研究简报与论文选登
课题研究简报怎么写
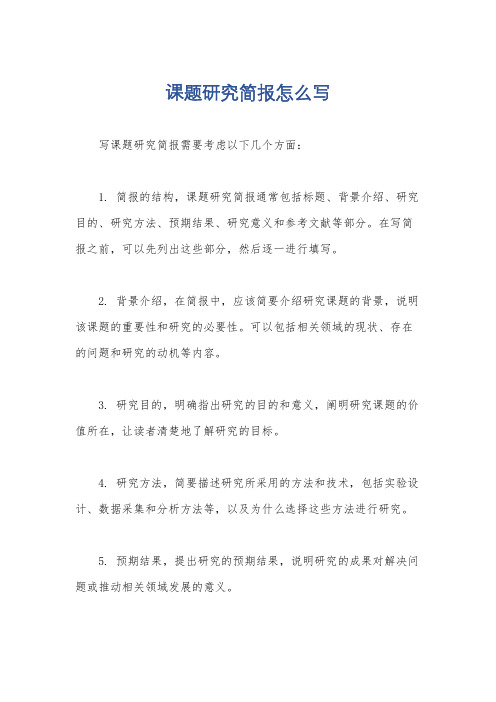
课题研究简报怎么写
写课题研究简报需要考虑以下几个方面:
1. 简报的结构,课题研究简报通常包括标题、背景介绍、研究目的、研究方法、预期结果、研究意义和参考文献等部分。
在写简报之前,可以先列出这些部分,然后逐一进行填写。
2. 背景介绍,在简报中,应该简要介绍研究课题的背景,说明该课题的重要性和研究的必要性。
可以包括相关领域的现状、存在的问题和研究的动机等内容。
3. 研究目的,明确指出研究的目的和意义,阐明研究课题的价值所在,让读者清楚地了解研究的目标。
4. 研究方法,简要描述研究所采用的方法和技术,包括实验设计、数据采集和分析方法等,以及为什么选择这些方法进行研究。
5. 预期结果,提出研究的预期结果,说明研究的成果对解决问题或推动相关领域发展的意义。
6. 研究意义,阐明研究的意义和价值,说明研究成果对学术、实践或社会的影响,以及可能带来的改变和发展。
7. 参考文献,列出研究过程中所参考的文献和资料,以及对研究有重要影响的先前研究成果。
在写课题研究简报时,需要注意言之有物、简明扼要,突出重点,尽量避免冗长和啰嗦的描述。
同时,要确保简报的逻辑性和连贯性,使读者能够清晰地理解研究课题及其意义。
最后,对简报进行反复修改和润色,确保语言通顺、准确。
希望这些建议能帮助你写出一份完整全面的课题研究简报。
中文核心期刊论文研究简报
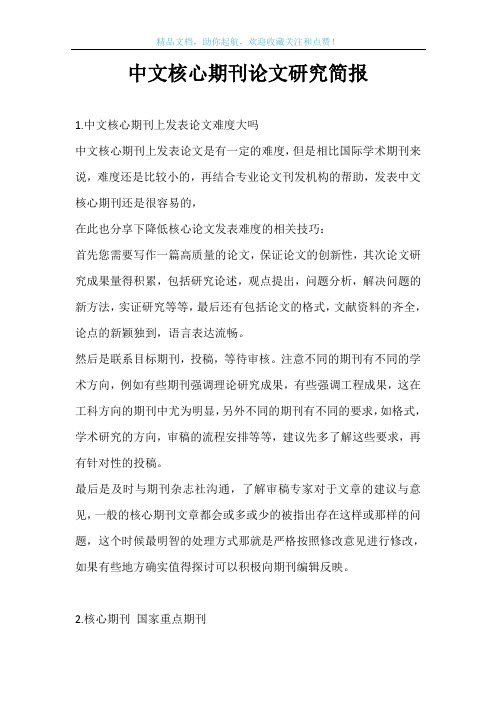
中文核心期刊论文研究简报1.中文核心期刊上发表论文难度大吗中文核心期刊上发表论文是有一定的难度,但是相比国际学术期刊来说,难度还是比较小的,再结合专业论文刊发机构的帮助,发表中文核心期刊还是很容易的,在此也分享下降低核心论文发表难度的相关技巧:首先您需要写作一篇高质量的论文,保证论文的创新性,其次论文研究成果量得积累,包括研究论述,观点提出,问题分析,解决问题的新方法,实证研究等等,最后还有包括论文的格式,文献资料的齐全,论点的新颖独到,语言表达流畅。
然后是联系目标期刊,投稿,等待审核。
注意不同的期刊有不同的学术方向,例如有些期刊强调理论研究成果,有些强调工程成果,这在工科方向的期刊中尤为明显,另外不同的期刊有不同的要求,如格式,学术研究的方向,审稿的流程安排等等,建议先多了解这些要求,再有针对性的投稿。
最后是及时与期刊杂志社沟通,了解审稿专家对于文章的建议与意见,一般的核心期刊文章都会或多或少的被指出存在这样或那样的问题,这个时候最明智的处理方式那就是严格按照修改意见进行修改,如果有些地方确实值得探讨可以积极向期刊编辑反映。
2.核心期刊国家重点期刊科技论文期刊按其学术档次及学术影响共分为四级:一、国内一级学术期刊包括《SCI》、《SSCI》、《AHCI》统计源期刊等二、国内二级学术期刊1、《中国科技论文统计源期刊》、《中文核心期刊要目总览》所列的理科学术期刊。
2、《CSSCI统计源期刊》、《中国人文社会科学核心期刊要览》、《中文核心期刊要目总览》中所列的文科学术期刊。
1.在《人民日报》、《光明日报》理论版上发表的学术论文视作一级,在《中国教育报》、《文汇报》理论版上发表的学术论文视作二级。
3、公开出版的国际专业学术会议或国家一级学会举办的全国性专业学术会议的论文集论文视作二级三、国内三级学术期刊公开出版的国家二级学会或省级学会举办的学术会议的论文集论文视作三级期刊,各级学术期刊的增刊、专刊、专辑等均降一级。
课程研究成果简报
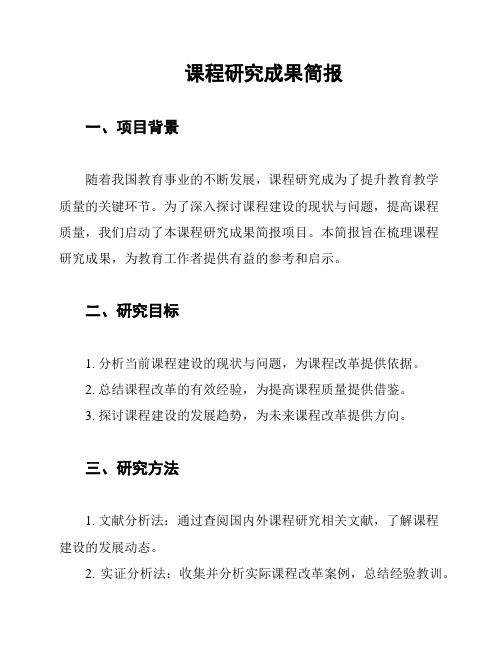
课程研究成果简报一、项目背景随着我国教育事业的不断发展,课程研究成为了提升教育教学质量的关键环节。
为了深入探讨课程建设的现状与问题,提高课程质量,我们启动了本课程研究成果简报项目。
本简报旨在梳理课程研究成果,为教育工作者提供有益的参考和启示。
二、研究目标1. 分析当前课程建设的现状与问题,为课程改革提供依据。
2. 总结课程改革的有效经验,为提高课程质量提供借鉴。
3. 探讨课程建设的发展趋势,为未来课程改革提供方向。
三、研究方法1. 文献分析法:通过查阅国内外课程研究相关文献,了解课程建设的发展动态。
2. 实证分析法:收集并分析实际课程改革案例,总结经验教训。
3. 比较分析法:对比不同地区、不同学科的课程建设情况,挖掘共性与个性。
4. 专家访谈法:邀请课程研究领域专家,咨询意见与建议。
四、研究内容1. 当前课程建设的现状与问题分析。
2. 课程改革的有效经验总结。
3. 课程建设的发展趋势探讨。
4. 对未来课程改革的建议。
五、研究成果1. 现状与问题分析:通过文献分析、实证分析等方法,我们发现当前课程建设存在以下问题:(列出问题)2. 经验总结:通过比较分析、专家访谈等方法,我们总结出以下课程改革的有效经验:(列出经验)3. 发展趋势探讨:结合文献分析、实证分析等方法,我们预测课程建设未来可能发展趋势:(列出趋势)4. 建议:针对现状与问题,结合经验与趋势,我们提出以下建议:(列出建议)六、结论本课程研究成果简报通过多种研究方法,深入分析了当前课程建设的现状与问题,总结了课程改革的有效经验,探讨了课程建设的发展趋势,并提出了对未来课程改革的建议。
我们希望这份简报能为教育工作者提供有益的参考,共同为提高我国课程质量贡献力量。
七、参考文献(列出参考文献)八、附录(如有必要,可附上相关数据、图表等材料){content}。
中国卫生统计投稿要求

投稿邮箱:yyxbzz@1 研究报告与研究简报1.1 文字量:研究报告一般不超过5个版面(约9000字),研究简报一般不超过4个版面。
1.2 题名:务求简明确切,一般不超过20个字,应包括主要关键词,避免使用不常见缩写词。
英文题目内容与论文中文题目应相符,一般不超过10个实词。
1.3 作者及单位:作者应限于参加研究工作者。
单位应包括单位全称,市名,邮政编码。
汉语姓名译法采用姓前名后,姓全部大写,名首字母大写,名为两个字时,中间加一半字线“-”,如:LIU Xu, WANG Hu-qu。
英文单位名称应为本单位公布或认可的标准英文名称。
1.4 摘要:要求简明扼要。
内容按目的、方法、结果、结论依次编写;在叙述“目的”的文字前冠以(目的)字样,其余同。
英文摘要要求语法正确,无拼写错误,符合英语表达习惯,按Objective, Method, Result和Conclusion依次编写;在叙述“Objective”的文字前冠以(Obje ctive)字样,其余同。
1.5 关键词: 选用在文题和摘要中出现的能反映论文特征内容、通用性比较强的3~8个规范词。
1.6 首页脚注标识项目1.6.1 资助项目:注明基金项目、攻关项目、专项项目等的名称,并在括号内注明项目编号。
1.6.2 作者简介:第一作者姓名(出生年-),性别,民族(汉族省略),籍贯,职称,学位(包括博士生导师),主要研究方向,E-mail地址,手机或座机号码(后两者以便编辑部和作者联系)。
*通讯作者姓名,E-mail地址。
征稿范围卫生统计的基本理论和方法(包括统计调查设计和实验设计,资料收集、统计计算及分析方法,统计预测等),居民健康统计(包括人口统计、疾病统计、健康发育统计及计划生育统计等),以及卫生业务统计(包括农村卫生统计、工业卫生统计、医院统计、卫生事业基本情况统计)等方面的学术论文、专题笔谈、学术讨论、方法介绍、讲座、文献综述、问题解答、实例分析、资料等文稿。
课题调研活动简报

课题调研活动简报课题调研活动简报时间:2022年5月1日地点:XX大学图书馆参与人员:研究小组成员及相关专家一、活动目的和背景本次课题调研活动旨在对某特定领域的相关问题进行深入研究,为后续研究工作提供参考和依据。
通过调研活动,了解现有研究情况,分析问题现状,明确研究方向。
二、活动内容1.文献调研研究小组成员针对所研究的课题进行了大量的文献调研工作。
通过查阅相关书籍、已发表的学术论文、行业报告等资源,对该领域的研究现状进行了充分了解,收集并整理了大量的文献资料。
2.专家访谈研究小组成员邀请了多位该领域的专家进行访谈,就相关问题进行探讨和交流。
通过与专家的深入对话,了解了专家们的研究经验和观点,并得到了一些宝贵的建议和意见。
3.问卷调查为了更全面地了解该领域的现状和问题,研究小组成员设计了一份问卷并进行了调查。
该问卷面向相关领域的从业人员和研究人员,通过统计和分析问卷结果,得出了一些有关该领域的数据和趋势。
三、调研结果与发现根据文献调研、专家访谈和问卷调查的结果,研究小组对该课题的研究现状和相关问题进行了总结和分析,得出了以下几点调研结果和发现:1.该领域的研究现状较为薄弱,目前尚缺乏系统性和深入性的研究成果。
2.某特定问题在该领域中存在广泛关注和研究,但解决该问题的方法和方案尚未有明确共识。
3.相关领域的专家普遍认为该课题的研究具有重要意义,并给出了一些研究的思路和方向。
四、下一步工作计划基于对该课题的调研结果和发现,研究小组将进一步开展以下工作:1.深入研究该课题,结合调研结果,进行理论分析和实证研究。
2.准备相关实验和数据收集工作,为后续研究工作做好准备。
3.继续与专家保持沟通和交流,汲取经验和意见,并请教专家关于研究方法和方案的建议。
五、其他事项2022年5月20日,研究小组将召开一个内部会议,对本次调研的结果进行讨论,制定下一步具体工作的计划和安排。
科研项目研究简报范文
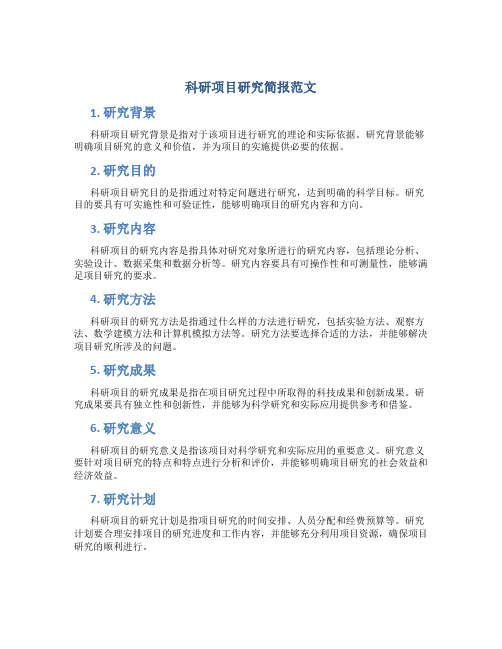
科研项目研究简报范文1. 研究背景科研项目研究背景是指对于该项目进行研究的理论和实际依据。
研究背景能够明确项目研究的意义和价值,并为项目的实施提供必要的依据。
2. 研究目的科研项目研究目的是指通过对特定问题进行研究,达到明确的科学目标。
研究目的要具有可实施性和可验证性,能够明确项目的研究内容和方向。
3. 研究内容科研项目的研究内容是指具体对研究对象所进行的研究内容,包括理论分析、实验设计、数据采集和数据分析等。
研究内容要具有可操作性和可测量性,能够满足项目研究的要求。
4. 研究方法科研项目的研究方法是指通过什么样的方法进行研究,包括实验方法、观察方法、数学建模方法和计算机模拟方法等。
研究方法要选择合适的方法,并能够解决项目研究所涉及的问题。
5. 研究成果科研项目的研究成果是指在项目研究过程中所取得的科技成果和创新成果。
