[2010]Cross-media Retrieval Based on CSRN Clustering
基于十字注意力机制改进U-Transformer_的新冠肺炎影像分割
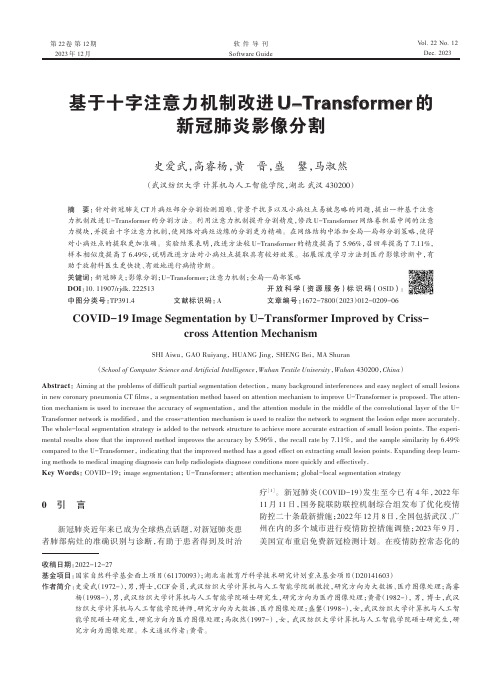
第 22卷第 12期2023年 12月Vol.22 No.12Dec.2023软件导刊Software Guide基于十字注意力机制改进U-Transformer的新冠肺炎影像分割史爱武,高睿杨,黄晋,盛鐾,马淑然(武汉纺织大学计算机与人工智能学院,湖北武汉 430200)摘要:针对新冠肺炎CT片病灶部分分割检测困难、背景干扰多以及小病灶点易被忽略的问题,提出一种基于注意力机制改进U-Transformer的分割方法。
利用注意力机制提升分割精度,修改U-Transformer网络卷积层中间的注意力模块,并提出十字注意力机制,使网络对病灶边缘的分割更为精确。
在网络结构中添加全局—局部分割策略,使得对小病灶点的提取更加准确。
实验结果表明,改进方法较U-Transformer的精度提高了5.96%,召回率提高了7.11%,样本相似度提高了6.49%,说明改进方法对小病灶点提取具有较好效果。
拓展深度学习方法到医疗影像诊断中,有助于放射科医生更快捷、有效地进行病情诊断。
关键词:新冠肺炎;影像分割;U-Transformer;注意力机制;全局—局部策略DOI:10.11907/rjdk.222513开放科学(资源服务)标识码(OSID):中图分类号:TP391.4 文献标识码:A文章编号:1672-7800(2023)012-0209-06COVID-19 Image Segmentation by U-Transformer Improved by Criss-cross Attention MechanismSHI Aiwu, GAO Ruiyang, HUANG Jing, SHENG Bei, MA Shuran(School of Computer Science and Artificial Intelligence,Wuhan Textile University,Wuhan 430200,China)Abstract:Aiming at the problems of difficult partial segmentation detection, many background interferences and easy neglect of small lesions in new coronary pneumonia CT films, a segmentation method based on attention mechanism to improve U-Transformer is proposed. The atten‐tion mechanism is used to increase the accuracy of segmentation, and the attention module in the middle of the convolutional layer of the U-Transformer network is modified, and the cross-attention mechanism is used to realize the network to segment the lesion edge more accurately. The whole-local segmentation strategy is added to the network structure to achieve more accurate extraction of small lesion points. The experi‐mental results show that the improved method improves the accuracy by 5.96%, the recall rate by 7.11%, and the sample similarity by 6.49% compared to the U-Transformer, indicating that the improved method has a good effect on extracting small lesion points. Expanding deep learn‐ing methods to medical imaging diagnosis can help radiologists diagnose conditions more quickly and effectively.Key Words:COVID-19; image segmentation; U-Transformer; attention mechanism; global-local segmentation strategy0 引 言新冠肺炎近年来已成为全球热点话题,对新冠肺炎患者肺部病灶的准确识别与诊断,有助于患者得到及时治疗[1]。
西氏公司丁基胶塞介绍材料
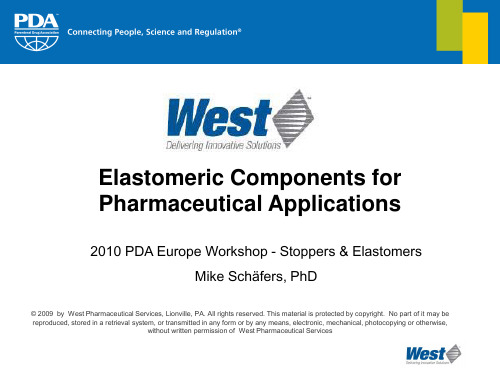
Final Inspection
Mixing control
Visual, dimensional Inspection
Compounding
Molding
Inspection Of Trimming Edge
Final Inspection List of Defects
Trimming
Final Treatment
Manufacturing Process
Raw Materials and Auxiliaries Weighing Mixing Dimensioning
Incoming Inspection
Overview of the rubber production process
Molding B2-Coating* Trimming Washing/Siliconization Automated Vision Inspection* Packaging Sterilization* Shipping
Lab Testing ( chemical)
Chemical/Physical Tests Acc. EP Other Pharmacopoeias Such As USP, JP Silicone Oil-Testing Functional Tests e.g. ISO 8536, Part 1, POF, etc. Pyrolysis - IR
Specification Limit: ≤ 5 CFUs per 100 cm2 of closure surface area
Proved Clean Index (PCI)
Rinse of ´Ready to Sterilize´ closures with appropriate solution, followed by filtration and counting of particles on filter Quantifies visible particulate into size ranges 25µ - 50µ; 51µ - 100µ; > 100µ Index calculated with larger particles having higher weight Does NOT count silicone particles
融合多尺度通道注意力的开放词汇语义分割模型SAN
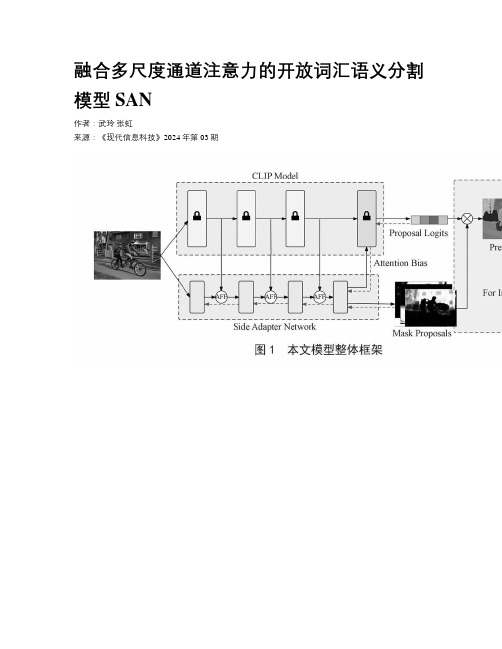
融合多尺度通道注意力的开放词汇语义分割模型SAN作者:武玲张虹来源:《现代信息科技》2024年第03期收稿日期:2023-11-29基金项目:太原师范学院研究生教育教学改革研究课题(SYYJSJG-2154)DOI:10.19850/ki.2096-4706.2024.03.035摘要:随着视觉语言模型的发展,开放词汇方法在识别带注释的标签空间之外的类别方面具有广泛应用。
相比于弱监督和零样本方法,开放词汇方法被证明更加通用和有效。
文章研究的目标是改进面向开放词汇分割的轻量化模型SAN,即引入基于多尺度通道注意力的特征融合机制AFF来改进该模型,并改进原始SAN结构中的双分支特征融合方法。
然后在多个语义分割基准上评估了该改进算法,结果显示在几乎不改变参数量的情况下,模型表现有所提升。
这一改进方案有助于简化未来开放词汇语义分割的研究。
关键词:开放词汇;语义分割;SAN;CLIP;多尺度通道注意力中图分类号:TP391.4;TP18 文献标识码:A 文章编号:2096-4706(2024)03-0164-06An Open Vocabulary Semantic Segmentation Model SAN Integrating Multi Scale Channel AttentionWU Ling, ZHANG Hong(Taiyuan Normal University, Jinzhong 030619, China)Abstract: With the development of visual language models, open vocabulary methods have been widely used in identifying categories outside the annotated label. Compared with the weakly supervised and zero sample method, the open vocabulary method is proved to be more versatile and effective. The goal of this study is to improve the lightweight model SAN for open vocabularysegmentation, which introduces a feature fusion mechanism AFF based on multi scale channel attention to improve the model, and improve the dual branch feature fusion method in the original SAN structure. Then, the improved algorithm is evaluated based on multiple semantic segmentation benchmarks, and the results show that the model performance has certain improvement with almost no change in the number of parameters. This improvement plan will help simplify future research on open vocabulary semantic segmentation.Keywords: open vocabulary; semantic segmentation; SAN; CLIP; multi scale channel attention 0 引言識别和分割任何类别的视觉元素是图像语义分割的追求。
海康威视产品说明书:无接触体温检测与戴口罩检测系统

Four adjustable lenses in one camera cover up to a 360° field of view, ensuring there are no monitoring blindspots. The monitoring tilt angle can also be adjusted.
