谷歌无人驾驶月度实测数据2015年12月
谷歌开发自动驾驶汽车技术以减少交通事故

随着 中国新农村建设 的深入 ,一些新 问题 也
应 运 而生 。 如农 民住 宅实 行 统一 规 划 、统 一 设
( 3 以上 则来源于美国加州大学洛杉矶分校
周江评 ,2 1 —1 —1) 0 0 1 0
计 、统 一建设和统 一配套基础设 施后 ,农 民的交
机场停车策略评估和相关技术 在 中 国 ,伴 随着 小 汽 车 越来 越 多地 进入 家 庭 ,人们也在 更 多的场所 需要停车场地 ,例如机
巴士转换到 自行车这一有效 的方式来 降低二氧化
碳 的排放量。
( 经济参考报 ,2 1 —1 —1) 00 1 2
场 、火 车站 、汽 车站 等城市 重要 对外 交通节 点 。
素 。 人 们 更 加 关 注 气 候 变化 ,通 过 从 小 汽 车 或 者
趣 的读者 ,可访问 :
h p / p . . gc f dT B tr et sl . p t :a sr o / e /R Ne o c p y s? t / p t r ms e b P j Di a a Poet = 9 7 r cD 28 。 j l
年均 2 %~ 0 5 3 %的速度增长 ,近 2 %的成年人使用 0 头盔 ,9 岁以下儿童的头盔使用率高达 7%。 5
利 用 与交 通 关联 的研 究 常 常默认 是 针 对城 市 地 区 ,而在 美 国 ,类似 的现 象也 不 同程度地 存 在。 最近 ,美 国交通研 究会 为改变这一现象 ,提 供 了 6 万美 元开放 性资金 ,面 向全 美研究人 员招 标 , 5
世界各 国研 究者 从不同侧面研究交通行 为的 l 篇 8
间 ,未来还能实现 汽车共享 ,并大大降低 汽车使 用 ,帮助创造 “ 未来公路列车” 。
综述无人驾驶汽车的主要功能

综述无人驾驶汽车的主要功能摘要:随着技术模式的不断更迭与改进,无人驾驶已成为世界各国竞相投入开发的新技术。
原因在于无人驾驶技术涉及多项领域,其中包括无人控制,人工智能,计算机视觉等高新技术,还与互联网紧密结合。
将无人驾驶技术做好做强,掌握的不仅是一门精尖技术,更是对本国科技水平的一次整合与提升。
本文简要介绍了无人驾驶汽车的一些主要功能及实现方法,对无人驾驶的一些问题进行了思考,简述了无人驾驶技术的一些优势和不足。
关键词:无人驾驶、路线规划、车内信息采集、车外信息采集1. 引言无人驾驶汽车在20世纪已经有了数十年的历史,在21世纪呈现出了实用趋势,国内外几家公司也在快速推进无人驾驶汽车实用化。
当前,人们对无人驾驶汽车的需求不断提高,无人驾驶技术也日益成熟。
因此开展对无人驾驶方面的研究具有重要的意义。
2. 无人驾驶技术的简介2.1 无人驾驶汽车的发展情况无人驾驶这一概念最先由英、德、美等发达国家在上世纪七八十年代提出。
发展至今,国外的许多公司都取得了不错的成绩。
如谷歌,其在2014年12月中下旬,就首次展示了无人驾驶原型车成品,该车可全功能运行。
2015年5月8日在美国内华达州允许测试3个月后,谷歌的无人驾驶汽车就取得了合法牌照。
在我国,无人驾驶技术日臻成熟。
在1992年国防科技大学成功研制出中国第一辆真正意义上的无人驾驶汽车。
现今,百度与北汽合作打造的无人驾驶汽车已经试运营,并且达到L3级别。
未来,我国将推进立法,使无人驾驶汽车真正合法化。
美国汽车工程师学会(SAE)对自动驾驶有明确的分级并已经被 NHTSA 确定为标准,从辅助驾驶到完全不需要人干预的自动驾驶有明确界定[1]。
根据NHTSA对无人驾驶汽车的分级,无人驾驶汽车分为L0、L1、L2、L3、L4五个等级,如表1。
美国摩根斯丹利研究报告中指出在2025 年自动驾驶技术在美国的潜在经济影响为 2000 亿到 1.9万亿美元左右。
[2]2.2 无人汽车的组成部分无人汽车的组成部分主要有:GPS、测距雷达、激光发射器、中央处理器、视频采集器。
谷歌无人驾驶月度实测数据2016年3月

ACTIVITY SUMMARYAll metrics as of March 31, 2016Vehicles:●21 Lexus RX450h SUVs currently self-driving on public streets: 13 in Mountain View, CA; 8 inAustin, TX●33 prototypes currently self-driving on public streets: 24 in Mountain View, CA; 7 in Austin, TX; 2in Kirkland, WAMiles driven since start of project in 2009“Autonomous mode” means the software is driving the vehicle, and test drivers are not touching the manual controls. “Manual mode” means the test drivers are driving the car.●Autonomous mode:1,498,214●Manual mode:1,046,386●We average around 10,000—15,000 autonomous miles per week on public streets BUILDING MAPS FOR A SELF-DRIVINGCARWe’re often asked how we build mapsspecifically for a fully autonomous car. A mapfor self-driving cars has a lot more detail thanconventional maps (e.g. the height of a curb,width of an intersection, and the exact locationof a traffic light or stop sign), so we’ve had todevelop a whole new way of mapping theworld.Before we drive in a new city or new part oftown, we build a detailed picture of what’saround us using the sensors on our self-drivingcar. As we drive around town, our lasers sendout pulses of light that help us paint a three-dimensional portrait of the world. We’re able to tell the distance and dimensions of road features based on the amount of time it takes for the laser beam to bounce back to our sensors (see image above). Our mapping team then turns this into useful information for our cars by categorizing interesting features on the road, such as driveways, fire hydrants, and intersections.This level of detail helps our car know exactly where it is in the world. As our cars drive autonomously on the road, our software matches what the car sees in real-time with the maps we’ve already built, allowing the car to know its position on the road to within 10cm of accuracy. That means we don’t have to rely on GPS technology, or a single point of data such as lane markings, to navigate the streets. Another benefit of knowing permanent features of the road is that our sensors and software can focus more on moving objects, like pedestrians, vehicles, and construction zones. This allows us to do a better job of anticipating — and avoiding — tricky situations.Self-driving cars can use a much greater level of detail than you’d find on Google Maps. Our mapping team highlights road features such as the length of a crosswalk, height of a traffic light, and the curve of a turn.Of course our streets are ever-changing, so our cars need to be able to recognize new conditions and make adjustments in real-time. For example, we can detect signs of construction (orange cones, workmen in vests, etc.) and understand that we may have to merge to bypass a closed lane, or that other road users may behave differently.To keep our maps up-to-date, our cars automatically send reports back to our mapping team whenever they detect changes like these. The team can then quickly update the map and share information with the whole autonomous fleet.SCENES FROM THE STREETEach month we’ll give examples of situations we encounter on the roadWhat do a 1980s Japanese arcade gameand our self-driving car have in common? This month we showed a compilation of odd encounters we’ve recently had on the streets while out testing. One of these included half a dozen people leap-frogging through traffic in front of one of our self-driving cars (if you’re finding that difficult to imagine, you can watch Chris Urmson show this encounter in his S XSW speechat 26:00).Despite never encountering humans posing as an army