Arc-consistency for non-binary dynamic CSPs
人工智能英文参考文献(最新120个)
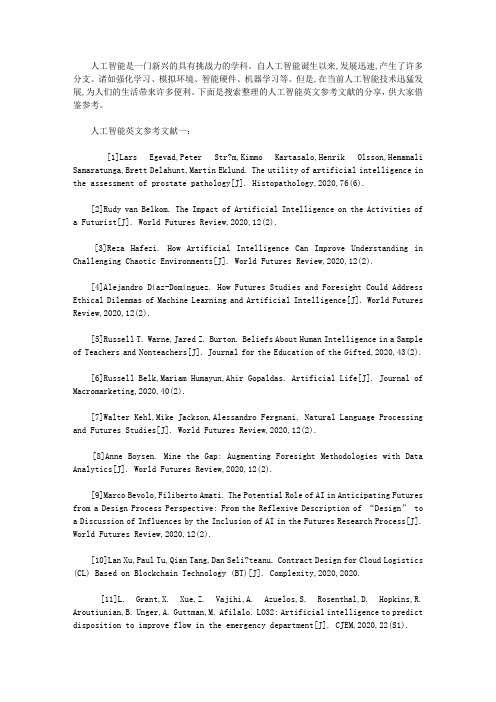
人工智能是一门新兴的具有挑战力的学科。
自人工智能诞生以来,发展迅速,产生了许多分支。
诸如强化学习、模拟环境、智能硬件、机器学习等。
但是,在当前人工智能技术迅猛发展,为人们的生活带来许多便利。
下面是搜索整理的人工智能英文参考文献的分享,供大家借鉴参考。
人工智能英文参考文献一:[1]Lars Egevad,Peter Str?m,Kimmo Kartasalo,Henrik Olsson,Hemamali Samaratunga,Brett Delahunt,Martin Eklund. The utility of artificial intelligence in the assessment of prostate pathology[J]. Histopathology,2020,76(6).[2]Rudy van Belkom. The Impact of Artificial Intelligence on the Activities ofa Futurist[J]. World Futures Review,2020,12(2).[3]Reza Hafezi. How Artificial Intelligence Can Improve Understanding in Challenging Chaotic Environments[J]. World Futures Review,2020,12(2).[4]Alejandro Díaz-Domínguez. How Futures Studies and Foresight Could Address Ethical Dilemmas of Machine Learning and Artificial Intelligence[J]. World Futures Review,2020,12(2).[5]Russell T. Warne,Jared Z. Burton. Beliefs About Human Intelligence in a Sample of Teachers and Nonteachers[J]. Journal for the Education of the Gifted,2020,43(2).[6]Russell Belk,Mariam Humayun,Ahir Gopaldas. Artificial Life[J]. Journal of Macromarketing,2020,40(2).[7]Walter Kehl,Mike Jackson,Alessandro Fergnani. Natural Language Processing and Futures Studies[J]. World Futures Review,2020,12(2).[8]Anne Boysen. Mine the Gap: Augmenting Foresight Methodologies with Data Analytics[J]. World Futures Review,2020,12(2).[9]Marco Bevolo,Filiberto Amati. The Potential Role of AI in Anticipating Futures from a Design Process Perspective: From the Reflexive Description of “Design” to a Discussion of Influences by the Inclusion of AI in the Futures Research Process[J]. World Futures Review,2020,12(2).[10]Lan Xu,Paul Tu,Qian Tang,Dan Seli?teanu. Contract Design for Cloud Logistics (CL) Based on Blockchain Technology (BT)[J]. Complexity,2020,2020.[11]L. Grant,X. Xue,Z. Vajihi,A. Azuelos,S. Rosenthal,D. Hopkins,R. Aroutiunian,B. Unger,A. Guttman,M. Afilalo. LO32: Artificial intelligence to predict disposition to improve flow in the emergency department[J]. CJEM,2020,22(S1).[12]A. Kirubarajan,A. Taher,S. Khan,S. Masood. P071: Artificial intelligence in emergency medicine: A scoping review[J]. CJEM,2020,22(S1).[13]L. Grant,P. Joo,B. Eng,A. Carrington,M. Nemnom,V. Thiruganasambandamoorthy. LO22: Risk-stratification of emergency department syncope by artificial intelligence using machine learning: human, statistics or machine[J]. CJEM,2020,22(S1).[14]Riva Giuseppe,Riva Eleonora. OS for Ind Robots: Manufacturing Robots Get Smarter Thanks to Artificial Intelligence.[J]. Cyberpsychology, behavior and social networking,2020,23(5).[15]Markus M. Obmann,Aurelio Cosentino,Joshy Cyriac,Verena Hofmann,Bram Stieltjes,Daniel T. Boll,Benjamin M. Yeh,Matthias R. Benz. Quantitative enhancement thresholds and machine learning algorithms for the evaluation of renal lesions using single-phase split-filter dual-energy CT[J]. Abdominal Radiology,2020,45(1).[16]Haytham H. Elmousalami,Mahmoud Elaskary. Drilling stuck pipe classification and mitigation in the Gulf of Suez oil fields using artificial intelligence[J]. Journal of Petroleum Exploration and Production Technology,2020,10(10).[17]Rüdiger Schulz-Wendtland,Karin Bock. Bildgebung in der Mammadiagnostik –Ein Ausblick <trans-title xml:lang="en">Imaging in breast diagnostics—an outlook [J]. Der Gyn?kologe,2020,53(6).</trans-title>[18]Nowakowski Piotr,Szwarc Krzysztof,Boryczka Urszula. Combining an artificial intelligence algorithm and a novel vehicle for sustainable e-waste collection[J]. Science of the Total Environment,2020,730.[19]Wang Huaizhi,Liu Yangyang,Zhou Bin,Li Canbing,Cao Guangzhong,Voropai Nikolai,Barakhtenko Evgeny. Taxonomy research of artificial intelligence for deterministic solar power forecasting[J]. Energy Conversion and Management,2020,214.[20]Kagemoto Hiroshi. Forecasting a water-surface wave train with artificial intelligence- A case study[J]. Ocean Engineering,2020,207.[21]Tomonori Aoki,Atsuo Yamada,Kazuharu Aoyama,Hiroaki Saito,Gota Fujisawa,Nariaki Odawara,Ryo Kondo,Akiyoshi Tsuboi,Rei Ishibashi,Ayako Nakada,Ryota Niikura,Mitsuhiro Fujishiro,Shiro Oka,Soichiro Ishihara,Tomoki Matsuda,Masato Nakahori,Shinji Tanaka,Kazuhiko Koike,Tomohiro Tada. Clinical usefulness of a deep learning‐based system as the first screening on small‐bowel capsule endoscopy reading[J]. Digestive Endoscopy,2020,32(4).[22]Masashi Fujii,Hajime Isomoto. Next generation of endoscopy: Harmony with artificial intelligence and robotic‐assisted devices[J]. Digestive Endoscopy,2020,32(4).[23]Roberto Verganti,Luca Vendraminelli,Marco Iansiti. Innovation and Design in the Age of Artificial Intelligence[J]. Journal of Product Innovation Management,2020,37(3).[24]Yuval Elbaz,David Furman,Maytal Caspary Toroker. Modeling Diffusion in Functional Materials: From Density Functional Theory to Artificial Intelligence[J]. Advanced Functional Materials,2020,30(18).[25]Dinesh Visva Gunasekeran,Tien Yin Wong. Artificial Intelligence in Ophthalmology in 2020: A Technology on the Cusp for Translation and Implementation[J]. Asia-Pacific Journal of Ophthalmology,2020,9(2).[26]Fu-Neng Jiang,Li-Jun Dai,Yong-Ding Wu,Sheng-Bang Yang,Yu-Xiang Liang,Xin Zhang,Cui-Yun Zou,Ren-Qiang He,Xiao-Ming Xu,Wei-De Zhong. The study of multiple diagnosis models of human prostate cancer based on Taylor database by artificial neural networks[J]. Journal of the Chinese Medical Association,2020,83(5).[27]Matheus Calil Faleiros,Marcello Henrique Nogueira-Barbosa,Vitor Faeda Dalto,JoséRaniery Ferreira Júnior,Ariane Priscilla Magalh?es Tenório,Rodrigo Luppino-Assad,Paulo Louzada-Junior,Rangaraj Mandayam Rangayyan,Paulo Mazzoncini de Azevedo-Marques. Machine learning techniques for computer-aided classification of active inflammatory sacroiliitis in magnetic resonance imaging[J]. Advances in Rheumatology,2020,60(1078).[28]Balamurugan Balakreshnan,Grant Richards,Gaurav Nanda,Huachao Mao,Ragu Athinarayanan,Joseph Zaccaria. PPE Compliance Detection using Artificial Intelligence in Learning Factories[J]. Procedia Manufacturing,2020,45.[29]M. Stévenin,V. Avisse,N. Ducarme,A. de Broca. Qui est responsable si un robot autonome vient à entra?ner un dommage ?[J]. Ethique et Santé,2020.[30]Fatemeh Barzegari Banadkooki,Mohammad Ehteram,Fatemeh Panahi,Saad Sh. Sammen,Faridah Binti Othman,Ahmed EL-Shafie. Estimation of Total Dissolved Solids (TDS) using New Hybrid Machine Learning Models[J]. Journal of Hydrology,2020.[31]Adam J. Schwartz,Henry D. Clarke,Mark J. Spangehl,Joshua S. Bingham,DavidA. Etzioni,Matthew R. Neville. Can a Convolutional Neural Network Classify Knee Osteoarthritis on Plain Radiographs as Accurately as Fellowship-Trained Knee Arthroplasty Surgeons?[J]. The Journal of Arthroplasty,2020.[32]Ivana Nizetic Kosovic,Toni Mastelic,Damir Ivankovic. Using Artificial Intelligence on environmental data from Internet of Things for estimating solar radiation: Comprehensive analysis[J]. Journal of Cleaner Production,2020.[33]Lauren Fried,Andrea Tan,Shirin Bajaj,Tracey N. Liebman,David Polsky,Jennifer A. Stein. Technological advances for the detection of melanoma: Part I. Advances in diagnostic techniques[J]. Journal of the American Academy of Dermatology,2020.[34]Mohammed Amoon,Torki Altameem,Ayman Altameem. Internet of things Sensor Assisted Security and Quality Analysis for Health Care Data Sets Using Artificial Intelligent Based Heuristic Health Management System[J]. Measurement,2020.[35]E. Lotan,C. Tschider,D.K. Sodickson,A. Caplan,M. Bruno,B. Zhang,Yvonne W. Lui. Medical Imaging and Privacy in the Era of Artificial Intelligence: Myth, Fallacy, and the Future[J]. Journal of the American College of Radiology,2020.[36]Fabien Lareyre,Cédric Adam,Marion Carrier,Juliette Raffort. Artificial Intelligence in Vascular Surgery: moving from Big Data to Smart Data[J]. Annals of Vascular Surgery,2020.[37]Ilesanmi Daniyan,Khumbulani Mpofu,Moses Oyesola,Boitumelo Ramatsetse,Adefemi Adeodu. Artificial intelligence for predictive maintenance in the railcar learning factories[J]. Procedia Manufacturing,2020,45.[38]Janet L. McCauley,Anthony E. Swartz. Reframing Telehealth[J]. Obstetrics and Gynecology Clinics of North America,2020.[39]Jean-Emmanuel Bibault,Lei Xing. Screening for chronic obstructive pulmonary disease with artificial intelligence[J]. The Lancet Digital Health,2020,2(5).[40]Andrea Laghi. Cautions about radiologic diagnosis of COVID-19 infection driven by artificial intelligence[J]. The Lancet Digital Health,2020,2(5).人工智能英文参考文献二:[41]K. Orhan,I. S. Bayrakdar,M. Ezhov,A. Kravtsov,T. ?zyürek. Evaluation of artificial intelligence for detecting periapical pathosis on cone‐beam computed tomography scans[J]. International Endodontic Journal,2020,53(5).[42]Avila A M,Mezi? I. Data-driven analysis and forecasting of highway traffic dynamics.[J]. Nature communications,2020,11(1).[43]Neri Emanuele,Miele Vittorio,Coppola Francesca,Grassi Roberto. Use of CT andartificial intelligence in suspected or COVID-19 positive patients: statement of the Italian Society of Medical and Interventional Radiology.[J]. La Radiologia medica,2020.[44]Tau Noam,Stundzia Audrius,Yasufuku Kazuhiro,Hussey Douglas,Metser Ur. Convolutional Neural Networks in Predicting Nodal and Distant Metastatic Potential of Newly Diagnosed Non-Small Cell Lung Cancer on FDG PET Images.[J]. AJR. American journal of roentgenology,2020.[45]Coppola Francesca,Faggioni Lorenzo,Regge Daniele,Giovagnoni Andrea,Golfieri Rita,Bibbolino Corrado,Miele Vittorio,Neri Emanuele,Grassi Roberto. Artificial intelligence: radiologists' expectations and opinions gleaned from a nationwide online survey.[J]. La Radiologia medica,2020.[46]?. ? ? ? ? [J]. ,2020,25(4).[47]Savage Rock H,van Assen Marly,Martin Simon S,Sahbaee Pooyan,Griffith Lewis P,Giovagnoli Dante,Sperl Jonathan I,Hopfgartner Christian,K?rgel Rainer,Schoepf U Joseph. Utilizing Artificial Intelligence to Determine Bone Mineral Density Via Chest Computed Tomography.[J]. Journal of thoracic imaging,2020,35 Suppl 1.[48]Brzezicki Maksymilian A,Bridger Nicholas E,Kobeti? Matthew D,Ostrowski Maciej,Grabowski Waldemar,Gill Simran S,Neumann Sandra. Artificial intelligence outperforms human students in conducting neurosurgical audits.[J]. Clinical neurology and neurosurgery,2020,192.[49]Lockhart Mark E,Smith Andrew D. Fatty Liver Disease: Artificial Intelligence Takes on the Challenge.[J]. Radiology,2020,295(2).[50]Wood Edward H,Korot Edward,Storey Philip P,Muscat Stephanie,Williams George A,Drenser Kimberly A. The retina revolution: signaling pathway therapies, genetic therapies, mitochondrial therapies, artificial intelligence.[J]. Current opinion in ophthalmology,2020,31(3).[51]Ho Dean,Quake Stephen R,McCabe Edward R B,Chng Wee Joo,Chow Edward K,Ding Xianting,Gelb Bruce D,Ginsburg Geoffrey S,Hassenstab Jason,Ho Chih-Ming,Mobley William C,Nolan Garry P,Rosen Steven T,Tan Patrick,Yen Yun,Zarrinpar Ali. Enabling Technologies for Personalized and Precision Medicine.[J]. Trends in biotechnology,2020,38(5).[52]Fischer Andreas M,Varga-Szemes Akos,van Assen Marly,Griffith L Parkwood,Sahbaee Pooyan,Sperl Jonathan I,Nance John W,Schoepf U Joseph. Comparison of Artificial Intelligence-Based Fully Automatic Chest CT Emphysema Quantification to Pulmonary Function Testing.[J]. AJR. American journal ofroentgenology,2020,214(5).[53]Moore William,Ko Jane,Gozansky Elliott. Artificial Intelligence Pertaining to Cardiothoracic Imaging and Patient Care: Beyond Image Interpretation.[J]. Journal of thoracic imaging,2020,35(3).[54]Hwang Eui Jin,Park Chang Min. Clinical Implementation of Deep Learning in Thoracic Radiology: Potential Applications and Challenges.[J]. Korean journal of radiology,2020,21(5).[55]Mateen Bilal A,David Anna L,Denaxas Spiros. Electronic Health Records to Predict Gestational Diabetes Risk.[J]. Trends in pharmacological sciences,2020,41(5).