Gene selection in cancer classification using sparse logistic regression with bayesian regu
生物信息学方法筛选胰腺癌进展相关的核心基因
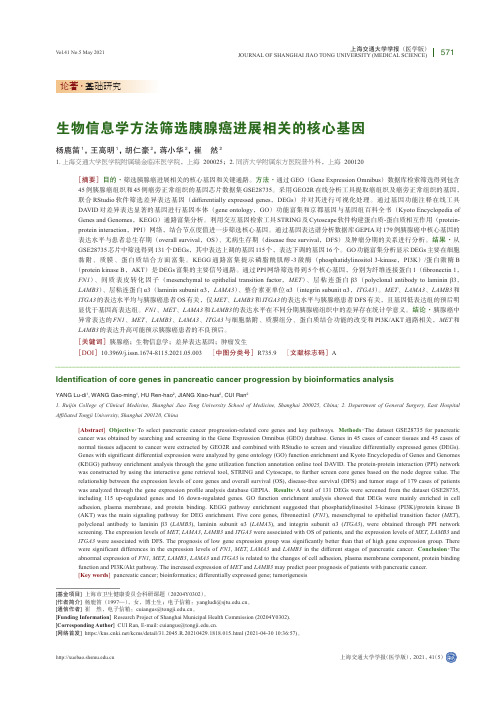
Vol.41No.5May 2021上海交通大学学报(医学版)JOURNAL OF SHANGHAI JIAO TONG UNIVERSITY (MEDICAL SCIENCE)生物信息学方法筛选胰腺癌进展相关的核心基因杨鹿笛1,王高明1,胡仁豪2,蒋小华2,崔然21.上海交通大学医学院附属瑞金临床医学院,上海200025;2.同济大学附属东方医院普外科,上海200120[摘要]目的·筛选胰腺癌进展相关的核心基因和关键通路。
方法·通过GEO (Gene Expression Omnibus )数据库检索筛选得到包含45例胰腺癌组织和45例癌旁正常组织的基因芯片数据集GSE28735。
采用GEO2R 在线分析工具提取癌组织及癌旁正常组织的基因,联合RStudio 软件筛选差异表达基因(differentially expressed genes ,DEGs )并对其进行可视化处理。
通过基因功能注释在线工具DAVID 对差异表达显著的基因进行基因本体(gene ontology ,GO )功能富集和京都基因与基因组百科全书(Kyoto Encyclopedia of Genes and Genomes ,KEGG )通路富集分析。
利用交互基因检索工具STRING 及Cytoscape 软件构建蛋白质-蛋白质相互作用(protein-protein interaction ,PPI )网络,结合节点度值进一步筛选核心基因。
通过基因表达谱分析数据库GEPIA 对179例胰腺癌中核心基因的表达水平与患者总生存期(overall survival ,OS )、无病生存期(disease free survival ,DFS )及肿瘤分期的关系进行分析。
结果·从GSE28735芯片中筛选得到131个DEGs ,其中表达上调的基因115个,表达下调的基因16个。
GO 功能富集分析显示DEGs 主要在细胞黏附、质膜、蛋白质结合方面富集。
非小细胞肺癌患者外周血和肺癌组织中ZIC1启动子甲基化检测的临床意义
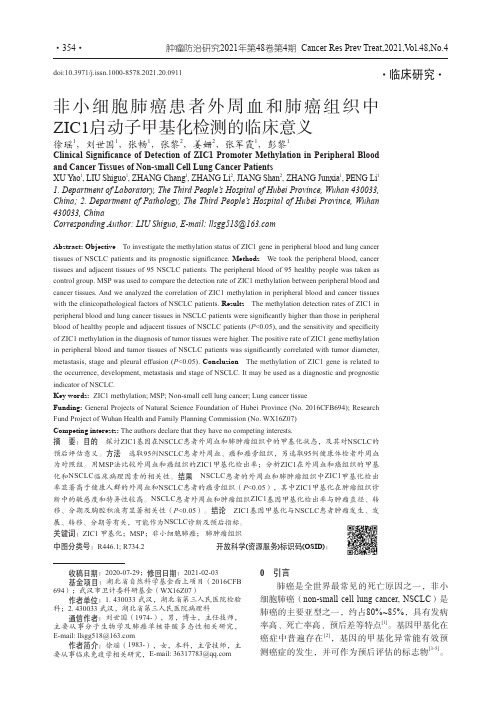
doi:10.3971/j.issn.1000-8578.2021.20.0911·临床研究·非小细胞肺癌患者外周血和肺癌组织中ZIC1启动子甲基化检测的临床意义徐瑶1,刘世国1,张畅1,张黎2,姜姗2,张军霞1,彭黎1Clinical Significance of Detection of ZIC1 Promoter Methylation in Peripheral Bloodand Cancer Tissues of Non-small Cell Lung Cancer Patients XU Yao1, LIU Shiguo1, ZHANG Chang1, ZHANG Li2, JIANG Shan2, ZHANG Junxia1, PENG Li11. Department of Laboratory, The Third People’s Hospital of Hubei Province, Wuhan 430033,China; 2. Department of Pathology, The Third People’s Hospital of Hubei Province, Wuhan430033, ChinaCorrespondingAuthor:LIUShiguo,E-mail:****************Abstract: Objective To investigate the methylation status of ZIC1 gene in peripheral blood and lung cancer tissues of NSCLC patients and its prognostic significance. Methods We took the peripheral blood, cancer tissues and adjacent tissues of 95 NSCLC patients. The peripheral blood of 95 healthy people was taken as control group. MSP was used to compare the detection rate of ZIC1 methylation between peripheral blood and cancer tissues. And we analyzed the correlation of ZIC1 methylation in peripheral blood and cancer tissues with the clinicopathological factors of NSCLC patients. Results The methylation detection rates of ZIC1 in peripheral blood and lung cancer tissues in NSCLC patients were significantly higher than those in peripheralblood of healthy people and adjacent tissues of NSCLC patients (P<0.05), and the sensitivity and specificityof ZIC1 methylation in the diagnosis of tumor tissues were higher. The positive rate of ZIC1 gene methylation in peripheral blood and tumor tissues of NSCLC patients was significantly correlated with tumor diameter,metastasis, stage and pleural effusion (P<0.05). Conclusion The methylation of ZIC1 gene is related to the occurrence, development, metastasis and stage of NSCLC. It may be used as a diagnostic and prognostic indicator of NSCLC.Key words: ZIC1 methylation; MSP; Non-small cell lung cancer; Lung cancer tissueFunding: General Projects of Natural Science Foundation of Hubei Province (No. 2016CFB694); Research Fund Project of Wuhan Health and Family Planning Commission (No. WX16Z07)Competing interests: The authors declare that they have no competing interests.摘 要:目的 探讨ZIC1基因在NSCLC患者外周血和肺肿瘤组织中的甲基化状态,及其对NSCLC的预后评估意义。
基于随机森林相似度矩阵差异性的特征选择

=
1 m-
1 i =1
( Pi
-
P) ,
(2)
式中 P 是 m 个 Pro x 矩阵的均值 , P = ( 1/ m) ·
m
∑| Pi | .