of frogs, our car still knew how to behave safely (though your parents would probably tell you this is unsafe behavior anyway, so kids don't try this at home!). That’s because rather than teaching the car to handle very specific things, we give the car fundamental capabilities for detecting other road users or unfamiliar objects, and then we give it lots of practice in a wide range of situations.On our private test track, we’ve dreamt up and recreated hundreds of odd scenarios to gauge our car’s response (e.g we even had someone jump out of a porta potty on the side of the road), but situations like these demonstrate why public testing of our self-driving cars is important to developing our cars for the road. We can try to come up with lots of wacky situations for our cars to handle, but the real world can defy even our wildest imaginations.TRAFFIC COLLISIONS INVOLVING AUTONOMOUS FLEETIn this section, we detail any accidents our self-driving fleet has been involved in while testing on public roads. Given the time we’re spending on busy streets, we’ll inevitably be involved in collisions; sometimes it’s impossible to overcome the realities of speed and distance. Thousands of minor accidents happen every day on typical American streets, 94% of them involving human error, and as many as 55% of them go unreported. (And we think this number is low; for more, see h ere.)March 14, 2016:A Google Lexus-model autonomous vehicle (“Google AV”) travelling westbound on W. Anderson Ln. in Austin, TX in autonomous mode was rear-ended while stopped at a traffic light. The Google AV was stopped for approximately 3 seconds behind traffic waiting at a red light at Burnet Road, when a vehicle (Volkswagen Passat) approaching from behind collided with the rear bumper of the Google AV. The Google AV’s speed at the time of the collision was 0 mph. The other vehicle’s approximate speed at the time of the collision was 10 mph.The driver of the other vehicle appeared disoriented to the Google AV test driver, so the Google AV test driver called 911, and the 911 dispatcher sent emergency vehicles to the scene. The Google AV sustained minor damage to its rear bumper. The other vehicle sustained moderate damage to its front bumper.WHAT WE’VE BEEN READING●SxSW: W atch Chris Urmson explain Google’s self-driving car project [video](March 2016)●Washington Post: I rode in Google’s self-driving car. This what impressed me the most.(March2016)●The Verge:G oogle's bus crash is changing the conversation around self-driving cars(March2016)●USA Today:S elf-driving car leaders ask for national laws(March 2016)●AP:A utonomous cars aren't perfect, but how safe must they be?(March 2016)。
四川省2020年公需科目试题及答案
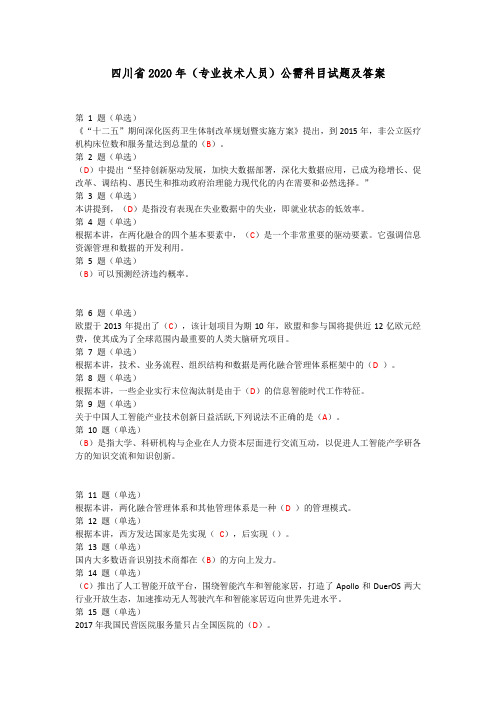
四川省2020年(专业技术人员)公需科目试题及答案第1 题(单选)《“十二五”期间深化医药卫生体制改革规划暨实施方案》提出,到2015年,非公立医疗机构床位数和服务量达到总量的(B)。
第2 题(单选)(D)中提出“坚持创新驱动发展,加快大数据部署,深化大数据应用,已成为稳增长、促改革、调结构、惠民生和推动政府治理能力现代化的内在需要和必然选择。
”第3 题(单选)本讲提到,(D)是指没有表现在失业数据中的失业,即就业状态的低效率。
第4 题(单选)根据本讲,在两化融合的四个基本要素中,(C)是一个非常重要的驱动要素。
它强调信息资源管理和数据的开发利用。
第5 题(单选)(B)可以预测经济违约概率。
第6 题(单选)欧盟于2013年提出了(C),该计划项目为期10年,欧盟和参与国将提供近12亿欧元经费,使其成为了全球范围内最重要的人类大脑研究项目。
第7 题(单选)根据本讲,技术、业务流程、组织结构和数据是两化融合管理体系框架中的(D )。
第8 题(单选)根据本讲,一些企业实行末位淘汰制是由于(D)的信息智能时代工作特征。
第9 题(单选)关于中国人工智能产业技术创新日益活跃,下列说法不正确的是(A)。
第10 题(单选)(B)是指大学、科研机构与企业在人力资本层面进行交流互动,以促进人工智能产学研各方的知识交流和知识创新。
第11 题(单选)根据本讲,两化融合管理体系和其他管理体系是一种(D)的管理模式。
第12 题(单选)根据本讲,西方发达国家是先实现(C),后实现()。
第13 题(单选)国内大多数语音识别技术商都在(B)的方向上发力。
第14 题(单选)(C)推出了人工智能开放平台,围绕智能汽车和智能家居,打造了Apollo和DuerOS两大行业开放生态,加速推动无人驾驶汽车和智能家居迈向世界先进水平。
第15 题(单选)2017年我国民营医院服务量只占全国医院的(D)。
第16 题(单选)下列有关人工智能的说法中,不正确的是(C)。
谷歌自动驾驶测试报告(2015年12月)

Google Self-Driving Car Testing Reporton Disengagements of Autonomous ModeDecember 2015IntroductionIn accordance with regulations issued by the the California Department of Motor Vehicles (DMV), Google Auto LLC (“Google”) submits this report of disengagements from autonomous mode that have occurred when operating its self-driving cars (SDCs) on public roads in California. In accordance with the DMV rule , this report covers the period from the date of issuance of Google’s1Manufacturer’s Testing Permit (September 24, 2014) through November 30, 2015.As of the end of November, Google had operated its self-driving cars in autonomous mode for more than 1.3 million miles. Of those miles, 424,331 occurred on public roads in California during the period covered by this report -- with the vast majority on surface streets in the typical suburban city environment of Mountain View, CA and neighboring communities. We’re self-driving 30,000-40,000 miles or more per month, which is equal to two to four years of typical US adult driving.The setting in which our SDCs and our drivers operate most frequently is important. M astering autonomous driving on city streets -- rather than freeways, interstates or highways -- requires us to navigate complex road environments such as m ulti-lane intersectionsor unprotected left-hand turns, a larger variety of road users including cyclists and pedestrians, and more unpredictable behavior from other road users. This differs from the driving undertaken by an average American driver who will spend a larger proportion of their driving miles on less complex roads such as freeways. N ot surprisingly, 89 percent of our