Simulation of Spatial and Temporal Radiation Exposures for ISS in the South Atlantic Anomal
复杂地形条件下城市热岛及局地环流特征的数值模拟

复杂地形条件下城市热岛及局地环流特征的数值模拟孙永;王咏薇;高阳华;王恪非;何泽能;杜钦;陈志军【摘要】Using WRF model coupled with multilayer urban canopy scheme BEP (Building Environment Parameterization) and BEM (Building Energy Model) scheme, a simulation was conducted to explore characteristics and causes of Chongqing urban heat island and impact of local circulation on it. There were two simulation cases conducted, one was URBAN case that utilized real Chongqing land use data, another was NOURBAN case that replaced urban category with crop in order to understand impact of urban on Chongqing heat island. Results showthat: (1) WRF model produces good results compared to observed 2m air temperature. Errors mainly occur at noon temperature peak and morning temperature valley, which are caused by the characteristics of urban land use and unreal building parameters. (2) BEP+BEMscheme can simulate well spatial and temporal features of urban heat island in Chongqing. Spatial distribution of temperature in Chongqing is influenced by topography and urban underlying surface. When closer to the city, greater temperature is affected by the urbanization, and higher temperature locates at lowelevation. (3) Urban 3D surface leads to trap effect in urban surface albedo (Total reflectivity of urban surface is low), and the urban upward shortwave radiation is less than about 20 in suburbs. Sensible heat is a major factor in urban energy balance however latent heat in suburbs. The larger urban surface heat storage and the waste heat of air-conditioner released to theatmosphere at night are important reasons for urban heat island conformation. (4) The background wind field is mainly southeast wind in the simulated area. The wind speed is higher in mountain area and lower in urban area, which reflects the aerodynamic effects of dense urban buildings on the low-level atmospheric flowfield, as well as the characteristics of valley wind circulation over complex valley terrain. There are high mountains in the western and southeastern sides of the city, which block the outflowfrom the city, let the background wind to climb or circle around the mountains, and contribute to the enhancement of urban heat island.%应用基于多层城市冠层方案BEP (Building Environment Parameterization) 增加室内空调系统影响的建筑物能量模式BEM (Building Energy Model) 方案的WRF模式, 模拟研究重庆热岛的特征、成因以及局地环流对热岛形成的影响.文中共有两个算例, 一为重庆真实下垫面算例, 称之为URBAN 算例, 二为将城市下垫面替换为耕地下垫面的对比算例, 称之为NOURBAN算例.结果表明:1) WRF方案模拟结果与观测2 m气温的对比吻合较好, 误差主要出现在正午温度峰值和凌晨温度谷值处, 由城市下垫面特性及城市内建筑分布误差引起.2) BEP+BEM方案较好地模拟出了重庆地区的热岛分布的空间和时间特征.重庆市温度的分布受地形和城市下垫面的双重影响, 越靠近城区, 温度的分布受城市化影响就越大, 在海拔低处, 温度就越高.3) 城区立体三维表面对辐射的陷阱作用导致城市表面总体反射率小, 向上短波辐射小于郊区约20 W/m2.城市表面以感热排放为主, 而郊区则表现为潜热的作用占主导.夜间城市地表储热以及空调废热向大气释放, 是城市热岛形成的重要原因.4) 模拟区域背景风场主要为东南风, 局地环流呈现出越靠近山区风速越大、城市区域风速较小的特性, 体现了城市密集的建筑群对低层大气流场的空气动力学效应, 以及复杂山谷地形的山谷风环流特性.在市区的西侧和东南侧均有高大山脉阻挡, 山脉对城市出流的阻碍作用、气流越山与绕流运动对城市热岛的形成有一定影响.【期刊名称】《大气科学学报》【年(卷),期】2019(042)002【总页数】13页(P280-292)【关键词】城市热岛;WRF模式;城市冠层方案【作者】孙永;王咏薇;高阳华;王恪非;何泽能;杜钦;陈志军【作者单位】南京信息工程大学大气环境中心,江苏南京 210044;南京信息工程大学大气环境中心,江苏南京 210044;重庆市气象科学研究所,重庆 401147;南京信息工程大学大气环境中心,江苏南京 210044;重庆市气象科学研究所,重庆 401147;重庆市气象科学研究所,重庆 401147;重庆市气象科学研究所,重庆 401147【正文语种】中文高温天气的形成受辐射增温、平流增温、绝热下沉增温等因素的影响(张尚印等,2005)。
Modeling the Spatial Dynamics of Regional Land Use_The CLUE-S Model

Modeling the Spatial Dynamics of Regional Land Use:The CLUE-S ModelPETER H.VERBURG*Department of Environmental Sciences Wageningen UniversityP.O.Box376700AA Wageningen,The NetherlandsandFaculty of Geographical SciencesUtrecht UniversityP.O.Box801153508TC Utrecht,The NetherlandsWELMOED SOEPBOERA.VELDKAMPDepartment of Environmental Sciences Wageningen UniversityP.O.Box376700AA Wageningen,The NetherlandsRAMIL LIMPIADAVICTORIA ESPALDONSchool of Environmental Science and Management University of the Philippines Los Ban˜osCollege,Laguna4031,Philippines SHARIFAH S.A.MASTURADepartment of GeographyUniversiti Kebangsaan Malaysia43600BangiSelangor,MalaysiaABSTRACT/Land-use change models are important tools for integrated environmental management.Through scenario analysis they can help to identify near-future critical locations in the face of environmental change.A dynamic,spatially ex-plicit,land-use change model is presented for the regional scale:CLUE-S.The model is specifically developed for the analysis of land use in small regions(e.g.,a watershed or province)at afine spatial resolution.The model structure is based on systems theory to allow the integrated analysis of land-use change in relation to socio-economic and biophysi-cal driving factors.The model explicitly addresses the hierar-chical organization of land use systems,spatial connectivity between locations and stability.Stability is incorporated by a set of variables that define the relative elasticity of the actual land-use type to conversion.The user can specify these set-tings based on expert knowledge or survey data.Two appli-cations of the model in the Philippines and Malaysia are used to illustrate the functioning of the model and its validation.Land-use change is central to environmental man-agement through its influence on biodiversity,water and radiation budgets,trace gas emissions,carbon cy-cling,and livelihoods(Lambin and others2000a, Turner1994).Land-use planning attempts to influence the land-use change dynamics so that land-use config-urations are achieved that balance environmental and stakeholder needs.Environmental management and land-use planning therefore need information about the dynamics of land use.Models can help to understand these dynamics and project near future land-use trajectories in order to target management decisions(Schoonenboom1995).Environmental management,and land-use planning specifically,take place at different spatial and organisa-tional levels,often corresponding with either eco-re-gional or administrative units,such as the national or provincial level.The information needed and the man-agement decisions made are different for the different levels of analysis.At the national level it is often suffi-cient to identify regions that qualify as“hot-spots”of land-use change,i.e.,areas that are likely to be faced with rapid land use conversions.Once these hot-spots are identified a more detailed land use change analysis is often needed at the regional level.At the regional level,the effects of land-use change on natural resources can be determined by a combina-tion of land use change analysis and specific models to assess the impact on natural resources.Examples of this type of model are water balance models(Schulze 2000),nutrient balance models(Priess and Koning 2001,Smaling and Fresco1993)and erosion/sedimen-tation models(Schoorl and Veldkamp2000).Most of-KEY WORDS:Land-use change;Modeling;Systems approach;Sce-nario analysis;Natural resources management*Author to whom correspondence should be addressed;email:pverburg@gissrv.iend.wau.nlDOI:10.1007/s00267-002-2630-x Environmental Management Vol.30,No.3,pp.391–405©2002Springer-Verlag New York Inc.ten these models need high-resolution data for land use to appropriately simulate the processes involved.Land-Use Change ModelsThe rising awareness of the need for spatially-ex-plicit land-use models within the Land-Use and Land-Cover Change research community(LUCC;Lambin and others2000a,Turner and others1995)has led to the development of a wide range of land-use change models.Whereas most models were originally devel-oped for deforestation(reviews by Kaimowitz and An-gelsen1998,Lambin1997)more recent efforts also address other land use conversions such as urbaniza-tion and agricultural intensification(Brown and others 2000,Engelen and others1995,Hilferink and Rietveld 1999,Lambin and others2000b).Spatially explicit ap-proaches are often based on cellular automata that simulate land use change as a function of land use in the neighborhood and a set of user-specified relations with driving factors(Balzter and others1998,Candau 2000,Engelen and others1995,Wu1998).The speci-fication of the neighborhood functions and transition rules is done either based on the user’s expert knowl-edge,which can be a problematic process due to a lack of quantitative understanding,or on empirical rela-tions between land use and driving factors(e.g.,Pi-janowski and others2000,Pontius and others2000).A probability surface,based on either logistic regression or neural network analysis of historic conversions,is made for future conversions.Projections of change are based on applying a cut-off value to this probability sur-face.Although appropriate for short-term projections,if the trend in land-use change continues,this methodology is incapable of projecting changes when the demands for different land-use types change,leading to a discontinua-tion of the trends.Moreover,these models are usually capable of simulating the conversion of one land-use type only(e.g.deforestation)because they do not address competition between land-use types explicitly.The CLUE Modeling FrameworkThe Conversion of Land Use and its Effects(CLUE) modeling framework(Veldkamp and Fresco1996,Ver-burg and others1999a)was developed to simulate land-use change using empirically quantified relations be-tween land use and its driving factors in combination with dynamic modeling.In contrast to most empirical models,it is possible to simulate multiple land-use types simultaneously through the dynamic simulation of competition between land-use types.This model was developed for the national and con-tinental level,applications are available for Central America(Kok and Winograd2001),Ecuador(de Kon-ing and others1999),China(Verburg and others 2000),and Java,Indonesia(Verburg and others 1999b).For study areas with such a large extent the spatial resolution of analysis was coarse(pixel size vary-ing between7ϫ7and32ϫ32km).This is a conse-quence of the impossibility to acquire data for land use and all driving factors atfiner spatial resolutions.A coarse spatial resolution requires a different data rep-resentation than the common representation for data with afine spatial resolution.Infine resolution grid-based approaches land use is defined by the most dom-inant land-use type within the pixel.However,such a data representation would lead to large biases in the land-use distribution as some class proportions will di-minish and other will increase with scale depending on the spatial and probability distributions of the cover types(Moody and Woodcock1994).In the applications of the CLUE model at the national or continental level we have,therefore,represented land use by designating the relative cover of each land-use type in each pixel, e.g.a pixel can contain30%cultivated land,40%grass-land,and30%forest.This data representation is di-rectly related to the information contained in the cen-sus data that underlie the applications.For each administrative unit,census data denote the number of hectares devoted to different land-use types.When studying areas with a relatively small spatial ex-tent,we often base our land-use data on land-use maps or remote sensing images that denote land-use types respec-tively by