1.Yu and Moyeed(2001)Bayesian quantile regression
Analysis of a predator–prey model with modified Leslie–Gower
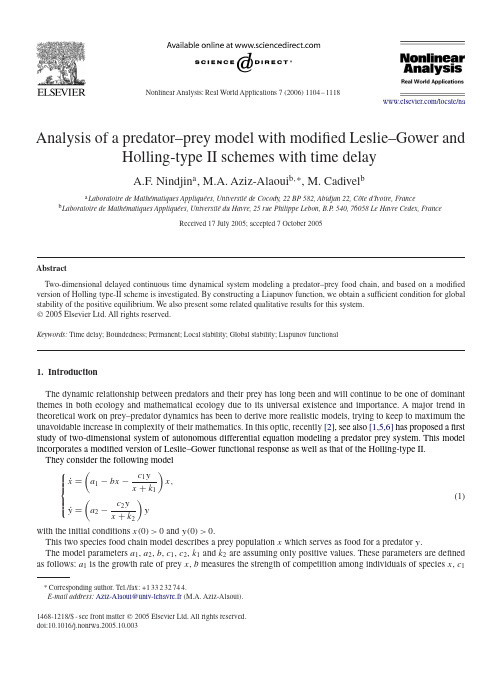
Nonlinear Analysis:Real World Applications7(2006)1104–1118/locate/naAnalysis of a predator–prey model with modified Leslie–Gower and Holling-type II schemes with time delayA.F.Nindjin a,M.A.Aziz-Alaoui b,∗,M.Cadivel ba Laboratoire de Mathématiques Appliquées,Universitéde Cocody,22BP582,Abidjan22,Côte d’Ivoire,Franceb Laboratoire de Mathématiques Appliquées,Universitédu Havre,25rue Philippe Lebon,B.P.540,76058Le Havre Cedex,FranceReceived17July2005;accepted7October2005AbstractTwo-dimensional delayed continuous time dynamical system modeling a predator–prey food chain,and based on a modified version of Holling type-II scheme is investigated.By constructing a Liapunov function,we obtain a sufficient condition for global stability of the positive equilibrium.We also present some related qualitative results for this system.᭧2005Elsevier Ltd.All rights reserved.Keywords:Time delay;Boundedness;Permanent;Local stability;Global stability;Liapunov functional1.IntroductionThe dynamic relationship between predators and their prey has long been and will continue to be one of dominant themes in both ecology and mathematical ecology due to its universal existence and importance.A major trend in theoretical work on prey–predator dynamics has been to derive more realistic models,trying to keep to maximum the unavoidable increase in complexity of their mathematics.In this optic,recently[2],see also[1,5,6]has proposed afirst study of two-dimensional system of autonomous differential equation modeling a predator prey system.This model incorporates a modified version of Leslie–Gower functional response as well as that of the Holling-type II.They consider the following model⎧⎪⎪⎨⎪⎪⎩˙x=a1−bx−c1yx+k1x,˙y=a2−c2yx+k2y(1)with the initial conditions x(0)>0and y(0)>0.This two species food chain model describes a prey population x which serves as food for a predator y.The model parameters a1,a2,b,c1,c2,k1and k2are assuming only positive values.These parameters are defined as follows:a1is the growth rate of prey x,b measures the strength of competition among individuals of species x,c1∗Corresponding author.Tel./fax:+133232744.E-mail address:Aziz-Alaoui@univ-lehavre.fr(M.A.Aziz-Alaoui).1468-1218/$-see front matter᭧2005Elsevier Ltd.All rights reserved.doi:10.1016/j.nonrwa.2005.10.003A.F.Nindjin et al./Nonlinear Analysis:Real World Applications7(2006)1104–11181105 is the maximum value of the per capita reduction rate of x due to y,k1(respectively,k2)measures the extent to which environment provides protection to prey x(respectively,to the predator y),a2describes the growth rate of y,and c2 has a similar meaning to c1.It wasfirst motivated more by the mathematics analysis interest than by its realism as a model of any particular natural dynamical system.However,there may be situations in which the interaction between species is modelized by systems with such a functional response.It may,for example,be considered as a representation of an insect pest–spider food chain.Furthermore,it is afirst step towards a predator–prey model(of Holling–Tanner type)with inverse trophic relation and time delay,that is where the prey eaten by the mature predator can consume the immature predators.Let us mention that thefirst equation of system(1)is standard.By contrast,the second equation is absolutely not standard.This intactness model contains a modified Leslie–Gower term,the second term on the right-hand side in the second equation of(1).The last depicts the loss in the predator population.The Leslie–Gower formulation is based on the assumption that reduction in a predator population has a reciprocal relationship with per capita availability of its preferred food.Indeed,Leslie introduced a predator prey model where the carrying capacity of the predator environment is proportional to the number of prey.He stresses the fact that there are upper limits to the rates of increase of both prey x and predator y,which are not recognized in the Lotka–V olterra model.In case of continuous time,the considerations lead to the following:d y d t =a2y1−yx,in which the growth of the predator population is of logistic form,i.e.d y d t =a2y1−yC.Here,“C”measures the carry capacity set by the environmental resources and is proportional to prey abundance,C= x, where is the conversion factor of prey into predators.The term y/ x of this equation is called the Leslie–Gower term.It measures the loss in the predator population due to the rarity(per capita y/x)of its favorite food.In the case of severe scarcity,y can switch over to other population,but its growth will be limited by the fact that its most favorite food,the prey x,is not available in abundance.The situation can be taken care of by adding a positive constant to the denominator,hence the equation above becomes,d y d t =a2y1−yand thus,d y d t =y⎛⎜⎝a2−a2.yx+d⎞⎟⎠that is the second equation of system(1).In this paper,we introduce time delays in model(1),which is a more realistic approach to the understanding of predator–prey dynamics.Time delay plays an important role in many biological dynamical systems,being particularly relevant in ecology,where time delays have been recognized to contribute critically to the stable or unstable outcome of prey densities due to predation.Therefore,it is interesting and important to study the following delayed modified Leslie–Gower and Holling-Type-II schemes:⎧⎪⎪⎨⎪⎪⎩˙x(t)=a1−bx(t)−c1y(t)x(t)+k1x(t),˙y(t)=a2−c2y(t−r)x(t−r)+k2y(t)(2)for all t>0.Here,we incorporate a single discrete delay r>0in the negative feedback of the predator’s density.1106 A.F .Nindjin et al./Nonlinear Analysis:Real World Applications 7(2006)1104–1118Let us denote by R 2+the nonnegative quadrant and by int (R 2+)the positive quadrant.For ∈[−r,0],we use the following conventional notation:x t ( )=x( +t).Then the initial conditions for this system take the formx 0( )= 1( ),y 0( )= 2( )(3)for all ∈[−r,0],where ( 1, 2)∈C([−r,0],R 2+),x(0)= 1(0)>0and y(0)= 2(0)>0.It is well known that the question of global stability of the positive steady state in a predator–prey system,with a single discrete delay in the predator equation without instantaneous negative feedback,remains a challenge,see [3,5,7].Our main purpose is to present some results about the global stability analysis on a system with delay containing modified Leslie–Gower and Holling-Type-II terms.This paper is organized as follows.In the next section,we present some preliminary results on the boundedness of solutions for system (2)–(3).Next,we study some equilibria properties for this system and give a permanence result.In Section 5,the analysis of the global stability is made for a boundary equilibrium and sufficient conditions are provided for the positive equilibrium of both instantaneous system (1)and system with delay (2)–(3)to be globally asymptotically stable.Finally,a discussion which includes local stability results for system (2)–(3)is given.2.PreliminariesIn this section,we present some preliminary results on the boundedness of solutions for system (2)–(3).We consider (x,y)a noncontinuable solution,see [4],of system (2)–(3),defined on [−r,A [,where A ∈]0,+∞].Lemma 1.The positive quadrant int (R 2+)is invariant for system (2).Proof.We have to show that for all t ∈[0,A [,x(t)>0and y(t)>0.Suppose that is not true.Then,there exists 0<T <A such that for all t ∈[0,T [,x(t)>0and y(t)>0,and either x(T )=0or y(T )=0.For all t ∈[0,T [,we havex(t)=x(0)expt0a 1−bx(s)−c 1y(s)x(s)+k 1d s (4)andy(t)=y(0)expt0a 2−c 2y(s −r)x(s −r)+k 2d s .(5)As (x,y)is defined and continuous on [−r,T ],there is a M 0such that for all t ∈[−r,T [,x(t)=x(0)expt0a 1−bx(s)−c 1y(s)x(s)+k 1d s x(0)exp (−T M)andy(t)=y(0)expt0a 2−c 2y(s −r)x(s −r)+k 2d sy(0)exp (−T M).Taking the limit,as t →T ,we obtainx(T ) x(0)exp (−T M)>0andy(T ) y(0)exp (−T M)>0,which contradicts the fact that either x(T )=0or y(T )=0.So,for all t ∈[0,A [,x(t)>0and y(t)>0.A.F.Nindjin et al./Nonlinear Analysis:Real World Applications7(2006)1104–11181107 Lemma2.For system(2)–(3),A=+∞andlim supt→+∞x(t) K(6) andlim supt→+∞y(t) L(7) where K=a1/b and L=a2/c2(K+k2)e a2r.Proof.From thefirst equation of system(2)–(3),we have for all t∈[0,A[,˙x(t)<x(t)(a1−bx(t)).A standard comparison argument shows that for all t∈[0,A[,x(t) ˜x(t)where˜x is the solution of the following ordinary differential equation˙˜x(t)=˜x(t)(a1−b˜x(t)),˜x(0)=x(0)>0.As lim t→+∞˜x(t)=a1/b,then˜x and thus x is bounded on[0,A[.Moreover,from Eq.(5),we can define y on all interval[kr,(k+1)r],with k∈N,and it is easy to see that y is bounded on[0,A[if A<+∞.Then A=+∞,see [4,Theorem2.4].Now,as for all t 0,x(t) ˜x(t),thenlim sup t→+∞x(t) lim supt→+∞˜x(t)=K.From the predator equation,we have˙y(t)<a2y(t),hence,for t>r,y(t) y(t−r)e a2r,which is equivalent,for t>r,toy(t−r) y(t)e−a2r.