[56]Yao Xiang,Mao Ling,Lv Shunli,Ren Zhenghong,Li Wentao,Ren Ke. CT radiomics features as a diagnostic tool for classifying basal ganglia infarction onset time.[J]. Journal of the neurological sciences,2020,412.[57]van Assen Marly,Banerjee Imon,De Cecco Carlo N. Beyond the Artificial Intelligence Hype: What Lies Behind the Algorithms and What We Can Achieve.[J]. Journal of thoracic imaging,2020,35 Suppl 1.[58]Guzik Tomasz J,Fuster Valentin. Leaders in Cardiovascular Research: Valentin Fuster.[J]. Cardiovascular research,2020,116(6).[59]Fischer Andreas M,Eid Marwen,De Cecco Carlo N,Gulsun Mehmet A,van Assen Marly,Nance John W,Sahbaee Pooyan,De Santis Domenico,Bauer Maximilian J,Jacobs Brian E,Varga-Szemes Akos,Kabakus Ismail M,Sharma Puneet,Jackson Logan J,Schoepf U Joseph. Accuracy of an Artificial Intelligence Deep Learning Algorithm Implementing a Recurrent Neural Network With Long Short-term Memory for the Automated Detection of Calcified Plaques From Coronary Computed Tomography Angiography.[J]. Journal of thoracic imaging,2020,35 Suppl 1.[60]Ghosh Adarsh,Kandasamy Devasenathipathy. Interpretable Artificial Intelligence: Why and When.[J]. AJR. American journal of roentgenology,2020,214(5).[61]M.Rosario González-Rodríguez,M.Carmen Díaz-Fernández,Carmen Pacheco Gómez. Facial-expression recognition: An emergent approach to the measurement of tourist satisfaction through emotions[J]. Telematics and Informatics,2020,51.[62]Ru-Xi Ding,Iván Palomares,Xueqing Wang,Guo-Rui Yang,Bingsheng Liu,Yucheng Dong,Enrique Herrera-Viedma,Francisco Herrera. Large-Scale decision-making: Characterization, taxonomy, challenges and future directions from an Artificial Intelligence and applications perspective[J]. Information Fusion,2020,59.[63]Abdulrhman H. Al-Jebrni,Brendan Chwyl,Xiao Yu Wang,Alexander Wong,Bechara J. Saab. AI-enabled remote and objective quantification of stress at scale[J]. Biomedical Signal Processing and Control,2020,59.[64]Gillian Thomas,Elizabeth Eisenhauer,Robert G. Bristow,Cai Grau,Coen Hurkmans,Piet Ost,Matthias Guckenberger,Eric Deutsch,Denis Lacombe,Damien C. Weber. The European Organisation for Research and Treatment of Cancer, State of Science in radiation oncology and priorities for clinical trials meeting report[J]. European Journal of Cancer,2020,131.[65]Muhammad Asif. Are QM models aligned with Industry 4.0? A perspective on current practices[J]. Journal of Cleaner Production,2020,258.[66]Siva Teja Kakileti,Himanshu J. Madhu,Geetha Manjunath,Leonard Wee,Andre Dekker,Sudhakar Sampangi. Personalized risk prediction for breast cancer pre-screening using artificial intelligence and thermal radiomics[J]. Artificial Intelligence In Medicine,2020,105.[67]. Evaluation of Payer Budget Impact Associated with the Use of Artificial Intelligence in Vitro Diagnostic, Kidneyintelx, to Modify DKD Progression:[J]. American Journal of Kidney Diseases,2020,75(5).[68]Rohit Nishant,Mike Kennedy,Jacqueline Corbett. Artificial intelligence for sustainability: Challenges, opportunities, and a research agenda[J]. International Journal of Information Management,2020,53.[69]Hoang Nguyen,Xuan-Nam Bui. Soft computing models for predicting blast-induced air over-pressure: A novel artificial intelligence approach[J]. Applied Soft Computing Journal,2020,92.[70]Benjamin S. Hopkins,Aditya Mazmudar,Conor Driscoll,Mark Svet,Jack Goergen,Max Kelsten,Nathan A. Shlobin,Kartik Kesavabhotla,Zachary A Smith,Nader S Dahdaleh. Using artificial intelligence (AI) to predict postoperative surgical site infection: A retrospective cohort of 4046 posterior spinal fusions[J]. Clinical Neurology and Neurosurgery,2020,192.[71]Mei Yang,Runze Zhou,Xiangjun Qiu,Xiangfei Feng,Jian Sun,Qunshan Wang,Qiufen Lu,Pengpai Zhang,Bo Liu,Wei Li,Mu Chen,Yan Zhao,Binfeng Mo,Xin Zhou,Xi Zhang,Yingxue Hua,Jin Guo,Fangfang Bi,Yajun Cao,Feng Ling,Shengming Shi,Yi-Gang Li. Artificial intelligence-assisted analysis on the association between exposure to ambient fine particulate matter and incidence of arrhythmias in outpatients of Shanghai community hospitals[J]. Environment International,2020,139.[72]Fatemehalsadat Madaeni,Rachid Lhissou,Karem Chokmani,Sebastien Raymond,Yves Gauthier. Ice jam formation, breakup and prediction methods based on hydroclimatic data using artificial intelligence: A review[J]. Cold Regions Science and Technology,2020,174.[73]Steve Chukwuebuka Arum,David Grace,Paul Daniel Mitchell. A review of wireless communication using high-altitude platforms for extended coverage and capacity[J]. Computer Communications,2020,157.[74]Yong-Hong Kuo,Nicholas B. Chan,Janny M.Y. Leung,Helen Meng,Anthony Man-Cho So,Kelvin K.F. Tsoi,Colin A. Graham. An Integrated Approach of Machine Learning and Systems Thinking for Waiting Time Prediction in an Emergency Department[J]. International Journal of Medical Informatics,2020,139.[75]Matteo Terzi,Gian Antonio Susto,Pratik Chaudhari. Directional adversarial training for cost sensitive deep learning classification applications[J]. Engineering Applications of Artificial Intelligence,2020,91.[76]Arman Kilic. Artificial Intelligence and Machine Learning in Cardiovascular Health Care[J]. The Annals of Thoracic Surgery,2020,109(5).[77]Hossein Azarmdel,Ahmad Jahanbakhshi,Seyed Saeid Mohtasebi,Alfredo Rosado Mu?oz. Evaluation of image processing technique as an expert system in mulberry fruit grading based on ripeness level using artificial neural networks (ANNs) and support vector machine (SVM)[J]. Postharvest Biology and Technology,2020,166.[78]Wafaa Wardah,Abdollah Dehzangi,Ghazaleh Taherzadeh,Mahmood A. Rashid,M.G.M. Khan,Tatsuhiko Tsunoda,Alok Sharma. Predicting protein-peptide binding sites with a deep convolutional neural network[J]. Journal of Theoretical Biology,2020,496.[79]Francisco F.X. Vasconcelos,Róger M. Sarmento,Pedro P. Rebou?as Filho,Victor Hugo C. de Albuquerque. Artificial intelligence techniques empowered edge-cloud architecture for brain CT image analysis[J]. Engineering Applications of Artificial Intelligence,2020,91.[80]Masaaki Konishi. Bioethanol production estimated from volatile compositions in hydrolysates of lignocellulosic biomass by deep learning[J]. Journal of Bioscience and Bioengineering,2020,129(6).人工智能英文参考文献三:[81]J. Kwon,K. Kim. Artificial Intelligence for Early Prediction of Pulmonary Hypertension Using Electrocardiography[J]. Journal of Heart and Lung Transplantation,2020,39(4).[82]C. Maathuis,W. Pieters,J. van den Berg. Decision support model for effects estimation and proportionality assessment for targeting in cyber operations[J]. Defence Technology,2020.[83]Samer Ellahham. Artificial Intelligence in Diabetes Care[J]. The American Journal of Medicine,2020.[84]Yi-Ting Hsieh,Lee-Ming Chuang,Yi-Der Jiang,Tien-Jyun Chang,Chung-May Yang,Chang-Hao Yang,Li-Wei Chan,Tzu-Yun Kao,Ta-Ching Chen,Hsuan-Chieh Lin,Chin-Han Tsai,Mingke Chen. Application of deep learning image assessment software VeriSee? for diabetic retinopathy screening[J]. Journal of the Formosan Medical Association,2020.[85]Emre ARTUN,Burak KULGA. Selection of candidate wells for re-fracturing in tight gas sand reservoirs using fuzzy inference[J]. Petroleum Exploration and Development Online,2020,47(2).[86]Alberto Arenal,Cristina Armu?a,Claudio Feijoo,Sergio Ramos,Zimu Xu,Ana Moreno. Innovation ecosystems theory revisited: The case of artificial intelligence in China[J]. Telecommunications Policy,2020.[87]T. Som,M. Dwivedi,C. Dubey,A. Sharma. Parametric Studies on Artificial Intelligence Techniques for Battery SOC Management and Optimization of Renewable Power[J]. Procedia Computer Science,2020,167.[88]Bushra Kidwai,Nadesh RK. Design and Development of Diagnostic Chabot for supporting Primary Health Care Systems[J]. Procedia Computer Science,2020,167.[89]Asl? Bozda?,Ye?im Dokuz,?znur Begüm G?k?ek. Spatial prediction of PM 10 concentration using machine learning algorithms in Ankara, Turkey[J]. Environmental Pollution,2020.[90]K.P. Smith,J.E. Kirby. Image analysis and artificial intelligence in infectious disease diagnostics[J]. Clinical Microbiology and Infection,2020.[91]Alklih Mohamad YOUSEF,Ghahfarokhi Payam KAVOUSI,Marwan ALNUAIMI,Yara ALATRACH. Predictive data analytics application for enhanced oil recovery in a mature field in the Middle East[J]. Petroleum Exploration and Development Online,2020,47(2).[92]Omer F. Ahmad,Danail Stoyanov,Laurence B. Lovat. Barriers and pitfalls for artificial intelligence in gastroenterology: Ethical and regulatory issues[J]. Techniques and Innovations in Gastrointestinal Endoscopy,2020,22(2).[93]Sanne A. Hoogenboom,Ulas Bagci,Michael B. Wallace. Artificial intelligence in gastroenterology. The current state of play and the potential. How will it affect our practice and when?[J]. Techniques and Innovations in Gastrointestinal Endoscopy,2020,22(2).[94]Douglas K. Rex. Can we do resect and discard with artificial intelligence-assisted colon polyp “optical biopsy?”[J]. Techniques and Innovations in Gastrointestinal Endoscopy,2020,22(2).[95]Neal Shahidi,Michael J. Bourke. Can artificial intelligence accurately diagnose endoscopically curable gastrointestinal cancers?[J]. Techniques and Innovations in Gastrointestinal Endoscopy,2020,22(2).[96]Michael Byrne. Artificial intelligence in gastroenterology[J]. Techniques and Innovations in Gastrointestinal Endoscopy,2020,22(2).[97]Piet C. de Groen. Using artificial intelligence to improve adequacy of inspection in gastrointestinal endoscopy[J]. Techniques and Innovations in Gastrointestinal Endoscopy,2020,22(2).[98]Robin Zachariah,Andrew Ninh,William Karnes. Artificial intelligence for colon polyp detection: Why should we embrace this?[J]. Techniques and Innovations in Gastrointestinal Endoscopy,2020,22(2).[99]Alexandra T. Greenhill,Bethany R. Edmunds. A primer of artificial intelligence in medicine[J]. Techniques and Innovations in Gastrointestinal Endoscopy,2020,22(2).[100]Tomohiro Tada,Toshiaki Hirasawa,Toshiyuki Yoshio. The role for artificial intelligence in evaluation of upper GI cancer[J]. Techniques and Innovations in Gastrointestinal Endoscopy,2020,22(2).[101]Yahui Jiang,Meng Yang,Shuhao Wang,Xiangchun Li,Yan Sun. Emerging role of deep learning‐based artificial intelligence in tumor pathology[J]. Cancer Communications,2020,40(4).[102]Kristopher D. Knott,Andreas Seraphim,Joao B. Augusto,Hui Xue,Liza Chacko,Nay Aung,Steffen E. Petersen,Jackie A. Cooper,Charlotte Manisty,Anish N. Bhuva,Tushar Kotecha,Christos V. Bourantas,Rhodri H. Davies,Louise A.E. Brown,Sven Plein,Marianna Fontana,Peter Kellman,James C. Moon. The Prognostic Significance of Quantitative Myocardial Perfusion: An Artificial Intelligence–Based Approach Using Perfusion Mapping[J]. Circulation,2020,141(16).[103]Muhammad Asad,Ahmed Moustafa,Takayuki Ito. FedOpt: Towards Communication Efficiency and Privacy Preservation in Federated Learning[J]. Applied Sciences,2020,10(8).[104]Wu Wenzhi,Zhang Yan,Wang Pu,Zhang Li,Wang Guixiang,Lei Guanghui,Xiao Qiang,Cao Xiaochen,Bian Yueran,Xie Simiao,Huang Fei,Luo Na,Zhang Jingyuan,Luo Mingyan. Psychological stress of medical staffs during outbreak of COVID-19 and adjustment strategy.[J]. Journal of medical virology,2020.[105]. Eyenuk Fulfills Contract for Artificial Intelligence Grading of Retinal Images[J]. Telecomworldwire,2020.[106]Kim Tae Woo,Duhachek Adam. Artificial Intelligence and Persuasion: A Construal-Level Account.[J]. Psychological science,2020,31(4).[107]McCall Becky. COVID-19 and artificial intelligence: protecting health-care workers and curbing the spread.[J]. The Lancet. Digital health,2020,2(4).[108]Alca?iz Mariano,Chicchi Giglioli Irene A,Sirera Marian,Minissi Eleonora,Abad Luis. [Autism spectrum disorder biomarkers based on biosignals, virtual reality and artificial intelligence].[J]. Medicina,2020,80 Suppl 2.[109]Cong Lei,Feng Wanbing,Yao Zhigang,Zhou Xiaoming,Xiao Wei. Deep Learning Model as a New Trend in Computer-aided Diagnosis of Tumor Pathology for Lung Cancer.[J]. Journal of Cancer,2020,11(12).