On stability in fuzzy linear programming problems
非线性薛定谔方程数值解的MATLAB仿真

admin[非线性薛定谔方程数值解的MATLAB仿真]——利用分步快速傅里叶变换对光纤中光信号的传输方程进行数值求解1、非线性薛定谔方程非线性薛定谔方程(nonlinear Schrodinger equation ,NLSE)是奥地利物理学家薛定谔于1926 年提出的,应用在量子力学系统中。
由于量子力学主要研究粒子的动力学运动状态,所以不能运用牛顿力学公式来表示。
通常在量子力学中,研究系统的状态一般通过波函数(x ,t)来表示。
而对波函数的研究主要是求解非线性薛定谔方程。
本文主要研究光脉冲在光纤中传输状态下的演变。
一般情况下,光脉冲信号在光纤中传输时,同时受到光纤的色散和非线性效应的影响。
通过Maxwell 方程,考虑到光纤的色散和非线性效应,可以推导出光信号在光纤中的传输方程,即非线性薛定谔方程。
NLSE 是非线性偏微分方程,一般很难直接求出解析解,于是通过数值方法进行求解。
具体分为两大类:(1)分布有限差分法(split-step finite differencemethod ,SSFD);(2)分步傅里叶变换法(split-step Fourier transform method ,SSFT)。
一般情况,在达到相同精度,由于分步傅里叶变换法采用运算速度快的快速傅里叶变换,所以相比较有限差分法运算速度快一到两个数量级。
于是本文介绍分步傅里叶变换法来对光纤中光信号的传输方程,即非线性薛定谔方程进行数值求解。
并通过MATLAB 软件对结果数值仿真。
非线性薛定谔方程的基本形式为:22||t xx iu u u u =+其中u 是未知的复值函数.目前,采用分步傅立叶算法(Split step Fourier Method)求解非线性薛定谔方程的数值解应用比较多。
分步傅立叶方法最早是在1937年开始应用的,这种方法己经被证明是相同精度下数值求解非线性薛定愕方程最快的方法,部分原因是它采用了快速傅立叶变换算法(Fast Fourier Transform Algorithm)。
巴特沃斯滤波器求阶数n
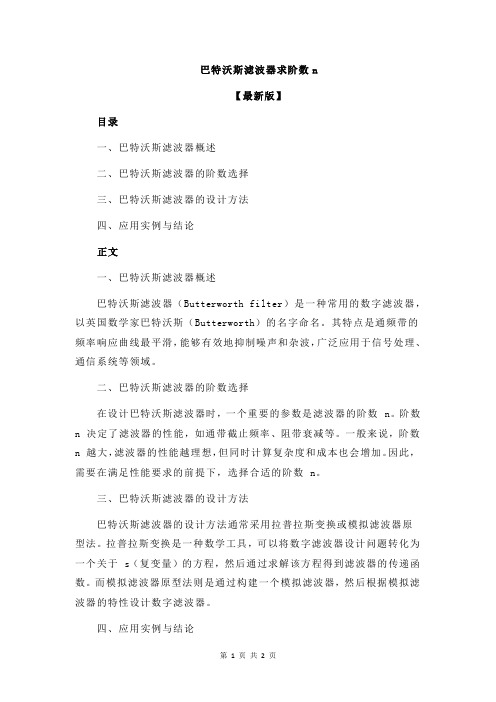
巴特沃斯滤波器求阶数n
【最新版】
目录
一、巴特沃斯滤波器概述
二、巴特沃斯滤波器的阶数选择
三、巴特沃斯滤波器的设计方法
四、应用实例与结论
正文
一、巴特沃斯滤波器概述
巴特沃斯滤波器(Butterworth filter)是一种常用的数字滤波器,以英国数学家巴特沃斯(Butterworth)的名字命名。
其特点是通频带的频率响应曲线最平滑,能够有效地抑制噪声和杂波,广泛应用于信号处理、通信系统等领域。
二、巴特沃斯滤波器的阶数选择
在设计巴特沃斯滤波器时,一个重要的参数是滤波器的阶数 n。
阶数n 决定了滤波器的性能,如通带截止频率、阻带衰减等。
一般来说,阶数n 越大,滤波器的性能越理想,但同时计算复杂度和成本也会增加。
因此,需要在满足性能要求的前提下,选择合适的阶数 n。
三、巴特沃斯滤波器的设计方法
巴特沃斯滤波器的设计方法通常采用拉普拉斯变换或模拟滤波器原
型法。
拉普拉斯变换是一种数学工具,可以将数字滤波器设计问题转化为一个关于 s(复变量)的方程,然后通过求解该方程得到滤波器的传递函数。
而模拟滤波器原型法则是通过构建一个模拟滤波器,然后根据模拟滤波器的特性设计数字滤波器。
四、应用实例与结论
巴特沃斯滤波器在信号处理和通信系统中有广泛的应用。
例如,在音频处理中,可以使用巴特沃斯滤波器对音频信号进行降噪和音质改善;在通信系统中,可以使用巴特沃斯滤波器对信号进行预处理,以提高信号的可靠性和抗干扰性。
总之,巴特沃斯滤波器是一种优秀的数字滤波器,具有良好的性能和实用性。
线性不确定随机系统时滞相关的H∞滤波

tr c u rn n b t a n vm arc sa d s se m arc sa ea od db nr d cn o r eweg tn e msa c r ig i o h Ly pu o tie n y tm tie r v ie y ito u igs mefe ih ig
模 型 转 换 , 立 了新 的 L a u o - a o s i 函数 。 通 过 引入 自由加 权 矩 阵 , 除 了 I a u o 建 y p n v Krs v ki 消 p n v矩 阵 和 系统 矩 阵 的 y
乘 积 项 , 而 无 需 在 滤 波 器设 计 过 程 中对 L a u o 矩 阵 作 任 何 约 束 , 在 很 大 程 度 上 降 低 了滤 波 器 设 计 的 保 守 从 ypn v 这
Jn 2 1 a. 00
线 性 不 确 定 随 机 系统 。 。 ,罗 小元
( .新疆 大学数 学 与系统科 学 学院 ,新疆 乌 鲁木齐 8 0 4 ; 1 3 0 6 2 .燕 山大学 电气工 程 学院 ,河北 秦皇 岛 0 6 0 ) 6 0 4
第 3 2卷
第 1 期
系 统 工 程 与 电子 技 术
Sys e s Eng n e i d El c r i s tm i e rng an e ton c
Vo . 2 No 1 13 .