reportable disengagements have occurred in this complex street environment (see Table 6 below).Disengagements are a critical part of the testing process that allows our engineers to expand the software’s capabilities and identify areas of improvement. Our objective is not to minimize disengagements; rather, it is to gather, while operating safely, as much data as possible to enable us to improve our self-driving system. Therefore, we set disengagement thresholds conservatively, and each is carefully recorded. We have an evaluation process in which we identify disengagements that may signal any safety issues, and we resolve them by refining our software, firmware, or hardware and incorporating those changes across our entire fleet.As we continue to develop our technology, the rate of safety significant disengagements has fallen even as we drive more autonomous miles on public roads.Disengagements Covered by This ReportThe DMV rule defines disengagements as deactivations of the autonomous mode in two situations: (1) “when a failure of the autonomous technology is detected,” or (2) “when the safe operation of the vehicle requires that the autonomous vehicle test driver disengage the autonomous mode and take immediate manual control of the vehicle.” In adopting this definition, the DMV noted:1 S ection 227.46 of Article 3.7 (Autonomous Vehicles) of Title 13, Division 1, Chapter 1, California Code of Regulations“This clarification is necessary to ensure that manufacturers are not reporting each common or routine disengagement.”2As part of testing, our cars switch in and out of autonomous mode many times a day. These disengagements number in the many thousands on an annual basis though the vast majority are considered routine and not related to safety. Safety is our highest priority and Google test drivers are trained to take manual control in a multitude of situations, not only when safe operation “requires” that they do so. Our drivers err on the side of caution and take manual control if they have any doubt about the safety of continuing in autonomous mode (for example, due to the behavior of the SDC or any other vehicle, pedestrian, or cyclist nearby), or in situations where other concerns may warrant manual control, such as improving ride comfort or smoothing traffic flow. Similarly, the SDC’s computer hands over control to the driver in many situations that do not involve a “failure of the autonomous technology” and do not require an immediate takeover of control by the driver. We explain more in each relevant section below.Failure of the Autonomous Technology DetectedIn events where the software has detected a technology “failure” -- i.e. an issue with the autonomous technology that may affect the safe operation of the vehicle -- the SDC will immediately hand over control to the driver; we categorize these as “immediate manual control” disengagements. In these cases, the test driver is given a distinct audio and visual signal, indicating that immediate takeover is required.3“Immediate manual control” disengage thresholds are set conservatively. Our objective is not to minimize disengages; rather, it is to gather as much data as possible to enable us to improve our self-driving system. Our self-driving system runs thousands of checks on itself every second. Immediate manual control disengages are triggered primarily when we detect a communication failure between the primary and secondary (back-up) self-driving systems (for example, a broken wire); when we detect anomalies in sensor readings related to our acceleration or position in the world (accelerometers or GPS); or when we detect anomalies in the monitoring of key functions like steering and braking.During the reporting period, Google’s fleet of SDCs experienced 272 such disengagements. Our test drivers are trained and prepared for these events and the average driver response time of all measurable events was 0.84 seconds.As we continue to develop and refine the self-driving software, we are seeing fewer disengagements of this type despite a growing number of miles driven each month (Table 1). The number of autonomous miles we are driving between immediate manual control disengagements is increasing steadily over time. The rate of this type of disengagement has dropped significantly from 785 miles per disengagement in the fourth quarter of 2014 to 5318 miles per disengagement in the fourth quarter of 2015. Figure 1 illustrates this improvement.2 DMV’s Final Statement of Reasons at page 2.3 D uring this testing phase of the software, our SDC hands over control to test drivers on many other occasions that are not “failures” of the autonomous technology. As we calibrate our software and hardware, we closely monitor its performance and alert our drivers and engineers to any minor anomalies.Table 1: Disengagements related to detection of a failure of the autonomous technologyMonthNumberDisengagesAutonomous mileson public roads2014/09 0 4207.22014/10 14 23971.12014/11 14 15836.62014/12 40 9413.12015/01 48 18192.12015/02 12 18745.12015/03 26 22204.22015/04 47 31927.32015/05 9 38016.82015/06 7 42046.62015/07 19 34805.12015/08 4 38219.82015/09 15 36326.62015/10 11 47143.52015/11 6 43275.9Total 272 424331Figure 1: Autonomous miles