研究成果要具有独立性和创新性,并能够为科学研究和实际应用提供参考和借鉴。
6. 研究意义科研项目的研究意义是指该项目对科学研究和实际应用的重要意义。
研究意义要针对项目研究的特点和特点进行分析和评价,并能够明确项目研究的社会效益和经济效益。
7. 研究计划科研项目的研究计划是指项目研究的时间安排、人员分配和经费预算等。
研究计划要合理安排项目的研究进度和工作内容,并能够充分利用项目资源,确保项目研究的顺利进行。
8. 结论科研项目研究简报的结论是对项目研究所取得的主要成果进行总结和归纳。
结论要准确、简明地表达项目研究的核心观点和重要结论,并提出进一步的研究建议。
9. 参考文献在科研项目研究简报中,需要列出所引用的参考文献。
参考文献可以是已经发表的学术论文、研究报告和专著等,要求准确标注文献的作者、题目、出版时间和出版地点等信息。
10. 致谢在科研项目研究简报中,需要对对项目研究提供支持和帮助的机构或个人表示感谢。
致谢部分要真实客观地表达对帮助和支持的感激之情,并表达对帮助和支持的认可和赞赏。
课题研究任务分配简报范文
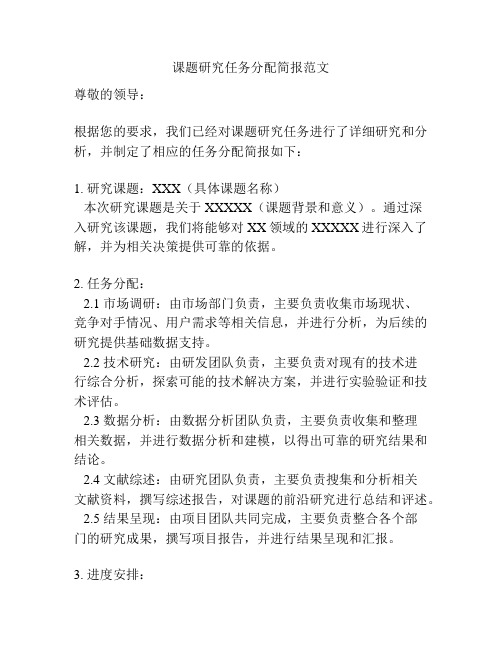
课题研究任务分配简报范文尊敬的领导:根据您的要求,我们已经对课题研究任务进行了详细研究和分析,并制定了相应的任务分配简报如下:1. 研究课题:XXX(具体课题名称)本次研究课题是关于XXXXX(课题背景和意义)。
通过深入研究该课题,我们将能够对XX领域的XXXXX进行深入了解,并为相关决策提供可靠的依据。
2. 任务分配:2.1 市场调研:由市场部门负责,主要负责收集市场现状、竞争对手情况、用户需求等相关信息,并进行分析,为后续的研究提供基础数据支持。
2.2 技术研究:由研发团队负责,主要负责对现有的技术进行综合分析,探索可能的技术解决方案,并进行实验验证和技术评估。
2.3 数据分析:由数据分析团队负责,主要负责收集和整理相关数据,并进行数据分析和建模,以得出可靠的研究结果和结论。
2.4 文献综述:由研究团队负责,主要负责搜集和分析相关文献资料,撰写综述报告,对课题的前沿研究进行总结和评述。
2.5 结果呈现:由项目团队共同完成,主要负责整合各个部门的研究成果,撰写项目报告,并进行结果呈现和汇报。
3. 进度安排:按照初步的安排,我们计划在X月X日前完成市场调研和文献综述工作,并在X月X日前完成技术研究和数据分析工作,从而为结果呈现提供充足的时间。
4. 风险和问题:在研究过程中,我们预计可能会面临的风险和问题主要包括数据获取困难、技术难题等方面的挑战。
我们将采取相应的措施,如与相关部门合作、加强技术交流等,以解决可能出现的问题,并确保研究工作顺利进行。
5. 预期成果:我们预期通过本次研究能够获得相关领域的前沿技术和市场现状的全面了解,提供具体可行的解决方案,并为相关决策提供可靠的依据。
同时,我们将通过发表学术论文、参加相关学术会议等方式将研究成果进行推广和应用。
以上是我们制定的课题研究任务分配简报,希望得到您的批示和指导。
如果对任务分配有任何改动或调整的意见,烦请尽快告知,我们将按照您的要求进行相应的修改。
论文研究情况汇报模板
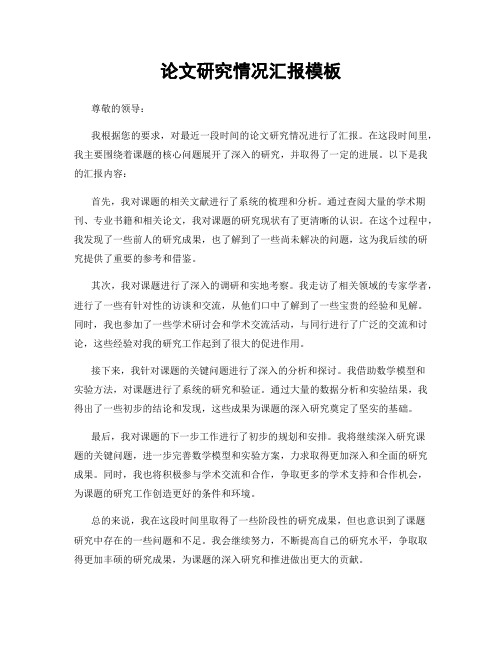
论文研究情况汇报模板尊敬的领导:我根据您的要求,对最近一段时间的论文研究情况进行了汇报。
在这段时间里,我主要围绕着课题的核心问题展开了深入的研究,并取得了一定的进展。
以下是我的汇报内容:首先,我对课题的相关文献进行了系统的梳理和分析。
通过查阅大量的学术期刊、专业书籍和相关论文,我对课题的研究现状有了更清晰的认识。
在这个过程中,我发现了一些前人的研究成果,也了解到了一些尚未解决的问题,这为我后续的研究提供了重要的参考和借鉴。
其次,我对课题进行了深入的调研和实地考察。
我走访了相关领域的专家学者,进行了一些有针对性的访谈和交流,从他们口中了解到了一些宝贵的经验和见解。
同时,我也参加了一些学术研讨会和学术交流活动,与同行进行了广泛的交流和讨论,这些经验对我的研究工作起到了很大的促进作用。
接下来,我针对课题的关键问题进行了深入的分析和探讨。
我借助数学模型和实验方法,对课题进行了系统的研究和验证。
通过大量的数据分析和实验结果,我得出了一些初步的结论和发现,这些成果为课题的深入研究奠定了坚实的基础。
最后,我对课题的下一步工作进行了初步的规划和安排。
我将继续深入研究课题的关键问题,进一步完善数学模型和实验方案,力求取得更加深入和全面的研究成果。
同时,我也将积极参与学术交流和合作,争取更多的学术支持和合作机会,为课题的研究工作创造更好的条件和环境。
总的来说,我在这段时间里取得了一些阶段性的研究成果,但也意识到了课题研究中存在的一些问题和不足。
我会继续努力,不断提高自己的研究水平,争取取得更加丰硕的研究成果,为课题的深入研究和推进做出更大的贡献。
以上就是我的论文研究情况汇报,请领导批示。
- 1、下载文档前请自行甄别文档内容的完整性,平台不提供额外的编辑、内容补充、找答案等附加服务。
- 2、"仅部分预览"的文档,不可在线预览部分如存在完整性等问题,可反馈申请退款(可完整预览的文档不适用该条件!)。
- 3、如文档侵犯您的权益,请联系客服反馈,我们会尽快为您处理(人工客服工作时间:9:00-18:30)。
P1Implementation of a Parallel Multi-dimensional Fast Fourier TransformationP20 COUPL+:并行PDE求解函数库P37 PETSc求解二维拉普拉斯方程P50 初值问题的微分-代数方程系统求解器P64 SMP集群系统上可扩展特征问题并行求解器的研究P73 基础并行软件平台建设与应用综述(续)P81 加卸载响应比理论(LURR)预测中国大陆地区2005年地震趋势取得新进展P82 2005年中科院超级计算用户成果交流会圆满结束征 稿 示启《超级计算通讯》是中国科学院计算机网络信息中心超级计算中心主办的综合性刊物,每季一期。
本刊兼具学术性、科普、要闻报道和趣味性,不定期开辟有“研究简报、论文选登”、“学习园地”、“网格论坛”、“超级计算机”、“专家论坛”、“信息港”、“交流之家”、“他山之石——文摘”、“本期人物”、“企业之星”、“会议信息”等栏目。
希望可以为广大高性能计算研究人员、应用开发人员、各级管理人员和高等院校师生提供一个相互学习交流的园地,一个获取信息的窗口,一个展示高性能计算及其应用成果的平台。
热烈欢迎所有致力于高性能计算研发的工作人员,以及社会各界人士为本刊撰稿。
来稿请注明作者姓名、工作单位、通讯地址、邮政编码、email地址。
稿件一经录用,酌付稿酬,并赠送本期《超级计算通讯》。
来稿请寄:100080北京市中关村南四街四号 北京349信箱中国科学院计算机网络信息中心超级计算中心《超级计算通讯》编辑部 徐 薇(收)或发送电子邮件至 wxu@研究简报与论文选登Implementation of a Parallel Multi-dimensional FastFourier TransformationX.B. Chi E. van den Berg Q. Cheng J. ChenComputer Network Information CenterChinese Academy of Sciences, Beijing, 100080, P.R. Chinachi@ ewout@ walls@Abstract: In this article we consider the parallel implementation of themultidimensional fast Fourier transformation and describe in detail a number ofaspects that need addressing; data flow planning, communication and data layout. Weintroduce three different data layouts and discuss their implications on the parallelFFT algorithm. We then consider the performance of the algorithms on a clustercomputer and evaluate the resulting speedup curves.1 IntroductionThe Fourier transformation is a widely known mathematical tool and has applications in a wide range of scientific areas such as signal processing, speech recognition and synthesis, geophysical research, and image processing, but also in less obvious fields such as the solution of certain partial differential equations, due to a number of useful properties of the transformation [1]. The 1-dimensional Fourier transformation and its inverse are defined as22()() (forward transformation)()() (inverse trasformation)kx kx F k f x e dx f x F k e dk ππ∞−−∞∞−−∞==∫∫ (1)When considering problems that require computational, rather than analytical mathematics, either because no explicit solution to the above functions can be found for the given f(x) or F(k), or