Analysis
HikCentral
Trip & Fall Incidents
Loitering
Violent Motion
Guards check situation
Reception - Facial recognition & monitoring abnormal temperature.
NEED TO RECOGNIZE A VIP FROM YOUR BRANCH?
O ce & Customer Service Area - Indoor Panoramic Monitoring.
CAPTURE 360° IMAGES
MINI PANOVU CAMERAS
Hikvision’s PanoVu DS-2PT3326IZ-DE3 PanoVu Mini-Series Network PTZ camera, with integrated panoramic and PTZ cameras, is able to capture 360° images with its panoramic cameras, as well as detailed close-up images with the PTZ camera.
基于视觉特征的跨域图像检索算法的研究

摘要摘要随着成像传感器性能的不断发展和类型的不断丰富,同一事物来自不同成像载体、不同成像光谱、不同成像条件的跨域图像也日益增多。
为了高效地利用这些数字资源,人们往往需要综合多种不同的成像传感器以获得更加全面丰富的信息。
跨域图像检索就是研究如何在不同视觉域图像进行检索,相关课题已经成为当今计算机视觉领域的研究热点之一,并在很多领域都有广泛的应用,例如:异质图像配准与融合、视觉定位与导航、场景分类等。
因此,深入研究跨域图像之间的检索问题具有重要的理论意义和应用价值。
本文详细介绍了跨域图像检索的研究现状,深入分析了不同视觉域图像之间的内在联系,重点研究了跨域视觉显著性检测、跨域特征的提取与描述、跨域图像相似度度量这三个关键问题,并实现了基于显著性检测的跨域视觉检索方法、基于视觉词汇翻译器的跨域图像检索方法和基于共生特征的跨域图像检索方法。
论文的主要研究工作如下:(1)分析了跨域图像的视觉显著性,提出了一种基于显著性检测的跨域视觉检索方法。
该方法首先利用图像超像素区域的边界连接值,赋予各个区域不同的显著性值,获得主体目标区域;然后通过线性分类器进一步优化跨域特征,并对数据库图像进行多尺度处理;最后计算图像间的相似度,返回相似度得分最高的图像作为检索结果。
该方法有效地降低了背景等无关区域的干扰,提高了检索准确率。
(2)针对跨域图像特征差异较大无法直接进行匹配的问题,提出了一种基于视觉词汇翻译器的跨域图像检索方法。
该方法受语言翻译机制的启发,利用视觉词汇翻译器,建立了不同视觉域之间的联系。
该翻译器主要包含两部分:一是视觉词汇树,它可以看作是每个视觉域的字典;二是从属词汇树叶节点的索引文件,其中保存了不同视觉域间的翻译关系。
通过视觉词汇翻译器,跨域检索问题被转化为同域检索问题,从新的角度实现了跨视觉域间的图像检索。
实验验证了算法的性能。
(3)利用不同视觉域间的跨域共生相关性,提出了一种基于视觉共生特征的跨域图像检索方法。
移动互联网跨媒体信息检索技术
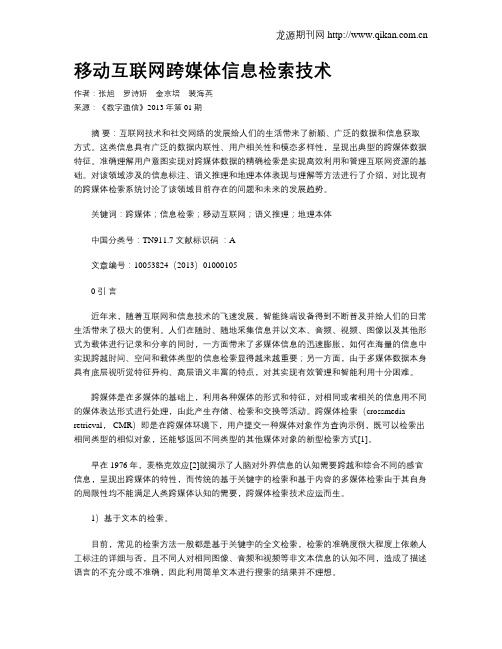
移动互联网跨媒体信息检索技术作者:张旭罗诗妍金京培裴海英来源:《数字通信》2013年第01期摘要:互联网技术和社交网络的发展给人们的生活带来了新颖、广泛的数据和信息获取方式。
这类信息具有广泛的数据内联性、用户相关性和模态多样性,呈现出典型的跨媒体数据特征。
准确理解用户意图实现对跨媒体数据的精确检索是实现高效利用和管理互联网资源的基础。
对该领域涉及的信息标注、语义推理和地理本体表现与理解等方法进行了介绍,对比现有的跨媒体检索系统讨论了该领域目前存在的问题和未来的发展趋势。
关键词:跨媒体;信息检索;移动互联网;语义推理;地理本体中国分类号:TN911.7 文献标识码:A文章编号:10053824(2013)010001050 引言近年来,随着互联网和信息技术的飞速发展,智能终端设备得到不断普及并给人们的日常生活带来了极大的便利。
人们在随时、随地采集信息并以文本、音频、视频、图像以及其他形式为载体进行记录和分享的同时,一方面带来了多媒体信息的迅速膨胀,如何在海量的信息中实现跨越时间、空间和载体类型的信息检索显得越来越重要;另一方面,由于多媒体数据本身具有底层视听觉特征异构、高层语义丰富的特点,对其实现有效管理和智能利用十分困难。
跨媒体是在多媒体的基础上,利用各种媒体的形式和特征,对相同或者相关的信息用不同的媒体表达形式进行处理,由此产生存储、检索和交换等活动。
跨媒体检索(crossmedia retrieval, CMR)即是在跨媒体环境下,用户提交一种媒体对象作为查询示例,既可以检索出相同类型的相似对象,还能够返回不同类型的其他媒体对象的新型检索方式[1]。
早在1976年,麦格克效应[2]就揭示了人脑对外界信息的认知需要跨越和综合不同的感官信息,呈现出跨媒体的特性,而传统的基于关键字的检索和基于内容的多媒体检索由于其自身的局限性均不能满足人类跨媒体认知的需要,跨媒体检索技术应运而生。
1)基于文本的检索。
cross-modal retrieval 指标

《探究跨模态检索指标》跨模态检索(cross-modal retrieval)是指在不同的数据模态之间进行相关内容的搜索和检索。
在信息检索领域,跨模态检索已经成为一个热门的话题,因为我们现在可以访问到各种类型的数据,比如文本、图像、视频和音频等。
针对这个主题,我们将首先从跨模态检索的定义开始,逐步深入探讨其相关指标及重要性。
1. 跨模态检索的定义跨模态检索是指在不同的模态之间进行信息检索和相似性匹配的过程。
在现实场景中,我们可能会面对不同类型的数据,比如从视觉图像到文本描述,或者从音频到视频内容。
跨模态检索的目标是在这些不同的数据模态之间建立联系,实现信息的交叉检索和匹配。
2. 跨模态检索指标的种类在跨模态检索任务中,有许多不同的指标可以用来评价检索结果的质量。
常见的指标包括但不限于以下几种:- 结构化相似度指标:用于衡量不同数据模态之间的结构化相似性,比如文本与图像之间的关联程度。
- 语义相关性指标:通过自然语言处理技术来度量文本和其他模态数据之间的语义相关性,比如使用词向量或者语义表示模型来衡量语义相似度。
- 图像相似度指标:针对图像模态数据,可以使用各种图像特征提取和相似度度量方法来评估图像之间的相似程度。
- 融合指标:结合多个模态数据的特征来计算综合的相似性指标,以更全面地评估跨模态检索结果的质量。
3. 指标选择的重要性在进行跨模态检索任务时,选择合适的指标非常重要。
不同的任务和应用场景可能需要不同的指标来评价检索结果。
比如在文本与图像的关联检索中,我们更关注语义相关性指标;而在音频与视频内容的匹配中,可能更关注结构化相似度指标。
在实际应用中,我们需要根据具体的任务需求来选择合适的指标进行评价。
4. 个人观点和理解在我看来,跨模态检索是一个非常具有挑战性和前沿的研究领域。
随着多模态数据的不断涌现,跨模态检索技术将对信息检索和智能推荐等领域产生重大影响。
在未来,我期待能够看到更多深度学习和多模态融合的技术应用于跨模态检索任务中,以提升检索结果的质量和效率。
参考文献

[1]卢汉清,刘静.基于图学习的自动图像标注[J]. 计算机学报,2008,31(9):1630-1645.[2]李志欣,施智平,李志清,史忠植.融合语义主题的图像自动标注[J].软件学报,2011,22(4):801-812.[3]Minyi Ke, Shuaihao Li, Yong Sun, Shengjun Cao . Research on similarity comparison by quantifying grey histogram based on multi-feature in CBIR [J]// Proceedings of the 3th International Conference on Education Technology and Training .IEEE Press.2010:422-424.[4] Wang Ke-Gang, Qi Li-Ying. Classification and Application of Images Based on Color Texture Feature[C]// Proceedings of 4th IEEE International Conference on Computer Science and Information Technology .IEEE Press. 2011:284-290.[5]Mohamed Maher Ben Ismail. Image Database Categorization based on a Novel Possibilistic Clustering and Feature Weighting Algorithm[C] // Proceedings of 2012 International Conference on Network and Computational Intelligence. 2012:122-127.[6]Du Gen-yuana, Miao Fang, Tian Sheng-li, Liu Ye.A modified fuzzy C-means algorithm in remote sensing image segmentation[C]// Proceedings of Environmental Science and Information Application Technology. 2009: 447-450.[7]Jeon J., Lavrenko V., Manmatha R. Automatic image annotation and retrieval using cross- media relevance models[C]// ACM SIGIR.ACM Press,2003:119- 126.[8]苗晓光,袁平波,何芳,俞能海. 一种新颖的自动图像标注方法[C].// 第十三届中国图象图形学术会议.2006:581-584参考文献正解[1] Smeulders A W M, Worring M, Santini S, et al. Content-based image retrieval at the end ofthe early years[J]. Pattern Analysis and Machine Intelligence, IEEE Transactions on,22(12),2000: 1349-1380.[2] Datta R, Joshi D, Li J, et al. Image retrieval: ideas, influences, and trends of the new age[J].ACM Computing Surveys (CSUR),40(2),2008: 5.[3] Mller H, Mller W, Squire D M G, et al. Performance evaluation in content-based imageretrieval: overview and proposals[J]. Pattern Recognition Letters,22(5),2001: 593-601.[4]Müller H, SO H E S. Text-based (image) retrieval[J]. HES SO//Valais, Sierre, Switzerland[Online] http://thomas. deselaers. de/teaching/files/tutorial_icpr08/03text Based Retrieval. pdf [Accessed 25 July 2010], 2007.[5]Zhao R, Grosky W I. Narrowing the semantic gap-improved text-based web document retrievalusing visual features[J]. Multimedia, IEEE Transactions on, 2002, 4(2): 189-200.[6]卢汉清,刘静.基于图学习的自动图像标注[J]. 计算机学报,2008,31(9):1630-1645.[7]李志欣,施智平,李志清.史忠植.融合语义主题的图像自动标注[J].软件学报,2011,22(4):801-812.[8] Li J, Wang JZ. Automatic linguistic indexing of pictures by a statistical modeling approach.IEEE Trans. on Pattern Analysis and Machine Intelligence, 2003,25(9):1075−1088. [doi:10.1109/TPAMI.2003.1227984][9] Chang E, Goh K, Sychay G, Wu G. CBSA: Content-Based soft annotation for multimodalimage retrieval using Bayes point machines. IEEE Trans. on Circuits and Systems for Video Technolo gy, 2003,13(1):26− 38. [doi: 10.1109/TCSVT.2002.808079][10]Carneiro G, Chan AB, Moreno PJ, Vasconcelos N. Supervised learning of semantic classes forimage annotation and retrieval. IEEE Trans. on Pattern Analysis and Machine Intelligence, 2007,29(3):394 − 410. [doi: 10.1109/TPAMI.2007.61][11]Blei DM, Jordan MI. Modeling annotated data. In: Proc. of the 26th Int’l ACM SIGIR Conf.on Research and Development in Information Retrieval. New York: ACM Press, 2003. 127− 134. [doi: 10.1145/860435.860460][12]Barnard K, Duygulu P, Forsyth D, de Freitas N, Blei DM, Jordan MI. Matching words andpictures. Journal of Machine Learning Research, 2003,3(2):1107 − 1135. [doi:10.1162/153244303322533214][13] LA VRENKO V, JEON J. Automatic image annotation and retrieval using cross-mediarelevance models. [C]//Proceeding of the 26th ACM SIGIR Conf. on Research and Development in Information Retrieval . New York: ACM, 2003: 119 − 126.