i=1
利用统计量 d 在 iris , zoo , SAheart , derma2 tology 4 个 U CI 标准数据库上 ,对全部样本生成 的 Pro x 矩阵的鲁棒性进行试验 ,试验结果如图 1 所示.
周绮凤 洪文财 杨 帆 罗林开
(厦门大学 自动化系 , 福建 厦门 361005)
摘要 : 将随机森林的相似度矩阵看做一种特殊的核度量 ,利用该度量对模型参数的鲁棒性和特征变化的敏感 性 ,提出一种特征选择的方法. 采用相似度矩阵 ,计算训练样本类内和类间相似性比率. 再利用特征值随机置 换技术 ,将相似性比率的变化量作为特征重要性度量指标 ,从而对所有特征进行排序. 试验结果表明 ,该方法 能充分利用全部样本的信息 ,有效地进行特征选择 ,且其性能优于基于袋外数据误差率估计的特征选择方法. 关 键 词 : 特征选择 ; 度量 ; 差异性 ; 相似度矩阵 ; 随机森林 ; 随机置换 中图分类号 : TP391 文献标识码 : A 文章编号 : 167124512 (2010) 0420058204
第4期
周绮凤等 : 基于随机森林相似度矩阵差异性的特征选择
·59 ·
分析与实验比较 ,验证了该方法能有效地对特征 进行排序 ,从而选出合适的特征样本相似性度量 随机森林在建模的同时 ,还提供了样本相似
性度量 ,即相似度矩阵 (简记为 Pro x 矩阵) . 当用 一棵树对所有数据进行判别时 ,这些数据最终都 将达到该树的某个叶节点上. 可以用两个样本在 每棵树的同一个节点上出现的频率大小 ,来衡量 这两个样本之间的相似程度 ,或两个样本属于同 一类的概率大小. Pro x 矩阵生成过程如下 :a. 对 于样本数为 n 的训练集 ,首先生成一个 n ×n 的零 元素矩阵 Pro x ,记为 P = { pij } ; b. 对于任意两个 样本 xi 和 x j ,若它们出现在所建树的同一个叶节 点上 ,则 p′ij = pij + 1 ; c. 重复上述过程直至 m 棵 树全部建好 , 得到相应的矩阵 ; d. 进行归一化处 理 p′ij = pij / m ( i , j = 1 , 2 , …, n) , 得 到 最 后 的 Pro x 矩阵.
癌症基因突变数据挖掘与生物信息学分析

癌症基因突变数据挖掘与生物信息学分析近年来,癌症的发病率持续上升,成为威胁人类健康的主要疾病之一。
癌症的发生往往与基因突变密切相关。
因此,对于癌症基因突变数据的挖掘与生物信息学分析变得尤为重要。
本文将详细探讨如何利用生物信息学的方法分析癌症基因突变数据,为癌症的早期预测和治疗提供理论依据。
首先,为了进行癌症基因突变数据的挖掘,我们需要获取相应的数据集。
目前,公开的癌症基因突变数据库包括COSMIC、TCGA等,这些数据库收集了大量患者样本的基因突变信息。
通过下载已公开的数据集,我们可以进行后续的生物信息学分析。
在数据集准备完毕后,我们可以开始对癌症基因突变数据进行挖掘和分析。
生物信息学分析的第一步是对数据进行预处理。
预处理的目的是去除噪声数据,保留有效的突变信息。
常见的预处理方法包括数据清洗和特征选择。
数据清洗主要涉及到对数据中的缺失值、异常值等进行处理。
对于缺失值,可以选择删除或者填充。
删除缺失值的方法包括删除含有缺失值的行或列,填充缺失值的方法包括平均值、中值或者最近邻值等。
异常值的处理可以采用平滑法或者替换法。
特征选择是生物信息学分析中的关键步骤,其目的是从大量的基因特征中筛选出与癌症发生相关的特征。
特征选择方法包括过滤式方法、包裹式方法和嵌入式方法。
常用的过滤式方法包括方差过滤和相关系数过滤,包裹式方法包括递归特征消除和遗传算法,嵌入式方法包括LASSO和岭回归等。
通过特征选择,我们可以减少数据集的维度,提高分析的效率。
在数据预处理完成后,我们可以进行癌症基因突变数据的挖掘和分析。
常见的分析方法包括聚类分析、关联规则挖掘、决策树和支持向量机等。
聚类分析可以将癌症样本划分为不同的簇,从而识别出不同亚型的癌症。
关联规则挖掘可以找出基因之间的关联性,从而发现潜在的癌症相关基因。
决策树和支持向量机可以建立预测模型,帮助诊断和预测癌症。
这些方法的选择根据数据的特点和问题的要求进行。
除了基本的挖掘和分析方法,还可以引入更复杂的模型和算法进行癌症基因突变数据的分析。
肿瘤细胞致癌基因的筛选及其功能分析

肿瘤细胞致癌基因的筛选及其功能分析肿瘤是人类面临的重大健康问题之一。
而致癌基因的突变与肿瘤的发生密切相关。
为了更好地了解肿瘤的形成机制以及开发有效的治疗方法,对致癌基因的筛选和功能分析变得尤为关键。
首先,致癌基因是什么?致癌基因普遍存在于人类细胞中,它们是维持正常细胞生长和分裂所必需的。
这些基因在正常情况下发挥着重要作用,但当它们发生突变或失去调控时,就会导致细胞的异常增殖和致癌。
目前已经确定了许多致癌基因,如RAS和p53等。
其次,如何筛选致癌基因?为了筛选出致癌基因,通常采用基因组学、转基因模型和细胞系等方法。
其中最常用的方法是测序技术,通过对患有某种癌症的病人的癌细胞和正常细胞进行基因测序,可以发现致癌基因的突变位置和类型。
此外,利用转基因技术将已知致癌基因的异常表达载入小鼠等模型生物中,可以观察到小鼠出现癌症的情况,而分析这些肿瘤组织的基因序列就可以筛选出致癌基因。
最后,致癌基因的功能分析是什么?通过筛选出致癌基因,需要进一步了解它们的功能和对细胞生长和分裂的调控方式。
这项工作依赖于细胞系和转基因模型的构建,包括肿瘤组织、细胞和动物模型的建立。
这些分析方法包括:1. 基因敲除和过度表达:可以通过操纵致癌基因的表达水平来观察对细胞生长和分裂的影响。
2. 基因互作分析:通过物质互作数据库等方法,了解致癌基因与其他调节基因的相互关系,以及与信号通路的互作情况。
3. 进一步性质分析:如对生物化学特性、功能、结构等方面进行深入研究。
致癌基因的筛选和功能分析是了解癌症机制、开发治疗方法的关键一步。
近年来,随着技术手段和分析方法的不断更新和拓展,我们对致癌基因作用的了解也变得更加深入和全面。
可以预想,这些研究成果将推动癌症研究领域的不断发展和进步。
从DNA序列中的突变寻找癌症生物标志物
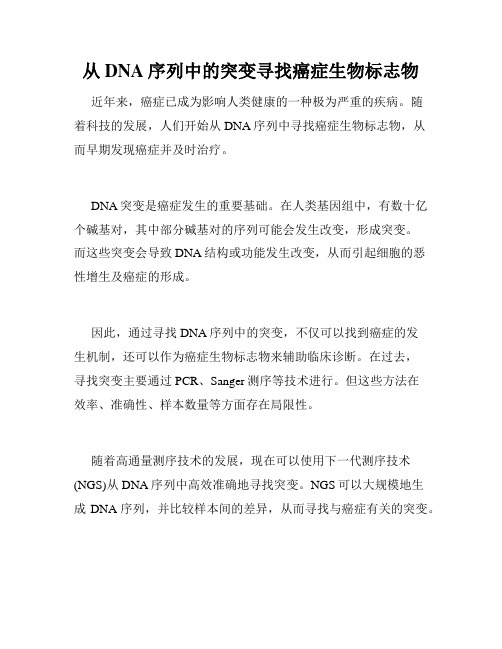
从DNA序列中的突变寻找癌症生物标志物近年来,癌症已成为影响人类健康的一种极为严重的疾病。
随着科技的发展,人们开始从DNA序列中寻找癌症生物标志物,从而早期发现癌症并及时治疗。
DNA突变是癌症发生的重要基础。
在人类基因组中,有数十亿个碱基对,其中部分碱基对的序列可能会发生改变,形成突变。
而这些突变会导致DNA结构或功能发生改变,从而引起细胞的恶性增生及癌症的形成。
因此,通过寻找DNA序列中的突变,不仅可以找到癌症的发生机制,还可以作为癌症生物标志物来辅助临床诊断。
在过去,寻找突变主要通过PCR、Sanger测序等技术进行。
但这些方法在效率、准确性、样本数量等方面存在局限性。
随着高通量测序技术的发展,现在可以使用下一代测序技术(NGS)从DNA序列中高效准确地寻找突变。
NGS可以大规模地生成DNA序列,并比较样本间的差异,从而寻找与癌症有关的突变。
在通过NGS寻找突变的过程中,有两种方法最为常见:全外显子组测序(whole exome sequencing,WES)和全基因组测序(whole genome sequencing,WGS)。
WES仅对编码蛋白的区域进行测序,而WGS则对整个基因组进行测序。
WES技术相对来说更便宜,但是易错因为不对低频突变检测准确,WGS技术覆盖面更广,但是成本较高。
在医学领域,一般先通过WES(全外显子组测序)进行筛查,再通过WGS(全基因组组测序)进行深度分析。
通过全外显子组测序筛查后,通过全基因组测序可以更加准确地找到癌症的相关突变。
与PCR和Sanger测序相比,NGS技术速度更快、准确度更高,并且可以覆盖更多的序列。
而且NGS技术可以同时比较多个样本,对大规模样本的处理非常高效。
这些特点使得它在寻找突变和检测癌症生物标志物方面非常有应用前景。
总之,通过搜索DNA序列中的突变,可以帮助探明癌症的发生、发展机制,从而研发更有效的治疗方法。
随着科技不断创新和发展,NGS技术将会更进一步地推进癌症诊断和治疗的进程,让癌症真正成为“可防可治”的疾病。
基于临床与基因图谱的结肠癌基因标签提取

摘要由于基因间的调控和相互作用表现为“功能基因组合”形式,基因的功能与作用是集体作用的结果,而非单个基因单独作用的结果,表现在分类特征对样本的分类能力方面就是以特征集合的形式整体体现出来的。
根据这个生物学知识,本文考察由多个基因构成的基因簇作为区分正常人和癌症患者的分类因素,利用独立成分分析(ICA)技术对已给出的基因表达采样数据进行分析,最大程度地降低基因之间强烈的相互影响,从而获得对判断是否患有肿瘤或者癌症的最有直接关系但数目较少的潜在因素,即基因簇信息。
随后,我们采用了支持向量机(SVM)依据提取出的潜在因素(基因簇)进行分类,筛选出致病的癌症基因15个。
另外,我们还运用基于灵敏度的支持向量机对基因本身进行分类,而不是基于基因簇。
摘利用得到的结果与基于独立成分分析的方法所提取的基因提供比较。
发现所筛选的基因簇中有三个基因与灵敏度支持向量机方法筛选的基因相同。
对预处理过后的1908个基因,通过独立成分分析提取出61个基因簇,这些基因簇中含有与分类无关的基因簇,即噪声,以及与分类相关的分类因素5个。
事实上,为了能够得到最好的分类因素,我们将问题转化为一类信号稀疏表示的优化问题。
此外,为了进一步进行基因分类,我们利用含噪声的ICA和带松弛因子的非光滑优化模型研究带有噪声的基因图谱信息。
通过含噪声模型与不含噪声模型进行对比,说明含噪模型的优势。
最后,借助于条件概率模型,对病人数据进行了筛选,将临床结论与基因图谱相结合,通过已有文献以及生物信息网站所获取资料发现,所筛选的大部分基因标签与当今临床医学所得到的直肠癌研究结论相吻合。
关键词:含噪基因簇独立成分分析支持向量机非光滑优化模型临床基因标签一、问题的重述癌症起源于正常组织在物理或化学致癌物的诱导下基因组发生的突变,即基因在结构上发生碱基对的组成或排列顺序的改变,因而改变了基因原来的正常分布(即所包含基因的种类和各类基因以该基因转录的mRNA的多少来衡量的表达水平)。
CSE1L与恶性肿瘤的临床研究进展
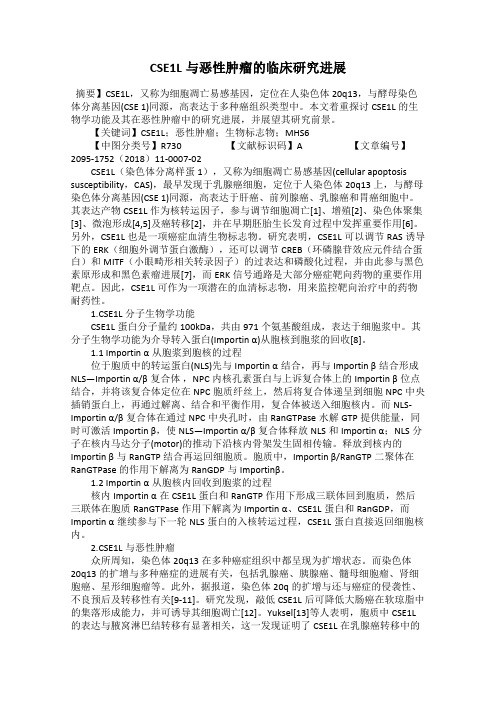
CSE1L与恶性肿瘤的临床研究进展摘要】CSE1L,又称为细胞凋亡易感基因,定位在人染色体20q13,与酵母染色体分离基因(CSE 1)同源,高表达于多种癌组织类型中。
本文着重探讨CSE1L的生物学功能及其在恶性肿瘤中的研究进展,并展望其研究前景。