because the functions are altogether unknown and we only know their evaluation at a number of regularly spaced values for x or k, or simply because it is computationally more efficient, we can turn to the discrete Fourier transformation (DFT) which is defined by210(),, with 0n i rk n n r k k y F x y x e r n π−===≤∑<(2)for 1-dimensional problems, with x m , the known sample values in the spatial domain—1—《超级计算通讯》 Vol.3,No.3,2005,9and y k , 0 ≤ k ≤ n - 1, the dual values in the frequency domain. By interchanging x k and y r , and k and r k at all other positions, and negating the exponent of e , we get the inverse transformation x = F n −1 (y ). The DFT defined above can easily be extended to an arbitrarily high d -dimensional DFT as follows;121122*********11,,...,,,...,000...... (with 0,1,...,-1, for 1)d d d d d d d n n n r k r k r k r r r n n n k k k i i k k k y x r n ωωω−−−=====∑∑∑i d ≤≤ (3)where we defined2i n n e πω= (4)for conciseness of notation. For the 1-dimensional DFT, we need to evaluate n summations, each consisting of n terms, so that the overall complexity of this operations seems to be O (n 2). However, in 1965 Cooley and Tukey [2] showed that by exploiting the inherent symmetry in the transformation, this complexity can be reduced to O (n log n ) using an algorithms commonly known at the fast Fourier transformation (FFT). For two and higher dimensional transformations similar symmetry exists and the concept underlying the FFT can be extended for the fast computation of these transforms. Perhaps more convenient is the decoupling of the dimensions reducing the problem to a series of 1-dimensional DFTs. This idea is best illustrated on the 2-dimensional DFT;121211221122121212121210201201111,,0(n n n n r k r k r k r k r r n n k k n n k k k k k k y x ωωωω===−−−−===∑∑∑∑,).x (5)For a fixed k 1, the term between brackets reduces to22212212201,,n r k r r n k k k y x ω=−=∑ (6)which is simply a 1-dimensional DFT, similar to the one defined in equation 2. Since we have n1 different values for k 1, and hence n 1 independent transformations, thecomputation of has a complexity of O (n 1 · n 2 log n 2). Using these intermediate values, we can complete the original DFT by computingy11112112101,,n r k r r n k k k y y ω=−=∑ (7)for all values of k 2. This amounts to a time complexity of O (n 2n 1 log n 1), leading to an overall complexity of O (n 1n 2 log n 1n 2). In terms of matrices, this means that we can first apply Fourier transforms on all rows, followed by transformations over all columns on the results of the first step. Decomposition of higher-dimensional transforms proceeds completely analogously, successively computing 1-dimensional—2—研究简报与论文选登 discrete Fourier transformations along each dimension.2 Sequential algorithmThe sequential algorithm of a multi-dimensional fast Fourier transformation could be as simple as subsequently performing one-dimensional transformations along each dimension. Direct implementation of this approach, however, will result in an algorithm with rather poor performance due to the hierarchical nature of computer memories in which high memory locality is preferred. To achieve this desired level of memory locality an additional matrix transposition step for two-dimensional transformations and dimension reordering steps for higher-order dimensions is needed, so that the input data for the Fourier transformation are stored in adjacent locations in memory. Such an operation, even at its cost of O(nm) for an n × m matrix, contributes significantly to the performance of the FFT as a whole. As such, the resulting sequence of operations for transformations becomes as is outlined in figure 1(a) for a two-dimensional, and as figures 1(c,d) for multi-dimensional transforms.The letters in the figures represent arrays that serve as the input and output of the different operations, with A denoting the array holding the initial data to be transformed, and B and C serving as intermediate arrays. Array B, in addition is designated to store the final transformation result. At any time only two different arrays are used which means that either B or C can coincide with A to save the amount of memory used.