[110]Wang Fengdan,Gu Xiao,Chen Shi,Liu Yongliang,Shen Qing,Pan Hui,Shi Lei,Jin Zhengyu. Artificial intelligence system can achieve comparable results to experts for bone age assessment of Chinese children with abnormal growth and development.[J]. PeerJ,2020,8.[111]Hu Wenmo,Yang Huayu,Xu Haifeng,Mao Yilei. Radiomics based on artificial intelligence in liver diseases: where we are?[J]. Gastroenterology report,2020,8(2).[112]Batayneh Wafa,Abdulhay Enas,Alothman Mohammad. Prediction of the performance of artificial neural networks in mapping sEMG to finger joint angles via signal pre-investigation techniques.[J]. Heliyon,2020,6(4).[113]Aydin Emrah,Türkmen ?nan Utku,Namli G?zde,?ztürk ?i?dem,Esen Ay?e B,Eray Y Nur,Ero?lu Egemen,Akova Fatih. A novel and simple machine learning algorithm for preoperative diagnosis of acute appendicitis in children.[J]. Pediatric surgery international,2020.[114]Ellahham Samer. Artificial Intelligence in Diabetes Care.[J]. The Americanjournal of medicine,2020.[115]David J. Winkel,Thomas J. Weikert,Hanns-Christian Breit,Guillaume Chabin,Eli Gibson,Tobias J. Heye,Dorin Comaniciu,Daniel T. Boll. Validation of a fully automated liver segmentation algorithm using multi-scale deep reinforcement learning and comparison versus manual segmentation[J]. European Journal of Radiology,2020,126.[116]Binjie Fu,Guoshu Wang,Mingyue Wu,Wangjia Li,Yineng Zheng,Zhigang Chu,Fajin Lv. Influence of CT effective dose and convolution kernel on the detection of pulmonary nodules in different artificial intelligence software systems: A phantom study[J]. European Journal of Radiology,2020,126.[117]Georgios N. Kouziokas. A new W-SVM kernel combining PSO-neural network transformed vector and Bayesian optimized SVM in GDP forecasting[J]. Engineering Applications of Artificial Intelligence,2020,92.[118]Qingsong Ruan,Zilin Wang,Yaping Zhou,Dayong Lv. A new investor sentiment indicator ( ISI ) based on artificial intelligence: A powerful return predictor in China[J]. Economic Modelling,2020,88.[119]Mohamed Abdel-Basset,Weiping Ding,Laila Abdel-Fatah. The fusion of Internet of Intelligent Things (IoIT) in remote diagnosis of obstructive Sleep Apnea: A survey and a new model[J]. Information Fusion,2020,61.[120]Federico Caobelli. Artificial intelligence in medical imaging: Game over for radiologists?[J]. European Journal of Radiology,2020,126.以上就是关于人工智能参考文献的分享,希望对你有所帮助。
Demons非刚性配准算法拓扑保持性的研究

第36卷第1期自动化学报Vol.36,No.1 2010年1月ACTA AUTOMATICA SINICA January,2010Demons非刚性配准算法拓扑保持性的研究林相波1,2邱天爽1阮素2NICOLIER Frédéric2摘要在基于配准的图像分割应用中,拓扑保持性是非刚性图像配准算法的一个重要约束.本文从矢量场特性出发,分析了Demons非刚性图像配准算法导致目标拓扑改变时变形场的特点.根据变形场特点与其雅可比行列式之间的关系,给出了校正该算法拓扑保持性的方法.实验表明,改进后的变形场具有了拓扑保持性.关键词非刚性配准,拓扑,雅可比行列式,矢量场分析DOI10.3724/SP.J.1004.2010.00179Research on the Topology Preservation of the Demons Non-rigid RegistrationAlgorithmLIN Xiang-Bo1,2QIU Tian-Shuang1RUAN Su2NICOLIER Frédéric2Abstract Topology preservation is an important constraint of image non-rigid registration algorithms in the applications of registration based image segmentation.In this paper,the defor-mationfield of the Demons non-rigid registration that make the object s topology change is analyzed according to the features of the vectorfield.An method that can enforce the algorithm s topology preservation is provided on the basis of relation between the deformationfield and its Jacobian.The experiment results show that the modified deformationfield is topology preserving. Key words Non-rigid registration,topology,Jacobian deter-minant,vectorfield analysis非刚性医学图像配准是近年来的一个研究热点,实现不同个体间的配准是其中的一个主题,它的一个重要应用是利用图谱提供的信息,分割不同个体的组织器官结构[1].图像配准是通过将模板图像变形为目标图像,实现两图像中相同结构间的良好对应,这种对应性用配准得到的空间变形场T 表达.设可以变形的模板图像为R,目标图像为S,则T表示从模板图像的支撑域ΩR向目标图像的支撑域ΩS变形的一个空间变换,即T:ΩR→ΩS.通常可以通过迭代方式求解能量最小化问题得到最优解T,其配准能量的一般表达式如下E(S,R◦T)=E dis(S,R◦T)+E reg(T)(1)其中E dis(S,R◦T)表示图像特征之间的匹配程度,可以直接采用图像的灰度信息[2],也可以采用图像的其他特征[3];收稿日期2008-10-06录用日期2009-04-13Manuscript received October6,2008;accepted April13,2009国家自然科学基金(30170259,30570475,60372081),教育部博士点基金项目(20050141025)资助Supported by National Natural Science Foundation of China (30170259,30570475,60372081),Ph.D.Programs Foundation of Ministry of Education of China(20050141025)1.大连理工大学电子与信息工程学院电子工程系大连1160242.兰斯大学特鲁瓦技术学院通用电气与计算机工程系100261.Department of Electronic Engineering,School of Electronic and Information Engineering,Dalian University of Technology,Dalian 116024,P.R.China2.Department of Electrical Engineering and Computer Engineering,University of Reims,10026,France E reg(T)是惩罚项,旨在保证得到的变形场满足某些必要的属性,如平滑性、正反变换的一致性、拓扑保持性等,其中要求变形场具有拓扑保持性在完全基于配准的图像分割中是非常重要的,因为从医学上讲,不同正常个体的同源结构虽大小不同,但具有同样的拓扑.变形场具有拓扑保持性意味着各结构在变形后的图像中仍保持内部的连接性和结构之间的邻接关系不变,不会发生撕裂或粘贴,不会有新的结构出现,原有的结构也不会消失.通过将这一约束加在变形场上,可以限制式(1)的最优解空间,使它符合真实材料的变形特性.判断给定的变形场是否具有拓扑保持性,一般是考察该变形场的雅可比行列式的值是否总是大于零[4].在3D情况下,变形场向量可以表示成T=(X,Y,Z),X,Y,Z分别表示点p(x,y,z)变形后的位置,则变形场在p点处的雅可比行列式可以表示成J T(p)=∂X∂x∂X∂y∂X∂z∂Y∂x∂Y∂y∂Y∂z∂Z∂x∂Z∂y∂Z∂z(2)也就是说,只有保证在任意点处J T(p)>0,所得到的变形场才具有拓扑保持性[5−8].由于在变形场迭代寻优过程中约束雅可比行列式的值大于零的计算代价太大,文献[9]通过对正反变换进行处理以保证变形场的双射属性,然后再进行平滑处理,力求其具有拓扑保持性.然而,研究表明,很难从理论上证明利用双射和平滑技术可以保证变形场具有拓扑不变性[7,10],实践中,我们也通过实验发现该算法得到的变形场并不总是具有拓扑不变属性.为此,本文从矢量场的特点出发,详细分析了该算法得到的变形场拓扑性质,并在此基础上给出了强化变形场拓扑保持性的方法.1算法简介文献[9]提出的Demons算法应用于医学图像领域时,常基于光流场理论求解其变形场.光流场理论的假设前提是图像在运动的过程中保持灰度不变,即I(x(t),y(t),z(t),t)=const(3) I表示图像的灰度,是像素点坐标的函数.对式(3)求微分得∂I∂x∂x∂t+∂I∂y∂y∂t+∂I∂z∂z∂t=−∂I∂t(4)若将待配准的两幅图像看成是单位时间间隔内同一目标的成像结果,则上式变为v·∇s=r−s(5)其中v=(∂x∂t,∂y∂t,∂z∂t),r和s分别表示图像R和S的灰度.经过近似处理,得到运动速度的表达式为v=(r−s)∇s[||∇s||2+(r−s)2](6)这个速度代表了变形网格点受到的作用力.其寻优过程如下:1)初始化变换矩阵T;180自动化学报36卷2)相似度函数寻优,得到源图像中每个点p 的位移矢量:u (p )=−(r ◦T (p )−s (p ))(r ◦T (p )−s (p ))2+||∇s (p )||2∇s (p )(7)3)对得到的位移矢量进行高斯平滑处理:u ←G σ∗u 4)T ←T +u .重复迭代,直到满足停止条件.2变形场分析图像配准得到的变形场是一个矢量场,它反映了两图像中相对应结构间的变化.为了更好地理解变形场所模拟的物理过程和其中隐含的拓扑信息,有必要对得到的矢量变形场进行特性分析.矢量场的特性可以用它的特征点来表征[11],其中最重要的几个特征点是源点、汇点和漩涡中心点,如图1所示.图1矢量场的主要特征点Fig.1The important attribute points of a vector field根据式(7)可知,位移矢量是对标量场求梯度得到的,而梯度场是无旋场,所以本文所用配准算法的特征点主要是源点和汇点.矢量场的特征点代表了与应用相关的不同的物理属性.以不同个体间脑部核磁共振图像的配准应用为例,源点表征了对应脑部器官的增大,而汇点则表示对应脑部器官的缩小,它与变形场雅可比行列式的值所表征的物理特性是相吻合的.J T >1表征了对应脑部器官的增大,J T <1表征了对应脑部器官的缩小,J T=1表征了物质既没有增加也没有减少,J T ≤0表示存在拓扑改变[7−8,12].如前所述,图像变形带来的拓扑改变主要表现在图像中的目标结构发生粘贴或撕裂,参考矢量场特征点的图示(图1),可见汇点处容易发生挤压粘贴和反折,源点处容易因膨胀过度而撕裂.2D 情况下常见的几种情况如图2所示.(a)反折粘贴(a)Fold(b)挤压粘贴(b)Overlap(c)撕裂(c)Tear图22D 空间中变形导致的拓扑改变示例Fig.2Examples of the changed topology in 2D space due tothe deformations图2中的编号1、2、3、4表示初始状态下一个矩形网格的四个顶点,粗实线表示变形后的网格,其顶点为1 、2 、3 、4 ,分别与初始状态下的四个顶点相对应,带箭头的虚线表示各顶点的变化路径.图2(a)表示网格变形后出现了反折粘贴,就像是一个平面不仅形状发生了变化,而且由正面翻扣过来变成了背面;图2(b)表示网格变形后出现了挤压粘贴,2、3两点在变形过程中有交叉现象,导致网格扭曲;图2(c)表示网格变形后向两侧膨胀的幅度过大,超过了两点间连线允许的拉伸强度而撕裂.5 和6 表示网格断裂后插入的新顶点.研究表明,Demons 算法得到的变形场有拓扑改变现象,在某些区域得到的雅可比行列式的值不为正.观察这些区域的变形场特点,可见到与图2示例相近的情况,如图3所示.图3(a)为可变的模板图像,图3(b)为目标图像,图3(c)为模板图像在Demons 变形场作用下变形得到的图像,可以看出,二者具有较高的相似度.上面的白斑表示变形场雅可比行列式的值不大于零的位置,意味着得到该结果的变形场在某些位置不具有拓扑保持性.我们选择了三个拓扑发生改变的区域1,2,3,在图3(c)中用白色矩形框标出.图3(d)∼(f)给出了这三个区域的变形场图示,可以看到在这些矢量场的特征点附近有交叉或反折现象.(a)模板图像(a)Template(b)目标图像(b)Target(c)变形后的模板图像(c)Deformed template(d)1区放大(d)Enlarged region 1(e)2区放大(e)Enlarged region 2(f)3区放大(f)Enlarged region 3图3原Demons 算法得到的变形场特点Fig.3The deformation field obtained from the originalDemons algorithm1期林相波等:Demons非刚性配准算法拓扑保持性的研究181从图2可以看出,变形时发生反折主要表现在沿变形方向上相邻点的位移幅度不匹配,后面的点位移后的位置超过前面的点位移后的位置.变形时发生交叉主要是在矢量场汇点附近,相邻的点位移方向相对,各点的位移幅度相对较大导致相互交叉.因此,既直观又简单的解决方案应是在尽量保持原有变形场几何特性的前提下合理地改变这些点处的位移,达到拓扑校正的目的.3增强变形场拓扑保持属性的方法若连续可微的变形场具有拓扑保持性,则其雅可比行列式的值在其定义域内应处处为正.数字图像受分辨率的限制,是定义在离散网格点上的,不是一个连续函数,因而得到的变形场也是离散的.文献[10]指出,连续变形场是离散变形场经过插值得到的,它的特性决定于离散场的特性.如果离散变形场在各个离散网格点处雅可比行列式的值为正,则经过线性插值后得到的连续变形场在定义域内具有拓扑保持性.设连续变形场T =(X,Y,Z )可以表示成X (x,y,z )=x +u x (x,y,z )(8)Y (x,y,z )=y +u y (x,y,z )(9)Z (x,y,z )=z +u z (x,y,z )(10)其中u x (x,y,z )、u y (x,y,z )和u z (x,y,z )分别表示点p (x,y,z )在x,y,z 坐标方向上的位移,该点处雅可比行列式的值为J (x,y,z ).定义另外一个变形场T k ,其表达式为X k (x,y,z )=x +ku x (x,y,z )(11)Y k (x,y,z )=y +ku y (x,y,z )(12)Z k (x,y,z )=z +ku z (x,y,z )(13)该变形场在p (x,y,z )点处的雅可比行列式的值为J k (x,y,z ).显然,当k =1时,J k (x,y,z )=J (x,y,z );若p (x,y,z )点处J (x,y,z )<0,意味着其不具有拓扑保持性.J k (x,y,z )是k 的连续函数,根据函数的连续性可知,至少存在一个k ∗∈[0,1],使得J k ∗(x,y,z )>0.离散场是对连续场的采样,则对于离散场,通过在原有位移场上乘以一个0∼1之间的系数,可以校正原离散变形场的拓扑性.变形场的雅可比行列式建立在对变形场求梯度的基础上.在离散条件下,我们采用中间差分方式求梯度,任一点的梯度在不同坐标方向上的分量由该坐标方向上待求点两侧的点(可以称这些点为关联点)确定.具体校正过程如下:步骤1.根据得到的变形场T 计算各点的雅可比行列式值J (x,y,z );步骤2.若在点(x,y,z )处J (x,y,z )<0,则从k =1开始,等步长减小k 值,得到点(x,y,z )的关联点的新位移.对每一个k ,根据这些新位移计算J k (x,y,z ).重复这个过程,直到找到k ∗,使所有的J k ∗(x,y,z )>0;步骤3.根据得到的T k ∗,检验变形后的模板图像与目标图像的匹配程度,若不满足要求,则以新得到的变形后的模板图像为可变形模板,重新配准,对得到的T 重复这一过程,直到满足条件为止.4实验本文实验采用真实的脑部医学核磁共振图像.其中模板图像为SPL [13]标准图谱,T1加权,包含256×256×160个体素.目标图像的采集仪器为1.5T GE 核磁共振仪,T1加权,256×256×124个体素.实验目的主要是验证本文提出的增强变形矢量场拓扑保持性方法的性能,主要进行了两方面的对比,分别比较了变形场拓扑校正前后其矢量空间特性的变化和不同的目标结构分割结果.图4所示为原Demons 变形场经过拓扑校正处理后的结果.实验中改变k 值时步长设为0.01,迭代终止条件为变形后的模板图像与目标图像的互相关系数达到给定的阈值或者以新得到的图像为可变形模板重新配准次数到达上限(本实验中设为3次).本文实验的互相关系数阈值设置方式为:设模板图像与目标图像的初始互相关系数为CC 0,则阈值CC t =(1−CC 0)/α+CC 0,参数α的值设为1.2.分别比较图4(a)∼(d)与图3(c)∼(f),可以看出校正后的变形场雅可比行列式的值满足拓扑保持性的要求,而且校正后的变形场更加规则、平滑.从图3(c)给出的实验结果可以看出,原变形场雅可比行列式的值不大于零的点所占比例较小,也就是说需要校正的点所占比例较小,因此相对于原算法其计算量只大约增加了5%.(a)变形后的模板图像(a)Deformed template(b)1区放大(b)Enlarged region 1(c)2区放大(c)Enlarged region 2(d)3区放大(d)Enlarged region 2图4拓扑校正后的变形场Fig.4The deformation field with corrected topologies图5所示为变形场拓扑校正后,各点位移量的变化值,颜色越浅表示该位置处的位移矢量的幅度改变得越多,最大改变量为1.86个像素,说明校正后的变形场基本保持了原有182自动化学报36卷场的几何特性.同时,图5表明位移幅值发生明显变化的像素点的个数相对较少,也证明了采用本文的拓扑校正方法不会使计算量大幅增加.图6比较了采用拓扑校正前和校正后的变形场分割丘脑结构的效果.图6(a)为可变形模板图像,上面叠加了丘脑的专家分割结果.图6(b)为待分割的目标图像,图6(c)中的白斑给出了校正前的变形场拓扑发生改变的区域,采用该变形场得到的丘脑分割结果见图6(d),显然得到的结果有撕裂现象,丘脑的拓扑结构发生了改变,相应位置的变形场有交叉现象,见图6(e).经过本文提出的拓扑校正处理后,表征雅可比行列式的值不为正的白斑消失,见图6(f),说明此时的变形场具有拓扑保持性,用该变形场进行同样的分割,避免了丘脑结构的撕裂,如图6(g)所示.对比校正前后该位置的变形场(图6(e)和图6(h)),可以看出本文提出的方法消除了其中的矢量交叉,使变形场更平滑、更合理.不过,从图6(g)可以看出,虽然校正后的变形场具有了拓扑保持性,但其分割结果仍存在误分割处.这是因为原配准算法是单纯基于图像灰度信息的,当邻近的不同目标的灰度值相似时,得到的变形场可能是局部最优解,而本算法在对变形场进行拓扑校正时,遵循尽可能少地改变原有变形场几何特性这一原则,一旦满足拓扑保持条件,即停止校正,所以不能完全改变这一局部最优变形场的情况.可以考虑增加配准时所用的信息(如位置,形状等),尽量避免初始变形场陷入局部最优.图5变形场校正前后的幅度差(以像素值度量)Fig.5The magnitude differences of the deformation fields before and after topology correction (measured in pixels)5结论在非刚性图像配准的研究中,保证变形场的拓扑不变性是一个重要问题.研究表明,经典的基于灰度的Demons 配准算法虽然采用了双射技术和平滑处理,所得变形场仍然存在拓扑改变问题.本文从矢量场几何特性的角度,分析了该变形场导致拓扑改变的原因,提出在尽量保持原有变形场几何特性的前提下直接调整点位移的方法校正原变形场的拓扑性,并给出了具体的理论支持.实验表明,本文给出的方法能够校正原变形场在拓扑保持方面的缺陷,得到更平滑、合理且具有拓扑保持性的变形场.(a)模板图像(a)Template(b)目标图像(b)Target(c)校正前拓扑状况(c)Topology beforecorrection(d)校正前丘脑分割(d)Thalams segmentationbefore correction(e)校正前的场放大图(e)Enlarged field beforecorrection(f)校正后拓扑状况(f)Topology aftercorrection(g)校正后丘脑分割(g)Thalams segmentationafter correction(h)校正后的场放大图(h)Enlarged field beforecorrection图6变形场校正前后脑结构分割对比Fig.6Comparison of brain structures segmentation based on the deformation fields with and without topology corrections1期林相波等:Demons非刚性配准算法拓扑保持性的研究183References1Gholipou A,Kehtarnavaz N,Briggs R,Devous M,Gopinath K.Brain functional localization:a survey of image regis-tration techniques.IEEE Transactions on Medical Imaging, 2007,26(4):427−4512Cachier P,Bardinet E,Dormont D,Pennec X,Ayache N.Iconic feature based nonrigid registration:the PASHA puter Vision and Image Understanding,2003, 89(2-3):272−2983Shen D G,Davatzikos C.HAMMER:hierarchical attribute matching mechanism for elastic registration.IEEE Transac-tions on Medical Imaging,2002,21(11):1421−14394Holden M.A review of geometric transformations for non-rigid body registration.IEEE Transactions on Medical Imag-ing,2008,27(1):111−1285Noblet V,Heinrich C,Heitz F,Armspach J P.Retrospec-tive evaluation of a topology preserving non-rigid registra-tion method.Medical Image Analysis,2006,10(3):366−3846Musse O,Heitz F,Armspach J P.Topology preserving deformable image matching using constrained hierarchical parametric models.IEEE Transactions on Image Processing, 2001,10(7):1081−10937Noblet V,Heinrich C,Heitz F,Armspach J P.3-D de-formable image registration:a topology preservation scheme based on hierarchical deformation models and interval anal-ysis optimization.IEEE Transactions on Image Processing, 2005,14(5):553−5668Leow A D,Yanovsky I,Chiang M C,Lee A D,Klunder A D, Lu A.Statistical properties of jacobian maps and the realiza-tion of unbiased large-deformation nonlinear image registra-tion.IEEE Transactions on Medical Imaging,2007,26(6): 822−8329Thirion J P.Image matching as a diffusion process:an anal-ogy with Maxwell s demons.Medical Image Analysis,1998, 2(3):243−26010Karacah B,Davatzikos C.Estimating topology preserving and smooth displacementfields.IEEE Transactions on Med-ical Imaging,2004,23(7):868−88011Tittgemeyer M,Wollny G,Kruggel F.Visualising deforma-tionfields computed by non-linear image -puting and Visualization in Science,2002,5(1):45−5112Rohlfing T,Maurer C R J,Bluemke D A,Jacobs M A.Volume-preserving nonrigid registration of mr breast images using free-form deformation with an incompressibility con-straint.IEEE Transactions on Medical Imaging,2003,22(6): 730−74113Kikinis R,Shenton M E,Iosifescu D V,McCarley R W, Saiviroonporn P,Hokama H H.A digital brain atlas for surgical planning,model-driven segmentation,and teaching.IEEE Transactions on Visualization and Computer Graph-ics,1996,2(3):232−241林相波大连理工大学电子与信息工程学院博士研究生.