion.The Euler angles are then integrated into the external quaternion and reset to zero,ready for the next update.4Feature Measurement4.1ColourColour tracking is implemented using a colourfilter,which is sufficient for simple scenes.Alternative colour tracking tech-niques provide greater robustness against lighting variations and clutter[Agbinya and Rees,1999;McKenna et al.,1997] and may replace the colourfilter in future work.Thefilter is generated automatically from the texture information in the object model by compiling an RGB colour histogram,calcu-lated by partitioning RGB space into uniform cells(16cells along each axis)and counting the number of texture pixels within the bounds of each cell.Outlier rejection is applied by eliminating cells with the least pixels until no fewer than85% of the original pixels remain.The remaining non-empty cells form the pass-band of the colourfilter.During tracking,thefilter is applied to the region of interest in the captured frame.Figure4shows the result for the track-ing scenario in Figure2,where white areas represent regions of colour present in the object model.The colour cue mea-surement is recovered by applying binary connectivity to the filter output and calculating the centroid of the largest blob. Finally,the IEKF requires a measurement function to predict the observed centroid from thefilter state.For this purpose, we approximate the location of the colour centroid as the pro-(a)Edgefilteroutput.(b)Jump boundarypixels.(c)Detected edges.Figure5:Edge detection and matching.jection of the object’s centre of mass(calculated as the aver-age position of vertices in the model).The bias introduced by this simplification is readily overcome by other visual cues.4.2EdgesThe predicted edges for the tracking scenario in Figure2are shown in Figure3(a).Only the jump boundaries outlining the object are considered,since internal edges are easily con-fused with texture features.The j th predicted edge segment in a given camera is represented by the end-points a j and b j, from which we also calculate the normal direction n j and the perpendicular distance d j to the image plane origin.These parameters are used to guide edge detection in the captured frame.As usual,thefirst step in edge detection is to calculate intensity gradients using a central difference approximation:g x(x,y)=I(x+1,y)−I(x−1,y)(5)g y(x,y)=I(x,y+1)−I(x,y−1)(6) where I(x,y)is the intensity channel.An edge is detected at pixel position p i=(x i,y i) when g x>e th and g y>e th for the difference threshold e th,and the orientation of the detected edge is calculated asθi=tan−1(g x/g y).Figure5(a)shows the output of the edge detector for the region of interest in Figure2,with orientation indicated by grey level.The output in Figure5(a)contains numerous spurious mea-surements due to variations in texture and lighting.These distracting features are eliminated by combining edge detec-tion with the output from the colourfilter(see Figure4):only edges near the boundary of the largest colour blob are consid-ered as possible candidates for the desired jump boundaries. Skeletonization is applied to reduce edge pixels to a minimal set of candidates,and Figure5(b)shows the result.An association algorithm then attempts to match edge pix-els with predicted line segments.The i th edge pixel,with position p i and orientationθi,is associated the the j th line segment when the following conditions are satisfied:n T j p i+d j<r th(7)|tan−1(n y j/n x j)−θi|<θth(8) 0≤(p i−a j) (b j−a j)≤|b j−a j|2(9)where n j=(n x j,n y j) .Condition(7)requires the candidate pixel to satisfy the line equation to within an error threshold r th.Condition(8)enforces a maximum angular differenceθth between the orientation of the edge pixel and line segment. Finally,(9)ensures that the pixel lies within the end points of the edge.Ambiguous associations are resolved by assigning candidates to the segment with minimal residual error in(7). After edge pixel association,the j th predicted segment has n j matched pixels from which feature measurements are cal-culated.For segments with sufficient matches(n j>n th),two measurements are generated:the average position m j of edge pixels and the normal direction˜n j of afitted line,using prin-cipal component analysis(PCA).The robustness of the mea-surements is improved by performing PCA twice and apply-ing outlier rejection after thefirst iteration.Figure5(c)shows the mean position and orientation of observed edges for the tracking example in Figure2.The observed normals˜n j are added directly to the mea-surement vector in the IEKF,and additional data is supplied to indicate the associated model edges.The measurement equation in the IEKF then predicts˜n j from the image plane projection of the associated model edge for a given pose of the object.Conversely,the observed means m j cannot be di-rectly added to the measurement vector since they cannot be predicted from thefilter state.Instead,the measurement is treated as the distance between m j and the associated edge, which is implicitly zero.The measurement equation then cal-culates the distance between the observed means and pro-jected model edges for the given pose,so that model edges tend to coincide with m j for the optimal pose.4.3TextureTexture tracking algorithms typically employ small greyscale image templates as tracking features,which are matched to the captured image using sum of squared difference(SSD)(a)Texturequality.