Low-Complexity ML Decoding for Convolutional Tail-Biting Codes
低密度校验码的混合比特反转译码算法

低密度校验码的混合比特反转译码算法
周立媛;张立军;陈常嘉
【期刊名称】《北京交通大学学报》
【年(卷),期】2005(029)002
【摘要】提出了低密度校验(LDPC)码的混合比特反转(HBF)译码算法,该算法充分利用了LDPC码置信传播(BP)输出的软信息,对经典的比特反转(BF)算法的误码性能有明显改善.AWGN信道下的仿真结果表明,在相同的译码复杂度情况下,HBF算法的性能明显优于BP算法,并呈现出更低的误码平台.
【总页数】4页(P47-50)
【作者】周立媛;张立军;陈常嘉
【作者单位】北京交通大学,电子信息工程学院,北京,100044;北京交通大学,电子信息工程学院,北京,100044;北京交通大学,电子信息工程学院,北京,100044
【正文语种】中文
【中图分类】TN929.5
【相关文献】
1.一种简化的低密度校验码译码算法的研究 [J], 许成谦;杨兴丽;霍新整
2.基于低密度校验码的OFDM编码调制译码算法 [J], 徐志江;李式巨;官军
3.SαS与高斯的混合噪声中低密度校验码的译码算法 [J], 徐志江;王亢;孟利民
4.LDPC码混合加权比特翻转译码算法 [J], 刘冰;高俊;窦高奇
5.两种低密度校验码译码算法的性能比较与分析 [J], 宋挥师;张平
因版权原因,仅展示原文概要,查看原文内容请购买。
沉积物扩散模型的参数修正

沉积物扩散模型的参数修正第一章沉积物扩散模型简介沉积物是水污染治理中一个重要的研究对象,因为它是许多重金属、有机物等污染物的主要储存和释放介质。
沉积物扩散模型(Sediment Diffusion Model)是一种数学模型,用于描述沉积物在水体中的输移和扩散规律。
该模型是一种基于物理过程的模型,它可以计算沉积物的输移速度、分布特征等参数,为沉积物的迁移评估提供了重要的工具。
沉积物扩散模型通常包括两个主要部分:输移模型和扩散模型。
输移模型用于计算沉积物在水体中的运动,包括水流对沉积物的起到作用、沉积物在水中悬浮的时间等因素;扩散模型用于估算沉积物在水体中的水平和垂直分布规律。
这些参数需要在模型中进行修正,以达到更为准确的模拟结果。
第二章沉积物扩散模型中的参数修正沉积物扩散模型的参数修正是指对模型中的输移和扩散参数进行修正,以提高模拟结果的准确性。
这些参数可能会受到许多因素的影响,如沉积物的性质、水流速度、底部土壤的类型等等。
在现有的研究中,许多学者已经探寻了这些影响,并提出了各自的修正方法。
2.1 输移参数修正输移参数的修正通常包括三个主要方面:水流速度影响、沉积物的性质影响和不同底质类型的影响。
2.1.1 水流速度影响水流速度是沉积物扩散模型中输移参数的一个重要因素。
当水流速度变化时,沉积物的输移速率也将随之改变。
然而,在实际应用中,水流速度通常难以准确测量,因此需要利用其他参数对水流速度进行修正。
例如,一些研究者尝试使用底质粗糙度、底部摩擦系数等参数来修正水流速度对输移速率的影响。
2.1.2 沉积物的性质影响沉积物的性质也是影响输移速率的一个因素。
有些沉积物具有较高的比重、较大的颗粒大小等特征,这些特征会减慢沉积物的输移速率。
因此,在修正输移参数时,需要考虑沉积物的性质对输移速率的影响。
2.1.3 不同底质类型的影响沉积物的输移速率可能会因为不同底质类型而有所不同。
例如,沙底质和泥底质的摩擦系数、粘性等性质均不同,这些特征会影响沉积物的输移速率。
一种改进的高斯频率域压缩感知稀疏反演方法(英文)
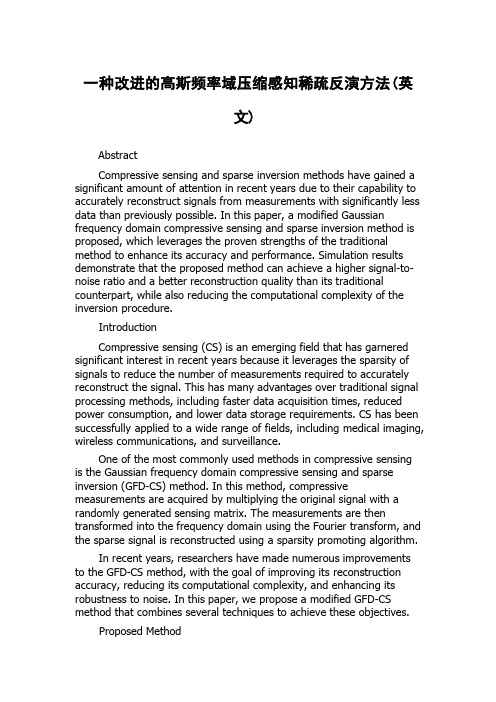
AbstractCompressive sensing and sparse inversion methods have gained a significant amount of attention in recent years due to their capability to accurately reconstruct signals from measurements with significantly less data than previously possible. In this paper, a modified Gaussian frequency domain compressive sensing and sparse inversion method is proposed, which leverages the proven strengths of the traditional method to enhance its accuracy and performance. Simulation results demonstrate that the proposed method can achieve a higher signal-to- noise ratio and a better reconstruction quality than its traditional counterpart, while also reducing the computational complexity of the inversion procedure.IntroductionCompressive sensing (CS) is an emerging field that has garnered significant interest in recent years because it leverages the sparsity of signals to reduce the number of measurements required to accurately reconstruct the signal. This has many advantages over traditional signal processing methods, including faster data acquisition times, reduced power consumption, and lower data storage requirements. CS has been successfully applied to a wide range of fields, including medical imaging, wireless communications, and surveillance.One of the most commonly used methods in compressive sensing is the Gaussian frequency domain compressive sensing and sparse inversion (GFD-CS) method. In this method, compressive measurements are acquired by multiplying the original signal with a randomly generated sensing matrix. The measurements are then transformed into the frequency domain using the Fourier transform, and the sparse signal is reconstructed using a sparsity promoting algorithm.In recent years, researchers have made numerous improvementsto the GFD-CS method, with the goal of improving its reconstruction accuracy, reducing its computational complexity, and enhancing its robustness to noise. In this paper, we propose a modified GFD-CS method that combines several techniques to achieve these objectives.Proposed MethodThe proposed method builds upon the well-established GFD-CS method, with several key modifications. The first modification is the use of a hierarchical sparsity-promoting algorithm, which promotes sparsity at both the signal level and the transform level. This is achieved by applying the hierarchical thresholding technique