【关键词】CSE1L;恶性肿瘤;生物标志物;MHS6【中图分类号】R730 【文献标识码】A 【文章编号】2095-1752(2018)11-0007-02CSE1L(染色体分离样蛋1),又称为细胞凋亡易感基因(cellular apoptosis susceptibility,CAS),最早发现于乳腺癌细胞,定位于人染色体20q13上,与酵母染色体分离基因(CSE 1)同源,高表达于肝癌、前列腺癌、乳腺癌和胃癌细胞中。
其表达产物CSE1L作为核转运因子,参与调节细胞凋亡[1]、增殖[2]、染色体聚集[3]、微泡形成[4,5]及癌转移[2],并在早期胚胎生长发育过程中发挥重要作用[6]。
另外,CSE1L也是一项癌症血清生物标志物。
研究表明,CSE1L可以调节RAS诱导下的ERK(细胞外调节蛋白激酶),还可以调节CREB(环磷腺苷效应元件结合蛋白)和MITF(小眼畸形相关转录因子)的过表达和磷酸化过程,并由此参与黑色素原形成和黑色素瘤进展[7],而ERK信号通路是大部分癌症靶向药物的重要作用靶点。
因此,CSE1L可作为一项潜在的血清标志物,用来监控靶向治疗中的药物耐药性。
1.CSE1L分子生物学功能CSE1L蛋白分子量约100kDa,共由971个氨基酸组成,表达于细胞浆中。
其分子生物学功能为介导转入蛋白(Importin α)从胞核到胞浆的回收[8]。
1.1 Importin α从胞浆到胞核的过程位于胞质中的转运蛋白(NLS)先与Importin α结合,再与Importin β结合形成NLS—Importin α/β复合体,NPC内核孔素蛋白与上诉复合体上的Importin β位点结合,并将该复合体定位在NPC胞质纤丝上,然后将复合体递呈到细胞NPC中央插销蛋白上,再通过解离、结合和平衡作用,复合体被送入细胞核内。
- 1、下载文档前请自行甄别文档内容的完整性,平台不提供额外的编辑、内容补充、找答案等附加服务。
- 2、"仅部分预览"的文档,不可在线预览部分如存在完整性等问题,可反馈申请退款(可完整预览的文档不适用该条件!)。
- 3、如文档侵犯您的权益,请联系客服反馈,我们会尽快为您处理(人工客服工作时间:9:00-18:30)。
Vol.22no.192006,pages2348–2355doi:10.1093/bioinformatics/btl386 BIOINFORMATICS ORIGINAL PAPERGene expressionGene selection in cancer classification using sparse logistic regression with Bayesian regularizationGavin C.CawleyÃand Nicola L.C.TalbotSchool of Computing Sciences,University of East Anglia,Norwich NR47TJ,UKReceived on March29,2006;revised on June22,2006;accepted on July6,2006Advance Access publication July14,2006Associate Editor:David RockeABSTRACTMotivation:Gene selection algorithms for cancer classification,based on the expression of a small number of biomarker genes,have been the subject of considerable research in recent years.Shevade and Keerthi propose a gene selection algorithm based on sparse logistic regression(SLogReg)incorporating a Laplace prior to promote sparsity in the model parameters,and provide a simple but efficient training procedure.The degree of sparsity obtained is determined by the value of a regularization parameter,which must be carefully tuned in order to optimize performance.This normally involves a model selec-tion stage,based on a computationally intensive search for the mini-mizer of the cross-validation error.In this paper,we demonstrate that a simple Bayesian approach can be taken to eliminate this regu-larization parameter entirely,by integrating it out analytically using an uninformative Jeffrey’s prior.The improved algorithm(BLogReg)is then typically two or three orders of magnitude faster than the original algorithm,as there is no longer a need for a model selection step.The BLogReg algorithm is also free from selection bias in performance estimation,a common pitfall in the application of machine learning algorithms in cancer classification.Results:The SLogReg,BLogReg and Relevance Vector Machine (RVM)gene selection algorithms are evaluated over the well-studied colon cancer and leukaemia benchmark datasets.The leave-one-out estimates of the probability of test error and cross-entropy of the BLogReg and SLogReg algorithms are very similar,however the BlogReg algorithm is found to be considerably faster than the original SLogReg ing nested cross-validation to avoid selection bias,performance estimation for SLogReg on the leukaemia dataset takes almost48h,whereas the corresponding result for BLogReg is obtained in only1min24s,making BLogReg by far the more practical algorithm.BLogReg also demonstrates better estimates of conditional probability than the RVM,which are of great importance in medical applications,with similar computational expense.Availability:A MATLAB implementation of the sparse logistic regres-sion algorithm with Bayesian regularization(BLogReg)is available from /~gcc/cbl/blogreg/Contact:gcc@1INTRODUCTIONCancer classification based on microarray gene-expression data, ideally identifying a small number of discriminatory biomarker genes,provides one of the earliest applications of machine learning methods in computational biology.A wide variety of machine learning algorithms have been applied to this problem,including the support vector machine(Guyon et al.,2002),sparse logistic regression(SLogReg)(Shevade and Keerthi,2003),the relevance vector machine(RVM)(Li et al.,2002),Gaussian Process models (Chu et al.,2005)and simple decision rules(Tan et al.,2005).The common aims of such algorithms are2-fold:primarily to distinguish between patients suffering from subtly different forms of cancer, with the highest possible degree of accuracy,on the basis of their gene expression profiles obtained by broad-spectrum microarray analysis.The second goal is to identify a small sub-set of biomarker genes,for which expression patterns are highly indicative of a particular form of cancer,and are therefore implicated by asso-ciation.This second goal is concerned with improving our