主要研究方向为生物医学图像与信号处理,模式识别.E-mail:linxbo@ (LIN Xiang-Bo Ph.D.candidate in the School of Electronic and Information Engineering,Dalian University of Technology. Her research interest covers biomedical image and signal pro-cessing,pattern recognition.)邱天爽大连理工大学电子与信息工程学院教授.主要研究方向为非高斯、非平稳信号分析与处理理论,生物医学信号与图像处理,通信信号处理理论.本文通信作者.E-mail:qiutsh@ (QIU Tian-Shuang Professor in the School of Electronic and Information Engineering,Dalian University of Technology. His research interest covers non-Gaussian,non-stable signal pro-cessing theory,biomedical signal and image processing,commu-nication signal processing theory.Corresponding author of this paper.)阮素法国兰斯大学通用电气与计算机工程系教授.主要研究方向为图像分割与分类,数据融合,模式识别,医学图像处理.E-mail:su.ruan@univ-reims.fr(RUAN Su Professor in the Department of Electrical Engi-neering and Computer Engineering,University of Reims,France. Her research interest covers image segmentation and classifica-tion,data fusion and pattern recognition,medical image pro-cessing.)NICOLIER Frédéric法国兰斯大学通用电气与计算机工程系副教授.主要研究方向为图像比较,分割评价.E-mail:frederic.nicolier@univ-reims.fr(NICOLIER Frédéric Associate professor in the Depart-ment of Electrical Engineering and Computer Engineering,Uni-versity of Reims,France.His research interest covers image com-parison by similarity measurements,segmentation evaluation.)。
代数中常用英语词汇

(0,2) 插值||(0,2) interpolation0#||zero-sharp; 读作零井或零开。
0+||zero-dagger; 读作零正。
1-因子||1-factor3-流形||3-manifold; 又称“三维流形”。
AIC准则||AIC criterion, Akaike information criterionAp 权||Ap-weightA稳定性||A-stability, absolute stabilityA最优设计||A-optimal designBCH 码||BCH code, Bose-Chaudhuri-Hocquenghem codeBIC准则||BIC criterion, Bayesian modification of the AICBMOA函数||analytic function of bounded mean oscillation; 全称“有界平均振动解析函数”。
BMO鞅||BMO martingaleBSD猜想||Birch and Swinnerton-Dyer conjecture; 全称“伯奇与斯温纳顿-戴尔猜想”。
B样条||B-splineC*代数||C*-algebra; 读作“C星代数”。
C0 类函数||function of class C0; 又称“连续函数类”。
CA T准则||CAT criterion, criterion for autoregressiveCM域||CM fieldCN 群||CN-groupCW 复形的同调||homology of CW complexCW复形||CW complexCW复形的同伦群||homotopy group of CW complexesCW剖分||CW decompositionCn 类函数||function of class Cn; 又称“n次连续可微函数类”。
Cp统计量||Cp-statisticC。
四大安全会议论文题目
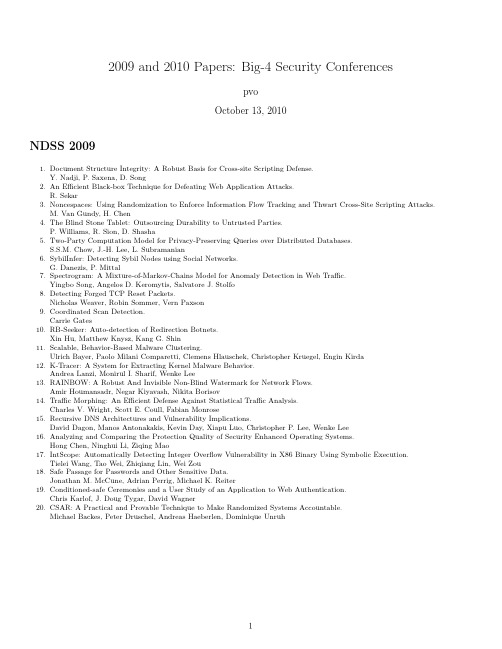
2009and2010Papers:Big-4Security ConferencespvoOctober13,2010NDSS20091.Document Structure Integrity:A Robust Basis for Cross-site Scripting Defense.Y.Nadji,P.Saxena,D.Song2.An Efficient Black-box Technique for Defeating Web Application Attacks.R.Sekar3.Noncespaces:Using Randomization to Enforce Information Flow Tracking and Thwart Cross-Site Scripting Attacks.M.Van Gundy,H.Chen4.The Blind Stone Tablet:Outsourcing Durability to Untrusted Parties.P.Williams,R.Sion,D.Shasha5.Two-Party Computation Model for Privacy-Preserving Queries over Distributed Databases.S.S.M.Chow,J.-H.Lee,L.Subramanian6.SybilInfer:Detecting Sybil Nodes using Social Networks.G.Danezis,P.Mittal7.Spectrogram:A Mixture-of-Markov-Chains Model for Anomaly Detection in Web Traffic.Yingbo Song,Angelos D.Keromytis,Salvatore J.Stolfo8.Detecting Forged TCP Reset Packets.Nicholas Weaver,Robin Sommer,Vern Paxson9.Coordinated Scan Detection.Carrie Gates10.RB-Seeker:Auto-detection of Redirection Botnets.Xin Hu,Matthew Knysz,Kang G.Shin11.Scalable,Behavior-Based Malware Clustering.Ulrich Bayer,Paolo Milani Comparetti,Clemens Hlauschek,Christopher Kruegel,Engin Kirda12.K-Tracer:A System for Extracting Kernel Malware Behavior.Andrea Lanzi,Monirul I.Sharif,Wenke Lee13.RAINBOW:A Robust And Invisible Non-Blind Watermark for Network Flows.Amir Houmansadr,Negar Kiyavash,Nikita Borisov14.Traffic Morphing:An Efficient Defense Against Statistical Traffic Analysis.Charles V.Wright,Scott E.Coull,Fabian Monrose15.Recursive DNS Architectures and Vulnerability Implications.David Dagon,Manos Antonakakis,Kevin Day,Xiapu Luo,Christopher P.Lee,Wenke Lee16.Analyzing and Comparing the Protection Quality of Security Enhanced Operating Systems.Hong Chen,Ninghui Li,Ziqing Mao17.IntScope:Automatically Detecting Integer Overflow Vulnerability in X86Binary Using Symbolic Execution.Tielei Wang,Tao Wei,Zhiqiang Lin,Wei Zou18.Safe Passage for Passwords and Other Sensitive Data.Jonathan M.McCune,Adrian Perrig,Michael K.Reiter19.Conditioned-safe Ceremonies and a User Study of an Application to Web Authentication.Chris Karlof,J.Doug Tygar,David Wagner20.CSAR:A Practical and Provable Technique to Make Randomized Systems Accountable.Michael Backes,Peter Druschel,Andreas Haeberlen,Dominique UnruhOakland20091.Wirelessly Pickpocketing a Mifare Classic Card.(Best Practical Paper Award)Flavio D.Garcia,Peter van Rossum,Roel Verdult,Ronny Wichers Schreur2.Plaintext Recovery Attacks Against SSH.Martin R.Albrecht,Kenneth G.Paterson,Gaven J.Watson3.Exploiting Unix File-System Races via Algorithmic Complexity Attacks.Xiang Cai,Yuwei Gui,Rob Johnson4.Practical Mitigations for Timing-Based Side-Channel Attacks on Modern x86Processors.Bart Coppens,Ingrid Verbauwhede,Bjorn De Sutter,Koen De Bosschere5.Non-Interference for a Practical DIFC-Based Operating System.Maxwell Krohn,Eran Tromer6.Native Client:A Sandbox for Portable,Untrusted x86Native Code.(Best Paper Award)B.Yee,D.Sehr,G.Dardyk,B.Chen,R.Muth,T.Ormandy,S.Okasaka,N.Narula,N.Fullagar7.Automatic Reverse Engineering of Malware Emulators.(Best Student Paper Award)Monirul Sharif,Andrea Lanzi,Jonathon Giffin,Wenke Lee8.Prospex:Protocol Specification Extraction.Paolo Milani Comparetti,Gilbert Wondracek,Christopher Kruegel,Engin Kirda9.Quantifying Information Leaks in Outbound Web Traffic.Kevin Borders,Atul Prakash10.Automatic Discovery and Quantification of Information Leaks.Michael Backes,Boris Kopf,Andrey Rybalchenko11.CLAMP:Practical Prevention of Large-Scale Data Leaks.Bryan Parno,Jonathan M.McCune,Dan Wendlandt,David G.Andersen,Adrian Perrig12.De-anonymizing Social Networks.Arvind Narayanan,Vitaly Shmatikov13.Privacy Weaknesses in Biometric Sketches.Koen Simoens,Pim Tuyls,Bart Preneel14.The Mastermind Attack on Genomic Data.Michael T.Goodrich15.A Logic of Secure Systems and its Application to Trusted Computing.Anupam Datta,Jason Franklin,Deepak Garg,Dilsun Kaynar16.Formally Certifying the Security of Digital Signature Schemes.Santiago Zanella-Beguelin,Gilles Barthe,Benjamin Gregoire,Federico Olmedo17.An Epistemic Approach to Coercion-Resistance for Electronic Voting Protocols.Ralf Kuesters,Tomasz Truderung18.Sphinx:A Compact and Provably Secure Mix Format.George Danezis,Ian Goldberg19.DSybil:Optimal Sybil-Resistance for Recommendation Systems.Haifeng Yu,Chenwei Shi,Michael Kaminsky,Phillip B.Gibbons,Feng Xiao20.Fingerprinting Blank Paper Using Commodity Scanners.William Clarkson,Tim Weyrich,Adam Finkelstein,Nadia Heninger,Alex Halderman,Ed Felten 21.Tempest in a Teapot:Compromising Reflections Revisited.Michael Backes,Tongbo Chen,Markus Duermuth,Hendrik P.A.Lensch,Martin Welk22.Blueprint:Robust Prevention of Cross-site Scripting Attacks for Existing Browsers.Mike Ter Louw,V.N.Venkatakrishnan23.Pretty-Bad-Proxy:An Overlooked Adversary in Browsers’HTTPS Deployments.Shuo Chen,Ziqing Mao,Yi-Min Wang,Ming Zhang24.Secure Content Sniffing for Web Browsers,or How to Stop Papers from Reviewing Themselves.Adam Barth,Juan Caballero,Dawn Song25.It’s No Secret:Measuring the Security and Reliability of Authentication via’Secret’Questions.Stuart Schechter,A.J.Bernheim Brush,Serge Egelman26.Password Cracking Using Probabilistic Context-Free Grammars.Matt Weir,Sudhir Aggarwal,Bill Glodek,Breno de MedeirosUSENIX Security2009promising Electromagnetic Emanations of Wired and Wireless Keyboards.(Outstanding Student Paper)Martin Vuagnoux,Sylvain Pasini2.Peeping Tom in the Neighborhood:Keystroke Eavesdropping on Multi-User Systems.Kehuan Zhang,XiaoFeng Wang3.A Practical Congestion Attack on Tor Using Long Paths,Nathan S.Evans,Roger Dingledine,Christian Grothoff4.Baggy Bounds Checking:An Efficient and Backwards-Compatible Defense against Out-of-Bounds Errors.Periklis Akritidis,Manuel Costa,Miguel Castro,Steven Hand5.Dynamic Test Generation to Find Integer Bugs in x86Binary Linux Programs.David Molnar,Xue Cong Li,David A.Wagner6.NOZZLE:A Defense Against Heap-spraying Code Injection Attacks.Paruj Ratanaworabhan,Benjamin Livshits,Benjamin Zorn7.Detecting Spammers with SNARE:Spatio-temporal Network-level Automatic Reputation Engine.Shuang Hao,Nadeem Ahmed Syed,Nick Feamster,Alexander G.Gray,Sven Krasser8.Improving Tor using a TCP-over-DTLS Tunnel.Joel Reardon,Ian Goldberg9.Locating Prefix Hijackers using LOCK.Tongqing Qiu,Lusheng Ji,Dan Pei,Jia Wang,Jun(Jim)Xu,Hitesh Ballani10.GATEKEEPER:Mostly Static Enforcement of Security and Reliability Policies for JavaScript Code.Salvatore Guarnieri,Benjamin Livshits11.Cross-Origin JavaScript Capability Leaks:Detection,Exploitation,and Defense.Adam Barth,Joel Weinberger,Dawn Song12.Memory Safety for Low-Level Software/Hardware Interactions.John Criswell,Nicolas Geoffray,Vikram Adve13.Physical-layer Identification of RFID Devices.Boris Danev,Thomas S.Heydt-Benjamin,Srdjan CapkunCP:Secure Remote Storage for Computational RFIDs.Mastooreh Salajegheh,Shane Clark,Benjamin Ransford,Kevin Fu,Ari Juels15.Jamming-resistant Broadcast Communication without Shared Keys.Christina Popper,Mario Strasser,Srdjan Capkun16.xBook:Redesigning Privacy Control in Social Networking Platforms.Kapil Singh,Sumeer Bhola,Wenke Lee17.Nemesis:Preventing Authentication and Access Control Vulnerabilities in Web Applications.Michael Dalton,Christos Kozyrakis,Nickolai Zeldovich18.Static Enforcement of Web Application Integrity Through Strong Typing.William Robertson,Giovanni Vigna19.Vanish:Increasing Data Privacy with Self-Destructing Data.