(8) Moreover,for any >1,there exists positive T ,such that for t>T ,x(t)< K.According to(8),we have,fort>T +r,˙y(t)<y(t)a2−c2e−a2rK+k2y(t),which implies by the same arguments use for x that,lim supt→+∞y(t) L ,where L =a2/c2( K+k2)e a2r.Conclusion of this lemma holds by letting →1.3.EquilibriaIn this section we study some equilibria properties of system(2)–(3).These steady states are determined analytically by setting˙x=˙y=0.They are independent of the delay r.It is easy to verify that this system has three trivial boundary equilibria,E0=(0,0),E1=(a1/b,0)and E2(0,a2k2/c2).1108 A.F .Nindjin et al./Nonlinear Analysis:Real World Applications 7(2006)1104–1118Proposition 3.System (2)–(3)has a unique interior equilibrium E ∗=(x ∗,y ∗)(i.e.x ∗>0and y ∗>0)if the following condition holdsa 2k 2c 2<a 1k 1c 1.(9)Proof.From system (2)–(3),such a point satisfies(a 1−bx ∗)(x ∗+k 1)=c 1y ∗(10)andy ∗=a 2(x ∗+k 2)c 2.(11)If (9)holds,this system has two solutions (x ∗+,y ∗+)and (x ∗−,y ∗−)given by ⎧⎪⎪⎨⎪⎪⎩x ∗±=12c 2b (−(c 1a 2−a 1c 2+c 2bk 1)± 1/2),y ∗±=a 2(x ∗±+k 2)c 2,where =(c 1a 2−a 1c 2+c 2bk 1)2−4c 2b(c 1a 2k 2−c 2a 1k 1)>0.Moreover,it is easy to see that,x ∗+>0and x ∗−<0. Linear analysis of system (2)–(3)shows that point E 0is unstable (it repels in both x and y directions)and point E 1is also unstable (it attracts in the x -direction but repels in the y -direction).For r >0,the characteristic equation of the linearized system at E 2takes the formP 2( )+Q 2( )e − r =0,whereP 2( )= 2−A ,Q 2( )=a 2( −A),andA =a 1−c 1a 2k 2c 2k 1.Let us defineF 2(y)=|P 2(iy)|2−|Q 2(iy)|2.It is easy to verify that the equation F 2(y)=0has one positive root.Therefore,if E 2is unstable for r =0,it will remain so for all r >0,and if it is stable for r =0,there is a positive constant r 2,such that for r >r 2,E 2becomes unstable.It is easy to verify that for r =0,E 2is asymptotically stable if a 2k 2/c 2>a 1k 1/c 1,stable (but not asymptotically)if a 2k 2/c 2=a 1k 1/c 1and unstable if a 2k 2/c 2<a 1k 1/c 1.4.Permanence resultsDefinition 4.System (2)–(3)is said to be permanent,see [4],if there exist , ,0< < ,independent of the initial condition,such that for all solutions of this system,min lim inf t →+∞x(t),lim inf t →+∞y(t)A.F .Nindjin et al./Nonlinear Analysis:Real World Applications 7(2006)1104–11181109andmaxlim sup t →+∞x(t),lim sup t →+∞y(t).Theorem 5.System (2)–(3)is permanent ifL <a 1k 1c 1.(12)Proof.By Lemma (2),there is a =max {K,L }>0independent of the initial condition such thatmax lim sup t →+∞x(t),lim sup t →+∞y(t) .We only need to show that there is a >0,independent of the initial data,such thatmin lim inf t →+∞x(t),lim inf t →+∞y(t) .It is easy to see that,for system (2)–(3),for any >1and for t large enough,we have y(t)< L .Thus,we obtain˙x >xa 1−bx −c 1 Lk 1 .By standard comparison arguments,it follows thatlim inf t →+∞x(t)1ba 1−c 1 L k 1 and letting →1,we obtainlim inf t →+∞x(t)1ba 1−c 1Lk 1.(13)Let us denote by N 1=1/b(a 1−c 1L/k 1).If (12)is satisfied,N 1>0.From (13)and Lemma 2and for any >1,there exists a positive constant,T ,such that for t >T ,x(t)>N 1/ and y(t)< L .Then,for t >T +r ,we have˙y(t)>y(t)a 2−c 2N 1+ k 2y(t −r) .(14)On the one hand,for t >T +r ,these inequalities lead to˙y(t)>−2c 2LN 1+ k 2y(t),which involves,for t >T +r ,y(t −r)<y(t)exp2c 2LN 12r .(15)On the other hand,from (14)and (15),we have for t >T +r ,˙y(t)>y(t)a 2−c 2N 1+ k 2exp 2c 2LN 1+ k 2r y(t) which yieldslim inf t →+∞y(t)a 2(N 1+ k 2)2exp − 2c 2LN 12r =y .1110 A.F .Nindjin et al./Nonlinear Analysis:Real World Applications 7(2006)1104–1118Letting →1,we getlim inf t →+∞y(t) a 2(N 1+k 2)c 2exp −c 2LN 1+k 2r=y 1.Let be =min {N 1,y 1}>0.Then we have shown that system (2)–(3)is permanent.5.Global stability analysis 5.1.Stability of E 2Theorem 6.If a 1−c 1y 1(k 1<0,then E 2is globally asymptotically stable for system (2)–(3).Proof.As lim inf t →+∞y(t) y 1and lim sup t →+∞x(t) K ,from the prey’s equation we obtain:∀ 1,∃T >0,∀t >T ,˙x(t)<x(t)a 1−bx −c 1y 1(k 1+ K).Asa 1−c 1y 1(k 1+K)<0,there exists >1such thata 1−c 1y 1(k 1<0.Then,by standard comparison arguments,it follows that lim sup t →+∞x(t) 0and thus,lim t →+∞x(t)=0.The -limit set of every solution with positive initial conditions is then contained in {(0,y),y 0}.Now from (7),we obviously obtain ⊂{(0,y),0 y L }.As E 0/∈ ,(E 0is unstable is repels in both x and y directions)and as is nonempty closed and invariant set,therefore ={E 2}. 5.2.Stability of E ∗without delayFirst,we give some sufficient conditions which insure that the steady state in the instantaneous system,i.e.without time delay,is globally asymptotically stable.Theorem 7.The interior equilibrium E ∗is globally asymptotically stable ifa 1+c 1<b(k 1+x ∗),(16)a 1a 2<bk 2(c 2−a 2).(17)Proof.The proof is based on constructing a suitable Lyapunov function.We defineV (x,y)=(x −x ∗)−x ∗ln x x ∗ + (y −y ∗)−y ∗ln yy ∗ ,where =k 2c 1/k 1a 2.A.F .Nindjin et al./Nonlinear Analysis:Real World Applications 7(2006)1104–11181111This function is defined and continuous on int(R 2+).It is obvious that the function V is zero at the equilibrium E∗and is positive for all other values of x and y ,and thus,E ∗is the global minimum of V .The time derivative of V along a solution of system (2)–(3)is given byd V d t =(x −x ∗) a 1−bx −c 1y x +k 1 + (y −y ∗) a 2−c 2y x +k 2.Centering d V /d t on the positive equilibrium,we getd V d t =−b +a 1−bx ∗x +k 1 (x −x ∗)2−c 2x +k 2(y −y ∗)2+a 2 x +k 2−c 1x +k 1 (x −x ∗)(y −y ∗)= −b +a 1−bx ∗x +k 1(x −x ∗)2−c 2x +k 2(y −y ∗)2(18)+a 2x +k 2+c 1x +k 1 (x −x ∗)2+(y −y ∗)22. −b +a 1−bx ∗k 1+c 1k1(x −x ∗)2+−c 2 x +k 2+c 1k 1(y −y ∗)2 −b +a 1−bx ∗k 1+c 1k 1(x −x ∗)2+c 1k 1(x +k 2)x +k 2−c 2k 2a 2 (y −y ∗)2.(19)From (16),we obtain−b +a 1−bx ∗k 1+c 1k 1<0.From (6)and (17),there exists >1and T >0,such thatK +k 2−c 2k 2a 2<0,and for t >T ,d V d t−b +a 1−bx ∗k 1+c 1k 1(x −x ∗)2+c 1k 1(x +k 2)K +k 2−c 2k 2a 2(y −y ∗)2.Thus,d V /d t is negative definite provided that (16)and (17)holds true.Finally,E ∗is globally asymptoticallystable.If in particular,we suppose that environment provides the same protection to both prey and predator (i.e.k 1=k 2)then Theorem 7can be simplified as follows.Corollary 8.The interior equilibrium E ∗is globally asymptotically stable ifk 1=k 2(20)anda 1<b(k 1+x ∗).(21)1112 A.F .Nindjin et al./Nonlinear Analysis:Real World Applications 7(2006)1104–1118Proof.From (18),we haved Vd t =−b +a 1−bx ∗x +k 1 (x −x ∗)2−c 2 x +k 2(y −y ∗)2+c 1(k 2−k 1)xk 1(x +k 2)(x +k 1) (x −x ∗)(y −y ∗) −b +a 1−bx ∗k 1(x −x ∗)2−c 2x +k 2(y −y ∗)2+c 1(k 2−k 1)xk 1(x +k 2)(x +k 1)(x −x ∗)(y −y ∗),which is negative definite provided that (20)and (21)holds true and thus E ∗is globally asymptotically stable.5.3.Stability of E ∗with delayIn this subsection we shall give a result on the global asymptotic stability of the positive equilibrium for the delayed system.Theorem 9.Assume that parameters of system (2)–(3)satisfya 1k 1c 1>maxa 12c 2,32a 2k 2c 2.(22)Then ,for b large enough ,there exists r 0>0such that ,for r ∈[0,r 0],the interior equilibrium E ∗is globally asymp-totically stable in R 2+.Proof.First of all,we rewrite Eqs.(2)to center it on its positive equilibrium.By using the following change of variables,X(t)=lnx(t)x ∗ andY (t)=lny(t)y ∗,the system becomes⎧⎪⎨⎪⎩˙X(t)=x ∗ −b +a 1−bx ∗x(t)+k 1 (e X(t)−1)−c 1y ∗x(t)+k 1(e Y (t)−1),˙Y (t)=−c 2y ∗x(t −r)+k 2(e Y (t −r)−1)+a 2x ∗x(t −r)+k 2(e X(t −r)−1).(23)According to the global existence of solutions established in Lemma 2,we can assume that the initial data exists on [−2r,0](this can be done by changing initial time).Now,let >1be fixed and let us define the following Liapunov functional V :C ([−2r ;0],R 2)→R ,V ( 1, 2)= 1(0)0(e u −1)d u + 2(0)(e u−1)d u +a 2x ∗2k 2 0−r (e 1(u)−1)2d u+c 2y ∗2k 2 0−r 0v e 2(s) c 2y ∗k 2(e 2(s −r)−1)2+a 2x ∗k 2(e 1(s −r)−1)2 d s d v +c 22k 2r L 0−r c 2y ∗k 2(e 2(s)−1)2+a 2x ∗k 2(e 1(s)−1)2 d s .Let us define the continuous and nondecreasing function u :R +→R +byu(x)=e x −x −1.A.F .Nindjin et al./Nonlinear Analysis:Real World Applications 7(2006)1104–11181113We have u(0)=0,u(x)>0for x >0andu(| (0)|) u( 1(0))+u( 2(0)) V ( 1, 2),where =( 1, 2)and |·|denotes the infinity norm in R 2.Let v :R +→R +be define byv(x)=2u(x)+(e x −1)2 a 2x ∗r 2k 2+ c 2y ∗k 2+a 2x ∗k 2 c 2y ∗r 24k 2e x +c 22k 2r 2 L .It is clear that v is a continuous and nondecreasing function and satisfies v(0)=0,v(x)>0andV ( 1, 2) v( ),where · denotes the infinity norm in C ([−2r ;0],R 2).Now,let (X,Y )be a solution of (23)and let us compute˙V(23),the time derivative of V along the solutions of (23).First,we start with the functionV 1( 1, 2)= 1(0)0(e u −1)d u + 2(0)0(e u −1)d u +a 2x ∗2k 2 0−r (e 1(u)−1)2d u .We haveV 1(X t ,Y t )=X(t)(e u−1)d u +Y (t)(e u−1)d u +a 2x ∗2k 2t t −r(e X(u)−1)2d u .Then,˙V1|(23)=(e X(t)−1)˙X(t)+(eY (t)−1)˙Y (t)+a 2x ∗2k 2((e X(t)−1)2−(e X(t −r)−1)2).System (23)gives us˙V 1|(23)=x ∗ −b +a 1−bx ∗x(t)+k 1 (e X(t)−1)2−c 1y ∗x(t)+k 1(e Y (t)−1)(e X(t)−1)−c 2y∗x(t −r)+k 2(e Y (t)−1)(e Y (t −r)−1)+a 2x ∗x(t −r)+k 2(e Y (t)−1)(e X(t −r)−1)+a 2x ∗2k 2((e X(t)−1)2−(e X(t −r)−1)2).By using several times the obvious inequalities of type−c 1y ∗x(t)+k 1(e Y (t)−1)(e X(t)−1) c 1y ∗x(t)+k 1 (e Y (t)−1)22+(e X(t)−1)22c 1y ∗2k 1((e Y (t)−1)2+(e X(t)−1)2),we get˙V1|(23)x ∗−b +a 1−bx ∗k 1+a 22k 2 +c 1y ∗2k 1 (e X(t)−1)2+c 1y ∗2k 1+a 2x ∗2k 2 (e Y (t)−1)2−c 2y ∗x(t −r)+k 2(e Y (t)−1)(e Y (t −r)−1).As,e Y(t−r)=e Y(t)− tt−re Y(s)˙Y(s)d s,we obtain˙V1|(23)x∗−b+a1−bx∗k1+a22k2+c1y∗2k1(e X(t)−1)2+c1y∗2k1+a2x∗2k2−c2y∗x(t−r)+k2(e Y(t)−1)2+c2y∗x(t−r)+k2(e Y(t)−1)tt−re Y(s)˙Y(s)d s.