under-standing of the underlying causes of the cancer.In this paper,we propose a substantial improvement to the sparse logistic regression(SLogReg)approach of Shevade and Keerthi (2003).The SLogReg algorithm employs an L1-norm regularization term(Tikhonov and Arsenin,1977),corresponding to a Laplace prior over the model parameters(c.f Williams,1995),in order to identify a sparse sub-set of the most discriminatory features corre-sponding to biomarker genes.Both the generalization ability of the classifier and the level of sparsity achieved are critically depen-dent on the value of a regularization parameter,which must be carefully tuned to optimize performance.This is normally achieved by a computationally intensive search for the minimizer of a cross-validation based estimate of generalization performance.Instead, we adopt a Bayesian approach,in which the regularization parame-ter is integrated out analytically,using an uninformative Jeffery’s prior,in the style of Buntine and Weigend(1991)(see also Lehrach et al.,2006).The resulting parameterless classification algorithm (BLogReg)is very much easier to use,is comparable in perfor-mance with the original sparse logistic regression algorithms,but is two or three orders of magnitude faster,as there is no longer a need for a model selection stage to optimize the regularization parameter. The remainder of this paper is structured as follows:The existing sparse logistic regression(SLogReg)algorithm(Shevade and Keerthi,2003)is reviewed in Section2.The modified Bayesian logistic regression(BLogReg)algorithm is then introduced in Section 3.Experimental results obtained on the well-studied colon cancer(Alon et al.,1999)and leukaemia(Golub et al., 1999)benchmark problems are presented in Section4,demonstrat-ing the competitiveness of the improved algorithm.Finally,the work is summarized and conclusions drawn in Section5.ÃTo whom correspondence should be addressed.2348ÓThe Author2006.Published by Oxford University Press.All rights reserved.For Permissions,please email:journals.permissions@2SPARSE LOGISTIC REGRESSIONWe are commonly faced with statistical pattern recognition prob-lems,where we must learn some decision rule distinguishing between objects belonging to one of two classes,based on a set of‘training examples,D¼fðx i‚y iÞg‘i¼1‚x i2X&R d‚y i2fÀ1‚þ1g‚where x i represents a vector of measurements describing the i-th example,and y i indicates the class to which the i-th example belongs,with y i¼+1representing class C1and y i¼À1representing class C2.Logistic regression is a classical approach to this problem, that attempts to estimate the a-posteriori probability of class mem-bership based on a linear combination of the input features,pðC1j xÞ¼11þexp fÀfðxÞg‚ð1Þwherefðx iÞ¼X dj¼1a j x ijþa0:ð2ÞThe parameters of the logistic regression model, a¼ða0‚a1‚...‚a dÞ,can be found by maximizing the likelihood of the training examples,or equivalently by minimizing the nega-tive log-likelihood.Assuming D represents an independent and identically distributed(i.i.d.)sample from a Bernoulli distribution, the negative log-likelihood is given byE D¼X‘i¼1g fÀy i fðx iÞg‚whereg f j g¼log f1þexpðjÞg:Minimizing the negative log-likelihood is relatively straightfor-ward as thefirst and second derivatives,with respect to individual model parameters,are continuous and easily computed,@E D @a j ¼ÀX‘i¼1exp fÀy i fðx iÞg y i x ij1þexp fÀyfðx iÞgð3Þand@2E D @a j ¼X‘i¼1exp fÀy i fðx iÞg y2i x2ij½1þexp fÀyfðx iÞg:ð4ÞThe resulting model is however fully dense,in the sense that none of the model parameters a are in general exactly zero.Ideally we would prefer a model based on a small selection of the most infor-mative features,with the remaining features being‘pruned’from the model.A sparse model can be introduced by adding a regularization term to the negative log-likelihood(e.g.Williams,1995),corre-sponding to a Laplace prior over a,to give a modified training criterion,M¼E Dþl E a;where E a¼X di¼1j a i jð5Þand l is a regularization parameter,controlling the bias-variance trade-off and simultaneously the sparsity of the resulting model. Note that the usual bias parameter a0is normally left unregularized.At a minima of M,the partial derivatives of M with respect to the model parameters will be uniformly zero,giving@E D@a i¼l if j a i j>0and@E D@a i<l if j a i j¼0:This implies that if the sensitivity of the negative log-likelihood with respect to a model parameter,a i,falls below l,then the value of that parameter will be set exactly to zero and the corresponding input feature can be pruned from the model.The principal short-comings of this approach lie in the