圆
圆的问题专题

专题-圆的问题专题知识回顾一、与圆有关的概念与规律1.圆:平面上到定点的距离等于定长的所有点组成的图形叫做圆。
定点称为圆心,定长称为半径。
圆的半径或直径决定圆的大小,圆心决定圆的位置。
2.圆的性质:(1)圆具有旋转不变性;(2)圆具有轴对称性;(3)圆具有中心对称性。
3.垂径定理:垂直于弦的直径平分弦,并且平分弦所对的两条弧。
4.推论:平分弦(不是直径)的直径垂直于弦,并且平分弦所对的两条弧.5.圆心角:顶点在圆心上的角叫做圆心角。
圆心角的度数等于它所对弧的度数。
6.在同圆或等圆中,相等的圆心角所对的弧相等,所对的弦相等,所对的弦心距也相等。
在同圆或等圆中,如果两条弧相等,那么他们所对的圆心角相等,所对的弦相等,所对的弦心距也相等。
在同圆或等圆中,如果两条弦相等,那么他们所对的圆心角相等,所对的弧相等,所对的弦心距也相等。
7.圆周角:顶点在圆周上,并且两边分别与圆相交的角叫做圆周角。
8.在同圆或等圆中,同弧或等弧所对的圆周角相等,都等于这条弧所对的圆心角的一半.9.半圆(或直径)所对的圆周角是直角,90°的圆周角所对的弦是直径.10. 点和圆的位置关系:① 点在圆内点到圆心的距离小于半径② 点在圆上点到圆心的距离等于半径③ 点在圆外点到圆心的距离大于半径11. 过三点的圆:不在同一直线上的三个点确定一个圆。
12. 外接圆和外心:经过三角形的三个顶点可以做一个圆,这个圆叫做三角形的外接圆。
外接圆的圆心,叫做三角形的外心。
外心是三角形三条边垂直平分线的交点。
外心到三角形三个顶点的距离相等。
13.若四边形的四个顶点都在同一个圆上,这个四边形叫做圆内接四边形,这个圆叫做这个四边形的外接圆。
14.圆内接四边形的特征:⇔⇔⇔①圆内接四边形的对角互补;②圆内接四边形任意一个外角等于它的内对角。
15.直线与圆有3种位置关系:如果⊙O 的半径为r ,圆心O 到直线的距离为d ,那么① 直线和⊙O 相交;② 直线和⊙O 相切;③ 直线和⊙O 相离。
第一章 圆(讲义)(含答案)
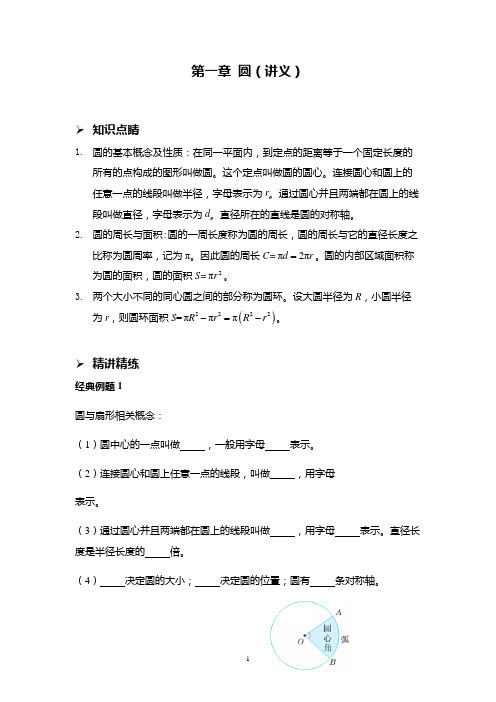
第一章圆(讲义)➢知识点睛1.圆的基本概念及性质:在同一平面内,到定点的距离等于一个固定长度的所有的点构成的图形叫做圆。
这个定点叫做圆的圆心。
连接圆心和圆上的任意一点的线段叫做半径,字母表示为r。
通过圆心并且两端都在圆上的线段叫做直径,字母表示为d。
直径所在的直线是圆的对称轴。
2.圆的周长与面积:圆的一周长度称为圆的周长,圆的周长与它的直径长度之比称为圆周率,记为π。
因此圆的周长C=rπ=。
圆的内部区域面积称dπ2为圆的面积,圆的面积S=2πr。
3.两个大小不同的同心圆之间的部分称为圆环。
设大圆半径为R,小圆半径为r,则圆环面积S=()2222-=-。
R r R rπππ➢精讲精练经典例题1圆与扇形相关概念:(1)圆中心的一点叫做,一般用字母表示。
(2)连接圆心和圆上任意一点的线段,叫做,用字母表示。
(3)通过圆心并且两端都在圆上的线段叫做,用字母表示。
直径长度是半径长度的倍。
(4)决定圆的大小;决定圆的位置;圆有条对称轴。
(5)图中涂色部分是一个。
圆上A、B两点之间的部分叫做。
顶点在圆心,两条半径组成的∠AOB,叫做。
(6)圆的周长式:;圆的面积公式:。
经典例题2(1)图中圆的周长是多少?圆的面积是多少?(单位:厘米,π取3.14)(2)下图的周长及面积分别是多少?(π取3.14,单位:米)经典例题3计算下图涂色部分的面积。
(π取3.14)经典例题4如图,有五个同心圆的半径分别是1、2、3、4、5,求图中阴影部分的面积。