[14]MINVI KE, SHUAIHAO LI, YONG SUN, SHENGJUN CAO. Research on similaritycomparison by quantifying grey histogram based on multi-feature in CBIR [C]//Proceeding of the 3rd International Conference on Education Technology and Training .IEEE,2010:422-424.[15] WANGKE GANG, QILI YING. Classification and application of images based on colortexture feature[C]// Proceedings of 4th IEEE International Conference on Computer Science and Information Technology .IEEE, 2011:284-290.[16]Mohamed Maher Ben Ismail. Image Database Categorization based on a Novel PossibilisticClustering and Feature Weighting Algorithm[C] // Proceedings of 2012 International Conference on Network and Computational Intelligence. 2012:122-127.[17]Du Gen-yuana, Miao Fang, Tian Sheng-li, Liu Ye.A modified fuzzy C-means algorithm inremote sensing image segmentation[C]// Proceedings of Environmental Science and Information Application Technology. 2009: 447-450.[18] Wang Ke-Gang, Qi Li-Ying. Classification and Application of Images Based on ColorTexture Feature[C]// Proceedings of 4th IEEE International Conference on Computer Science and Information Technology .IEEE Press. 2011:284-290.[19]Jeon J., Lavrenko V., Manmatha R. Automatic image annotation and retrieval using cross-media relevance models[C]// ACM SIGIR.ACM Press,2003:119- 126.[20]苗晓光,袁平波,何芳,俞能海. 一种新颖的自动图像标注方法[C].// 第十三届中国图象图形学术会议.2006:581-584.[21] Duygulu P, Barnard K, de Freitas J F G, et al. Object recognition as machine translation:Learning a lexicon for a fixed image vocabulary[M]//Computer Vision—ECCV 2002.Springer Berlin Heidelberg, 2002: 97-112.[22]王科平,王小捷,钟义信.加权特征自动图像标注方法[J].北京邮电大学学报,.2011:34(5):6-9.[23] Chen K, Li J, Ye L. Automatic Image Annotation Based on Region Feature[M]//Multimediaand Signal Processing. Springer Berlin Heidelberg, 2012: 137-145.[24]刘丽, 匡纲要. 图像纹理特征提取方法综述[J]. 中国图象图形学报, 2009, 14(4): 622-635.[25] 杨红菊, 张艳, 曹付元. 一种基于颜色矩和多尺度纹理特征的彩色图像检索方法[J]. 计算机科学, 2009, 36(9): 274-277.[26]Minyi Ke, Shuaihao Li, Yong Sun, Shengjun Cao . Research on similarity comparison byquantifying grey histogram based on multi-feature in CBIR [J]// Proceedings of the 3th International Conference on Education Technology and Training .IEEE Press.2010:422-424 [27] Mohamed Maher Ben Ismail. Image Database Categorization based on a Novel Possibilistic Clustering and Feature Weighting Algorithm[C] // Proceedings of 2012 International Conference on Network and Computational Intelligence. 2012:122-127.[28]Khalid Y I A, Noah S A. A framework for integrating DBpedia in a multi-modality ontologynews image retrieval system[C]//Semantic Technology and Information Retrieval (STAIR).IEEE, 2011: 144-149.[29]Celik T, Tjahjadi T. Bayesian texture classification and retrieval based on multiscale featurevector[J]. Pattern recognition letters, 2011, 32(2): 159-167.[30]Min R, Cheng H D. Effective image retrieval using dominant color descriptor and fuzzysupport vector machine[J]. Pattern Recognition, 2009, 42(1): 147-157.[31]Feng H, Shi R, Chua T S. A bootstrapping framework for annotating and retrieving WWWimages[C]//Proceedings of the 12th annual ACM international conference on Multimedia.ACM, 2004: 960-967.[22]Ke X, Chen G. Automatic Image Annotation Based on Multi-scale SalientRegion[M]//Unifying Electrical Engineering and Electronics Engineering.New York, 2014: 1265-1273.[33]Wartena C, Brussee R, Slakhorst W. Keyword extraction using wordco-occurrence[C]//Database and Expert Systems Applications (DEXA).IEEE, 2010: 54-58. [34]刘松涛, 殷福亮. 基于图割的图像分割方法及其新进展[J]. 自动化学报, 2012, 38(6):911-922.[35]陶文兵, 金海. 一种新的基于图谱理论的图像阈值分割方法[J]. 计算机学报, 2007, 30(1):110-119.[36]谭志明. 基于图论的图像分割及其嵌入式应用研究[D][J]. 博士学位论文) 上海交通大学,2007.[37] Shi J, Malik J. Normalized cuts and image segmentation[J]. Pattern Analysis and MachineIntelligence,2000, 22(8): 888-905.[38] Huang Z C, Chan P P K, Ng W W Y, et al. Content-based image retrieval using color momentand Gabor texture feature[C]//Machine Learning and Cybernetics (ICMLC), 2010 International Conference on. IEEE, 2010, 2: 719-724.[39]王涛, 胡事民, 孙家广. 基于颜色-空间特征的图像检索[J]. 软件学报, 2002, 13(10).[40] 朱兴全, 张宏江. iFind: 一个结合语义和视觉特征的图像相关反馈检索系统[J]. 计算机学报,2002, 25(7): 681-688.[41]Sural S, Qian G, Pramanik S. Segmentation and histogram generation using the HSV colorspace for image retrieval[C]//Image Processing. 2002. Proceedings. 2002 International Conference on. IEEE, 2002, 2: II-589-II-592 vol. 2.[42]Ojala T, Rautiainen M, Matinmikko E, et al. Semantic image retrieval with HSVcorrelograms[C]//Proceedings of the Scandinavian conference on Image Analysis. 2001: 621-627.[43]Yu H, Li M, Zhang H J, et al. Color texture moments for content-based imageretrieval[C]//Image Processing. 2002. Proceedings. 2002 International Conference on. IEEE, 2002, 3: 929-932.[44]Sun L, Ge H, Yoshida S, et al. Support vector description of clusters for content-based imageannotation[J]. Pattern Recognition, 2014, 47(3): 1361-1374.[45]Hiremath P S, Pujari J. Content based image retrieval using color, texture and shapefeatures[C]//Advanced Computing and Communications, 2007. ADCOM 2007.International Conference on. IEEE, 2007: 780-784.[46]Zhang D, Lu G. Generic Fourier descriptor for shape-based image retrieval[C]//Multimediaand Expo, 2002. ICME'02. Proceedings. 2002 IEEE International Conference on. IEEE, 2002, 1: 425-428.[47]Gevers T, Smeulders A W M. Pictoseek: Combining color and shape invariant features forimage retrieval[J]. Image Processing, IEEE Transactions on, 2000, 9(1): 102-119.[48] Bailloeul T, Zhu C, Xu Y. Automatic image tagging as a random walk with priors on thecanonical correlation subspace[C]//Proceedings of the 1st ACM international conference on Multimedia information retrieval. ACM, 2008: 75-82.[16]MOHAMED MAHER, BEN ISMAIL. Image database categorization based on a novelprobability clustering and feature weighting algorithm[C] // Proceedings of 2012 International Conference on Network and Computational Intelligence, 2012:122-127.[17]DU GENYUANA, TIAN SHENGLI, LIU YE.A modified fuzzy c-means algorithm in remotesensing image segmentation[C]// Proceedings of Environmental Science and Information Application Technology, 2009: 447-450.[18]SIVIC J, RUSSELL BC. Discovering objects and their location in images.[C] //Proceedingsof the 10th IEEE Int’l Conf. on Computer Vision .IEEE Computer Society, 2005:370 − 377.[19] DUYGULU P, BARNARD K, FORSYTH D. Object recognition as machine translation [J].//Learning a lexicon for a fixed image vocabulary In: HEYDEN A, NIELSEN M, JOHANSEN P, eds. Lecture Notes in Computer Science 2353,2002,45(1): 97−112.