training algorithm no longer involving an optimization problem with continuous derivatives and in the need for lengthy cross-validation trials to determine a good value for the regularization parameter l.Shevade and Keerthi (2003)provide a solution to thefirst problem,described in the remainder of this section.This paper proposes a Bayesian solution to the second problem,where the regularization parameter is inte-grated out analytically.2.1An efficient optimization procedureThe training algorithm proposed by Shevade and Keerthi(2003) seeks to minimize the cost function(5)by optimizing one parameter at a time via Newton’s method.However,owing to the discontinuity in thefirst derivative at the origin,care must be exercised when the value of a model parameter passes through zero.This is achieved by bracketing the optimal value for a model parameter,a i,by upper and lower limits(H and L respectively)such that the interval does not include0,except perhaps at a boundary.These limits can be computed using the gradient of M with respect to a i computed at its current value and at zero,from both above and below,as shown in Table1and illustrated by Figure1.A model parameter must be selected for optimization at each iteration,the parameter with the gradient of the greatest magnitude is a sensible choice.In order to improve the speed of convergence, we begin by optimizing only active parameters(those with non-zero values),and only consider inactive parameters if no active parame-ter can be found with a non-zero gradient.Iterative optimization procedures do not generally reduce the gradient exactly to zero,and so in practice we only consider parameters for optimization if they have a gradient exceeding a pre-defined tolerance parameter t. The algorithm terminates when no such parameter can be found. Table1.Special cases that must be considered in optimizing M with respect to a i in order to avoid difficulties due to the discontinuity in the first deriva-tive at the originCase a i@M@a ia i@M@a i0À@M@a i0þL H10—<0<00+1 20—>0>00À1 3<0>0——À1a i 4>0<0——a i+1 5<0<0>0—a i0 6>0>0—<00a i 7<0<0—>00+1 8>0>0<0—À10 9<0<00!000 10>0>00!000Sparse Bayesian gene selection2349For a complete description of the training algorithm,see Shevade and Keerthi (2003).3BAYESIAN REGULARIZATIONIn this section,we demonstrate how the regularization parameter may be eliminated,following the methods of Buntine and Weigend (1991)and Williams (1995),before going on to describe the modi-fication of the training procedure of Shevade and Keerthi (2003)required to accommodate the revised optimization problem.3.1Eliminating the regularization parameter lMinimization of (5)has a straight-forward Bayesian interpretation;the posterior distribution for a ,the parameters of the model given by (1and 2),can be written asp ða jD ‚l Þ/p ðDj a Þp ða j l Þ:M is then,up to an additive constant,the negative logarithm of the posterior density.The prior over model parameters,a ,is then given by a separable Laplace distributionp ða j l Þ¼ l 2 N exp fÀl E a g ¼Y N i ¼1l2exp fÀl j a i jg ‚ð6Þwhere N is the number of active (non-zero)model parameters.Agood value for the regularization parameters l can be estimated,within a Bayesian framework,by maximizing the evidence (MacKay,1992a,b,c)or alternatively it may be integrated out analytically (Buntine and Weigend,1991;Williams,1995).Here we take the latter approach,where the prior distribution over model parameters is given by marginalizing over l ,p ða Þ¼Zp ða j l Þp ðl Þd l :As l is a scale parameter,an appropriate ignorance prior is givenby the improper Jeffrey’s prior,p ðl Þ/1/l ,corresponding to a uniform prior over log l .Substituting Equation (6)and noting that l is strictly positive,p ða Þ¼12N Z 10l N À1exp fÀl E a g d l :Using the Gamma integral,R 10x n À1e Àm x d x ¼G ðn Þm n(Gradshteyn and Ryzhic,1994,equation 3.384),we obtainp ða Þ¼12N G ðN ÞE N a)Àlog p ða Þ/N log E a ‚giving a revised optimization criterion for sparse logistic regression with Bayesian regularization,Q ¼E D þN log E a ‚ð7Þin which the regularization parameter has been eliminated,for fur-ther details and theoretical justification,see Williams (1995).The use of a Laplace prior and Jeffrey’s hyper-prior in sparse supervised learning has also been proposed by Figueiredo (2003),along with an Expectation-Maximization (EM)style training algorithm.Unfortu-nately this training procedure involves solving a system of ‘linear equations,analogous to the normal equations to be solved in linear regression,and so is not suitable for large-scale applications,such as the analysis of microarray data.Fortunately the method of Shevade and Keerthi (2003)can easily be adapted to sparse logistic regres-sion with Bayesian regularization.3.2Minimizing the Bayesian training criterionShevade and Keerthi (2003)demonstrate that the cost function for sparse logistic regression using a Laplace prior can be iteratively minimized in an efficient manner one parameter at a time.Note that the objective function is non-smooth,as the first derivatives exhibit discontinuities at a i ¼0‚8i 2f 1‚2‚...