(a)(b)—3—《超级计算通讯》 Vol.3,No.3,2005,9(c)(d)Figure 1: Possible data-flows for (a) two-dimensional, and (c) even and (d) odd number higher- dimensional FFT transformations. In parallel execution a distributed transpose (b) replaces the common transpose operation.The arrows indicate operations on input data and point to the array to store the result of the operation, and hence represent the data flow of the algorithm. For a convenient discussion of this data flow, we introduce a parity value that is associated with each operation, with the exception of the first FFT. For outof-place operations, ie operations for which the input and output arrays differ, the parity value is set to 1, whereas for in-place operations, ie those where the input and output arrays coincide, the parity is set to 0.Since the initial and final arrays as well as all operations are known in advance, the only thing that remains to be determined is the exact data flow path. Although the given graphs show all possible connections, some paths will result in better performance than others and in certain cases some transitions may not exist and therefore cannot be used in the path.Fourier transformations can generally be done both in-place and out-of-place, depending on the implementation of the FFT routines. The FFT routines provided by FFTW 3.0.1 support both possibilities, and it was found that transformations with a length 2n were best done in-place, whereas all other transformations were faster out-of-place.—4—研究简报与论文选登The transposition operations are more demanding in that in-place transposition can only be done efficiently when the matrix is square, which also proved to be the best choice. For all other matrices we are required to use out-of-place transposition, reducing the number of possible paths.If all preferred transitions were taken the situation might well arise that the final output end up in array C, instead of B. This problem can be overcome in two ways; either add an addition step in which the data are simply copied to B or change the destination array of one of the oper- ations. A number of time measurement clearly showed that the second solution was superior to the first one, giving rise to the question which operation to change the parity of. Since transpositions can only be changed when the matrix is square, it is best to select FFT operations for this purpose.The use of transposition for reorganizing the data fails to work for the third dimension and above and a complete reordering is needed as illustrated in figures 1(c,d). Reordering is done by sequentially reading through the data and writing it with strides and offsets such that the desired dimension order is obtained. By grouping dimensions in pairs we can limit the use of reorder operations to roughly half of the higher-order dimensions. As an example, consider an n1 × n2 × n3 × . . . × n d array. After the Fourier transformation has been applied on all n1 × n2 sub-arrays, we reorder the array such that all dimensions along which no Fourier transformation has been applied are moved to the front, ie to locations with smaller strides between elements. In the first step, for an array with d = 5 dimensions, this would leave us an array of size n3×n4×n5×n1×n2. Then, we can compute the FFTs for n3, transpose the first two dimensions and compute the FFTs over the fourth dimension, resulting in an array of size n4×n3×n5×n1×n2. We continue with another reordering step, shifting the frontmost two dimensions towards the back of the dimensions that have not yet been transformed and shift these latter dimensions to the front; n5×n4×n3×n1×n2. In this case only the fifth dimension remains so we simply compute the FFT along this dimension. As a final step the dimension order of the array is restored by special reordering operation that reverses all but the original first two dimensions. This is done to allow the transformation to return data in transposed state (with the first two dimensions interchanged) which saves time, especially when an inverse Fourier transformation is performed at a later stage. Transposed data results from omitting the rightmost transposition step in figure 1(a). By deciding this final order at this stage of the algorithm allows for a strict separation of the transformation of the first two—5—《超级计算通讯》 Vol.3,No.3,2005,9dimensions and the remaining higher-order dimensions, as shown in parts (c) and (d) of the given figure.When considering higher-order dimensions for data planning, we only need to take into account the reorder operations which are always done out-of-place. The overall planning of the