(Outstanding Student Paper)Roxana Geambasu,Tadayoshi Kohno,Amit A.Levy,Henry M.Levy20.Efficient Data Structures for Tamper-Evident Logging.Scott A.Crosby,Dan S.Wallach21.VPriv:Protecting Privacy in Location-Based Vehicular Services.Raluca Ada Popa,Hari Balakrishnan,Andrew J.Blumberg22.Effective and Efficient Malware Detection at the End Host.Clemens Kolbitsch,Paolo Milani Comparetti,Christopher Kruegel,Engin Kirda,Xiaoyong Zhou,XiaoFeng Wang 23.Protecting Confidential Data on Personal Computers with Storage Capsules.Kevin Borders,Eric Vander Weele,Billy Lau,Atul Prakash24.Return-Oriented Rootkits:Bypassing Kernel Code Integrity Protection Mechanisms.Ralf Hund,Thorsten Holz,Felix C.Freiling25.Crying Wolf:An Empirical Study of SSL Warning Effectiveness.Joshua Sunshine,Serge Egelman,Hazim Almuhimedi,Neha Atri,Lorrie Faith Cranor26.The Multi-Principal OS Construction of the Gazelle Web Browser.Helen J.Wang,Chris Grier,Alex Moshchuk,Samuel T.King,Piali Choudhury,Herman VenterACM CCS20091.Attacking cryptographic schemes based on”perturbation polynomials”.Martin Albrecht,Craig Gentry,Shai Halevi,Jonathan Katz2.Filter-resistant code injection on ARM.Yves Younan,Pieter Philippaerts,Frank Piessens,Wouter Joosen,Sven Lachmund,Thomas Walter3.False data injection attacks against state estimation in electric power grids.Yao Liu,Michael K.Reiter,Peng Ning4.EPC RFID tag security weaknesses and defenses:passport cards,enhanced drivers licenses,and beyond.Karl Koscher,Ari Juels,Vjekoslav Brajkovic,Tadayoshi Kohno5.An efficient forward private RFID protocol.Come Berbain,Olivier Billet,Jonathan Etrog,Henri Gilbert6.RFID privacy:relation between two notions,minimal condition,and efficient construction.Changshe Ma,Yingjiu Li,Robert H.Deng,Tieyan Li7.CoSP:a general framework for computational soundness proofs.Michael Backes,Dennis Hofheinz,Dominique Unruh8.Reactive noninterference.Aaron Bohannon,Benjamin C.Pierce,Vilhelm Sjoberg,Stephanie Weirich,Steve Zdancewicputational soundness for key exchange protocols with symmetric encryption.Ralf Kusters,Max Tuengerthal10.A probabilistic approach to hybrid role mining.Mario Frank,Andreas P.Streich,David A.Basin,Joachim M.Buhmann11.Efficient pseudorandom functions from the decisional linear assumption and weaker variants.Allison B.Lewko,Brent Waters12.Improving privacy and security in multi-authority attribute-based encryption.Melissa Chase,Sherman S.M.Chow13.Oblivious transfer with access control.Jan Camenisch,Maria Dubovitskaya,Gregory Neven14.NISAN:network information service for anonymization networks.Andriy Panchenko,Stefan Richter,Arne Rache15.Certificateless onion routing.Dario Catalano,Dario Fiore,Rosario Gennaro16.ShadowWalker:peer-to-peer anonymous communication using redundant structured topologies.Prateek Mittal,Nikita Borisov17.Ripley:automatically securing web2.0applications through replicated execution.K.Vikram,Abhishek Prateek,V.Benjamin Livshits18.HAIL:a high-availability and integrity layer for cloud storage.Kevin D.Bowers,Ari Juels,Alina Oprea19.Hey,you,get offof my cloud:exploring information leakage in third-party compute clouds.Thomas Ristenpart,Eran Tromer,Hovav Shacham,Stefan Savage20.Dynamic provable data possession.C.Christopher Erway,Alptekin Kupcu,Charalampos Papamanthou,Roberto Tamassia21.On cellular botnets:measuring the impact of malicious devices on a cellular network core.Patrick Traynor,Michael Lin,Machigar Ongtang,Vikhyath Rao,Trent Jaeger,Patrick Drew McDaniel,Thomas Porta 22.On lightweight mobile phone application certification.William Enck,Machigar Ongtang,Patrick Drew McDaniel23.SMILE:encounter-based trust for mobile social services.Justin Manweiler,Ryan Scudellari,Landon P.Cox24.Battle of Botcraft:fighting bots in online games with human observational proofs.Steven Gianvecchio,Zhenyu Wu,Mengjun Xie,Haining Wang25.Fides:remote anomaly-based cheat detection using client emulation.Edward C.Kaiser,Wu-chang Feng,Travis Schluessler26.Behavior based software theft detection.Xinran Wang,Yoon-chan Jhi,Sencun Zhu,Peng Liu27.The fable of the bees:incentivizing robust revocation decision making in ad hoc networks.Steffen Reidt,Mudhakar Srivatsa,Shane Balfe28.Effective implementation of the cell broadband engineTM isolation loader.Masana Murase,Kanna Shimizu,Wilfred Plouffe,Masaharu Sakamoto29.On achieving good operating points on an ROC plane using stochastic anomaly score prediction.Muhammad Qasim Ali,Hassan Khan,Ali Sajjad,Syed Ali Khayam30.On non-cooperative location privacy:a game-theoretic analysis.Julien Freudiger,Mohammad Hossein Manshaei,Jean-Pierre Hubaux,David C.Parkes31.Privacy-preserving genomic computation through program specialization.Rui Wang,XiaoFeng Wang,Zhou Li,Haixu Tang,Michael K.Reiter,Zheng Dong32.Feeling-based location privacy protection for location-based services.Toby Xu,Ying Cai33.Multi-party off-the-record messaging.Ian Goldberg,Berkant Ustaoglu,Matthew Van Gundy,Hao Chen34.The bayesian traffic analysis of mix networks.Carmela Troncoso,George Danezis35.As-awareness in Tor path selection.Matthew Edman,Paul F.Syverson36.Membership-concealing overlay networks.Eugene Y.Vasserman,Rob Jansen,James Tyra,Nicholas Hopper,Yongdae Kim37.On the difficulty of software-based attestation of embedded devices.Claude Castelluccia,Aurelien Francillon,Daniele Perito,Claudio Soriente38.Proximity-based access control for implantable medical devices.Kasper Bonne Rasmussen,Claude Castelluccia,Thomas S.Heydt-Benjamin,Srdjan Capkun39.XCS:cross channel scripting and its impact on web applications.Hristo Bojinov,Elie Bursztein,Dan Boneh40.A security-preserving compiler for distributed programs:from information-flow policies to cryptographic mechanisms.Cedric Fournet,Gurvan Le Guernic,Tamara Rezk41.Finding bugs in exceptional situations of JNI programs.Siliang Li,Gang Tan42.Secure open source collaboration:an empirical study of Linus’law.Andrew Meneely,Laurie A.Williams43.On voting machine design for verification and testability.Cynthia Sturton,Susmit Jha,Sanjit A.Seshia,David Wagner44.Secure in-VM monitoring using hardware virtualization.Monirul I.Sharif,Wenke Lee,Weidong Cui,Andrea Lanzi45.A metadata calculus for secure information sharing.Mudhakar Srivatsa,Dakshi Agrawal,Steffen Reidt46.Multiple password interference in text passwords and click-based graphical passwords.Sonia Chiasson,Alain Forget,Elizabeth Stobert,Paul C.van Oorschot,Robert Biddle47.Can they hear me now?:a security analysis of law enforcement wiretaps.Micah Sherr,Gaurav Shah,Eric Cronin,Sandy Clark,Matt Blaze48.English shellcode.Joshua Mason,Sam Small,Fabian Monrose,Greg MacManus49.Learning your identity and disease from research papers:information leaks in genome wide association study.Rui Wang,Yong Fuga Li,XiaoFeng Wang,Haixu Tang,Xiao-yong Zhou50.Countering kernel rootkits with lightweight hook protection.Zhi Wang,Xuxian Jiang,Weidong Cui,Peng Ning51.Mapping kernel objects to enable systematic integrity checking.Martim Carbone,Weidong Cui,Long Lu,Wenke Lee,Marcus Peinado,Xuxian Jiang52.Robust signatures for kernel data structures.Brendan Dolan-Gavitt,Abhinav Srivastava,Patrick Traynor,Jonathon T.Giffin53.A new cell counter based attack against tor.Zhen Ling,Junzhou Luo,Wei Yu,Xinwen Fu,Dong Xuan,Weijia Jia54.Scalable onion routing with torsk.Jon McLachlan,Andrew Tran,Nicholas Hopper,Yongdae Kim55.Anonymous credentials on a standard java card.Patrik Bichsel,Jan Camenisch,Thomas Gros,Victor Shouprge-scale malware indexing using function-call graphs.Xin Hu,Tzi-cker Chiueh,Kang G.Shin57.Dispatcher:enabling active botnet infiltration using automatic protocol reverse-engineering.Juan Caballero,Pongsin Poosankam,Christian Kreibich,Dawn Xiaodong Song58.Your botnet is my botnet:analysis of a botnet takeover.Brett Stone-Gross,Marco Cova,Lorenzo Cavallaro,Bob Gilbert,MartinSzydlowski,Richard A.Kemmerer,Christopher Kruegel,Giovanni VignaNDSS20101.Server-side Verification of Client Behavior in Online Games.Darrell Bethea,Robert Cochran and Michael Reiter2.Defeating Vanish with Low-Cost Sybil Attacks Against Large DHTs.S.Wolchok,O.S.Hofmann,N.Heninger,E.W.Felten,J.A.Halderman,C.J.Rossbach,B.Waters,E.Witchel3.Stealth DoS Attacks on Secure Channels.Amir Herzberg and Haya Shulman4.Protecting Browsers from Extension Vulnerabilities.Adam Barth,Adrienne Porter Felt,Prateek Saxena,and Aaron Boodman5.Adnostic:Privacy Preserving Targeted Advertising.Vincent Toubiana,Arvind Narayanan,Dan Boneh,Helen Nissenbaum and Solon Barocas6.FLAX:Systematic Discovery of Client-side Validation Vulnerabilities in Rich Web Applications.Prateek Saxena,Steve Hanna,Pongsin Poosankam and Dawn Song7.Effective Anomaly Detection with Scarce Training Data.William Robertson,Federico Maggi,Christopher Kruegel and Giovanni Vignarge-Scale Automatic Classification of Phishing Pages.Colin Whittaker,Brian Ryner and Marria Nazif9.A Systematic Characterization of IM Threats using Honeypots.Iasonas Polakis,Thanasis Petsas,Evangelos P.Markatos and Spiros Antonatos10.On Network-level Clusters for Spam Detection.Zhiyun Qian,Zhuoqing Mao,Yinglian Xie and Fang Yu11.Improving Spam Blacklisting Through Dynamic Thresholding and Speculative Aggregation.Sushant Sinha,Michael Bailey and Farnam Jahanian12.Botnet Judo:Fighting Spam with Itself.A.Pitsillidis,K.Levchenko,C.Kreibich,C.Kanich,G.M.Voelker,V.Paxson,N.Weaver,S.Savage13.Contractual Anonymity.Edward J.Schwartz,David Brumley and Jonathan M.McCune14.A3:An Extensible Platform for Application-Aware Anonymity.Micah Sherr,Andrew Mao,William R.Marczak,Wenchao Zhou,Boon Thau Loo,and Matt Blaze15.When Good Randomness Goes Bad:Virtual Machine Reset Vulnerabilities and Hedging Deployed Cryptography.Thomas Ristenpart and Scott Yilek16.InvisiType:Object-Oriented Security Policies.Jiwon Seo and Monica m17.A Security Evaluation of DNSSEC with NSEC3.Jason Bau and John Mitchell18.On the Safety of Enterprise Policy Deployment.Yudong Gao,Ni Pan,Xu Chen and Z.Morley Mao19.Where Do You Want to Go Today?Escalating Privileges by Pathname Manipulation.Suresh Chari,Shai Halevi and Wietse Venema20.Joe-E:A Security-Oriented Subset of Java.Adrian Mettler,David Wagner and Tyler Close21.Preventing Capability Leaks in Secure JavaScript Subsets.Matthew Finifter,Joel Weinberger and Adam Barth22.Binary Code Extraction and Interface Identification for Security Applications.Juan Caballero,Noah M.Johnson,Stephen McCamant,and Dawn Song23.Automatic Reverse Engineering of Data Structures from Binary Execution.Zhiqiang Lin,Xiangyu Zhang and Dongyan Xu24.Efficient Detection of Split Personalities in Malware.Davide Balzarotti,Marco Cova,Christoph Karlberger,Engin Kirda,Christopher Kruegel and Giovanni VignaOakland20101.Inspector Gadget:Automated Extraction of Proprietary Gadgets from Malware Binaries.Clemens Kolbitsch Thorsten Holz,Christopher Kruegel,Engin Kirda2.Synthesizing Near-Optimal Malware Specifications from Suspicious Behaviors.Matt Fredrikson,Mihai Christodorescu,Somesh Jha,Reiner Sailer,Xifeng Yan3.Identifying Dormant Functionality in Malware Programs.Paolo Milani Comparetti,Guido Salvaneschi,Clemens Kolbitsch,Engin Kirda,Christopher Kruegel,Stefano Zanero4.Reconciling Belief and Vulnerability in Information Flow.Sardaouna Hamadou,Vladimiro Sassone,Palamidessi5.Towards Static Flow-Based Declassification for Legacy and Untrusted Programs.Bruno P.S.Rocha,Sruthi Bandhakavi,Jerry I.den Hartog,William H.Winsborough,Sandro Etalle6.Non-Interference Through Secure Multi-Execution.Dominique Devriese,Frank Piessens7.Object Capabilities and Isolation of Untrusted Web Applications.Sergio Maffeis,John C.Mitchell,Ankur Taly8.TrustVisor:Efficient TCB Reduction and Attestation.Jonathan McCune,Yanlin Li,Ning Qu,Zongwei Zhou,Anupam Datta,Virgil Gligor,Adrian Perrig9.Overcoming an Untrusted Computing Base:Detecting and Removing Malicious Hardware Automatically.Matthew Hicks,Murph Finnicum,Samuel T.King,Milo M.K.Martin,Jonathan M.Smith10.Tamper Evident Microprocessors.Adam Waksman,Simha Sethumadhavan11.Side-Channel Leaks in Web Applications:a Reality Today,a Challenge Tomorrow.Shuo Chen,Rui Wang,XiaoFeng Wang Kehuan Zhang12.Investigation of Triangular Spamming:a Stealthy and Efficient Spamming Technique.Zhiyun Qian,Z.Morley Mao,Yinglian Xie,Fang Yu13.A Practical Attack to De-Anonymize Social Network Users.Gilbert Wondracek,Thorsten Holz,Engin Kirda,Christopher Kruegel14.SCiFI-A System for Secure Face Identification.(Best Paper)Margarita Osadchy,Benny Pinkas,Ayman Jarrous,Boaz Moskovich15.Round-Efficient Broadcast Authentication Protocols for Fixed Topology Classes.Haowen Chan,Adrian Perrig16.Revocation Systems with Very Small Private Keys.Allison Lewko,Amit Sahai,Brent Waters17.Authenticating Primary Users’Signals in Cognitive Radio Networks via Integrated Cryptographic and Wireless Link Signatures.Yao Liu,Peng Ning,Huaiyu Dai18.Outside the Closed World:On Using Machine Learning For Network Intrusion Detection.Robin Sommer,Vern Paxson19.All You Ever Wanted to Know about Dynamic Taint Analysis and Forward Symbolic Execution(but might have been afraid to ask).Thanassis Avgerinos,Edward Schwartz,David Brumley20.State of the Art:Automated Black-Box Web Application Vulnerability Testing.Jason Bau,Elie Bursztein,Divij Gupta,John Mitchell21.A Proof-Carrying File System.Deepak Garg,Frank Pfenning22.Scalable Parametric Verification of Secure Systems:How to Verify Ref.Monitors without Worrying about Data Structure Size.Jason Franklin,Sagar Chaki,Anupam Datta,Arvind Seshadri23.HyperSafe:A Lightweight Approach to Provide Lifetime Hypervisor Control-Flow Integrity.Zhi Wang,Xuxian Jiang24.How Good are Humans at Solving CAPTCHAs?A Large Scale Evaluation.Elie Bursztein,Steven Bethard,John C.Mitchell,Dan Jurafsky,Celine Fabry25.Bootstrapping Trust in Commodity Computers.Bryan Parno,Jonathan M.McCune,Adrian Perrig26.Chip and PIN is Broken.