(b)Candidatefeatures.(c)Validatedfeatures.(d)Matched features.Figure6:Texture feature selection and matching.or correlation based measures.The templates are generally view-based and therefore dependent on the pose of the object. Most tracking algorithms address this issue by maintaining a quality measure to determine when a template no longer rep-resents the current appearance of a feature.Conversely,our algorithm solves the problem of view dependence by select-ing an entirely new set of texture features for each updated pose.This approach is only made possible by optimizing the feature selection calculations to operate in real time.Figure3(b)shows the predicted appearance of textures for the object in Figure2.Clearly,some regions constrain the tracking problem better than others;areas with omni-directional spatial frequency content such as corner and salt-and-pepper textures are generally considered the most suit-able.A widely accepted technique for locating such features is the quality measure proposed by[Shi and Tomasi,1994]. For each pixel in the rendered image,matrix Z is computed:Z=∑Wg2x g x g yg x g y g2y(10)where g x and g y are spatial intensity gradients calculated from (5)-(6),and W is the m×m template window surrounding the pixel.Good texture features are identified as satisfyingmin(λ1,λ2)>λth(11) whereλ1andλ2are the eigenvalues of Z.Figure6(a)shows the minimum eigenvalue at each pixel of the rendered im-age in Figure3(b).While the object is outlined by a high response to the quality measure,these areas usually straddle a jump boundary and are not considered suitable for track-ing.The feature selector therefore examines only the interior of the object using a window-based search to identify local maxima.An m×m template in the window surrounding each local maxima is extracted from the rendered image,forming a set of candidate texture features.The templates are matched to the captured image using SSD minimization to determine the offset d i between the pre-dicted and measured feature locations.The SSD for the i th feature is evaluated as:εi(d)=∑x∈W i[J(x)−I(x+d)−M(d)]2(12)where W i is the template window,J is the rendered image, I is the captured frame,and M(d)compensates for the mean intensity difference between predicted and measured features:M(d)=1m2∑x∈W i[J(x)−I(x+d)](13)The minimum SSD within a search window D i determines the displacement d i of the i th feature:d i=argmin d∈Diε(d)(14) Figure6(b)shows the candidate features and measured dis-placement vectors(scaled for clarity)for the object tracked in Figure2.A number of measured displacements will typically result from false matches,as the object model is only an ap-proximation to the actual appearance.A two-stage validation process is therefore applied before adding the results to the measurement vector of the IEKF.Let the initial candidate displacements be represented by the set of image plane vectors C={v i}.Assuming the pose error is approximately translational in the image plane(a common requirement for template-based tracking),the valid displacements will be identical.Thefirst validation step therefore attempts tofind the largest subsetˆC⊆C contain-ing approximately equal elements.For each candidate vector v i,wefirst construct a set S i of similar candidates:S i={v j:||v j−v i||<d th;v i,v j∈C}(15) where d th determines the maximum allowed distance between elements of S i.We then construct a set M i of mutually sup-porting vectors for each candidate,such that v i∈S j and v j∈S i for all v i,v j∈M i.This can be calculated as the in-tersection:M i={S i:v i∈S j}(16)The largest mutually supporting set of measurementsˆC= argmax|M i|are classified as valid.A second validation test requires template matching to be invertible for valid features.For each vector v i∈ˆC,a feature template is extracted from the measured position in the cap-tured frame and matched to the rendered image by SSD mini-mization,giving a reverse displacement vector r i.Finally,the valid measurements are the subset V⊆ˆC given byV={v i:||v i+r i||<r th}(17)for an empirically determined error threshold r th.Figure6(c) gives thefinal set of validated features from the initial can-didates in Figure6(b),and Figure6(d)shows the location of matched features in the captured frame.The observed template positions are added to the measure-ment vector of the IEKF,and a suitable function is then re-quired to predict these measurements given the pose of the object.A3D model of the texture cues is constructed by back-projecting a ray through the position of each template in the rendered image onto the surface of the object.The resulting set of3D surface points correspond to the location of texture cues in the frame of the object.The measurement function in the IEKF then simply transforms these points to the specified pose and projects them onto the image plane of the associated camera.5Experimental ResultsThe proposed tracking algorithm was implemented on the ex-perimental humanoid platform shown in Figure1.Stereo cameras are mounted on a pan/tilt/verge robotic head and cap-ture images at half PAL resolution(384×288pixels)and PAL framerate(25Hz).Projective rectification and radial distortion correction are applied to each frame prior to fea-ture extraction,so the tracking system can assume rectilinear stereo.Manual calibration was used to