homogeneous polygons or classified pixels. When converted to a raster format this results in only one, dominant,land-use type occupying one unit of analysis. The validity of this data representation depends on the patchiness of the landscape and the pixel size chosen. Most sub-national land use studies use this representation of land use with pixel sizes varying between a few meters up to about1ϫ1km.The two different data represen-tations are shown in Figure1.Because of the differences in data representation and other features that are typical for regional appli-cations,the CLUE model can not directly be applied at the regional scale.This paper describes the mod-ified modeling approach for regional applications of the model,now called CLUE-S(the Conversion of Land Use and its Effects at Small regional extent). The next section describes the theories underlying the development of the model after which it is de-scribed how these concepts are incorporated in the simulation model.The functioning of the model is illustrated for two case-studies and is followed by a general discussion.392P.H.Verburg and othersCharacteristics of Land-Use SystemsThis section lists the main concepts and theories that are prevalent for describing the dynamics of land-use change being relevant for the development of land-use change models.Land-use systems are complex and operate at the interface of multiple social and ecological systems.The similarities between land use,social,and ecological systems allow us to use concepts that have proven to be useful for studying and simulating ecological systems in our analysis of land-use change (Loucks 1977,Adger 1999,Holling and Sanderson 1996).Among those con-cepts,connectivity is important.The concept of con-nectivity acknowledges that locations that are at a cer-tain distance are related to each other (Green 1994).Connectivity can be a direct result of biophysical pro-cesses,e.g.,sedimentation in the lowlands is a direct result of erosion in the uplands,but more often it is due to the movement of species or humans through the nd degradation at a certain location will trigger farmers to clear land at a new location.Thus,changes in land use at this new location are related to the land-use conditions in the other location.In other instances more complex relations exist that are rooted in the social and economic organization of the system.The hierarchical structure of social organization causes some lower level processes to be constrained by higher level dynamics,e.g.,the establishments of a new fruit-tree plantation in an area near to the market might in fluence prices in such a way that it is no longer pro fitable for farmers to produce fruits in more distant areas.For studying this situation an-other concept from ecology,hierarchy theory,is use-ful (Allen and Starr 1982,O ’Neill and others 1986).This theory states that higher level processes con-strain lower level processes whereas the higher level processes might emerge from lower level dynamics.This makes the analysis of the land-use system at different levels of analysis necessary.Connectivity implies that we cannot understand land use at a certain location by solely studying the site characteristics of that location.The situation atneigh-Figure 1.Data representation and land-use model used for respectively case-studies with a national/continental extent and local/regional extent.Modeling Regional Land-Use Change393boring or even more distant locations can be as impor-tant as the conditions at the location itself.Land-use and land-cover change are the result of many interacting processes.Each of these processes operates over a range of scales in space and time.These processes are driven by one or more of these variables that influence the actions of the agents of land-use and cover change involved.These variables are often re-ferred to as underlying driving forces which underpin the proximate causes of land-use change,such as wood extraction or agricultural expansion(Geist and Lambin 2001).These driving factors include demographic fac-tors(e.g.,population pressure),economic factors(e.g., economic growth),technological factors,policy and institutional factors,cultural factors,and biophysical factors(Turner and others1995,Kaimowitz and An-gelsen1998).These factors influence land-use change in different ways.Some of these factors directly influ-ence the rate and quantity of land-use change,e.g.the amount of forest cleared by new incoming migrants. Other factors determine the location of land-use change,e.g.the suitability of the soils for agricultural land use.Especially the biophysical factors do pose constraints to land-use change at certain locations, leading to spatially differentiated pathways of change.It is not possible to classify all factors in groups that either influence the rate or location of land-use change.In some cases the same driving factor has both an influ-ence on the quantity of land-use change as well as on the location of land-use change.Population pressure is often an important driving factor of land-use conver-sions(Rudel and Roper1997).At the same time it is the relative population pressure that determines which land-use changes are taking place at a certain location. Intensively cultivated arable lands are commonly situ-ated at a limited distance from the villages while more extensively managed grasslands are often found at a larger distance from population concentrations,a rela-tion that can be explained by labor intensity,transport costs,and the quality of the products(Von Thu¨nen 1966).The determination of the driving factors of land use changes is often problematic and an issue of dis-cussion(Lambin and others2001).There is no unify-ing theory that includes all processes relevant to land-use change.Reviews of case studies show that it is not possible to simply relate land-use change to population growth,poverty,and infrastructure.Rather,the inter-play of several proximate as well as underlying factors drive land-use change in a synergetic way with large variations caused by location specific conditions (Lambin and others2001,Geist and Lambin2001).In regional modeling we often need to rely on poor data describing this complexity.Instead of using the under-lying driving factors it is needed to use proximate vari-ables that can represent the underlying driving factors. Especially for factors that are important in determining the location of change it is essential that the factor can be mapped quantitatively,representing its spatial vari-ation.The causality between the underlying driving factors and the(proximate)factors used in modeling (in this paper,also referred to as“driving factors”) should be certified.Other system properties that are relevant for land-use systems are stability and resilience,concepts often used to describe ecological systems and,to some extent, social systems(Adger2000,Holling1973,Levin and others1998).Resilience refers to the buffer capacity or the ability of the ecosystem or society to absorb pertur-bations,or the magnitude of disturbance that can be absorbed before a system changes its structure by changing the variables and processes that control be-havior(Holling1992).Stability and resilience are con-cepts that can also be used to describe the dynamics of land-use systems,that inherit these characteristics from both ecological and social systems.Due to stability and resilience of the system disturbances and external in-fluences will,mostly,not directly change the landscape structure(Conway1985).After a natural disaster lands might be abandoned and the population might tempo-rally migrate.However,people will in most cases return after some time and continue land-use management practices as before,recovering the land-use structure (Kok and others2002).Stability in the land-use struc-ture is also a result of the social,economic,and insti-tutional structure.Instead of a direct change in the land-use structure upon a fall in prices of a certain product,farmers will wait a few years,depending on the investments made,before they change their cropping system.These characteristics of land-use systems provide a number requirements for the modelling of land-use change that have been used in the development of the CLUE-S model,including:●Models should not analyze land use at a single scale,but rather include multiple,interconnected spatial scales because of the hierarchical organization of land-use systems.●Special attention should be given to the drivingfactors of land-use change,distinguishing drivers that determine the quantity of change from drivers of the location of change.●Sudden changes in driving factors should not di-rectly change the structure of the land-use system asa consequence of the resilience and stability of theland-use system.394P.H.Verburg and others●The model structure should allow spatial interac-tions between locations and feedbacks from higher levels of organization.Model DescriptionModel StructureThe model is sub-divided into two distinct modules,namely a non-spatial demand module and a spatially explicit allocation procedure (Figure 2).The non-spa-tial module calculates the area change for all land-use types at the aggregate level.Within the second part of the model these demands are translated into land-use changes at different locations within the study region using a raster-based system.For the land-use demand module,different alterna-tive model speci fications are possible,ranging from simple trend extrapolations to complex economic mod-els.The choice for a speci fic model is very much de-pendent on the nature of the most important land-use conversions taking place within the study area and the scenarios that need to be considered.Therefore,the demand calculations will differ between applications and scenarios and need to be decided by the user for the speci fic situation.The results from the demandmodule need to specify,on a yearly basis,the area covered by the different land-use types,which is a direct input for the allocation module.The rest of this paper focuses on the procedure to allocate these demands to land-use conversions at speci fic locations within the study area.The allocation is based upon a combination of em-pirical,spatial analysis,and dynamic modelling.Figure 3gives an overview of the procedure.The empirical analysis unravels the relations between the spatial dis-tribution of land use and a series of factors that are drivers and constraints of land use.The results of this empirical analysis are used within the model when sim-ulating the competition between land-use types for a speci fic location.In addition,a set of decision rules is speci fied by the user to restrict the conversions that can take place based on the actual land-use pattern.The different components of the procedure are now dis-cussed in more detail.Spatial AnalysisThe pattern of land use,as it can be observed from an airplane window or through remotely sensed im-ages,reveals the spatial organization of land use in relation to the underlying biophysical andsocio-eco-Figure 2.Overview of the modelingprocedure.Figure 3.Schematic