to the coefficients corresponding to the higher frequency components of the transformed signal.The second modification is the use of a novel error feedback mechanism, which reduces the impact of measurement noise on the reconstructed signal. Specifically, the proposed method utilizes an iterative algorithm that updates the measurement error based on the difference between the reconstructed signal and the measured signal. This feedback mechanism effectively increases the signal-to-noise ratio of the reconstructed signal, improving its accuracy and robustness to noise.The third modification is the use of a low-rank approximation method, which reduces the computational complexity of the inversion algorithm while maintaining reconstruction accuracy. This is achieved by decomposing the sensing matrix into a product of two lower dimensional matrices, which can be subsequently inverted using a more efficient algorithm.Simulation ResultsTo evaluate the effectiveness of the proposed method, we conducted simulations using synthetic data sets. Three different signal types were considered: a sinusoidal signal, a pulse signal, and an image signal. The results of the simulations were compared to those obtained using the traditional GFD-CS method.The simulation results demonstrate that the proposed method outperforms the traditional GFD-CS method in terms of signal-to-noise ratio and reconstruction quality. Specifically, the proposed method achieves a higher signal-to-noise ratio and lower mean squared error for all three types of signals considered. Furthermore, the proposed method achieves these results with a reduced computational complexity compared to the traditional method.ConclusionThe results of our simulations demonstrate the effectiveness of the proposed method in enhancing the accuracy and performance of the GFD-CS method. The combination of sparsity promotion, error feedback, and low-rank approximation techniques significantly improves the signal-to-noise ratio and reconstruction quality, while reducing thecomputational complexity of the inversion procedure. Our proposed method has potential applications in a wide range of fields, including medical imaging, wireless communications, and surveillance.。
纹理物体缺陷的视觉检测算法研究--优秀毕业论文
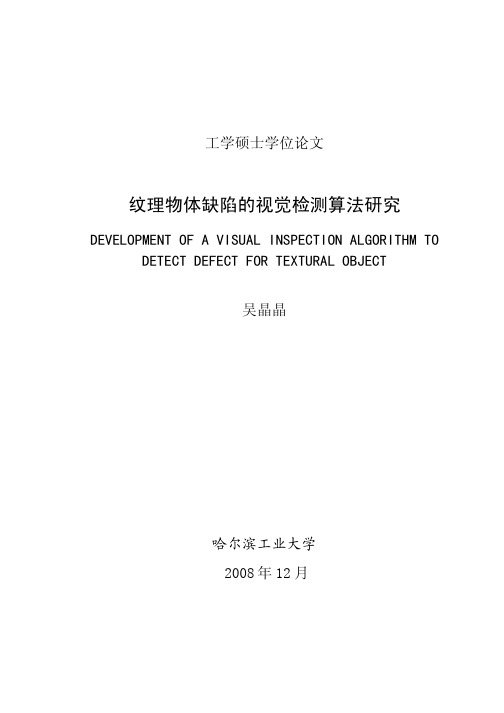
摘 要
在竞争激烈的工业自动化生产过程中,机器视觉对产品质量的把关起着举足 轻重的作用,机器视觉在缺陷检测技术方面的应用也逐渐普遍起来。与常规的检 测技术相比,自动化的视觉检测系统更加经济、快捷、高效与 安全。纹理物体在 工业生产中广泛存在,像用于半导体装配和封装底板和发光二极管,现代 化电子 系统中的印制电路板,以及纺织行业中的布匹和织物等都可认为是含有纹理特征 的物体。本论文主要致力于纹理物体的缺陷检测技术研究,为纹理物体的自动化 检测提供高效而可靠的检测算法。 纹理是描述图像内容的重要特征,纹理分析也已经被成功的应用与纹理分割 和纹理分类当中。本研究提出了一种基于纹理分析技术和参考比较方式的缺陷检 测算法。这种算法能容忍物体变形引起的图像配准误差,对纹理的影响也具有鲁 棒性。本算法旨在为检测出的缺陷区域提供丰富而重要的物理意义,如缺陷区域 的大小、形状、亮度对比度及空间分布等。同时,在参考图像可行的情况下,本 算法可用于同质纹理物体和非同质纹理物体的检测,对非纹理物体 的检测也可取 得不错的效果。 在整个检测过程中,我们采用了可调控金字塔的纹理分析和重构技术。与传 统的小波纹理分析技术不同,我们在小波域中加入处理物体变形和纹理影响的容 忍度控制算法,来实现容忍物体变形和对纹理影响鲁棒的目的。最后可调控金字 塔的重构保证了缺陷区域物理意义恢复的准确性。实验阶段,我们检测了一系列 具有实际应用价值的图像。实验结果表明 本文提出的纹理物体缺陷检测算法具有 高效性和易于实现性。 关键字: 缺陷检测;纹理;物体变形;可调控金字塔;重构
Keywords: defect detection, texture, object distortion, steerable pyramid, reconstruction
II
数字图像修复的DI灢StyleGANv2模型
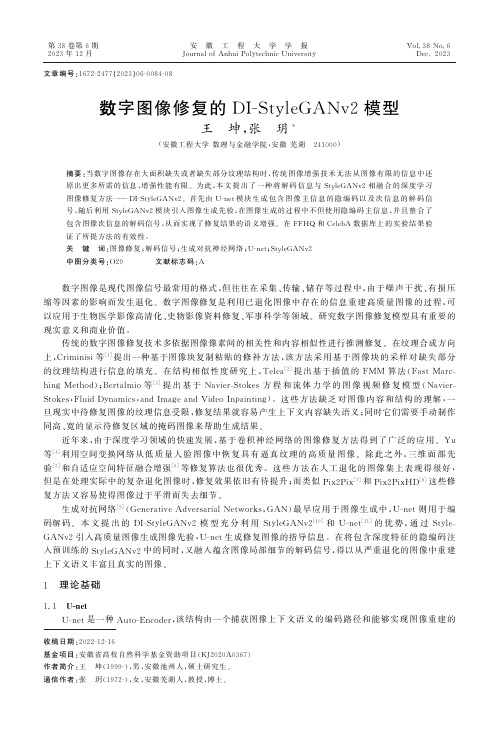
第38卷第6期2023年12月安 徽 工 程 大 学 学 报J o u r n a l o fA n h u i P o l y t e c h n i cU n i v e r s i t y V o l .38N o .6D e c .2023文章编号:1672-2477(2023)06-0084-08收稿日期:2022-12-16基金项目:安徽省高校自然科学基金资助项目(K J 2020A 0367)作者简介:王 坤(1999-),男,安徽池州人,硕士研究生㊂通信作者:张 玥(1972-),女,安徽芜湖人,教授,博士㊂数字图像修复的D I -S t yl e G A N v 2模型王 坤,张 玥*(安徽工程大学数理与金融学院,安徽芜湖 241000)摘要:当数字图像存在大面积缺失或者缺失部分纹理结构时,传统图像增强技术无法从图像有限的信息中还原出更多所需的信息,增强性能有限㊂为此,本文提出了一种将解码信息与S t y l e G A N v 2相融合的深度学习图像修复方法 D I -S t y l e G A N v 2㊂首先由U -n e t 模块生成包含图像主信息的隐编码以及次信息的解码信号,随后利用S t y l e G A N v 2模块引入图像生成先验,在图像生成的过程中不但使用隐编码主信息,并且整合了包含图像次信息的解码信号,从而实现了修复结果的语义增强㊂在F F HQ 和C e l e b A 数据库上的实验结果验证了所提方法的有效性㊂关 键 词:图像修复;解码信号;生成对抗神经网络;U -n e t ;S t y l e G A N v 2中图分类号:O 29 文献标志码:A 数字图像是现代图像信号最常用的格式,但往往在采集㊁传输㊁储存等过程中,由于噪声干扰㊁有损压缩等因素的影响而发生退化㊂数字图像修复是利用已退化图像中存在的信息重建高质量图像的过程,可以应用于生物医学影像高清化㊁史物影像资料修复㊁军事科学等领域㊂研究数字图像修复模型具有重要的现实意义和商业价值㊂传统的数字图像修复技术多依据图像像素间的相关性和内容相似性进行推测修复㊂在纹理合成方向上,C