driven per disengagement related to detection of a failure of the autonomous technologyDisengagements Where Safe Operation of the Vehicle Requires Control by the DriverOur test drivers play a critical role in refining our technology and ensuring the safe operation of the vehicles while we are in this development phase. They are directed to take control of the vehicle as often as they feel is necessary and for a variety of reasons relating to the comfort of the ride, the safety of the vehicle, or the erratic or unpredictable behavior of other road users.Each time a test driver takes manual control of the vehicle, our system automatically records the circumstances leading up to the disengagement from autonomous mode and flags them for review by the software team. This information, along with feedback given by the test driver, is used to evaluate the software for any potential safety issues or areas of improvement, such as making our self-driving car drive more smoothly.To help evaluate the significance of driver disengagements, we employ a powerful simulator program -- developed in-house by our engineers -- that allows the team to “replay” each incident and predict the behavior of the self-driving car (had the driver not taken control of it) as well as the behavior and positions of other road users in the vicinity (such as pedestrians, cyclists, and other vehicles). The simulator can also create thousands of variations on that core event so we can evaluatewhat would have happened under slightly different circumstances, such as our vehicle and other road users moving at different times, speeds, and angles.Through this process we can determine the events that have safety significance and should receive prompt and thorough attention from our engineers in resolving them. In the reporting period, there were 69 events across our fleet in which safe operation of the vehicle required disengagement by the driver.Each of these events is carefully studied to root out the underlying issue or family of issues, and our software is then refined. The revised software is tested extensively, in simulation, on closed courses and on public roads with our test drivers. Even with the vast majority of our autonomous miles being driven in complex city street environments, we only record a few safe operation disengagements each month (Table 2) .Table 2: Driver-initiated disengagements related to safe operation of the vehicleMonthNumberDisengagesAutonomous mileson public roads2014/09 2 4207.22014/10 5 23971.12014/11 7 15836.62014/12 3 9413.12015/01 5 18192.12015/02 2 18745.12015/03 4 22204.22015/04 4 31927.32015/05 4 38016.82015/06 4 42046.62015/07 10 34805.12015/08 3 38219.82015/09 1 36326.62015/10 5 47143.52015/11 10 43275.9Total69 424331Figure 2, below, displays how the number of autonomous miles driven between such disengagements has changed over the calendar quarters covered in the report. The low absolute number of events makes a trend hard to discern because an aberrational month can skew the data.Figure 2: Autonomous miles driven per driver-initiated disengagement related to safe operationof the vehicleOf the 69 reportable safe operation events, 13 were “simulated contacts” -- events in which, upon replaying the event in our simulator, we determined that the test driver prevented our vehicle from making contact with another object. The remaining 56 of the 69 events were safety-significant because, under simulation, we identified some aspect of the SDC’s behavior that could be a potential cause of contacts in other environments or situations if not addressed. This includes proper perception of traffic lights, yielding properly to pedestrians and cyclists, and violations of traffic laws. To be clear, however, these 56 events during the reporting period would very likely not have resulted in a real-world contact if the test driver had not taken over.In 10 of the 13 simulated contact events, the SDC’s predicted behavior would have, in simulation, caused contact (though 2 of these involved simulated contact with traffic cones). In 3 of the 13 occasions, a driver in another vehicle made a move that would have, in simulation, caused a contact with our car (e.g., in one case the other vehicle was driving the wrong way down the road in the SDC’s path); in these cases, we believe a human driver could have taken a reasonable action to avoid the contact but the simulation indicated the SDC would not have taken that action.These events are rare and our engineers carefully study these simulated contacts and refine the software to ensure the self-driving car performs safely. A software “fix” is tested against many miles of simulated driving, then tested on the road, and, after careful review and validation, rolled out to the entire fleet. The rate of these simulated contact disengagements is declining even as autonomous miles driven increase. Because the simulated contact events are so few in number, they do not lend themselves well to trend analysis, but, we are generally driving more autonomous miles between these events. From April 2015 to November 2015, our cars self-drove more than 230,000 miles without a single such event.Table 3: Disengagements related to simulated contacts of the autonomous