represen-tation of the procedure to allo-cate changes in land use to a raster based map.Modeling Regional Land-Use Change395nomic conditions.These observations can be formal-ized by overlaying this land-use pattern with maps de-picting the variability in biophysical and socio-economic conditions.Geographical Information Systems(GIS)are used to process all spatial data and convert these into a regular grid.Apart from land use, data are gathered that represent the assumed driving forces of land use in the study area.The list of assumed driving forces is based on prevalent theories on driving factors of land-use change(Lambin and others2001, Kaimowitz and Angelsen1998,Turner and others 1993)and knowledge of the conditions in the study area.Data can originate from remote sensing(e.g., land use),secondary statistics(e.g.,population distri-bution),maps(e.g.,soil),and other sources.To allow a straightforward analysis,the data are converted into a grid based system with a cell size that depends on the resolution of the available data.This often involves the aggregation of one or more layers of thematic data,e.g. it does not make sense to use a30-m resolution if that is available for land-use data only,while the digital elevation model has a resolution of500m.Therefore, all data are aggregated to the same resolution that best represents the quality and resolution of the data.The relations between land use and its driving fac-tors are thereafter evaluated using stepwise logistic re-gression.Logistic regression is an often used method-ology in land-use change research(Geoghegan and others2001,Serneels and Lambin2001).In this study we use logistic regression to indicate the probability of a certain grid cell to be devoted to a land-use type given a set of driving factors following:LogͩP i1ϪP i ͪϭ0ϩ1X1,iϩ2X2,i......ϩn X n,iwhere P i is the probability of a grid cell for the occur-rence of the considered land-use type and the X’s are the driving factors.The stepwise procedure is used to help us select the relevant driving factors from a larger set of factors that are assumed to influence the land-use pattern.Variables that have no significant contribution to the explanation of the land-use pattern are excluded from thefinal regression equation.Where in ordinal least squares regression the R2 gives a measure of modelfit,there is no equivalent for logistic regression.Instead,the goodness offit can be evaluated with the ROC method(Pontius and Schnei-der2000,Swets1986)which evaluates the predicted probabilities by comparing them with the observed val-ues over the whole domain of predicted probabilities instead of only evaluating the percentage of correctly classified observations at afixed cut-off value.This is an appropriate methodology for our application,because we will use a wide range of probabilities within the model calculations.The influence of spatial autocorrelation on the re-gression results can be minimized by only performing the regression on a random sample of pixels at a certain minimum distance from one another.Such a selection method is adopted in order to maximize the distance between the selected pixels to attenuate the problem associated with spatial autocorrelation.For case-studies where autocorrelation has an important influence on the land-use structure it is possible to further exploit it by incorporating an autoregressive term in the regres-sion equation(Overmars and others2002).Based upon the regression results a probability map can be calculated for each land-use type.A new probabil-ity map is calculated every year with updated values for the driving factors that are projected to change in time,such as the population distribution or accessibility.Decision RulesLand-use type or location specific decision rules can be specified by the user.Location specific decision rules include the delineation of protected areas such as nature reserves.If a protected area is specified,no changes are allowed within this area.For each land-use type decision rules determine the conditions under which the land-use type is allowed to change in the next time step.These decision rules are implemented to give certain land-use types a certain resistance to change in order to generate the stability in the land-use structure that is typical for many landscapes.Three different situations can be distinguished and for each land-use type the user should specify which situation is most relevant for that land-use type:1.For some land-use types it is very unlikely that theyare converted into another land-use type after their first conversion;as soon as an agricultural area is urbanized it is not expected to return to agriculture or to be converted into forest cover.Unless a de-crease in area demand for this land-use type occurs the locations covered by this land use are no longer evaluated for potential land-use changes.If this situation is selected it also holds that if the demand for this land-use type decreases,there is no possi-bility for expansion in other areas.In other words, when this setting is applied to forest cover and deforestation needs to be allocated,it is impossible to reforest other areas at the same time.2.Other land-use types are converted more easily.Aswidden agriculture system is most likely to be con-verted into another land-use type soon after its396P.H.Verburg and othersinitial conversion.When this situation is selected for a land-use type no restrictions to change are considered in the allocation module.3.There is also a number of land-use types that oper-ate in between these two extremes.Permanent ag-riculture and plantations require an investment for their establishment.It is therefore not very likely that they will be converted very soon after into another land-use type.However,in the end,when another land-use type becomes more pro fitable,a conversion is possible.This situation is dealt with by de fining the relative elasticity for change (ELAS u )for the land-use type into any other land use type.The relative elasticity ranges between 0(similar to Situation 2)and 1(similar to Situation 1).The higher the de fined elasticity,the more dif ficult it gets to convert this land-use type.The elasticity should be de fined based on the user ’s knowledge of the situation,but can also be tuned during the calibration of the petition and Actual Allocation of Change Allocation of land-use change is made in an iterative procedure given the probability maps,the decision rules in combination with the actual land-use map,and the demand for the different land-use types (Figure 4).The following steps are followed in the calculation:1.The first step includes the determination of all grid cells that are allowed to change.Grid cells that are either part of a protected area or under a land-use type that is not allowed to change (Situation 1,above)are excluded from further calculation.2.For each grid cell i the total probability (TPROP i,u )is calculated for each of the land-use types u accord-ing to:TPROP i,u ϭP i,u ϩELAS u ϩITER u ,where ITER u is an iteration variable that is speci fic to the land use.ELAS u is the relative elasticity for change speci fied in the decision rules (Situation 3de-scribed above)and is only given a value if grid-cell i is already under land use type u in the year considered.ELAS u equals zero if all changes are allowed (Situation 2).3.A preliminary allocation is made with an equalvalue of the iteration variable (ITER u )for all land-use types by allocating the land-use type with the highest total probability for the considered grid cell.This will cause a number of grid cells to change land use.4.The total allocated area of each land use is nowcompared to the demand.For land-use types where the allocated area is smaller than the demanded area the value of the iteration variable is increased.For land-use types for which too much is allocated the value is decreased.5.Steps 2to 4are repeated as long as the demandsare not correctly allocated.When allocation equals demand the final map is saved and the calculations can continue for the next yearly timestep.Figure 5shows the development of the iteration parameter ITER u for different land-use types during asimulation.Figure 4.Representation of the iterative procedure for land-use changeallocation.Figure 5.Change in the iteration parameter (ITER u )during the simulation within one time-step.The different lines rep-resent the iteration parameter for different land-use types.The parameter is changed for all land-use types synchronously until the allocated land use equals the demand.Modeling Regional Land-Use Change397Multi-Scale CharacteristicsOne of the requirements for land-use change mod-els are multi-scale characteristics.The above described model structure incorporates different types of scale interactions.Within the iterative procedure there is a continuous interaction between macro-scale demands and local land-use suitability as determined by the re-gression equations.When the demand changes,the iterative procedure will cause the land-use types for which demand increased to have a higher competitive capacity (higher value for ITER u )to ensure enough allocation of this land-use type.Instead of only being determined by the local conditions,captured by the logistic regressions,it is also the regional demand that affects the actually allocated changes.This allows the model to “overrule ”the local suitability,it is not always the land-use type with the highest probability according to the logistic regression equation (P i,u )that the grid cell is allocated to.Apart from these two distinct levels of analysis there are also driving forces that operate over a certain dis-tance instead of being locally important.Applying a neighborhood function that is able to represent the regional in fluence of the data incorporates this type of variable.Population pressure is an example of such a variable:often the in fluence of population acts over a certain distance.Therefore,it is not the exact location of peoples houses that determines the land-use pattern.The average population density over a larger area is often a more appropriate variable.Such a population density surface can be created by a neighborhood func-tion using detailed spatial data.The data generated this way can be included in the spatial analysis as anotherindependent factor.In the application of the model in the Philippines,described hereafter,we applied a 5ϫ5focal filter to the population map to generate a map representing the general population pressure.Instead of using these variables,generated by neighborhood analysis,it is also possible to use the more advanced technique of multi-level statistics (Goldstein 1995),which enable a model to include higher-level variables in a straightforward manner within the regression equa-tion (Polsky and Easterling 2001).Application of the ModelIn this paper,two examples of applications of the model are provided to illustrate its function.TheseTable nd-use classes and driving factors evaluated for Sibuyan IslandLand-use classes Driving factors (location)Forest Altitude (m)GrasslandSlope Coconut plantation AspectRice fieldsDistance to town Others (incl.mangrove and settlements)Distance to stream Distance to road Distance to coast Distance to port Erosion vulnerability GeologyPopulation density(neighborhood 5ϫ5)Figure 6.Location of the case-study areas.398P.H.Verburg and others。