r i m i n i s i 等[1]提出一种基于图像块复制粘贴的修补方法,该方法采用基于图像块的采样对缺失部分的纹理结构进行信息的填充㊂在结构相似性度研究上,T e l e a [2]提出基于插值的F MM 算法(F a s tM a r c -h i n g M e t h o d );B e r t a l m i o 等[3]提出基于N a v i e r -S t o k e s 方程和流体力学的图像视频修复模型(N a v i e r -S t o k e s ,F l u i dD y n a m i c s ,a n d I m a g e a n dV i d e o I n p a i n t i n g )㊂这些方法缺乏对图像内容和结构的理解,一旦现实中待修复图像的纹理信息受限,修复结果就容易产生上下文内容缺失语义;同时它们需要手动制作同高㊁宽的显示待修复区域的掩码图像来帮助生成结果㊂近年来,由于深度学习领域的快速发展,基于卷积神经网络的图像修复方法得到了广泛的应用㊂Y u等[4]利用空间变换网络从低质量人脸图像中恢复具有逼真纹理的高质量图像㊂除此之外,三维面部先验[5]和自适应空间特征融合增强[6]等修复算法也很优秀㊂这些方法在人工退化的图像集上表现得很好,但是在处理实际中的复杂退化图像时,修复效果依旧有待提升;而类似P i x 2P i x [7]和P i x 2P i x H D [8]这些修复方法又容易使得图像过于平滑而失去细节㊂生成对抗网络[9](G e n e r a t i v eA d v e r s a r i a lN e t w o r k s ,G A N )最早应用于图像生成中,U -n e t 则用于编码解码㊂本文提出的D I -S t y l e G A N v 2模型充分利用S t y l e G A N v 2[10]和U -n e t [11]的优势,通过S t y l e -G A N v 2引入高质量图像生成图像先验,U -n e t 生成修复图像的指导信息㊂在将包含深度特征的隐编码注入预训练的S t y l e G A N v 2中的同时,又融入蕴含图像局部细节的解码信号,得以从严重退化的图像中重建上下文语义丰富且真实的图像㊂1 理论基础1.1 U -n e t U -n e t 是一种A u t o -E n c o d e r ,该结构由一个捕获图像上下文语义的编码路径和能够实现图像重建的对称解码路径组成㊂其前半部分收缩路径为降采样,后半部分扩展路径为升采样,分别对应编码和解码,用于捕获和恢复空间信息㊂编码结构包括重复应用卷积㊁激活函数和用于下采样的最大池化操作㊂在整个下采样的过程中,伴随着特征图尺度的降低,特征通道的数量会增加,扩展路径的解码过程同理,同时扩展路径与收缩路径中相应特征图相连㊂1.2 生成对抗网络生成对抗网络框架由生成器G 和判别器D 这两个模块构成,生成器G 利用捕获的数据分布信息生成图像,判别器D 的输入是数据集的图像或者生成器G 生成的图像,判别器D 的输出刻画了输入图像来自真实数据的可能性㊂为了让生成器G 学习到数据x 的分布p g ,先定义了一个关于输入的噪声变量z ,z 到数据空间的映射表示为G (z ;θg ),其中G 是一个由参数为θg 的神经网络构造的可微函数㊂同时定义了第2个神经网络D (x ;θd ),其输出单个标量D (x )来表示x 来自于数据而不是P g 的概率㊂本训练判别器最大限度地区分真实样本和生成样本,同时训练生成器去最小化l o g(1-D (G (z ))),相互博弈的过程由以下公式定义:m i n G m a x D V (D ,G )=E x ~P d a t a (x )[l o g (D (x ))]+E z ~P z (z )[l o g (1-D (G (z )))],(1)通过生成器㊁判别器的相互对抗与博弈,整个网络最终到达纳什平衡状态㊂这时生成器G 被认为已经学习到了真实数据的内在分布,由生成器合成的生成图像已经能够呈现出与真实数据基本相同的特征,在视觉上难以分辨㊂1.3 S t y l e G A N v 2选用高分辨率图像生成方法S t y l e G A N v 2[10]作为嵌入D I -S t y l e G A N v 2的先验网络,S t yl e G A N v 2[10]主要分为两部分,一部分是映射网络(M a p p i n g N e t w o r k ),如图1a 所示,其中映射网络f 通过8个全连接层对接收的隐编码Z 进行空间映射处理,转换为中间隐编码W ㊂特征空间中不同的子空间信息对应着数据的不同类别信息或整体风格,因为其相互关联存在较高的耦合性,且存在特征纠缠的现象,所以利用映射网络f 使得隐空间得到有效的解耦,最后生成不必遵循训练数据分布的中间隐编码㊂另一部分被称为合成网络,如图1b 所示㊂合成网络由卷积和上采样层构成,其根据映射网络产生的隐编码来生成所需图像㊂合成网络用全连接层将中间隐编码W 转换成风格参数A 来影响不同尺度生成图像的骨干特征,用噪声来影响细节部分使生成的图片纹理更自然㊂S t y l e G A N v 2[10]相较于旧版本重新设计生成器的架构,使用了权重解调(W e i g h tD e m o d u t i o n )更直接地从卷积的输出特征图的统计数据中消除特征图尺度的影响,修复了产生特征伪影的缺点并进一步提高了结果质量㊂图1 S t yl e G A N v 2基本结构根据传入的风格参数,权重调制通过调整卷积权重的尺度替代性地实现对输入特征图的调整㊂w 'i j k =s i ㊃w i jk ,(2)式中,w 和w '分别为原始权重和调制后的权重;s i 为对应第i 层输入特征图对应的尺度㊂接着S t y l e -㊃58㊃第6期王 坤,等:数字图像修复的D I -S t y l e G A N v 2模型G A N v 2通过缩放权重而非特征图,从而在卷积的输出特征图的统计数据中消除s i 的影响,经过调制和卷积后,权值的标准偏差为:σj =∑i ,k w 'i j k 2,(3)权重解调即权重按相应权重的L 2范式进行缩放,S t y l e G A N v 2使用1/σj 对卷积权重进行操作,其中η是一个很小的常数以避免分母数值为0:w ″i j k =w 'i j k /∑i ,k w 'i j k 2+η㊂(4)解调操作融合了S t y l e G A N 一代中的调制㊁卷积㊁归一化,并且相比一代的操作更加温和,因为其是调制权重信号,而并非特征图中的实际内容㊂2 D I -S t yl e G A N v 2模型D I -S t y l e G A N v 2模型结构如图2所示:其主要部分由一个解码提取模块U -n e t 以及预先训练过的S t y l e G A N v 2组成,其中S t y l e G A N v 2包括了合成网络部分(S y n t h e s i sN e t w o r k )和判别器部分㊂待修复的输入图像在被输入到整个D I -S t yl e G A N v 2网络之前,需要被双线性插值器调整大小到固定的分辨率1024×1024㊂接着输入图像通过图2中左侧的U -n e t 生成隐编码Z 和解码信号(D e c o d i n g I n f o r m a t i o n ,D I )㊂由图2所示,隐编码Z 经过多个全连接层构成的M a p p i n g Ne t w o r k s 解耦后转化为中间隐编码W ㊂中间隐编码W 通过仿射变换产生的风格参数作为风格主信息,已经在F F H Q 数据集上训练过的S t yl e G A N v 2模块中的合成网络在风格主信息的指导下恢复图像㊂合成网络中的S t y l e G A N B l o c k 结构在图2下方显示,其中包含的M o d 和D e m o d 操作由式(2)和式(4)给出㊂同时U -n e t 解码层每一层的解码信息作为风格次信息以张量拼接的方式加入S t y l e G A NB l o c k 中的特征图,更好地让D I -S t y le G A N v 2模型生成细节㊂最后将合成网络生成的图片汇入到判别器中,由判别器判断是真实图像还是生成图像㊂图2 数字图像修复网络结构图模型鉴别器综合了3个损失函数作为总损失函数:对抗损失L A ㊁内容损失L C 和特征匹配损失L F ㊂其中,L A 为原始G A N 网络中的对抗损失,被定义为式(5),X 和^X 表示真实的高清图像和低质量的待修复图像,G 为训练期间的生成器,D 为判别器㊂L A =m i n G m a x D E (X )L o g (1+e x p (-D (G (X ^)))),(5)L C 定义为最终生成的修复图片与相应的真实图像之间的L 1范数距离;L F 为判别器中的特征层,其定义为式(6);T 为特征提取的中间层总数;D i (X )为判别器D 的第i 层提取的特征:㊃68㊃安 徽 工 程 大 学 学 报第38卷L F =m i n G E (X )(∑T i =0||D i (X )-D i (G (X ^))||2),(6)最终的总损失函数为:L =L