21 0 0年 1 月
文章 编 号 :0 15 6 ( 0 0 0 — 1 20 1 0 —0 X 2 1 ) 1 5 — 6 0
Ab t a t Th o u t H 。 i e i g p o l m o o tn o s tme l e r s o h s i s s e t i e v r sr c : e r b s 。fl rn r b e f r c n i u u —i i a t c a tc y t ms wih t — a y t n m
模糊控制器设计外文资料翻译--离散模糊双线性系统的静态输出反馈控制

模糊控制器设计外文资料翻译--离散模糊双线性系统的静态输出反馈控制中文2094字外文资料翻译Static Output Feedback Control for Discrete-time Fuzzy Bilinear System Abstract The paper addressed the problem of designing fuzzy static output feedback controller for T-S discrete-time fuzzy bilinear system (DFBS). Based on parallel distribute compensation method, some sufficient conditions are derived to guarantee the stability of the overall fuzzy system. The stabilization conditions are further formulated into linear matrix inequality (LMI) so that the desired controller can be easily obtained by using the Matlab LMI toolbox. In comparison with the existing results, the drawbacks such as coordinate transformation, same output matrices have been eliminated. Finally, a simulation example shows that the approach is effective.Keywords discrete-time fuzzy bilinear system (DFBS); static output feedback control; fuzzy control; linear matrix inequality (LMI)1 IntroductionIt is well known that T-S fuzzy model is an effective tool for control of nonlinear systems where the nonlinear model is approximated by a set of linear local models connected by IF-THEN rules. Based on T-S model, a great number of results have been obtained on concerning analysis and controllerdesign[1]-[11]. Most of the above results are designed based on either state feedback control or observer-based control[1]-[7].Very few results deal with fuzzy output feedback[8]-[11]. The scheme of static output feedback control is very important and must be used when the system states are not completely available for feedback. The static output feedback control for fuzzy systems with time-delay was addressed [9][10] and a robust H∞ controller via static output feedback was designed[11]. But the derived conditions are not solvable by the convex programming technique since they are bilinear matrix inequality problems. Moreover, it is noted that all of the aforementioned fuzzy systems were based on the T-S fuzzy model with linear rule consequence.Bilinear systems exist between nonlinear and linear systems, which provide much better approximation of the original nonlinear systems than the linear systems [12].The research of bilinear systems has been paid a lot of attention and a series of results have been obtained[12][13].Considering the advantages of bilinear systems and fuzzy control, the fuzzy bilinear system (FBS) based on the T-S fuzzy model with bilinear rule consequence was attracted the interest of researchers[14]-[16]. The paper [14] studied the robust stabilization for the FBS, then the result was extended to the FBS with time-delay[15]. The problem of robust stabilization for discrete-time FBS (DFBS) was considered[16]. But all the above results are obtained via state feedback controller.In this paper, a new approach for designing a fuzzy static output feedback controller for theDFBS is proposed. Some sufficient conditions for synthesis of fuzzy static output feedback controller are derived in terms of linear matrix inequality (LMI) and the controller can be obtained by solving a set of LMIs. In comparison with the existing literatures, the drawbacks such as coordinate transformation and same output matrices have been eliminated.Notation: In this paper, a real symmetric matrix 0P > denotes P being a positive definite matrix. In symmetric block matrices, an asterisk (*) is used to represent a symmetric term and {...}diag stands for a block-diagonal matrix. The notion ,,1si j l =∑means 111s s si j l ===∑∑∑. 2 Problem formulationsConsider a DFBS that is represented by T-S fuzzy bilinear model. The i th rule of the DFBS is represented by the following form11 ()...() (1)()()()()()()1,2,...,i i v vi i i i i R if t is M and and t is M then x t A x t B u t N x t u t y t C x t i sξξ+=++== (1)Where iR denotes the fuzzy inference rule, s is the number of fuzzy rules.,1,2...ji M j v=is fuzzy set and()j t ξis premise variable.