(24)From(23),we have(e Y(t)−1) tt−re Y(s)˙Y(s)d s= tt−re Y(s)−c2y∗x(s−r)+k2(e Y(t)−1)(e Y(s−r)−1)d s +tt−re Y(s)a2x∗x(s−r)+k2(e Y(t)−1)(e X(s−r)−1)d sc2y∗2k2+a2x∗2k2tt−re Y(s)d s.(e Y(t)−1)2+c2y∗2k2tt−re Y(s)(e Y(s−r)−1)2d s+a2x∗2k2tt−re Y(s)(e X(s−r)−1)2d sand as y∗e Y(s) L for s large enough,we obtain,for t large enough,(e Y(t)−1) tt−re Y(s)˙Y(s)d s Lrc22k2+a2x∗2k2y∗(e Y(t)−1)2+ tt−re Y(s)c2y∗2k2(e Y(s−r)−1)2+a2x∗2k2(e X(s−r)−1)2d sand as x(s−r) L for s large enough,(24)becomes,for t large enough,˙V1|(23)x∗−b+a1−bx∗k1+a22k2+c1y∗2k1(e X(t)−1)2+c1y∗2k1+a2x∗2k2−c2y∗x(t−r)+k2+c2Lrk2c2y∗2k2+a2x∗2k2(e Y(t)−1)2+c2y∗k2tt−re Y(s)c2y∗2k2(e Y(s−r)−1)2+a2x∗2k2(e X(s−r)−1)2d s.(25)The next step consists in computation of the time derivative along the solution of(23),of the termV2( 1, 2)=c2y∗2k2−rve 2(s)c2y∗k2(e 2(s−r)−1)2+a2x∗k2(e 1(s−r)−1)2d s d v+c22k2r L−rc2y∗k2(e 2(s)−1)2+a2x∗k2(e 1(s)−1)2d s.We haveV2(X t,Y t)=c2y∗2k2tt−rtve Y(s)c2y∗k2(e Y(s−r)−1)2+a2x∗k2(e X(s−r)−1)2d s d v+c22k2r Ltt−rc2y∗k2(e Y(s)−1)2+a2x∗k2(e X(s)−1)2d s.Then,˙V2|(23)=c2y∗2k2e Y(t)c2y∗k2(e Y(t−r)−1)2+a2x∗k2(e X(t−r)−1)2r−c2y∗2k2tt−re Y(s)c2y∗k2(e Y(s−r)−1)2+a2x∗k2(e X(s−r)−1)2d s+c22k2r Lc2y∗k2(e Y(t)−1)2+a2x∗k2(e X(t)−1)2−c22k2r Lc2y∗k2(e Y(t−r)−1)2+a2x∗k2(e X(t−r)−1)2=c2r2k2c2y∗k2(e Y(t−r)−1)2+a2x∗k2(e X(t−r)−1)2(y∗e Y(t)− L)−c2y ∗2k2 tt−re Y(s)c2y∗k2(e Y(s−r)−1)2+a2x∗k2(e X(s−r)−1)2d s+c22k2r Lc2y∗k2(e Y(t)−1)2+a2x∗k2(e X(t)−1)2.For t large enough,we have y∗e Y(t)− L<0and thus,˙V2|(23) −c2y∗2k2tt−re Y(s)c2y∗k2(e Y(s−r)−1)2+a2x∗k2(e X(s−r)−1)2d s+c22k2r Lc2y∗k2(e Y(t)−1)2+a2x∗k2(e X(t)−1)2.This inequality and(25)lead to,for t large enough,˙V| (23)x∗−b+a1−bx∗k1+a22k2+c1y∗2k1(e X(t)−1)2+c1y∗2k1+a2x∗2k2−c2y∗x(t−r)+k2+c2Lrk2c2y∗2k2+a2x∗2k2(e Y(t)−1)2+c22k2r Lc2y∗k2(e Y(t)−1)2+a2x∗k2(e X(t)−1)2−bx∗(k1+x∗)k1+a1x∗k1+a2x∗2k2+c1y∗2k1+c2a2x∗r L2k22(e X(t)−1)2+c1y∗2k1+a2x∗2k2−c2y∗x(t−r)+k2+c2Lrk2c2y∗k2+a2x∗2k2(e Y(t)−1)2.Now,if−bx ∗(k1+x∗)k1+a1x∗k1+a2x∗2k2+c1y∗2k1<0(26)andc1y∗2k1+a2x∗2k2−c2y∗x(t−r)+k2<0(27)then,for r small enough,we can conclude that ˙V|(23)is negative definite.Hence V satisfies all the assumptions of Corollary 5.2in [4]and the theorem follows.We now study when inequalities (26)and (27)hold.From (10),we have −bx ∗(k 1+x ∗)=c 1(y ∗−a 1(k 1+x ∗)/c 1)and using (11),(26)becomes32c 1k 1a 2(x ∗+k 2)c 2−a 1+a 2x ∗2k 2<0which is rewritten as x ∗a 2k 1c 1k 2+3a 2c 2<2a 1k 1c 1−3a 2k 2c 2.(28)As x ∗ a 1b ,then the following inequalitya 1a 2k 1c 1k 2+3a 2c 2 <b 2a 1k 1c 1−3a 2k 2c 2(29)implies (28).Now,if the following inequality holdsc 1y ∗2k 1+a 2x ∗2k 2−c 2y ∗a 1b+k 2<0,(30)then,for t large enough,(27)holds ing (11),(30)is reformulated asc 1y ∗2k 1+c 2y ∗−a 2k 22k 2<c 2y ∗a1b+k 2c 12k 1+c 22k 2<c 2a 1b+k 2+a 22y ∗c 12k 1+c 22k 2<c 2a 1b +k 2+c 22(x ∗+k 2)c 1k 1<c 2⎛⎜⎝2a 1b +k 2+1x ∗2−1k 2⎞⎟⎠.As x ∗ a 1/b ,then the last inequality is satisfied ifc 1k 1<c 2⎛⎜⎝3a 1b +k 2−1k 2⎞⎟⎠(31)that is ifa 1c 1k 2k 1+a 1c 2<b k 2k 1(2c 2k 1−c 1).(32)In conclusion,ifa 1k 1c 1>maxa 12c 2,32a 2k 2c 2and for b large enough,there exists a unique interior equilibrium and for r small enough it is globally asymptoticallystable.6.DiscussionIt is interesting to discuss the effect of time delay r on the stability of the positive equilibrium of system (2)–(3).We assume that positive equilibrium E ∗exists for this system.Linearizing system (2)–(3)at E ∗,we obtain˙X(t)=A 11X(t)+A 12Y (t),˙Y (t)=A 21X(t −r)+A 22Y (t −r),(33)whereA 11=−bx ∗+c 1x ∗y ∗(x ∗+k 1)2,A 12=−c 1x ∗x ∗+k 1,A 21=c 2(y ∗)2(x ∗+k 2)2=a 22c 2andA 22=−c 2y ∗x ∗2=−a 2.The characteristic equation for (33)takes the formP ( )+Q( )e − r =0(34)in whichP ( )= 2−A 11 andQ( )=−A 22 +(A 11A 22−A 12A 21).When r =0,we observe that the jacobian matrix of the linearized system isJ = A 11A 12A 21A 22 .One can verify that the positive equilibrium E ∗is stable for r =0provided thata 1<bk 1.(35)Indeed,when (35)holds,then it is easy to verify thatT r(J )=A 11+A 22<0andDet (J )=A 11A 22−A 12A 21>0.By denotingF (y)=|P (iy)|2−|Q(iy)|2,we haveF (y)=y 4+(A 211−A 222)y 2−(A 11A 22−A 12A 21)2.If (35)holds,then =0is not a solution of (34).It is also easy to verify that if (35)holds,then equation F (y)=0has at least one positive root.By applying standard theorem on the zeros of transcendental equation,see [4,Theorem4.1],we see that there is a positive constant r0(which can be evaluated explicitly),such that for r>r0,E∗becomes unstable.Then,the global stability of E∗involves restrictions on length of time delay r.Therefore,it is obvious that time delay has a destabilized effect on the positive equilibrium of system(2)–(3).References[1]M.A.Aziz-Alaoui,Study of Leslie–Gower-type tritrophic population,Chaos Solitons Fractals14(8)(2002)1275–1293.[2]M.A.Aziz-Alaoui,M.Daher Okiye,Boundeness and global stability for a predator–prey model with modified Leslie–Gower and Holling-typeIIschemes,Appl.Math.Lett.16(2003)1069–1075.[3]E.Beretta,Y.Kuang,Global analyses in some delayed ratio-depended predator–prey systems,Nonlinear Anal.Theory Methods Appl.32(3)(1998)381–408.[4]Y.Kuang,Delay Differential Equations,with Applications in Population Dynamics,Academic Press,New York,1993.[5]R.K.Upadhyay,V.Rai,Crisis-limited chaotic dynamics in ecological systems,Chaos Solitons Fractals12(2)(2001)205–218.[6]R.K.Upadhyay,S.R.K.Iyengar,Effect of seasonality on the dynamics of2and3species prey–predator system,Nonlinear Anal.:Real WorldAppl.6(2005)509–530.[7]R.Xu,M.A.J.Chaplain,Persistence and global stability in a delayed predator–prey system with Michaelis–Menten type functional response,put.130(2002)441–455.。
Bayes(贝叶斯)估计
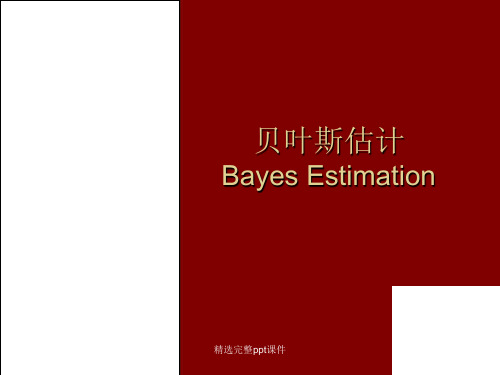
•
参数作为随机变量
• 条件分布: p(x1,x2,..xn | )
精选完整ppt课件
几个学派(3)
• 信念学派:
• 带头人:Fisher
• 观点:概率是频率
•
主观不是概率,而是信念度
•
参数不是随机变量,仅是普通变量
• 似然函数: L( | x1,x2,..xn)
精选完整ppt课件
批评1:置信区间
后验风险:
• Bayesian风险与后验风险
(L(,)p(x|) ()d)dx
• 后验分析最小=>Bayesian风险最小
精选完整ppt课件
两种常用损失函数:
• 平方损失:
L(,)()2
– 最小Bayesian风险估计:后验期望
• 点损失:
L(a,
)
0,|
a
|
1,|
a
|
– 最大后验密度估计
精选完整ppt课件
• 3、联合分布密度->条件分布密度
• p(x1,x2,..xn | ), 是随机变量
• 4、确定的先验分布() • 5、利用Bayesian公式求后验分布密度 • 6、使用后验分布做推断(参数估计、假设检验)
精选完整ppt课件
例1:两点分布b(1,p)的
• 1. 联合分布:p(x|)nxx(1)nx
• 使得 h ( |r ) p (x |)* ( )与先验分布同类型
• 若p(x|)服从正态分布,选正态分布 • 若p(x|)服从两点分布,选Beta分布 • 若p(x|)服从指数分布,选逆Gamma分布
精选完整ppt课件
Bayes统计推断问题
• 参数估计:
– 点估计 – 区间估计
贝叶斯混合效应模型
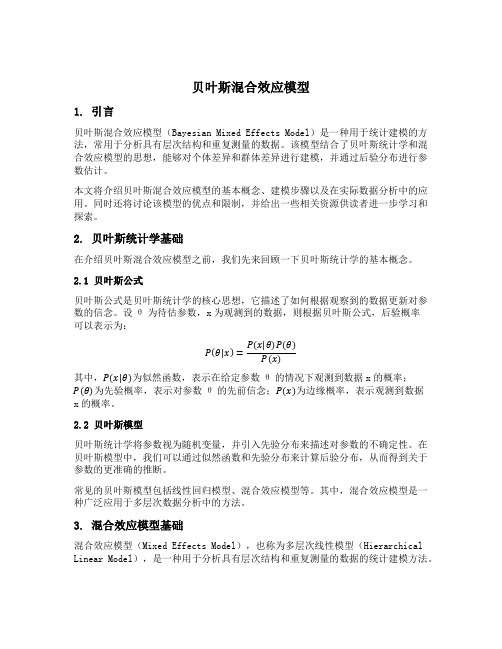
贝叶斯混合效应模型1. 引言贝叶斯混合效应模型(Bayesian Mixed Effects Model)是一种用于统计建模的方法,常用于分析具有层次结构和重复测量的数据。
该模型结合了贝叶斯统计学和混合效应模型的思想,能够对个体差异和群体差异进行建模,并通过后验分布进行参数估计。
本文将介绍贝叶斯混合效应模型的基本概念、建模步骤以及在实际数据分析中的应用。
同时还将讨论该模型的优点和限制,并给出一些相关资源供读者进一步学习和探索。
2. 贝叶斯统计学基础在介绍贝叶斯混合效应模型之前,我们先来回顾一下贝叶斯统计学的基本概念。
2.1 贝叶斯公式贝叶斯公式是贝叶斯统计学的核心思想,它描述了如何根据观察到的数据更新对参数的信念。
设θ为待估参数,x为观测到的数据,则根据贝叶斯公式,后验概率可以表示为:P(θ|x)=P(x|θ)P(θ)P(x)其中,P(x|θ)为似然函数,表示在给定参数θ的情况下观测到数据x的概率;P(θ)为先验概率,表示对参数θ的先前信念;P(x)为边缘概率,表示观测到数据x的概率。
2.2 贝叶斯模型贝叶斯统计学将参数视为随机变量,并引入先验分布来描述对参数的不确定性。
在贝叶斯模型中,我们可以通过似然函数和先验分布来计算后验分布,从而得到关于参数的更准确的推断。
常见的贝叶斯模型包括线性回归模型、混合效应模型等。
其中,混合效应模型是一种广泛应用于多层次数据分析中的方法。
3. 混合效应模型基础混合效应模型(Mixed Effects Model),也称为多层次线性模型(Hierarchical Linear Model),是一种用于分析具有层次结构和重复测量的数据的统计建模方法。
3.1 模型结构混合效应模型将数据分为不同层次,并假设每个层次具有不同的随机效应。
模型的基本结构可以表示为:y ij=X ijβ+Z ij b i+ϵij其中,y ij表示第i个个体在第j个层次上的观测值;X ij和Z ij分别为固定效应和随机效应的设计矩阵;β为固定效应系数;b i为第i个个体的随机效应;ϵij为误差项。
Bayesian and Non-Bayesian Estimation of PrYX
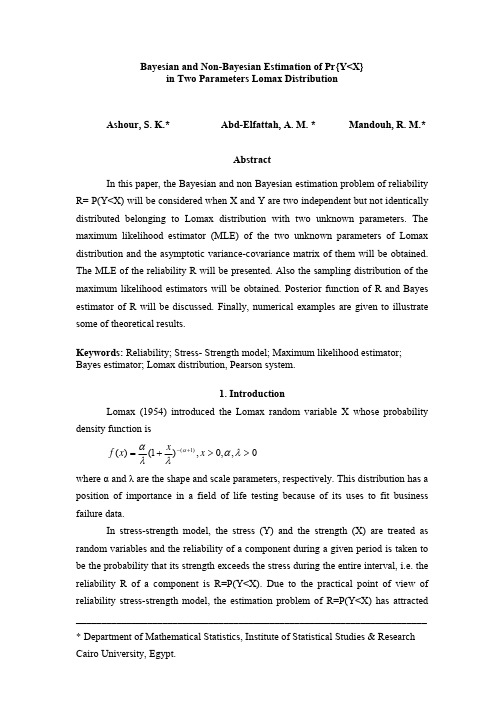
ห้องสมุดไป่ตู้
where α and λ are the shape and scale parameters, respectively. This distribution has a position of importance in a field of life testing because of its uses to fit business failure data. In stress-strength model, the stress (Y) and the strength (X) are treated as random variables and the reliability of a component during a given period is taken to be the probability that its strength exceeds the stress during the entire interval, i.e. the reliability R of a component is R=P(Y<X). Due to the practical point of view of reliability stress-strength model, the estimation problem of R=P(Y<X) has attracted _____________________________________________________________________ * Department of Mathematical Statistics, Institute of Statistical Studies & Research Cairo University, Egypt.
贝叶斯公式(BayesTheorem)
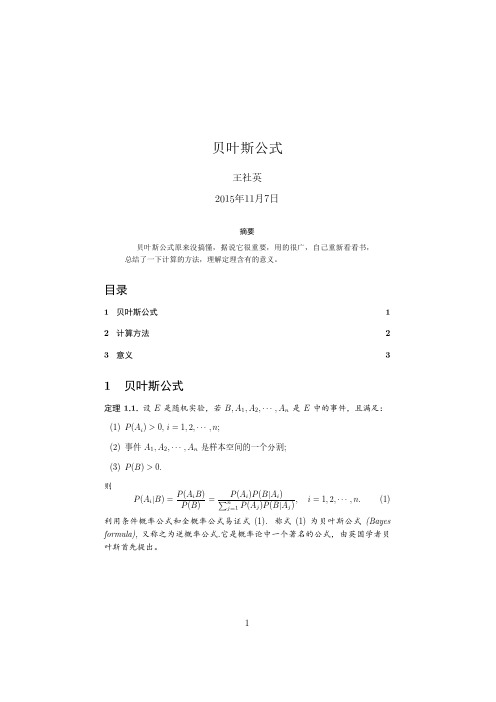
贝叶斯公式王社英2015年11月7日摘要贝叶斯公式原来没搞懂,据说它很重要,用的很广,自己重新看看书,总结了一下计算的方法,理解定理含有的意义。
目录1贝叶斯公式1 2计算方法2 3意义3 1贝叶斯公式定理1.1.设E是随机实验,若B,A1,A2,···,A n是E中的事件,且满足:质贱贩P质A i贩>贰贬i贽贱,贲,···,n贻质贲贩事件A1,A2,···,A n是样本空间的一个分割贻质贳贩P质B贩>贰贮则P质A i|B贩贽P质A i B贩P质B贩贽P质A i贩P质B|A i贩nj=1P质A j贩P质B|A j贩,i贽贱,贲,···,n.质贱贩利用条件概率公式和全概率公式易证式质贱贩.称式质贱贩为贝叶斯公式(Bayes formula),又称之为逆概率公式.它是概率论中一个著名的公式,由英国学者贝叶斯首先提出。
贱2计算方法贝叶斯公式的计算可以画一个图,或者叫做概率树贱P 质A 2贩P 质贖B|A 2贩P 质B |A 2贩P 质A 1贩P 质贖B|A 1贩P 质B |A 1贩贝叶斯公式的计算就是两层贮•第一层的子树数目不定,最常见的是两个;•第二层的子树是确定的,就是两个;•要把第二层的位置放整齐贬含有B 全部放在上面贮那么,贝叶斯公式的计算,就可以流程化了。