‚N g ,but is otherwise smooth.These properties of the objective function are clearly−20240102030Case #1−4−2020102030Case #2−4−2020102030Case #3−20240102030Case #4-4-2020102030Case #5−20240102030Case #6−20240102030Case #7−4−2020102030Case #8−20240102030Case #9−4−2020102030Case #10Fig.1.Graphical depiction of the special cases,listed in full in Table 1,to be considered in optimizing a model parameter in order to deal with the discontinuity in the first derivative at the origin.Note that even and odd numbered cases differ only by reflection.G.C.Cawley and L.C.Talbot2350evident from thefirst and second derivatives,@ @a i log E a¼a ij a i j1E aand@2@a2ilog E a¼À1E2a:The training criterion incorporating a fully Bayesian regulariza-tion term can be minimized via a simple modification of the existing training algorithm for sparse logistic regression.Differentiating the original and modified training criteria(5,7),we have thatr M¼r E Dþl r E a and r Q¼r E Dþ~l r E a,where1 ~l ¼1NX Ni¼1j a i j:ð8ÞFrom a gradient descent perspective,Minimizing Q effectively becomes equivalent to minimizing M,assuming that the regular-ization parameter,l,is continuously updated according to(8) following every change in the vector of model parameters,a (Williams,1995).This requires only a very minor modification of the code implementing the sparse logistic regression algorithm, whilst eliminating the only training parameter and hence the need for a model selection procedure infitting the model.3.3Relationship with the evidence frameworkIt has been observed that the‘integrate-out’approach to deal with the regularization parameter(Buntine and Weigend,1991)is likely to lead to over-regularized models that under-fit the data,for neural network models with a traditional Gaussian weight-decay prior,and that evidence framework(MacKey,1992a,b,c)is generally to be preferred(MacKay,1994).However,it is relatively straight forward to show that,in the case of the Laplace prior,the iterative update formula for the effective regularization parameter(8)is identical to the update formula for the regularization parameter under the evi-dence framework(Williams,1995).4RESULTSIn this section,we evaluate the performance of the proposed logistic regression method with Bayesian regularization using a Laplace prior against the sparse logistic regression method of Shevade and Keerthi(2003),on which it is based,and the RVM (Tipping,2001),which represents the most direct competing approach.The performance of all three classifiers are evaluated over two commonly used benchmark datasets:the colon cancer dataset,introduced by Alon et al.(1999)and the leukaemia dataset introduced by Golub et al.(1999).Sections4.1–4.3outline key aspects of the experimental methodology,the experimental results are given in Sections4.4and4.5.4.1Performance evaluationCross-validation(Stone,1974)is a commonly used procedure for evaluating the quality of statistical models.In k-fold cross-validations,the available data are partitioned into k disjoint sub-sets of approximately equal size.A set of k classifiers is then constructed;each classifier is trained on a different combina-tion of kÀ1sub-sets and tested on the remaining sub-set.The average test performance of the k classifiers generally provides a good estimate of the generalization performance of a single classifier trained on the entire dataset.Cross-validation is especially attractive in applications with relatively limited amounts of data as all observations are used as both training and test data.The most extreme form of cross-validation,where each partition contains a single pattern,is known as leave-one-out cross-validation,and has been shown to provide an almost unbiased estimate of the true test error(Luntz and Brailovsky,1969).Leave-one-out cross-validation is rarely used in performance evaluation owing its high computa-tional expense,but also because is has been observed to exhibit a higher variance than conventional k-fold cross-validation(Kohavi, 1995).However,if the amount of available data are severely lim-ited,leave-one-out cross-validation becomes the more attractive option,and not only because the computational expense involved becomes less