()nx t R ∈Is the statevector,()u t R ∈is the control input and T 12()[(),(),..,()]q q y t y t y t y t R =∈ is the system output. The matrices ,,,i i i iA B N C are known matrices with appropriatedimensions. Since the static output feedback control is considered in this paper,we simply set v q =and11()(),...,()()v q t y t t y t ξξ==.By using singleton fuzzifier, product inference and center-averagedefuzzifier, the fuzzy model(1) Can be expressed by the following global model11(1)(())[()()()()]()(())()si i i i i si i i x t h y t A x t B u t N x t u t y t h y t C x t ==+=++=∑∑(2)Where 11(())(())/(()),(())(())qsi i i i ij i j h y t y t y t y t y t ωωωμ====∑∏.(())ij y t μisthe grade of Membership of ()j y t in jiM . We assumethat (())0iy t ω≥and 1(())0si i y t ω=>∑. Then we have the following conditions:1(())0,(())1si i i h y t h y t =≥=∑.Based on parallel distribute compensation,the fuzzy controller shares the same premise parts with (1); that is, the static output controller for fuzzy rule i is written as11T T ()... () ()()1i i v vi i i i R if y t is M and and y t is M then u t F y t y F F yρ=+(3)Hence, the overall fuzzy control law can be represented as111()sin cos ()1ss si i i i i i i i i i T T i i u t h h h F y t y F F y ρθρθ======+∑∑∑ (4)WhereT TTTsin [,],1,2,...,2211i i i i i i i i i s y F F yy F F yππθθθ==∈-=++.1qi F R ⨯∈is a vector to be determined and 0ρ>is a scalar to be assigned.By substituting (4) into (2), the closed-loop fuzzy systems can be represented as,,11(1)()()()si j l ijl i j l si i i x t h h h x t y t h C x t ==+=Λ=∑∑ (5)wherecos sin ijl i i j l j i jA B F C N ρθρθΛ=++.The objective of this paper is to design fuzzy controller (4) such that the DFBS (5) is asymptotically stable. 3 Main resultsNow we introduce the following Lemma which will be used in our main results.Lemma 1 Given any matrices ,M N and 0P >with appropriate dimensionssuch that 0ε>, the inequality T T T 1TM PN N PM M PM N PN εε-+≤+holds.Proof: Note that 11112222()()()()T TTTM PN N PM P M P N P N P M +=+Applying Lemma 1 in [1]: 1T T T TM N N M M M N N εε-+≤+, the inequalityT T T 1T M PN N PM M PM N PN εε-+≤+can be obtained. Thus the proof iscompleted.Theorem 1 For given scalar 0ρ>and 0,,1,2,...,ij i j sε>=, the DFBS (5) is asymptotically stable in the large, if there exist matrices 0Q >and ,1,2,...,i F i s=satisfying the inequality (6).T T T 111()0000 ,,1,2,...,i i i j l ijl Q QA QN B F C Q a Q b Q b Q i j l s---⎡⎤-⎢⎥*-⎢⎥Φ=<⎢⎥**-⎢⎥***-⎣⎦= (6)Where211,(1)ij ij a b ερε-=+=+.Proof: Consider the Lyapunov function candidate asT ()()()V t x t Px t =(7)where 1P Q -= is to be selected.Define the difference ()(1)()V t V t V t ∆=+-, and then along the solution of (5), we haveT TT ,,1,,1T T T T 12,,1,,1T T T ,,1()()()()()()()()()() ()()()ssi j l m n p ijl mnp i j l m n p ssi j l m n p ijl mnp ijl mnp i j l m n p s i j l ijl ijl i j l V t h h h h h h x t P x t x t Px t h h h h h h x t P P x t x t Px t h h h x t P x t x t P =====∆=ΛΛ-=ΛΛ+ΛΛ-≤ΛΛ-∑∑∑∑∑()x t (8)Applying Lemma 1 again, it follows thatT T T 21T T (cos sin )(cos sin ) (1)(1)[()()]ijl ijl i i j l j i j i i j l j i j ij i i ij i j l i j l i i P A B F C N P A B F C N A PA B F C P B F C N PN ρθρθρθρθερε-ΛΛ=++++≤++++ (9)Substituting (9) into (8) leads toT T T T ,,1()()[()()]()si j l i i i j l i j l i i i j l V t h h h x t aA PA b B F C P B F C bN PN P x t =∆≤++-∑ (10)Applying the Schur complement, (6) is equivalent toT 1T 1T 1()(Q)0i i i j l i j l i i Q aQA Q A Q b B F C Q Q B F C bQN Q N Q ----+++<(11)Pre- and post multiplying both side of (11) with P , respectively, we haveT T T ()()0i i i j l i j l i i P aA PA b B F C P B F C bN PN -+++< (12)Therefore, it is noted that ()0V t ∆<, then the DFBS (5) is asymptotically stable. Thus the proof is completed.The matrix inequality (6) leads to BMI optimization, a non-convexprogramming problem. In the following theorem, we will derive a sufficient condition such that the matrix inequality (6) can be transformed into an LMI problem.Theorem 2 For given scalar 0ρ>and 0,,1,2,...,ij i j sε>=, the DFBS (5) is asymptotically stable in the large, if there exist matrices 0Q >and ,1,2,...,i F i s=satisfying the inequality (13).T T T 1110()000000 ,,1,2,...,i i l ijl i j Q QA QN C Q a Q I b Q I b Q B F I i j l s ---⎡⎤-⎢⎥*-+⎢⎥⎢⎥ψ=<**-+⎢⎥***-⎢⎥⎢⎥****-⎣⎦= (13)Proof: It is trivial thatT T ()()00000()()l l ijl i j i j C Q C Q I I B F B F φ⎡⎤⎢⎥*⎢⎥=>⎢⎥**⎢⎥***⎢⎥⎣⎦(14)Then ifijl ijl φΦ+<, we can conclude that 0ijl Φ<.TT T T 11T 1TTT111()()000()000000()()()0000 