(Best Practical Paper)Steven J.Murdoch,Saar Drimer,Ross Anderson,Mike Bond27.Experimental Security Analysis of a Modern Automobile.K.Koscher,A.Czeskis,F.Roesner,S.Patel,T.Kohno,S.Checkoway,D.McCoy,B.Kantor,D.Anderson,H.Shacham,S.Savage 28.On the Incoherencies in Web Browser Access Control Policies.Kapil Singh,Alexander Moshchuk,Helen J.Wang,Wenke Lee29.ConScript:Specifying and Enforcing Fine-Grained Security Policies for JavaScript in the Browser.Leo Meyerovich,Benjamin Livshits30.TaintScope:A Checksum-Aware Directed Fuzzing Tool for Automatic Software Vulnerability Detection.(Best Student Paper)Tielei Wang,Tao Wei,Guofei Gu,Wei Zou31.A Symbolic Execution Framework for JavaScript.Prateek Saxena,Devdatta Akhawe,Steve Hanna,Stephen McCamant,Dawn Song,Feng MaoUSENIX Security20101.Adapting Software Fault Isolation to Contemporary CPU Architectures.David Sehr,Robert Muth,CliffBiffle,Victor Khimenko,Egor Pasko,Karl Schimpf,Bennet Yee,Brad Chen2.Making Linux Protection Mechanisms Egalitarian with UserFS.Taesoo Kim and Nickolai Zeldovich3.Capsicum:Practical Capabilities for UNIX.(Best Student Paper)Robert N.M.Watson,Jonathan Anderson,Ben Laurie,Kris Kennaway4.Structuring Protocol Implementations to Protect Sensitive Data.Petr Marchenko,Brad Karp5.PrETP:Privacy-Preserving Electronic Toll Pricing.Josep Balasch,Alfredo Rial,Carmela Troncoso,Bart Preneel,Ingrid Verbauwhede,Christophe Geuens6.An Analysis of Private Browsing Modes in Modern Browsers.Gaurav Aggarwal,Elie Bursztein,Collin Jackson,Dan Boneh7.BotGrep:Finding P2P Bots with Structured Graph Analysis.Shishir Nagaraja,Prateek Mittal,Chi-Yao Hong,Matthew Caesar,Nikita Borisov8.Fast Regular Expression Matching Using Small TCAMs for Network Intrusion Detection and Prevention Systems.Chad R.Meiners,Jignesh Patel,Eric Norige,Eric Torng,Alex X.Liu9.Searching the Searchers with SearchAudit.John P.John,Fang Yu,Yinglian Xie,Martin Abadi,Arvind Krishnamurthy10.Toward Automated Detection of Logic Vulnerabilities in Web Applications.Viktoria Felmetsger,Ludovico Cavedon,Christopher Kruegel,Giovanni Vigna11.Baaz:A System for Detecting Access Control Misconfigurations.Tathagata Das,Ranjita Bhagwan,Prasad Naldurg12.Cling:A Memory Allocator to Mitigate Dangling Pointers.Periklis Akritidis13.ZKPDL:A Language-Based System for Efficient Zero-Knowledge Proofs and Electronic Cash.Sarah Meiklejohn,C.Chris Erway,Alptekin Kupcu,Theodora Hinkle,Anna Lysyanskaya14.P4P:Practical Large-Scale Privacy-Preserving Distributed Computation Robust against Malicious Users.Yitao Duan,John Canny,Justin Zhan,15.SEPIA:Privacy-Preserving Aggregation of Multi-Domain Network Events and Statistics.Martin Burkhart,Mario Strasser,Dilip Many,Xenofontas Dimitropoulos16.Dude,Where’s That IP?Circumventing Measurement-based IP Geolocation.Phillipa Gill,Yashar Ganjali,Bernard Wong,David Lie17.Idle Port Scanning and Non-interference Analysis of Network Protocol Stacks Using Model Checking.Roya Ensafi,Jong Chun Park,Deepak Kapur,Jedidiah R.Crandall18.Building a Dynamic Reputation System for DNS.Manos Antonakakis,Roberto Perdisci,David Dagon,Wenke Lee,Nick Feamster19.Scantegrity II Municipal Election at Takoma Park:The First E2E Binding Governmental Election with Ballot Privacy.R.Carback,D.Chaum,J.Clark,J.Conway,A.Essex,P.S.Herrnson,T.Mayberry,S.Popoveniuc,R.L.Rivest,E.Shen,A.T.Sherman,P.L.Vora20.Acoustic Side-Channel Attacks on Printers.Michael Backes,Markus Durmuth,Sebastian Gerling,Manfred Pinkal,Caroline Sporleder21.Security and Privacy Vulnerabilities of In-Car Wireless Networks:A Tire Pressure Monitoring System Case Study.Ishtiaq Rouf,Rob Miller,Hossen Mustafa,Travis Taylor,Sangho Oh,Wenyuan Xu,Marco Gruteser,Wade Trappe,Ivan Seskar 22.VEX:Vetting Browser Extensions for Security Vulnerabilities.(Best Paper)Sruthi Bandhakavi,Samuel T.King,P.Madhusudan,Marianne Winslett23.Securing Script-Based Extensibility in Web Browsers.Vladan Djeric,Ashvin Goel24.AdJail:Practical Enforcement of Confidentiality and Integrity Policies on Web Advertisements.Mike Ter Louw,Karthik Thotta Ganesh,V.N.Venkatakrishnan25.Realization of RF Distance Bounding.Kasper Bonne Rasmussen,Srdjan Capkun26.The Case for Ubiquitous Transport-Level Encryption.Andrea Bittau,Michael Hamburg,Mark Handley,David Mazieres,Dan Boneh27.Automatic Generation of Remediation Procedures for Malware Infections.Roberto Paleari,Lorenzo Martignoni,Emanuele Passerini,Drew Davidson,Matt Fredrikson,Jon Giffin,Somesh Jha28.Re:CAPTCHAs-Understanding CAPTCHA-Solving Services in an Economic Context.Marti Motoyama,Kirill Levchenko,Chris Kanich,Damon McCoy,Geoffrey M.Voelker,Stefan Savage29.Chipping Away at Censorship Firewalls with User-Generated Content.Sam Burnett,Nick Feamster,Santosh Vempala30.Fighting Coercion Attacks in Key Generation using Skin Conductance.Payas Gupta,Debin GaoACM CCS20101.Security Analysis of India’s Electronic Voting Machines.Scott Wolchok,Erik Wustrow,J.Alex Halderman,Hari Prasad,Rop Gonggrijp2.Dissecting One Click Frauds.Nicolas Christin,Sally S.Yanagihara,Keisuke Kamataki3.@spam:The Underground on140Characters or Less.Chris Grier,Kurt Thomas,Vern Paxson,Michael Zhang4.HyperSentry:Enabling Stealthy In-context Measurement of Hypervisor Integrity.Ahmed M.Azab,Peng Ning,Zhi Wang,Xuxian Jiang,Xiaolan Zhang,Nathan C.Skalsky5.Trail of Bytes:Efficient Support for Forensic Analysis.Srinivas Krishnan,Kevin Z.Snow,Fabian Monrose6.Survivable Key Compromise in Software Update Systems.Justin Samuel,Nick Mathewson,Justin Cappos,Roger Dingledine7.A Methodology for Empirical Analysis of the Permission-Based Security Models and its Application to Android.David Barrera,H.Gunes Kayacik,Paul C.van Oorschot,Anil Somayaji8.Mobile Location Tracking in Metropolitan Areas:malnets and others.Nathanial Husted,Steve Myers9.On Pairing Constrained Wireless Devices Based on Secrecy of Auxiliary Channels:The Case of Acoustic Eavesdropping.Tzipora Halevi,Nitesh Saxena10.PinDr0p:Using Single-Ended Audio Features to Determine Call Provenance.Vijay A.Balasubramaniyan,Aamir Poonawalla,Mustaque Ahamad,Michael T.Hunter,Patrick Traynor11.Building Efficient Fully Collusion-Resilient Traitor Tracing and Revocation Schemes.Sanjam Garg,Abishek Kumarasubramanian,Amit Sahai,Brent Waters12.Algebraic Pseudorandom Functions with Improved Efficiency from the Augmented Cascade.Dan Boneh,Hart Montgomery,Ananth Raghunathan13.Practical Leakage-Resilient Pseudorandom Generators.Yu Yu,Francois-Xavier Standaert,Olivier Pereira,Moti Yung14.Practical Leakage-Resilient Identity-Based Encryption from Simple Assumptions.Sherman S.M.Chow,Yevgeniy Dodis,Yannis Rouselakis,Brent Waters15.Testing Metrics for Password Creation Policies by Attacking Large Sets of Revealed Passwords.Matt Weir,Sudhir Aggarwal,Michael Collins,Henry Stern16.The Security of Modern Password Expiration:An Algorithmic Framework and Empirical Analysis.Yinqian Zhang,Fabian Monrose,Michael K.Reiter17.Attacks and Design of Image Recognition CAPTCHAs.Bin Zhu,JeffYan,Chao Yang,Qiujie Li,Jiu Liu,Ning Xu,Meng Yi18.Robusta:Taming the Native Beast of the JVM.Joseph Siefers,Gang Tan,Greg Morrisett19.Retaining Sandbox Containment Despite Bugs in Privileged Memory-Safe Code.Justin Cappos,Armon Dadgar,JeffRasley,Justin Samuel,Ivan Beschastnikh,Cosmin Barsan,Arvind Krishnamurthy,Thomas Anderson20.A Control Point for Reducing Root Abuse of File-System Privileges.Glenn Wurster,Paul C.van Oorschot21.Modeling Attacks on Physical Unclonable Functions.Ulrich Ruehrmair,Frank Sehnke,Jan Soelter,Gideon Dror,Srinivas Devadas,Juergen Schmidhuber22.Dismantling SecureMemory,CryptoMemory and CryptoRF.Flavio D.Garcia,Peter van Rossum,Roel Verdult,Ronny Wichers Schreur23.Attacking and Fixing PKCS#11Security Tokens.Matteo Bortolozzo,Matteo Centenaro,Riccardo Focardi,Graham Steel24.An Empirical Study of Privacy-Violating Information Flows in JavaScript Web Applications.Dongseok Jang,Ranjit Jhala,Sorin Lerner,Hovav Shacham25.DIFC Programs by Automatic Instrumentation.William Harris,Somesh Jha,Thomas Reps26.Predictive Black-box Mitigation of Timing Channels.Aslan Askarov,Danfeng Zhang,Andrew Myers27.In Search of an Anonymous and Secure Lookup:Attacks on Structured Peer-to-peer Anonymous Communication Systems.Qiyan Wang,Prateek Mittal,Nikita Borisov28.Recruiting New Tor Relays with BRAIDS.Rob Jansen,Nicholas Hopper,Yongdae Kim29.An Improved Algorithm for Tor Circuit Scheduling.Can Tang,Ian Goldberg30.Dissent:Accountable Anonymous Group Messaging.Henry Corrigan-Gibbs,Bryan Ford31.Abstraction by Set-Membership—Verifying Security Protocols and Web Services with Databases.Sebastian Moedersheim。
代数英语

(0,2) 插值||(0,2) interpolation0#||zero-sharp; 读作零井或零开。
0+||zero-dagger; 读作零正。
1-因子||1-factor3-流形||3-manifold; 又称“三维流形”。
AIC准则||AIC criterion, Akaike information criterionAp 权||Ap-weightA稳定性||A-stability, absolute stabilityA最优设计||A-optimal designBCH 码||BCH code, Bose-Chaudhuri-Hocquenghem codeBIC准则||BIC criterion, Bayesian modification of the AICBMOA函数||analytic function of bounded mean oscillation; 全称“有界平均振动解析函数”。
BMO鞅||BMO martingaleBSD猜想||Birch and Swinnerton-Dyer conjecture; 全称“伯奇与斯温纳顿-戴尔猜想”。
B样条||B-splineC*代数||C*-algebra; 读作“C星代数”。
C0 类函数||function of class C0; 又称“连续函数类”。
CA T准则||CAT criterion, criterion for autoregressiveCM域||CM fieldCN 群||CN-groupCW 复形的同调||homology of CW complexCW复形||CW complexCW复形的同伦群||homotopy group of CW complexesCW剖分||CW decompositionCn 类函数||function of class Cn; 又称“n次连续可微函数类”。
Cp统计量||Cp-statisticC。
基于CPN的安全协议形式化建模及安全分析方法
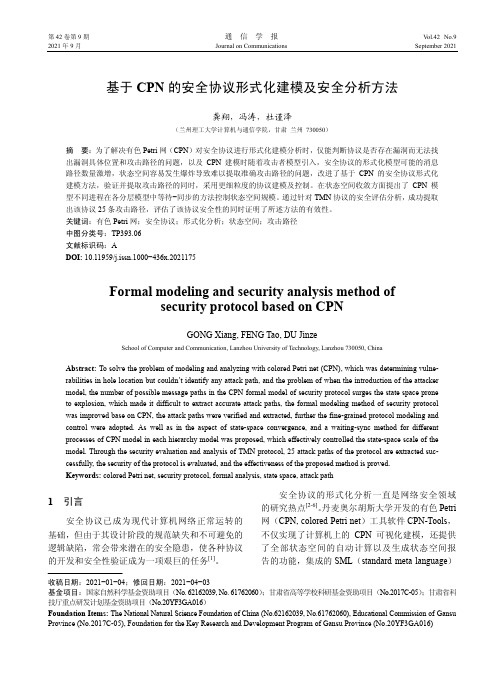
2021年9月Journal on Communications September 2021 第42卷第9期通信学报V ol.42No.9基于CPN的安全协议形式化建模及安全分析方法龚翔,冯涛,杜谨泽(兰州理工大学计算机与通信学院,甘肃兰州 730050)摘 要:为了解决有色Petri网(CPN)对安全协议进行形式化建模分析时,仅能判断协议是否存在漏洞而无法找出漏洞具体位置和攻击路径的问题,以及CPN建模时随着攻击者模型引入,安全协议的形式化模型可能的消息路径数量激增,状态空间容易发生爆炸导致难以提取准确攻击路径的问题,改进了基于CPN的安全协议形式化建模方法,验证并提取攻击路径的同时,采用更细粒度的协议建模及控制。
在状态空间收敛方面提出了CPN模型不同进程在各分层模型中等待−同步的方法控制状态空间规模。
通过针对TMN协议的安全评估分析,成功提取出该协议25条攻击路径,评估了该协议安全性的同时证明了所述方法的有效性。
关键词:有色Petri网;安全协议;形式化分析;状态空间;攻击路径中图分类号:TP393.06文献标识码:ADOI: 10.11959/j.issn.1000−436x.2021175Formal modeling and security analysis method ofsecurity protocol based on CPNGONG Xiang, FENG Tao, DU JinzeSchool of Computer and Communication, Lanzhou University of Technology, Lanzhou 730050, China Abstract: To solve the problem of modeling and analyzing with colored Petri net (CPN), which was determining vulne-rabilities in hole location but couldn’t identify any attack path, and the problem of when the introduction of the attacker model, the number of possible message paths in the CPN formal model of security protocol surges the state space prone to explosion, which made it difficult to extract accurate attack paths, the formal modeling method of security protocol was improved base on CPN, the attack paths were verified and extracted, further the fine-grained protocol modeling and control were adopted. As well as in the aspect of state-space convergence, and a waiting-sync method for different processes of CPN model in each hierarchy model was proposed, which effectively controlled the state-space scale of the model. Through the security evaluation and analysis of TMN protocol, 25 attack paths of the protocol are extracted suc-cessfully, the security of the protocol is evaluated, and the effectiveness of the proposed method is proved.Keywords: colored Petri net, security protocol, formal analysis, state space, attack path1 引言安全协议已成为现代计算机网络正常运转的基础,但由于其设计阶段的规范缺失和不可避免的逻辑缺陷,常会带来潜在的安全隐患,使各种协议的开发和安全性验证成为一项艰巨的任务[1]。