technologyMonthNumberDisengagesAutonomous mileson public roads2014/09 0 4207.22014/10 2 23971.12014/11 4 15836.62014/12 2 9413.12015/01 1 18192.12015/02 0 18745.12015/03 1 22204.22015/04 1 31927.32015/05 0 38016.82015/06 0 42046.62015/07 0 34805.12015/08 0 38219.82015/09 0 36326.62015/10 0 47143.52015/11 2 43275.9Total 13 424331Summary of All Reportable DisengagementsTable 4 summarizesa ll disengagements required to be reported to the DMV, i.e., both those where a failure of the autonomous technology was detected and those involving drivers taking control when required for safe operation. A brief description of each reportable disengagement is shown in Appendix A.Table 4: All Reportable DisengagementsMonthNumberDisengagesAutonomous mileson public roads2014/09 2 4207.22014/10 19 23971.12014/11 21 15836.62014/12 43 9413.12015/01 53 18192.12015/02 14 18745.12015/03 30 22204.22015/04 51 31927.32015/05 13 38016.82015/06 11 42046.62015/07 29 34805.12015/08 7 38219.82015/09 16 36326.62015/10 16 47143.52015/11 16 43275.9Total 341 424331 Figure 3, below, shows the relationship between all reportable disengagements and the number of autonomous miles driven.Figure 3: Autonomous miles driven per reportable disengagementTable 5 below provides the breakdown of disengagements by cause. Note that, while we have used, where applicable, the causes mentioned in the DMV rule (weather conditions, road surface conditions, construction, emergencies, accidents or collisions), those causes were infrequent in our experience. Far more frequent were the additional causes we have labeled as unwanted maneuver, perception discrepancy, software discrepancy, hardware discrepancy, incorrect behavior prediction, or other road users behaving recklessly.4Table 5: Disengagements by Cause4 O ur cause descriptions reflect the categories of disengagements that our experience has taught us are the most useful for analyzing any underlying issue. “Recklessly behaving road user” indicates that our driver disengaged from autonomous mode to respond to reckless behavior by another driver, cyclist, or pedestrian. “Hardware discrepancy” indicates that a hardware element is not performing as expected. “Unwanted maneuver of the vehicle” involves the SDC moving in a way that is undesirable (e.g., coming uncomfortably close to a parked car). “Perception discrepancy” refers to a situation in which the SDC’s sensors are not correctly perceiving an object (e.g., perceiving overhanging branches as an obstacle). “Incorrect behavior prediction of other traffic participants” involves not correctly predicting the behavior of another road user (e.g., incorrectly predicting that pedestrians on the sidewalk will jaywalk). “Software discrepancy” covers situations involving apparent software inadequacies that do not readily fall into other categories (e.g., map or calibration issues).Cause Sep2014Oct2014Nov2014Dec2014Jan2015Feb2015Mar2015Apr2015May2015Jun2015Jul2015Aug2015Sep2015Oct2015Nov2015Totaldisengage forweatherconditionsduring testing 0 0 0 0 1 5 0 6 0 0 0 0 0 0 1 13 disengage for arecklesslybehaving roaduser 1 0 1 1 1 3 3 7 0 0 0 2 1 0 3 23 disengage forhardwarediscrepancy 0 1 0 0 2 1 0 1 0 5 8 1 8 8 4 39 disengage forunwantedmaneuver ofthe vehicle 0 3 6 14 15 1 3 2 1 0 3 2 0 3 2 55 disengage for aperceptiondiscrepancy 1 2 3 18 19 2 20 30 4 4 8 0 4 3 1 119 disengage forincorrectbehaviorprediction ofother trafficparticipants 0 2 2 0 1 0 2 0 0 0 0 0 0 1 0 8 disengage for asoftwarediscrepancy 0 11 9 9 14 2 1 5 8 2 9 2 3 1 4 80 disengage forconstructionzone duringtesting 0 0 0 1 0 0 1 0 0 0 1 0 0 0 0 3 disengage foremergencyvehicle duringtesting 0 0 0 0 0 0 0 0 0 0 0 0 0 0 1 1 Total 2 19 21 43 53 14 30 51 13 11 29 7 16 16 16 341Table 6 provides information on the location of disengagements covered in this report.Table 6: Disengagements by LocationLocation Sep2014Oct2014Nov2014Dec2014Jan2015Feb2015Mar2015Apr2015May2015Jun2015Jul2015Aug2015Sep2015Oct2015Nov2015TotalInterstate 0 0 0 0 0 0 0 0 0 0 1 0 0 0 0 1 Freeway 0 0 0 0 0 0 0 0 1 0 3 0 0 0 0 4 Highway 0 1 2 0 1 1 4 2 3 2 2 2 5 4 3 32 Street 2 18 19 43 52 13 26 49 9 9 23 5 11 12 13 304 Total 2 19 21 43 53 14 30 51 13 11 29 7 16 16 16 341In its listing of possible disengagement causes, the DMV rule asks each manufacturer to state “whether the disengagement was the result of a planned test of the autonomous vehicle.” All thedisengagements reported here occurred during planned testing of the SDCs. However, if the rule isseeking information on whether the disengagement occurred during planned testing of thedisengagement function itself, we do not test that function on public roads. Instead, we test thefunction in our own facilities during vehicle preparation.Miles Driven by Autonomous VehiclesAppendix Bs hows the total number of miles each autonomous vehicle was tested in autonomous mode on public roads each month. The total miles driven on public roads in Californiaby Google’s fleet during the period, broken down by autonomous and manual modes, is shown inFigure 4.FIgure 4: Miles driven on public roads in California.Time Elapsed Between Technology Failure and Driver Assumption of ControlThe DMV rule requires that our report include in our summary of disengagements the “period of time elapsed