00l l i i i j l ijl ijl i j i j l i i i j C Q C Q Q QA QN B F C Q I a Q I b Q B F B F b Q C Q Q QA QN a Q I b Q IB F b Q φ------⎡⎤⎡⎤-⎢⎥⎢⎥**-⎢⎥⎢⎥Φ+=+⎢⎥⎢⎥****-⎢⎥⎢⎥******-⎢⎥⎣⎦⎣⎦⎡⎡⎤-⎢⎢⎥*-+⎢⎢⎥=+⎢⎥**-+⎢⎥***-⎣⎦⎣T 00()l i j C Q B F ⎤⎥⎥⎡⎤⎣⎦⎢⎥⎢⎥⎢⎥⎦(15)By applying Schur complement, (13) is equivalent to 0ijl ijl φΦ+<. Then wegetijl Φ<. According to Theorem 1, the DFBS (5) is asymptotically stable. Thusthe proof is completed.4 Numerical examplesIn this section, an example is used for illustration. The considered DFBS is1: (1)()()()()()() 1,2i ii i i i R ify is M then x t A x t B u t N x t u t y t C x t i +=++==Where1212129.78-1010101,,,510510011A A N N B B ----⎡⎤⎡⎤⎡⎤⎡⎤======⎢⎥⎢⎥⎢⎥⎢⎥---⎣⎦⎣⎦⎣⎦⎣⎦ [][]1210,10.C C ==-The membership functions are defined as111()(1cos())/2,M y y μ=-2111()1()M M y y μμ=-.By letting111221220.72,0.2, 1.51,ρεεεε===== applying Theorem 2 andsolving the corresponding LMIs, we can obtain the following solutions:[][]12-1.5014,11.0790 2.2785,-3.0452.2.27850.5984F Q F =⎡⎤=⎢⎥=⎣⎦Simulation results with the initial conditions: []T1.4-1.6 respective, areshown in Fig.1 and Fig.2. One can find that all these state converge to the equilibrium state after 17 seconds.tx (t )x 1x 2tu (t )Fig.1. State responses of system Fig.2. Control trajectory of system 5 ConclusionsIn this paper, a new and simple approach for designing a fuzzy static output feedback controller for the discrete-time fuzzy bilinear system is presented. The result is formulated in terms of a set of LMI-based conditions. By the proposed approach, the local output matrices are not necessary to be the same. Thus, the constraints had been relaxed and applicability of the static output feedback is increased.References[1] Wang R J, Lin W W and Wang W J. Stabilizability of linear quadratic state feedback for uncertain fuzzy time-delay systems [J]. IEEE Trans. Syst., Man, and Cybe., 2004, 34(2):1288-1292.[2] Cao Y Y and Frank P M. Analysis and synthesis of nonlinear time-delay systems via fuzzy control approach [J]. IEEE Trans. Fuzzy Syst., 2000, 18(2): 200-211.[3] Yoneyama J. Robust stability and stabilization for uncertain Takagi-Sugeno fuzzy time-delay systems [J]. Fuzzy Sets and Syst., 2007, 158(4): 115-134.[4] Shi X Y and Gao Z W. Stability analysis for fuzzy descriptor systems [J]. Systems Engineering and Electronics, 2005, 27(6):1087-1089. (In Chinese)[5] Jiang X F and Han Q L. On designing fuzzy controllers for a class of nonlinear networked control systems[J].IEEE Trans. Fuzzy Syst., 2008, 16(4): 1050-1060.[6] Lin C, Wang Q G, Lee T H, et al. Design of observer-based H∞ control for fuzzy time-delay systems[J]. IEEE Trans. Fuzzy Syst., 2008, 16(2): 534-543.[7] Kim S H and Park P G. Observer-based relaxed H∞ control for fuzzy systems using a multiple Lyapunov function[J]. IEEE Trans. Fuzzy Syst., 2009, 17(2): 476-484.[8] Zhang Y S, Xu S Y and Zhang B Y. Robust output feedback stabilization for uncertain discrete-time fuzzy markovian jump systems with time-varying delays[J]. IEEE Trans. Fuzzy Syst., 2009, 17(2): 411-420.[9] Chang Y C, Chen S S, Su S F, et al. Static output feedback stabilization for nonlinear interval time-delay systems via fuzzy control approach [J]. Fuzzy Sets and Syst., 2004, 148(3): 395-410.[10] Chen S S, Chang Y C, Su S F, et al. Robust static output-feedback stabilization for nonlinear discrete-time systems with time delay via fuzzy control approach[J]. IEEE Trans. Fuzzy Syst., 2005, 13(2): 263-272.[11] Hua ng D and Nguang S K. Robust H∞ static output feedback control of fuzzy systems: a LMIs approach [J]. IEEE Trans. Syst., Man, and Cybe., 2006, 36: 216-222.[12] Mohler R R. Nonlinear systems: Vol.2 Application to Bilinear control [M]. Englewood Cliffs, NJ: Prentice-Hall, 1991[13] Dong M and Gao Z W. H∞ fault-tolerant control for singular bilinear systems related to output feedback[J]. Systems Engineering and Electronics, 2006, 28(12):1866-1869. (In Chinese)[14] Li T H S and Tsai S H. T-S fuzzy bilinear model and fuzzy controller design for a class of nonlinear systems [J]. IEEE Trans. Fuzzy Syst., 2007, 3(15):494-505.[15] Tsai S H and Li T H S. Robust fuzzy control of a class of fuzzy bilinear systems with time-delay [J]. Chaos, Solitons and Fractals (2007), doi: 10.1016/j. chaos.2007.06.057.[16] Li T H S, Tsai S H, et al, Robust H∞ fuzzy control for a class of uncertain discrete fuzzy bilinear systems [J]. IEEE Trans. Syst., Man, and Cybe., 2008, 38(2) : 510-526.离散模糊双线性系统的静态输出反馈控制摘要:研究了一类离散模糊双线性系统(DFBS)的静态输出反馈控制问题。