of an issue.In these circumstances,k-fold cross-validation can also exhibit a high variance because more data are held out for testing in each fold,and the classifiers may then have too little data to form a stable decision rule(i.e.a small change in the training data may lead to a significant change in the decision rule). The estimator then becomes sensitive to issues such as the parti-tioning of the data.In microarray analysis,we typically have only a few tens or hundreds of training patterns with a few thousand fea-tures.We therefore use leave-one-out cross-validation as it is likely to provide a more reliable indicator of generalization performance thanfive-or ten-fold cross-validation.4.2The relevance vector machineThe RVM is included in the evaluation as it implements a logistic regression model,where the amount of regularization applied and the degree of sparsity obtained are also governed within a Bayesian framework,rather than by an explicit parameter which must be tuned by the user.The algorithm therefore generates a model of the same form as the proposed method,also without the need for a model selection stage to choose good settings for any hyper-parameters.The RVM,however,is based on a separable Gaussian prior over the model parameters,with a distinct regularization parameter for each weight.The regularization parameters are adjusted so as to maximize the marginal likelihood of the model, which tends to force the values of redundant weights strongly towards zero,so that they can be identified and pruned from the model,via a process known as automatic relevance determination (ARD).The implementation used here is based on the fast marginal likelihood approach described by Faul and Tippline(2002,2003), however rather than re-fitting the Laplace approximation after each update of a regularization,it is only updated when a significant increase in the marginal likelihood is no longer possible via updates of the regularization parameters.This strategy was found to be considerably faster in practice.4.3Model selection for sparse logistic regressionThe existing sparse logistic regression model of Shevade and Keerthi(2003)includes a regularization parameter,controlling the complexity of the model and the sparsity of the model para-meters,which must be chosen by the user or alternatively optimized in an additional model selection stage.In this study,the value of this parameter is found via a(computationally expensive)minimization of the leave-one-out cross-validation estimate of the cross-entropy loss.Again,leave-one-out cross-validation is appropriate as the amount of training data is severely limited.However,we cannot use the same leave-one-out cross-validation estimate for both modelSparse Bayesian gene selection2351selection and performance evaluation as this would introduce a (possibly quite strong)selection bias in favour of the existing sparse logistic regression model.A nested leave-one-out cross-validation procedure is therefore used instead.Leave-one-out cross-validation is used for performance evaluation in the‘outer loop’of the pro-cedure,in each iteration of which model selection is performed individually for each classifier based on a separate leave-one-out cross-validation procedure.Obviously this is computationally expensive,but provides an almost unbiased assessment of general-ization performance as well as a sensible automatic method of setting the value of the regularization parameter.4.4Results on the colon cancer datasetThe colon cancer dataset(Alon et al.,1999)describes the expres-sion of2000genes in40cancer and22normal tissue samples,the aim being to construct a classifier capable of distinguishing between cancer and normal tissues.Table2shows the leave-one-out cross-validation estimate of the cross-entropy and error rate for the RVM, SLogReg and BlogReg algorithms over the colon dataset.All three classifiers achieve the same error rate of17.7%.The cross-entropy provides a more refined indicator of the discriminative ability of a classifier,and in this case shows that the SLogReg and BLogReg algorithms clearly outperform the RVM,but that the difference in performance between the SLogReg and BLogReg algorithms is minimal.The higher cross-entropy of the RVM is however offset by the use of a much smaller sub-set of the available features. Figure2shows the frequency of selection and the mean weight for each feature comprising the colon dataset for RVM,SLogReg and BlogReg algorithms,averaged over1000bootstrap realizations of the data.Note that in each case,a small sub-set of features are selected on a regular basis with significant weights,however the