[20]JEON J, MANMA THA R. Automatic image annotation and retrieval using cross- mediarelevance models[C]// ACM SIGIR.ACM,2003:119- 126.[21]G K RAMANI and T ASSUDANI. Tag recommendation for photos[J].In Stanford CS229Class Project, 2009,23(1):130 − 145.[22] D. ZHANG and G. LU. A review on automatic image annotation techniques[J]. PatternRecognition, 2011,145(1):346–362.[23]K.BARNARD,P.DUGGULU,N FREITAS,D FORSYTH, D BLEI. Matching words andpictures [J].Journal of Machine Learning Research,2003,132(2):1107-1135.[24]苗晓光,袁平波,何芳,俞能海. 一种新颖的自动图像标注方法[C].// 第十三届中国图象图形学术会议.2006:581-584.[25] 王科平,王小捷, 钟义信.加权特征自动图像标注方法[J].北京邮电大学学报,2011,34(5):6−9.[26] JIN R, KANG F, SUKTHANKAR R. Correlated label propagation with application tomulti-label learning [C]. //Proceedings of the IEEE Computer Society Conference on Computer Vision and Pattern Recognition.2006:119-126.[27]YANG C.B, DONG, M, HUA J. Region-based image annotation using asymmetrical supportvector machine-based multiple-instance learning[C].// Proceeding of the CVPR.2006:2057–2063.[28] CARNEIRO G, V ASCONCELOS N. A database centric view of semantic image annotationand retrieval[C].// Proceeding of ACM SIGIR. 2005:559–566.[29] CUSANO C, CIOCCA G, SCHETTINI R. Image annotation using SVM[C].// Proceeding ofthe Internet Imaging, 2004: 330–338.[30]R Y AN, A HAUPTMANN, R JIN. Multimedia search with pseudo-relevancefeedback[C].//Proceeding of IEEE conference on Content-based Image and Video Retrieval.2007:238-247.[31] J W ANG, S KUMAR, S CHANG. Semi-supervised hashing for scalable imageretrieval[C].//Proceeding of IEEE conference on Computer Vision.2009:1-8.[32]M KOKARE, B CHATTERJI, P BISWAS. Comparison of similarity metrics for texture imageretrieval[C].//Proceeding of IEEE conference on Convergent Technologies for Asia-Pacific Region.2003:571-575.[33]EDWARD CHANG, KINGSHY GOH, GERARD SYCHAY, GANG WU. Content-base softannotation for multimodal image retrieval using bays point machines[J].CirSysVideo,2003,13(1):26-38.[34]SHI J, MALIK J. Normalized cuts and image segmentation[J].IEEE Transactions on PatternAnalysis and Machine Intelligence,2000,22(8):888-905.[35]ZHOU D, BOUSQUET O. Learning with local and global consistency[C].//Proceeding ofAdvances in Neural Information Proceeding Systems,2004:321-328.[36]Minyi Ke, Shuaihao Li, Yong Sun, Shengjun Cao . Research on similarity comparison byquantifying gray histogram based on multi-feature in CBIR [J]// Proceedings of the 3th International Conference on Education Technology and Training .IEEE Press.2010:422-424 [37] Wang Ke-Gang, Qi Li-Ying. Classification and Application of Images Based on ColorTexture Feature[C]// Proceedings of 4th IEEE International Conference on Computer Science and Information Technology .IEEE Press. PP:284-290 ,2011[38] Chen K, Li J, Ye L. Automatic Image Annotation Based on Region Feature[M]//Multimediaand Signal Processing. Springer Berlin Heidelberg, 2012: 137-145.[39]Lu Z, Ip H H S. Generalized relevance models for automatic image annotation [M]//Advancesin Multimedia Information Processing-PCM 2009. Springer Berlin Heidelberg, 2009: 245-255.[40]Fournier J, Cord M. A Flexible Search-by-Similarity Algorithm for Content-Based ImageRetrieval[C]//JCIS. 2002: 672-675.论文3[1]Zhang C, Chai J Y, Jin R. User term feedback in interactive text-based image retrieval[C]//Proceedings of the 28th annual international ACM SIGIR conference on Research and development in information retrieval. ACM, 2005: 51-58.[2]Akgül C B, Rubin D L, Napel S, et al. Content-based image retrieval in radiology: currentstatus and future directions[J]. Journal of Digital Imaging, 2011, 24(2): 208-222.[7]Zhang D, Islam M, Lu G. Structural image retrieval using automatic image annotation and region based inverted file[J]. Journal of Visual Communication and Image Representation, 2013, 24(7): 1087-1098.[8]Datta R, Joshi D, Li J, et al. Image retrieval: Ideas, influences, and trends of the new age[J]. ACM Computing Surveys (CSUR), 2008, 40(2): 111-115.[9]Li ZX, Shi ZP, Li ZQ, Shi ZZ. A survey of semantic mapping in image retrieval[J]. Journal of Computer-Aided Design and Computer Graphics, 2008,20(8):1085−1096 (in Chinese with English abstract).[10]Zhang D, Islam M M, Lu G. A review on automatic image annotation techniques[J]. Pattern Recognition, 2012, 45(1): 346-362.[11] Li J, Wang J Z. Automatic linguistic indexing of pictures by a statistical modeling approach[J]. Pattern Analysis and Machine Intelligence,2003, 25(9): 1075-1088.[12] Chang E, Goh K, Sychay G, et al. CBSA: content-based soft annotation for multimodal image retrieval using Bayes point machines[J]. Circuits and Systems for Video Technology,2003, 13(1): 26-38.[13]Jeon J, Lavrenko V, Manmatha R. Automatic image annotation and retrieval using cross-media relevance models[C]//Proceedings of the 26th annual international ACM SIGIR conference on Research and development in information retrieval. ACM,2003: 119−126.[14] Lavrenko V, Manmatha R, Jeon J. A Model for Learning the Semantics of Pictures[C]//NIPS. 2003: 11-18.[15]Feng S L, Manmatha R, Lavrenko V. Multiple bernoulli relevance models for image and video annotation[C]//Proceedings of the IEEE Computer Society conference onComputer Vision and Pattern Recognition.IEEE, 2005: 51-58.[16]Tian D, Zhao X, Shi Z. An Efficient Refining Image Annotation Technique by Combining Probabilistic Latent Semantic Analysis and Random Walk Model[J]. Intelligent Automation & Soft Computing, 2014 (ahead-of-print): 1-11.。
- 1、下载文档前请自行甄别文档内容的完整性,平台不提供额外的编辑、内容补充、找答案等附加服务。
- 2、"仅部分预览"的文档,不可在线预览部分如存在完整性等问题,可反馈申请退款(可完整预览的文档不适用该条件!)。
- 3、如文档侵犯您的权益,请联系客服反馈,我们会尽快为您处理(人工客服工作时间:9:00-18:30)。