(π取3.14)经典例题5如图是圆环的一半,面积是28.26平方厘米,那么图形的周长是多少?(π取3.14)【参考答案】经典例题1:(1)圆心,O(2)半径,r(3)直径,d ,2(4)半径,圆心,无数(5)扇形,弧AB ,圆心角(6)C =π2πd r ,S =2πr经典例题2:(1)周长:94.2cm ,面积:706.52cm(2)周长:40.56米,面积:105.12平方米经典例题3:84.78经典例题4:47.1经典例题5:24.84。
圆的16个公式
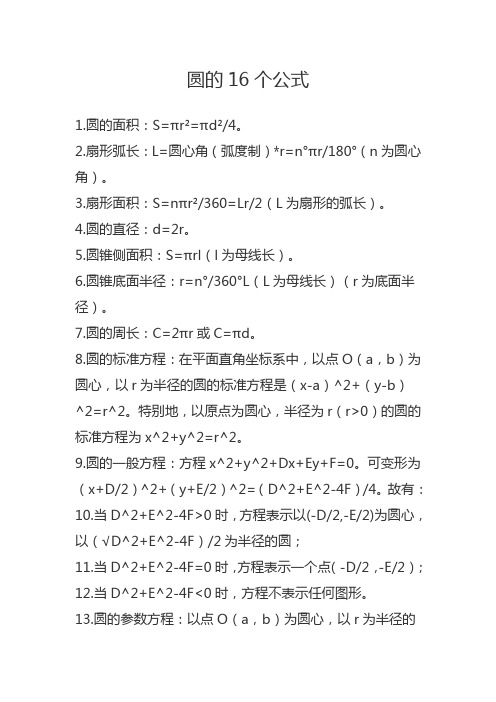
圆的16个公式1.圆的面积:S=πr²=πd²/4。
2.扇形弧长:L=圆心角(弧度制)*r=n°πr/180°(n为圆心角)。
3.扇形面积:S=nπr²/360=Lr/2(L为扇形的弧长)。
4.圆的直径:d=2r。
5.圆锥侧面积:S=πrl(l为母线长)。
6.圆锥底面半径:r=n°/360°L(L为母线长)(r为底面半径)。
7.圆的周长:C=2πr或C=πd。
8.圆的标准方程:在平面直角坐标系中,以点O(a,b)为圆心,以r为半径的圆的标准方程是(x-a)^2+(y-b)^2=r^2。
特别地,以原点为圆心,半径为r(r>0)的圆的标准方程为x^2+y^2=r^2。
9.圆的一般方程:方程x^2+y^2+Dx+Ey+F=0。
可变形为(x+D/2)^2+(y+E/2)^2=(D^2+E^2-4F)/4。
故有:10.当D^2+E^2-4F>0时,方程表示以(-D/2,-E/2)为圆心,以(√D^2+E^2-4F)/2为半径的圆;11.当D^2+E^2-4F=0时,方程表示一个点(-D/2,-E/2);12.当D^2+E^2-4F<0时,方程不表示任何图形。
13.圆的参数方程:以点O(a,b)为圆心,以r为半径的圆的参数方程是x=a+r*cosθ,y=b+r*sinθ,(其中θ为参数)。
14.圆的端点式:若已知两点A(a1,b1),B(a2,b2),则以线段AB为直径的圆的方程为(x-a1)(x-a2)+(y-b1)(y-b2)=0。
15.圆的离心率e=0,在圆上任意一点的半径都是r。
16.经过圆x^2+y^2=r^2上一点M(a0,b0)的切线方程为a0*x+b0*y=r^2。
圆的知识点总结

圆的相关知识点1、圆心:圆中心一点叫做圆心。
用字母“O"来表示。
半径:连接圆心和圆上任意一点的线段叫做半径,用字母“r"来表示.画圆时,圆规两脚间的距离就是半径.直径:通过圆心并且两端都在圆上的线段叫做直径,用字母“d”表示。
直径是圆中最长的线段。
2.圆心确定圆的位置,半径确定圆的大小。
圆是轴对称图形,直径所在的直线是圆的对称轴。
3.在同一个圆内,所有的半径都相等,所有的直径都相等。
在同一个圆内,有无数条半径,有无数条直径。
在同一个圆内,直径的长度是半径的2倍,半径的长度是直径的一半。
用字母表示为:d=2r r =d÷24、正方形中画最大的圆:先画正方形的两条对角线,交点就是圆心,再以边长的一半作半径画圆.边长也就是圆的直径。
5、圆中画最大的正方形:先画两条互相垂直的直径,直径和圆相交的四个点连接起来就成了一个圆。
在长方形中画最大的圆,宽就是圆的直径。
6、扇形:由两条半径和一段弧围成的图形就是扇形.顶点在圆心的角是圆心角。
圆上两点间的一段叫弧。
7、在同一个圆中,扇形的大小与圆心角的大小有关.在不同的圆中,扇形的大小与圆心角的大小和半径的长短有关。
8.圆的周长:围成圆的曲线的长度叫做圆的周长。
圆的周长总是直径的3倍多一些,这个比值是一个固定的数.我们把圆的周长和直径的比值叫做圆周率,用字母π表示。
圆周率是一个无限不循环小数.在计算时,π取3。
14。