from when the autonomous vehicle test driver was alerted of the technology failure and the driver assumed manual control of the vehicle.” This requirement is relevant only to the “technology failure” category of disengagements when the vehicle hands over control to the driver for immediate action. Appendix A shows this elapsed time for each disengagement where the data are available. In the vast majority of cases, the driver took control in one second or less after the immediate manual control message was received. The average time of all measurable events was 0.84 seconds.Appendix ASummary of Each Reportable DisengagementDate Location Type Time to manual CauseSep 2014 Street Safe Operation - Disengage for a perception discrepancy Sep 2014 Street Safe Operation - Disengage for a recklessly behaving agent Oct 2014 Street Safe Operation - Disengage for a perception discrepancy Oct 2014 Street Failure Detection 0.7s Disengage for hardware discrepancyOct 2014 Street Safe Operation - Disengage for incorrect behavior prediction of other traffic participantsOct 2014 Street Failure Detection 0.8s Disengage for unwanted maneuver of the vehicle Oct 2014 Street Failure Detection 0.8s Disengage for unwanted maneuver of the vehicle Oct 2014 Street Failure Detection 0.9s Disengage for a software discrepancyOct 2014 Street Safe Operation - Disengage for a perception discrepancyOct 2014 Highway Failure Detection 0.6s Disengage for a software discrepancyOct 2014 Street Failure Detection 0.9s Disengage for a software discrepancyOct 2014 Street Failure Detection 0.9s Disengage for a software discrepancyOct 2014 Street Failure Detection 1.0s Disengage for a software discrepancyOct 2014 Street Failure Detection 0.6s Disengage for a software discrepancyOct 2014 Street Failure Detection 0.9s Disengage for a software discrepancyOct 2014 Street Failure Detection 0.6s Disengage for a software discrepancyOct 2014 Street Failure Detection 0.6s Disengage for a software discrepancyOct 2014 Street Safe Operation - Disengage for unwanted maneuver of the vehicleOct 2014 Street Safe Operation - Disengage for incorrect behavior prediction of other traffic participantsOct 2014 Street Failure Detection 0.7s Disengage for a software discrepancy Oct 2014 Street Failure Detection * Disengage for a software discrepancy Nov 2014 Street Failure Detection 0.5s Disengage for a software discrepancy Nov 2014 Highway Failure Detection 0.8s Disengage for a software discrepancy Nov 2014 Street Failure Detection 0.7s Disengage for a software discrepancy Nov 2014 Street Failure Detection 0.2s Disengage for a software discrepancy Nov 2014 Street Failure Detection 0.7s Disengage for a software discrepancyNov 2014 Street Safe Operation - Disengage for a perception discrepancyNov 2014 Street Failure Detection 0.2s Disengage for incorrect behavior prediction of other traffic participantsNov 2014 Street Failure Detection 0.8s Disengage for a software discrepancyNov 2014 Street Failure Detection 0.6s Disengage for a software discrepancyNov 2014 Street Safe Operation - Disengage for unwanted maneuver of the vehicleNov 2014 Street Failure Detection * Disengage for incorrect behavior prediction of other traffic participantsNov 2014 Street Safe Operation - Disengage for a recklessly behaving agentNov 2014 Street Failure Detection 0.7s Disengage for unwanted maneuver of the vehicle Nov 2014 Street Safe Operation - Disengage for unwanted maneuver of the vehicle Nov 2014 Street Safe Operation - Disengage for a perception discrepancyNov 2014 Street Failure Detection 0.2s Disengage for unwanted maneuver of the vehicle Nov 2014 Highway Failure Detection 1.1s Disengage for a software discrepancyNov 2014 Street Safe Operation - Disengage for a perception discrepancyNov 2014 Street Failure Detection 2.2s Disengage for unwanted maneuver of the vehicle Nov 2014 Street Safe Operation - Disengage for unwanted maneuver of the vehicle Nov 2014 Street Failure Detection 2.2s Disengage for a software discrepancyDec 2014 Street Failure Detection 0.2s Disengage for a software discrepancyDec 2014 Street Safe Operation - Disengage for unwanted maneuver of the vehicle Dec 2014 Street Failure Detection * Disengage for a software discrepancyDec 2014 Street Failure Detection 1.8s Disengage for unwanted maneuver of the vehicle Dec 2014 Street Failure Detection 0.7s Disengage for unwanted maneuver of the vehicle Dec 2014 Street Failure Detection 0.8s Disengage for unwanted maneuver of the vehicle Dec 2014 Street Failure Detection * Disengage for unwanted maneuver of the vehicle Dec 2014 Street Failure Detection 0.3s Disengage for a perception discrepancyDec 2014 Street Failure Detection 1.2s Disengage for unwanted maneuver of the vehicle Dec 2014 Street Failure Detection 0.8s Disengage for unwanted maneuver of the vehicle Dec 2014 Street Failure Detection 0.3s Disengage for unwanted maneuver of the vehicle Dec 2014 Street Failure Detection 1.1s Disengage for a perception discrepancyDec 2014 Street Failure Detection 1.7s Disengage for a perception discrepancyDec 2014 Street Failure Detection 1.1s Disengage for unwanted maneuver of the vehicle Dec 2014 Street Failure Detection * Disengage for a perception discrepancyDec 2014 Street Failure Detection * Disengage for