Journal of Computational Information Systems 6:9(2010) 2821-2830Available at Cross-media Retrieval Based on CSRN ClusteringCheng ZENG1,†, Zhenzhen WANG1, Gang DU21State Key Lab of Software Engineering, Wuhan University, Wuhan, China, 4300722School of Computer, Wuhan University, Wuhan, China, 430072AbstractA novel cross-media retrieval methodology is proposed to help user more accurately and naturally describe theirrequirement and gain response. The first problem to be solved in this paper is how to build the bridge among heterogeneous information. An efficient approach is adopted to mine the semantic relationship among different multimedia data. We propose the scheme, called cross-media semantic relationship network (CSRN), to store the cross-media relationship knowledge, which is constructed by mining various potential relation information in the Internet. By hierarchical fuzzy clustering based on semantic relationship against CSRN, we obtain semantic vector bundle which could gather different modalities but same semantic of diverse media feature vectors. Furthermore, dynamic linear hash index is used in these vectors to adapt to the flexible expanding or updating of CSRN and quickly retrieval based on multiple examples without the limit of media modality by hash intersection. We show through experiment based on different modalities or different numbers of examples that our approach can provide more flexible retrieval mode and more accurate retrieval results.Keywords:Cross Media; Semantic Relationship; Semantic Vector Bundle; Hierarchical Fuzzy Clustering1. IntroductionCommercial multimedia search engines consider more on efficiency and realize search based on relevant technique of keywords or low-level features matching, such as Google.image, Bing.image and Like, GazoPa, etc. However, these systems provide only simple requirement expressing way to user so that user’s intention is difficult to be understood and the results are always poor amd single modality. On the contrary, academic circles are exploring better man-machine information exchanging technology, where the machine would adapt to the human’s habit of expressing and receiving information as much as possible, but not that the human have to adapt to the understanding ability of machine. Lots of new research topics emerge which generally refer to the concept “semantic”, such as semantic search, semantic multimedia, semantic computing and so on. Their common ground is to attempt to create, process or display information with the thinking way as human, e.g. IGroup[1] provides the semantic classifications of results. Hakia, Cuil, Kosmix and PlayAudioVideo support multimodal of results displaying, and Powerset focuses on natural language search. In addition, there are so many techniques[2-5] which realize semantic or personalized retrieval based on ontology.Many earlier cross-media studies [6,7] focused on integrating or managing heterogeneous multimedia data. Henceforth, cross-media technology is applied into diverse multimedia retrieval domain. Liu [8] proposes a cross-media manifold co-training mechanism between keyword-based metric space and vision-based metric space and deduce a best dual-space fusion by minimizing manifold-based visual & textual energy criterion. At last, he realizes image annotation and retrieval. In [9], a dual cross-media †Corresponding author.Email addresses: zengc@ (Cheng ZENG), zhenzhen_1987cn@ (Zhenzhen WANG),melephas@ (Gang DU)1553-9105/ Copyright © 2010 Binary Information PressSeptember, 20102822 C. Zeng et al. /Journal of Computational Information Systems 6:9(2010) 2821-2830 relevance model which considers both word-to-image and word-to-word relations is proposed to automatically annotate and retrieval images. Furthermore, there are lots of researches on video retrieval based on cross-media. Bruno et al. [10] designs a multimodal dissimilar space which is constructed based on positive and negative examples distribution and realizes video retrieval by space clustering and modalities fusing. Meanwhile, many researchers explore cross-media comprehensive retrieval way, such as a typical application about E-learning [11]. [12] utilizes the complementarity among different modalities of media data to mine co-occurrence relationships of news items, and then provides cross-media content complementation. Zhuang et al. [13,14] integrate the webpage structure analyzing and low-level features correlation mining of multimedia data to construct cross-media relationship graph UCCG, and short-term and long-term feedbacks play vital role in the method.Cross-media technology aims at making maximum use of relevance, cooperation, complementarity among diverse modalities of media to fuse all sorts of information. As a result, user can gain the desired information with higher accuracy, more quickly speed and lower cost. The kernel of realizing cross-media retrieval is to discover the semantic relationship among different multimedia data. However, the semantic “gap” and the feature diversity among various modalities of media make the development of this domain difficult in taking a step. Furthermore, the common character of high information density of multimedia data lead to that the relevant processes generally require a large amount of computing time and resources. It is very difficult to develop a universal cross-media retrieval engine at present. However, the technology has good application prospect in some certain domain, such as E-learning, E-business, tourism and entertainment, etc.2. Framework of Cross-media Retrieval SystemThis paper gains semantic relationships among diverse data from internet where multitudinous knowledge is contributed by various persons or software systems every moment. Initially, we don’t concern about the modality of data, but pay attention on the channels where we can get the relationship. All media objects, which are the results by processing multimedia document and semantic relationship among them will be stored into a unified model, called cross-media semantic relationship network (CSRN). For multimodal, time-based and text types of multimedia document, we segment them into the smaller granularity of unit. The kernel of our approach is how to construct and utilize CSRN. We give the definition of CSRN below: Definition 1. Let each node represent a modality of media object, the metadata structure of is as follows:, , , , , , , , , (1) Each edge , denotes semantic relationship between different media objects, which comes from 4 aspects:processes space dependency of web data based on visual structure and hyperlink in the webpage, e.g. adjacent text, image or other modality of media objects in a webpage have more similar semantic;analyzes the labels and ranking of search results coming from multiple multimedia search engines based on the same search condition;mines the inherent relevancy among different modalities of data or same modality of segmented data in a multimedia document, e.g. visual, audio and textual data in a news video, or all video scene clips in a sport video have certain relevancy each other;accumulates potential user feedback during the process of user browsing. E.g. those results clicked one after another generally have approximative topic or similar semantic.We call the the integration of these nodes and edges as CSRN.In formula (1), each media object has a globally unique identity SID and GID corresponds to the document ID. Moreover, not each media object corresponds to an example. Time-based media such as video, audio possibly contain too much semantic