世界上第一个把圆周率算出来的人是我国的数学家祖冲之.周长是直径的π倍,是半径的2π倍。
6.圆的周长公式:C=πd 或C=2πr 周长等于直径乘π,等于半径乘2π。
直径等于周长除以π,或等于半径乘2,半径等于周长除以π再除以2,或等于直径除以2。
圆的直径、半径扩大或缩小几倍,周长也扩大或缩小相同的倍数,周长、直径、半径间的变化相同。
两个圆的直径、半径和周长之间的倍数关系完全相同。
7、圆的面积:圆所占平面的大小叫圆的面积.8.把一个圆割拼成一个近似的长方形,割拼成的长方形的长相当于圆周长的一半,宽相当于圆的半径,因为长方形面积=长×宽,所以圆的面积= πr×r=πr²,要求圆的面积必须知道圆的半径(或知道半径的平方)。
关于圆的知识点
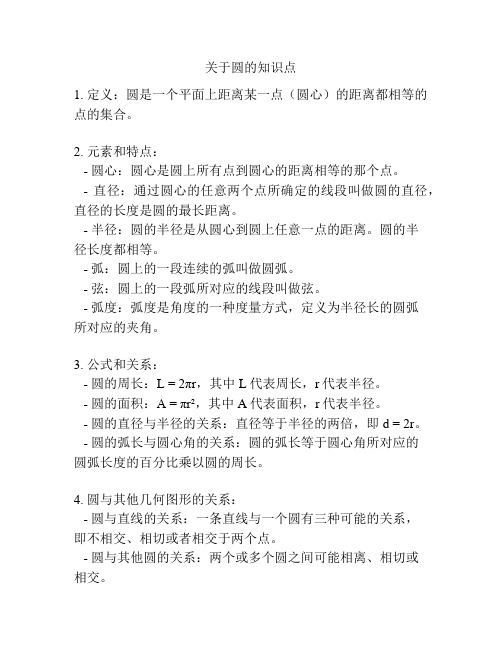
关于圆的知识点
1. 定义:圆是一个平面上距离某一点(圆心)的距离都相等的点的集合。
2. 元素和特点:
- 圆心:圆心是圆上所有点到圆心的距离相等的那个点。
- 直径:通过圆心的任意两个点所确定的线段叫做圆的直径,直径的长度是圆的最长距离。
- 半径:圆的半径是从圆心到圆上任意一点的距离。
圆的半
径长度都相等。
- 弧:圆上的一段连续的弧叫做圆弧。
- 弦:圆上的一段弧所对应的线段叫做弦。
- 弧度:弧度是角度的一种度量方式,定义为半径长的圆弧
所对应的夹角。
3. 公式和关系:
- 圆的周长:L = 2πr,其中L代表周长,r代表半径。
- 圆的面积:A = πr²,其中A代表面积,r代表半径。
- 圆的直径与半径的关系:直径等于半径的两倍,即d = 2r。
- 圆的弧长与圆心角的关系:圆的弧长等于圆心角所对应的
圆弧长度的百分比乘以圆的周长。
4. 圆与其他几何图形的关系:
- 圆与直线的关系:一条直线与一个圆有三种可能的关系,
即不相交、相切或者相交于两个点。
- 圆与其他圆的关系:两个或多个圆之间可能相离、相切或
相交。
这些是关于圆的基本知识点,可以帮助我们理解和解决与圆相关的问题。
圆的意义和特点

圆的意义和特点
一、圆的意义
1、理想:圆是一个完美的图形,它代表着完美无缺、统一完整,也象征着未来永恒的社会价值,代表着理想的力量。
2、和谐:圆的状态更具多元和谐的特性,象征着乐观有希望的未来,鼓舞着我们努力追求完美的社会。
3、虚无:圆形也代表世间万物的恒久,它体现出空无的真谛,象征着宇宙之无限,提醒我们要懂得把握生活的虚无本质以及学会珍惜身边的每一份礼物。
4、自由:圆形更象征着一种自由的生活方式,它传递着宽容他人的精神,鼓励每个人要平等自由的生活。
二、圆的特点
1、统一:圆形的边缘没有明显的起伏,所有的点都处于某种统一的状态,从而构成一个完整的圆形。
2、完美:每一个圆的状态,都处于完美程度的状态,这表明了一种高贵无比的气质和心态,更展示出完美的审美情趣。
3、动态:圆的状态是一种极具变化的状态,不断改变的空间和时间,它演化出不同的美丽,象征着一种优秀的平衡和活力。
4、平等:圆形的每一个点都处于同等的距离,这体现了一种平等的意义,鼓励我们要以一种平等的心态去看待世界。
圆组词造句
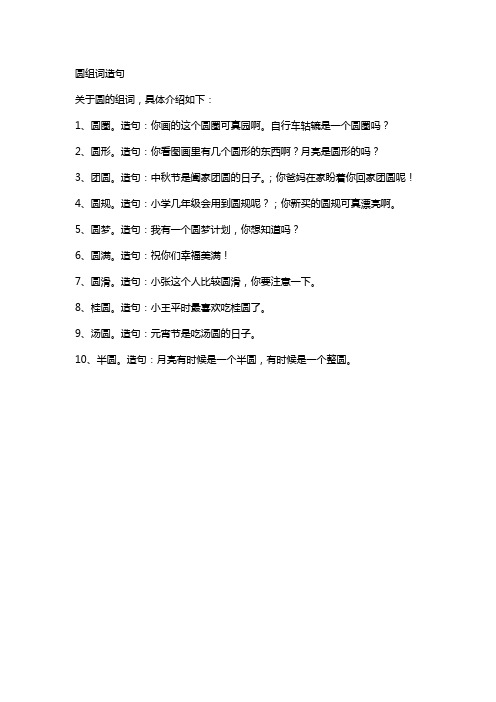
圆组词造句
关于圆的组词,具体介绍如下:
1、圆圈。
造句:你画的这个圆圈可真园啊。
自行车轱辘是一个圆圈吗?
2、圆形。
造句:你看图画里有几个圆形的东西啊?月亮是圆形的吗?
3、团圆。
造句:中秋节是阖家团圆的日子。
;你爸妈在家盼着你回家团圆呢!
4、圆规。
造句:小学几年级会用到圆规呢?;你新买的圆规可真漂亮啊。
5、圆梦。
造句:我有一个圆梦计划,你想知道吗?
6、圆满。
造句:祝你们幸福美满!