鞅差中心极限定理
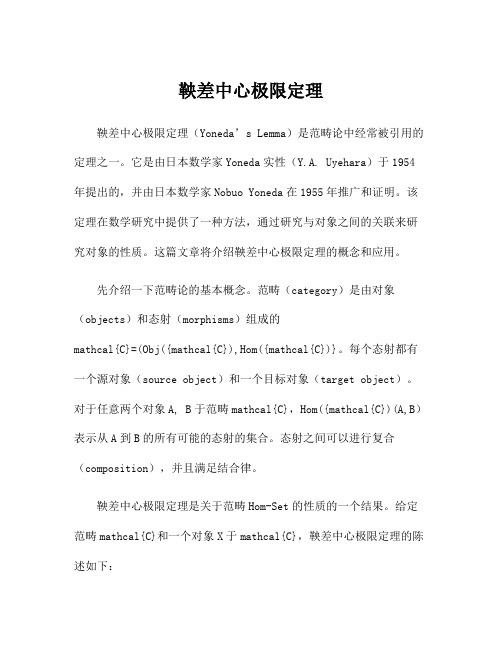
鞅差中心极限定理鞅差中心极限定理(Yoneda’s Lemma)是范畴论中经常被引用的定理之一。
它是由日本数学家Yoneda实性(Y.A. Uyehara)于1954年提出的,并由日本数学家Nobuo Yoneda在1955年推广和证明。
该定理在数学研究中提供了一种方法,通过研究与对象之间的关联来研究对象的性质。
这篇文章将介绍鞅差中心极限定理的概念和应用。
先介绍一下范畴论的基本概念。
范畴(category)是由对象(objects)和态射(morphisms)组成的mathcal{C}=(Obj({mathcal{C}),Hom({mathcal{C})}。
每个态射都有一个源对象(source object)和一个目标对象(target object)。
对于任意两个对象A, B于范畴mathcal{C},Hom({mathcal{C})(A,B)表示从A到B的所有可能的态射的集合。
态射之间可以进行复合(composition),并且满足结合律。
鞅差中心极限定理是关于范畴Hom-Set的性质的一个结果。
给定范畴mathcal{C}和一个对象X于mathcal{C},鞅差中心极限定理的陈述如下:对于任意对象A于mathcal{C},Hom({mathcal{C})(A,X)与Hom({mathcal{C})(A,X+n)之间存在一个自然变换。
其中n表示任意对象。
这个定理的关键在于“中心极限”(central limit)。
直观的来说,这个定理说明了给定一个对象A于范畴mathcal{C}和一个差n,在Hom-Set Hom({mathcal{C})(A,X)和Hom({mathcal{C})(A,X+n)之间存在一个特殊的变换。
它将每个态射映射到一个稍微偏移一点的态射,表示了A到X的“鞅差”或“波动”。
在实际应用中,鞅差中心极限定理可以帮助数学家在给定了一个对象A时,研究从A到X的态射的性质。
这些态射在形式上可能非常复杂,但鞅差中心极限定理提供了一种方法,将这些态射转化为一种更简单的形式。
robust solutions of uncertain linear programs文献讲解
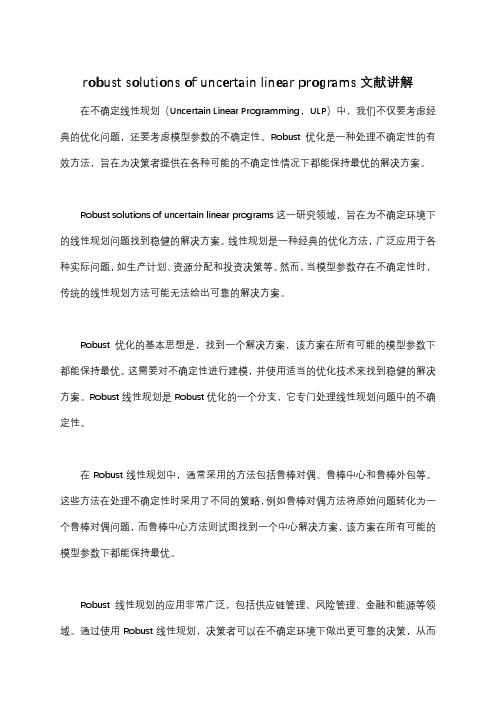
robust solutions of uncertain linear programs文献讲解在不确定线性规划(Uncertain Linear Programming,ULP)中,我们不仅要考虑经典的优化问题,还要考虑模型参数的不确定性。
Robust优化是一种处理不确定性的有效方法,旨在为决策者提供在各种可能的不确定性情况下都能保持最优的解决方案。
Robust solutions of uncertain linear programs这一研究领域,旨在为不确定环境下的线性规划问题找到稳健的解决方案。
线性规划是一种经典的优化方法,广泛应用于各种实际问题,如生产计划、资源分配和投资决策等。
然而,当模型参数存在不确定性时,传统的线性规划方法可能无法给出可靠的解决方案。
Robust优化的基本思想是,找到一个解决方案,该方案在所有可能的模型参数下都能保持最优。
这需要对不确定性进行建模,并使用适当的优化技术来找到稳健的解决方案。
Robust线性规划是Robust优化的一个分支,它专门处理线性规划问题中的不确定性。
在Robust线性规划中,通常采用的方法包括鲁棒对偶、鲁棒中心和鲁棒外包等。
这些方法在处理不确定性时采用了不同的策略,例如鲁棒对偶方法将原始问题转化为一个鲁棒对偶问题,而鲁棒中心方法则试图找到一个中心解决方案,该方案在所有可能的模型参数下都能保持最优。
Robust线性规划的应用非常广泛,包括供应链管理、风险管理、金融和能源等领域。
通过使用Robust线性规划,决策者可以在不确定环境下做出更可靠的决策,从而提高组织的效率和竞争力。
综上所述,Robust solutions of uncertain linear programs是一个重要的研究领域,它为不确定环境下的线性规划问题提供了稳健的解决方案。
通过使用适当的优化技术和不确定性建模方法,我们可以找到在各种可能的不确定性情况下都能保持最优的解决方案,从而提高组织的效率和竞争力。
模糊赋范线性空间的紧性与完备性
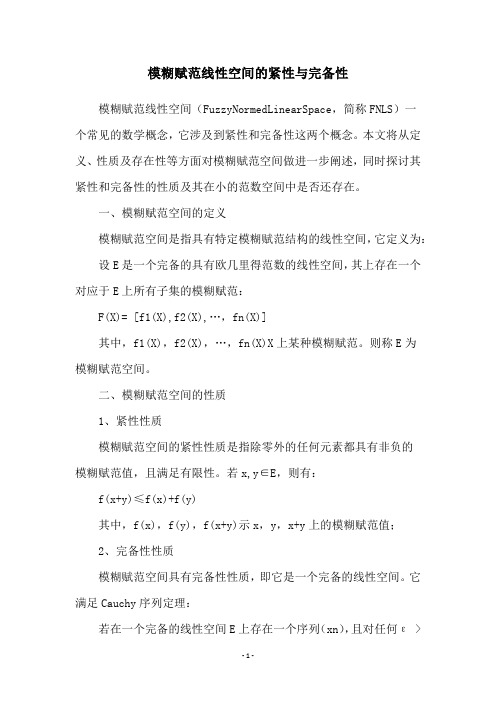
模糊赋范线性空间的紧性与完备性模糊赋范线性空间(FuzzyNormedLinearSpace,简称FNLS)一个常见的数学概念,它涉及到紧性和完备性这两个概念。
本文将从定义、性质及存在性等方面对模糊赋范空间做进一步阐述,同时探讨其紧性和完备性的性质及其在小的范数空间中是否还存在。
一、模糊赋范空间的定义模糊赋范空间是指具有特定模糊赋范结构的线性空间,它定义为:设E是一个完备的具有欧几里得范数的线性空间,其上存在一个对应于E上所有子集的模糊赋范:F(X)= [f1(X),f2(X),…,fn(X)]其中,f1(X),f2(X),…,fn(X)X上某种模糊赋范。
则称E为模糊赋范空间。
二、模糊赋范空间的性质1、紧性性质模糊赋范空间的紧性性质是指除零外的任何元素都具有非负的模糊赋范值,且满足有限性。
若x,y∈E,则有:f(x+y)≤f(x)+f(y)其中,f(x),f(y),f(x+y)示x,y,x+y上的模糊赋范值;2、完备性性质模糊赋范空间具有完备性性质,即它是一个完备的线性空间。
它满足Cauchy序列定理:若在一个完备的线性空间E上存在一个序列(xn),且对任何ε >0有lim(n→∞) f(xn)=0则称序列(xn)在E上模糊赋范收敛。
三、模糊赋范空间在小范数空间中的存在性模糊赋范空间既可以是一个大的完备空间,也可以是一个小的范数空间。
一个小的范数空间的模糊赋范空间是满足以下条件的:1、有边界:范数空间E是一个具有模糊赋范结构的边界空间,即其上存在一组模糊赋范;2、不改变结构:范数空间E上的模糊赋范不改变空间E的结构和性质;3、紧性:模糊赋范空间满足紧性性质;4、完备性:模糊赋范空间满足完备性性质。
因此,在满足上述性质的情况下,模糊赋范空间可以存在于小的范数空间中。
本文论述了模糊赋范空间的定义和性质,及其是否可以存在于小的范数空间中。
紧性性质和完备性性质是模糊赋范空间的关键,两者缺一不可。
紧性性质可以保证空间上的结构不变,完备性性质可以保证空间上的元素收敛。
LOGIC AND FUZZY SYSTEM Fuzzy Analogy of Linear Systems
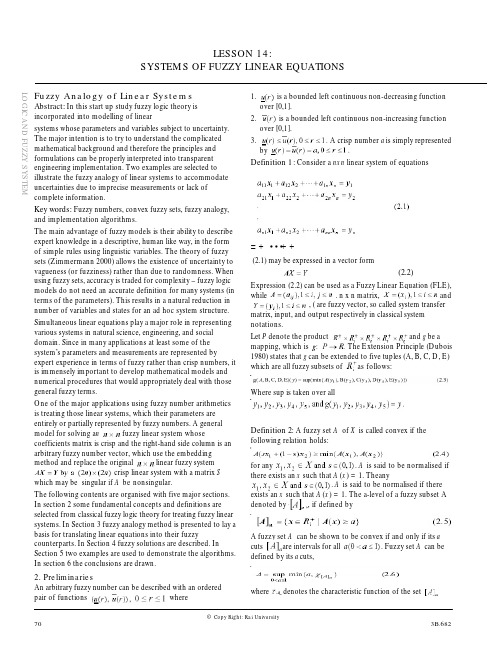
LOGIC AND FUZZY SYSTEMLESSON 14:SYSTEMS OF FUZZY LINEAR EQUATIONSFuzzy Analogy of Linear SystemsAbstract: In this start up study fuzzy logic theory is incorporated into modelling of linearsystems whose parameters and variables subject to uncertainty.The major intention is to try to understand the complicated mathematical background and therefore the principles and formulations can be properly interpreted into transparent engineering implementation. Two examples are selected to illustrate the fuzzy analogy of linear systems to accommodate uncertainties due to imprecise measurements or lack of complete information.Key words: Fuzzy numbers, convex fuzzy sets, fuzzy analogy,and implementation algorithms.The main advantage of fuzzy models is their ability to describe expert knowledge in a descriptive, human like way, in the form of simple rules using linguistic variables. The theory of fuzzy sets (Zimmermann 2000) allows the existence of uncertainty to vagueness (or fuzziness) rather than due to randomness. When using fuzzy sets, accuracy is traded for complexity – fuzzy logic models do not need an accurate definition for many systems (in terms of the parameters). This results in a natural reduction in number of variables and states for an ad hoc system structure.Simultaneous