a perception discrepancyDec 2014 Street Failure Detection 0.3s Disengage for a software discrepancyDec 2014 Street Failure Detection 1.0s Disengage for a perception discrepancyDec 2014 Street Failure Detection * Disengage for a perception discrepancyDec 2014 Street Failure Detection 0.7s Disengage for unwanted maneuver of the vehicle Dec 2014 Street Failure Detection 0.6s Disengage for a perception discrepancyDec 2014 Street Failure Detection * Disengage for a perception discrepancyDec 2014 Street Failure Detection 1.3s Disengage for a perception discrepancyDec 2014 Street Failure Detection 0.4s Disengage for a software discrepancyDec 2014 Street Failure Detection 0.2s Disengage for unwanted maneuver of the vehicle Dec 2014 Street Failure Detection 2.0s Disengage for a perception discrepancyDec 2014 Street Failure Detection 0.8s Disengage for unwanted maneuver of the vehicle Dec 2014 Street Failure Detection 0.8s Disengage for a software discrepancyDec 2014 Street Failure Detection 1.6s Disengage for a software discrepancyDec 2014 Street Failure Detection 0.8s Disengage for a perception discrepancyDec 2014 Street Failure Detection 0.3s Disengage for a software discrepancyDec 2014 Street Failure Detection 1.7s Disengage for unwanted maneuver of the vehicle Dec 2014 Street Failure Detection 0.3s Disengage for unwanted maneuver of the vehicle Dec 2014 Street Failure Detection 0.4s Disengage for a recklessly behaving agentDec 2014 Street Failure Detection 0.2s Disengage for a perception discrepancyDec 2014 Street Failure Detection 1.2s Disengage for a software discrepancyDec 2014 Street Failure Detection * Disengage for a perception discrepancyDec 2014 Street Safe Operation - Disengage for construction zone during testing Dec 2014 Street Safe Operation - Disengage for a perception discrepancyDec 2014 Street Failure Detection 0.6s Disengage for a perception discrepancyDec 2014 Street Failure Detection * Disengage for a perception discrepancyDec 2014 Street Failure Detection 1.3s Disengage for a perception discrepancyJan 2015 Street Failure Detection 1.9s Disengage for unwanted maneuver of the vehicle Jan 2015 Street Failure Detection * Disengage for a perception discrepancyJan 2015 Street Failure Detection 0.2s Disengage for a perception discrepancyJan 2015 Street Failure Detection * Disengage for a perception discrepancyJan 2015 Street Failure Detection 0.2s Disengage for a perception discrepancyJan 2015 Street Failure Detection 0.5s Disengage for a software discrepancyJan 2015 Street Failure Detection 0.3s Disengage for unwanted maneuver of the vehicle Jan 2015 Street Failure Detection 0.3s Disengage for a perception discrepancyJan 2015 Street Failure Detection 0.8s Disengage for a software discrepancyJan 2015 Street Failure Detection 0.3s Disengage for unwanted maneuver of the vehicle Jan 2015 Street Failure Detection 0.8s Disengage for a perception discrepancyJan 2015 Street Failure Detection 0.5s Disengage for unwanted maneuver of the vehicle Jan 2015 Street Failure Detection 0.7s Disengage for a perception discrepancyJan 2015 Street Failure Detection * Disengage for adverse road surface conditions such as road holes or bumpsJan 2015 Street Failure Detection 0.4s Disengage for unwanted maneuver of the vehicle Jan 2015 Street Failure Detection 0.3s Disengage for a perception discrepancyJan 2015 Street Failure Detection 0.7s Disengage for unwanted maneuver of the vehicle Jan 2015 Street Failure Detection * Disengage for a software discrepancyJan 2015 Street Failure Detection 0.3s Disengage for a perception discrepancyJan 2015 Street Failure Detection 1.0s Disengage for a software discrepancyJan 2015 Street Failure Detection 0.4s Disengage for unwanted maneuver of the vehicle Jan 2015 Street Failure Detection 1.4s Disengage for a perception discrepancyJan 2015 Street Failure Detection 1.9s Disengage for a perception discrepancyJan 2015 Street Failure Detection 0.3s Disengage for unwanted maneuver of the vehicle Jan 2015 Street Failure Detection 0.2s Disengage for a software discrepancyJan 2015 Street Failure Detection 0.2s Disengage for a software discrepancyJan 2015 Street Failure Detection 1.0s Disengage for a software discrepancyJan 2015 Street Failure Detection 2.0s Disengage for a software discrepancyJan 2015 Street Failure Detection 0.2s Disengage for unwanted maneuver of the vehicle Jan 2015 Street Failure Detection 0.8s Disengage for a perception discrepancyJan 2015 Highway Safe Operation - Disengage for a recklessly behaving agentJan 2015 Street Failure Detection 0.2s Disengage for a perception discrepancyJan 2015 Street Safe Operation - Disengage for incorrect behavior prediction of other traffic participantsJan 2015 Street Failure Detection 0.3s Disengage for a software discrepancyJan 2015 Street Failure Detection 1.4s Disengage for unwanted maneuver of the vehicle Jan 2015 Street Failure Detection 1.3s Disengage for unwanted maneuver of the vehicle Jan 2015 Street Failure Detection 0.9s Disengage for unwanted maneuver of the vehicle Jan 2015 Street Failure Detection * Disengage for unwanted maneuver of the vehicle Jan 2015 Street Failure Detection * Disengage for a perception discrepancyJan 2015 Street Failure Detection 0.6s Disengage for a software discrepancy。