data flow for the sequential algorithm is done in reverse, starting at output array B and going back towards the output array of the first FFT, granting the preferred parity values for all operations. This means that in some cases the parity desired by the first FFT operation may not be granted. However, we had already determined that the best operation to act as a spill would be the FFT, such that we obtain the desired result.A final, optional operation not shown in figure 1 is a scaling operation that multiplies all data elements by a fixed constant. This operation is always done in-place and therefore does not affect the planning phase.3 Parallel algorithmIn the parallel algorithm, the workload needs to be distributed among the available processors. This is done by partitioning the data along the first dimension ( the number of rows ) into blocks of roughly equivalent size. Each partition is then assigned to a processor and the Fourier transformation can commence. In the first step a one-dimensional FFT is applied on the vectors covering all columns, for each rows that is part of the local data. The second step again comprises a matrix transposition in which the rows and columns are interchanged and array is partitioned and distributed along the now-transposed columns. In order to transpose a column, we first need to gather its elements that are distributed across the processors and because each processor does this, an all-to-all communication operation is required. As a result, the transposition operations as depicted in figure 1(a) can no longer be used and needs to be replaced by the steps given in (b) which consists of a communication step followed by a local transposition step. An example of this process can be seen in figure 2 showing the initial data distribution on the left, the intermediate distribution, after data transfer in the middle and the final result of the global transposition operation at the right side. In the third step of the parallel algorithm Fourier transformation is performed on the transposed columns with a vector length equal to the original number of rows. The fourth, optional step is similar to the second step—6—研究简报与论文选登transposing the data back to the initial form and can be omitted when the results are to be given in transposed form.Communication in the global transposition phase can be done in blocking and non-blocking mode. In blocking mode, communication primitive functions do not return until after all communication is completed, whereas in nonblocking mode, the functions return much faster requiring additional function calls at a later stage to check the result of these operations. The advantage of non-blocking communication is that some computations can be done during data transfer thus allowing partial overlap of the two and reducing the total execution time of the algorithm.In the Fourier transformation phase a potentially large number of independent transformations are performed. After one or more FFTs are completed, transfer of the results can be initiated and another set of transformations computed. After that the transfer can be finalized and the newly available results transmitted, until no more transformations need to be computed. This way nonblocking communication can be effectively used to reduce the communication overhead that puts an upper bound to the possible speed-up.Once processing of the first two dimensions has been completed, the higherorder dimensions can be transformed. At this point the only difference between the sequential and parallel algorithms is the extend of the first or second dimension, depending on whether the results are given in normal or transposed form. Therefore the transformation along these dimensions is done as shown in figures 1(c,d) without the need for any modifications or additional steps at the end.Planning of the data flow on the other hand needs to be changed in order to make the parallel algorithm perform well and correctly. When using the planning method given for the sequential algorithm in conjunction with nonblocking communication primitives problems may arise. This is due to the lack of arrays available during the transformation. Suppose what happens when an out- of-place Fourier transformation is combined with the non-blocking communication step. First, an FFT operation is applied to a number of vectors in, say, array B and the results are