linear equations play a major role in representing various systems in natural science, engineering, and social domain. Since in many applications at least some of the system’s parameters and measurements are represented byexpert experience in terms of fuzzy rather than crisp numbers, it is immensely important to develop mathematical models and numerical procedures that would appropriately deal with those general fuzzy terms.One of the major applications using fuzzy number arithmetics is treating those linear systems, which their parameters are entirely or partially represented by fuzzy numbers. A general model for solving a n fuzzy linear system whosecoefficients matrix is crisp and the right-hand side column is an arbitrary fuzzy number vector, which use the embedding method and replace the original linear fuzzy systemcrisp linear system with a matrix Swhich may be singular if A be nonsingular.The following contents are organised with five major sections.In section 2 some fundamental concepts and definitions are selected from classical fuzzy logic theory for treating fuzzy linear systems. In Section 3 fuzzy analogy method is presented to lay a basis for translating linear equations into their fuzzycounterparts. In Section 4 fuzzy solutions are described. InSection 5 two examples are used to demonstrate the algorithms.In section 6 the conclusions are drawn.2. PreliminariesAn arbitrary fuzzy number can be described with an ordered pair of functions where1. is a bounded left continuous non-decreasing functionover [0,1].2. is a bounded left continuous non-increasing function over [0,1].3.A crisp number a is simply representedbyDefinition 1 : Consider a nxnlinear system of equations= + · · · + +(2.1) may be expressed in a vector form(2.2)Expression (2.2) can be used as a Fuzzy Linear Equation (FLE),while . n x n matrix, and, ( are fuzzy vector, so called system transfermatrix, input, and output respectively in classical system notations.Let P denote the product and g be a mapping, which is The Extension Principle (Dubois 1980) states that g can be extended to five tuples (A, B, C, D, E)which are all fuzzy subsets ofas follows:Where sup is taken over allDefinition 2: A fuzzy set A of X is called convex if thefollowing relation holds:for any . A is said to be normalised if there exists an x such that A (x ) = 1. Theany. A is said to be normalised if thereexists an x such that A (x ) = 1. The a-level of a fuzzy subset A denoted by, if defined byA fuzzy set A can be shown to be convex if and only if its a cuts are intervals for all . Fuzzy set A can be defined by its acuts,wheredenotes the characteristic function of the setLOGIC AND FUZZY SYSTEMDefinition 3 Fuzzy number A is called a number of the R L .type if its membership functionhas the following form:where L and R are continuous non-increasing functions, defined on ), strictly decreasing to zero in those subintervals of the interval ) , in which they are positive, and fulfilling the condition The parameters a and â are non-negative real numbers.3. Fuzzy AnalogyIf X is the set of real numbers, by a fuzzy number x N , this means a fuzzy subset of X where •, if and only if y = x.•is continuous.• is convex.•vanishes at infinity..denotes the degree of belief that the value of x is infact y. Consider a class of functional equations of the formwhere f is unknown, may be either addition or multiplication of real numbers. (3.1) may be put in a generalformwhereis a function relating the unknownquantities stands for andstands forLet denote the degree of belief that x equals to y . Using extension principle (Dubois 1980), (3.2) can be expressed in thefuzzy formwhere the sup is taken over all y 1and y2 for whichAccording to Nguyen (1978), (3.3) and (3.1) may be the implies as following For the alevels are equalis a closed and bounded interval. Hence,,where are the left endpointand the right endpoint respectively associated with the a level of the fuzzy number w . If F is non-decreasing in its argument and Fis continuous, then it givesMoreover solutions to (3.5) and (3.6), say functions H 1and H2, respectively, are continuous and no-decreasing. ThusSo, the solution of (3.3) is given bywhere H 1 and H 2 are the solutions of (3.5) and (3.6),respectively. According to (3.3), the solution of (3.8) may alsobe represented bywhere the sup is taken over allis thecharacteristic function over the interval4. Fuzzy Solutions A solution tto (2.1) one should recall that forarbitrary fuzzy numbers and realnumber k ,•x = yif and only if•Definition 4: A fuzzy number vector givenby is called a solution ofthe FSLE ifConsider the ith equation of the system (2.1)it hasFrom (2.4) two crisp linear systems for all i that there can be extended to. crisp linear system as follows:LOGIC AND FUZZY SYSTEMThus FLE (2.1) is extended to a crisp (2.5) where A= S 2 + S 1.