in a same document, which possibly lead to most media objects having semantic relaionship each other. Overly abundant semantic relationship in CSRN is equivalent to not be able to gain any valuable information. Moreover, a multimedia document includingC. Zeng et al. /Journal of Computational Information Systems 6:9(2010) 2821-2830 2823multiple modalities of data will also affect the analysis. Thus, it’s necessary to previously split or segment these multimedia documents before they are regarded as media objects. Likewise, text is segmented based on paragraph to reduce the semantic complexity of each media object.After CSRN is constructed, the hierarchical fuzzy clustering will be used on CSRN. The purpose is to put all media objects with similar semantic into same cluster, called semantic category (SC). It is possible that a media object belongs to multiple SC, and even several media objects belong to distinct or same SC in different conditions. Thus, the hierarchical fuzzy clustering method will be suitable and essential to these statuses. All media objects will be substituted for their feature vectors. Because there are a large number of different modalities of media object in same SC and many of them possess similar low-level features which are actually redundant, we quantify all feature vectors in a SC by feature vectors clustering against different modalities of media objects, respectively. Then the typical feature vectors(TV), called semantic vector bundle (SVB), will be selected to represent the SC. It means that we obtain the mapping among all possible feature vectors of different modalities but similar semantic. At last, hash indexs will be respectively created on these TV sets corresponding to different modalities of media objects. Thus, if a user submits several media examples with same or different modalities, they will be preprocessed and transformed into feature vectors, and then respectively locate their own SC sets. The architecture of our cross-media retrieval method is shown in Fig.1.3. Cross-media Semantic Relationship NetworkA great deal of researchers improve the system performance by mining implicit or explicit knowledge in the internet. Furthermore, most of commercial search engines use keywords and hyperlink extracted from Webpages to index the images, videos or other data. In our approach, all initial cross-media semanticrelationship knowledge comes from internet and these knowledge will be stored in CSRN.Fig.1 Architecture of Cross-media Retrieval System2824 C. Zeng et al. /Journal of Computational Information Systems 6:9(2010) 2821-28303.1. Webpage Visual Structures and Hyperlink AnalysisSome privious methods regard webpages as information units. A webpage generally contains multiple and different granularities of semantic so that these methods will confuse the semantic correlation among media objects. VIPS algorithm [15] is proposed to segment webpage by analyzing DOM tree based on visual structure. The highest sensitive granularity of VIPS is <TD> level because its purpose is to segment webpage block. But in our work, extracting the media objects and the relationship among them are the final purpose. Hence we improve VIPS algorithm so that it is sensitive to URL address, <BR> in continuous text and hyperlink etc.. Thus, each leaf node will actually correspond to a media object and the semantic relationship only exist between any two leaf nodes. Fig.2 shows an example of webpage visual segmentation and the final visual structure tree constructed by improved VIPS is shown in Fig.3.Fig.2 An Example of Webpage Visual Segmentation Fig.3 An Example of Webpage Visual Structure TreeThe similarity between any two nodes can be calculated based on the shortest path in the tree. Becausesemantic generalization will lose some semantic information while semantic specialization narrows therange of semantic description, we give different semantic attenuation constants α and βrespectivelycorresponding to upward and downward section in the path. The formula of relationship calculatingbetween any two media object and is as follows:, 1 0 1 0 (2) where n and m denote the numbers of upward and downward sections, respectively.3.2. Results Analysis of Multimedia Search EnginesMultimedia search engines return lots of related multimedia documents based on keywords, such asgoogle.image, Youtube, Flickr etc.. Hakia, Cuil even simultaneously returns different types of multimedia documents. A concept set is extracted from LSCOM, and then the set is extended with WordNet. These concepts will be selected or combined according to some certain rules in turn and be simultaneouslysubmited to all search engines. For each search, all returned results are expected to have similar semantic, and a sub-CSRN could be constructed. But polysemy confuses the results and affects the ranking.Contextual concepts in result labels are used to filter most confusing results. Thus, we syntheticallyconsider the result label which are preprocessed to only reserve noun and verb keywords, and result rankingof search engine to measure the semantic relationship among returned results.At first, , which denotes semantic similarity between search keywords set and concepts set corresponding to v th result label will be calculated:, , , ,,, , (3)In the above formula, KM denotes classical Kuhn-Munkres algorithm, and , denotes the semantic similarity between any two concepts , in and , respectively, and 0 , 0 where , are represented as the amount of retained search keywords and correspondingreturned results, respectively., represents the number of same concept between and , while,is the amount of all different concepts in and . is constant between 0 and 1 when theC. Zeng et al. /Journal of Computational Information Systems 6:9(2010) 2821-2830 2825 intersection between and is null after deleting same concepts from them. Semantic similarity function [16] based on WordNet is utilized to calculate the similarity between different concepts.,1 2 , ,, / , , ,(4) Detailed mathematical introduction of formula (4) can be found in [16]. We previously construct a concept similarity map (CSM) for avoiding repeatedly computing , .We define semantic relationship, of any two results (media objects) with same search keywords in formula (5). It is measured based on the cosine of angles between two result vectors constructed in the results semantic similarity space (R3S) where horizontal ordinate denotes the similarity degree between result label and search keywords, and vertical ordinate denotes ranking value., , ,, ,0 , (5)where , are decimal value which denote the results