stored in C. These results are then transferred to other processors and, because communication is out-of-place, incoming data must be stored in B. This means that the data that have just been transformed are overwritten by partially transposed data. As long as the amounts of data send and received are equal for all processors this will work, but as soon as these amounts differ there will be at least one processor for which the data—7—《超级计算通讯》 Vol.3,No.3,2005,9that have yet to be transformed are overwritten by other data thus corruption the whole computation.There are two ways to avoid this problem; the first one is to finish all transformations prior to the communication step at the cost of the advantage that could be gained by overlapping the two steps. The second solution is to compute the Fourier transformations that are followed by commu- nication in-place whenever possible. Since communication is a more time consuming operation than transformation, this second solution is preferred over the first one which should be used only in case an in-place transformation is absolutely impossible.The above scheme can be realized by planning according to a number of rules. First, communication that is followed by non-blocking communication is strongly preferred in-place if the dimensions of the data require this. Second, the preferred parity of the hindmost, rather than that the first, FFT operation is discarded to allow non-blocking communication as much as possible. The assignment of arrays to operations is still done from the end back to the start of the total transformation, which means that a pre-planning phase is needed to detect possible problems and set a flag to modify the parity of the first FFT operation encountered. If non-blocking commu- nication occurs when the flag is still set this must also be changed to retain correctness of the parallel transform. In case blocking communication is used we merely need to check if all prefer- red parities can be granted, otherwise change that of the last FFT using the above method.4 Data structureIn order for the parallel algorithm to perform well, the communication overhead should be as small as possible. Communication time in part depends on the data layout, ie how the rows are distributed over the processors. In the following we will consider three different data layouts and discuss their advantages, disadvantages and the effects on communication.4.1 Blocked layoutThe first data layout, illustrated in figure 2(a), partitions the data into regularly shaped blocks. The partitioning along the rows is a physical one in that data in—8—(a)(b)(c)Figure 2: Data layout for the regular block-padded structure.different partitions reside in the memory of different processors, the column-wise partitioning is logical and reflects the assign- ment of rows after a global transposition operation. Higher dimensional data can be imagined as replications of the matrix shown in the above figure, such that a three-dimensional block of data (the third dimension holding the aggregated higher-order dimensions) is formed. The partitioning of this data remains as indicated by the dashed lines. The size of the blocks formed by the lines is rows np ⎡⎤⎢⎥ by columns np ⎡⎤⎢⎥, where np is the number ofprocessors. Padding is required if the number of rows or columns does not divide by np and is appended after the row or column with the highest index, ie right and bottom in the figure. The computational load distribution arising from this partition scheme is slightly skewed towards the lower numbered processors that hold full blocks of data. This, however, does not significantly affect the performance of the algorithm as the maximum number of rows processed by any processor, a value that dictates potential parallel performance, is minimal.