A +αL C +βL F ,(7)式(7)中内容损失L C 判断修复结果和真实高清图像之间的精细特征与颜色信息上差异的大小;通过判别器中间特征层得到的特征匹配损失L F 可以平衡对抗损失L A ,更好地恢复㊁还原高清的数字图像㊂α和β作为平衡参数,在本文的实验中根据经验设置为α=1和β=0.02㊂3实验结果分析图3 F F HQ 数据集示例图3.1 数据集选择及数据预处理从图像数据集的多样性和分辨率考虑,本文选择在F F H Q (F l i c k rF a c e s H i g h Q u a l i t y )数据集上训练数字图像修复模型㊂该数据集包含7万张分辨率为1024×1024的P N G 格式高清图像㊂F F H Q 囊括了非常多的差异化的图像,包括不同种族㊁性别㊁表情㊁脸型㊁背景的图像㊂这些丰富属性经过训练可以为模型提供大量的先验信息,图3展示了从F F H Q 中选取的31张照片㊂训练过程中的模拟退化过程,即模型生成数据集对应的低质量图像这部分,本文主要通过以下方法实现:通过C V 库对图像随机地进行水平翻转㊁颜色抖动(包括对图像的曝光度㊁饱和度㊁色调进行随机变化)以及转灰度图等操作,并对图像采用混合高斯模糊,包括各向同性高斯核和各向异性高斯核㊂在模糊核设计方面,本文采用41×41大小的核㊂对于各向异性高斯核,旋转角度在[-π,π]之间均匀采样,同时进行下采样和混入高斯噪声㊁失真压缩等处理㊂整体模拟退化处理效果如图4所示㊂图4 模拟退化处理在模型回测中,使用C e l e b A 数据集来生成低质量的图像进行修复并对比原图,同时定量比较本模型与近年来提出的其他方法对于数字图像的修复效果㊂3.2 评估指标为了公平地量化不同算法视觉质量上的优劣,选取图像质量评估方法中最广泛使用的峰值信噪比(P e a kS i g n a l -t o -n o i s eR a t i o ,P S N R )以及结构相似性指数(S t r u c t u r a l S i m i l a r i t y I n d e x ,S S I M )指标,去量化修复后图像和真实图像之间的相似性㊂P S N R 为信号的最大可能功率和影响其精度的破坏性噪声功率的比值,数值越大表示失真越小㊂P S N R 基于逐像素的均方误差来定义㊂设I 为高质量的参考图像;I '为复原后的图像,其尺寸均为m ×n ,那么两者的均方误差为:M S E =1m n ∑m i =1∑n j =1(I [i ,j ]-I '[i ,j ])2,(8)P S N R 被定义为公式(9),P e a k 表示图像像素强度最大的取值㊂㊃78㊃第6期王 坤,等:数字图像修复的D I -S t yl e G A N v 2模型P S N R =10×l o g 10(P e a k 2M S E )=20×l o g 10(P e a k M S E ),(9)S S I M 是另一个被广泛使用的图像相似度评价指标㊂与P S N R 评价逐像素的图像之间的差异,S S I M 仿照人类视觉系统实现了其判别标准㊂在图像质量的衡量上更侧重于图像的结构信息,更贴近人类对于图像质量的判断㊂S S I M 用均值估计亮度相似程度,方差估计对比度相似程度,协方差估计结构相似程度㊂其范围为0~1,越大代表图像越相似;当两张图片完全一样时,S S I M 值为1㊂给定两个图像信号x 和y ,S S I M 被定义为:S S I M (x ,y )=[l (x ,y )α][c (x ,y )]β[s (x ,y )]γ,(10)式(10)中的亮度对比l (x ,y )㊁对比度对比c (x ,y )㊁结构对比s (x ,y )三部分定义为:l (x ,y )=2μx μy +C 1μ2x +μ2y +C 1,c (x ,y )=2σx σy +C 2σ2x +σ2y +C 2,l (x ,y )=σx y +C 3σx σy +C 3,(11)其中,α>0㊁β>0㊁γ>0用于调整亮度㊁对比度和结构之间的相对重要性;μx 及μy ㊁σx 及σy 分别表示x 和y 的平均值和标准差;σx y 为x 和y 的协方差;C 1㊁C 2㊁C 3是常数,用于维持结果的稳定㊂实际使用时,为简化起见,定义参数为α=β=γ=1以及C 3=C 2/2,得到:S S I M (x ,y )=(2μx μy +C 1)(2σx y +C 2)(μ2x +μ2y +C 1)(σ2x +σ2y +C 2)㊂(12)在实际计算两幅图像的结构相似度指数时,我们会指定一些局部化的窗口,计算窗口内信号的结构相似度指数㊂然后每次以像素为单位移动窗口,直到计算出整幅的图像每个位置的局部S S I M 再取均值㊂3.3 实验结果(1)D I -S t y l e G A N v 2修复结果㊂图5展示了D I -S t yl e G A N v 2模型在退化图像上的修复结果,其中图5b ㊁5d 分别为图5a ㊁5c 的修复结果㊂通过对比可以看到,D I -S t y l e G A N v 2修复过的图像真实且还原,图5b ㊁5d 中图像的头发㊁眉毛㊁眼睛㊁牙齿的细节清晰可见,甚至图像背景也被部分地修复,被修复后的图像通过人眼感知,整体质量优异㊂图5 修复结果展示(2)与其他方法的比较㊂本文用C e l e b A -H Q 数据集合成了一组低质量图像,在这些模拟退化图像上将本文的数字图像修复模型与G P E N [12]㊁P S F R G A N [13]㊁H i F a c e G A N [14]这些最新的深度学习修复算法的修复效果进行比较评估,这些最近的修复算法在实验过程中使用了原作者训练过的模型结构和预训练参数㊂各个模型P S N R 和L P I P S 的测试结果如表1所示,P S N R 和S S I M 的值越大,表明修复图像和真实高清图像之间的相似度越高,修复效果越好㊂由表1可以看出,我们的数字图像修复模型获得了与其他顶尖修复算法相当的P S N R 指数,L P I P S 指数相对于H i F a c e G A N 提升了12.47%,同时本实验环境下修复512×512像素单张图像耗时平均为1.12s ㊂表1 本文算法与相关算法的修复效果比较M e t h o d P S N R S S I M G P E N 20.40.6291P S F R G A N 21.60.6557H i F a c e G A N 21.30.5495o u r 20.70.6180值得注意的是P S N R 和S S I M 大小都只能作为参考,不能绝对反映数字图像修复算法的优劣㊂图6展示了D I -S t y l e G A N v 2㊁G P E N ㊁P S F R G A N ㊁H i F a c e G A N 的修复结果㊂由图6可以看出,无论是全局一致性还是局部细节,D I -S t y l e G A N v 2都做到了很好得还原,相比于其他算法毫不逊色㊂在除人脸以外的其他自然场景的修复上,D I -S t yl e G A N v 2算法依旧表现良好㊂图7中左侧为修复前㊃88㊃安 徽 工 程 大 学 学 报第38卷图像,右侧为修复后图像㊂从图7中红框处标记出来的细节可以看出,右侧修复后图像的噪声相比左图明显减少,观感更佳,这在图7下方放大后的细节比对中表现得尤为明显㊂从图像中招牌的字体区域可见对比度和锐化程度的提升使得图像内部图形边缘更加明显,整体更加清晰㊂路面的洁净度上噪声也去除很多,更为洁净㊂整体上修复后图像的色彩比原始的退化图像要更丰富,层次感更强,视觉感受更佳㊂图6 修复效果对比图图7 自然场景图像修复结果展示(左:待修复图像;右:修复后图像)4 结论基于深度学习的图像修复技术近年来在超分辨图像㊁医学影像等领域得到广泛的关注和应用㊂本文针对传统修复技术处理大面积缺失或者缺失部分纹理结构的图像时容易产生修复结果缺失图像语义的问题,在国内外图像修复技术理论与方法的基础上,由卷积神经网络U -n e t 结合近几年效果极佳的生成对抗网络S t yl e G A N v 2,提出了以图像解码信息㊁隐编码㊁图像生成先验这三类信息指导深度神经网络对图像进行修复㊂通过在F F H Q 数据集上进行随机图像退化模拟来训练D I -S t y l e G A N v 2网络模型,并由P S N R 和S S I M 两个指标来度量修复样本和高清样本之间的相似性㊂㊃98㊃第6期王 坤,等:数字图像修复的D I -S t y l e G A N v 2模型㊃09㊃安 徽 工 程 大 学 学 报第38卷实验表明,D I-S t y l e G A N v2网络模型能够恢复清晰的面部细节,修复结果具有良好的全局一致性和局部精细纹理㊂其对比现有技术具有一定优势,同时仅需提供待修复图像而无需缺失部分掩码就能得到令人满意的修复结果㊂这主要得益于D I-S t y l e G A N v2模型能够通过大样本数据的训练学习到了丰富的图像生成先验,并由待修复图像生成的隐编码和解码信号指导神经网络学习到更多的图像结构和纹理信息㊂参考文献:[1] A N T O N I O C,P A T R I C KP,K E N T A R O T.R e g i o n f i l l i n g a n do b j e c t r e m o v a l b y e x e m p l a r-b a s e d i m a g e i n p a i n t i n g[J].I E E ET r a n s a c t i o n s o n I m a g eP r o c e s s i n g:A P u b l i c a t i o no f t h eI E E E S i g n a lP r o c e s s i n g S o c i e t y,2004,13(9):1200-1212.