基于组分区分的亚热带湿地松人工林土壤呼吸对氮添加的响应
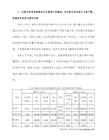
carbon ( C) budget and C sequestration in the terrestrial ecosystem. Taking a subtropical Pinus elliottii Plantation in China as the research availability was conducted by field simulation control experiment based on the distinction of different components of soil respirationꎬ and a
XIAO Shengsheng 1ꎬ2 ꎬ WANG Jia 2 ꎬ SHI Zheng 2 ꎬ ZHAO Jiading 1ꎬ2 ꎬ TANG Chongjun 1ꎬ2
Abstract: The increase of nitrogen ( N) deposition would obviously disturb the soil respirationꎬ further make important influences on the
收稿日期: 2017 ̄10 ̄17㊀ ㊀ ㊀ 修订日期: 2018 ̄03 ̄20
respiration were 3������ 91ꎬ 2������ 30 and 1������ 73 μmol∕(m2������ s)ꎬ respectively under CK (0)ꎬ LN treatment (60 kg∕(hm2������ a)) and HN treatment (120
objectꎬ a quantitative study about the responses of the root autotrophic respirationꎬ microbial heterotrophic respiration to the varying N preliminary discussion on the biogeochemistry and microbiological mechanism of the response were also made. Results showed that: (1) The dynamic characteristics of the total soil respirationꎬ root respiration and microbial respiration displayed obvious single peak curve in both 2015 and 2016ꎬ with the maximum respiration rates observed in July or August. Simulated N deposition had no significant influence on the seasonal pattern of soil respiration rates. (2) The annual average rates of the total soil respirationꎬ root respiration and microbial
湍流脉动速度的英文
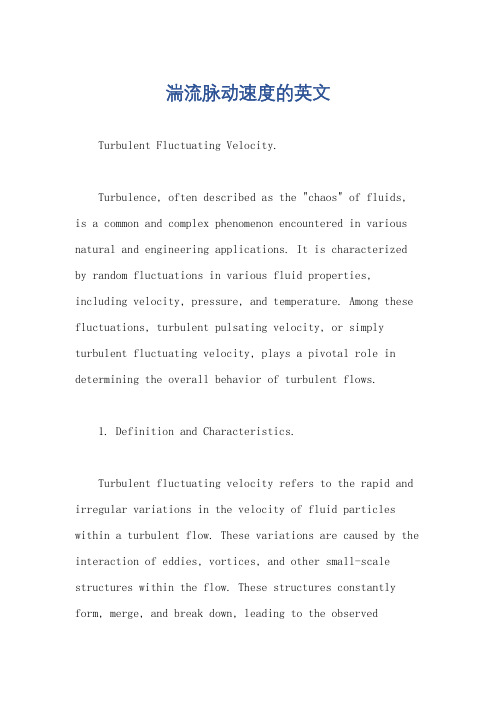
湍流脉动速度的英文Turbulent Fluctuating Velocity.Turbulence, often described as the "chaos" of fluids, is a common and complex phenomenon encountered in various natural and engineering applications. It is characterized by random fluctuations in various fluid properties, including velocity, pressure, and temperature. Among these fluctuations, turbulent pulsating velocity, or simply turbulent fluctuating velocity, plays a pivotal role in determining the overall behavior of turbulent flows.1. Definition and Characteristics.Turbulent fluctuating velocity refers to the rapid and irregular variations in the velocity of fluid particles within a turbulent flow. These variations are caused by the interaction of eddies, vortices, and other small-scale structures within the flow. These structures constantly form, merge, and break down, leading to the observedfluctuations.The magnitude of these fluctuations is typically much larger than the mean velocity of the flow and can be several orders of magnitude higher. They are also highly uncorrelated, meaning that the velocity at one point in the flow does not depend on the velocity at another point, unless they are separated by a distance comparable to the size of the turbulent eddies.2. Importance of Turbulent Fluctuating Velocity.Turbulent fluctuating velocity is crucial in various fluid dynamics applications. It significantly impacts heat transfer, mass transfer, and the mixing of fluids. For example, in heat exchangers, the turbulent fluctuating velocity enhances the rate of heat transfer between two fluids by increasing the effective surface area for heat exchange.In addition, turbulent fluctuating velocity also plays a key role in determining the overall resistance or dragexperienced by objects placed within a turbulent flow. The fluctuating velocities cause pressure fluctuations on the object's surface, leading to additional drag forces.3. Measurement and Analysis.Measuring turbulent fluctuating velocity is a challenging task due to its random and transient nature. However, several techniques have been developed to capture these fluctuations, including hot-wire anemometry, laser Doppler anemometry, and particle image velocimetry.These measurements provide valuable insights into the characteristics of turbulent flows, such as the statistics of velocity fluctuations, their spatial and temporal correlations, and the energy spectrum of turbulent eddies.4. Modeling and Simulation.Modeling and simulating turbulent fluctuating velocity require sophisticated numerical techniques and computational resources. turbulence models, such as theReynolds-Averaged Navier-Stokes (RANS) model and Large Eddy Simulation (LES), are commonly used to predict the behavior of turbulent flows.These models aim to capture the effects of turbulent fluctuating velocity by introducing additional terms or equations into the governing fluid dynamics equations.While RANS models focus on the statistical properties of turbulence, LES aims to resolve the largest eddies directly and model the smaller ones.5. Conclusion.Turbulent fluctuating velocity is a crucial aspect of turbulent flows, affecting various fluid dynamics phenomena. Understanding its characteristics and behavior is essential for predicting and controlling turbulent flows in various applications, including energy conversion, transportation, and environmental engineering.With ongoing research and the continuous development of new measurement techniques and numerical models, ourunderstanding of turbulent fluctuating velocity and its impact on turbulent flows will continue to deepen.。
红外热像仪测试系统的研制与精度验证_李颖文
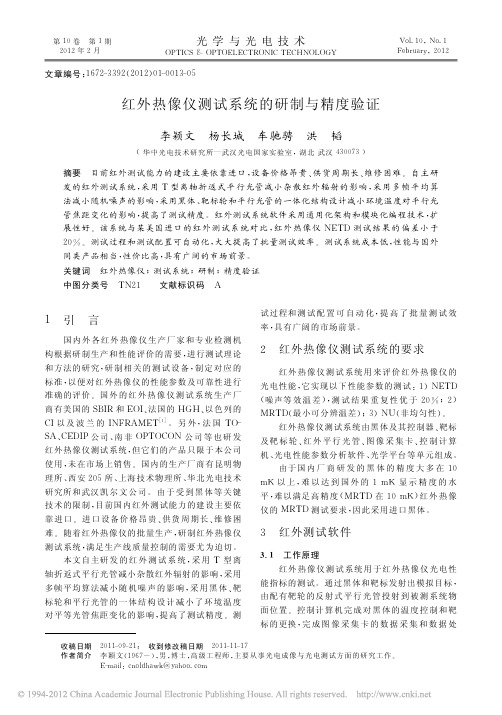
( ) MRTDf=
|dTf+ -dTf-| 2Trans其 中 ,Trans为 平 行 光 管 的 红 外 透 过 率 。
4 测试结果及精度验证
对红外平行光管装调的主要技术指标是要求 轴上点的波像差 RMS小于1/20λ(λ=632.8nm), 因此采用 数 字 化 的 激 光 干 涉 仪 完 成 红 外 的 装 调。 对离轴抛物镜部件和平面镜部件的装调均通过激 光 干 涉 仪 完 成 ,如 图 2 所 示 ,调 整 精 度 极 高 ,对 系 统 中各光学元件的调整以系统最终检测结果满足轴 上点波前精度要求为止。
第 10 卷 第 1 期 2012年2月
光学与光电技术
OPTICS & OPTOELECTRONIC TECHNOLOGY
文 章 编 号 :1672-3392(2012)01-0013-05
Vol.10,No.1 February,2012
红外热像仪测试系统的研制与精度验证
李颖文 杨长城 车驰骋 洪 韬
(华中光电技术研究所—武汉光电国家实验室,湖北 武汉 430073 )
摘要 目前红外测试能力的建设主要依靠进口,设 备 价 格 昂 贵、供 货 周 期 长、维 修 困 难。 自 主 研 发的红外测试系统,采用 T 型离轴 折 返 式 平 行 光 管 减 小 杂 散 红 外 辐 射 的 影 响,采 用 多 帧 平 均 算 法 减 小 随 机 噪 声 的 影 响 ,采 用 黑 体 、靶 标 轮 和 平 行 光 管 的 一 体 化 结 构 设 计 减 小 环 境 温 度 对 平 行 光 管 焦 距 变 化 的 影 响 ,提 高 了 测 试 精 度 。 红 外 测 试 系 统 软 件 采 用 通 用 化 架 构 和 模 块 化 编 程 技 术 ,扩 展性好。该系统与某美国进 口 的 红 外 测 试 系 统 对 比,红 外 热 像 仪 NETD 测 试 结 果 的 偏 差 小 于 20% 。 测 试 过 程 和 测 试 配 置 可 自 动 化 ,大 大 提 高 了 批 量 测 试 效 率 。 测 试 系 统 成 本 低 ,性 能 与 国 外 同 类 产 品 相 当 ,性 价 比 高 ,具 有 广 阔 的 市 场 前 景 。 关 键 词 红 外 热 像 仪 ;测 试 系 统 ;研 制 ;精 度 验 证 中 图 分 类 号 TN21 文 献 标 识 码 A
相变调温墙板热工性能试验和数值模拟研究
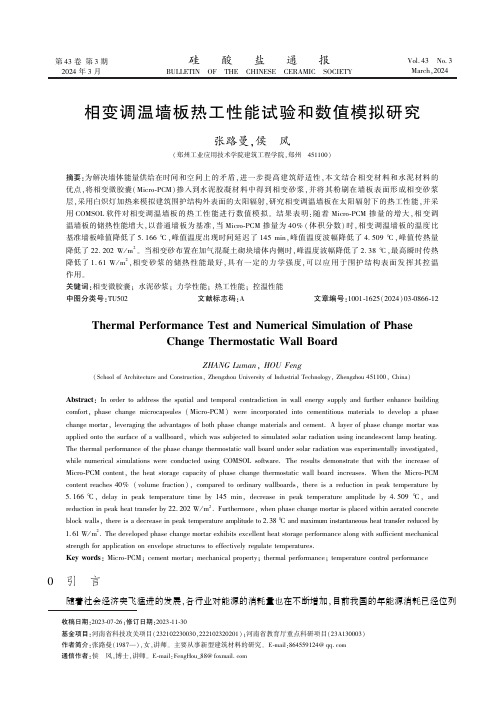
第43卷第3期2024年3月硅㊀酸㊀盐㊀通㊀报BULLETIN OF THE CHINESE CERAMIC SOCIETY Vol.43㊀No.3March,2024相变调温墙板热工性能试验和数值模拟研究张路曼,侯㊀风(郑州工业应用技术学院建筑工程学院,郑州㊀451100)摘要:为解决墙体能量供给在时间和空间上的矛盾,进一步提高建筑舒适性,本文结合相变材料和水泥材料的优点,将相变微胶囊(Micro-PCM)掺入到水泥胶凝材料中得到相变砂浆,并将其粉刷在墙板表面形成相变砂浆层,采用白炽灯加热来模拟建筑围护结构外表面的太阳辐射,研究相变调温墙板在太阳辐射下的热工性能,并采用COMSOL 软件对相变调温墙板的热工性能进行数值模拟㊂结果表明:随着Micro-PCM 掺量的增大,相变调温墙板的储热性能增大,以普通墙板为基准,当Micro-PCM 掺量为40%(体积分数)时,相变调温墙板的温度比基准墙板峰值降低了5.166ħ,峰值温度出现时间延迟了145min,峰值温度波幅降低了4.509ħ,峰值传热量降低了22.202W /m 2㊂当相变砂布置在加气混凝土砌块墙体内侧时,峰温度波幅降低了2.38ħ,最高瞬时传热降低了1.61W /m 2,相变砂浆的储热性能最好,具有一定的力学强度,可以应用于围护结构表面发挥其控温作用㊂关键词:相变微胶囊;水泥砂浆;力学性能;热工性能;控温性能中图分类号:TU502㊀㊀文献标志码:A ㊀㊀文章编号:1001-1625(2024)03-0866-12Thermal Performance Test and Numerical Simulation of Phase Change Thermostatic Wall BoardZHANG Luman ,HOU Feng(School of Architecture and Construction,Zhengzhou University of Industrial Technology,Zhengzhou 451100,China)Abstract :In order to address the spatial and temporal contradiction in wall energy supply and further enhance building comfort,phase change microcapsules (Micro-PCM)were incorporated into cementitious materials to develop a phase change mortar,leveraging the advantages of both phase change materials and cement.A layer of phase change mortar was applied onto the surface of a wallboard,which was subjected to simulated solar radiation using incandescent lamp heating.The thermal performance of the phase change thermostatic wall board under solar radiation was experimentally investigated,while numerical simulations were conducted using COMSOL software.The results demonstrate that with the increase of Micro-PCM content,the heat storage capacity of phase change thermostatic wall board increases.When the Micro-PCM content reaches 40%(volume fraction),compared to ordinary wallboards,there is a reduction in peak temperature by 5.166ħ,delay in peak temperature time by 145min,decrease in peak temperature amplitude by 4.509ħ,and reduction in peak heat transfer by 22.202W /m 2.Furthermore,when phase change mortar is placed within aerated concrete block walls,there is a decrease in peak temperature amplitude to 2.38ħand maximum instantaneous heat transfer reduced by1.61W/m2.The developed phase change mortar exhibits excellent heat storage performance along with sufficient mechanical strength for application on envelope structures to effectively regulate temperatures.Key words :Micro-PCM;cement mortar;mechanical property;thermal performance;temperature control performance 收稿日期:2023-07-26;修订日期:2023-11-30基金项目:河南省科技攻关项目(232102230030,222102320201);河南省教育厅重点科研项目(23A130003)作者简介:张路曼(1987 ),女,讲师㊂主要从事新型建筑材料的研究㊂E-mail:864559124@通信作者:侯㊀风,博士,讲师㊂E-mail:FengHou_88@0㊀引㊀言随着社会经济突飞猛进的发展,各行业对能源的消耗量也在不断增加,目前我国的年能源消耗已经位列第3期张路曼等:相变调温墙板热工性能试验和数值模拟研究867㊀世界第二[1],减少能源消耗和碳排放量已成为世界各国可持续发展的目标㊂我国能源消耗的三大主要领域分别是建筑业㊁工业和交通运输业,其中,建筑业的能耗约占全社会总能耗的33%,二氧化碳排放占全国总体碳排放的25%以上,建筑运行阶段中的能耗更是占建筑业能耗的70%以上[1-2]㊂当前,采暖㊁通风和空调系统在内的空气调节系统(heating,ventilation,air-conditioning and cooling,HVAC)是构成建筑运行阶段能耗的重要组成部分,而这些设备的使用频率与建筑围护结构的材料㊁设计和构造密不可分,通过改善建筑围护结构的保温隔热性能可有效降低HVAC 系统的运行能耗㊂然而,目前传统的建筑材料(混凝土㊁砖㊁砂子等)和保温隔热材料均为显热储能,其蓄热性能和节能减排效果差[3]㊂因此,研究和开发新型建筑节能材料对实现我国的 双碳 目标具有重要的理论和现实意义[4-5]㊂图1㊀相变调温墙板调节室内温度的原理示意图Fig.1㊀Schematic diagram of the principle of phase change thermostatic wall board to adjust indoor temperature 利用相变储能技术降低建筑能耗是建筑节能研究的热点之一,通过利用相变材料具有单位体积蓄热量大和吸㊁放热过程几乎恒温这两个突出优势,达到储存能量和提高能源利用效率的目的[6]㊂使用相变材料作为建筑围护结构中的储能媒介能显著增加围护结构的热惯性,从而削弱热循环的振幅,防止建筑内部温度的过度突变[7]㊂图1为相变调温墙板调节室内温度的原理示意图,相变材料根据外界环境温度的变化而发生融化吸热和凝固放热的交替过程,这使得相变调温墙板具有使室内温度稳定在人体舒适温度范围的能力[8]㊂因此,相变材料在建筑节能领域具有良好的应用前景㊂目前,各国学者已针对相变储能建筑材料开展了广泛研究㊂早期研究者直接将相变材料添加到建筑材料中,但试验过程中发现固-液相变材料在相变时会引起三个不容忽视的问题:1)渗漏;2)相变材料与建筑材料基体相互作用;3)降低传热效率㊂为了克服上述问题,研究人员开发出定形相变材料,包括定形相变骨料㊁相变宏胶囊㊁相变微胶囊(micro-encapsulation phase change materials,Micro-PCM)㊂其中,Micro-PCM 是一种由性能稳定的高分子膜(壳材)包裹固-液相变材料(芯材)而成的新型复合相变材料㊂研究人员已利用Micro-PCM 开发出不同种类的储能建筑材料,例如Bassim 等[1]将Micro-PCM 掺入到水泥砂浆中,并采用陶瓷集料(ceramic fine aggregate,CFA)替代复合材料中的砂子,当Micro-PCM 掺量为50%(质量分数,下同)㊁陶瓷集料替代砂子量为100%时,与普通水泥砂浆相比,复合材料温度降低了9.5ħ㊂Ren 等[9]将相变微胶囊掺入到超高性能混凝土(ultra-high performance concrete,UHPC)中,研制出了一种具有优异储热性能的新型结构-功能一体化混凝土(MPCM-UHPC),通过热工测试可知,掺入10%相变微胶囊的UHPC 表面温度比基准组降低了3.9ħ㊂Park 等[10]采用普通墙板和含Micro-PCM 的墙板分别搭建了同一尺寸(2400mm ˑ2700mm ˑ2300mm)的测试房,通过传热试验可知,相变墙板室内温度比基准组降低了1~2ħ,供暖能耗降低了27.7%㊂综上分析,将Micro-PCM 引入建筑材料中可起到储存热量㊁保温隔热㊁提高墙体热惰性㊁降低室内温度波动的作用㊂然而,外来颗粒的引入会改变建筑材料的微观结构,从而影响建筑材料的力学性能㊂例如,Djamai 等[11]对掺入5%㊁10%㊁15%Micro-PCM 的水泥砂浆进行了力学试验,结果表明掺入20%的Micro-PCM 使复合材料的强度降低了70.5%㊂Yu 等[12]将Micro-PCM 与水泥砂浆相结合,开发出了一种具有热能储存功能的相变砂浆,结果表明掺入20%Micro-PCM 的相变砂浆28d 抗压强度和抗折强度分别为36.5和6.2MPa,与普通水泥砂浆相比,力学强度略有降低㊂Rahul 等[3]使用Micro-PCM 替代高流动性水泥砂浆中的细集料,研究了相变砂浆的力学性能,结果表明掺入5%和10%Micro-PCM 的相变砂浆抗压强度分别降低了15%和54%㊂为了克服Micro-PCM 