(a)(b)(c)Figure 3: Data layout for the irregular block structure.Communication For the communication phase we interpret the data as a two-dimensional array, where the number of rows, including padding, is multiplied by the product of all higher-order dimensions, as illustrated in figure 4(a), which agrees with a row-major memory layout. Because the dimensions of the data operated on by each processor are the same, no problems arise from using non-blocking communication primitives allowing maximum overlap of computation and communication. For the best performance, communication is divided into a number of smaller operations each with a fixed upper bound on the number of rows transferred at one time. The given number of rows is determined from the optimal message size for a given machine, the total number of aggregated rows, and the number of columns in each partition.4.2 Strided layoutThe blocked layout described above has the problem that padding is explicitly incorporated in the input and output data. This means that either the user needs to take this artefact into account, or that an additional reordering step needs to be performed prior to and following Fourier transformation possibly requiring an additional block of memory. Since neither of these two solutions is entirely satisfactory, a new data layout is needed. The strided layout, depicted in figure 3(a), partitions the data such that no padding is required in the input and output arrays. The rows and columns are partitioned similarly which is a necessary requirement for the transposition phase or an inverse Fourier transformation operating on transposed data. The only difference is that the division along the rows is physical, ie these partitions are distributed amongthe processors, while the columns partitions exist only logically and do not come intoexistence until the communication phase. Given np processors, a set of x rows (or columns) is partitioned by successively assigning a number of them to a processor 0 ≤ p < np . This number is determined as follows; each processor is initially assigned x np ⎢⎥⎣⎦ rows while one additional row is added to all processors p < x mod np , so that the remainder of the above division is accounted for. Higher dimensions are dealt with in the same way as in the blocked layout.Communication Unlike the blocked layout where data communication proceeds fairly naturally with fixed block sizes, the strided layout introduces a number of issues that complicate the communication phase. These all arise from the variations in block sizes sent and received. The most obvious complication is the all-to-all communication itself needing to deal with different block sizes and offsets. This problem is amplified when dealing with higher- dimensional transformations, as shown in figure 4(b) where the blocks received from processor p = 3 have fewer rows than the others. These gaps need to be padded before higher- dimensional instances of the two-dimensional array can be stored. Consequentially, due to the lack of padding in the data sent, the higher-order dimensions can no longer be merged with the first dimension (number of rows), unless this dimension divides by the number of processors. This too implies that for each instance of the two-dimensional array, a separate communication phase is needed which may seriously affect parallel performance. A final consequence of the uneven block size is that in-place transpositions ( with source and destination arrays equal ) are possible only if the number of rows and columns are equal and divide by the number of processors used.(a)(b)(c)Figure 4: Data layout multi-dimensional arrays (a) block padded; (b) strided; (c) continuous. 4.3 Contiguous layoutAlthough the strided layout eliminated the need for explicit padding it did introduce intermediate padding in the communication phase and as a result, problems with communication of higher-order dimensional data. The contiguous data layout was conceived to overcome these problems while maintaining a layout that does not require padding at the input and output level. The contiguous and strided data layouts are similar and differ only in the way received data are stored prior to the transposition phase. Thus, the assignment of rows and columns to processors remains unaltered from the strided layout. In figure 4(c) the data layout after receiving multi- dimensional data is given. Unlike the strided layout where data are stored in abutted blocks, the contiguous layout groups data according to the processor it originates from. Due of the lack of gaps in data, higher-order dimensions can once collated with the first matrix dimension allowing for more efficient communication. Another potentialadvantage of the contiguous layout is that the data need not be reordered into blocks that are subsequently transposed to yet other blocks which may have a negative effecton cache efficiency. Instead, the data is left in its sequential form and read likewisefor transposition.5 