[2] T E L E A A.A n i m a g e i n p a i n t i n g t e c h n i q u eb a s e do nt h e f a s tm a r c h i n g m e t h o d[J].J o u r n a l o fG r a p h i c sT o o l s,2004,9(1):23-34.[3] B E R T A L M I O M,B E R T O Z Z IA L,S A P I R O G.N a v i e r-s t o k e s,f l u i dd y n a m i c s,a n d i m a g ea n dv i d e o i n p a i n t i n g[C]//I E E EC o m p u t e r S o c i e t y C o n f e r e n c e o nC o m p u t e rV i s i o n&P a t t e r nR e c o g n i t i o n.K a u a i:I E E EC o m p u t e r S o c i e t y,2001:990497.[4] Y U X,P O R I K L I F.H a l l u c i n a t i n g v e r y l o w-r e s o l u t i o nu n a l i g n e d a n dn o i s y f a c e i m a g e s b y t r a n s f o r m a t i v e d i s c r i m i n a t i v ea u t o e n c o d e r s[C]//I nC V P R.H o n o l u l u:I E E EC o m p u t e r S o c i e t y,2017:3760-3768.[5] HU XB,R E N W Q,L AMA S T E RJ,e t a l.F a c e s u p e r-r e s o l u t i o n g u i d e db y3d f a c i a l p r i o r s.[C]//C o m p u t e rV i s i o n–E C C V2020:16t hE u r o p e a nC o n f e r e n c e.G l a s g o w:S p r i n g e r I n t e r n a t i o n a l P u b l i s h i n g,2020:763-780.[6] L IX M,L IW Y,R E ND W,e t a l.E n h a n c e d b l i n d f a c e r e s t o r a t i o nw i t hm u l t i-e x e m p l a r i m a g e s a n d a d a p t i v e s p a t i a l f e a-t u r e f u s i o n[C]//P r o c e e d i n g so f t h eI E E E/C V F C o n f e r e n c eo nC o m p u t e rV i s i o na n dP a t t e r n R e c o g n i t i o n.V i r t u a l:I E E EC o m p u t e r S o c i e t y,2020:2706-2715.[7] I S O L A P,Z HUJY,Z HO U T H,e t a l.I m a g e-t o-i m a g e t r a n s l a t i o nw i t hc o n d i t i o n a l a d v e r s a r i a l n e t w o r k s[C]//P r o-c e ed i n g s o f t h eI E E E C o n fe r e n c eo nC o m p u t e rV i s i o na n dP a t t e r n R e c o g n i t i o n.H o n o l u l u:I E E E C o m p u t e rS o c i e t y,2017:1125-1134.[8] WA N G TC,L I U M Y,Z HUJY,e t a l.H i g h-r e s o l u t i o n i m a g es y n t h e s i sa n ds e m a n t i cm a n i p u l a t i o nw i t hc o n d i t i o n a lg a n s[C]//P r o c e e d i n g so f t h eI E E E C o n f e r e n c eo nC o m p u t e rV i s i o na n dP a t t e r n R e c o g n i t i o n.S a l tL a k eC i t y:I E E EC o m p u t e r S o c i e t y,2018:8798-8807.[9] G O O D F E L L OWI A N,P O U G E T-A B A D I EJ,M I R Z A M,e t a l.G e n e r a t i v ea d v e r s a r i a ln e t s[C]//A d v a n c e s i n N e u r a lI n f o r m a t i o nP r o c e s s i n g S y s t e m s.M o n t r e a l:M o r g a nK a u f m a n n,2014:2672-2680.[10]K A R R A ST,L A I N ES,A I T T A L A M,e t a l.A n a l y z i n g a n d i m p r o v i n g t h e i m a g e q u a l i t y o f s t y l e g a n[C]//P r o c e e d i n g so f t h e I E E E/C V FC o n f e r e n c e o nC o m p u t e rV i s i o n a n dP a t t e r nR e c o g n i t i o n.S n o w m a s sV i l l a g e:I E E EC o m p u t e r S o c i e-t y,2020:8110-8119.[11]O L A FR O N N E B E R G E R,P H I L I P PF I S C H E R,T HOMA SB R O X.U-n e t:c o n v o l u t i o n a l n e t w o r k s f o r b i o m e d i c a l i m a g es e g m e n t a t i o n[C]//M e d i c a l I m a g eC o m p u t i n g a n dC o m p u t e r-A s s i s t e dI n t e r v e n t i o n-M I C C A I2015:18t hI n t e r n a t i o n a lC o n f e r e n c e.M u n i c h:S p r i n g e r I n t e r n a t i o n a l P u b l i s h i n g,2015:234-241.[12]Y A N G T,R E NP,X I EX,e t a l.G a n p r i o r e m b e d d e dn e t w o r k f o r b l i n d f a c e r e s t o r a t i o n i n t h ew i l d[C]//P r o c e e d i n g s o ft h eI E E E/C V F C o n f e r e n c eo n C o m p u t e r V i s i o na n d P a t t e r n R e c o g n i t i o n.V i r t u a l:I E E E C o m p u t e rS o c i e t y,2021: 672-681.