无人驾驶

2019年四季度上市
蔚来ES8
蔚来对产品的定义,ES8 将拥有特斯拉的性 能、雷克萨斯的质量以及接近汉兰达的价 格. 蔚来宣布 ES8 价格,基准版补贴前为 44.8 万价格,创始版补贴前价格为 54.8 万元。 ES8 基于电池租用方案价格,基准版补贴前 为 34.8 万价格,创始版补贴前价格为 44.8 万元。 ES8 是全球首个安装Mobileye EyeQ4芯片的 车型,EyeQ4的处理能力是EyeQ3的8倍,平 均功耗5瓦,响应时间20毫秒。 蔚来加入了国家电网的超级充电(2017年 12月31日总数42000根,2020年预计12万 根),都可以为蔚来的车型进行充电。3分 钟换电池。 2017年12月16日上市,ES8,7座SUV
0月底,与金龙合作率先实现无人驾 驶小巴车的小规模量产及试运营,并在 2019年与江淮、北汽,2020年与奇7.24
22015.12.10无人驾驶汽车项目启动无人车进行了第一次路测
3
2016.9.1
42017.04.17无人车获得美国加州 政府颁发的全球第 15 张无 人车上路测试牌照。
042015.12.10 无人车第一次路测18无人车的优势
6、节省时间:开车时间&拥堵时间 麦肯锡公司估计,无人驾驶汽车每天为全球司机节省的 时间总和高达10亿个小时。 整个城市都依靠导航地图来运行的场景。汽车之间会相 互合作,改道出行避免堵车。堵车将成为过去时,人们能 更快到达目的地。
智能汽车无人驾驶原理知识培训
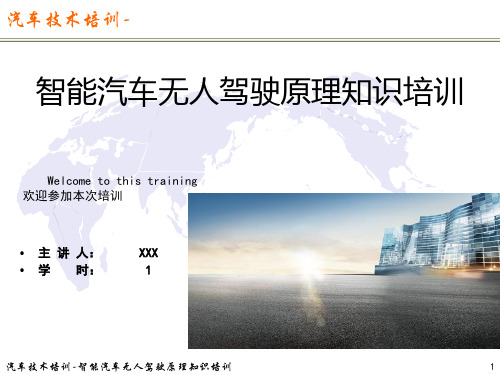
3、光测距系统 LIDAR
① 谷歌采用了Velodyne公司的车顶激光测距系统。 扫描器发射64束覆盖汽车周围360°角内的区域距 离可以精确到2cm以内的激光射线,然后激光碰到 车辆周围的物体,又反射回来,这样就计算出了物 体的距离。
② 另一套在底部的系统测量出车辆在三个方向上的加 速度、角速度等数据,然后再结合GPS数据计算出 车辆的位置,所有这些数据与车载摄像机捕获的图 像一起输入计算机,软件以极高的速度处理这些数 据。这样,系统就可以非常迅速的作出判断。
汽车技术培训-
19
汽车技术培训-
THANKS
汽车技术培训-智能汽车无人驾驶原理知识培训20源自Part汽车技术培训-
6、全球定位系统GPS
① 一个自动驾驶员需要知道他正在去哪儿。谷歌使用Applanix公司的定位 系统,以及他们自己的研制开发Google Map和GPS 技术。
汽车技术培训-智能汽车无人驾驶原理知识培训
16
3
Part
7、轮角度编码器 Wheel Encoder
① 轮载传感器可以在Google汽车穿梭于车流中 时测量它的速度。进而自动调节汽车的行驶 速度。帮助汽车在地图上找到准确的位置。
2
1 无人驾驶概述
Part
汽车技术培训-
无人驾驶汽车是一种智能汽车,也可以称之为轮式移动机器人,主要依靠车 内的以计算机系统为主的智能驾驶仪来实现无人驾驶。
无人驾驶汽车是通过车载传感系统感知道路环境,自动规划行车路线并控制 车辆到达预定目标的智能汽车。
无人驾驶汽车集自动控制、体系结构、人工智能、视觉计算等众多技术于一 体,是计算机科学、模式识别和智能控制技术高度发展的产物,也是衡量一 个国家科研实力和工业水平的一个重要标志,在国防和国民经济领域具有广 阔的应用前景 。
未卜先知的谷歌:15年前的14个先见之明
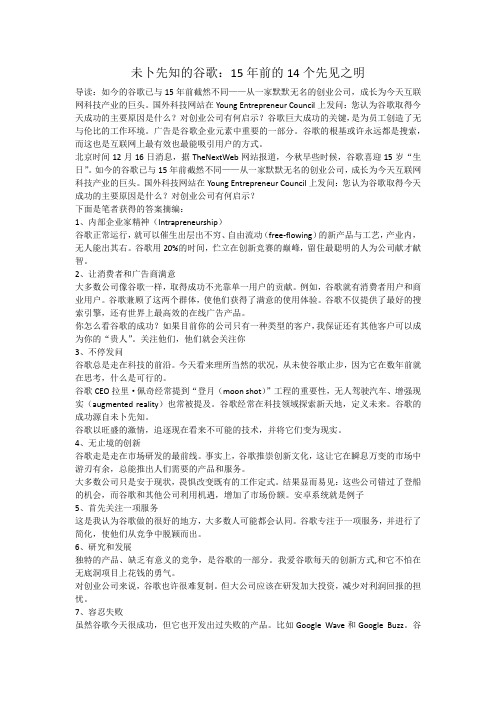
未卜先知的谷歌:15年前的14个先见之明导读:如今的谷歌已与15年前截然不同——从一家默默无名的创业公司,成长为今天互联网科技产业的巨头。
国外科技网站在Young Entrepreneur Council上发问:您认为谷歌取得今天成功的主要原因是什么?对创业公司有何启示?谷歌巨大成功的关键,是为员工创造了无与伦比的工作环境。
广告是谷歌企业元素中重要的一部分。
谷歌的根基或许永远都是搜索,而这也是互联网上最有效也最能吸引用户的方式。
北京时间12月16日消息,据TheNextWeb网站报道,今秋早些时候,谷歌喜迎15岁“生日”。
如今的谷歌已与15年前截然不同——从一家默默无名的创业公司,成长为今天互联网科技产业的巨头。
国外科技网站在Young Entrepreneur Council上发问:您认为谷歌取得今天成功的主要原因是什么?对创业公司有何启示?下面是笔者获得的答案摘编:1、内部企业家精神(Intrapreneurship)谷歌正常运行,就可以催生出层出不穷、自由流动(free-flowing)的新产品与工艺,产业内,无人能出其右。
谷歌用20%的时间,伫立在创新竞赛的巅峰,留住最聪明的人为公司献才献智。
2、让消费者和广告商满意大多数公司像谷歌一样,取得成功不光靠单一用户的贡献。
例如,谷歌就有消费者用户和商业用户。
谷歌兼顾了这两个群体,使他们获得了满意的使用体验。
谷歌不仅提供了最好的搜索引擎,还有世界上最高效的在线广告产品。
你怎么看谷歌的成功?如果目前你的公司只有一种类型的客户,我保证还有其他客户可以成为你的“贵人”。
关注他们,他们就会关注你3、不停发问谷歌总是走在科技的前沿。
今天看来理所当然的状况,从未使谷歌止步,因为它在数年前就在思考,什么是可行的。
谷歌CEO拉里·佩奇经常提到“登月(moon shot)”工程的重要性,无人驾驶汽车、增强现实(augmented reality)也常被提及。
- 1、下载文档前请自行甄别文档内容的完整性,平台不提供额外的编辑、内容补充、找答案等附加服务。
- 2、"仅部分预览"的文档,不可在线预览部分如存在完整性等问题,可反馈申请退款(可完整预览的文档不适用该条件!)。
- 3、如文档侵犯您的权益,请联系客服反馈,我们会尽快为您处理(人工客服工作时间:9:00-18:30)。
Activity Summary (all metrics are as of December 31, 2015)
Vehicles
●23 Lexus RX450h SUVs – currently self-driving on public streets; 18 in Mountain View, CA, 5 in Austin, TX
●30 prototypes – currently self-driving on public streets; 23 in Mountain View, CA & 7 in Austin, TX
Miles driven since start of project in 2009
“Autonomous mode” means the software is driving the vehicle, and test drivers are not touching the manual controls. “Manual mode” means the test drivers are driving the car.
●Autonomous mode: 1,372,111 miles
●Manual mode: 970,390 miles
●We’re currently averaging 10,000-15,000 autonomous miles per week on public streets
Sensing in the rain. The limits of self-driving in sunny California.
After a multi-year drought, we’re finally starting to get some rain in California. It’s not only a welcome relief for farmers and gardeners, but an opportunity for our cars to get more time learning in cold and rainy weather. Driving in rain makes many human drivers nervous due to reduced visibility, and some of our sensors -- particularly the cameras and lasers -- have to deal with similar issues. For example, we’ve had to come up with our own equivalent of a windscreen wiper on the dome to ensure our sensors have the best view possible. Our laser sensors are able to detect rain, so we have to teach our cars to see through the raindrops and clouds of exhaust on cold mornings, and continue to properly detect objects. We’re helped by our diversity of sensors, since our radars have no problem seeing through this sort of clutter.
As we’re developing the technology, we've made sure our
cars are aware of how rain may affect their ability to drive.
Our cars can determine the severity of the rain, and just
like human drivers they drive more cautiously in wet
conditions when roads are slippery and visibility is poor.
For now, if it’s particularly stormy, our cars automatically
pull over and wait until conditions improve (and of course,
our test drivers are always available to take over). To
explore even more challenging environments, we’re
beginning to collect data in all sorts of rainy and snowy
conditions as we work toward the goal of a self-driving car
that will be able to drive come rain, hail, snow or shine!
Traffic Accidents Reported to CA DMV
None for the month of December.
What we’ve been reading
●The Atlantic, “The High-Stakes Race to Rid the World of Human Drivers
”, (December 2015)
●The Atlantic: “D riverless Cars Are Like Elevators
”, (December 2015)
●Associated Press: “U S officials signal move toward embracing self-driving cars
”, (December 2015)
●San Jose Mercury News:
”, (December 2015)
Q uinn: The DMV puts a brake on our transportation future
“
●Fortune: “E lon Musk Says Tesla Vehicles Will Drive Themselves in Two Years”
, (December 2015)。