P 质A i 贩P 质B |A i 贩 n j =1P 质A j 贩P 质B |A j 贩,i 贽贱,贲,···,n.质贲贩可以把P 质A i 贩P 质B |A i 贩视为根节点P 质A i 贩与子节点P 质B |A i 贩的乘积。
把P 质A i 贩从上到下依次计算。
当我们计算时,•如果计算事件B 发生了,那么所有出现贖B的项可以全部忽略贻•如果计算事件贖B发生了,那么所有出现B 的项可以全部忽略如果我们定义向量•x 贽质P 质A 1贩,P 质A 2贩,···,P 质A n 贩贩贻•y 贽质P 质B |A 1贩,P 质B |A 2贩,···,P 质B |A n 贩贩贻•¯y 贽质P 质贖B|A 1贩,P 质贖B |A 2贩,···,P 质贖B |A n 贩贩贮那么贝叶斯公式的计算可以用向量表示如下贺贲•事件B已经发生的情况下,事件A i发生的概率更新为贺P质A i贩贽x i·y ix·y,i贽贱,贲,...,n.•事件贖B已经发生的情况下,事件A i发生的概率更新为贺P质A i贩贽x i·贖y i x·¯y3意义赛贱贬走赡赧赥赳贲贰购贲贳赝在全概率公式和贝叶斯公式中,如果我们把事件B看成“结果”,而把事件A1,A2,···,A n看成导致结果发生的可能“原因”,则可以形象的把全概率公式看成“由原因推结果”贻而贝叶斯公式恰好相反,其作用在于“由结果找原因”贮在贝叶斯公式中,称P质A i贩为事件A i的先验概率,称P质A i|B贩为事件A i的后验概率,贝叶斯公式是专门用于计算后验概率的贮也就是说,在没有更多的信息质不知事件B是否发生贩的情况下,人们对诸事件A i,A2,···,A n发生的可能性有一个最初的认识贮当有了新的信息质知道事件B已经发生贩贬人们对A i,A2,···,A n发生的可能性大小就有了新的估计贮下面的例子很好的说明了这一点贮例3.1.伊索寓言“孩子与狼”讲的是一个小孩每天到山上放羊,山里有狼出没贮有一天,他闲得无聊在山上喊:“狼来了!狼来了!”,山下的村民闻声便去打狼,可是到了山上发现并没有狼贮第二天仍是如此贮第三天狼真的来了,可是无论小孩怎么叫喊,也没有人来救他,因为前两次他说了谎,人们不再相信他了贮现在用贝叶斯公式来分析寓言中村民对这个小孩的可信程度是如何下降的贮首先记A贽{小孩说谎}贬B贽村民相信小孩的话质即小孩可信贩贮不妨设村民过去对这个小孩的印象为P质B贩贽贰.贸,P质贖B贩贽贰.贲,现在用贝叶斯公式来求P质B|A贩,即小孩说了一次谎后,村民对他可信程度的改变贮在贝叶斯公式中我们要用到概率P质A|B贩和P质A|贖B贩贬这两个概念的含义是:前者为“可信”的孩子说谎的可能性,后者为“不可信”的孩子说谎的可能性贮在此不妨设P质A|B贩贽贰.贱,P质A|贖B贩贽贰.贵.第一次村民上山打狼,发现狼没有来,即小孩说了谎贮村民根据这个信息,对这个小孩的可信程度改变为质由贝叶斯公式贩P质B|A贩贽P质B贩P质A|B贩P质B贩P质A|B贩贫P质贖B贩P质A|贖B贩贽贰.贸×贰.贱贰.贸×贰.贱贫贰.贲×贰.贵贽贰.贴贴贴,贳这表明村民上了一次当后,对这个小孩的可信程度由原来的贰.贸调整为贰.贴贴贴贬即P质B贩贽贰.贴贴贴,P质贖B贩贽贰.贵贵贶,在此基础上,我们再一次利用贝叶斯公式来计算P质B|A贩贬即小孩第二次说谎后,村民对他的可信度改变为P质B|A贩贽贰.贴贴贴×贰.贱贰.贴贴贴×贰.贱贫贰.贵贵贶贽贰.贱贳贸,这表明村民经过两次上当,对这个小孩的可信程度由原来的贰.贸下降到了贰.贱贳贸贬如此低的可信度,村名们听到第三次呼叫时怎么会再上山打狼呢贮贝叶斯公式解释了一种直观的现象,一个说谎的人真的说谎了,他的可信度更低,一个被检测有病的人真的有病了,那么以后就对诊断结果会更相信。
The Cross-Section of Volatility and Expected Returns
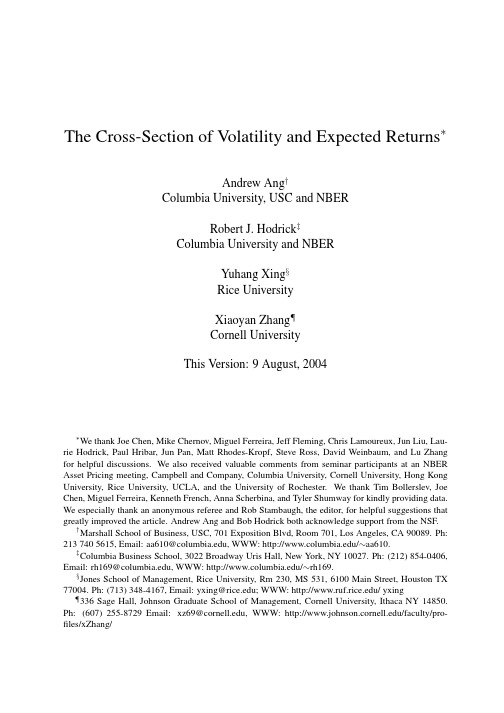
The Cross-Section of V olatility and Expected Returns∗Andrew Ang†Columbia University,USC and NBERRobert J.Hodrick‡Columbia University and NBERYuhang Xing§Rice UniversityXiaoyan Zhang¶Cornell UniversityThis Version:9August,2004∗We thank Joe Chen,Mike Chernov,Miguel Ferreira,Jeff Fleming,Chris Lamoureux,Jun Liu,Lau-rie Hodrick,Paul Hribar,Jun Pan,Matt Rhodes-Kropf,Steve Ross,David Weinbaum,and Lu Zhang for helpful discussions.We also received valuable comments from seminar participants at an NBER Asset Pricing meeting,Campbell and Company,Columbia University,Cornell University,Hong Kong University,Rice University,UCLA,and the University of Rochester.We thank Tim Bollerslev,Joe Chen,Miguel Ferreira,Kenneth French,Anna Scherbina,and Tyler Shumway for kindly providing data. We especially thank an anonymous referee and Rob Stambaugh,the editor,for helpful suggestions that greatly improved the article.Andrew Ang and Bob Hodrick both acknowledge support from the NSF.†Marshall School of Business,USC,701Exposition Blvd,Room701,Los Angeles,CA90089.Ph: 2137405615,Email:aa610@,WWW:/∼aa610.‡Columbia Business School,3022Broadway Uris Hall,New York,NY10027.Ph:(212)854-0406, Email:rh169@,WWW:/∼rh169.§Jones School of Management,Rice University,Rm230,MS531,6100Main Street,Houston TX 77004.Ph:(713)348-4167,Email:yxing@;WWW:/yxing ¶336Sage Hall,Johnson Graduate School of Management,Cornell University,Ithaca NY14850. Ph:(607)255-8729Email:xz69@,WWW:/faculty/pro-files/xZhang/AbstractWe examine the pricing of aggregate volatility risk in the cross-section of stock returns. Consistent with theory,wefind that stocks with high sensitivities to innovations in aggregate volatility have low average returns.In addition,wefind that stocks with high idiosyncratic volatility relative to the Fama and French(1993)model have abysmally low average returns. This phenomenon cannot be explained by exposure to aggregate volatility risk.Size,book-to-market,momentum,and liquidity effects cannot account for either the low average returns earned by stocks with high exposure to systematic volatility risk or for the low average returns of stocks with high idiosyncratic volatility.1IntroductionIt is well known that the volatility of stock returns varies over time.While considerable research has examined the time-series relation between the volatility of the market and the expected re-turn on the market(see,among others,Campbell and Hentschel(1992),and Glosten,Jagan-nathan and Runkle(1993)),the question of how aggregate volatility affects the cross-section of expected stock returns has received less attention.Time-varying market volatility induces changes in the investment opportunity set by changing the expectation of future market returns, or by changing the risk-return trade-off.If the volatility of the market return is a systematic risk factor,an APT or factor model predicts that aggregate volatility should also be priced in the cross-section of stocks.Hence,stocks with different sensitivities to innovations in aggregate volatility should have different expected returns.Thefirst goal of this paper is to provide a systematic investigation of how the stochastic volatility of the market is priced in the cross-section of expected stock returns.We want to de-termine if the volatility of the market is a priced risk factor and estimate the price of aggregate volatility risk.Many option studies have estimated a negative price of risk for market volatil-ity using options on an aggregate market index or options on individual stocks.1Using the cross-section of stock returns,rather than options on the market,allows us to create portfolios of stocks that have different sensitivities to innovations in market volatility.If the price of ag-gregate volatility risk is negative,stocks with large,positive sensitivities to volatility risk should have low average ing the cross-section of stock returns also allows us to easily con-trol for a battery of cross-sectional effects,like the size and value factors of Fama and French (1993),the momentum effect of Jegadeesh and Titman(1993),and the effect of liquidity risk documented by P´a stor and Stambaugh(2003).Option pricing studies do not control for these cross-sectional risk factors.Wefind that innovations in aggregate volatility carry a statistically significant negative price of risk of approximately-1%per annum.Economic theory provides several reasons why the price of risk of innovations in market volatility should be negative.For example,Campbell (1993and1996)and Chen(2002)show that investors want to hedge against changes in mar-ket volatility,because increasing volatility represents a deterioration in investment opportuni-ties.Risk averse agents demand stocks that hedge against this risk.Periods of high volatility also tend to coincide with downward market movements(see French,Schwert and Stambaugh (1987),and Campbell and Hentschel(1992)).As Bakshi and Kapadia(2003)comment,assets 1See,among others,Jackwerth and Rubinstein(1996),Bakshi,Cao and Chen(2000),Chernov and Ghysels (2000),Burashi and Jackwerth(2001),Coval and Shumway(2001),Benzoni(2002),Jones(2003),Pan(2002), Bakshi and Kapadia(2003),Eraker,Johannes and Polson(2003),and Carr and Wu(2003).with high sensitivities to market volatility risk provide hedges against market downside risk. The higher demand for assets with high systematic volatility loadings increases their price and lowers their average return.Finally,stocks that do badly when volatility increases tend to have negatively skewed returns over intermediate horizons,while stocks that do well when volatil-ity rises tend to have positively skewed returns.If investors have preferences over coskewness (see Harvey and Siddique(2000)),stocks that have high sensitivities to innovations in market volatility are attractive and have low returns.2The second goal of the paper is to examine the cross-sectional relationship between id-iosyncratic volatility and expected returns,where idiosyncratic volatility is defined relative to the standard Fama and French(1993)model.3If the Fama-French model is correct,forming portfolios by sorting on idiosyncratic volatility will obviously provide no difference in average returns.Nevertheless,if the Fama-French model is false,sorting in this way potentially provides a set of assets that may have different exposures to aggregate volatility and hence different aver-age returns.Our logic is the following.If aggregate volatility is a risk factor that is orthogonal to existing risk factors,the sensitivity of stocks to aggregate volatility times the movement in aggregate volatility will show up in the residuals of the Fama-French model.Firms with greater sensitivities to aggregate volatility should therefore have larger idiosyncratic volatilities relative to the Fama-French model,everything else being equal.Differences in the volatilities offirms’true idiosyncratic errors,which are not priced,will make this relation noisy.We should be able to average out this noise by constructing portfolios of stocks to reveal that larger idiosyncratic volatilities relative to the Fama-French model correspond to greater sensitivities to movements in aggregate volatility and thus different average returns,if aggregate volatility risk is priced.While high exposure to aggregate volatility risk tends to produce low expected returns,some economic theories suggest that idiosyncratic volatility