Performance evaluationEach of the three different data layouts were incorporated in the Parallel Multi-Dimensional Fast Fourier Transformation (pmdfft) library for testing. Writtenin C, the library benefits from the routines defined by the MPI standard and uses the one-dimensional Fouriertransformation routines provided by the FFTW library [3]. The library is freely available on-line and can be downloaded at . Similar to FFTW, pmdfft has a planning and an execution phase in which the transformations is prepared respectively computed. The planning phase includes the preparation of all one-dimensional Fourier transformations, the definition of all required MPI data types and the designation of arrays to operations. The execution phase takes the information that is stored in the plan and performs the actual transformation, communication and transposition operations.For the parallel performance evaluation, only the execution times are taken into consideration despite the fact that, depending on the dimension extends of the data,the planning phase can occasionally take a significant amount of time. However, when a great number of transformations are done, this overhead becomes negligiblefor individual transformations and can be safely omitted. As a reference, the planning and execution times of the sequential FFT are given in table 1 for a number of different array sizes.Table 1: Sequential planning and execution times for different matrix sizes.Normal TransposedExecution Planning Execution Size Planning1024×1024 0.010728 0.267979 0.009331 0.223688 2571×438 0.187368 2.511004 0.147429 2.418615 31×72×32×20 0.014203 1.070320 0.013491 0.987648 240×490×1000 0.008226 58.500558 0.008387 54.087522 The platform used for testing is the Deepcomp 6800 installed at theSupercomputing Center of the Computer Network Information Center – Chinese Academy of Sciences (SCCAS) in Beijing. The machine is an SMP cluster with a total of 256 nodes, equipped with four Itanium II processors running at 1.3 GHz and interconnected by a QsNet network, good for a peak performance of 5.324 GFlops.(a) 1024 × 1024, contiguous(b) 2571 × 438, contiguousFigure 5: Speed-up plotted against number of processors for parallel Fourier transformation of arrays of size 1024 × 1024 (a), and 2571 × 438 (b) for the contiguous data layout.Figures 5 and 6 give the speed-up curves for the arrays given in table 1. In figure 5 the results for the two-dimensional arrays 1024×1024 and 2571×438 with contiguous layout are given. The other two layouts are omitted here because of the similarity of their results. From the table we can see that although the amounts of dataof the two arrays differ only by approximately 7%, the executionand planning times differ considerable. This difference is caused by the favourable dimensions of the former matrix (powers of two) which are particularly suited for FFT, whereas the dimensions of the latter matrix (containing large prime factors) require different FFT algorithms that are much slower. This also results in a better parallel performance for the 2571×438 matrix, compared to the 1024×1024 matrix, because the relative communication overhead for the former is less and therefore has little impact on the speed-up of the algorithm; computation is the dominant factor. Note also that the non-blocking versions of the algorithm are generally faster than the blocking versions and as a result have better speed-up values. Leaving the output in tansposed form is not only faster in the sequential program but also leads to higher speed-up values because of the reduced communication overhead.In figure 6 the differences in performance between the three data layouts are more apparent. Most striking is the poor performance when using the strided layout. This is caused mainly because of its general inability to group together the higher-order dimensions thus prohibiting more efficient communication. The blocked and contiguous layouts show much better results both for the small 4-dimensional array, as well as for the large 3-dimensional array that requires much more communication. Also apparent is the difference in blocking and nonblocking communication modes, the latter typically being faster. In accordance with expectations, leaving output in transposed order gives better speed-up figures as the result of the reduction in communication.(a)31 × 72 × 32 × 20, blocked。