(4.5) can be write as follows:where the matrix S is nonsingular if and only if the matrices A=S1+S2 and S1-S2 are both non-singular.Definition 5: Let denote the unique solution of SX = Y . The fuzzy number vectoris defined byis called the fuzzy solution of SX = Y . Ifare all triangular fuzzy numbers thenand U is called a strongfuzzy solution. Otherwise, U is a weak fuzzy solution.In the general, the structure of S implies thatand thatWhere B contains the positive entries of A , C the absolute values of the negative entries of A , and A=B-C If linear system are fuzzy variables, for the inputsdenotes the degree of,inputThesolution of linear system are givenIf linear system are fuzzy coefficients and fuzzy variables, whichis in system (2.1)are fuzzy numbers, denotes the degree ofcoefficients and variable respectively.where so the solution of systemsAX = Yis5. Examples and SimulationsTwo examples were selected to demonstrate the understanding of the fuzzy of analogy of linear systems.Example 1:Consider a simple linear system B AX Y + = withfuzzy output and input variablesFig.1 shows a fuzzy line, which denotes what a line looks like with a fuzzy input.Example 2 :Consider a two inputs & single output fuzzy system with fuzzy outputsY = AX + B(5.3)Where .The extended 4 x 4 . matrix isThe fuzzy solution isFig.2 shows that variables x 1and x 2 are determined by the output y which is the ácut, when y = 1, an accurate value for thesolution can be obtained.LOGIC AND FUZZY SYSTEM6. ConclusionsIn this paper a general model structure is presented for solving of linear equations (systems) with fuzzy variables andparameters. With this structure, a fuzzy system with a matrix Ais transformed into a crisp linear system S . The system is then solved with crisp variables and parameters and thesolution vector is either a strong fuzzy solution or a weak fuzzy solution. Solutions of linear fuzzy equations have been well addressed in mathematics. However it is still a long distance to arrive in engineering field for applications. This study has attempted to bridge the two domains. Additionally fuzzy analogy of nonlinear systems is a new area and will be studied as the expansion of linear systems.References1.Zimmermann, H.J., Fuzzy Set Theory-and Its Applications,3rd Edition, Kluwer Academic Publishers, Dordrecht, 2000.2.Wang, L.X., Adaptive Fuzzy Systems and Control, New Jersey 07632, 1999.3.Klir, G.J., Folger, T.A., Fuzzy Sets, Uncerainty andInformation, Prentice-Hall, Englewood, Cli.s, NJ, 1988.4.Dubois, D., and Prade, H., Fuzzy Sets and Systems: Theory and Applications, Academic Press, New York, 1980.5.Deeba, E., On a fuzzy difference equation. IEEE Trans.Fuzzy Systems 3(3):469-472, 1995.6.Deeba, E., On a fuzzy logistic difference equation.Differential Equations Dynam. Systems 4(2):149-156, 19967.Deschrijver, G., On the relationship between someextensions of fuzzy set theory, Fuzzy Sets and Systems 133:227-235, 2003.8.Friedman, M., Fuzzy Linear Systems, Fuzzy Sets and Systems 96(2): 201-209, 1998.9.Soliman, S.A., Fuzzy linear parameter estimation algorithms:a new formulation, International Journal of Electrical Power & Energy Systems 24(5): 415-420, 200210.Nguyen, H.T., A note on the extension principle for fuzzy sets, J. Math. Anl. 64(2): 369-380, 1978Notes。
- 1、下载文档前请自行甄别文档内容的完整性,平台不提供额外的编辑、内容补充、找答案等附加服务。
- 2、"仅部分预览"的文档,不可在线预览部分如存在完整性等问题,可反馈申请退款(可完整预览的文档不适用该条件!)。
- 3、如文档侵犯您的权益,请联系客服反馈,我们会尽快为您处理(人工客服工作时间:9:00-18:30)。
Robert Full´ er rfuller@ra.abo.fi, http://www.abo.fi/˜rfuller/robert.html
Abstract This study focuses on the problem of stability (with respect to changes of centres of fuzzy parameters) of the solution in Fuzzy Linear Programming (FLP) problems with symmetrical triangular fuzzy numbers and extended operations and inequalities.