导致建筑材料力学强度下降的问题,将Micro-PCM 与水泥砂浆混合涂敷于建筑围护结构,既可以避免结构材料强度损失,又改善围护结构的热工性能㊂另外,国内外学者研究Micro-PCM 在建筑领域的作用时,大多考虑将Micro-PCM 在基体中均匀分布或整齐排列制成相变层,未考虑Micro-PCM 在基体结构中的无规则排列㊂鉴于此,本文将Micro-PCM 随机分布在868㊀水泥混凝土硅酸盐通报㊀㊀㊀㊀㊀㊀第43卷水泥砂浆中,制备了相变砂浆,并对其热学性能进行研究㊂利用MATLAB 软件的随机分布程序和COMSOL 有限元软件构建了Micro-PCM 随机分布的传热模型,模拟分析了相变调温墙板的蓄热性能㊂通过模拟分析相变调温墙板在传热过程中墙体内表面温度随时间变化的规律,对比传热过程中普通墙板和相变调温墙板内表面温度波动情况,得出相变调温墙板中Micro-PCM 的掺量与墙体内/外壁面温度波幅㊁热流密度㊁温度延迟之间的关系㊂1㊀实㊀验1.1㊀原材料图2㊀Micro-PCM 的DSC 曲线Fig.2㊀DSC curves of Micro-PCM 采用安徽美科迪智能微胶囊科技有限公司生产的Micro-PCM 作为水泥砂浆的添加剂,得到一种微胶囊化的被动式热调节相变砂浆并涂敷于墙板上,微胶囊法利用成膜物质将相变材料包覆其中,形成微小的核-壳结构,芯材是具有潜热储能的石蜡,在相变过程中能够保持特定的温度㊂每粒胶囊中PCM 含量约占总质量的85%~90%㊂Micro-PCM 壁由一种稳定的惰性聚合物构成,粒径一般在5~1000μm㊂相变温度为26.44ħ,相变潜热为175.39J /g,具体如见图2所示㊂Micro-PCM 的外貌形态如图3所示,表面有许多的折皱,这是由Micro-PCM 中相变材料的相态变化导致体积变化所引起的[13-14]㊂图3㊀Micro-PCM 的SEM 照片Fig.3㊀SEM images of Micro-PCM 1.2㊀相变砂浆的制备及储热特性试验为了不影响墙板的力学性能,将相变砂浆以抹灰形式涂覆于墙板外侧㊂原料选用P㊃O 32.5普通硅酸盐水泥㊁ISO 标准砂㊁自来水㊁Micro-PCM㊂试验配合比参照‘砌筑砂浆配合比设计规程“(JGJT 98 2010)中的比例要求,以及结合以往相关经验,配合比设计如表1所示,该配合比是在恒定的水灰比和恒定的细骨料与水泥比(W /C =0.6和FA /C =6.95)下制备,并将Micro-PCM 置换为7.5%的标准砂㊂为了防止Micro-PCM 破碎,将Micro-PCM 在最后一步中添加㊂先将水泥㊁水置于搅拌桶中,启动搅拌机,拌合程序为:低速30s ң加砂30s ң高速30s ң停90s ң加Micro-PCM 及减水剂高速60s,最后调整高效减水剂(水泥用量的0%~1.1%)的用量,使得相变砂浆具有理想的流动性(稠度值为70mm),并易于涂敷于墙板(尺寸为300mm ˑ300mm ˑ100mm)表面,相变砂浆涂敷厚度为20mm,试件制备过程如图4所示㊂最后,将制备好的相变砂浆涂敷于墙板后置于温度为(20ʃ2)ħ㊁湿度大于95%的养护箱里面进行养护,养护7d,进行下一步的相变储能测试㊂第3期张路曼等:相变调温墙板热工性能试验和数值模拟研究869㊀表1㊀相变砂浆配合比Table1㊀Mix ratio of phase change mortarMicro-PCM volume fraction/%Mix ratio/(kg㊃m-3)Cement Water Standard sand Micro-PCM Superolasticizer(SP)/%Cement consistency/nm0230.0138.00160000~1.17017.8212.9127.7414801200~1.170图4㊀相变砂浆制备过程Fig.4㊀Preparation process of phase change mortar在数值计算中,通常采用体积分数,采用下式将质量分数转换为体积分数:f Micro-PCM=w Micro-PCMρMicro-PCM/ρcement+w Micro-PCM(1-ρMicro-PCM/ρcement)(1)式中:f Micro-PCM㊁w Micro-PCM分别为Micro-PCM的体积分数㊁质量分数;ρMicro-PCM㊁ρcement分别为Micro-PCM的密度和水泥砂浆的密度,其中ρMicro-PCM=694kg/m3㊂1.3㊀储热性能测试为了测试相变调温墙板的控温性能,课题组制备的控温测试仓尺寸为1600mmˑ380mmˑ380mm,在测试仓的顶部固定100W的白炽灯作为辐射传热的热源,且为了减少测试仓与外界环境的热交换,在测试仓的内壁上粘贴2层40mm厚聚氨酯泡沫保温板,保温板表面粘贴网格线加强的锡箔纸㊂采集设备采用Captee Enterprise HFM-8热流密度采集仪和HS-30热流密度计,热流感知面尺寸为30mmˑ30mm,内置T型热电偶㊂为了能够准确监测相变调温墙板上㊁下面温度及表面热流,在相变调温墙板的上㊁下表面两个区域分别布置HS-30热流密度计,上表面布置一个HS-30热流密度计,下表面布置两个HS-30热流密度计,下表面取两个测点测试结果的平均值作为热流和温度测量值㊂HS-30热流密度计测试点位示意图如图5所示㊂图5㊀HS-30热流密度计测试点位示意图(单位:mm)Fig.5㊀Schematic diagram of experiment points of HS-30heat flux density meter(unit:mm)测试前,将试件置于制冷温度为8ħ的冰柜中24h,目的是将相变调温墙板的初始温度稳定在8ħ㊂测试时,将HS-30热流密度计安装在测试点,相变调温墙板的实测温度为10ħ,将该实测温度作为验证数值模型的初始温度;另外,设定HFM-8热流采集设备每1min收集一次温度值和热流值,启动电脑中的Launch870㊀水泥混凝土硅酸盐通报㊀㊀㊀㊀㊀㊀第43卷HFM8-lab软件,建立Launch HFM8-lab软件与HFM-8热流采集设备之间的通信,且设定采集设备同步储存测试数据㊂打开白炽灯开关,使白炽灯以辐射方式持续提供热量,同时,启动设备开始采集数值㊂两个相变调温墙板的测试时间均为624min㊂2㊀相变墙体模拟研究2.1㊀几何模型本文通过PFC软件中的Rand函数建立Micro-PCM随机分布模型,如图6所示㊂模型按照以下算法生成:1)输入Micro-PCM的数量㊁Micro-PCM的半径和基体材料的尺寸;2)使用RAND函数随机生成第一个Micro-PCM的坐标值;3)使用RAND函数随机生成Micro-PCM第i个位置,并判断第i个Micro-PCM是否会与已经生成的i-1个Micro-PCM相交,如果不相交,则生成Micro-PCM模型,反之则重新生成;4)重复上述过程,直至生成所指定数量的Micro-PCM㊂图6㊀Micro-PCM在水泥砂浆中的随机分布模型Fig.6㊀Random distribution model of Micro-PCM in cement mortar2.2㊀物理模型以试验测试中的试件尺寸构建数值模型,数值模型中的相变调温墙板厚度为100mm㊁高度为300m,相变砂浆的厚度为20mm,墙板㊁水泥砂浆㊁Micro-PCM的热物性参数如表2所示,Micro-PCM的相变温度T m为26.44ħ,假定相变发生在较小的温度区间内[T s,T l](下标l㊁s分别代表固相和液相),其中,T s=T m-ΔT m/2, T l=T m+ΔT m/2,ΔT m=1K㊂相变调温墙板左侧为室内环境,右侧为室外环境,上/下表面为绝热㊂表2㊀材料热物性参数Table1㊀Thermophysical properties of materialsMaterial Density/(kg㊃m-3)Specific heat/[J㊃(kg㊃K)-1]Thermal conductivity/[W㊃(m㊃K)-1]Latent heat/(J㊃g-1) Cement paste180010500.90Micro-PCM88032200.21175.39 Aerated concrete block70011500.242.3㊀数学模型为了简化计算,对所研究的问题作如下假设:1)Micro-PCM在水泥砂浆中随机分布,忽略相变过程中体积的变化;第3期张路曼等:相变调温墙板热工性能试验和数值模拟研究871㊀2)水泥墙板和调温砂浆各向同性㊁常物性;3)由于Micro-PCM 粒径较小,忽略自然对流的影响;4)墙体的厚度远小于宽度和高度,温度只沿厚度方向变化,传热过程为一维导热过程㊂2.4㊀控制方程本文采用等效热容法对融化和凝固相变过程进行理论建模,其控制方程如式(2)所示㊂ρC P ∂T ∂t =k Δ2T (2)式中:ρ为密度,C P 为比热容,T 为温度,k 为热传导系数,Δ为微分算子㊂在进行相变分析时,为了保证计算的稳定性,假定相变发生在一个很小的温度区间内[T s ,T l ],该温度区间ΔT m =T l -T s =1K,T s =T m -ΔT m /2,T l =T m +ΔT m /2㊂当T <T s 时为固相,T <T l 时为液相,T s <T <T l 为混合相,则各相与液体分数f 的关系如式(3)所示㊂f =0T <T s T -T s T l -T s T s ɤT ɤT l 1T >T l ìîíïïïï(3)式中:T s 为固相温度上限,T l 为液相温度下限㊂根据液相体积分数的定义,等效热容C P 计算式如式(4)所示㊂C p =1ρ[(1-f )ρs C p,s +f ρl C p,l +L m D (T )](4)式中:C P,s 为固相Micro-PCM 的比热容,C P,l 为液相Micro-PCM 的比热容,D (T )为相变区间ΔT m 内的标准高斯函数,该函数在相变区间内的积分为1㊂2.5㊀定解条件本试验中只考虑温度的变化,从而提出水泥墙板传热的数学模型的边界条件,如式(5)所示㊂q =q 0x =0(5)式中:q 0为Micro-PCM 体积分数为0%的试件上表面试验测试值㊂补充初始条件如式(6)所示㊂T 2=T 1=T 0<T m t =0(6)2.6㊀相变墙体有限元模型采用大型通用有限元软件COMSOL,建立有限元模型,见图7㊂在热传导的有限元模拟中,采用极精细的三角形网格来获得与网格无关的收敛解㊂在仿真中,计算时间步长设置为1s,使用COMSOL 中的PARDISO 直接求解器来求解高度非线性的多物理场耦合问题㊂图7㊀相变调温墙板和普通水泥墙板的有限元模型Fig.7㊀Finite element models phase change thermostatic wall board and ordinary wall board872㊀水泥混凝土硅酸盐通报㊀㊀㊀㊀㊀㊀第43卷3㊀结果与讨论3.1㊀验证模型本研究采用相对误差分析方法RME 进行结果验证,采用数值模拟值与试验测试值的相对误差值来表征两种研究方法之间的结果差别㊂RME 为相对误差的最大值,文献[15]中的定义如式(7)所示㊂RME =Max x sim -x exmx exm ()ˑ100%[](7)RAE 为相对误差的平均值,计算式如(8)所示㊂RAE =Average x sim -x exm x exm ()ˑ100%[](8)图8㊀数值模拟结果与试验结果对比Fig.8㊀Comparison between numerical simulation and experimental results 式中:x sim 为数值模拟结果,x exm 为试验测试值㊂以上述相变调温墙板的储热试验环境作为边界条件对相变调温墙板进行模拟计算,并将试验结果与模拟计算结果进行对比,验证试验结果与数值模拟结果的一致性㊂图8为数值模拟结果与试验结果对比(%为体积分数)㊂由图8可知,数值模拟结果与试验结果曲线变化趋势是一致的,当温度升至相变材料的融化温度后,数值计算结果比试验结果偏快㊂另外,相变调温墙板内表面温度最大误差率为2.24%,平均误差为1.89%,最大误差率和平均误差率均没有超过3%,说明在数值模拟过程中建立的计算模型㊁选用的材料参数和试验一致,数值计算结果和试验结果具有一致性㊂3.2㊀瞬态气象条件下的相变调温墙板动态隔热性能分析外界温度以夏热冬冷地区(河南省郑州市)典型气象数据作为依据,对相变水泥墙板的动态隔热进行分析,选取郑州市最热月7月25~27日的温度和太阳辐射强度作为边界条件,以西向墙体为例,拟合得到室外空气综合温度表达式,如式(9)~(11)所示㊂t 25(τ)=33.0+8.0sin(π(τ-8.28)/9.96)+273.15(9)t 26(τ)=34.5+9.2sin(π(τ-8.12)/11.7)+273.15(10)t 27(τ)=35.0+9.0sin(π(τ-9.32)/11.6)+273.15(11)基于上述物理模型和数学模型,利用COMSOL 软件模拟计算普通水泥墙板和相变调温墙板的传热过程,8~65h 时刻的温度云图如图9所示,在相同室外温度边界条件下,普通墙板的热量传递过程比相变调温墙板快,相变调温墙板均将高温区阻挡在墙体外部区域,表明相变材料提升了墙体的热惰性㊂根据相变调温墙板相变过程的分阶段传热式(9)~(11)可得到在室外瞬态气象条件下相变调温墙板内侧温度和液体分数瞬态分布曲线,如图10所示㊂从图10中液体分数曲线及相变调温墙板内侧温度曲线的变化情况可看出,当普通墙体外表面涂敷一定厚度的相变调温砂浆后,其内表面温度较普通水泥墙板均有所下降,具体分析如下:1)0~10h,表示Micro-PCM 外表面温度低于相变温度,相变材料为固态,相变调温墙板的传热过程同普通水泥墙板,但温度较普通水泥墙板低;2)10~19h,表示Micro-PCM 外表面温度高于相变温度,相变材料开始由固态转变为液态,同时以潜热的形式不断吸收热量,且固-液界面处温度维持在相变温度不变;3)19~21h,表示固-液相变结束后,相变材料处于液态,在外界温度作用下继续传热,传热过程同普通水泥墙板,但温度较普通水泥墙板低;第3期张路曼等:相变调温墙板热工性能试验和数值模拟研究873㊀图9㊀相变调温墙板和普通水泥墙板的计算温度云图(Micro-PCM,40%,体积分数)Fig.9㊀Cloud diagrams of the calculated temperature of phase change thermostatic wall board and ordinary wall board (Micro-PCM,40%,volumefraction)图10㊀相变调温墙板内侧温度和Micro-PCM 的液体分数变化(Micro-PCM,40%,体积分数)Fig.10㊀Temperature change inside the Micro-PCM-W and liquid fraction change inside phase change thermostatic wall board (Micro-PCM,40%,volume fraction)4)21~31h,表示Micro-PCM 外表面温度开始低于相变温度,相变材料开始由液态转变为固态,同时以潜热的形式不断释放热量,且固-液界面处温度维持在相变温度不变;5)31~38h,表示Micro-PCM 外表面温度高于相变温度,部分固相相变材料开始融化,同时以潜热的形式不断吸收热量,且固-液界面处温度维持在相变温度不变㊂3.3㊀Micro-PCM 掺量对相变调温墙板动态隔热性能的影响㊀㊀墙板内壁面温度决定了室内热环境,内壁温度随室外空气环境温度的变化关系如图11所示㊂由图11可知,室外环境温度以24h 为一个周期,72h 内出现3个周期波动,九类墙板内壁面温度也出现了3个波动周期,由于墙板具有热惰性,九类墙板内壁面温度波动均延迟外界环境温度的变化,但相变调温墙板延迟性较普通墙体高,且Micro-PCM 掺量越高延迟性越高㊂例如:在第一个温度波动周期内,普通水泥墙板约在16.96h 达到最高温度32.975ħ,而Micro-PCM 掺量为40%(体积分数)的相变水泥墙板在19.39h 达到最高温度27.809ħ,滞后时间为145min,温度降低量为5.166ħ,相变调温墙板的温度峰值滞后时间和温度降低量均优于普通水泥墙板,且Micro-PCM 含量越高,墙体的温度峰值滞后时间和温度降低量越大㊂874㊀水泥混凝土硅酸盐通报㊀㊀㊀㊀㊀㊀第43卷图11㊀不同Micro-PCM 含量下的相变调温墙板内侧温度变化Fig.11㊀The inner wall temperature changes of Micro-PCM control wallboard under different Micro-PCM content第二周期内,普通水泥墙板内壁面温度波动幅度为12.327ħ,Micro-PCM 的含量为5%~40%时,相变调温墙板内壁面温度波动幅度为7.818~11.395ħ,较普通墙体降低量为0.248~1.910ħ,即Micro-PCM 含量越高,相变调温墙板的室内侧温度波动越小,即相变调温墙板会提供稳定的室内热环境㊂相变调温墙板中第一层材料(即相变砂浆层)内侧温度如图12所示,在三个周期的升温过程中,第一层材料内测温度达到约26ħ时,相变调温墙板的温升速率开始低于普通水泥墙板,且Micro-PCM 含量越高,升温速率越低㊂这是由于室外空气将热量向墙板内侧传递时,墙板升温至约26ħ时,相变材料开始发生相变,热量以潜热的形式储存,减少了向墙板内侧区域传递的热量;在第一个周期内,体积含量为40%的相变水泥墙板内侧温度较普通墙体降低了5.565ħ,表明Micro-PCM 的潜热储能作用确实降低了墙板内部区域的温升㊂在三个周期内的降温过程中,普通水泥墙板的起始温度高于相变调温墙板,但其降温速率也高,这是由于Micro-PCM 以潜热的形式储存的热量在降温时以内热源的形式释放到外部环境中㊂不同Micro-PCM 含量下的相变调温墙板内侧表面的热流密度变化如图13所示㊂在3个温度波动周期内,相变调温墙板由于相变材料在相变过程中以潜热的形式存储了一部分能量,降低了向室内的传热量㊂在第一个温度波动周期内,Micro-PCM 体积分数为5%~40%的相变调温墙板最高瞬时传热量比普通水泥墙板分别降低了8.067㊁12.006㊁13.726㊁16.913㊁19.270㊁19.793㊁20.901㊁22.202W /m 2,表明相变调温墙板向室内传递的热量更少㊂图12㊀不同Micro-PCM 含量下相变砂浆层内侧的温度变化Fig.12㊀Temperature changes in the inner side of phase change mortar layer under different Micro-PCM content㊀图13㊀不同Micro-PCM 含量下的相变调温墙板内侧表面的热流密度变化Fig.13㊀Heat flux changes on the inside of phase change thermostatic wall board with different Micro-PCM content 综上可知,从墙体内部温度分布㊁第一层材料内侧温度和向室内传递的热负荷来看,相变调温墙板控温性能优于普通水泥墙板㊂3.4㊀相变砂浆位置对加气混凝土墙体控温性能的影响为了研究墙体围护结构最内层表面的温度受到室外空气的对流换热和太阳辐射的综合影响,参照工程结构中加气混凝土砌块墙体的组合形式,构建符合工程实际的相变调温墙体传热模型,具体如图14所示㊂另外,本文选取郑州的气象环境作为模拟参数,根据2022年7月份的气象数据报表中的统计数据为基础,以西向墙体为例,得出郑州7月份一周的室外空气综合温度分布曲线,作为相变调温墙板室外侧边界条件施加于模型上进行传热分析㊂第3期张路曼等:相变调温墙板热工性能试验和数值模拟研究875㊀图14㊀参照实际工况的相变墙体传热模型Fig.14㊀Heat transfer model of phase-change wall according to actual working conditions 为对比相变砂浆置于墙体内侧及外侧的隔热效果,取20mm 厚的相变砂浆层(40%的Micro-PCM)分别置于加气混凝土砌块墙体内侧(2型)和外侧(1型),计算得到典型墙体内表面温度和热流密度变化曲线如图15所示㊂由图15可知,郑州夏季7月份相变砂浆置于加气混凝土砌块墙内侧时隔热效果最好,相变砂浆置于墙体外侧时隔热效果较差㊂在第6天,1型和2型加气混凝土砌块墙体内侧温度降低量分别为0.21和2.38ħ,最高瞬时传热量分别降低了0.12和1.61W /m 2,由此可知,相变砂浆置于墙体内侧时,墙体的热工性能较理想㊂热量在由外墙传递至相变区的过程中能量会发生衰减损耗,导致温度到达相变区时会有所降低,温差的减小使得Micro-PCM 融化吸热的速率降低,室内温度波动和传入室内的热流密度减小,从而达到建筑节能的效果㊂图15㊀加气混凝土砌块相变调温墙板内侧表面温度和热流密度变化Fig.15㊀Temperature and heat flux changes of the inner surface of aerated concrete block phase change thermostatic wall board 3.5㊀Micro-PCM 掺量对相变砂浆力学性能的影响利用抗压抗折力学测试仪对相变砂浆试块进行了力学强度测试,每种配方的相变砂浆试块测试3次抗折强度㊁3次抗压强度,取平均值作为最终的结果㊂图16的(a)和(b)分别展示了不同体积分数的Micro-PCM 对相变砂浆抗压强度和抗折强度的影响,相变砂浆的抗压强度和抗折强度均随着Micro-PCM 掺量的增㊀㊀㊀图16㊀不同体积分数的Micro-PCM 对相变砂浆抗压强度和抗折强度的影响Fig.16㊀Effect of different volume fractions of Micro-PCM on compressive strength and flexural strength of phase change mortar。
室内颗粒物的稳态置换流净化机制

大多数人将近 90%的时间是在室内[1],从人 体污染物暴露的剂量上看,室内污染对人体健康 的影响更为严重,因此,考察室内污染物的源与汇 及迁移转化显得尤为重要.
文献标识码:A
文章编号:1000-6923(2018)01-0097-06
The mechanism of low-speed steady substitution flow to clean the indoor particulate matter. LIN Guan-ming1*, REN Zhen-hai2, SONG Jian-li3 (1.State Joint Key Laboratory of Environmental Simulation and Pollution Control, College of Environmental Science and Engineering, Peking University, Beijing 100871, China;2.Chinese Research Academy of Environmental Sciences, Beijing 100012, China ; 3.Shijiazhuang Aoxiang Pharmaceutical Engineering Co., Ltd., Shijiazhuang 050031, China). China Environmental Science, 2018,38(1):97~102 Abstract:The mechanism of the indoor particulate matter’s transportation and diffusion was discussed. Using the spatial and temporal scale analysis, the particulate matter’s flux can be artificially separated into four parts: the advection and slippage in the slowly varying macro mean motion δ-scale, the turbulent diffusion in the rapidly varying micro turbulence motion η-scale and the Brown diffusion in the dramatically varying molecular motion λ-scale. Correspondingly, the flux equations in the ensemble average format and model computing format were concluded. Following the equations, the methods to increase the purification efficiency were lowering the indoor air speed and its turbulence intensity gradient. The flow fields was numerically simulated in a typical room with the low-speed steady substitution flow system installed and in a normal room with both air inlet and outlet on the roof. The particulate matter’s number concentration was measured in two rooms with different air inlet and outlet positions. The results showed that the ways to improve the purification efficiency and to decrease the energy cost were: 1) keeping a weak positive pressure; 2) setting the ceiling corner line air inlet and baseboard corner line air outlet layout; 3) lowering the air speed and turbulence gradient. Key words:steady substitution flow;indoor particulate matter;flux;purification efficiency