should be positively related to expected returns.If investors demand compensation for not being able to diversify risk(see Malkiel and Xu(2002),and Jones and Rhodes-Kropf(2003)),then agents will demand a premium for holding stocks with high idiosyncratic volatility.Merton(1987)suggests that in an information-segmented market,firms with largerfirm-specific variances require higher average returns to compensate investors for holding imperfectly diversified portfolios.Some behavioral models, 2Bates(2001)and Vayanos(2004)provide recent structural models whose reduced form factor structures have a negative risk premium for volatility risk.3Recent studies examining total or idiosyncratic volatility focus on the average level offirm-level volatility. For example,Campbell,Lettau,Malkiel and Xu(2001),and Xu and Malkiel(2003)document that idiosyncratic volatility has increased over time.Brown and Ferreira(2003)and Goyal and Santa-Clara(2003)argue that id-iosyncratic volatility has positive predictive power for excess market returns,but this is disputed by Bali,Cakici, Yan and Zhang(2004).like Barberis and Huang(2001),also predict that higher idiosyncratic volatility stocks should earn higher expected returns.Our results are directly opposite to these theories.Wefind that stocks with high idiosyncratic volatility have low average returns.There is a strongly significant difference of-1.06%per month between the average returns of the quintile portfolio with the highest idiosyncratic volatility stocks and the quintile portfolio with the lowest idiosyncratic volatility stocks.In contrast to our results,earlier researchers either found a significantly positive relation between idiosyncratic volatility and average returns,or they failed tofind any statistically sig-nificant relation between idiosyncratic volatility and average returns.For example,Lintner (1965)shows that idiosyncratic volatility carries a positive coefficient in cross-sectional regres-sions.Lehmann(1990)alsofinds a statistically significant,positive coefficient on idiosyncratic volatility over his full sample period.Similarly,Tinic and West(1986)and Malkiel and Xu (2002)unambiguouslyfind that portfolios with higher idiosyncratic volatility have higher av-erage returns,but they do not report any significance levels for their idiosyncratic volatility premiums.On the other hand,Longstaff(1989)finds that a cross-sectional regression coeffi-cient on total variance for size-sorted portfolios carries an insignificant negative sign.The difference between our results and the results of past studies is that the past literature either does not examine idiosyncratic volatility at thefirm level or does not directly sort stocks into portfolios ranked on this measure of interest.For example,Tinic and West(1986)work only with20portfolios sorted on market beta,while Malkiel and Xu(2002)work only with 100portfolios sorted on market beta and size.Malkiel and Xu(2002)only use the idiosyncratic volatility of one of the100beta/size portfolios to which a stock belongs to proxy for that stock’s idiosyncratic risk and,thus,do not examinefirm-level idiosyncratic volatility.Hence,by not di-rectly computing differences in average returns between stocks with low and high idiosyncratic volatilities,previous studies miss the strong negative relation between idiosyncratic volatility and average returns that wefind.The low average returns to stocks with high idiosyncratic volatilities could arise because stocks with high idiosyncratic volatilities may have high exposure to aggregate volatility risk, which lowers their average returns.We investigate this issue andfind that this is not a complete explanation.Our idiosyncratic volatility results are also robust to controlling for value,size, liquidity,volume,dispersion of analysts’forecasts,and momentum effects.Wefind the effect robust to different formation periods for computing idiosyncratic volatility and for different holding periods.The effect also persists in both bull and bear markets,recessions and expan-sions,and volatile and stable periods.Hence,our results on idiosyncratic volatility represent a substantive puzzle.The rest of this paper is organized as follows.In Section2,we examine how aggregate volatility is priced in the cross-section of stock returns.Section3documents thatfirms with high idiosyncratic volatility have very low average returns.Finally,Section4concludes.2Pricing Systematic Volatility in the Cross-Section2.1Theoretical MotivationWhen investment opportunities vary over time,the multi-factor models of Merton(1973)and Ross(1976)show that risk premia are associated with the conditional covariances between as-set returns and innovations in state variables that describe the time-variation of the investment opportunities.Campbell’s(1993and1996)version of the Intertemporal CAPM(I-CAPM) shows that investors care about risks from the market return and from changes in forecasts of future market returns.When the representative agent is more risk averse than log utility,assets that covary positively with good news about future expected returns on the market have higher average returns.These assets command a risk premium because they reduce a consumer’s abil-ity to hedge against a deterioration in investment opportunities.The intuition from Campbell’s model is that risk-averse investors want to hedge against changes in aggregate volatility because volatility positively affects future expected market returns,as in Merton(1973).However,in Campbell’s set-up,there is no direct role forfluctuations in market volatility to affect the expected returns of assets because Campbell’s model is premised on homoskedastic-ity.Chen(2002)extends Campbell’s model to a heteroskedastic environment which allows for both time-varying covariances and stochastic market volatility.Chen shows that risk-averse in-vestors also want to directly hedge against changes in future market volatility.In Chen’s model, an asset’s expected return depends on risk from the market return,changes in forecasts of future market returns,and changes in forecasts of future market volatilities.For an investor more risk averse than log utility,Chen shows that an asset that has a positive covariance between its return and a variable that positively forecasts future market volatilities causes that asset to have a lower expected return.This effect arises because risk-averse investors reduce current consumption to increase precautionary savings in the presence of increased uncertainty about market returns.Motivated by these multi-factor models,we study how exposure to market volatility risk is priced in the cross-section of stock returns.A true conditional multi-factor representation of expected returns in the cross-section would take the following form:r i t+1=a it+βim,t(r mt+1−γm,t)+βiv,t(v t+1−γv,t)+Kk=1βik,t(f k,t+1−γk,t),(1)where r it+1is the excess return on stock i,βim,tis the loading on the excess market return,βiv,tis the asset’s sensitivity to volatility risk,and theβik,tcoefficients for k=1...K representloadings on other risk factors.In the full conditional setting in equation(1),factor loadings, conditional means of factors,and factor premiums potentially vary over time.The model inequation(1)is written in terms of factor innovations,so r mt+1−γm,t represents the innovation in the market return,v t+1−γv,t represents the innovation in the factor reflecting aggregate volatility risk,and innovations to the other factors are represented by f k,t+1−γk,t.The conditional mean of the market and aggregate volatility are denoted byγm,t andγv,t,respectively,while the conditional mean of the other factors are denoted byγk,t.In equilibrium,the conditional mean of stock i is given by:a i t =E t(r it+1)=βim,tλm,t+βiv,tλv,t+Kk=1βik,tλk,t,(2)whereλm,t is the price of risk of the market factor,λv,t is the price of aggregate volatility risk, and theλk,t are prices of risk of the other factors.Note that only if a factor is traded is the conditional mean of a factor equal to its conditional price of risk.The main prediction from the factor model setting of equation(1)that we examine is that stocks with different loadings on aggregate volatility risk have different average returns.4How-ever,the true model in equation(1)is infeasible to examine because the true set of factors is unknown and the true conditional factor loadings are unobservable.Hence,we do not attempt to directly use equation(1)in our empirical work.Instead,we simplify the full model in equation (1),which we now detail.2.2The Empirical FrameworkTo investigate how aggregate volatility risk is priced in the cross-section of equity returns we make the following simplifying assumptions to the full specification in equation(1).First,we use observable proxies for the market factor and the factor representing aggregate volatility risk. We use the CRSP value-weighted market index to proxy for the market factor.To proxy innova-tions in aggregate volatility,(v t+1−γv,t),we use changes in the V IX index from the Chicago 