7、圆滑。
造句:小张这个人比较圆滑,你要注意一下。
8、桂圆。
造句:小王平时最喜欢吃桂圆了。
9、汤圆。
造句:元宵节是吃汤圆的日子。
10、半圆。
造句:月亮有时候是一个半圆,有时候是一个整圆。
圆组词_圆的组词_圆字组词.doc
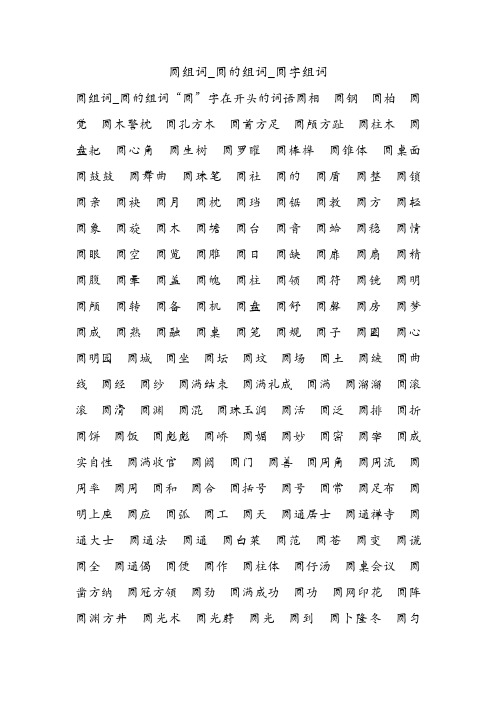
圆组词_圆的组词_圆字组词圆组词_圆的组词“圆”字在开头的词语圆相圆钢圆柏圆觉圆木警枕圆孔方木圆首方足圆颅方趾圆柱木圆盘耙圆心角圆生树圆罗曜圆棒榫圆锥体圆桌面圆鼓鼓圆舞曲圆珠笔圆社圆的圆盾圆整圆锁圆亲圆袂圆月圆枕圆珰圆锯圆教圆方圆轻圆象圆旋圆木圆蟾圆台圆音圆蛤圆稳圆情圆眼圆空圆览圆雕圆日圆缺圆扉圆扇圆精圆腹圆晕圆盖圆魄圆柱圆领圆符圆镜圆明圆颅圆转圆备圆机圆盘圆舒圆磬圆房圆梦圆成圆熟圆融圆桌圆笼圆规圆子圆圈圆心圆明园圆城圆坐圆坛圆坟圆场圆土圆绫圆曲线圆经圆纱圆满结束圆满礼成圆满圆溜溜圆滚滚圆滑圆渊圆混圆珠玉润圆活圆泛圆排圆折圆饼圆饭圆彪彪圆峤圆媚圆妙圆密圆宰圆成实自性圆满收官圆阙圆门圆善圆周角圆周流圆周率圆周圆和圆合圆括号圆号圆常圆足布圆明上座圆应圆弧圆工圆天圆通居士圆通禅寺圆通大士圆通法圆通圆白菜圆范圆苍圆变圆谎圆全圆通偈圆便圆作圆柱体圆仔汤圆桌会议圆凿方纳圆冠方领圆劲圆满成功圆功圆网印花圆阵圆渊方井圆光术圆光蔚圆光圆到圆卜隆冬圆匀圆美圆丽圆滑世故圆常无圆觉三观圆丘草圆丘圆乎乎“圆”字在结尾的词语踏圆簿圆旋圆转圆破镜难圆缺月重圆刓方爲圆青解毒圆月缺重圆预知子圆家好月圆青木香圆言方行圆月缺难圆税外方圆内方外圆橘瓣鱼圆字正腔圆破镜重圆的皪圆胜金圆外切圆梅月圆比金圆虎睛圆的的圆鸭蛋圆规圆高圆肉圆水圆蜡圆轻圆窝圆环圆鱼圆龙圆蹴圆肥圆踢圆松圆穹圆走圆上圆香圆青圆桂圆椭圆扁圆长圆重圆银圆复圆围圆团团圆圆合家团圆骨肉团圆回圆地格方圆缩砂圆缚虎圆滴圆满圆的溜圆溜圆滚瓜溜圆渫白圆清圆消食圆消暑圆玉润珠圆空心汤圆泡汤圆汤圆外接圆指方画圆贰拾圆毁方投圆拟规画圆扒圆破饮圆煨姜圆如神圆好梦难圆阖家团圆膀阔腰圆和胃圆同心圆幅圆戊已圆神应圆广圆弄圆开胃圆平圆狗头骨圆天心月圆大已寒圆膀大腰圆大惊圆大团圆大圆对圆寸金圆通圆连翘圆月边圆御圆香薷圆白薇圆蓬煎圆山药汤圆紫石英圆花好月圆玉芝圆诜诜圆卖镜重圆半圆偏圆顺倾转圆面面俱圆体规画圆润体圆人月圆行满功圆神功圆缺月再圆外方内圆随方就圆百部圆防风圆匾圆光圆滚瓜儿圆剔团圆内切圆包圆匀圆美圆十五月亮十六圆八圆破觚为圆面为底圆削方为圆刓方为圆破矩为圆斫方为圆底为面圆磨盘两圆菟丝子圆不以规矩,不能成方圆不以规矩,不成方圆明月不常圆不二圆不圆无规矩不成方圆万病圆七子团圆小丁香圆一裹圆一圆乌荆圆乌圆事缓则圆事寛即圆小理中圆“圆”字在中间的词语方圆几里虎目圆睁文圆质方规圆矩方方圆百里怒目圆睁方领圆冠方圆殊趣怒目圆瞪智圆行方椭圆体走圆场跑圆场滚圆溜胖珠圆玉滑珠圆玉润浑圆球肾沥圆方打圆场戴圆履方月圆花好镜圆璧合打圆台方圆可施纱帽圆领方底圆盖右手画圆,左手画方科头圆子大圆镜智大圆智团圆节谈圆说通地圆说重圆记方圆十里做圆活幼圆体凿枘圆方方枘圆凿凿圆枘方功行圆满功德圆满外圆内方卵圆孔蛾眉倒蹙,凤眼圆睁竹刻圆雕刻圆仪划圆防守金圆券包圆儿自圆其说八面圆通世故圆滑方圆难周,异道不安万枘圆凿诸事圆满处事圆滑。
- 1、下载文档前请自行甄别文档内容的完整性,平台不提供额外的编辑、内容补充、找答案等附加服务。
- 2、"仅部分预览"的文档,不可在线预览部分如存在完整性等问题,可反馈申请退款(可完整预览的文档不适用该条件!)。
- 3、如文档侵犯您的权益,请联系客服反馈,我们会尽快为您处理(人工客服工作时间:9:00-18:30)。
Circle detection on images using genetic algorithmsVictor Ayala-Ramirez *,Carlos H.Garcia-Capulin,Arturo Perez-Garcia,Raul E.Sanchez-YanezUniversidad de Guanajuato FIMEE,Electronics and Communications Engineering Department,Tampico 912,Colonia Bellavista,Guanajuato,36730Salamanca,MexicoReceived 23December 2004;received in revised form 6October 2005Available online 28November 2005Communicated by G.BorgeforsAbstractIn this paper,we present a circle detection method based on genetic algorithms.Our genetic algorithm uses the encoding of three edge points as the chromosome of candidate circles (x ,y ,r )in the edge image of the scene.Fitness function evaluates if these candidate circles are really present in the edge image.Our encoding scheme reduces the search space by avoiding trying unfeasible individuals,this results in a fast circle detector.Our approach detects circles with sub-pixellic accuracy on synthetic images.Our method can also detect circles on natural images with sub-pixellic precision.Partially occluded circles can be located in both synthetic and natural images.Examples of the application of our method to the recognition of hand-drawn circles are also shown.Detection of several circles in a single image is also handled by our method.Ó2005Elsevier B.V.All rights reserved.Keywords:Object recognition;Circle detection;Genetic algorithms;Hand-drawn shape location1.IntroductionShape detection is needed in many computer vision tasks because shape is an important cue for modelling objects in scenes (da Fontoura Costa and Marcondes Cesar Jr.,2001).Object location problems are mainly solved by two types of techniques:In one hand,deterministic techniques include application of Hough transform based methods (for example,Yuen et al.,1990),geometric hashing and template or model matching techniques (Iivarinen et al.,1997;Jones et al.,1990).In the other hand,stochastic techniques include random sample consensus techniques (Fischer and Bolles,1981),simulated annealing and genetic algorithms (GA)(Roth and Levine,1994).Template and model matching techniques were the first approaches to shape detection.Shape coding techniquesand combination of shape properties were used to repre-sent objects.Plenty of methods have been developed to solve the shape detection problem (Peura and Iivarinen,1997).Main drawback of these techniques is related to the contour extraction step from real images.Additionally,it is difficult for models to deal with pose invariance except maybe for very simple objects.Hough transform based methods (Muammar and Nixon,1989)have shown to be robust enough to deal with noisy images.However,Hough transform techniques are not efficient for high dimensional problems because they are computationally or memory intensive even if they have a robust behavior.In order to overcome these problems,some other researchers have proposed new approaches to the Hough transform.We can find in the literature,for example,the probabilistic Hough transform (Shaked et al.,1996),the randomized Hough transform (RHT)(Xu et al.,1990),the fuzzy Hough transform (Han et al.,1993).We can even find alternative transforms as the pro-posed by Becker et al.(2002).0167-8655/$-see front matter Ó2005Elsevier B.V.All rights reserved.doi:10.1016/j.patrec.2005.10.003*Corresponding author.Tel.:+524646480911;fax:+524646472400.E-mail address:ayalav@salamanca.ugto.mx (V.Ayala-Ramirez)./locate/patrecStochastic techniques can attain a good solution quickly without the needing of high memory or computation power requirements.Nevertheless,they can not guarantee tofind an optimal solution.Genetic programming,in particular GA,are used to optimize afitness function by mimicking natural evolution for organisms.Individuals for this evolu-tion are computational representations of potential solu-tions for the problem to be solved.Each individual is represented as a binary string also known as a computa-tional chromosome.The entire set of individuals examined at a time is called the population.In particular,genetic algorithms have been recently used for some shape detec-tion tasks:Roth and Levine(1993,1994)has proposed to use GA for primitive extraction on images.A further improvement on Roth approach has been presented by Lutton et al.