[13]C H E NCF,L IX M,Y A N GLB,e t a l.P r o g r e s s i v e s e m a n t i c-a w a r e s t y l e t r a n s f o r m a t i o n f o r b l i n d f a c e r e s t o r a t i o n[C]//P r o c e e d i n g s o f t h e I E E E/C V FC o n f e r e n c e o nC o m p u t e rV i s i o n a n dP a t t e r nR e c o g n i t i o n.V i r t u a l:I E E EC o m p u t e r S o c i-e t y,2021:11896-11905.[14]Y A N GLB,L I U C,WA N GP,e t a l.H i f a c e g a n:f a c e r e n o v a t i o nv i a c o l l a b o r a t i v e s u p p r e s s i o na n dr e p l e n i s h m e n t[C]//P r o c e e d i n g s o f t h e28t hA C MI n t e r n a t i o n a l C o n f e r e n c e o n M u l t i m e d i a.S e a t t l e:A s s o c i a t i o n f o rC o m p u t i n g M a c h i n e r y, 2020:1551-1560.D I -S t y l e G A N v 2M o d e l f o rD i g i t a l I m a geR e s t o r a t i o n WA N G K u n ,Z H A N G Y u e*(S c h o o l o fM a t h e m a t i c s a n dF i n a n c e ,A n h u i P o l y t e c h n i cU n i v e r s i t y ,W u h u241000,C h i n a )A b s t r a c t :W h e n t h e r e a r e l a r g e a r e a s o fm i s s i n g o r c o m p l e x t e x t u r e s t r u c t u r e s i n d i g i t a l i m a g e s ,t r a d i t i o n -a l i m a g e e n h a n c e m e n t t e c h n i q u e s c a n n o t r e s t o r em o r e r e q u i r e d i n f o r m a t i o n f r o mt h e l i m i t e d i n f o r m a t i o n i n t h e i m a g e ,r e s u l t i n g i n l i m i t e d e n h a n c e m e n t p e r f o r m a n c e .T h e r e f o r e ,t h i s p a p e r p r o p o s e s a d e e p l e a r n -i n g i n p a i n t i n g m e t h o d ,D I -S t y l e G A N v 2,w h i c hc o m b i n e sd e c o d i n g i n f o r m a t i o n (D I )w i t hS t y l e G A N v 2.F i r s t l y ,t h eU -n e tm o d u l e g e n e r a t e s ah i d d e n e n c o d i n g s i g n a l c o n t a i n i n g t h em a i n i n f o r m a t i o no f t h e i m -a g e a n d a d e c o d i n g s i g n a l c o n t a i n i n g t h e s e c o n d a r y i n f o r m a t i o n .T h e n ,t h e S t y l e G A N v 2m o d u l e i s u s e d t o i n t r o d u c e a n i m a g e g e n e r a t i o n p r i o r .D u r i n g t h e i m a g e g e n e r a t i o n p r o c e s s ,n o t o n l y t h eh i d d e ne n c o d i n gm a i n i n f o r m a t i o n i s u s e d ,b u t a l s o t h e d e c o d i n g s i g n a l c o n t a i n i n g t h e s e c o n d a r y i n f o r m a t i o no f t h e i m a g e i s i n t e g r a t e d ,t h e r e b y a c h i e v i n g s e m a n t i c e n h a n c e m e n t o f t h e r e p a i r r e s u l t s .T h e e x pe r i m e n t a l r e s u l t s o n F F H Qa n dC e l e b Ad a t a b a s e s v a l i d a t e t h e ef f e c t i v e n e s s o f t h e p r o p o s e da p p r o c h .K e y w o r d s :i m ag e r e s t o r a t i o n ;d e c o d e s i g n a l ;g e n e r a t i v e a d v e r s a r i a l n e t w o r k ;U -n e t ;S t y l e G A N v 2(上接第83页)P r o g r e s s i v e I n t e r p o l a t i o nL o o p S u b d i v i s i o n M e t h o d w i t hD u a lA d ju s t a b l eF a c t o r s S H IM i n g z h u ,L I U H u a y o n g*(S c h o o l o fM a t h e m a t i c s a n dP h y s i c s ,A n h u i J i a n z h uU n i v e r s i t y ,H e f e i 230601,C h i n a )A b s t r a c t :A i m i n g a t t h e p r o b l e mt h a t t h e l i m i ts u r f a c e p r o d u c e db y t h ea p p r o x i m a t eL o o p s u b d i v i s i o n m e t h o d t e n d s t o s a g a n d s h r i n k ,a p r o g r e s s i v e i n t e r p o l a t i o nL o o p s u b d i v i s i o nm e t h o dw i t hd u a l a d j u s t a -b l e f a c t o r s i s p r o p o s e d .T h i sm e t h o d i n t r o d u c e s d i f f e r e n t a d j u s t a b l e f a c t o r s i n t h e t w o -p h a s eL o o p s u b d i -v i s i o nm e t h o d a n d t h e p r o g r e s s i v e i t e r a t i o n p r o c e s s ,s o t h a t t h e g e n e r a t e d l i m i t s u r f a c e i s i n t e r p o l a t e d t o a l l t h e v e r t i c e s o f t h e i n i t i a l c o n t r o lm e s h .M e a n w h i l e ,i t h a s a s t r i n g e n c y ,l o c a l i t y a n d g l o b a l i t y .I t c a nn o t o n l y f l e x i b l