4While an I-CAPM implies joint time-series as well as cross-sectional predictability,we do not examine time-series predictability of asset returns by systematic volatility.Time-varying volatility risk generates intertemporal hedging demands in partial equilibrium asset allocation problems.In a partial equilibrium setting,Liu(2001)and Chacko and Viceira(2003)examine how volatility risk affects the portfolio allocation of stocks and risk-free assets, while Liu and Pan(2003)show how investors can optimally exploit the variation in volatility with options.Guo and Whitelaw(2003)examine the intertemporal components of time-varying systematic volatility in a Campbell (1993and1996)equilibrium I-CAPM.Board Options Exchange(CBOE).5Second,we reduce the number of factors in equation(1) to just the market factor and the proxy for aggregate volatility risk.Finally,to capture the con-ditional nature of the true model,we use short intervals,one month of daily data,to take into account possible time-variation of the factor loadings.We discuss each of these simplifications in turn.Innovations in the V IX IndexThe V IX index is constructed so that it represents the implied volatility of a synthetic at-the-money option contract on the S&P100index that has a maturity of one month.It is constructed from eight S&P100index puts and calls and takes into account the American features of the option contracts,discrete cash dividends and microstructure frictions such as bid-ask spreads (see Whaley(2000)for further details).6Figure1plots the V IX index from January1986to December2000.The mean level of the daily V IX series is20.5%,and its standard deviation is7.85%.Because the V IX index is highly serially correlated with afirst-order autocorrelation of 0.94,we measure daily innovations in aggregate volatility by using daily changes in V IX, which we denote as∆V IX.Dailyfirst differences in V IX have an effective mean of zero(less than0.0001),a standard deviation of2.65%,and also have negligible serial correlation(the first-order autocorrelation of∆V IX is-0.0001).As part of our robustness checks in Section 2.3,we also measure innovations in V IX by specifying a stationary time-series model for the conditional mean of V IX andfind our results to be similar to using simplefirst differences. While∆V IX seems an ideal proxy for innovations in volatility risk because the V IX index is representative of traded option securities whose prices directly reflect volatility risk,there are two main caveats with using V IX to represent observable market volatility.Thefirst concern is that the V IX index is the implied volatility from the Black-Scholes 5In previous versions of this paper,we also considered sample volatility,following Schwert and Stambaugh (1987);a range-based estimate,following Alizadeh,Brandt and Diebold(2002);and a high-frequency estima-tor of volatility from Andersen,Bollerslev and Diebold(2003).Using these measures to proxy for innovations in aggregate volatility produces little spread in cross-sectional average returns.These tables are available upon request.6On September22,2003,the CBOE implemented a new formula and methodology to construct its volatility index.The new index is based on the S&P500(rather than the S&P100)and takes into account a broader range of strike prices rather than using only at-the-money option contracts.The CBOE now uses V IX to refer to this new index.We use the old index(denoted by the ticker V XO).We do not use the new index because it has been constructed by back-filling only to1990,whereas the V XO is available in real-time from1986.The CBOE continues to make both volatility indices available.The correlation between the new and the old CBOE volatility series is98%from1990-2000,but the series that we use has a slightly broader range.(1973)model,and we know that the Black-Scholes model is an approximation.If the true stochastic environment is characterized by stochastic volatility and jumps,∆V IX will reflect total quadratic variation in both diffusion and jump components(see,for example,Pan(2002)). Although Bates(2000)argues that implied volatilities computed taking into account jump risk are very close to original Black-Scholes implied volatilities,jump risk may be priced differ-ently from volatility risk.Our analysis does not separate jump risk from diffusion risk,so our aggregate volatility risk may include jump risk components.A more serious reservation about the V IX index is that V IX combines both stochastic volatility and the stochastic volatility risk premium.Only if the risk premium is zero or constant would∆V IX be a pure proxy for the innovation in aggregate volatility.Decomposing∆V IX into the true innovation in volatility and the volatility risk premium can only be done by writing down a formal model.The form of the risk premium depends on the parameterization of the price of volatility risk,the number of factors and the evolution of those factors.Each different model specification implies a different risk premium.For example,many stochastic volatility option pricing models assume that the volatility risk premium can be parameterized as a linear function of volatility(see,for example,Chernov and Ghysels(2000),Benzoni(2002),and Jones(2003)).This may or may not be a good approximation to the true price of risk.Rather than imposing a structural form,we use an unadulterated∆V IX series.An advantage of this approach is that our analysis is simple to replicate.The Pre-Formation RegressionOur goal is to test if stocks with different sensitivities to aggregate volatility innovations(prox-ied by∆V IX)have different average returns.To measure the sensitivity to aggregate volatility innovations,we reduce the number of factors in the full specification in equation(1)to two,the market factor and∆V IX.A two-factor pricing kernel with the market return and stochastic volatility as factors is also the standard set-up commonly assumed by many stochastic option pricing studies(see,for example,Heston,1993).Hence,the empirical model that we examine is:r i t =β0+βiMKT·MKT t+βi∆V IX·∆V IX t+εit,(3)where MKT is the market excess return,∆V IX is the instrument we use for innovations inthe aggregate volatility factor,andβiMKT andβi∆V IXare loadings on market risk and aggregatevolatility risk,respectively.Previous empirical studies suggest that there are other cross-sectional factors that have ex-planatory power for the cross-section of returns,such as the size and value factors of the Fama and French(1993)three-factor model(hereafter FF-3).We do not directly model these effectsin equation(3),because controlling for other factors in constructing portfolios based on equa-tion(3)may add a lot of noise.Although we keep the number of regressors in our pre-formation portfolio regressions to a minimum,we are careful to ensure that we control for the FF-3factors and other cross-sectional factors in assessing how volatility risk is priced using post-formation regression tests.We construct a set of assets that are sufficiently disperse in exposure to aggregate volatility innovations by sortingfirms on∆V IX loadings over the past month using the regression(3) with daily data.We run the regression for all stocks on AMEX,NASDAQ and the NYSE,with more than17daily observations.In a setting where coefficients potentially vary over time,a 1-month window with daily data is a natural compromise between estimating coefficients with a reasonable degree of precision and pinning down conditional coefficients in an environment with time-varying factor loadings.P´a stor and Stambaugh(2003),among others,also use daily data with a1-month window in similar settings.At the end of each month,we sort stocks into quintiles,based on the value of the realizedβ∆V IX coefficients over the past month.Firms in quintile1have the lowest coefficients,whilefirms in quintile5have the highestβ∆V IX loadings. Within each quintile portfolio,we value-weight the stocks.We link the returns across time to form one series of post-ranking returns for each quintile portfolio.Table1reports various summary statistics for quintile portfolios sorted by pastβ∆V IX over the previous month using equation(3).Thefirst two columns report the mean and standard deviation of monthly total,not excess,simple returns.In thefirst column under the heading ‘Factor Loadings,’we report the pre-formationβ∆V IX coefficients,which are computed at the beginning of each month for each portfolio and are value-weighted.The column reports the time-series average of the pre-formationβ∆V IX loadings across the whole sample.By con-struction,since the portfolios are formed by ranking on pastβ∆V IX,the pre-formationβ∆V IX loadings monotonically increase from-2.09for portfolio1to2.18for portfolio5.The columns labelled‘CAPM Alpha’and‘FF-3Alpha’report the time-series alphas of these portfolios relative to the CAPM and to the FF-3model,respectfully.Consistent with the negative price of systematic volatility risk found by the option pricing studies,we see lower average raw returns,CAPM