(1994).Yuen and Ma(2000)uses GA for tem-plate matching when the pattern has been the subject of an unknown affine transformation.Circle and ellipse detection problem has attracted many researchers.Most of them use Hough transform based tech-niques.For example,Lam and Yuen(1996)propose an approach based on hypothesisfiltering and Hough trans-forms to detect circles.Yuen and Lo(1994)propose to use a multi-resolution approach for the circle detection prob-lem.Mainzer(2002a,b)uses circle detection in a traffic sign detector.Rosin and Nyongesa(2000)use a soft computing approach to shape classification.For the ellipses,Rosin pro-poses an ellipsefitting algorithm that usesfive points(Rosin et al.,1997)and he has extended tofit data on superellipses (Zhang and Rosin,2003).Yao et al.(2004)propose to use a multiple population GA to detect ellipses.In our work,we present a GA-based circle detector.Our system uses a three edge point circle representation that lets the system to reduce the search space by eliminating unfea-sible circle locations in our image.This approach results in a sub-pixellic circle detector that can detect circles in real images even when the circular object has a significative occluded portion.We present the results of the application of our method to synthetic images,real-world images and hand-drawn circles.This paper is organized as follows:In Section2,we for-mulate our approach and we also present the main charac-teristics of the GA method used to detect circles in images. Section3shows our test protocol and the results of apply-ing our method to synthetic and real-world images.Our conclusions are presented in Section4,we also discuss here some future work to be done.2.Circle detection using GA2.1.Our approachWe represent circles by using the parameters of the sec-ond degree equation passing through three edge points in the edge space of the image.Images are preprocessed by using an edge detection step.We store locations for all the edge points.The detected edge points are the only potential candidates to define circles in our image,taken in triplets.We need an edge detection method that obtains a single pixel contour for objects in the image.We have used a classical Sobel detector as implemented by Matlab software.All the edge points in the image are stored in a vector array V¼f v0;v1;...;v Neg with N e the total number of edge points in the image.We store the(x i,y i)coordinates for each edge pixel v i in the edge vector.We encode each individual as the concatenation of the binary codes of the indexes i1,i2and i3of three edge points.We consider the circle passing through the points v i1;v i2;v i3as a potential solution to the detection of circles in images.A number of these candidate solutions are generated for the initial population of the GA.We evolve this solution by using genetic operators in the simple GA approach.When a given threshold is obtained in thefitness evolution of the population,best individual is taken as the solution of the circle detection problem.Even if RHT for circle detection uses also three edge points to cast a vote for the corresponding point in the parameter space,to obtain a subpixel resolution(as our method is capable to detect for circle parameters),RHT would require huge amounts of memory and it would spend a lot of computational time.The evidence collecting step used in RHT is also performed in our method but it is done along the evolution process,sofitness function improves at each generation by discriminating non plausi-ble circles.In the following paragraphs,we detail the different steps used to formulate the circle detection problem as a GA optimization problem.2.2.Individual representationEach individual C uses three edge points as chromo-somes.In this representation,edge points are stored as an index to their relative position in the edge array V of the image.That will encode an individual as the circle that passes through the three points v i,v j and v k.We represent each circle C by the three parameters x0,y0 and r.With(x0,y0)being the(x,y)coordinates of the center of the circle and r being its radius.We can compute the equation of the circle passing through the three edge points as:ðxÀx0Þ2þðyÀy0Þ2¼r2ð1Þwith:x0¼x2jþy2jÀðx2iþy2iÞ2ðy jÀy iÞx2kþy2kÀðx2iþy2iÞ2ðy kÀy iÞ4ððx jÀx iÞðy kÀy iÞÀðx kÀx iÞðy jÀy iÞÞ;ð2Þy¼2ðx jÀx iÞx2jþy2jÀðx2iþy2iÞ2ðx kÀx iÞx2kþy2kÀðx2iþy2iÞ4ððx jÀx iÞðy kÀy iÞÀðx kÀx iÞðy jÀy iÞÞð3ÞV.Ayala-Ramirez et al./Pattern Recognition Letters27(2006)652–657653andr¼ffiffiffiffiffiffiffiffiffiffiffiffiffiffiffiffiffiffiffiffiffiffiffiffiffiffiffiffiffiffiffiffiffiffiffiffiffiffiffiffiffiðxÀx0Þ2þðyÀy0Þ2qð4ÞWe can then represent the shape parameters(for the circle, [x0,y0,r])as a transformation T of the edge vector indexes i, j,k.