y c o n t r o l t h e s h a p e o f l i m i t s u r f a c e ,b u t a l s o e x p a n d t h e c o n t r o l l a b l e r a n g e o f s h a p e t o a c e r -t a i ne x t e n t .F r o mt h e n u m e r i c a l e x p e r i m e n t s ,i t c a nb e s e e n t h a t t h em e t h o d c a n r e t a i n t h e c h a r a c t e r i s t i c s o f t h e i n i t i a l t r i a n g u l a rm e s hb e t t e rb y c h a n g i n g t h ev a l u eo f d u a l a d j u s t a b l e f a c t o r s ,a n d t h e g e n e r a t e d l i m i t s u r f a c eh a s a s m a l l d e g r e e o f s h r i n k a g e ,w h i c h p r o v e s t h em e t h o d t ob e f e a s i b l e a n d e f f e c t i v e .K e y w o r d s :L o o p s u b d i v i s i o n ;p r o g r e s s i v e i n t e r p o l a t i o n ;d u a l a d j u s t a b l e f a c t o r s ;a s t r i n g e n c y ㊃19㊃第6期王 坤,等:数字图像修复的D I -S t y l e G A N v 2模型。
0-1损失边界松弛化推导

0-1损失边界松弛化推导
0-1损失边界松弛化(LBRC)是一种用于二分类问题的损失函数,其可以将决策边界松弛到0-1损失边界以外的区域,从而提高模型的鲁棒性。
下面是LBRC的推导过程:
假设原始损失函数为J(W),其中W为模型参数向量。
LBRC损失函数为J LBRC(W),它由原始损失函数的梯度和0-1损失函数的平均值构成,即:
其中,m表示样本数量,n i表示第i个样本的特征向量维度,y ij表示第i个样本第j个特征的取值(0或1)。
现在,我们来推导LBRC损失函数的梯度。
首先,对于每个样本i,我们可以将LBRC损失函数写成以下形式:
这个近似式的误差是非常小的,因此我们可以将上式视为LBRC损失函数的梯度。
基于SVD和GMD的水下可见光通信研究

SVD 的预编码方案好。 同时,改变水下信道中的传播
距离、水下信道中的水质类型,验证这些参数对数据
通信传输质量的影响。
2. 1 验证所提方案的有效性
如图 3 和图 4 所示,在水下信道中分别设置对数
辐照方差 σ 2I = 0. 261 4 和 σ 2I = 2. 352 6,即分别验证强
Decomposition, SVD)的预编码方案会得到信噪比(Signal - to - Noise Ratio,SNR) 相差较大的子信道,为此
文章提出了一种基于 SVD 和几何均值分解( Geometric Mean Decomposition,GMD) 的预编码方案。 该
方案可以有效地抑制用户间干扰,得到 SNR 相同的子信道,并且消除复杂的比特分配过程,并通过仿
量高于基于双 SVD 的预编码方案。
图 5 水下信道下不同水质类型的比较
3 500 m 的时候仿真结果。 可看出随着通信距离的增
加,误码率也越来越高。
图 3 水下信道弱湍流的实验比较
图 6 水下信道不同传播距离的比较
图 4 水下信道下强湍流的实验比较
2. 2 改变信道参数的仿真结果
基于 SVD 和 GMD 的预编码方案在不同传播距
向量,从而可以得到 PEP 的表达式:
(
Γ‖R( x i - x j ) ‖2F
)
(9)
4
其中,Γ 是信噪比, Q(·) 是误差互补函数。
在得到每个符号的条件成对错误概率之后,利用
遍历搜索方法得到最小欧氏距离 d imin = min‖R( x i PEP( x i → x j R) = Q
梯度下降 收敛证明-解释说明
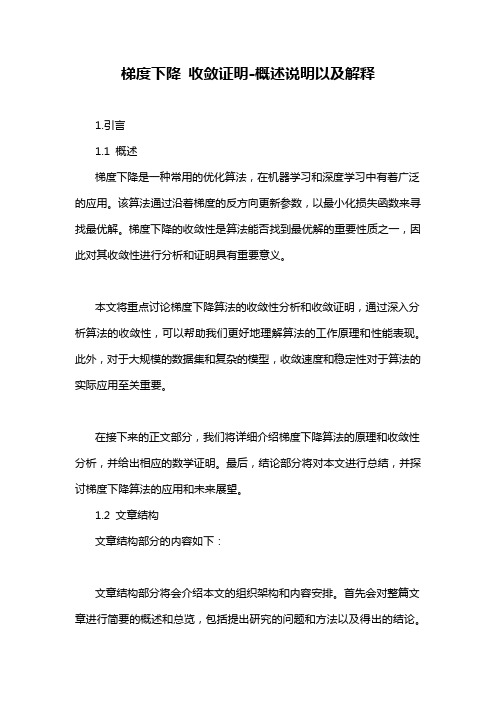
梯度下降收敛证明-概述说明以及解释1.引言1.1 概述梯度下降是一种常用的优化算法,在机器学习和深度学习中有着广泛的应用。
该算法通过沿着梯度的反方向更新参数,以最小化损失函数来寻找最优解。
梯度下降的收敛性是算法能否找到最优解的重要性质之一,因此对其收敛性进行分析和证明具有重要意义。
本文将重点讨论梯度下降算法的收敛性分析和收敛证明,通过深入分析算法的收敛性,可以帮助我们更好地理解算法的工作原理和性能表现。
此外,对于大规模的数据集和复杂的模型,收敛速度和稳定性对于算法的实际应用至关重要。
在接下来的正文部分,我们将详细介绍梯度下降算法的原理和收敛性分析,并给出相应的数学证明。
最后,结论部分将对本文进行总结,并探讨梯度下降算法的应用和未来展望。
1.2 文章结构文章结构部分的内容如下:文章结构部分将会介绍本文的组织架构和内容安排。
首先会对整篇文章进行简要的概述和总览,包括提出研究的问题和方法以及得出的结论。
然后对本文的具体结构进行介绍,包括各个章节的主题和内容概要,以及章节之间的逻辑关系和衔接。
最后,会阐明本文的目的和意义,以及对读者的预期效果和帮助。
通过文章结构部分的介绍,读者可以快速了解本文的框架和内容,对整篇文章有一个清晰的预期和理解。
1.3 目的本文的目的是对梯度下降算法的收敛性进行深入分析和证明。
通过对梯度下降算法的原理和数学推导进行全面阐述,旨在揭示该算法在迭代优化过程中的收敛规律和特性。
通过本文的研究,读者将能够更加深入地理解梯度下降算法在解决优化问题时的收敛机制,为算法的应用和改进提供理论支持。
同时,本文也将探讨梯度下降算法在实际应用中的限制和局限性,为进一步的研究提供发展方向。
2.正文2.1 梯度下降算法梯度下降算法是一种常用的优化方法,用于找到函数的局部最小值。
其基本原理是通过不断沿着负梯度方向调整参数,使得函数值逐渐减小,最终达到最优解。
具体来说,对于一个函数f(x),我们希望找到使得f(x)取得最小值的参数x*。
- 1、下载文档前请自行甄别文档内容的完整性,平台不提供额外的编辑、内容补充、找答案等附加服务。
- 2、"仅部分预览"的文档,不可在线预览部分如存在完整性等问题,可反馈申请退款(可完整预览的文档不适用该条件!)。
- 3、如文档侵犯您的权益,请联系客服反馈,我们会尽快为您处理(人工客服工作时间:9:00-18:30)。
I.L Tail-Biting Codes (CTBC) can overcome the loss on the code rate, and induces less performance degradation [2], [3]. In the trellis of CTBC, there is a one-to-one correspondence between a codeword and a path with the same initial and final state, which is called a tail-biting path.1 If the number of initial states (equivalently, final states) of the convolutional tail-biting code is Ns , the trellis is composed of Ns subtrellises with the same initial and final state. These subtrellises are called tail-biting subtrellises, or simply subtrellises, and will be denoted by Ti for the ith subtrellis. Several suboptimal decoding algorithms for the CTBC have been proposed [2], [4]. Among them, the wrap-around Viterbi algorithm (WAVA) is the one with the least decoding complexity [2]. A straightforward optimal decoding algorithm for the CTBC codes is to perform the Viterbi algorithm on all of the tailbiting subtrellises; however, such approach may be impractical due to its high computational complexity. Recently, an ML decoding algorithm of practical decoding complexity has been proposed [1]. This scheme has two phases. In the first phase, the Viterbi algorithm (VA) is applied to the trellis of the convolutional tail-biting code to obtain the trellis information. Based upon the trellis information, the algorithm A* is then performed on all subtrellises in the second phase to yield the