of ranking number i and j divided by , respectively.3.3. Other Aspects of Semantic Relationship and Their SynthesisFor mining aspect of relationship, we require to adjust the size of each time-based or multimodal media object in initial CSRN or even decompose it based on modality so as to simplify its semantic. Currently, there are lots of mature technique to support splitting diverse modalities of data from multimodal document, such as splitting audio from video. Then different modalities of time-based data contained in the same document will be jointly segmented with same boundary, where audio data are segmented referring to the results of video segmentation based on scene. Each video or audio segment would eventually correspond to a node (media object) in CSRN. The semantic relationship between different modalities of data segment within same time range are always 1, namely, 1. While semantic relationship between different data segments with same modality is defined based on adjacency distance:,1 01√ 1⁄ 1 (6)where x denotes the interval number of segment between and , and is a constant representing degeneration degree of semantic relationship.aspect will be able to optimize the relationship knowledge in CSRN after each time of cross-media search feedback, such as browsing, printing, downloading, etc., where the system will save operating results with same search condition. These are regarded as implicit user contributing. The relationship among the results will be used to update CSRN:, ∑ · 2, 0 (7)where denotes the amount that and have same operation in same search condition and represents the influence degree of each kind of operation. If the result of updated, is greater than 1, it will be directly replaced by 1.4. CSRN Index for Efficiently Retrieval and ExpandingYou could submit an image example and find a most similar image in CSRN with traditional feature matching technique, and then gain related diverse modalities of multimedia documents based on cross-media semantic relationship. However, when user submits several examples which are heterogeneous/homogeneous, the above way is possible to return several irrelative results sets. Furthermore, the retrieval efficiency will be low and CSRN expanding is difficult when new multimedia documents are inserted. Thus, we will introduce an efficient cross-media index mechanism in this section.2826 C. Zeng et al. /Journal of Computational Information Systems 6:9(2010) 2821-28304.1. Hierarchical Fuzzy ClusteringBy clustering on CSRN, all media objects with similar semantic can be congregated into the same semantic category (SC). However, most media objects possess complex semantic, and they are impossible to simply belong to a certain SC. Moreover, the clustering granularity is difficult to make certain. If the granularity is fine, we can obtain relatively accurate results but lose the recall ratio. Thus, it’s necessary to use hierarchical fuzzy clustering (HFC) algorithm against CSRN.The whole process of HFC includes two phases. In the first phase, K-distance neighbor is used to construct the initial status of HFC. Each media object and its K-distanced neighbors set can be picked out easily from CSRN which is stored with symmetric matrix. The average distance between and any object in can be calculated and denoted as . Then the relative density of K-distance neighbor of object is defined as follows:,…, , ,…,,…, , ,…, (8)Given the threshold value 0, is considered as a core object when 1 . For with , core objects set is defined as | where O is the list of entire core objects } which is the initial status of any cluster. In other words, a core object with current global minimum and without class mark will be picked out, and is obtained to form a new marked cluster . Then all objects in of cluster will be gradually extended by adding the K-distance neighbors of each core object in turn. Assume that is one core object in cluster , which has not been extended, and its K-distance neighbors can be obtained, denoted as 1,…, . If is a core object of other cluster, it will be ignored. Otherwise, it will be added into cluster . The cluster can be extended until no more objects in it can be extended. The process continues until all core objects have been extended. Here we can find that core objects are pivotal for each cluster and it is impossible that a core object belongs to more than one cluster, while other objects can exist in multiple clusters in the same time. During the course of extension, two clusters will be marked as adjacent clusters when part of objects they include are the same. Thus, we obtain the highest granularity of fuzzy clustering result.In the second phase, the clusters obtained in the first phase will be fuzzily clustered further. First, fuzzy similarity , of any two adjacent clusters and is calculated as:, ,, (9)Then descending threshold value set 1,…, is selected for measuring similariy from fine granularity to coarse granularity. For each cluster , similar cluster set under certain granularity level ∆ is defined as | , . The clusters in can be united into one cluster on level ∆. As the value of descend, we can obtain hierarchical clustering of objects and the granularities of clustering are from fine to coarse, until they are united into one cluster.4.2. Index on CSRNMedia feature vector quantification is the first process step which does not reduce the dimension number of vector but the amount of vectors. However, the dimension and implication of heterogeneous media feature vectors are different. Thus, we firstly distinguish feature vectors according to media modality, and then cluster these vectors with FEC algorithm [17], and the average vector (called typical vector, TV) is regarded as the representative of a cluster, shown in Fig.4. All TVs in same SC make up a semantic vector bundle (SVB). Each SVB enumerates various feature vectors which come from different modalities of media objects but represent similar semantic. We build the mapping relation , between TV and feature vectors in corrrespondng cluster and , between TV and SC where , σ respectively denotes the granularity level in HFC and media modality.For flexibly expanding or updating CSRN, and efficiently searching feature vector, we create dynamic linear hash index on which computes the hash value of TV only one times, stores hash values usingC. Zeng et al. /Journal of Computational Information Systems 6:9(2010) 2821-2830 2827as few buckets as possible and linearly increases buckets. Several vector hash functions are respectively defined for different dimension of vector corresponding to different media modality . The actual active bit number of could be calculated as follows, which always use the low order bits:(10)Where is represented as the amount of TV of modality σ.If a user submits an example , the system will extract its feature vector at first, and then invoke the corresponding . The returned result determines a unique hash bucket which stores a TV set having same hash value. The media object whose feature vector is most similar with must also exist in this bucket. Then, we use vector matching technique to locate the media object. Suppose Top( ) denoted the most similar TV with in hash bucket . We can get many SC sets of different granularity levels based on , .