½x0;y0;r ¼Tði;j;kÞð5Þwith T being the transformation composed of the previous computations for x0,y0and r.Using the indexes as computational chromosomes,we can sweep a continuous space for the shape parameters but keeping a binary string representation of the GA indi-vidual.This approach reduces the search space by eliminat-ing unfeasible solutions.2.3.Fitness evaluationIn order to compute thefitness value of an individual C, we compute the coordinates of the edge points in a virtual sampled shape.We then validate if they actually exist in the feature space.The test set for these points is S¼f s1;s2;...;s Ns g with N s,the number of test points where the existenceof an edge border will be seek.The test point set S is generated by the uniform sam-pling of the shape boundary.In our case,N s test points are generated around the circumference of the candidate circle.Each point s i is a2D-point where its coordinates (x i,y i)are computed as follows:x i¼x0þrÁcos 2p iN sð6Þx i¼x0þrÁsin 2p iN sð7ÞFitness function F(C)accumulates the number of expected edge points(i.e.the points in the set S)that actually are present in the edge image.That is:FðCÞ¼X N sÀ1i¼0Eðx i;yiÞN sð8Þwith E(x i,y i)being the evaluation of edge features in the image coordinate(x i,y i)and N s being the number of pixels in the perimeter of the circle corresponding to the individ-ual C under test.As the perimeter is a function of the ra-dius,this serves as a normalization function with respect to the radius.That is,F(C)measures completeness of the candidate circle encoded by the computational chromo-some.Our objective is then to maximize F(C)because a larger value implies a better response to this circularity operator.We can stop the optimization process byfixing a number of epochs to evolve the chromosomes or by sat-isfying a completeness threshold.Each of these procedures implies some a priori knowledge about the specific applica-tion context of the circle detector.In our tests,we have fixed a number of epochs for population evolution.There is also a penalty cost for small radius circles in order to favor detection of larger circles.This penalty cost is applied by multiplying F(C)by a factor f(r)defined as follows:fðrÞ¼krÃr if r<rÃ1:0otherwise8<:ð9Þwith r*a threshold depending on the specific application of the circle detector.2.4.Genetic operators and GA parameters tuningWe have developed a generic library for genetic algo-rithms implementation.Functions included in this tool are described in(Perez-Garcia et al.,2004).Our implemen-tation uses three genetic operators:selection,crossover and mutation.For the selection operator we have used a roulette wheel implementation.In this method,each individual is assigned a slice in the roulette wheel.The size of the slice is propor-tional to its normalizedfitness value.This selection strategy favors bestfitted individuals but it gives a chance to the less fitted individuals to survive.A steady state policy is also used,this implies to have elite individuals that will be kept in the next population.For the crossover operator,we have used a1-point crossover implementation.This method uses a crossover probability value to determine where we will divide the genetic material of the two parents to recombine into a new individual.A bit mutation operator is used in our implementation. In this method,a bit is inverted or not depending on a mutation probability.We have tuned the population size by running our circle detection algorithm on an image with a circle and salt and pepper noise.We have chosen the population sizes that minimizes detection time.After experimental tuning,GA parameters were configured as shown in Table1.3.Experimental workExperimental tests have been developed in order to eval-uate the performance of the circle detection environment. Test platform was implemented in C language,on a PC Table1List of parameters for the genetic algorithms used in the circle detection problemParameter ValuePopulation size70Crossover probability0.55Mutation probability0.10Number of elite individuals2Selection method Roulette wheel Crossover method1-point crossover654V.Ayala-Ramirez et al./Pattern Recognition Letters27(2006)652–657using an AMD XP processor running at 2.07GHz.These experiments mainly address tasks such as (1)circle localization,(2)shape discrimination,(3)hand written circle detection and (4)multiple circle localization.A set containing more than an hundred of synthetic and natural images has been used.Error analysis and elapsed time during experiments are also discussed for the system.3.1.Circle detection tests3.1.1.Synthetic imagesThe experimental setup includes the use of 20synthetic images of 256·256pixels.We have generated images with only one randomly located circle in them.Parameters of interest are the (x ,y )center of the circle position and its radius r .The algorithm has been run 100times for each test image.We can say that the average error for localization is 0.06pixels and the worst case (maximum error)is 0.31pixels.That is,the proposed algorithm finds the circle parameters in a sub-pixel level and with an error lower than a tenth of pixel.The detection is robust to translation and scale and the elapsed time is