Graph Regularized Nonnegative Matrix
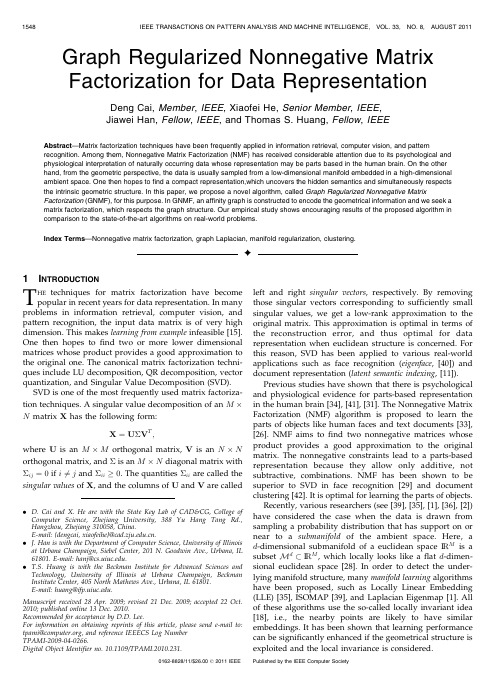
Ç
1 INTRODUCTION
HE
techniques for matrix factorization have become popular in recent years for data representation. In many problems in information retrieval, computer vision, and pattern recognition, the input data matrix is of very high dimension. This makes learning from example infeasible [15]. One then hopes to find two or more lower dimensional matrices whose product provides a good approximation to the original one. The canonical matrix factorization techniques include LU decomposition, QR decomposition, vector quantization, and Singular Value Decomposition (SVD). SVD is one of the most frequently used matrix factorization techniques. A singular value decomposition of an M Â N matrix X has the following form: X ¼ UÆVT ; where U is an M Â M orthogonal matrix, V is an N Â N orthogonal matrix, and Æ is an M Â N diagonal matrix with Æij ¼ 0 if i 6¼ j and Æii ! 0. The quantities Æii are called the singular values of X, and the columns of U and V are called
基于YOLO的复杂环境视觉SLAM优化方法
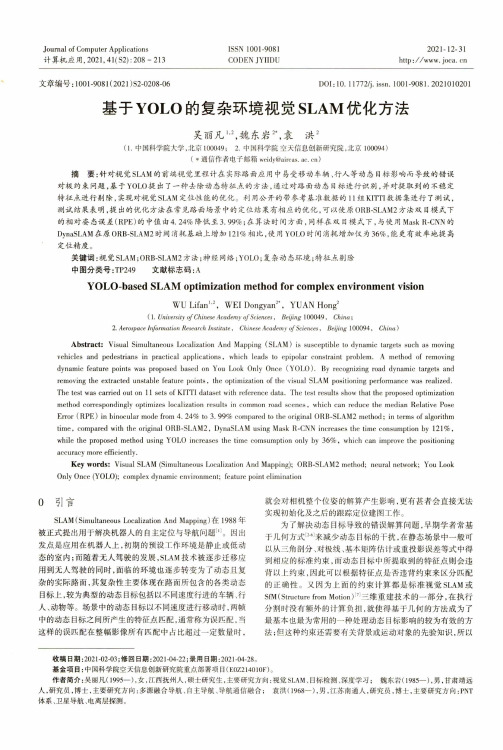
Journal of Computer Applications ISSN 1001-9081 2021-12-31
计算机应用,2021,41 (S2): 208 - 213 CODEN JYIIDU http://www.joca.cn
文章编号:1001-9081(2021)S2-0208-06 DOI: 10. 11772/j. issn. 1001-9081. 2021010201基于YOLO的复杂环境视觉SLAM优化方法
吴丽凡'魏东岩2•,袁洪2(1.中国科学院大学,北京100049; 2.中国科学院空天信息创新研究院,北京100094)(*通信作者电子邮箱weidy@aircas. ac. cn)
摘要:针对视觉SLAM的前端视觉里程计在实际路面应用中易受移动车辆、行人等动态目标影响而导致的错误
对极约束问题,基于Y0L0提出了 一种去除动态特征点的方法,通过对路面动态目标进行识别,并对提取到的不稳定 特征点进行剔除,实现对视觉SLAM定位性能的优化。利用公开的带参考基准数据的11组KITTI数据集进行了测试, 测试结果表明,提出的优化方法在常见路面场景中的定位结果有相应的优化,可以使原0RB-SLAM2方法双目模式下 的相对姿态误差(RPE)的中值由4. 24%降低至3. 99%;在算法时间方面,同样在双目模式下,与使用Mask R-CNN的 DynaSLAM在原0RB-SLAM2时间消耗基础上增加121%相比,使用Y0L0时间消耗增加仅为36%,能更有效率地提高
定位精度。关键词:视觉SLAM; 0RB-SLAM2方法;神经网络;Y0L0;复杂动态环境;特征点剔除
中图分类号:TP249 文献标志码:A
YOLO-based SLAM optimization method for complex environment vision
WU Lifan1,2, WEI Dongyan2*, YUAN Hong2(1. University of Chinese Academy of Sciences, Beijing 100049, China;2. Aerospace Information Research Institute, Chinese Academy of Sciences, Beijing 100094, China)
- 1、下载文档前请自行甄别文档内容的完整性,平台不提供额外的编辑、内容补充、找答案等附加服务。
- 2、"仅部分预览"的文档,不可在线预览部分如存在完整性等问题,可反馈申请退款(可完整预览的文档不适用该条件!)。
- 3、如文档侵犯您的权益,请联系客服反馈,我们会尽快为您处理(人工客服工作时间:9:00-18:30)。
Arc-Consistency for Non-Binary Dynamic CSPsChristian BessièreLIRMM (UMR C 9928 CNRS / Université Montpellier II)860, rue de Saint Priest 34090 Montpellier, FranceEmail: bessiere@crim.frAbstract. Constraint satisfaction problems (CSPs) provide a model often used in Artificial Intelligence. Since the problem of the existence of a solution in a CSP is an NP-complete task, many filtering techniques have been developed for CSPs. The most used filtering techniques are those achieving arc-consistency. Nevertheless, many reasoning problems in AI need to be expressed in a dynamic environment and almost all the techniques already developed to solve CSPs deal only with static CSPs. So, in this paper, we first recall what we name a dynamic CSP, and then, generalize the incremental algorithm achieving arc-consistency on binary dynamic CSPs to general dynamic CSPs. Like for the binary version of this algorithm, there is an advantage to use our specific algorithm for dynamic CSPs instead of the best static one, GAC4.1. IntroductionConstraint satisfaction problems (CSPs) provide a simple and good framework to encode systems of constraints and are widely used to express static problems. Nevertheless, many problems in Artificial Intelligence involve reasoning in dynamic environments. For instance, in a design process, the designer may add constraints to specify further the problem, or relax constraints when there are no more solutions (see the system to design peptide synthesis plans: SYNTHIA[1]). Every time a constraint has been added or removed we need to check if there still exist solutions in the CSP.Proving the existence of solutions or finding a solution in a CSP are NP-complete tasks. So, a filtering step is often applied to CSPs before searching solutions. The most used filtering algorithms are those achieving arc-consistency. Almost all arc-consistency algorithms are written for static CSPs. So, if we add or retract constraints in a CSP and achieve arc-consistency after each modification with one of these algorithms, we will probably do many times almost the same work. The only arc-consistency algorithm written for dynamic CSPs, DnAC-4 in [2], deals only with binary CSPs.So, in this paper we recall that a Dynamic CSP (DCSP) is a sequence of static CSPs each resulting from the addition or retraction of a constraint in the preceding one ([3], [2]). We propose a generalized version of DnAC-4 to maintain arc-consistency in general DCSPs which outperforms those written for static CSPs.The paper is organized as follows. Section 2 presents the CSP model (2.1) and defines what is a Dynamic CSP (2.2). Arc-consistency filtering method is introduced and the best algorithm achieving it (GAC4 in [4]) is described (2.3). Why this algorithm is not optimal in DCSPs is underlined in 2.4. Section 3 presents a new method which adapts the idea of GAC4 to reach better performances on DCSPs. Section 4 contains a summary and some final remarks.2. Definitions and preliminaries2.1. Constraint Satisfaction ProblemsA s t a t i c constraint satisfaction problem (CSP) (X, dom, c) involves a set of n variables, X={i, j…}, each taking value in its respective domain, dom(i), dom(j),…, elements of dom, and a set of constraints c. A constraint C p(i1,…,i q)constraining the subset {i1,...,i q} of X is a subset of the Cartesian product dom(i1)x…x dom(i q), that specifies which values of the variables are compatible with each other. A constraint is usually represented by the set of all tuples which are not forbidden by it. A s o l u t i o n of a CSP is an assignment of values to all the variables such that all the constraints are satisfied. The task, in a CSP, is to find one, or all the solutions. Consider for instance the CSP presented in fig. 1. Each node represents a variable whose values are explicitly indicated. Each constraint is explicitly given by the list of its admissible tuples (C1 and C5 are a strict lexicographic order along the arrows and C2, C3, C4 are equality constraints).12cb62baab cc25aabc cbc c23aab b34aabc cb5abcC1C2C 5C4C34C3Figure 1: An example of CSP2.2. Dynamic Constraint Satisfaction ProblemsA dynamic constraint satisfaction problem (DCSP) p is a sequence of static CSPs p(0),...p(α), p(α+1),..., each resulting from a change in the preceding one imposed by "the outside world". This change can be a r e s t r i c t i o n (a new constraint is imposed on a subset of variables) or a relaxation(a constraint which was present in the CSP is removed because it is no longer interesting or because the current CSP has no solution).So, if we have p(α)=(X, dom, c(α)), we will have p(α+1)=(X, dom, c(α+1)) where c(α+1)=c(α) ± C, C being a constraint. p(0)=(X, dom, ∅).2.3. Arc-consistencyThe task of finding solutions in a CSP has been treated by several authors, and since the problem is NP-complete, some of them have suggested that a preprocessing or filtering step be applied before the search (or backtracking) procedures. Then, consistency algorithms were proposed ([5], [6], [7]). These algorithms do not solve a CSP completely but they eliminate once and for all local inconsistencies that cannot participate in any solutions. These inconsistencies would otherwise have been repeatedly discovered by most backtracking procedures. The most widely used consistency algorithms are those achieving arc-consistency.Definition. A tuple u in a constraint C p is viable iff for all variable i constrained by C p, u[i] is a viable value. A value a for a variable i is viable iff for all constraint C p constraining i there exists a viable tuple u in C p such that u[i]=a. A CSP is arc-consistent iff for all i∈X, for all a∈dom(i), a is viable.The complexity of the best algorithm building the largest arc-consistent domain in general CSPs (GAC4 in [4]) is O(K) with K the sum of the lengths of all tuples for all constraints. It has been shown that it is an optimal algorithm.The efficiency of GAC4 comes from the data structure it handles which is suitable to the arc-consistency property. Arc-consistency can be seen as based on the notion of support. A value a for the variable i is viable if there exists at least one tuple which "supports" it at each constraint. GAC4 makes this support evident by assigning a set of supports to each constraint-value pair. The sets designed by S iap contain the tuples in C p that support the value a for the variable i. The algorithm is founded on a recursive value pruning, deleting a value when it has no support on a constraint, and then removing at their turn all tuples including this value. And so on until all remaining values have support on all the constraints. So, the CSP is arc-consistent. And it is the maximal arc-consistent domain.2.4. Arc-consistency in DCSPsMohr's and Masini's algorithm, GAC4, can be used in DCSPs. It keeps all its goods properties when we do a restriction, starting filtering from the current arc-consistent domain and pruning a new value when one of its support sets is empty after addition of constraints. But, when we remove a constraint (making a relaxation), GAC4 cannot find which value must be put back and which one must not: as it has "forgotten" the reason why a value has been removed, it cannot make the opposite propagation it has done during restrictions. So, we have to start filtering from the initial domain. 3. The incremental method3.1. IntroductionAs we have seen above, GAC4 does not have good properties (incrementality) for processing relaxations. So, in this section, we propose DnGAC4, a new arc-consistency algorithm for DCSPs. In DnGAC4 we extend GAC4 by recording some informations during restrictions while keeping its good properties. Then, DnGAC4 remains incremental for relaxations.More precisely, during a restriction, for every value deleted, we keep track of the constraint origin of the deletion as the "justification" of the value deleted. The justification is the first constraint on which the value is without support. For every tuple deleted, we keep track of the value origin of its deletion as the "killer" of the tuple deleted. The killer is the first value retracted in the tuple. During a relaxation, with the help of justifications and killers, we can incrementally add to the current domain values which belong to the new maximal arc-consistent domain. But we need to be careful because after the relaxation the system must be in the same state as if the algorithm had started with the initial CSP p(0)and had done only restrictions with all the new set of constraints: the set of justifications and killers must remain well-founded to ensure that the new domain is the maximal arc-consistent domain.Definition. Justifications and killers are well-founded iff in any set E of retracted values there exists a value (i, a) such that, its justification being the constraint C p, every tuple of C p including (i, a) has its killer out of E.In other words, well-foundness means that every removed value is justified by a non-cyclic chain of justifications and killers. The value is not recorded as one of the reasons of its self deletion.3.2. The algorithm DnGAC4The algorithm we propose works with the following data structures:• a table D of booleans keeps track of which values are in the current domain or not (the current domain being named D we confuse (i, a)∈D and D(i, a)=true)• a set of supports for each value on each constraint: S iap={u∈C p /(i, a)∈u and u is viable}• for each value, the constraint origin of its deletion is stored in justif(i, a).if (i, a) is viable, justif(i, a)=nilelse justif(i, a)=the number of the first constraint on which (i, a) had got an empty support.