IEEE COMMUNICATIONS LETTERS, VOL. 12, NO. 12, DECEMBER 2008
883
Low-Complexity ML Decoding for Convolutional Tail-Biting Codes
Hung-Ta Pai, Member, IEEE, Yunghsiang S. Han, Senior Member, IEEE, Ting-Yi Wu, Po-Ning Chen, Senior Member, IEEE, and Shin-Lin Shieh
Abstract—Recently, a maximum-likelihood (ML) decoding algorithm with two phases has been proposed for convolutional tailbiting codes [1]. The first phase applies the Viterbi algorithm to obtain the trellis information, and then the second phase employs the algorithm A* to find the ML solution. In this work, we improve the complexity of the algorithm A* by using a new evaluation function. Simulations showed that the improved A* algorithm has over 5 times less average decoding complexity in the second phase when Eb /N0 ≥ 4 dB. Index Terms—Viterbi algorithm, maximum-likelihood, tailbiting codes, algorithm A*.
(i) (i) n−1) (i) n−1 j =0 (i) (i) (i) (i)
ML decision. It has been shown that the decoding complexity can be reduced from Ns VA trials to equivalently 1.3 VA trials without sacrificing the optimality in performance. In this work, an improved algorithm A* with a new evaluation function is presented. Simulations showed that the complexity in the second phase can be further reduced down to 1/5 of the original scheme at medium-to-high signal to noise ratio (SNR). II. M AXIMUM -L IKELIHOOD D ECODING OF THE CTBC U SING I MPROVED A LGORITHM A* Let ∼ C be an (n, 1, m) convolutional tail-biting code of L information bits, where n is the number of output bits per information bit, and m is the memory order. Hence, the trellis of ∼ C has Ns = 2m states at each level, and is of L + 1 levels. As aforementioned, the corresponding tail-biting paths for codewords of ∼ C should constrain on the same initial and final state. By relaxing such constraint, we denote the super code of ∼ C, which consists of all paths that may end at a final state different from the initial state, by ∼ Cs . Denote by v (v0 , v1 , . . . , vN −1 ) ∈ {0, 1}N the binary codeword of ∼ C, where N = nL. Define the hard-decision sequence y = (y0 , y1 , . . ., yN −1 ) corresponding to the received vector r = (r0 , r1 , . . ., rN −1 ) as yj 1, if φj < 0; 0, otherwise,
where φj ln[Pr(rj |0)/ Pr(rj |1)]. Then, it can be derived by the Wagner rule that the ML decoding output v ∗ for received vector r satisfies
N −1 j =0 ∗ (vj ⊕ yj )|φj | ≤ N −1
(vj ⊕ yj )|φj | for all v ∈ ∼ C,
j =0
where “⊕” is the exclusive-or operation. We thereby define a new metric for the path in a subtrellis as follows. (i) Definition 1: For a path with zero-one labels x( n−1) = (x0 , x1 , . . . , x n−1 ), which ends at level define the metric associated with it as M x(
Manuscript received December 27, 2007. The associate editor coordinating the review of this letter and approving it for publication was R. Nabar. This work was supported by the National Science Council, Taiwan, R.O.C., under the project No. NSC 96-2628-E-305-001 and NSC 96-2221-E-305-003. H.-T. Pai, Y. S. Han, and T.-Y. Wu are with the Graduate Institute of Communication Engineering, National Taipei University (e-mail: {htpai, yshan}@.tw, mavericktywu@). P.-N. Chen is with the Dept. of Communications Engineering, National Chiao Tung University (e-mail: qponing@.tw). S.-L. Shieh is with Sunplus mMobile Inc., Hsinchu 300, Taiwan, R.O.C., and also the Dept. of Communications Engineering, National Chiao Tung University (e-mail: shinlinshieh@). Digital Object Identifier 10.1109/LCOMM.2008.072181. 1 Hence, “tail-biting paths” and “codewords” are interchangeably used in this work.