reasonably low (typically under 5ms).3.1.2.Natural imagesCircle detection on natural images is discussed now.Twenty images of 640·480pixels form the test set.The 8bits depth monochromatic images are captured using a digital camera.Each natural scene include a circle shape among other objects.Images are preprocessed using an edge detection algorithm and each output image is fed into the genetic algorithm-based detection.A particular case among these 20is shown on Fig.1.Due to the absence of ground truth reference data,results on detection have been statistically analyzed forcomparison purposes.We have computed averages and standard deviation for the detected parameters for a 100run test for each of a 10images set.Standard deviation is in the 0.3pixels range for the tests.These are good results,considering the existing noise,irregularities in the shape and inclusive,the partial occlusion of circles in some cases.Again,the system is robust to translation and scale in the field of view.3.2.Circle discrimination testsThe ability of the system on the circle detection from a set of diverse shapes is discussed here.Firstly,five synthetic images of 256·256pixels are considered.For each test image,the algorithm in run 100times and both,the num-ber of right detections and the elapsed time are registered.A maximum of 500generations is the limit for the detection process.A number of different shapes are present in the images,as indicated on Table 2.A sample image is the one in Fig.2(labeled as 5in Table 2).The same experiment was repeated using natural images.An example of such an image is Fig.3.Table 3resumes the system performance.From Tables 2and 3we can say that circles are detected an 100%in most cases and we have a 92%as the worst detection percent.That is,circle discrimination from other shapes is feasible in natural images.Translation and change of scale are well handled by thesystem.Fig.1.Circle detection on a natural image.Detected circle is shown as an overlay.Table 2Discrimination results on synthetic images Image Shapes in image Time (s)Accuracy rate (%)12 1.6410023 1.56100340.28100412 3.81925112.39100Fig.2.Sample synthetic image with 11different shapes.V.Ayala-Ramirez et al./Pattern Recognition Letters 27(2006)652–6576553.3.Hand-drawn sketch recognitionOne of the main characteristics offered by the environ-ment is the capability of detecting shapes deformed to a certain degree.This can be shown when processing images such as those in Fig.4.We can say the system performanceis adequate to both,discriminate the hand-drawn circle from the other shapes present in the image and determine the best approximation for this circle.The observed flexibility is due to the fact that the envi-ronment requires a minimum information for shape recon-struction.In this case,a minimum of edge points.Once a circle has been proposed,this shape is evaluated in order to see the concordance with the edge image.This leads to always finding the best approximation for a circle,be it present or not.This feature is the one that enables a suc-cessful detection when dealing with noisy,incomplete,or partially occluded circular shapes.3.4.Multiple circle detectionOur method can also detect several circles on a real image.We need to set a maximal number of circular shapes to find.The method works in the original edge image until convergence for the first circle detection.This shape is then masked on the edge image and the GA circle detector is run on the modified image.This procedure is repeated until the maximum number of detection is attained.A validation of all the detected circles is then performed by analyzing con-tinuity of the circumference segments detected as proposed by Kelly and Levine (1997).This procedure is necessary because we could ask to detect more circular shapes than the number of circles actually present in the image.The sys-tem can then provide a no circle response if none of the detected shapes satisfies circular completeness or also to detect the best circular shapes if there are more circular shapes in the image than the required number.Fig.5(a)shows a real-world image including several cir-cular shapes on it.Detection results are shown overlaid on the image.In that example,we asked the algorithm to find the 6best circular shapes.The edge image obtained after application of the Sobel mask used by our algorithm is shown in Fig.5(b).4.Conclusions and perspectivesIn this paper,we have presented a GA-based circle detector.Our system is capable of detecting circles withTable 3Discrimination results on natural images Image Shapes in image Time (s)Accuracy rate (%)120.9392230.34100340.38100490.43100595.0598Fig.3.Sample natural image with a variety of different shapes.