alphas,and FF-3alphas with higher past loadings ofβ∆V IX.All the differences between quintile portfolios5and1are significant at the1%level,and a joint test for the alphas equal to zero rejects at the5%level for both the CAPM and the FF-3model.In particular,the5-1spread in average returns between the quintile portfolios with the highest and lowestβ∆V IX coefficients is-1.04%per month.Controlling for the MKT factor exacerbates the5-1spread to-1.15%per month,while controlling for the FF-3model decreases the5-1 spread to-0.83%per month.Requirements for a Factor Risk ExplanationWhile the differences in average returns and alphas corresponding to differentβ∆V IX loadings are very impressive,we cannot yet claim that these differences are due to systematic volatility risk.We will examine the premium for aggregate volatility within the framework of an uncon-ditional factor model.There are two requirements that must hold in order to make a case for a factor risk-based explanation.First,a factor model implies that there should be contemporane-ous patterns between factor loadings and average returns.For example,in a standard CAPM, stocks that covary strongly with the market factor should,on average,earn high returns over the same period.To test a factor model,Black,Jensen and Scholes(1972),Fama and French(1992 and1993),Jagannathan and Wang(1996),and P´a stor and Stambaugh(2003),among others,all form portfolios using various pre-formation criteria,but examine post-ranking factor loadings that are computed over the full sample period.While theβ∆V IX loadings show very strong patterns of future returns,they represent past covariation with innovations in market volatility. We must show that the portfolios in Table1also exhibit high loadings with volatility risk over the same period used to compute the alphas.To construct our portfolios,we took∆V IX to proxy for the innovation in aggregate volatil-ity at a daily frequency.However,at the standard monthly frequency,which is the frequency of the ex-post returns for the alphas reported in Table1,using the change in V IX is a poor approximation for innovations in aggregate volatility.This is because at lower frequencies,the effect of the conditional mean of V IX plays an important role in determining the unanticipated change in V IX.In contrast,the high persistence of the V IX series at a daily frequency means that thefirst difference of V IX is a suitable proxy for the innovation in aggregate volatility. Hence,we should not measure ex-post exposure to aggregate volatility risk by looking at how the portfolios in Table1correlate ex-post with monthly changes in V IX.To measure ex-post exposure to aggregate volatility risk at a monthly frequency,we follow Breeden,Gibbons and Litzenberger(1989)and construct an ex-post factor that mimics aggre-gate volatility risk.We term this mimicking factor F V IX.We construct the tracking portfolio so that it is the portfolio of asset returns maximally correlated with realized innovations in volatility using a set of basis assets.This allows us to examine the contemporaneous relation-ship between factor loadings and average returns.The major advantage of using F V IX to measure aggregate volatility risk is that we can construct a good approximation for innovations in market volatility at any frequency.In particular,the factor mimicking aggregate volatility innovations allows us to proxy aggregate volatility risk at the monthly frequency by simply cumulating daily returns over the month on the underlying base assets used to construct the mimicking factor.This is a much simpler method for measuring aggregate volatility innova-。
贝叶斯
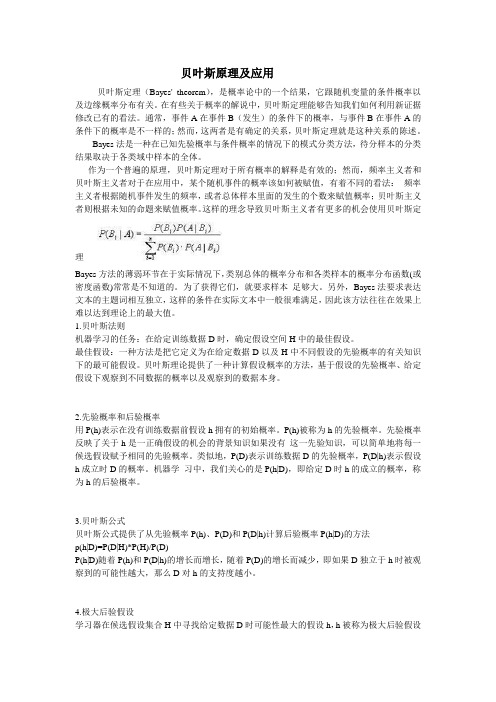
贝叶斯原理及应用贝叶斯定理(Bayes' theorem),是概率论中的一个结果,它跟随机变量的条件概率以及边缘概率分布有关。
在有些关于概率的解说中,贝叶斯定理能够告知我们如何利用新证据修改已有的看法。
通常,事件A在事件B(发生)的条件下的概率,与事件B在事件A的条件下的概率是不一样的;然而,这两者是有确定的关系,贝叶斯定理就是这种关系的陈述。
Bayes法是一种在已知先验概率与条件概率的情况下的模式分类方法,待分样本的分类结果取决于各类域中样本的全体。
作为一个普遍的原理,贝叶斯定理对于所有概率的解释是有效的;然而,频率主义者和贝叶斯主义者对于在应用中,某个随机事件的概率该如何被赋值,有着不同的看法:频率主义者根据随机事件发生的频率,或者总体样本里面的发生的个数来赋值概率;贝叶斯主义者则根据未知的命题来赋值概率。
这样的理念导致贝叶斯主义者有更多的机会使用贝叶斯定理Bayes方法的薄弱环节在于实际情况下,类别总体的概率分布和各类样本的概率分布函数(或密度函数)常常是不知道的。
为了获得它们,就要求样本足够大。
另外,Bayes法要求表达文本的主题词相互独立,这样的条件在实际文本中一般很难满足,因此该方法往往在效果上难以达到理论上的最大值。
1.贝叶斯法则机器学习的任务:在给定训练数据D时,确定假设空间H中的最佳假设。
最佳假设:一种方法是把它定义为在给定数据D以及H中不同假设的先验概率的有关知识下的最可能假设。
贝叶斯理论提供了一种计算假设概率的方法,基于假设的先验概率、给定假设下观察到不同数据的概率以及观察到的数据本身。
2.先验概率和后验概率用P(h)表示在没有训练数据前假设h拥有的初始概率。
P(h)被称为h的先验概率。
先验概率反映了关于h是一正确假设的机会的背景知识如果没有这一先验知识,可以简单地将每一候选假设赋予相同的先验概率。
类似地,P(D)表示训练数据D的先验概率,P(D|h)表示假设h成立时D的概率。
维基百科统计学家列表

A - E∙Odd Aalen(英语:Odd Aalen) (1947–)∙戈特弗里德·阿亨瓦尔(英语:Gottfried Achenwall) (1719–1772) ∙亚伯拉罕·曼尼·艾德斯坦(英语:Abraham Manie Adelstein) (1916–1992)∙约翰·艾奇逊(英语:John Aitchison) (1926–)∙亚历山大·艾特肯(英语:Alexander Aitken) (1895–1967)∙赤池弘次 (1927–)∙奥斯卡·安德尔森(英语:Oskar Anderson) (1887–1960)∙彼得·阿米蒂奇(英语:Peter Armitage) (1924–)∙Raghu Raj Bahadur(英语:Raghu Raj Bahadur) (1924-1997)∙乔治·阿尔弗雷德·巴纳德(英语:George Alfred Barnard) (1915–2002)∙莫里斯·史蒂文森·巴特利特(英语:M. S. Bartlett) (1910–2002) ∙Debabrata Basu(英语:Debabrata Basu) (1924–2001)∙劳伦斯·巴克斯特(英语:Laurence Baxter) (1954–1996)∙迈克尔·巴克斯特(英语:Michael Baxter) (1956-)∙托马斯·贝叶斯 (1702–1761)∙唐·贝瑞(英语:Don Berry (statistician))∙艾伦·伯恩鲍姆(英语:Allan Birnbaum) (1923–1976)∙戴维·布莱克威尔(英语:David Blackwell) (1919–)∙拉迪斯劳斯·博特基威茨(英语:Ladislaus Bortkiewicz) (1868–1931) ∙钱德拉·鲍斯(英语:Raj Chandra Bose) (1901–1987)∙亨利·伯顿利(Henry Bottomley) (1963–)∙亚瑟·里昂·鲍利(英语:Arthur Lyon Bowley) (1869–1957)∙George Box(英语:George Box) (1919–)∙Bento de Jesus Caraça(英语:Bento de Jesus Caraça) (1901–1948) ∙伊恩·卡索耳(英语:Ian Castles)∙乔治·查尔默斯(英语:George Chalmers) (1742–1825)∙戴维·高恩·钱珀瑙恩(英语:D. G. Champernowne) (1912–2000) ∙卡尔·沙利叶(英语:Carl Charlier) (1862–1934)∙切比雪夫 (1821–1894)∙赫尔曼·切尔诺夫(英语:Herman Chernoff) (1923–)∙Alexey Chervonenkis(英语:Alexey Chervonenkis) (1938–)∙Alexander Alexandrovich Chuprov(英语:Alexander Alexandrovich Chuprov) (1874–1926)∙科林·克拉克(英语:Colin Clark) (1905–1989)∙理查德·威廉·巴恩斯·克拉克(英语:Richard W. B. Clarke) (1910–1975)∙罗伯特·汉密尔顿·考特(英语:Robert H. Coats) (1874–1960) ∙科克伦(英语:William Gemmell Cochran) (1909–1980)∙Timothy Augustine Coghlan(英语:Timothy Augustine Coghlan) (1856–1926)∙勒拿·库克(英语:Len Cook) (1949–)∙Gauss Moutinho Cordeiro(英语:Gauss Moutinho Cordeiro) (1952–) ∙大卫·科斯(英语:David Cox (statistician)) (1924–)∙Gertrude Mary Cox(英语:Gertrude Mary Cox) (1900–1978)∙理查德·思雷尔克德·考克斯(英语:Richard Threlkeld Cox) (1898–1991)∙哈拉尔德·克拉梅尔 (1893 – 1985)∙菲利普·大卫(英语:Philip Dawid) (1946–)∙德福内梯(英语:Bruno de Finetti) (1906–1985)∙爱德华兹·戴明 (1900–1993)∙戴康尼斯 (1945–)∙理查德·多尔 (1912–2005)∙Karen Dunnell(英语:Karen Dunnell) (1946–)∙ A. Ross Eckler(英语:A. Ross Eckler) (1901–1991)∙ A. Ross Eckler, Jr.(英语:A. Ross Eckler, Jr.) (1927–)∙弗雷德里克·莫尔顿·艾登(英语:Frederick Morton Eden) (1766–1809)∙弗朗西斯·伊西德罗·埃奇沃思(英语:Francis Ysidro Edgeworth)(1845–1926)∙Anthony William Fairbank Edwards(英语:A. W. F. Edwards) (1935–) ∙邱吉尔·艾森哈特(英语:Churchill Eisenhart) (1913–1994)∙恩格尔 (1821–1896)∙安格那·克瑞乐普·尔蓝(英语:Agner Krarup Erlang) (1878–1929) ∙陈希孺(1934-2005)(数理统计学家)[编辑] F - J∙威廉·法尔(英语:William Farr) (1807–1883)∙费希纳(英语:Gustav Fechner) (1801–1887)∙费利奇(英语:Ivan Fellegi) (1935–)∙霍华德·芬克尔(英语:Howard Finkel) (1950-)∙欧文·费雪 (1867–1947)∙罗纳德·费雪 (1890–1962)∙William Fleetwood(英语:William Fleetwood) (1656–1723)∙约翰·福克斯(英语:John Fox (statistician)) (1945–)∙Arnoldo Frigessi(英语:Arnoldo Frigessi) (1959–)∙乔治·盖洛普(英语:George Gallup) (1901–1984)∙法兰西斯·高尔顿 (1822–1911)∙Seymour Geisser(英语:Seymour Geisser) (1929–2004)∙Lyndhurst Giblin(英语:Lyndhurst Giblin) (1872–1951)∙罗伯特·吉芬 (1837–1910)∙科拉多·基尼(英语:Corrado Gini) (1884–1965)∙格拉斯 (统计学家)(英语:Gene V. Glass) (1940–)∙本杰明·贡培兹(英语:Benjamin Gompertz) (1779–1865)∙I. J. Good(英语:I. J. Good) (1916–)∙Phillip Good(英语:Phillip Good)(1937–)∙威廉·戈塞 (1876–1937) - 以其笔名“学生氏”(Student)著称∙约翰·格朗特(英语:John Graunt) (1620–1674)∙格林伍德(英语:Major Greenwood) (1880–1949)∙Emil Julius Gumbel(英语:Emil Julius Gumbel) (1891–1966)∙Dimitrie Gusti(英语:Dimitrie Gusti) (1880–1955)∙Pierre Gy(英语:Pierre Gy) (1924–)∙Steven Haberman(英语:Steven Haberman) (1951–)∙安德斯·哈尔德 (1913–)∙Jotun Hein(英语:Jotun Hein) (1956–)∙赫尔默特(英语:Friedrich Robert Helmert) (1843–1917)∙吉姆·亨迪(英语:Jim Hendy) (1905-1961)∙杰克·希伯特(英语:Jack Hibbert) (1932–2005)∙Joseph Hilbe(英语:Joseph Hilbe) (1944-)∙奥斯汀·布莱德福·希尔(英语:Austin Bradford Hill) (1897–1991) ∙Nils Lid Hjort(英语:Nils Lid Hjort) (1953–)∙Wassily Hoeffding(英语:Wassily Hoeffding) (1914–1991)∙赫尔曼·荷勒里斯(英语:Herman Hollerith) (1860–1929)∙蒂姆·霍尔特(英语:Tim Holt (statistician)) (1943–)∙Reginald Hawthorn Hooker(英语:Reginald Hawthorn Hooker) (1867–1944)∙哈罗德·霍特林 (1895–1973)∙达莱尔.