determine the extrin-sic and intrinsic parameters of the stereo rig.Image process-ing and Kalmanfilter calculations are performed on a dual 2.2GHz Intel Xeon.A number of optimizations were im-plemented to achieve real time(25Hz)performance:stereo fields are processed in parallel,and MMX/SSE code opti-mizations are used for parallel pixel operations.Our algorithm was applied to the task of tracking the tex-tured box shown in Figure2,which was manually handled to provide dynamics.The complete tracking sequence is shown in Video1,and Figure7reproduces selected frames from the left camera.Each image shows the predicted region of inter-est,detected features and estimated pose overlaid as a wire-frame model.Figure8shows the tracking performance of individual cues,measured as the number of observed features per frame(the plot shows every tenth frame for clarity). Initially the model is manipulated through large pose vari-ations as shown in Figures7(a)and7(b).Good contrast and low clutter allow the tracking algorithm to detect a high num-ber of features during this portion of the sequence.In Figures51015200100200300400500600 NumberoffeaturesframeCue performance in box tracking sequenceedgescolour+textureFigure8:Experimental tracking performance of each cue.7(c)and7(d)the object is manipulated towards an area of low contrast,with a corresponding drop in the number of detected edges(between frames200and300).However,colour and texture features are unaffected and allow tracking to continue despite a lack of edges.Finally,the object isflipped to reveal its hidden surfaces in Figures7(e)and7(f).Since the textures on these surfaces were obscured during model construction, the number of detected texture features drops to zero.In this case,tracking is maintained by the uninterrupted detection of colour and edges.By maintaining track of the pose during the entire sequence,these results demonstrate how fusing multi-modal features provides robustness against tracking losses in individual cues.6Conclusion and Future WorkWe have presented a tracking algorithm that fuses colour, edge and texture cues from stereo cameras in a Kalmanfil-ter framework to track the pose of a3D model.Cues are fused through the measurement function in the Kalmanfilter, which relate the observed multimodal features to the pose of the object.This framework is completely extensible;addi-tional cameras and cues can be added to the tracking algo-rithm provided a suitable measurement function exists.Ex-perimental results demonstrate that multiple cue fusion al-lows the tracking algorithm to overcome short-term losses in individual cues due to changes in visual conditions that would otherwise distract single-cue algorithms.The obvious extension to this work is the addition of modalities that have not been exploited,such as motion and depth.The algorithm may also be refined in a number of other areas.Calculation of thefilter weight and state covariance re-quires estimates of the observation errors,which are currently fixed values determined empirically.It may be possible to re-cover uncertainties as part of the measurement process,which would provide better weighting of observations and more ro-bust adaptation to actual tracking conditions. Observations are restricted to afixed window of interest to reduce computational expense and eliminate background(a)frame0(b)frame100(c)frame180(d)frame250(e)frame350(f)frame500Figure7:Selected frames from box tracking sequence.clutter.However,the restricted window also hinders recov-ery after a tracking loss.Tracking performance could beimproved by varying the size of the tracking window withthe covariance of the pose,providing an automatically largersearch space when conditions degrade.Finally,the current system model employs a constant ve-locity state update equation,which is sufficient for free-moving objects with smooth dynamics.However,our ulti-mately aim is to provide a robot with robust grasping andmanipulation skills.Once an object is grasped,the trackingfilter should employ a constrained dynamics model imposedby the motion of the robot to improve tracking performance.AcknowledgementThis project was funded by the Strategic Monash UniversityResearch Fund for the Humanoid Robotics:Perception,In-telligence and Control project at IRRC.References[Agbinya and Rees,1999]J.I.Agbinya and D.Rees.Multi-object tracking in video.Real-Time Imaging,5:295–304,1999.[Bar-Shalom and Li,1993]Y.Bar-Shalom and X-R.Li.Es-timation and Tracking:Principles,Techniques and Soft-ware.Artech House,1993.[Bentivegna et al.,2002]D.C.Bentivegna, A.Ude, C.G.Atkeson,and G.Cheng.Humanoid robot learning andgame playing using PC-based vision.In Proc.IEEE/RSJ2002Int.Conf.on Intelligent Robots and Systems,vol-ume3,pages2449–2454,2002.[Darrell et al.,2000]T.Darrell,G.Gordon,M.Harville,andJ.Woodfill.Integrated perfon tracking using stereo,colorand pattern detection.Int.Journal of Computer Vision,37(2):175–185,2000.[Davison,1988]A.Davison.Mobile Robot Navigation Us-ing Active Vision.PhD thesis,University of Oxford,1988.[Hutchinson et al.,1996]S.Hutchinson,G.D.Hagar,andP.I.Corke.A tutorial on visual servo control.IEEE Trans.on Robotics and Automation,12(5):651–670,1996.[Kleeman,1992]L.Kleeman.Optimal estimation of posi-tion and heading for mobile robots using ultrasonic bea-cons and dead-reckoning.In IEEE International Con-ference on Robotics and Automation,pages2582–2587,1992.。