(a)A sample input and (b)the correspondingedges.Fig.4.Sample images for hand-drawn circlesdetection.Fig.5.Multiple circle detection on real-world images:(a)the original image with an overlay of the detected circles and (b)edge image obtained by the application of the Sobel operator.656V.Ayala-Ramirez et al./Pattern Recognition Letters 27(2006)652–657sub-pixellic accuracy in synthetic images.We can also detect circles in real-world images with sub-pixellic preci-sion.The encoding scheme used in this method encodes cir-cles by using the equation for the ideal circle passing through three edge points.The search space is reduced because we only try the circles that can pass through the actual edge points in the scene.This results in a fast detec-tor as compared to recent results in literature.Our circle detector can reliably detect circles even if they present sig-nificative occlusions,discontinuities or incompleteness.We show also that we can apply the same method to detect hand-drawn circles.Our system is also capable of detecting multiple circles on real images.The main drawback of our system arises when it deals with small circles.This problem is derived of the ability of our system to deal with imperfect circles,that is,when some circular sectors can be inscribed in a small shape,it will be detected as an imperfect but plausible circle.Behavior of our system with respect to small circles can be tuned according to the specific applica-tion context.Given the performance characteristics of the method presented here,our future work will be directed towards applying this detector for part inspection in manufacturing and for artificial landmark detection in robotics. AcknowledgementsThis work has been partially funded by the France–Mexico LAFMI Project‘‘Concepcio´n de funciones de per-cepcio´n y planificacio´n para la navegacio´n topolo´gica de un robot mo´vil en ambiente semi-estructurado interior o natural’’and the Mexico PROMEP project‘‘Navegacio´n topolo´gica de robots usando ana´lisis difuso de textura’’. ReferencesBecker J.,Grousson S.,Coltuc D.,2002.From Hough transforms to integral transforms.In:Proc.Int.Geoscience and Remote Sensing Symp.,2002IGARSSÕ02,vol.3,pp.1444–1446.da Fontoura Costa,L.,Marcondes Cesar Jr.,R.,2001.Shape Analysis and Classification.CRC Press,Boca Raton FL.Fischer,M.,Bolles,R.,1981.Random sample consensus:A paradigm to modelfitting with applications to image analysis and automated cartography.CACM24(6),381–395.Han,J.H.,Koczy,L.T.,Poston,T.,1993.Fuzzy Hough transform.In: Proc.2nd Int.Conf.on Fuzzy Systems,vol.2,pp.803–808. Iivarinen,J.,Peura,M.,Sarela,J.,Visa, A.,parison of combined shape descriptors for irregular objects.In:Proc.8th British Machine Vision Conf.,Cochester,UK,pp.430–439.Jones,G.,Princen,J.,Illingworth,J.,Kittler,J.,1990.Robust estimation of shape parameters.In:Proc.British Machine Vision Conf.,pp.43–48.Kelly,M.,Levine,M.,1997.Advances in Image Understanding.Finding and Describing Objects in Complex Images.IEEE Computer Society Press,pp.209–225.Lam,W.,Yuen,S.,1996.Efficient techniques for circle detection using hypothesisfiltering and Hough transform.IEEE Proc.Visual Image Signal Process.143(5),292–300.Lutton,E.,Martinez,P.,1994.A genetic algorithm for the detection2-D geometric primitives on images.In:Proc.of the12th Int.Conf.on Pattern Recognition(ICPRÕ94),vol.1,Jerusalem,Israel,pp.526–528. Mainzer,T.,2002a.Genetic algorithm for shape detection,Technical Report DCSE/TR-2002-06,University of West Bohemia in Pilsen, Pilsen,Czech Republic(March).Mainzer,T.,2002b.Genetic algorithm for traffic sign detection.In:Proc.Int.Conf.Applied Electronics2002,Pilsen,Czech Republic,pp.129–132.Muammar,H.,Nixon,M.,1989.Approaches to extending the Hough transform.In:Proc.Int.Conf.on Acoustics,Speech and Signal Processing ICASSPÕ89,vol.3,pp.1556–1559.Perez-Garcia,A.,Ayala-Ramirez,V.,Jaime-Rivas,R.,2004.A genetic algorithms generic library for application in optimization problems(in spanish).In:Proc.of the5th Mexican Conf.on Robotics,pp.141–146. Peura,M.,Iivarinen,J.,1997.Efficiency of simple shape descriptors.In: Arcelli,C.,Cordella,L.P.,di Baja,G.S.(Eds.),Advances in Visual Form Analysis.World Scientific,Singapore,pp.443–451.Rosin,P.L.,Nyongesa,H.O.,bining evolutionary,connection-ist,and fuzzy classification algorithms for shape analysis.In:Cagnoni, S.et al.(Eds.),Proc.EvoIASP,Real-World Applications of Evolu-tionary Computing,pp.87–96.Rosin,P.L.,1997.Furtherfive pointfit ellipsefitting.In:Proc.8th British Machine Vision Conf.,Cochester,UK,pp.290–299.Roth,G.,Levine,M.D.,1993.Extracting geometric primitives.CVGIP: Image Understanding58(1),1–22.Roth,G.,Levine,M.D.,1994.Geometric primitive extraction using a genetic algorithm.IEEE Trans.Pattern Anal.Machine Intell.16(9), 901–905.Shaked,D.,Yaron,O.,Kiryati,N.,1996.Deriving stopping rules for the probabilistic Hough transform by sequential put.Vision Image Understanding63,512–526.Xu,L.,Oja,E.,Kultanen,P.,1990.A new curve detection method: Randomized Hough transform(RHT).Pattern Recognition Lett.11(5),331–338.Yao,J.,Kharma,N.,Grogono,P.,2004.Fast robust GA-based ellipse detection.In:Proc.17th Int.Conf.on Pattern Recognition ICPRÕ04, vol.2,Cambridge,UK,pp.859–862.Yuen,S.,Ma, C.,2000.Genetic algorithm with competitive image labelling and least square.Pattern Recognition33,1949–1966. Yuen,H.,Princen,J.,Illingworth,J.,Kittler,J.,parative study of Hough transform methods for circlefinding.Image Vision Comput.8(1),71–77.Yuen,K.S.,Lo,E.K.,1994.A coarse-to-fine approach for circle detection.In:Proc.1994Int.Symp.on Speech,Image Processing and Neural Networks,Hong Kong,HK,pp.523–526.Zhang,X.,Rosin,P.L.,2003.Superellipsefitting to partial data.Pattern Recognition36,743–752.V.Ayala-Ramirez et al./Pattern Recognition Letters27(2006)652–657657。