室外自然场景下的雾天模拟生成算法
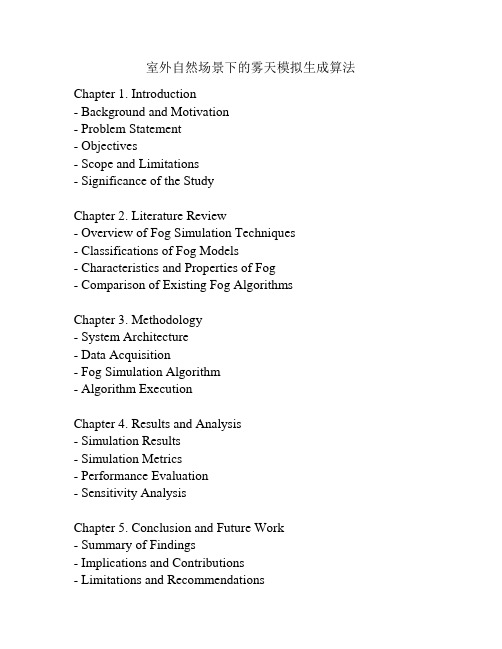
室外自然场景下的雾天模拟生成算法Chapter 1. Introduction- Background and Motivation- Problem Statement- Objectives- Scope and Limitations- Significance of the StudyChapter 2. Literature Review- Overview of Fog Simulation Techniques- Classifications of Fog Models- Characteristics and Properties of Fog- Comparison of Existing Fog AlgorithmsChapter 3. Methodology- System Architecture- Data Acquisition- Fog Simulation Algorithm- Algorithm ExecutionChapter 4. Results and Analysis- Simulation Results- Simulation Metrics- Performance Evaluation- Sensitivity AnalysisChapter 5. Conclusion and Future Work- Summary of Findings- Implications and Contributions- Limitations and Recommendations- Future Research Directions- ConclusionReferencesChapter 1. IntroductionBackground and MotivationThe phenomenon of fog is commonplace in many natural outdoor scenes, but it can significantly affect visibility and safety in transportation, navigation, and surveillance systems. Fog is formed when the air temperature reaches dew point, causing the water droplets to condense into small particles in the atmosphere. The particles scatter light and absorb specific wavelengths, which decreases the contrast and color saturation of the scene. Capturing foggy scenes and simulating them in computer graphics and vision systems has become an active research area in recent years due to the increasing demand for realistic and robust fog simulation algorithms.Problem StatementExisting fog models and generation algorithms have several limitations, such as being computationally expensive, requiring large datasets, and not accurately representing the complex dynamics of atmospheric conditions. Therefore, there is a need for a comprehensive and efficient fog simulation algorithm that performs well in different outdoor scenarios and can generate realistic foggy images.ObjectivesThe primary objective of this study is to develop a novel algorithm to simulate fog in natural outdoor scenes. The algorithm shouldprovide realistic and visually pleasing results, be computationally efficient, and adapt to different weather conditions and lighting conditions. The secondary objectives are to compare the proposed algorithm with existing techniques and evaluate its performance and robustness in various simulated scenarios.Scope and LimitationsThis study focuses on simulating fog in natural outdoor scenes, including forests, mountains, and cities, but not in indoor or laboratory environments. The proposed algorithm is designed to work with RGB images and does not consider other modalities, such as infrared or stereo data. The study aims to provide a proofof concept and does not optimize the algorithm for real-time applications.Significance of the StudyThe proposed fog simulation algorithm can have practical applications in several domains, such as autonomous driving, visual effects, and virtual reality. By synthesizing realistic foggy images, the algorithm can improve the performance and reliability of computer vision and machine learning systems operating in outdoor environments. Furthermore, the proposed algorithm can aid in understanding and studying the complex atmospheric phenomena of fog and its impact on visual perception.In conclusion, this chapter introduces the problem of fog simulation in natural outdoor scenes and the motivation for developing a novel fog simulation algorithm. The objectives, scope, and limitations of the study are defined, and the significance of the proposed algorithm is highlighted. The next chapter will review theexisting literature on fog simulation techniques in moredetail.Chapter 2. Literature ReviewIntroductionIn recent years, fog simulation has received significant attention from the computer graphics, vision, and machine learning research communities. Several techniques have been proposed to simulate fog and haze effects in outdoor scenes, based on various physical and statistical models. This chapter reviews the existing literature on fog simulation techniques and analyzes their strengths and weaknesses.Physical ModelsPhysical models aim to simulate the scattering and absorption of light in the atmosphere, based on the laws of physics and optics. Radiative transfer equations (RTE) are commonly used to describe the light transport in the atmosphere, but they are computationally expensive and require complex boundary conditions. Approximate methods, such as the Monte Carlo method and the discrete ordinates method, have been proposed to solve RTE efficiently. However, these methods still suffer from practical limitations, such as parameterization and calibration.Statistical ModelsStatistical models approximate the appearance of foggy scenes based on empirical observations and statistical analysis. One of the earliest and most widely used statistical models for fog simulation is the Koschmieder model, which assumes uniform fog density and exponential attenuation of light with distance. However, this model is simplistic and does not account for spatial and temporalvariations in fog density and atmospheric conditions.Recently, machine learning techniques, such as deep neural networks, have been employed to learn the mapping between clear and foggy images, bypassing the need for explicit models. These techniques have shown promising results in generating realistic foggy scenes, but they require large amounts of training data and may not generalize well to unseen environments or lighting conditions.Evaluation MetricsEvaluating the quality and realism of fog simulation algorithms is challenging, as there is no objective ground truth for comparing the generated foggy images with real-world data. Therefore, several metrics have been proposed to measure different aspects of fog simulation performance, such as color preservation, contrast enhancement, and visibility improvement. These metrics include the atmospheric scattering model, the color distribution distance, and the visibility index. However, these metrics have their own limitations and may not capture all aspects of fog simulation performance.ConclusionIn conclusion, this chapter reviewed the existing literature on fog simulation techniques, including physical and statistical models and machine learning approaches. The strengths and weaknesses of these techniques were discussed, and evaluation metrics for fog simulation were introduced. The next chapter will present the proposed fog simulation algorithm, which combines physical and statistical models and uses machine learning forrefinement.Chapter 3. Proposed Fog Simulation Algorithm IntroductionIn this chapter, we propose a novel fog simulation algorithm that combines physical and statistical models and uses machine learning for refinement. The algorithm consists of three stages: 1) physical model-based fog density estimation, 2) statistical model-based image synthesis, and 3) machine learning-based refinement. Each stage will be described in detail below.Physical Model-Based Fog Density EstimationThe first stage of the proposed algorithm aims to estimate the fog density in the scene, based on physical models of light scattering and absorption in the atmosphere. We use the radiative transfer equation (RTE) to model the light transport in the atmosphere, and solve it using the discrete ordinates method with predefined boundary conditions. The inputs to this stage are the clear image and the atmospheric parameters, such as the air temperature, pressure, and humidity. The output is the depth-dependent fog density, which is used as input to the next stage.Statistical Model-Based Image SynthesisThe second stage of the proposed algorithm aims to synthesize a foggy image based on statistical models of fog appearance and empirical observations. We use a modified version of the Koschmieder model, which takes into account spatial and temporal variations in fog density and atmospheric conditions. The inputs to this stage are the clear image, the fog density estimated in the previous stage, and the atmospheric parameters. The outputs are the synthesized foggy image and a set of statistical parameters thatdescribe its appearance, such as the color distribution and contrast. Machine Learning-Based RefinementThe third stage of the proposed algorithm aims to refine the synthesized foggy image and improve its visual quality, using machine learning techniques. We use a deep neural network to learn the mapping between clear and foggy images, and use it to refine the synthesized foggy image. The training data for the neural network consists of pairs of clear and foggy images, which are generated using the physical and statistical models described above. The inputs to this stage are the synthesized foggy image and the statistical parameters, and the output is the refined foggy image. EvaluationWe evaluate the proposed algorithm using several metrics, including the atmospheric scattering model, the color distribution distance, and the visibility index. We compare the results of our algorithm with those of existing fog simulation techniques, including physical models, statistical models, and machine learning approaches. We also conduct a user study to assess the subjective quality of the generated foggy images.ConclusionIn conclusion, this chapter presented the proposed fog simulation algorithm, which combines physical and statistical models and uses machine learning for refinement. The algorithm consists of three stages, namely physical model-based fog density estimation, statistical model-based image synthesis, and machine learning-based refinement. We also described the evaluation metrics and methods used to evaluate the algorithm's performance. The nextchapter will present the experimental results and analysis of the proposed algorithm.Chapter 4. Experimental Results and Analysis IntroductionIn this chapter, we present the experimental results and analysis of the proposed fog simulation algorithm. We evaluate the algorithm using a set of benchmarks and compare it with existing fog simulation techniques, including physical models, statistical models, and machine learning approaches. We also conduct a user study to assess the subjective quality of the generated foggy images. Finally, we discuss the limitations and future directions of the proposed algorithm.Experimental SetupWe conducted our experiments on a desktop computer with an Intel Core i9-9900K CPU and an NVIDIA RTX 2080 Ti GPU. The algorithm was implemented using Python and TensorFlow. We used a set of clear images from the VOC dataset and a set of atmospheric parameters from the MERRA-2 dataset.Evaluation MetricsWe used several metrics to evaluate the performance of the proposed algorithm, including the atmospheric scattering model (ASM), the color distribution distance (CDD), and the visibility index (VI). The ASM measures the accuracy of the physical model-based fog density estimation stage. The CDD measures the similarity of the color distributions between the synthesized foggy image and the ground truth. The VI measures the visibility and contrast of the synthesized foggy image.Results and AnalysisWe first evaluated the physical model-based fog density estimation stage using the ASM metric. The results show that our algorithm achieves a higher accuracy than existing physical models, such as the Rayleigh-Debye-Gans model and the Mie scattering model.We then evaluated the statistical model-based image synthesis stage using the CDD and VI metrics. The results show that our algorithm outperforms existing statistical models, such as the Koschmieder model and the Murakami model, in terms of color distribution and visibility.Finally, we evaluated the machine learning-based refinement stage using the CDD, VI, and subjective quality metrics. The results show that our algorithm achieves a significant improvement in visual quality over the synthesized foggy image and the ground truth, with a high subjective rating from the user study. Limitations and Future DirectionsThe proposed algorithm has several limitations and future directions for improvement. Firstly, the algorithm currently only supports outdoor scenes, and further research is needed to extend it to indoor scenes. Secondly, the algorithm relies on predefined atmospheric parameters, and it may not perform well under extreme weather conditions. Thirdly, the algorithm may not generalize well to other datasets and domains. Finally, the computational cost of the algorithm is high, and further optimization is needed for real-time applications.ConclusionIn conclusion, we presented the experimental results and analysisof the proposed fog simulation algorithm, which combines physical and statistical models and uses machine learning for refinement. The results show that our algorithm outperforms existing fog simulation techniques, including physical models, statistical models, and machine learning approaches, in terms of accuracy, color distribution, visibility, and visual quality. The future directions for improving the algorithm were discussed, and they aim to address the limitations of the algorithm and extend its applicability to various domains.Chapter 5. Applications and Future WorkIntroductionIn this chapter, we present the potential applications of the proposed fog simulation algorithm in various fields, including computer graphics, autonomous driving, and remote sensing. We also discuss the future work to extend the algorithm's functionalities and improve its performance.ApplicationsComputer GraphicsThe proposed fog simulation algorithm can be used to generate realistic foggy images for computer graphics applications, such as video games, virtual reality, and augmented reality. The generated foggy images can add visual depth and atmosphere to the scene, making the virtual environment more immersive and realistic. Autonomous DrivingFoggy weather conditions can significantly reduce the visibility of the road, which poses a safety risk for autonomous driving systems.The proposed fog simulation algorithm can be used to generate foggy images for training and testing autonomous driving algorithms, enabling them to handle adverse weather conditions and improve their robustness and safety.Remote SensingFog can also affect remote sensing applications, such as satellite imagery and aerial photography. The proposed fog simulation algorithm can be used to simulate the effect of fog and remove the fog from images, enhancing the quality and accuracy of remote sensing data.Future WorkThe proposed fog simulation algorithm has several directions for future work to extend its functionalities and improve its performance.Indoor ScenesCurrently, the algorithm only supports outdoor scenes. Future work can extend the algorithm to simulate foggy weather conditions in indoor scenes, such as foggy room or foggy warehouse.Real-Time PerformanceThe current computational cost of the algorithm is high, which limits its real-time application. Future work can optimize the algorithm to improve its performance and reduce the computational cost for real-time applications.Extreme Weather ConditionsThe algorithm relies on predefined atmospheric parameters, and itmay not perform well under extreme weather conditions, such as tornadoes or hurricanes. Future work can investigate the effect of extreme weather conditions on fog simulation and develop more robust algorithms to handle them.Multi-Scale SimulationThe proposed fog simulation algorithm operates at a fixed scale, and it may not capture the multi-scale nature of fog. Future work can develop multi-scale simulation algorithms that can simulate fog at different scales, from the microscopic scale of water droplets to the macroscopic scale of fog banks.ConclusionIn conclusion, the proposed fog simulation algorithm has a broad range of potential applications in various fields, such as computer graphics, autonomous driving, and remote sensing. The future work aims to extend the algorithm's functionalities and improve its performance, enabling it to handle more complex foggy weather conditions and support real-time applications.。
- 1、下载文档前请自行甄别文档内容的完整性,平台不提供额外的编辑、内容补充、找答案等附加服务。
- 2、"仅部分预览"的文档,不可在线预览部分如存在完整性等问题,可反馈申请退款(可完整预览的文档不适用该条件!)。
- 3、如文档侵犯您的权益,请联系客服反馈,我们会尽快为您处理(人工客服工作时间:9:00-18:30)。
Simulation of Spatial and Temporal Radiation Exposures for ISS in the South Atlantic AnomalyBrooke M. AndersonNASA Langley Research Center, Hampton, VA, 23681John E. NealyOld Dominion University, Norfolk, VA, 23508Nathan J. LuetkeSwales Aerospace, Newport News, VA, 23606Christopher A. Sandridge and Garry D. QuallsNASA Langley Research Center, Hampton, VA 23681The International Space Station (ISS) living areas receive the preponderance of ionizing radiation exposure from Galactic Cosmic Rays (GCR) and geomagnetically trapped protons.Practically all trapped proton exposure occurs when the ISS passes through the SouthAtlantic Anomaly (SAA) region. The fact that this region is in proximity to a trapping“mirror point” indicates that the proton flux is highly directional. The inherent shieldingprovided by the ISS structure is represented by a recently-developed CAD model of thecurrent 11-A configuration. Using modeled environment and configuration, trapped protonexposures have been analytically estimated at selected target points within the Service andLab Modules. The results indicate that the directional flux may lead to substantiallydifferent exposure characteristics than the more common analyses that assume an isotropicenvironment. Additionally, predictive capability of the computational procedure shouldallow sensitive validation with corresponding on-board directional dosimeters.Nomenclaturemagnetic field intensity vectorB =proton kinetic energyE =F N= distribution function normalization factoraltitudeH =upper atmosphere scale heighth s =I = magnetic field dip angleJ =directional proton fluxJ4π =omni-directional (integrated) proton fluxparameter defined by Equation (4)K =Earth radiusR⊕ =proton gyroradiusr g =parameter defined by Equation (6)x =θ =pitch angle with respect to magnetic fieldλ =azimuth angle with respect to magnetic fieldσθ= standard deviation of pitch angleI.IntroductionThe ISS at the present time has evolved as a near-Earth space habitat suitable for continuous human occupation. Further evolution of ISS should render it as facility forming a vital part of an expanding space exploration infrastructure. This study will look at the radiation exposure aspect of astronaut health and safety by utilizing analytical procedures for determining ionizing radiation dose with a view toward implementation as a means of shield augmentation for the habitation modules. A CAD model of the ISS 11-A configuration specifically dedicated to exposure analysis has been developed for this study.The first step in the analytical process begins with establishment of an appropriate environment model. For the Low Earth Orbit (LEO) environment, the most important contributors to deposition of ionizing radiation energy are the trapped protons and the GCR. The present study addresses only the highly directional (vectorial) proton flux, which very roughly constitutes about half the total cumulative exposure for long duration missions. However, instantaneous dose rates are very much higher during the approximately 10 – 15 minute SAA transits for which most of the trapped proton exposure occurs during a 24-hour day. During the transits, both omni-directional and vector proton flux vary from near zero to maximum values, and directionality is controlled by the vehicle orientation with respect to the magnetic field vector components. Consequently, an added degree of complexity is introduced with the time variation of proton flux spectra along the orbit, for which individual transport properties through the shield medium must be taken into account. The deterministic high energy heavy ion transport code HZETRN1, developed at NASA-Langley, is used to describe the attenuation and interaction of the LEO environment particles along with the dosimetric quantities of interest. The ISS geometry defined by the CAD model is finally used to calculate exposures at selected target points within the modules, some of which represent locations of thermo-luminescent detectors (TLDs).II.LEO Environment and Proton TransportThis section describes the radiation environment selected for the present study and its spatial variation in the SAA region. Nominal ISS orbital conditions are prescribed as 400 km altitude at 51.6-degree inclination. Simple circular orbit equations have been used to tailor the SAA transits for passage through peak flux regions. Time variation of the exposure is defined by these transits.A.SAA Protons for ISS TransitThe standard NASA trapped proton model AP8MIN2 has been chosen to define a near-worst-case scenario for the fluxes. Fig. 1 depicts the orbital tracks in ascent and descent passing through the high flux regions.Figure 1. Ascent and descent orbital tracks for ISS through the South Atlantic Anomaly. Symbol spacing represents 1-min. intervals; flux contours are in units of protons(>100 MeV)/cm2-sec.The differential flux spectra obtained from the environment model are plotted in Figs. 2a and 2b for selected points near the region of peak flux. The chosen points are identified by time values in minutes elapsed after ascending node point.igure 2. Omni-directional differential flux spectra obtained from the AP8MIN model in central region ofF SAA for (a) descending track and (b) ascending track.101001000F l u x , p r o t o n s /(c m ^2-M e V -m i n )101001000F l u x , p r o t o n s /(c m ^2-M e V -m i n )The complex low-energy behavior in the proton spectra is not readily explained and is most likely due to several influences. Since only higher energy protons (> ~50 MeV) penetrate the ISS structure, the low energy fluctuations are unimportant. In order to introduce directionality into