∑, (11) By now, user actually gains a result set containing multiple modalities of media objects which have been classified through SC in different granularity levels. is the highest granularity. If a user pays attention on precision, he can select fine granularity. When user submits multiple examples which are even different modalities, we use hash intersection to realize search. The search examples set is represented as . The system returns a corresponding to each example so that we can calculate the intersection among these SC sets of different examples in each granularity level.∑, (12)5. Experiments5.1. Data sources and CSRNOur experiments data all come from internet through webpage analysis which includes two aspects of pages. The first is the common pages of Web encyclopedia 1, Yahoo!news, etc.. We manually select the initial pages as entrance, and then the related webpages will be automatically crawled based on hyperlinks. The another is results page of multimedia search engines such as Google.image, Hakia, Flickr, etc. We extract 118 concepts with distinct visual or audio characteristic from LSCOM and expand them with synonym and cognate words in WordNet. At last, 203 concepts are used and they compose into 1065 keywords set, each set of which includes 1 to 3 concepts. These keywords set are simultaneously submited to all search engines in turn and are projected into the same R3S to calculate results similarity each other for same search condition. For ensuring relatively high accuracy and avoiding overfull results set, we only analyze and store 1; ; .Fig.4 The Schematic Diagram of CSRN Index2828 the top from 3some a minute For (mean,contras and th feature 5.2. Th Fig.5(1multip text by as lowest quantit contain semant video a and ge ways t higher filters reduce examp For by mu previou multip examp modali audio m precisi consid of com search 2http://w C p 15 results fo 352 video, 134audio material e data for all ti image, we ex , variance, ske st, Orientation he motion fea es slope, roll-o he Performan 1)-(3) show th le same moda y image as ex spect where te precision due ty in asp ns the feature tic relationshi and audio hav enerate relation to get audio d average searc the semantic e the deviatio les is similar a (1) Cross-media Image ExaFig.better reflecti ultiple modalit us dataset, sh le modalities les and more ities of media media data as ion of image+erable role du mbinational ex examples as/sm C. Zeng et al. /or each search 4,798 text par l websites suc ime-based me xtract 3 types o ewness), and 3n). For video ature histogra off, centroid an nce of Cross-m he experimen ality of media xample has the ext and imag e to less relati ect. Although e in key fram ip informatio ve better searc nship in data. It can be ch accuracies distinction am n between se as Zhuang’s a Retrieval by ample 5 Comparison of ng the charac ties of media hown in Fig.6s of example e modalities o a data as exam s examples ha +audio and v uring the proce xamples. Thes many as posmzy/dongwu-yx-7/Journal of Co h. We totally c ragraphs and 2ch as smzy 2 an edia.of color featur 3 types of text segment, we am in whole nd OER are u media Retrieva nt results of q a data as exam e highest prec e are in the m ionship which h video has sam me which is th n about imag h accuracies e aspect. But th e seen that m than single d mong differen earch example approach [14], b (2) f Cross-media Ret teristic of ind a data combin 6. We can ob es generally r of examples c mples, we can ave higher ac video+audio ess of cross-m se phenomeno sible will mak711-6.htmlomputational Icollect 40,610 2,809 audios nd so on. We re including c ture features i mainly reserv segment. For used.alquerying diffe mples which a cision because majority. But h are mainly l me situation a he same as an ge and enhan each other bec his situation w multiple docum document in a nt examples an es and user r ut we do not r Cross-media Ret Video Example trieval among Di ex intersection nation as exam bserve severa receives high can receive hi still get good ccuracy than p are higher th media retrieval on demonstratke the system Information S images and 3which are ext filter voice in color histogram including MR ve the feature r audio, cepst erent modaliti are all out of e we extract a querying aud limited in as audio, the fe n image. So a nce relational cause most of will be possibl ments as exam ll three figure nd receives th requirement. rely on iterativ trieval by e fferent Modalitie n in our appro mples where al interesting her search ac igher accuracy d results. Seco pure visual m han that of im l which signif te the principlm more accura Systems 6:9(203,379 video se tracted from v n audio and on m, color cohe RSAR, Gabor, e of key fram tral features ies of media our dataset. I a mass of sem dio by image and asp feature vector actually, video search accur f audio data ar ly changed if mples together es owing to SC heir semantic The search a ve short-term (3) Cross- Au es of Search Exam oach, we test c all examples phenomenon ccuracy than y. Even thoug ond, the comb media combina mage+video. ficantly raises le that using vately understa 010) 2821-283egments which videos or com nly analyze the rence, color m Tamura (coar me regarded as MFCC and s objects by sin In Fig.5(1), qu mantic relation as example h pects and has extracted from o indirectly u racy. In Fig.5e splitted from f we have mor r receive rema C intersection in common s accuracy of m feedback.-media Retrieval udio Example mples cross-media re are also out n. First, query single moda gh we only u ination of visu ation, e.g. the Third, text p s the search pr various modaland user requi 30h come me frome first 1moment rseness, s image spectral ngle or uerying nship in has the a small m video uses the (2)-(3), m video re other arkably n which so as to multiplebyetrieval t of the ying by ality of use two ual and search plays a recision lities ofirement。