哈夫(英语:Darrell Huff) (1913–2001)∙Ion Ionescu de la Brad(英语:Ion Ionescu de la Brad) (1818–1891) ∙约瑟夫·奥斯卡·欧文(英语:Joseph Oscar Irwin) (1898–1982) ∙石川馨 (1915–1989)∙比尔·詹姆斯(英语:Bill James) (1949-)∙埃德温·汤普森·杰尼斯(英语:Edwin Thompson Jaynes) (1922–1998) ∙Gwilym Jenkins(英语:Gwilym Jenkins) (1933–1982)∙William H. Jefferys(英语:William H. Jefferys) (1940–)∙哈罗德·杰弗里斯(英语:Harold Jeffreys) (1891–1989)∙ E. Morton Jellinek(英语:E. Morton Jellinek) (1890–1963)∙威廉姆·斯坦利·杰文斯 (1835–1882)∙诺曼·劳埃德·约翰逊(英语:Norman Lloyd Johnson) (1917–2004) ∙约瑟夫·M·朱兰(英语:Joseph M. Juran) (1904–)∙James Jurin(英语:James Jurin) (1684-1750)∙方开泰∙郭祖超(1912-)[编辑] K - N∙大卫·乔治·肯德尔(英语:David George Kendall) (1918–)∙莫里斯·肯得尔(英语:Maurice Kendall) (1907–1983)∙约翰·景文(英语:John Kingman) (1939–)∙莱斯利·基什(英语:Leslie Kish) (1910–2000)∙安德雷·柯尔莫哥洛夫 (1903–1987)∙Jaromír Korčák(英语:Jaromír Korčák) (1895–1989)∙Joseph Kruskal(英语:Joseph Kruskal) (1929–)∙William Kruskal(英语:William Kruskal) (1919–2005)∙蓝光国(英语:Gordon Lan)∙埃蒂恩·拉斯贝尔(英语:Etienne Laspeyres) (1834–1913)∙约翰·劳(英语:John Law (economist)) (1671–1729)∙Lucien le Cam(英语:Lucien le Cam) (1924–2000)∙威尔赫姆·莱克希斯(英语:Wilhelm Lexis) (1837–1914)∙Jarl Waldemar Lindeberg(英语:Jarl Waldemar Lindeberg) (1876–1932)∙Dennis Lindley(英语:Dennis Lindley) (1923–)∙Yuri Vladimirovich Linnik(英语:Yuri Vladimirovich Linnik) (1915–1972)∙阿弗雷德·洛特卡 (1880–1949)∙菲利普·伦德伯格(英语:Filip Lundberg) (1876–1965)∙Prasanta Chandra Mahalanobis(英语:Prasanta Chandra Mahalanobis)(1893–1972)∙南希·曼特尔(英语:Nathan Mantel) (1919–2002)∙唐纳德·麦夸尔特(英语:Donald Marquardt) (1929–1997)∙Motosaburo Masuyama(英语:Motosaburo Masuyama) (1912–2005) ∙Anderson Gray McKendrick(英语:Anderson Gray McKendrick) (1876–1943)∙克劳斯·莫瑟(英语:Claus Moser, Baron Moser) (1922–)∙菲德里克·莫斯提勒(英语:Frederick Mosteller) (1916–2006) ∙威廉·纽马奇(英语:William Newmarch) (1820–1882)∙耶日·奈曼(英语:Jerzy Neyman) (1894–1981)∙弗罗伦斯·南丁格尔 (1820–1910)∙刘大钧∙孟晓犁 (1963–)[编辑] P - T∙伊曼纽尔·帕尔逊(英语:Emanuel Parzen) (1929–∙伊根·皮尔逊(英语:Egon Pearson) (1895–1980)∙卡尔·皮尔逊 (1857–1936)∙威廉·配第 (1623–1687)∙ E.J.G. Pitman(英语:E.J.G. Pitman) (1897–1993)∙威廉·普莱菲(英语:William Playfair) (1759–1823)∙乔治·波利亚 (1887–1985)∙Alwyn Pritchard(英语:Alwyn Pritchard) (1947–)∙凯特勒 (1796–1874)∙霍华德·瑞法(英语:Howard Raiffa)∙Ştefan Ralescu(英语:Ştefan Ralescu) (1952-)∙ C.R. 劳(英语:C.R. Rao) (1920–)∙Georg Rasch(英语:Georg Rasch) (1901–1980)∙Šarūnas Raudys(英语:Šarūnas Raudys) (1941-)∙Olav Reiersøl(英语:Olav Reiersøl) (1908–2001)∙Brian Ripley(英语:Brian Ripley) (1952–)∙赫伯特·罗宾斯(英语:Herbert Robbins) (1922–2001)∙Irving Rosenwater(英语:Irving Rosenwater) (1932–2006)∙S. N. Roy(英语:S. N. Roy) (1906–1966)∙Jeff Sagarin(英语:Jeff Sagarin)∙Leonard Jimmie Savage(英语:Leonard Jimmie Savage) (1917–1971) ∙罗伯特·施赖弗(英语:Robert Schlaifer) (1915–1994)∙阿瑟·舒斯特(英语:Arthur Schuster) (1851–1934)∙豪威·斯奇瓦布(英语:Howie Schwab)∙Tore Schweder(英语:Tore Schweder) (1943–)∙伊丽莎白·斯科特(英语:Elizabeth Scott) (1917–1988)∙Pyotr Semenov-Tyan-Shansky(英语:Pyotr Semenov-Tyan-Shansky)(1827–1914)∙劳埃德·夏普利(英语:Lloyd Shapley) (1923–)∙William Fleetwood Sheppard(英语:William Fleetwood Sheppard)(1863–1936)∙沃特·阿曼德·休哈特(英语:Walter A. Shewhart) (1891–1967) ∙赫伯特·希雪(英语:Herbert Sichel) (1915–1995)∙约翰·辛克莱(英语:John Sinclair (writer)) (1754–1835)∙尤金·斯勒茨基(英语:Eugen Slutsky) (1880–1948)∙阿德里安·史密斯(英语:Adrian Smith (academic))∙Cedric Smith(英语:Cedric Smith) (1917–2002)∙斯内德克(英语:George W. Snedecor) (1881–1974)∙卡尔斯奈德(英语:Carl Snyder) (1869–1946)∙查尔斯·斯皮尔曼(英语:Charles Spearman) (1863–1945)∙David Spiegelhalter(英语:David Spiegelhalter) (1953–)∙乔赛亚·斯坦普(英语:Josiah Stamp, 1st Baron Stamp) (1880–1941) ∙斯蒂芬·施蒂茨勒(英语:Stephen Stigler)∙Dietrich Stoyan(英语:Dietrich Stoyan) (1940–)∙田口玄一 (1924–)∙Thorvald N. Thiele(英语:Thorvald N. Thiele) (1838–1910)∙约翰·塔基(英语:John Tukey) (1915–2000)[编辑] U - Z∙杰西卡·乌兹(英语:Jessica Utts)∙弗拉基米尔·万普尼克 (1935 - )∙亚伯拉罕·瓦尔德(英语:Abraham Wald) (1902–1950)∙克里斯·华莱仕(英语:Chris Wallace (computer scientist)) (1933–2004)∙Waloddi Weibull(英语:Waloddi Weibull) (1887–1979)∙阿诺德·温斯托克(英语:Arnold Weinstock) (1924–2002)∙Harald Ludvig Westergaard(英语:Harald Ludvig Westergaard) (1853–1936)∙Peter Whittle(英语:Peter Whittle) (1927–)∙威尔科克森(英语:Frank Wilcoxon) (1892–1965)∙Martin Wilk(英语:Martin Wilk) (1922–)∙Samuel Stanley Wilks(英语:Samuel Stanley Wilks) (1906–1964) ∙约翰·维希特(英语:John Wishart (statistician)) (1898–1956) ∙Herman Wold(英语:Herman Wold) (1908–1992)∙伍佛维兹(英语:Jacob Wolfowitz) (1910–1981)∙Frank Yates(英语:Frank Yates) (1902–1994)∙Arthur Young(英语:Arthur Young (writer)) (1741–1820)∙犹勒(英语:Udny Yule) (1871–1951)∙齐普夫(英语:George Kingsley Zipf) (1902–1950)∙阿诺德·泽尔纳(英语:Arnold Zellner) (1927–)∙许宝騄(1910-1970)∙薛仲三。
- 1、下载文档前请自行甄别文档内容的完整性,平台不提供额外的编辑、内容补充、找答案等附加服务。
- 2、"仅部分预览"的文档,不可在线预览部分如存在完整性等问题,可反馈申请退款(可完整预览的文档不适用该条件!)。
- 3、如文档侵犯您的权益,请联系客服反馈,我们会尽快为您处理(人工客服工作时间:9:00-18:30)。
Abstract The paper introduces the idea of Bayesian quantile regression employing a likelihood function that is based on the asymmetric Laplace distribution. It is shown that irrespective of the original distribution of the data, the use of the asymmetric Laplace distribution is a very natural and e ective way for modelling Bayesian quantile regression. The paper also demonstrates that improper uniform priors for the unknown model parameters yield a proper joint posterior. The approach is illustrated via a simulated and two real data sets. c 2001 Elsevier Science B.V. All rights reserved Keywords: Asymmetric Laplace distribution; Bayesian inference; Markov chain Monte Carlo methods; Quantile regression
Statistics & Probability Letters 54 (2001) 437 – 447
Bayesian quantile regression
Keming Yu, Rana A. Moyeed ∗
Department of Mathematics and Statistics, University of Plymouth, Drake circus, Plymouth PL4 8AA, UK Received January 2001; received in revised form April 2001
t
is the value, qp , for which P ( t ¡ qp ) = p. (1)
where ÿ(p) is a vector of coe cients dependent on p. ˆ(p), to the quantile regression The pth regression quantile (0 ¡ p ¡ 1) is deÿned as any solution, ÿ minimisation problem min
∗
Corresponding author. E-mail address: r.moyeed@ (R.A. Moyeed).
0167-7152/01/$ - see front matter c 2001 Elsevier Science B.V. All rights reserved PII: S 0 1 6 7 - 7 1 5 2 ( 0 1 ) 0 0 1 2 4 - 9
438
K. Yu, R.A. Moyeed / Statistics & Probability Letters 54 (2001) 437 – 447
term as it is allowed to take any form. Typically, (xt ) = xt ÿ for a vector of coe cients ÿ. The pth (0 ¡ p ¡ 1) quantile of The pth conditional quantile of yt given xt is then simply qp (yt |xt ) = xt ÿ(p);
1. Introduction The classical theory of linear models is essentially a theory for models of conditional expectations. In many applications, however, it is fruitful to go beyond these models. Quantile regression is gradually emerging as a comprehensive approach to the statistical analysis of linear and nonlinear response models. A number of papers have recently appeared on the application of quantile regression (see Cole and Green, 1992; Royston and Altman, 1994; Buchinsky, 1998; Yu and Jones, 1998; He et al., 1998; Koenker and Machado, 1999). Quantile regression supplements the exclusive focus of least squares based methods on the estimation of conditional mean functions with a general technique for estimating families of conditional quantile functions. This greatly expands the exibility of both parametric and nonparametric regression methods. In strictly linear models, a simple approach to estimating the conditional quantiles is suggested in Koenker and Bassett (1978). Consider the following standard linear model: yt = (xt ) + t ; where (xt ) may be thought of as the conditional mean of yt given the vector of regressors xt , and t is the error term with mean zero and constant variance. It is not necessary to specify the distribution of the error
ÿ
t p (yt
− xt ÿ);
(2)
where the loss function
p (u) = u(p
− I (u ¡ 0)): − (1 − p)I (u ¡ 0));
(3)
Equivalently, we may write (3) as
p (u) = u(pI (u ¿ 0)
or |u| + (2p − 1)u · 2 Contrary to the commonly used quadratic loss function for mean regression estimation, the quantile regression links to a special class of loss functions which has robust properties (see Huber, 1981). For example, the conditional median regression is more robust than the conditional mean regression in terms of outliers in the observations. The use of Bayesian inference in generalized linear and additive models is quite standard these days. The relative ease with which Markov chain Monte Carlo (MCMC) methods may be used for obtaining the posterior distributions, even in complex situations, has made Bayesian inference very useful and attractive. Unlike conventional methods, Bayesian inference provides one with the entire posterior distribution of the parameter of interest. In addition, it allows for parameter uncertainty to be taken into account when making predictions. However, in the area of quantile regression, there is very little in the literature along the Bayesian lines, the only exception being Fatti and Senaoana (1998). In this paper, we adopt a Bayesian approach to quantile regression which is quite di erent from what has been done previously in this context. Irrespective of the actual distribution of the data, Bayesian inference for quantile regression proceeds by forming the likelihood function based on the asymmetric Laplace distribution (Koenker and Bassett, 1978). In general one may choose any prior, but we show that the use of improper uniform priors produces a proper joint posterior. Section 2 of the paper, gives a brief overview of the asymmetric Laplace distribution. Section 3 describes the theoretical and computational framework of quantile regression from a Bayesian perspective. Section 4 shows that the use of improper priors for the quantile regression parameters leads to proper posterior distributions. In Section 5, we illustrate the implementation of Bayesian quantile regression through a simulated case-study and two real examples. We end with a short discussion in Section 6.