i,j
max |bi − bi (δ )| ≤ δ,
i
(11)
Then we get the following FLP problem:
< (bi (δ ), di ), i = 0, . . . , m. (ai1 (δ ), α)x1 + · · · + (ain (δ ), α)xn ∼
(12)
< (ai1 , α)x1 + · · · + (ain , α)xn ∼ (bi , di ), i = 0, . . . , m.
(6)
(7)
We denote by µi (x) the degree of satisfaction of the i-th restriction at the point x ∈ I Rn in (7), i.e. µi (x) = υ (˜ ai1 x1 + · · · + a ˜in xn , ˜ bi ). Then the solution of the FLP problem (7) is n defined as a fuzzy set on I R whose membership function is given by µ(x) = min µi (x)
3
Stability of the solution for the FLP problem
a0 , x → min; subject to Ax ≤ b.
The conventional model of LP can be stated as (5)
In many real-world problems instead of minimization of the objective function (5) it may be sufficient to determine an x such that a01 x1 + · · · + a0n xn ≤ b0 , a11 x1 + · · · + a1n xn ≤ b1 , . . . am1 x1 + · · · + amn xn ≤ bm , where b0 is a predetermined aspiration level. Assume that all parameters in (6) are fuzzy and are described by symmetrical triangular fuzzy numbers. Then the following FLP problem can be obtained by replacing crisp parameters aij , bi with fuzzy numbers (1) a ˜ij = (aij , α), ˜ bi = (bi , di ) respectively and by < replacing crisp relation ≤ with extended relation ∼.
<˜ The degree of satisfaction of the assertion ”a ˜ is less than ˜ b”, which we write a ˜∼ b, is υ (˜ a, ˜ b) defined in [1] as
υ (˜ a, ˜ b) = sup a ˜(x) ∧ ˜ b(y ).
x∈ I Rn
(13)
In the following theorem we establish a stability of the solution of the FLP problem (7). Theorem 3.1. Let µ(x) and µδ (x) be solution of FLP problems (7) and (12) respectively. Then ||µ − µδ ||C = sup |µ(x) − µδ (x)| ≤ δ
1
Let a ˜ = (a, α) and ˜ b = (b, α ) be two fuzzy numbers of the form (1), λ ∈ R. Then it is easily verified from Zadeh’s extension principle that we have a ˜+˜ b = (a + b, α + α ), a ˜−˜ b = (a − b, α + α ), λa ˜ = (λa, |λ|α). (2)
It will be sufficient to show that |µi (x) − µδ i (x)| ≤ δ because from (16) follows (14). Let x ∈ I Rn and i ∈ {0, . . . , m} be arbitrarily fixed. Consider the following cases: (1) µi (x) = µδ i (x). In this case (16) is trivially obtained. (2) 0 < µi (x) < 1 and 0 < µδ i (x) < 1. In this case from (10), (11) we have |µi (x) − µδ i (x)| = |1 − ai (δ ), x − bi (δ ) ai , x − b i − [1 − ]| = α|x|1 + di α|x|1 + di |bi − bi (δ ) + ai (δ ), x − ai , x | ≤ α|x|1 + di |bi − bi (δ ) + | ai (δ ), −ai , x | δ + |ai (δ ) − ai |∞ |x|1 ≤ ≤ α|x|1 + di α|x|1 + di δ + δ |x|1 1 1 1 1 ≤ δ( + ) ≤ δ + , α|x|1 + di α di α d where ai (δ ) = (ai1 (δ ), . . . , ain (δ )) and |ai (δ ) − ai |∞ = maxj |aij (δ ) − aij |. (3) µi (x) = 1 and 0 < µδ i (x) < 1. In this case from (10) we have ai , x ≤ b i . Hence |µi (x) − µδ i (x)| = |1 − [1 − ai (δ ), x − bi (δ ) ]| = α|x|1 + di (17) 1 1 + , ∀x ∈ I Rn , i = 0, . . . , m, α d (16)
x≤y
(3)
This formula uses once more Zadeh’s extension principle. Let a ˜ = (a, α) and b = (b, α ) be two fuzzy numbers of the form (1). Then it can be easily checked that 1 if a ≤ b, a−b (4) υ (˜ a, ˜ b) = if b < a ≤ b + α + α , 1 − α+α 0 if a > b + α + α .
2
Preliminaries
Definition 2.1. A fuzzy set of the real line given by the membership function |a − t| if |a − t| ≤ α, 1− a ˜(t) = α 0 otherwise,
(1)
i=0,... ,m
(8)
2
and the maximizing solution x∗ of the FLP problem (7) satisfies the equation µ(x). µ(x∗ ) = µ∗ = max n
x∈ I R
(9)
From (2) and (4) it follows that the degree of satisfaction of the i-th restriction at the point x∈I Rn in (7) is the following: 1 if ai , x ≤ bi , ai , x − b i µi (x) = (10) otherwise, 1− α | x | + d ) 1 i 0 if ai , x > bi + α|x|1 + di , where |x|1 = |x1 | + · · · + |xn | and ai , x = ai1 x1 + · · · + ain xn , i = 0, 1, . . . , m. Remark 3.1. In the extremal case α = 0 but di > 0 in (10), we get a linear membership function, i.e. Zimmermann’s principle [4]. Assume that instead of exact centers aij , bi in (7) only their approximations aij (δ ), bi (δ ) are known such that max |aij − aij (δ )| ≤ δ,