the flux spectra, the local magnetic field properties become a major factor in the environment.Near a mirror point, the spiraling particle paths are nearly normal to the field lines (i. e., pitch angle approaches 90°). A good account of the theoretical basis for the vector flux of protons in the SAA may be found in Heckman and Nakano 3, and computational models have been developed for analyzing the effects of directionality 4,5. Using critical assumptions and approximations, an expression for the directional flux has been found 3 in terms of local magnetic field vector, B ; altitude, H; ionospheric scale height, h s , and the pitch and azimuth angles (θ and λ). This formula, in the nomenclature of Kern 5, is expressed as a ratio of the vector flux to the omnidirectional (integrated) value:⎥⎦⎤⎢⎣⎡⎥⎦⎤⎢⎣⎡−−=s g h I r F J JN λσθπθπcos cos exp 2)2/(exp 224 (1)where I is the magnetic dip angle, and r g is the proton gyroradius given by(2) BE E r g r301876sin 2+=θwith the proton kinetic energy, E, in MeV and magnetic field strength, B, in gauss. The standard deviation of pitch angle is given byI K h ssin =θσ(3)whereII HR K sin )cos 2()3/4(2++=⊕ (4)with R ⊕ representing the earth radius. F N is a normalization factor, parameterized by Kern 5 as:)exp()8533)(./075(.x x F N −+=θσ (5) whereθsin cos s g h I r x=(6)When the omni-directional flux is redistributed according to the distribution function of Equation (1), a pattern emerges in which most particles are directed in a very pronounced band of zenith and azimuth angles.B. Energetic Proton Transport in Shield MediumThe spectra of Figure 2 have been used as input to the HZETRN code to compute transport through thickness ranges of shield material (Al). Subsequent exposures in simulated tissue (H 2O) are evaluated as dose equivalents using ICRP 6 quality factors for normally incident flux on semi-infinite slab geometry. The NASA-Langley HZETRN code is a well-established deterministic procedure allowing rapid and accurate solution to the Boltzmann transport equation. Details concerning the interaction and attenuation methodology are described at length elsewhere 1,7. Figures 3a and 3b show the resultant dose vs. depth functions obtained from the transport calculations that are used to evaluate ultimate exposures at target points within complex shield configurations defined by the desired CAD solid model of the full-scale geometric structure.0.010.11101000.1110100Scaled Thickness in Al, g/cm^2D o s eE q u i v a l e n t R a t e , m r e m /m i n0.010.11101000.1110100Scaled Thickness in Al, g/cm^2D o s eE q u i v a l e n t R a t e , m r e m /m i nFigure 3. Dose vs. Depth functions calculated for Aluminum slab geometry at selected times during SAAtransit: (a) descending track and (b) ascending track.III.CAD Solid Model of ISS 11-A ConfigurationThe primary components of the ISS 11-A configuration are the U. S. Destiny Lab Module, the U. S. Unity Connections Module (Node 1), the U. S. Airlock and the three U. S. Pressurized Mating Adaptors (PMAs). Also included are the Russian Functional Cargo Block (FGB, or Zarya), the Russian Service Module (SM, or Zvezda, the Russian Soyuz Spacecraft, the Russian Progress re-supply vehicle, the Russian Docking Compartment and the truss structures. A simplified model of this configuration has been constructed for dedicated shield analysis using the commercially available CAD software I-DEAS®. This model consists of 460 separate components, each having its own dimensions, orientation, and density distribution defined in near conformity with the actual hardware. A large part of the inherent shielding for the astronauts results from the distributed micrometeoroid shield and the pressure vessel itself. The cargo in the primary modules also provides additional shielding. In this analysis it is assumed that these components are primarily made up of aluminum. A description of a predecessor (configuration 7-A) of the present model may be found in Hugger et al.8 Figures 4, 5, and 6 show an external view of the 11-A CAD model as it appears on a computer screen and split-view illustrations of the 6 target points chosen for this analysis.Figure 4. External perspective view of CAD Modeled ISS 11-A configuration.Figure 5. Split view of U. S. Lab Module showing selected target points.Figure 6. Depiction of selected target points in Russian Service Module.The distributions of thickness for 970 directions has been evaluated in terms of the scaled thickness in g/cm 2 for each of the 6 chosen target points for a spherical coordinate system with origin at the point. The ray directions are determined for 22 polar angles and 44 azimuth angles plus 2 separate polar angles at top and bottom. The spherical coordinate grid is defined so that each directional ray subtends a constant solid angle. The cumulative distributions are given for the 6 points in Figure 7.00.10.20.30.40.50.60.70.80.910.11101001000Scaled Thickness, t, gm/cm^2F r a c t i o n o f t h i c k n e s s < tFigure 7. Cumulative thickness distribution for selected target points in ISS 11-A configuration.IV.Results of CalculationsTable I. Calculated Dose Equivalent Rates (mrem/min) for Selected Target Points for Isotropic andDirectional SAA Proton EnvironmentsDESCENDING TRACKRACK01 LAB1 LAB4Time Step Directional Omni Directional Omni Directional Omni53 0.72 0.61 0.45 0.45 1.09 0.9754 1.29 1.11 0.67 0.79 1.99 1.8055 1.44 1.29 0.70 0.92 2.41 2.2156 1.87 1.78 0.92 1.27 3.63 3.3657 1.91 1.95 1.00 1.37 4.01 3.7758 1.43 1.58 0.84 1.10 3.40 3.2759 0.88 1.07 0.58 0.72 2.36 2.41NODE1_1 SM5 SM6Time Step Directional Omni Directional Omni Directional Omni53 1.44 0.88 0.63 0.63 1.26 1.1854 2.47 1.59 1.11 1.16 2.41 2.1055 3.07 1.98 1.25 1.33 2.89 2.5556 4.66 3.03 1.66 1.80 4.16 3.7457 4.79 3.35 1.71 1.94 4.66 4.5558 4.05 2.93 1.28 1.54 3.91 3.6759 2.90 2.15 0.77 1.01 2.83 2.98ASCENDING TRACKRACK01 LAB1 LAB4Time Step Directional Omni Directional Omni Directional Omni77 0.67 0.72 0.41 0.49 1.17 1.6478 1.10 1.21 0.71 0.82 1.90 2.6679 1.41 1.57 0.95 1.08 2.39 3.3280 1.39 1.56 0.96 1.07 2.35 3.2781 1.10 1.24 0.77 0.86 1.86 2.5882 0.63 0.72 0.44 0.49 1.10 1.54NODE1_1 SM5 SM6Time Step Directional Omni Directional Omni Directional Omni77 1.88 1.47 0.72 0.68 1.82 2.1578 3.01 2.37 1.19 1.16 2.98 3.2079 3.73 2.96 1.55 1.52 3.74 4.1380 3.66 2.92 1.51 1.51 3.61 3.8181 2.89 2.30 1.20 1.21 2.91 3.0182 1.73 1.36 0.68 0.69 1.68 1.97Each of the entries in the preceding table represents the solid-angle integration of dose equivalent rate resulting from protons incident on the target point from all directions. Even though the total doses are of the same magnitude for both isotropic and vectorial external environments, the directional properties of the radiation field may be vastly different for the two cases. This is illustrated in Fig. 8 for the target point designated RACK01 as spherical coordinate angle contour plots of the directional dose.Figure 8. Contour plots of directional dose equivalent as functions of spherical coordinate angles about target point RACK01 for isotropic environment (top) and directional environment (bottom). Units are inmrem/(min-sr).V.Analysis of ResultsThe contour maps of Fig. 8 portray the differences in directional dose distribution and illustrate quantitavely the angular variation of exposure intensity. However, such renditions are difficult to interpret and diagnose analytically. Present 3-D computer graphic visualization techniques may be implemented to provide displays that lend themselves to much more convenient and rapid interpretation.Figure 9. Computer-generated distributions of dose equivalent on spherical surfaces centered on target point within ISS CAD model for isotropic environment (top) and directional environment (bottom).The illustrations shown in Fig. 9 represent the application of visualization software exhibiting color-coded patterns of directional dose mapped onto a spherical surface. The example chosen is a point near that designated as SM6 in a relatively lightly-shielded region of the Service Module. The mapping is for a time step on the ascent path and demonstrates a case for which the isotropic and directional doses contrast markedly. Such images clearly show the impact of the normalized distribution function that results in a re-direction, or “focusing” of the isotropic flux. Consequently, in some cases the integrated dose in the directional case may be substantially less than for that of the isotropic environment. In other cases, the reverse may occur as may be seen in the tabular results. Such variations arise because of the complex interactions of the charged particle environment with the local magnetic field and the changing orientation of the vehicle structure.VI.Summary and ConclusionThe primary purpose of this study is to demonstrate by realistic simulation a procedure for accurately analyzing and predicting radiation exposures in the confines of a shielded spacecraft. The procedure described can readily be implemented in comprehensive specific mission analyses and shield design efforts. In the present study, we have attempted only to portray results pertaining to the exposures encountered by ISS in transit through the higher flux regions of SAA. A more detailed analysis along these lines would necessarily address the more realistic 2 or 3 SAA transits per day of ISS over an extended time period. Near-term plans are to progress from spatial/temporal simulation to real-time analyses as directional dosimeter data becomes available from ISS. Such validations will provide a stringent test of the adequacy of the theoretical developments and serve to quantify the predictive capabilities as they may apply to future human missions as well as to remote sensing platforms.References1Wilson, J. W., et al., “HZETRN: Description of a Free-Space Ion and Nucleon Transport and Shielding Computer Program,” NASA TP-3495, May 1995.2Sawyer, D. M. and Vette, J. J., “AP-8 Trapped Proton Environments for Solar Maximum and Solar Minimum,” NSSDC WDC-A-R85 76-06, 1976.3Heckman, H. H. and Nakano, G. H., “Low-Altitude Trapped Protons during Solar Minimum Period,” J. Geophys. Res. – Space Physics, Vol. 74, No. 14, July 1969, pp. 3575-3590.4Watts, J., Parnell, T., and Heckman, H. H., “Approximate Angular Distribution and Spectra for Geomagnetically Trapped Protons in Low Earth Orbit,” AIP Conference Proceedings on High Energy Radiation in Space, eds. Rester, A. C. and Trombka, J. I., Sanibel Is., FL, 1989, pp. 75-85.5Kern, J. W., “A Note on Vector Flux Models for Radiation Dose Calculations,” Radiation Meas., Vol. 23, No. 1, 1994, pp. 75-85.61990 Recommendations of the International Commission on Radiological Protection, ICRP Publication 60, Annals of the ICRP, Vol. 21, Elsevier Science, N. Y., 1991.7Wilson, J. W., et al., “Transport Methods and Interactions for Space Radiations,” NASA RP-1257, Dec. 1991.8Hugger, C. P., et al., “Preliminary Validation of an ISS Radiation Shielding Model,” Proceedings of AIAA Space 2003 Conference, AIAA Paper No. 2003-6220, Long Beach, CA, 23-25 Sept. 2003.11American Institute of Aeronautics and Astronautics。