sub-sets of features chosen in each iteration exhibit substantial variation.The BLogReg algorithm is marginally more expensive than the RVM,each fold of the leave-one-out cross-validation pro-cedure taking on average1.03and0.84s respectively.The SLogReg algorithm is very much more expensive,owing to the need for a model selection stage to choose a good value for the regularization parameter,l,with each fold of the leave-one-out cross-validation procedure taking$317s.The choice of algorithm is then dependent on whether sparsity or predictive power(as measured by the cross-entropy)is most important.Given the minimal difference in performance and substantial difference in computational expense there is little reason to prefer the SLogReg over the BLogReg algorithm.4.5Results on the leukaemia datasetThe aim of the leukaemia benchmark(Golub et al.,1999)is to form a decision rule capable of distinguishing between acute myeloid leukaemia(AML)and acute lymphoblastic leukaemia(ALL).The data describe the expression of7128genes in47ALL samples and 25AML samples.The original benchmark partitions the data into training and test sets,however as leave-one-out cross-validation is used in this study,owing to the small size of the dataset,we have neglected this division.Again the leave-one-out cross-validation error rates are very similar,with the original SLogReg model gen-erating four leave-one-out errors and the BLogReg and RVM mod-els both generatingfive.The RVM and SLogReg models both produce very sparse models,however the BLogReg model still uses on average only11.59of the7128features(only0.16%). However,the additional features used by the BLogReg model do provide additional discriminatory power,as the BLogReg model achieves the lowest cross-entropy score.The BlogReg model is slightly faster than the RVM model,in this case each fold of the leave-one-out cross-validation process taking an average of 1.16s for the BlogReg model and 2.78s for the RVM model.The BLogReg model is however more than three orders of magnitude faster than the existing SLogReg model, which requires an average of2392.2s,owing to the model selection stage required to optimize the value of the regularization parameter (Fig.3and Table3).4.6DiscussionIt is interesting to compare the BLogReg and RVM approaches from a theoretical perspective as BLogReg,which integrates out the hyper-parameters and subsequently optimizes the parameters implements a strategy that is diametrically opposed to that of the RVM,which integrates over the model parameters and optimizes the hyper-parameters.The proper Bayesian approach to dealing with hyper-parameters seeks to define a suitable hyper-prior and integrate them out analytically(Buntine and Weigend,1991; Willams,1995),or via Markov Chain Monte Carlo(MCMC)meth-ods(Williams and Rasmussen,1996).On the other hand,MacKay (1999)notes that,at least in the case of a Gaussian prior,optimizing the hyper-parameters under the evidence framework is often pre-ferable.In the presence of many ill-defined parameters,the integrate-out approach often leads to over-regularization of the model,also the skewness of the posterior means that the usual Laplace approximation is not representative of the volume of the true posterior under the integrate-out approach.However,in the case of the Laplace prior,the pruning action of the regularizer eliminates any ill-determined parameters,and so the model will not generally be overly regularized.Also the model being sparse and composed solely of well-determined parameters,the posterior is likely to be comparatively compact,and so the Laplace approxima-tion under the Laplace prior can be expected to be relatively accu-rate.Indeed,the integrate-out and optimization approaches have been shown to be equivalent in the case of the Laplace prior (Williams,1995).The theoretical justification for the BLogReg algorithm is thus at least as sound as that of the RVM.The BLogReg and RVM have also been evaluated for the task of discriminative detection of regulatory elements(Cawley et al.2006a),where the BLogReg algorithm generally out-performed the RVM,although the difference in performance was relatively slight.Table2.Leave-one-out cross-validation estimate of the cross-entropy and error rate for RVM,SLogReg and BLogReg algorithms on the colon bench-mark and a bootstrap estimate of the average number of features used. Algorithm Cross-entropy Error rate#FeaturesRVM0.567±0.1780.177±0.049 5.60±0.040 SLogReg0.506±0.0940.177±0.04915.54±0.103 BLogReg0.510±0.0980.177±0.04911.74±0.033All averages are given with the associated standard error of the mean.G.C.Cawley and L.C.Talbot2352。