• for each tuple, we store in killer(u) the first value deleted in it.if u is viable, killer(u)=nilelse killer(u)=the first value in u retracted from D.• a set of killed tuples for each value on each constraint: T iap={u∈C p /killer(u)=(i, a)}• the lists S L and R L respectively control the propagation of deletions and additions of values along the constraints; the list UL records for reconsideration the tuples put back to ensure well-foundness but that still have a killer.Before giving the algorithms, we define the function find-killer which will be used in the following.function find-killer(u: tuple): value;return (j, b ) ∈u /D(j, b )=falseelse return nilWhen the algorithm starts with p(0), the tables are initialized:for all (i, a ) ∈dom doD(i, a ) ,true; justif(i, a ) ,nil ;Add a constraint C p to the DCSP is done by calling the procedure Add.procedure Add (C p(i1,…,i q): constraint);Put C p(i1,…,i q) in c;SL,∅ ;Init-Add (C p(i1,…,i q), SL );Propag-Suppress (SL );• the procedure Init-Add creates lists S iap and T iap for all (i, a) such that i is constrained by C p. It updates killer for all tuple u in C p, adding u in all S jbp such that (j, b)∈u if u is viable or in T killer(u)p if not. All (i, a, p) without support (S iap=∅) are added to SL.procedure Init-Add (in C p(i1,…,i q): constraint;in out SL: list);1for all i ∈ {i1,…,i q } do2for all a∈dom(i ) do S iap,∅ ; T iap,∅3for all u∈C p do4killer(u ) , find-killer(u );5if killer(u ) = nil then6for all (j, b ) ∈u do Append(S jbp, u );7else Append(T killer(u)p, u )8for all i ∈ {i1,…,i q } do9for all a∈dom(i ) do10if D(i, a ) and S iap = ∅then Append(SL, (i, a, p ));• the procedure Propag-Suppress takes constraint-value pairs (i, a, m) in SL, removes (i, a) from D, updates justif(i, a) and also killer(u) for all u viable such that (i, a) ∈u. These tuples u are added to T iap and removed from all S jbp which they were belonging. If a S jbp becomes empty, (j, b, p) is added in SL. And so on. procedure Propag-Suppress (in out SL: list);1while SL≠∅do2keep(SL, (i, a, m ));3if D(i, a ) and S iam = ∅then4justif(i, a ) ,m ;5D(i, a ) ,false;6for all C p in c constraining i do7for all u∈S iap do8killer(u ) , (i, a ); Append(T iap, u );9for all (j, b ) ∈u do10Suppress(S jbp, u );11if D(j, b ) and S jbp = ∅thenAppend(SL, (j, b, p ));To retract the constraint C m(i1,…,i q) from the DCSP we call the procedure Relax.procedure Relax (C m(i1,…,i q): constraint);SL,∅ ;Init-Propag-Relax (C m(i1,…,i q), SL );Propag-Suppress (SL )Two parts are needed in the relaxation process to maintain the well-founded property.• part 1. first, the procedure Init-Propag-Relax adds to D and puts in RL all values (i, a) for which deletion was justified by C m(i.e. justif(i, a)=m). In a second step, the consequences of the addition of these values (i, a) to D are propagated. Tuples u deleted by them (killer(u)=(i, a)) are put back in the supports lists S jbp of values (j, b) that they support (lines 13-15), except if there is another value of u for which we are sure that retraction is independant of u's retraction (lines 10-12). At each tuple put back, we add in D and in RL every (j, b) which has the supports set S jbp that becomes non empty on its justification constraint (p=justif(j, b)) (line 16). Additions of values are recursively propagated: this second step processes while RL is non empty. In this second step, if a tuple is added when itincludes values not in D because we are not sure of the independance of the retraction of these values, we add it in a list UL which records these "suspended" tuples (line 17). In the third step, we check if these suspended tuples are really saved (their killers were not independent, they had not a well-founded justification) or if their killers are still present. In this latter case, we kill again the tuple and remove it from the supports sets of the values it supports.During all the procedure Init-Propag-Relax, each time a support set S jbp of an added value (j, b) is found empty, we put the constraint-value pair (j, b, p) in SL. procedure Init-Propag-Relax (in C m(i1,…,i q): constraint;in out SL: list);{Step 1: values with justification equal to C m are added to D and put in RL for propagation}1RL,∅ ;2for all i ∈ {i1,…,i q } do3for all a∈dom(i ) do4if justif(i, a )=m thenAppend(RL, (i, a ));justif(i, a ) ,nil; D(i, a ) ,true5Remove C m from c;{Step 2: consequences of the addition to D of the values in RL are propagated }6while RL≠∅do7keep (RL, (i, a ));8for all C p in c constraining i do9for all u∈T iap do10if u!D and (∀(j, b)∈u, justif(j, b)≠p) then11killer(u) , find-killer(u);12Append(T killer(u)p, u )else13killer(u ) ,nil;14for all (j, b ) ∈u do15Append(S jbp, u );16if justif(j, b )=p thenAppend(RL, (j, b ));justif(j, b ) ,nil; D(j, b ) ,true17if u!D then Append(UL, u );18T iap,∅ ;19if S iap = ∅then Append(SL, (i, a, p ));{endwhile}{Step 3: "suspended" tuples are checked }20while UL≠∅do21keep(UL, u );{ let C p being the constraint including u }22killer(u ) , find-killer(u );23if killer(u ) ≠nil then24Append(T killer(u)p, u );25for all (j, b ) ∈u do26Suppress(S jbp, u );27if D(j, b ) and S jbp = ∅thenAppend(SL, (j, b, p ))• part 2. Propag-Suppress takes constraint-value pairs (i, a, m) in SL and removes again from D values (i, a) which still are without support on the constraint C m(S iam=∅). These retractions are propagated on the tuples and on the other values: the classic arc-consistency process restarts.We develop here DnGAC4 on the DCSP of fig.1, to show the mechanism of justifications and killers (notation: u p q is the q th tuple of the constraint C p).Add C1.(2, a) and (2, b) deleted: justif(2, a),1 and justif(2, b),1Add C2.killer(u21),(2, a) and killer(u22),(2, b).(3, a) and (3, b) deleted: justif(3, a),2 ; justif(3, b),2Add C3.killer(u31),(3, a) and killer(u32),(3, b).(4, a), (4, b), (5, a), (5, b) deleted: justif(4, a),3; justif(4, b),3; justif(5, a),3; justif(5, b),3Add C4.killer(u41),(2, a) and killer(u42),(2, b).Add C5.killer(u51),(2, b).Relax C1.(2, a) and (2, b) are added because justif(2, a)=1and justif(2, b)=1. All tuples killed by (2, a) and (2, b) are examined. u21, u22, u51 are restored but not u41 and u42 because we can find another killer for them without losing well-foundness. So, new killers are recorded: killer(u41),(5, a) and killer(u42),(5, b). (2, a) and (2, b) are without support on C4 so we add them to SL. The consequence of u21 and u22 rehabilitation is the addition of (3, a) and (3, b) to D. So, u31and u32are restored and (4, a), (4, b), (5, a) and (5, b) are added to D. Then, u41 and u42are restored. The first part of the relaxation process is finished. The second part is the classical arc-consistency process, examining values in SL and propagating deletions. (2, b) is in SL but has now a support on C4so nothing is done. (2, a) is without support on C5so it is discarded and justif(2, a),5. So, killer(u21),(2, a) and killer(u41),(2, a). Then, (3, a) and (5, a) are discarded (justif(3, a),2, justif(5, a),4). Then, killer(u31),(3, a) and (4, a) is discarded (justif(4, a),3). We have the new maximal arc-consistent domain.3.3. Correctness of DnGAC4We give here the properties true after each procedure of DnGAC4 which are needed to prove the correctness of the algorithm. For a complete proof we will see [8]. Basic properties.(1)S iap={u∈C p / (i, a) ∈u and u⁄D}(2)T iap={u∈C p / (i, a)=killer(u)}Justifications and killers properties.(3)justif(i, a)=p⇒ (i, a) ∉D and S iap=∅(4){(i, a) ∉D / justif(i, a)=nil}=∅(5){(i, a, p)/ S iap=∅and (i, a) ∈D} ⁄SL(6)killer(u)=(i, a) ⇒ (i, a) ∈u and (i, a) ∉D(7)u !D⇒killer(u)≠nil(8)∀E⁄dom\D: ∃(i, a)∈E: justif(i, a)=p and∀u∈C p /(i, a)∈u: killer(u) ∉E {well-founded property}From (5) we can deduce that D is arc-consistent at the end of Propag-Suppress, so at the end of Add or Relax too. From Propag-Suppress construction we can see that if D includes the maximal arc-consistent domain at its begining, this remains true at its end. Now, we need only to prove that when Propag-Suppress begins, we always have D including the maximal arc-consistent domain. It is evident in Add, and for Relax, we can prove this using properties (6), (7), (8) and looking Init-Propag-Relax construction.3.4. Complexity of DnGAC4In DnGAC4, the bigger data structures are the S iap and T iap lists of tuples. They take the same space as all the constraints C p in the CSP. This space is linear in the sum of the lengths of all the tuples of all the constraints (it is linear in the size of the problem).The upper bound time complexity of DnGAC4, like in GAC4, is linear in the size of the problem since the atomic operations are first Append(S iap, u), and then Suppress(S iap, u) (the total size of the S iap sets being bounded by the size of the problem). But these operations must be processed in constant time. A suitable data structure is shown in [4].If we want to specify the complexity of DnGAC4, we can say that, on one hand, during a restriction, DnGAC4 does a little more processing than GAC4, building a more complex data structure (for each tuple a killer is stored, and for each value we record a justification). On the other hand, during a relaxation, DnGAC4 only checks values and tuples needed to verify the well-founded property of justifications and killers. GAC4 handles all the new CSP.3.5. Performance comparisonThere are too many parameters in general DCSPs to provide significant tests on randomly generated DCSPs but it seems that DnGAC4 on general DCSPs works at least as better as DnAC-4 on binary DCSPs. If DnGAC4 is applied on CSPs containing only ternary constraints, we can see that it generally outperforms GAC4 after the first relaxation of constraint, reaching a gain of 35% (average of the classes of CSPs tested) on the sum of the running time of all restrictions plus the relaxation. More, if we modify the DCSP several times, adding and removing constraints until we find a "good" consistent CSP (what is done in all design problems) we remark that the gain of DnGAC4 fastly grows and overtops 50% after two or three relaxations. More changes will be done, more the use of DnGAC4 will be interesting.DnGAC4 is now under implementation on SYNTHIA [1].4. ConclusionWe have defined what we call Dynamic CSPs and have underlined that there does not exist algorithms incrementally maintaining arc-consistency in general DCSPs (there exists one only for binary DCSPs). We have noticed that arc-consistency is the most used filtering technique in CSPs, and so, it is important to have an incremental algorithm for all DCSPs. Then, we have provided an efficient algorithm (DnGAC4) incrementally achieving arc-consistency in general DCSPs. DnGAC4 can be useful for many systems which work in a dynamic environment. AcknowledgementsI would like to thank particularly Marie-Catherine Vilarem for the implementation of my work on SYNTHIA and Philippe David who gives me constructive suggestions for the final draft.References[1] P. Janssen, P. Jégou, B. Nouguier, M.C. Vilarem, B. Castro: "Synthia: Assisted design of peptide synthesis plans"; New Journal of Chemistry, 14-12, 1990, 969-976[2] C. Bessière: "Arc-Consistency in Dynamic Constraint Satisfaction Problems"; Proceedings AAAI-91, Anaheim CA, 221-226[3] R. Dechter, A. Dechter: "Belief Maintenance in Dynamic Constraint Networks"; Proceedings AAAI-88, St Paul MN, 37-42 [4] R. Mohr, G. Masini: "Good Old Discrete Relaxation"; Proceedings ECAI-88, München, FRG, 651-656[5] U. Montanari: "Networks of Constraints: Fundamental Properties and Applications to Picture Processing"; Information Science 7 (1974), 95-132[6] A.K. Mackworth: "Consistency in Networks of Relations"; Artificial Intelligence 8 (1977) 99-118[7] E.C. Freuder: "Synthesizing Constraint Expressions"; Communications of the ACM Nov. 1978 Vol.21 No.11, 958-966 [8] C. Bessière: "Algorithmes d'arc-consistance pour les CSP dynamiques"; Tech.Rep. 91-086, LIRMM Montpellier II, France, August 1991 (in French)。